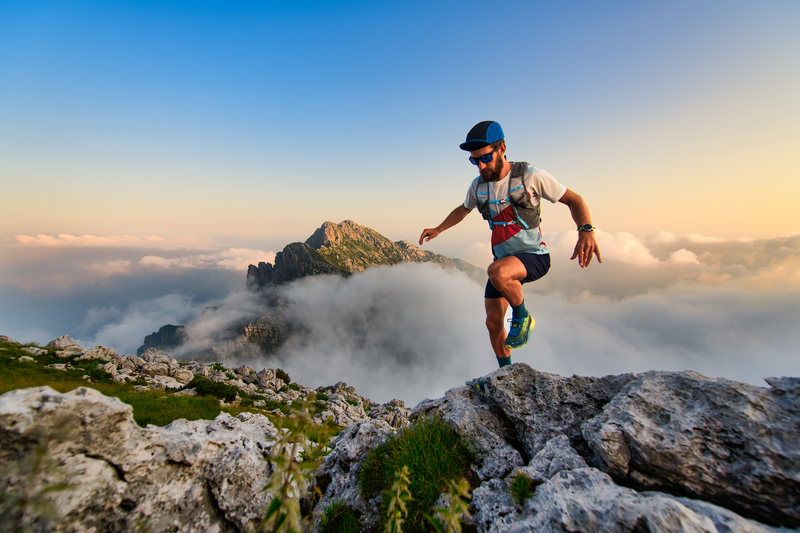
94% of researchers rate our articles as excellent or good
Learn more about the work of our research integrity team to safeguard the quality of each article we publish.
Find out more
ORIGINAL RESEARCH article
Front. Genet. , 17 May 2022
Sec. Livestock Genomics
Volume 13 - 2022 | https://doi.org/10.3389/fgene.2022.812828
This article is part of the Research Topic Microbiome Genomics for Livestock Production View all 8 articles
Background: The impact of extreme changes in weather patterns on the economy and human welfare is one of the biggest challenges our civilization faces. From anthropogenic contributions to climate change, reducing the impact of farming activities is a priority since it is responsible for up to 18% of global greenhouse gas emissions. To this end, we tested whether ruminal and stool microbiome components could be used as biomarkers for methane emission and feed efficiency in bovine by studying 52 Brazilian Nelore bulls belonging to two feed intervention treatment groups, that is, conventional and by-product-based diets.
Results: We identified a total of 5,693 amplicon sequence variants (ASVs) in the Nelore bulls’ microbiomes. A Differential abundance analysis with the ANCOM approach identified 30 bacterial and 15 archaeal ASVs as differentially abundant (DA) among treatment groups. An association analysis using Maaslin2 software and a linear mixed model indicated that bacterial ASVs are linked to the host’s residual methane emission (RCH4) and residual feed intake (RFI) phenotype variation, suggesting their potential as targets for interventions or biomarkers.
Conclusion: The feed composition induced significant differences in both abundance and richness of ruminal and stool microbial populations in ruminants of the Nelore breed. The industrial by-product-based dietary treatment applied to our experimental groups influenced the microbiome diversity of bacteria and archaea but not of protozoa. ASVs were associated with RCH4 emission and RFI in ruminal and stool microbiomes. While ruminal ASVs were expected to influence CH4 emission and RFI, the relationship of stool taxa, such as Alistipes and Rikenellaceae (gut group RC9), with these traits was not reported before and might be associated with host health due to their link to anti-inflammatory compounds. Overall, the ASVs associated here have the potential to be used as biomarkers for these complex phenotypes.
The Anthropocene Epoch is marked by the continuous degradation of the biosphere promoted by human activities, culminating in the ongoing climate and environmental crisis (Williams et al., 2015; Cook et al., 2016). One of the current challenges is to mitigate its effects and sustain an ever-growing human population by developing methods for efficient food production. Cattle farming, a valuable source of animal protein, is alone responsible for a significant environmental impact due to the occupation and degradation of land for pasture, contamination of water sources by cattle manure (Sahoo et al., 2016), and the emission of methane (CH4) produced by enteric fermentation, a greenhouse gas 28 times stronger than CO2 (Pachauri and Mayer, 2015).
Key phenotypes for the reduction of the meat industry’s environmental and economic burden, such as feed efficiency and methane emission, are linked to interactions between the host and its associated microbial communities, also known as the microbiota (Guan et al., 2008; Chang et al., 2019). Although studies targeting these phenotypes have been published over the years (Mudadu et al., 2016; Pszczola et al., 2018; Lassen and Difford, 2020), only recently the microbiota started to be considered as an important subject to increase efficiency and reduce costs and the environmental impact of cattle farming (Shi et al., 2014; Noel et al., 2019).
These microorganisms can shape their host biology through beneficial interactions and influence health, development, and immune system modulation (Flint et al., 2007; Belkaid and Hand, 2014). However, most of these microorganisms are elusive and utterly unknown to science due to inherent difficulties in cultivation procedures (Solden et al., 2016). Nowadays, it is possible to access microorganisms’ genomic material directly and investigate their identity, distribution, relatedness, and functionality using approaches from the meta-omics field, such as deep sequencing metagenomics, metabarcoding, and metatranscriptomics (Gilbert and Dupont, 2011).
The microbiome structure of the Nelore Brazilian beef cattle has gained the attention of the scientific community in the past years, being the subject of different studies (de Oliveira et al., 2013; Lopes et al., 2021), including a previous study by our research group (Andrade et al., 2020) in which we investigated the microbiome profiles of two segments of 26 Nelore bulls’ gastrointestinal tract (GIT). We showed that a significant part of the stool archaeal population co-occurred with the rumen archaeal population, suggesting the use of stool as a proxy for the rumen archaeal population.
Herein, we extended that the study by the introduction of an additional experimental group under a different diet and compared the microbiome populations from two distant sections of the Nelore GIT-rumen and rectal ampulla to 1) identify the impact of the dietary treatment on the microbiome diversity and abundance; 2) identify associations between microbiome components and phenotypes, such as residual CH4 emission (RCH4) and residual feed intake (RFI).
All experimental procedures were conducted following animal welfare guidelines and were approved by the EMBRAPA Livestock Science Ethics Committee on Animal Experimentation, São Carlos, São Paulo (Protocol No. 09/2016). The experimental population consisted of animals born in 2014, and the experiment was conducted at the feedlot facility of “Embrapa Pecuária Sudeste”. It lasted 105 days, which included 15 days for animal adaptation to the feedlot, 30 days for growth, and 60 days for animal finishing. Animals were divided into two groups based on dietary treatment. The first experimental group (conventional group, n = 26) consisted of animals fed with a conventional diet based on corn silage, corn, soybean meals, rumen-protected fat, and urea as a concentrate, as described in Andrade et al. (2020). The second experimental group (by-product group, n = 26) replaced concentrates with the industrial by-products citrus pulp, corn germ, corn germ oil meal, and peanut shell meal. In both treatment groups, animals received mineral supplements, active dry yeast, virginiamycin, and monensin.
Feedlots were divided based on the dietary treatment and initial weights, with heavyweight and lightweight animals grouped separately. The facility has collective stalls with an automatic feeding system (GrowSafe Systems Ltd., Airdrie, Alberta, Canada), in order to collect data regarding live weight and daily food consumption. The animals were then sent for slaughter at 23–24 months of age, following the Guidelines for Humane Handling, Transport, and Slaughter of Livestock. All animal data used in this study are available in Supplementary Table S1.
5 mL of blood samples were collected from each animal, and DNA extractions were performed by a salting-out method (Meo et al., 2009). DNA concentration was measured by spectrophotometry, and quality was verified by the 260/280 optical density ratio, followed by integrity inspection through agarose gel electrophoresis. All animals were genotyped using the GGP Bos indicus 50 k. Genotypes were called in the Illumina GenomeStudio software. We applied the following filtering parameters with PLINK software (Chang et al., 2015): 1) SNP call rate ≤95%, 2) SNP minor allele frequency (MAF) ≤ 5%, 3) animals with ≥10% missing genotypes, and 4) SNPs that did not pass the HWE test (p ≤ 0.001). A total of 41,869 SNPs of all 52 animals were available for further analysis.
Individual dry matter intake (DMI, kg/d) was obtained by the difference between the weight of the diet provided and refusal, and average daily gain (ADG, kg/d) was estimated by linear regression of body weight (BW) on days in feedlot. Residual feed intake (RFI, kg/d) was computed as the residuals from the regression of DMI on mid-test BW0.75 and ADG (Koch et al., 1963). The metabolic body weight (MBW, kg) was obtained with the following equation: MBW = BW0.75. The contemporary group (CG) was defined as the weighing group and the slaughter group, which were considered fixed effects by MIXED procedure of the SAS statistical program (SAS Institute, Cary, NC, United States, 2011), according to the following equation:
where DMIi is the dry matter intake predicted for animal i; ADGi is the average daily gain of animal i; MBWi is the metabolic body weight of animal i; β0 is the regression intercept; β1 is the partial regression coefficient of ADG; β2 is the partial regression coefficient of MBW; and β3 is the partial regression coefficient of CG, and RFIi is the RFI of animal i (Koch et al., 1963).
The methane emission was measured during the finishing period in the feedlot using the GreenFeed system (Clock Inc., Rapid City, SD, United States). The residual methane emission (RCH4) was obtained by the regression of methane emission using individual DMI (Donoghue et al., 2016), and CG as covariables in the MIXED procedure of SAS statistical program (SAS Institute, Cary, NC, United States, 2011), according to the following equation:
where MEi is the methane emission predicted for animal i; DMIi is the dry matter intake predicted for animal i; β0 is the regression intercept; β1 is the partial regression coefficient of DMI; β2 is the partial regression coefficient of CG; and, as proposed by Donoghue et al. (2016), the model residual error of animal i was considered as the residual methane emission (RCH4).
After the finishing phase, approximately 10 g of stool was obtained from each animal 2 weeks before slaughtering, and 50 ml of rumen content was collected immediately after slaughter. All samples were frozen in liquid nitrogen and permanently stored at −80°C before analysis. DNA extraction was performed using the Quick-DNA™ Fecal/Soil Microbe Miniprep Kit (ZYMO Research Corp., Irvine, CA), using 150 mg of each sample and following the standard protocol. PCR target amplification for the bacterial and archaeal 16S rRNA and protozoal 18S rRNA coding genes was performed using the primers 341-b-S-17F and 341-b-S-17F (Klindworth et al., 2013), Ar915aF and Ar1386R (Kittelmann et al., 2013), and Reg1320R and RP841F (Henderson et al., 2015), respectively, following Andrade et al. (2020). Amplicons were sequenced in an Illumina Miseq platform (2 × 250 bp) using the Illumina V3 sequencing kit at the ESALQ Genomics Center (Piracicaba, SP, Brazil).
In addition to the dataset generated in this study, raw reads generated by our previous study with bulls fed conventional diet were retrieved from the SRA database [accession number PRJNA525838] and processed to infer the impact of dietary treatments and to search for association with phenotypes.
Raw reads from conventional and by-product groups were filtered for quality (>Q25) and trimmed at positions 220 (forward) and 175 (reverse) using QIIME 2 version 2018.8 (Bolyen et al., 2019). We selected these positions based on aggregation plots provided by QIIME 2. The filtered data was submitted to the DADA2 package to generate amplicon sequence variants (ASVs) with the option just-concatenate and exclude chimeric sequences (Callahan et al., 2016). Bacterial sequences were annotated using the SILVA database version 132 (Quast et al., 2013), archaeal sequences using the Rumen and Intestinal Methanogen database (RIM-DB) (Seedorf et al., 2014), and protozoa using a curated database containing protozoa 18S rRNA gene sequences (Kittelmann et al., 2015). We used the resulting ASV table to determine alpha (number of ASVs and the Shannon–Wiener index) and beta diversities (unweighted UniFrac distance) with QIIME 2.
We contrasted the microbiome of groups submitted to different dietary treatments using the Analysis of Composition of Microbiomes (ANCOM) version 2.1 (Kaul et al., 2017), with significance values adjusted for multiple tests using the Benjamin–Hochberg method (α < 0.05). We applied a conservative W-statistic (W-statistic cutoff = 0.9) in which an ASV was considered as differentially abundant if its composition varied when compared to 90% of the rest of the dataset, being the W-value the number of times the model rejected the null hypothesis for a given ASV across two groups. ANCOM is a statistical approach that compares Aitchison’s centered log-ratio transformed abundances of each ASV individually with all the remaining ASVs without any distributional assumptions (Kaul et al., 2017).
ASV abundances were tested for associations with animal phenotypes, such as RCH4 and RFI phenotypes using the Microbiome Multivariable Association with Linear Models (Maaslin) version 2 (Mallick et al., 2021). Maaslin is a multivariate model developed for the microbiome data analysis, as it considers the compositional nature of these datasets. The analysis was adjusted for treatment and contemporary groups using the following formula:
where CLR is the ASV abundance transformed using the centered log-ratio method, and e is the residual error. ASVs identified as significant by the Maaslin analysis, and that was prevalent in more than 20% of animals with a minimum abundance of 0.001%, had their effect on the phenotypic variation (response variable) tested by using the linear mixed model implemented in the lme4qtl package (Ziyatdinov et al., 2018). Genetic relatedness was included as a random effect in the form of a kinship matrix to rule out the population structure effect in the phenotypic variation, jointly with diet and CG fitted as fixed effects. We built the genomic relatedness matrix using the AGHmatrix package (Amadeu et al., 2016) of R (v. 4.0.3), based on the genomic matrix proposed elsewhere (VanRaden, 2008).
A likelihood ratio test was used to contrast models (m1 and m2) to investigate the ASV impact on the phenotype. Our model can be described by the following formula:
m1: phenotype (RFI or RCH4) ∼ Diet + CG + Kinship Matrix + residual error.
m2: phenotype (RFI or RCH4) ∼ centered log-ratio (ASV) + Diet + CG + Kinship Matrix + residual error.
Significant values for all models were adjusted for multiple tests using the false discovery rate (FDR) method (α<= 0.05).
The sequencing of microbiome rDNA amplicons from ruminal and stool samples of the by-product group yielded a total of 10,573,763 paired-end reads (4,628,604 paired-end reads for bacteria, 4,443,390 for archaea, and 1,501,769 for protozoa), reaching 20,241,296 paired-end reads with the addition of sequencing data from animals fed conventional diet. After quality control and singleton exclusion, we identified a total of 4,519 bacterial ASVs (2,680 ruminal ASVs and 1,839 stool ASVs), 1,023 archaeal ASVs (421 ruminal ASVs and 602 stool ASVs), and 151 ruminal protozoa ASVs across treatments. Rarefaction curves based on the alpha diversity metrics of the Shannon–Wiener index (diversity) reached a plateau, which indicated that additional sequences would not likely result in additional features (Supplementary Figure S1).
Comparison of samples from different treatment groups using alpha diversity metrics (observed ASVs and Shannon–Wiener indexes) by the Kruskal–Wallis testing method revealed that rumen bacterial microbiome was significantly more abundant and richer in animals fed the conventional diet than those fed the by-product diet (p = 0.006 and p = 0.04, respectively), whereas the ruminal archaea diversity was richer (p = 0.0004) but not more abundant. There was no significant difference between diets when contrasting alpha diversity metrics of stool samples. Conversely, comparisons of the beta diversity metric unweighted UniFrac using the PERMANOVA approach revealed that samples of archaea and bacteria tended to form two significant clusters, which represented the treatment groups (adjusted p < 0.01) (Figures 1, 2), a tendency most pronounced in stool populations. Protozoa populations showed no significant differences between treatment groups (Supplementary Figure S2).
FIGURE 1. (A) Comparison between observed ASV (amplicon sequence variants) metric between treatment groups showed no significant difference in the bacterial population of animals submitted to different diets in both rumen (green line) and stool (yellow line). (B) Shannon index comparisons showing a significant difference (p < 0.01) in the richness of bacteria from the rumen microbiome. (C) PCoA using the rumen microbiome unweighted UniFrac distance showing a tendency of clustering of samples from conventional group (blue) and B (orange). (D) PCoA using the stool microbiome unweighted UniFrac distance showing an almost linear separation of samples from animals fed conventional diet (blue) and by-product diet (orange).
FIGURE 2. (A) Comparison between observed ASV (amplicon sequence variants) metric between treatment groups showed no significant difference in archaea populations of animals submitted to different diets in both rumen (green line) and stool (yellow line). (B) Shannon index comparisons showing a significant difference (p < 0.01) in the richness of archaea in the rumen microbiome. (C) PCoA using the rumen microbiome unweighted UniFrac distance showing no significant difference between groups (p > 0.1). (D) PCoA using the stool microbiome unweighted UniFrac distance showing a tendency of clustering of samples from animals fed conventional diet (blue) and by-product diet (orange).
We applied ANCOM to investigate the influence of dietary treatments in the microbiome composition at the ASV level. Seventeen ruminal ASVs of bacterial origin were differentially abundant (DA) with higher abundance in the conventional group, from which the most prominent were classified as Bacteroidales (group F082) (ASV 20 and 23), Christensenellaceae (ASV 112), Pedosphaeraceae families (ASV 145), and the genus Succiniclasticum (ASV 170). Ten DA ASVs had higher abundance in the by-product group, of which the most abundant were classified as Succiniclasticum (ASV 97), Acetitomaculum (ASV 116), Lachnospiraceae family (ASV 247), Fibrobacter (ASV 96), and Succinivibrio genus (ASV 118) (Supplementary Figure S3). Also, three stool ASVs were DA in our experimental groups; one was classified as a member of the family Rikenellaceae (ASV 361) and was more abundant animals fed conventional diet, while the ASV 332, classified as a member of the family Prevotellaceae, and the ASV 526, classified as the genus Oscillibacter, were more abundant in animals fed the by-product diet (Supplementary Figure S4).
Eight archaeal ASVs were DA among treatment groups in the rumen microbiome. Four ASVs classified as M. gottschalkii (ASVs 1, 2, 13, and 11), one as M. ruminantium (ASV 23), and one ASV belonging to the family Methanomassiliiicoccaceae (ASV 36) were all more abundant in animals fed conventional diet. In contrast, one classified as M. ruminantium (ASV 4) and the other as Methanosphaera (group ISO3-F5) (ASV 33) were more abundant in the by-product group (Supplementary Figure S5). Seven archaeal ASVs were DA in the stool microbiome. From these, the ASVs classified as M. gottschalkii (ASVs 2, 13, and 11) and M. smithii (ASV 28) were more abundant in animals fed a conventional diet. At the same time, M. ruminantium (ASV 4) and Methanosphaera (group ISO3-F5) (ASVs 5 and 33) were more abundant in animals fed by-product diet (Supplementary Figure S6). No ASVs of protozoa origin were observed as DA for any biome or group.
We applied a generalized linear model (GLM) within the Maaslin2 framework to investigate ASVs associated with RCH4 emission variation. This analysis allowed us to identify significant associations between bacteria and RCH4 in both environments. A second model was used to validate the direction and strength of association of ASVs identified by Maaslin2. We identified a single bacterial ASV associated with High-RCH4 (High emission) in the rumen, the ASV 3873 (coef_CLR = 0.54, coef_RCH4 = 0.48), classified as a Solobacterium (Figure 3A), and one associated with Low-RCH4 (Low emission) in the stool environment, the ASV 85 (coef_CLR = −1.18, coef_RCH4 = −0.48), classified as Alistipes (Figure 3B). There were no significant associations between RCH4 emission and archaea or protozoa ASVs.
FIGURE 3. Standardized beta coefficient for the RCH4 trait versus the module abundance (CLR) variation of amplicon sequence variants (ASV) in (A) rumen; (B) stool. Both the phenotypic variation and ASV abundance variation were retrieved from the beta coefficients of mixed models and Maaslin2 GLM regressions. Taxonomic information generated by QIIME 2 was included.
Association analysis between RFI and microbiome components was performed using the same GLM and mixed models described for the RCH4 analysis. We identified four bacterial ASVs associated with RFI in the rumen environment, three of these associated with feed inefficient (High-RFI) and one ASV associated with feed efficient animals (Low-RFI) (Figure 4). We also identified one ASV associated with High-RFI and one with Low-RFI in the stool environment (Figure 2).
FIGURE 4. Standardized beta coefficient for the RFI trait vs. the module abundance (CLR) variation of amplicon sequence Vvriants (ASV) in (A) rumen; (B) stool. Both the phenotypic and ASV abundance variations were retrieved from the beta coefficients of mixed models and Maaslin2 GLM regressions. Taxonomic information generated by QIIME 2 was included in the legend.
Among those associated with High-RFI, were the ASV 3718 (coef_CLR = 0.54, coef_RFI = 0.54), classified as Christensenellaceae (gut group R-7), the ASV 1452 (coef_CLR = 0.71, coef_RFI = 0.49), classified as Succinivibrio, ASV 813 (coef_CLR = 0.82, coef_RFI = 0.45), classified as Prevotella in the rumen, and the ASV 4228 (coef_CLR = 0.49, coef_RFI = 0.50), classified as Ruminococcaceae UCG-005, in stool environments. The ASV 1083 (coef_CLR = −0.91, coef_RFI = −0.48) and ASV 260 (coef_CLR = −1.00, coef_RFI = −0.46), both classified as Rikenellaceae RC9, were significant in Low-RFI (Figure 4) in rumen and stool environments, respectively. There were no significant associations between RFI, and archaea or protozoa ASVs.
Our previous study extensively explored the microbiome structure from two different sections of the Nelore cattle GIT under a single dietary treatment (Andrade et al., 2020). Herein, we expand this study by introducing a new experimental group under a different diet and related the microbiome to production phenotypes. We compared these groups to investigate the impact of the diet on microbial abundance and diversity, and the contribution of individual ASVs on complex phenotypes, such as RFI and RCH4.
Analysis of alpha diversity metrics showed that both bacteria and archaea only differed in the rumen environment, being less rich in animals of the by-product group. This richness difference could be explained by the presence of citrus pectin on the by-product diet formulation. Citrus pectin is a polysaccharide that can selectively stimulate the microbiome, affecting its composition (Larsen et al., 2019), and is linked to the decrease of Prevotella copri, a bacteria associated with rheumatoid arthritis in humans (Pianta et al., 2017). Also, PCoA analysis of the unweighted UniFrac beta diversity metric highlighted distinct clusters in treatment groups for bacteria (rumen and stool microbiomes) and archaea (stool microbiome), but not for protozoa. Altogether, these results indicate the diet as an essential microbiome modulator for bacteria and archaea populations, in agreement with previous studies in which different diets and feed components’ impact on microbiome diversity was evaluated (Dill-McFarland et al., 2019; Popova et al., 2019). Such differences in the microbiome composition had little to no impact on the phenotypes studied herein, in agreement with the lack of diet effect on phenotypes. We hypothesize, however, that this lack of observable effect could be explained by the microbiome functional redundancy, in which the taxonomic composition can vary between individuals, but its functional capacity is conserved (Tian et al., 2020). However, this hypothesis resides outside the scope of this paper, and further studies have to be implemented to test it.
Differential abundance analysis with individual bacterial ASVs revealed a significant impact of the dietary treatment in the bacterial populations of both environments. In the rumen, the ASVs classified as belonging to the Christensenellaceae family and the Prevotella and Fibrobacter genera were identified as more abundant in animals fed a conventional diet. These microorganisms are known producers of short-chain fatty acids (SCFAs), such as acetate, butyrate, and propionate (Neumann et al., 2017; Waters and Ley, 2019). Besides, ASVs classified within genera known to produce succinate and propionate, that is, Succiniclasticum and Succinivibrio (Hespell, 1992; van Gylswyk, 1995), were identified as more abundant in animals fed by-product diet. Differently from acetate and butyrate production, which increases H2 concentration in the rumen (Wolin, 1960), propionate is an electron acceptor end product of rumen fermentation and a viable alternative to methanogenesis to decrease the ruminal H2, a process known as H2 sink (Ungerfeld, 2015; Wang et al., 2018). This alternative H2 sink process decreases energy loss caused by methanogenesis and the resulting surplus of feed energy has the potential of diluting the host maintenance costs, increasing its feed efficiency.
The three DA ASVs identified in the stool samples were classified as bacteria that commonly inhabit the hindgut, including the Oscillibacter genus and Prevotellaceae family, both more abundant in animals fed by-product diet, and Rikenellaceae family, more abundant in animals fed conventional diet (Marounek and Duskova, 1999; Chen et al., 2011; Lee et al., 2012). The identification of a small number of DA ASVs in the stool microbiome is consistent with the previous alpha diversity analysis, in which there was no significant difference in both abundance and richness among experimental groups.
The dietary treatment also significantly impacted the archaea populations, e.g., an increased abundance of ASVs classified as M. gottschalkii in both rumen and stool environment of animals fed conventional diet, and M. ruminantium in both environments of animals fed by-product diet. A study on sheep with contrasting phenotypes for CH4 emission found a higher abundance of the archaea M. gottschalkii in the higher emitter group and M. ruminantium in the lower emitter group (Shi et al., 2014).
The GLM model analysis identified two bacterial ASVs associated with the RCH4 phenotype in the rumen and stool microbiomes. This reduced number of significant results was expected since this phenotype is complex and caused by different microbe–microbe and host–microbiome interactions. In addition, the microbiome functional redundancy may also play an essential role in it, reducing the likelihood of pinpointing a biomarker using metabarcoding alone.
Regarding the rumen microbiome, an ASV classified as belonging to the Solobacterium genus was identified as the most abundant bacterial ASV in animals with High-RCH4. This genus was first described in 1999 (Kageyama and Benno, 2000) and had the species Solobacterium moorei as its sole representative. This bacterium is an important producer of volatile sulfur compounds (VSC) such as hydrogen sulfide (H2S) in the human oral microbiome (Stephen et al., 2014). This compound was previously identified in vitro as having inhibitory properties against methane oxidation (Lee et al., 2015), preventing methanotrophs from metabolizing CH4 by using it as a carbon source, which could have, in turn, contributed to the increase in CH4 concentration.
In the stool microbiome, the ASV belonging to the Alistipes genus was associated with the decrease of the RCH4. This genus comprises Gram-negative and anaerobic bacteria commonly identified in the bovine GIT microbiome (Dowd et al., 2008; Holman and Gzyl, 2019). Like other members of the Rikenellaceae family, bacteria from this genus produce acetate and propionate, both fatty acids with anti-inflammatory properties in the gut of humans and chickens (Polansky et al., 2015; Parker et al., 2020).
Although we were not able to identify a direct link between anti-inflammatory compounds and methane production, researchers have been describing the potential use of different species of seaweed as a feed component to reduce the enteric methane production in dairy and beef cattle (Machado et al., 2014; Abbott et al., 2020). These seaweed species, especially the red seaweed, are rich to some extent in alkaloids, a nitrogenous compound with microbiome modulator capabilities and anti-inflammatory activity (Abbott et al., 2020). Although this link is hypothetical, the relationship between these taxa with methane emission and their biomarker/probiotic potential has to be further investigated using more layers of metagenomic information, such as metagenomes and metatranscriptomes. Another possible mechanism for this association comes from the observation of an increase in peristaltic movements in humans with higher abundance of the genus Alistipes (Scarpato et al., 2019; Sugitani et al., 2021). If this effect holds for ruminants, it could affect rumen emptying, thus providing a mechanism for the reduction in methane emission.
Surprisingly, we could not identify archaeal ASVs associated with RCH4, in both environments. Known significant contributors to methane production, species of archaea belonging to the Methanobrevibacter genus, such as M. gottschalkii and M. ruminantium, were highly abundant in the rumen microbiome of both experimental groups, with the diet having a significant impact on the archaea population structure. Still, archaeal ASVs failed to reach the significance threshold regarding this phenotype.
The ASVs identified as associated with High-RFI (decreasing the feed efficiency) in the rumen environment were taxonomically classified as Prevotella and Succinivibrio genus, and Christensenellaceae (gut group R-7). These results agreed with a recent study with rumen samples from Nelore cattle, in which OTUs classified as belonging to these same genera were linked to High-RFI (Lopes et al., 2021). Species from the genus Prevotella have a major role in the digestion of complex polysaccharides, such as cellulose and hemicellulose, and have been identified as abundant in both spectra of feed efficiency in cattle (Carberry et al., 2012; Hernandez-Sanabria et al., 2012; Myer et al., 2015), suggesting that the effect of Prevotella in the feed efficiency phenotype in cattle is species-specific (Perea et al., 2017).
Succinivibrio dextrinosolvens, the only representative species of the Succinivibrio genus, presented an increased relative abundance in the rumen fluid of High-RFI Hereford × Aberdeen Angus steers (Hernandez-Sanabria et al., 2012) and Nelore (Lopes et al., 2021). This species is usually abundant in animals fed with high starch diets (O’Herrin and Kenealy, 1993) and produces formate and SCFA, such as acetate and succinate (Russell and Hespell, 1981). Formate can be reduced to CO2, H2, and CH4 by the action of rumen methanogens (Lovley et al., 1984), which can lead to a significant loss of feed energy and, consequently, a reduced feed efficiency.
The Christensenellaceae (gut group R-7) produces acetate and butyrate as fermentation end products in the rumen and was identified as dominant in the ileum of high-feed conversion rate (FCR) pigs (Quan et al., 2018). This taxon was also associated with methane emission in Holstein cows (Ramayo-Caldas et al., 2020) a phenotype that can negatively influence feed efficiency (Hegarty et al., 2007). Interestingly, contrary to our results with the rumen microbiome, the family Christensenellaceae was linked to Low-RFI in the stool microbiome of Angus steers (Welch et al., 2021).
Regarding the stool environment, the only bacterial ASV associated with High-RFI was classified as belonging to the Ruminococcaceae UCG-005 genus-level cluster. Although the family Ruminococcaceae is highly abundant in the stool microbiome of ruminants (Andrade et al., 2020; Dai et al., 2021), there is limited literature exploring the relationship between members of this microbiome and feed efficiency phenotypes in ruminants. Nonetheless, members of this family were correlated with the FCR trait in pigs (McCormack et al., 2017; Aliakbari et al., 2021).
The ASVs abundancy associated with an increase of the feed efficiency trait in both rumen and stool environments were taxonomically classified as belonging to the Rikenellaceae (gut group RC9). The Rikenellaceae (gut group RC9), as other members of the Rikenellaceae family, can produce different SCFAs, such as propionate, acetate, and succinate, some with anti-inflammatory properties (Tedelind et al., 2007; Parada Venegas et al., 2019). Gut microbes associated with anti-inflammatory effects have been linked to gut health and with feed-efficient pigs, as inflammation may redirect feed energy that, otherwise, could be used for host growth and weight gain (Gardiner et al., 2020).
Besides, Welch et al. (2021) hypothesized that the link between highly feed-efficient animals and stool bacteria belonging to the Rikenellaceae family resides in the abundance of glycosaminoglycans present in their hindgut, as individuals from this family can use mucin as carbon and energy sources (Bomar et al., 2011), they could metabolize and have a competitive edge over other bacteria. Although this area is still in its infancy, recent studies have linked OTUs classified as belonging to this gut group, or to a lower taxonomic level such as the Rikenellaceae family. Species from this family have been linked to Low-RFI in the rumen of Nelore steers and in the stool microbiome of Angus steers, being also linked to Low-FCR in the stool microbiome of pigs (Quan et al., 2018; Lopes et al., 2021; Welch et al., 2021). Altogether, these results indicate a role of this specific taxon on this phenotype and suggest it as an RFI biomarker for highly efficient animals.
The GIT is a continuous and interconnected system, but the knowledge regarding the stool microbiome in ruminants is limited compared to the rumen microbiome for obvious reasons; however, it is a non-invasive sample that might represent a proxy for the rumen environment (Andrade et al., 2020). Nonetheless, identifying biomarkers for important production phenotypes in stool is advantageous as the sampling is less stressful and can be implemented in the animal routine. Understanding the biology of specific microorganisms that contribute to complex phenotypes may help to develop successful interventions for methane mitigation and feed efficiency improvement in bovines. Still, additional experiments have to be performed to assess the potential biomarkers identified in this study.
The feed composition induced significant differences in both abundance and richness of ruminal and stool microbial populations in ruminants of the Nelore breed. The dietary treatment based on industrial by-products applied to one of our experimental groups influenced the microbiome diversity of bacteria and archaea but not of protozoa. ASVs were associated with RCH4 emission and RFI in ruminal and stool microbiomes. While ruminal ASVs are expected to influence CH4 emission and feed efficiency, the relationship of stool taxa with these traits might be associated with host health, through their link to anti-inflammatory compounds. Overall, the ASVs identified have the potential to be used as biomarkers for these complex phenotypes.
All sequencing data are available in the NCBI Sequence Read Archive (SRA), under the bioproject number PRJNA638250.
Experimental procedures were conducted following Brazilian guidelines on animal welfare and approved by the Ethics Committee on the Use ofAnimals, College of Veterinary and Animal Science, São Paulo State University under protocol n° 8510190118.
BA, JK, AB, JP, JR, LC and LR conceived the experiment; BA and FB performed the experiments; BA, RC, HA, JP and GM performed analysis; BA, HA, RC, PO, AN, JR, SR, JM and LR interpreted the results and BA and LR drafted and revised the manuscript. All authors read and approved the final manuscript.
This research was conducted with funding from EMBRAPA, São Paulo Research Foundation scholarship to BGNA (FAPESP, grant number 2019/15557-7), São Paulo Research Foundation (FAPESP, grant number 2019/04089-2), and the National Council for Scientific and Technological Development (CNPq, grant number 428153/2018) and fellowships to LR and LC. This research has received funding from the European Union’s research and innovation program Horizon 2020 under the Marie Skłodowska-Curie grant agreement No. 801522 and Science Foundation Ireland and co-funded by the European Regional Development Fund through the ADAPT Centre for Digital Content Technology, grant number 13/RC/2106. This research has partially received funding by the Horizon 2020 projects STOP Obesity Platform under Grant Agreement No. 823978.
The authors declare that the research was conducted in the absence of any commercial or financial relationships that could be construed as a potential conflict of interest.
The handling editor SA declared a shared affiliation with the authors JP, GM, LC at the time of review.
All claims expressed in this article are solely those of the authors and do not necessarily represent those of their affiliated organizations, or those of the publisher, the editors, and the reviewers. Any product that may be evaluated in this article, or claim that may be made by its manufacturer, is not guaranteed or endorsed by the publisher.
The authors thank Marcela Morelli for collecting sample data and Leandro Sannomiya Sakamoto and the staff of the Embrapa Southeast Cattle Animal biotechnology laboratory for assistance in sample collection.
The Supplementary Material for this article can be found online at: https://www.frontiersin.org/articles/10.3389/fgene.2022.812828/full#supplementary-material
Supplementary Figure S1 | Rarefaction curves using the Shannon index. All datasets (A) bacteria, (B) archaea, and (C) protozoa achieved an early saturation based on this metric. Blue indicates rarefaction curves with rumen samples, and black, with stool samples.
Supplementary Figure S2 | (A) Comparison between observed ASV (amplicon sequence variant) metric between treatment groups showed no significant difference in protozoa populations of animals submitted to different diets. (B) Shannon index comparisons showed no significant difference (P<0.01) in the richness of protozoa populations. (C) PCoA using the rumen microbiome unweighted UniFrac distance showed no significant difference between groups (P > 0.1).
Supplementary Figure S3 | Vulcan plot showing 27 differentially abundant bacterial ASVs (amplicon sequence variants) of different genera and families in the rumen with W-value >0.9.
Supplementary Figure S4 | Vulcan plot showing three differentially abundant bacterial ASVs (amplicon sequence variants) of different genera and families in the stool with W-value >0.9.
Supplementary Figure S5 | Vulcan plot showing eight differentially abundant archaea ASVs (amplicon sequence variants) of different species, genera, and families in the rumen with W-value >0.9.
Supplementary Figure S6 | Vulcan plot showing seven differentially abundant archaea ASVs (amplicon sequence variants) of different species in the stool with W-value >0.9.
ASV, amplicon sequence variant; CLR, centered log-ratio; DA, differentially abundant; FCR, feed conversion rate; GIT, gastrointestinal tract; RCH4, residual methane emission; RFI, residual feed intake; and SCFA, short-chain fatty acid.
Abbott, D. W., Aasen, I. M., Beauchemin, K. A., Grondahl, F., Gruninger, R., Hayes, M., et al. (2020). Seaweed and Seaweed Bioactives for Mitigation of Enteric Methane: Challenges and Opportunities. Animals 10, 2432. doi:10.3390/ani10122432
Aliakbari, A., Zemb, O., Billon, Y., Barilly, C., Ahn, I., Riquet, J., et al. (2021). Genetic Relationships between Feed Efficiency and Gut Microbiome in Pig Lines Selected for Residual Feed Intake. J. Anim. Breed. Genet. 138, 491–507. doi:10.1111/jbg.12539
Amadeu, R. R., Cellon, C., Olmstead, J. W., Garcia, A. A. F., Resende, M. F. R., and Muñoz, P. R. (2016). AGHmatrix: R Package to Construct Relationship Matrices for Autotetraploid and Diploid Species: A Blueberry Example. Plant Genome 9. doi:10.3835/plantgenome2016.01.0009
Andrade, B. G. N., Bressani, F. A., Cuadrat, R. R. C., Tizioto, P. C., de Oliveira, P. S. N., Mourão, G. B., et al. (2020). The Structure of Microbial Populations in Nelore GIT Reveals Inter-dependency of Methanogens in Feces and Rumen. J. Anim. Sci Biotechnol 11. doi:10.1186/s40104-019-0422-x
Belkaid, Y., and Hand, T. W. (2014). Role of the Microbiota in Immunity and Inflammation. Cell 157, 121–141. doi:10.1016/j.cell.2014.03.011
Bolyen, E., Rideout, J. R., Dillon, M. R., Bokulich, N. A., Abnet, C. C., Al-Ghalith, G. A., et al. (2019). Reproducible, Interactive, Scalable and Extensible Microbiome Data Science Using QIIME 2. Nat. Biotechnol. 37, 852–857. doi:10.1038/s41587-019-0209-9
Bomar, L., Maltz, M., Colston, S., and Graf, J. (2011). Directed Culturing of Microorganisms Using Metatranscriptomics. mBio 2. doi:10.1128/mBio.00012-11
Callahan, B. J., McMurdie, P. J., Rosen, M. J., Han, A. W., Johnson, A. J. A., and Holmes, S. P. (2016). DADA2: High-Resolution Sample Inference from Illumina Amplicon Data. Nat. Methods 13, 581–583. doi:10.1038/nmeth.3869
Carberry, C. A., Kenny, D. A., Han, S., McCabe, M. S., and Waters, S. M. (2012). Effect of Phenotypic Residual Feed Intake and Dietary Forage Content on the Rumen Microbial Community of Beef Cattle. Appl. Environ. Microbiol. 78, 4949–4958. doi:10.1128/AEM.07759-11
Chang, C. C., Chow, C. C., Tellier, L. C., Vattikuti, S., Purcell, S. M., and Lee, J. J. (2015). Second-generation PLINK: Rising to the challenge of Larger and Richer Datasets. GigaSci 4, 7. doi:10.1186/s13742-015-0047-8
Chang, J., Peng, S., Ciais, P., Saunois, M., Dangal, S., Herrero, M., et al. (2019). Revisiting Enteric Methane Emissions from Domestic Ruminants and Their δ13CCH4 Source Signature Reflecting Diet and Environmental Shifts. doi:10.22022/ESM/06-2019.45
Chen, X., Zuo, Q., Hai, Y., and Sun, X. J. (2011). Lactulose: An Indirect Antioxidant Ameliorating Inflammatory Bowel Disease by Increasing Hydrogen Production. Med. Hypotheses 76, 325–327. doi:10.1016/j.mehy.2010.09.026
Cook, J., Oreskes, N., Doran, P. T., Anderegg, W. R. L., Verheggen, B., Maibach, E. W., et al. (2016). Consensus on Consensus: A Synthesis of Consensus Estimates on Human-Caused Global Warming. Environ. Res. Lett. 11, 048002. doi:10.1088/1748-9326/11/4/048002
Dai, Q., Ma, J., Cao, G., Hu, R., Zhu, Y., Li, G., et al. (2021). Comparative Study of Growth Performance, Nutrient Digestibility, and Ruminal and Fecal Bacterial Community between Yaks and Cattle-Yaks Raised by Stall-Feeding. AMB Expr. 11, 98. doi:10.1186/s13568-021-01259-9
de Oliveira, M. N. V., Jewell, K. A., Freitas, F. S., Benjamin, L. A., Tótola, M. R., Borges, A. C., et al. (2013). Characterizing the Microbiota across the Gastrointestinal Tract of a Brazilian Nelore Steer. Vet. Microbiol. 164, 307–314. doi:10.1016/j.vetmic.2013.02.013
Dill-McFarland, K. A., Weimer, P. J., Breaker, J. D., and Suen, G. (2019). Diet Influences Early Microbiota Development in Dairy Calves without Long-Term Impacts on Milk Production. Appl. Environ. Microbiol. 85, 1–12. doi:10.1128/AEM.02141-18
Donoghue, K. A., Bird-Gardiner, T., Arthur, P. F., Herd, R. M., and Hegarty, R. S. (2016). Repeatability of Methane Emission Measurements in Australian Beef Cattle. Anim. Prod. Sci. 56, 213. doi:10.1071/AN15573
Dowd, S. E., Callaway, T. R., Wolcott, R. D., Sun, Y., McKeehan, T., Hagevoort, R. G., et al. (2008). Evaluation of the Bacterial Diversity in the Feces of Cattle Using 16S rDNA Bacterial Tag-Encoded FLX Amplicon Pyrosequencing (bTEFAP). BMC Microbiol. 8, 125. doi:10.1186/1471-2180-8-125
Flint, H. J., Duncan, S. H., Scott, K. P., and Louis, P. (2007). Interactions and Competition within the Microbial Community of the Human colon: Links between Diet and Health. Environ. Microbiol. 9, 1101–1111. doi:10.1111/j.1462-2920.2007.01281.x
Gardiner, G. E., Metzler-Zebeli, B. U., and Lawlor, P. G. (2020). Impact of Intestinal Microbiota on Growth and Feed Efficiency in Pigs: A Review. Microorganisms 8, 1886. doi:10.3390/microorganisms8121886
Gilbert, J. a., and Dupont, C. L. (2011). Microbial Metagenomics: Beyond the Genome. Annu. Rev. Mar. Sci. 3, 347–371. doi:10.1146/annurev-marine-120709-142811
Guan, L. L., Nkrumah, J. D., Basarab, J. A., and Moore, S. S. (2008). Linkage of Microbial Ecology to Phenotype: Correlation of Rumen Microbial Ecology to Cattle's Feed Efficiency. FEMS Microbiol. Lett. 288, 85–91. doi:10.1111/j.1574-6968.2008.01343.x
Hegarty, R. S., Goopy, J. P., Herd, R. M., and McCorkell, B. (2007). Cattle Selected for Lower Residual Feed Intake Have Reduced Daily Methane Production1,2. J. Anim. Sci. 85, 1479–1486. doi:10.2527/jas.2006-236
Henderson, G., Cox, F., Cox, F., Ganesh, S., Jonker, A., Young, W., et al. (2015). Rumen Microbial Community Composition Varies with Diet and Host, but a Core Microbiome Is Found across a Wide Geographical Range. Sci. Rep. 5, 14567. doi:10.1038/srep14567
Hernandez-Sanabria, E., Goonewardene, L. A., Wang, Z., Durunna, O. N., Moore, S. S., and Guan, L. L. (2012). Impact of Feed Efficiency and Diet on Adaptive Variations in the Bacterial Community in the Rumen Fluid of Cattle. Appl. Environ. Microbiol. 78, 1203–1214. doi:10.1128/AEM.05114-11
Hespell, R. B. (1992). “The Genera Succinivibrio and Succinimonas,” in The Prokaryotes (New York, NYNew York: Springer), 3979–3982. doi:10.1007/978-1-4757-2191-1_60
Holman, D. B., and Gzyl, K. E. (2019). A Meta-Analysis of the Bovine Gastrointestinal Tract Microbiota. FEMS Microbiol. Ecol. 95, fiz072. doi:10.1093/femsec/fiz072
Kageyama, A., and Benno, Y. (2000). Phylogenic and Phenotypic Characterization of SomeEubacterium-like Isolates from Human Feces: Description ofSolobacterium mooreiGen. Nov., Sp. Nov. Microbiol. Immunol. 44, 223–227. doi:10.1111/j.1348-0421.2000.tb02487.x
Kaul, A., Mandal, S., Davidov, O., and Peddada, S. D. (2017). Analysis of Microbiome Data in the Presence of Excess Zeros. Front. Microbiol. 8, 1–10. doi:10.3389/fmicb.2017.02114
Kittelmann, S., Devente, S. R., Kirk, M. R., Seedorf, H., Dehority, B. A., and Janssen, P. H. (2015). Phylogeny of Intestinal Ciliates, Including Charonina Ventriculi, and Comparison of Microscopy and 18S rRNA Gene Pyrosequencing for Rumen Ciliate Community Structure Analysis. Appl. Environ. Microbiol. 81, 2433–2444. doi:10.1128/aem.03697-14
Kittelmann, S., Seedorf, H., Walters, W. A., Clemente, J. C., Knight, R., Gordon, J. I., et al. (2013). Simultaneous Amplicon Sequencing to Explore Co-occurrence Patterns of Bacterial, Archaeal and Eukaryotic Microorganisms in Rumen Microbial Communities. PloS one 8, e47879. doi:10.1371/journal.pone.0047879
Klindworth, A., Pruesse, E., Schweer, T., Peplies, J., Quast, C., Horn, M., et al. (2013). Evaluation of General 16S Ribosomal RNA Gene PCR Primers for Classical and Next-Generation Sequencing-Based Diversity Studies. Nucleic Acids Res. 41, e1. doi:10.1093/nar/gks808
Koch, R. M., Swiger, L. A., Chambers, D., and Gregory, K. E. (1963). Efficiency of Feed Use in Beef Cattle. J. Anim. Sci. 22, 486–494. doi:10.2527/jas1963.222486x
Larsen, N., Bussolo de Souza, C., Krych, L., Barbosa Cahú, T., Wiese, M., Kot, W., et al. (2019). Potential of Pectins to Beneficially Modulate the Gut Microbiota Depends on Their Structural Properties. Front. Microbiol. 10, 1–13. doi:10.3389/fmicb.2019.00223
Lassen, J., and Difford, G. F. (2020). Review: Genetic and Genomic Selection as a Methane Mitigation Strategy in Dairy Cattle. Animal 14, s473–s483. doi:10.1017/S1751731120001561
Lee, E.-H., Moon, K.-E., Kim, T. G., Lee, S.-D., and Cho, K.-S. (2015). Inhibitory Effects of Sulfur Compounds on Methane Oxidation by a Methane-Oxidizing Consortium. J. Biosci. Bioeng. 120, 670–676. doi:10.1016/j.jbiosc.2015.04.006
Lee, G.-H., Kumar, S., Lee, J.-H., Chang, D.-H., Kim, D.-S., Choi, S.-H., et al. (2012). Genome Sequence of Oscillibacter Ruminantium Strain GH1, Isolated from Rumen of Korean Native Cattle. J. Bacteriol. 194, 6362. doi:10.1128/JB.01677-12
Lopes, D. R. G., de Souza Duarte, M., La Reau, A. J., Chaves, I. Z., de Oliveira Mendes, T. A., Detmann, E., et al. (2021). Assessing the Relationship between the Rumen Microbiota and Feed Efficiency in Nellore Steers. J. Anim. Sci Biotechnol 12, 79. doi:10.1186/s40104-021-00599-7
Lovley, D. R., Greening, R. C., and Ferry, J. G. (1984). Rapidly Growing Rumen Methanogenic Organism that Synthesizes Coenzyme M and Has a High Affinity for Formate. Appl. Environ. Microbiol. 48, 81–87. doi:10.1128/aem.48.1.81-87.1984
Machado, L., Magnusson, M., Paul, N. A., de Nys, R., and Tomkins, N. (2014). Effects of Marine and Freshwater Macroalgae on In Vitro Total Gas and Methane Production. PLoS ONE 9, e85289. doi:10.1371/journal.pone.0085289
Mallick, H., Rahnavard, A., McIver, L. J., Ma, S., Zhang, Y., Nguyen, L. H., et al. (2021). Multivariable Association Discovery in Population-Scale Meta-Omics Studies. PLoS Comput. Biol. 17, e1009442. doi:10.1371/journal.pcbi.1009442
Marounek, M., and Duskova, D. (1999). Metabolism of Pectin in Rumen Bacteria Butyrivibrio Fibrisolvens and Prevotella Ruminicola. Lett. Appl. Microbiol. 29, 429–433. doi:10.1046/j.1472-765X.1999.00671.x
McCormack, U. M., Curião, T., Buzoianu, S. G., Prieto, M. L., Ryan, T., Varley, P., et al. (2017). Exploring a Possible Link between the Intestinal Microbiota and Feed Efficiency in Pigs. Appl. Environ. Microbiol. 83, 1–16. doi:10.1128/AEM.00380-17
Meo, S. C., Ferreira, C. R., Chiaratti, M. R., Meirelles, F. V., Regitano, L. C. A., Alencar, M. M., et al. (2009). Characterization of Mitochondrial Genotypes in the Foundation Herd of the Canchim Beef Cattle Breed. Genet. Mol. Res. 8, 261–267. doi:10.4238/vol8-1gmr577
Mudadu, M. A., Porto-Neto, L. R., Mokry, F. B., Tizioto, P. C., Oliveira, P. S. N., Tullio, R. R., et al. (2016). Genomic Structure and Marker-Derived Gene Networks for Growth and Meat Quality Traits of Brazilian Nelore Beef Cattle. BMC Genomics 17, 235. doi:10.1186/s12864-016-2535-3
Myer, P. R., Smith, T. P. L., Wells, J. E., Kuehn, L. A., and Freetly, H. C. (2015). Rumen Microbiome from Steers Differing in Feed Efficiency. PLoS ONE 10, e0129174–17. doi:10.1371/journal.pone.0129174
Neumann, A. P., McCormick, C. A., and Suen, G. (2017). Fibrobactercommunities in the Gastrointestinal Tracts of Diverse Hindgut-Fermenting Herbivores Are Distinct from Those of the Rumen. Environ. Microbiol. 19, 3768–3783. doi:10.1111/1462-2920.13878
Noel, S. J., Olijhoek, D. W., Mclean, F., Løvendahl, P., Lund, P., and Højberg, O. (2019). Rumen and Fecal Microbial Community Structure of Holstein and Jersey Dairy Cows as Affected by Breed, Diet, and Residual Feed Intake. Animals 9, 498. doi:10.3390/ani9080498
O’Herrin, S. M., and Kenealy, W. R. (1993). Glucose and Carbon Dioxide Metabolism by Succinivibrio Dextrinosolvens. Appl. Environ. Microbiol. 59, 748–755.
Pachauri, R. K., and Mayer, L. (2015). “Climate Change 2014: Synthesis Report,” in Intergovernmental Panel on Climate Change (Geneva, Switzerland: Intergovernmental Panel on Climate Change).
Parada Venegas, D., De la Fuente, M. K., Landskron, G., González, M. J., Quera, R., Dijkstra, G., et al. (2019). Short Chain Fatty Acids (SCFAs)-Mediated Gut Epithelial and Immune Regulation and its Relevance for Inflammatory Bowel Diseases. Front. Immunol. 10, 277. doi:10.3389/fimmu.2019.00277
Parker, B. J., Wearsch, P. A., Veloo, A. C. M., and Rodriguez-Palacios, A. (2020). The Genus Alistipes: Gut Bacteria with Emerging Implications to Inflammation, Cancer, and Mental Health. Front. Immunol. 11, 906. doi:10.3389/fimmu.2020.00906
Perea, K., Perz, K., Olivo, S. K., Williams, A., Lachman, M., Ishaq, S. L., et al. (2017). Feed Efficiency Phenotypes in Lambs Involve Changes in Ruminal, Colonic, and Small-Intestine-Located Microbiota1. J. Anim. Sci. 95, 2585–2592. doi:10.2527/jas.2016.1222
Pianta, A., Arvikar, S., Strle, K., Drouin, E. E., Wang, Q., Costello, C. E., et al. (2017). Evidence of the Immune Relevance of Prevotella Copri , a Gut Microbe, in Patients with Rheumatoid Arthritis. Arthritis Rheumatol. 69, 964–975. doi:10.1002/art.40003
Polansky, O., Sekelova, Z., Faldynova, M., Sebkova, A., Sisak, F., and Rychlik, I. (2016). Important Metabolic Pathways and Biological Processes Expressed by Chicken Cecal Microbiota. Appl. Environ. Microbiol. 82, 1569–1576. doi:10.1128/AEM.03473-15
Popova, M., Guyader, J., Silberberg, M., Seradj, A. R., Saro, C., Bernard, A., et al. (2019). Changes in the Rumen Microbiota of Cows in Response to Dietary Supplementation with Nitrate, Linseed, and Saponin Alone or in Combination. Appl. Environ. Microbiol. 85, 1–16. doi:10.1128/AEM.02657-18
Pszczola, M., Strabel, T., Mucha, S., and Sell-Kubiak, E. (2018). Genome-wide Association Identifies Methane Production Level Relation to Genetic Control of Digestive Tract Development in Dairy Cows. Sci. Rep. 8, 15164. doi:10.1038/s41598-018-33327-9
Quan, J., Cai, G., Ye, J., Yang, M., Ding, R., Wang, X., et al. (2018). A Global Comparison of the Microbiome Compositions of Three Gut Locations in Commercial Pigs with Extreme Feed Conversion Ratios. Sci. Rep. 8, 1–10. doi:10.1038/s41598-018-22692-0
Quast, C., Pruesse, E., Yilmaz, P., Gerken, J., Schweer, T., Yarza, P., et al. (2013). The SILVA Ribosomal RNA Gene Database Project: Improved Data Processing and Web-Based Tools. Nucleic Acids Res. 41, D590–D596. doi:10.1093/nar/gks1219
Ramayo‐Caldas, Y., Zingaretti, L., Popova, M., Estellé, J., Bernard, A., Pons, N., et al. (2020). Identification of Rumen Microbial Biomarkers Linked to Methane Emission in Holstein Dairy Cows. J. Anim. Breed. Genet. 137, 49–59. doi:10.1111/jbg.12427
Russell, J. B., and Hespell, R. B. (1981). Microbial Rumen Fermentation. J. Dairy Sci. 64, 1153–1169. doi:10.3168/jds.S0022-0302(81)82694-X
Sahoo, P. K., Kim, K., and Powell, M. A. (2016). Managing Groundwater Nitrate Contamination from Livestock Farms: Implication for Nitrate Management Guidelines. Curr. Pollut. Rep 2, 178–187. doi:10.1007/s40726-016-0033-5
Scarpato, E., Coppola, V., and Staiano, A. (2019). “Probiotics and Applications to Constipation,” in Dietary Interventions in Gastrointestinal Diseases (Elsevier), 193–196. doi:10.1016/B978-0-12-814468-8.00015-6
Seedorf, H., Kittelmann, S., Henderson, G., and Janssen, P. H. (2014). RIM-DB: a Taxonomic Framework for Community Structure Analysis of Methanogenic Archaea from the Rumen and Other Intestinal Environments. PeerJ 2, e494. doi:10.7717/peerj.494
Shi, W., Moon, C. D., Leahy, S. C., Kang, D., Froula, J., Kittelmann, S., et al. (2014). Methane Yield Phenotypes Linked to Differential Gene Expression in the Sheep Rumen Microbiome. Genome Res. 24, 1517–1525. doi:10.1101/gr.168245.113.1
Solden, L., Lloyd, K., and Wrighton, K. (2016). The Bright Side of Microbial Dark Matter: Lessons Learned from the Uncultivated Majority. Curr. Opin. Microbiol. 31, 217–226. doi:10.1016/j.mib.2016.04.020
Stephen, A. S., Naughton, D. P., Pizzey, R. L., Bradshaw, D. J., and Burnett, G. R. (2014). In Vitro growth Characteristics and Volatile Sulfur Compound Production of Solobacterium Moorei. Anaerobe 26, 53–57. doi:10.1016/j.anaerobe.2014.01.007
Sugitani, Y., Inoue, R., Inatomi, O., Nishida, A., Morishima, S., Imai, T., et al. (2021). Mucosa-associated Gut Microbiome in Japanese Patients with Functional Constipation. J. Clin. Biochem. Nutr. 68, 187–192. doi:10.3164/jcbn.20-93
Tedelind, S., Westberg, F., Kjerrulf, M., and Vidal, A. (2007). Anti-inflammatory Properties of the Short-Chain Fatty Acids Acetate and Propionate: A Study with Relevance to Inflammatory Bowel Disease. WJG 13, 2826. doi:10.3748/wjg.v13.i20.2826
Tian, L., Wang, X.-W., Wu, A.-K., Fan, Y., Friedman, J., Dahlin, A., et al. (2020). Deciphering Functional Redundancy in the Human Microbiome. Nat. Commun. 11, 6217. doi:10.1038/s41467-020-19940-1
Ungerfeld, E. M. (2015). Shifts in Metabolic Hydrogen Sinks in the Methanogenesis-Inhibited Ruminal Fermentation: a Meta-Analysis. Front. Microbiol. 6. doi:10.3389/fmicb.2015.00037
van Gylswyk, N. O. (1995). Succiniclasticum Ruminis Gen. nov., Sp. nov., a Ruminal Bacterium Converting Succinate to Propionate as the Sole Energy-Yielding Mechanism. Int. J. Syst. Bacteriol. 45, 297–300. doi:10.1099/00207713-45-2-297
VanRaden, P. M. (2008). Efficient Methods to Compute Genomic Predictions. J. Dairy Sci. 91, 4414–4423. doi:10.3168/jds.2007-0980
Wang, K., Nan, X., Chu, K., Tong, J., Yang, L., Zheng, S., et al. (2018). Shifts of Hydrogen Metabolism from Methanogenesis to Propionate Production in Response to Replacement of Forage Fiber with Non-forage Fiber Sources in Diets In Vitro. Front. Microbiol. 9, 1–12. doi:10.3389/fmicb.2018.02764
Waters, J. L., and Ley, R. E. (2019). The Human Gut Bacteria Christensenellaceae Are Widespread, Heritable, and Associated with Health. BMC Biol. 17, 1–11. doi:10.1186/s12915-019-0699-4
Welch, C. B., Lourenco, J. M., Krause, T. R., Seidel, D. S., Fluharty, F. L., Pringle, T. D., et al. (2021). Evaluation of the Fecal Bacterial Communities of Angus Steers with Divergent Feed Efficiencies across the Lifespan from Weaning to Slaughter. Front. Vet. Sci. 8, 597405. doi:10.3389/fvets.2021.597405
Williams, M., Zalasiewicz, J., Haff, P., Schwägerl, C., Barnosky, A. D., and Ellis, E. C. (2015). The Anthropocene Biosphere. Anthropocene Rev. 2, 196–219. doi:10.1177/2053019615591020
Wolin, M. J. (1960). A Theoretical Rumen Fermentation Balance. J. Dairy Sci. 43, 1452–1459. doi:10.3168/jds.S0022-0302(60)90348-9
Keywords: association, archaea, bacteria, biomarkers, Bos indicus, feed efficiency, methane emission
Citation: Andrade BGN, Bressani FA, Cuadrat RRC, Cardoso TF, Malheiros JM, de Oliveira PSN, Petrini J, Mourão GB, Coutinho LL, Reecy JM, Koltes JE, Neto AZ, R. de Medeiros S, Berndt A, Palhares JCP, Afli H and Regitano LCA (2022) Stool and Ruminal Microbiome Components Associated With Methane Emission and Feed Efficiency in Nelore Beef Cattle. Front. Genet. 13:812828. doi: 10.3389/fgene.2022.812828
Received: 10 November 2021; Accepted: 02 March 2022;
Published: 17 May 2022.
Edited by:
Sonia Andrade, University of São Paulo, BrazilReviewed by:
Androniki Psifidi, Royal Veterinary College (RVC), United KingdomCopyright © 2022 Andrade, Bressani, Cuadrat, Cardoso, Malheiros, de Oliveira, Petrini, Mourão, Coutinho, Reecy, Koltes, Neto, R. de Medeiros, Berndt, Palhares, Afli and Regitano. This is an open-access article distributed under the terms of the Creative Commons Attribution License (CC BY). The use, distribution or reproduction in other forums is permitted, provided the original author(s) and the copyright owner(s) are credited and that the original publication in this journal is cited, in accordance with accepted academic practice. No use, distribution or reproduction is permitted which does not comply with these terms.
*Correspondence: Haithem Afli, aGFpdGhlbS5hZmxpQG10dS5pZQ==; Luciana C. A. Regitano, bHVjaWFuYS5yZWdpdGFub0BlbWJyYXBhLmJy
Disclaimer: All claims expressed in this article are solely those of the authors and do not necessarily represent those of their affiliated organizations, or those of the publisher, the editors and the reviewers. Any product that may be evaluated in this article or claim that may be made by its manufacturer is not guaranteed or endorsed by the publisher.
Research integrity at Frontiers
Learn more about the work of our research integrity team to safeguard the quality of each article we publish.