- 1Benchmark Genetics Norway AS (formerly Akvaforsk Genetics Center AS), Sunndalsøra, Norway
- 2Laboratory of Ichthyology, Aquaculture and Diseases of Aquatic Animals, Department of Marine Sciences, University of The Aegean, Mytilene, Greece
- 3Nireus Aquaculture SA., Koropi, Greece
- 4CNRS, IRD, EPHE, ISEM, Université de Montpellier, Montpellier, France
- 5Biotechnology and Aquaculture (IMBBC), Hellenic Centre for Marine Research (HCMR), Institute of Marine Biology, Heraklion, Greece
- 6Nofima AS, Tromsø, Norway
Viral nervous necrosis (VNN) is an infectious disease caused by the red-spotted grouper nervous necrosis virus (RGNNV) in European sea bass and is considered a serious concern for the aquaculture industry with fry and juveniles being highly susceptible. To understand the genetic basis for resistance against VNN, a survival phenotype through the challenge test against the RGNNV was recorded in populations from multiple year classes (YC2016 and YC2017). A total of 4,851 individuals from 181 families were tested, and a subset (n∼1,535) belonging to 122 families was genotyped using a ∼57K Affymetrix Axiom array. The survival against the RGNNV showed low to moderate heritability with observed scale estimates of 0.18 and 0.25 obtained using pedigree vs. genomic information, respectively. The genome-wide association analysis showed a strong signal of quantitative trait loci (QTL) at LG12 which explained ∼33% of the genetic variance. The QTL region contained multiple genes (ITPK1, PLK4, HSPA4L, REEP1, CHMP2, MRPL35, and SCUBE) with HSPA4L and/or REEP1 genes being highly relevant with a likely effect on host response in managing disease-associated symptoms. The results on the accuracy of predicting breeding values presented 20–43% advantage in accuracy using genomic over pedigree-based information which varied across model types and applied validation schemes.
Introduction
The aquaculture industry is expected to fulfill an increased demand of fish for human consumption as fisheries realize their resources reach their limits. The need to increase production and fulfill current and future demands comes together with challenges in long-term sustainability and production value, such as diseases outbreaks, feed supplies, water pollution, and global warming.
Diseases are a major challenge affecting fish welfare and financial losses for the aquaculture industry, particularly those from viral nature due to high mortalities and reduced growth on affected fish. Viral nervous necrosis (VNN)—also known as viral encephalopathy and retinopathy (VER)—is one of the main infectious diseases affecting marine aquaculture. The disease is caused by the red-spotted grouper nervous necrosis virus (RGNNV) which belongs to the genus Betanodavirus of the family Nodaviridae (OIE, 2019). VNN has been found in up to 120 species from marine and freshwater environments including European sea bass (Dicentrarchus labrax), the most cultured species in the Mediterranean whose annual production reached 168,642.57 tonnes in 2018 valued on $1,03 billion USD, FAO. Recently, a reassortant betanodavirus (RGNNV/SJNNV) has also been reported in gilthead sea bream (Sparus aurata) with a postulated asymptomatic contagious host for transmission of disease to European sea bass (Toffan et al., 2017).
Viral nervous necrosis can be transmitted horizontally and vertically (Hazreen-Nita et al., 2019). The acute phase of the disease has been associated to the elevated sea temperature where nervous signs appear, while the sub-acute form is described as necrosis on the upper jaw and head regions and has been linked to lower temperatures (Le Breton et al., 1997). VNN is cataloged as the most important problem in the Mediterranean mariculture (Vendramin et al., 2016), leading a number of attempts to produce a vaccine (e.g., Stein et al., 2013; Valero et al., 2016; Gonzalez-Silvera et al., 2019). However, despite the approval of a commercial vaccine to protect sea bass against the most common VNN genotype in the Mediterranean, its use on mass scale is still limited due to its cost as single vaccine and technical and logistic problems presented when combined with other vaccines against vibriosis and pasteurellosis. In addition, the global warming trend and the temperature dependent nature of VNN remain a threat for the sustainable production of this species (Costa and Thompson, 2016).
Selective breeding is an effective tool to continuously improve traits such as disease resistance (Gjedrem and Rye, 2018), usually by evaluating the trait on sibs of breeding candidates. Reduction in the genotyping cost and turnaround time for a large number of markers per sample has opened the door to move and implement individual selection in sib-evaluated traits such as disease resistance; this is performed either by using markers linked to a major gene influencing the trait or by implementing genomic selection (GS), leading to higher genetic gains compared to more traditional methods (Dekkers, 2007). Such programs have become a routine to improve economically important traits such as survival against pathogens in species such as Atlantic salmon, rainbow trout, and Nile tilapia.
Major factors influencing the success of a breeding program are high quality, robust and reproducible phenotypic data, and the maintenance of a reliable pedigree; furthermore, when introducing advanced genomic methods, this extends to the use of genome-wide distributed, reliable, reproducible molecular genetic markers (e.g., single nucleotide polymorphisms, SNPs). The GS provides the opportunity to rank individuals within and across families with higher accuracy (Meuwissen et al., 2001). The availability of resources including reference genomes, linkage maps, genotyping arrays, etc., convenes the applicability of the GS along with genome-wide association studies. Not long ago, the reference genome of European sea bass became available (Tine et al., 2014), and very recently, the SNP genotyping Axiom arrays were also developed, which are a part of the public domain now (Griot et al., 2021; Peñaloza et al., 2021). There are several studies on different aquaculture species where quantitative trait loci (QTL) were detected for traits of economic and welfare importance using medium- to high-density SNP arrays. The major QTLs detected in Atlantic salmon for different traits include resistance against the infectious pancreatic necrosis virus (Houston et al., 2008; Moen et al., 2009), resistance against the piscine myocarditis virus (Boison et al., 2019; Hillestad and Moghadam, 2019), age at sexual maturity (Barson et al., 2015), and fillet color (Baranski et al., 2010). QTLs have also been detected in European sea bass with moderate to low impact. The main targeted traits in sea bass for QTL detections included body weight, resistance against VNN disease, and stress resistance (Chatziplis et al., 2007; Palaiokostas et al., 2018; Chatziplis et al., 2020; Griot et al., 2021). The detected QTLs with major effects can assist in implementation of cost-effective marker-assisted selection (MAS). However, among other factors, the nature of trait(s) in a breeding program affected by a few vs. many genes along with the strategy of selection/culling within the course of the growth cycle plays a major role for the adoption/application of advanced selection methods (MAS vs. GS). The GS has shown the advantage over the classical pedigree method by enabling the prediction of breeding values for the candidates with higher accuracy (Tsai et al., 2016; Correa et al., 2017; Vallejo et al., 2017; Aslam M. L. et al., 2020; Aslam M. L. et al., 2020). The application of the GS becomes highly important and efficient for lowly heritable and difficult traits which are usually not recorded on live candidates (e.g., carcass quality and disease resistance traits which are currently recorded on sibs of live selection candidates) and also allows selecting the best individuals for such traits instead of best families with traditional methods.
In this study, we investigated the genetic variation for resistance to the RGNNV from a challenge test in a commercial European sea bass breeding program performed during two consecutive years. In addition, fish from the same challenge tests were genotyped to detect QTL for survival against the RGNNV and assess the potential of the GS to improve resistance against VNN.
Materials and Methods
Experimental Population
The presented results are based on challenge testing of two different year classes in the Nireus SA’s breeding nucleus of European sea bass (Thorland et al., 2017; Nirea et al., 2021). The commercial family-based breeding program operates with overlapping generations, and the presented experimental population is part of year classes 2016 (YC2016) and 2017 (YC2017). These year classes have a selection history of between three and four full cycles of selection from families in base population (year-classes 2004 and 2005) and are in the program defined in generations between F3 and F4. Families in the breeding program are produced by artificial stripping of mature females and males and subsequent controlled crossings in the family design including both maternal and paternal half-sibs.
Parents to both year classes included in this study were photoperiod-manipulated to mature out of the natural spawning season. YC2016, consisting of 89 full-sibs families produced from 65 sires and 30 dams, generated between September 3 and October 10, 2016; YC2017 consisted of 92 full-sib families derived from 85 sires and 35 dams produced by artificial stripping in the period September 17 to October 13, 2017. In the mating design, each female parent was mated with one to four males with an average of 2.8 for YC2016 and 2.6 for YC2017. Each male parent was mated with one to four females (on average 1.4 females per male) in YC2016, whereas in YC2017 each male parent was mated to one to two with an average of 1.1 females per male. The average age of the male broodstock was 3.9 years post-hatching for YC2016 and 3.6 years for YC2017, while for the female broodstock, the average age was 3.5 and 4.0 years for YC2016 and YC2017, respectively. The use of broodstock of different years of age and the structure with overlapping generations builds a strong genetic tie between year classes in the breeding nucleus which allows for combined analysis of data from both year classes in this experimental population.
Families were reared in separate circular tanks of 315-L capacity at the Enalios-Breeding Programme hatchery of Nireus, S.A., located in Central Evia. Approximately 30 individuals per family were individually PIT-tagged using 8-mm Biomark tags. The PIT tagging for YC2016 was performed at an average body weight of 10.9 g (sd = 2.5 g) between March 21 and April 12, 2017, whereas the PIT tagging for YC2017 was performed from March 20 to March 23, 2018, at an average weight of 8.9 g (sd = 1.7 g). All PIT-tagged individuals within each year class for the specific challenge test were stocked in a single tank after transportation to the challenge test facilities at the laboratory of Ichthyology, Aquaculture and Diseases of Aquatic Organisms (ICHTHYAI), Department of Marine Sciences, at the University of Aegean in Lesvos, Greece. The date of transportation of individuals to the challenge test facility was April 24 in 2017 (YC2016) and June 13 and 15 in 2018 (YC2017).
Challenge Tests for Viral Nervous Necrosis
Both year classes (YC2016 and YC2017) were challenged according to similar protocols using a European sea bass RGNNV isolate from a commercial fish farm outbreak in 2012, and the virus challenge was performed with intramuscular (IM) injection. In YC2016, sea bass individuals with an average weight of 14.4 g were injected with a challenge dose of 106 TCID50/ml, defined from in-house pre-trials. The fish were injected on May 13, 2017 and distributed randomly to three experimental tanks of 2 m3, and mortalities were recorded every 4 h for a period of 28 days after infection (with no exceptions including recordings during nights and weekends), until mortality had stopped. The second challenge test was conducted on YC2017 (June 24, 2018) with similar conditions and environment as on YC2016, but the infection dose was 5 × 106 TCID50/ml. YC2017 had an initial average body weight of 25.3 g in the test. All fish were fin-clipped before the challenge test, and tissues were preserved in 95% ethanol and stored at −4°C.
Genotyping
In order to reduce genotyping costs, challenge-tested individuals (n = 4,851) were subsided from both year classes (YC2016 and YC2017). Hence, out of total 4,851 individuals, a subsample of 1,535 individuals were selected for genotyping comprising 767 individuals belonging to 30 families of YC2016 and 768 individuals from 92 families of YC2017. Since the accuracy of selection using genomic information would be highly dependent on the relationship of training (the training group is referred to the individuals with phenotype information available from the same generation, normally the full sibs of candidates) and tested (referred to individuals without phenotypic records i.e., the candidate group) population, pedigree relationships were considered to select individuals from families with genetic links to contemporary families. Tissue samples from selected fish were used for the DNA extraction and genotyping using the SNPs-based ∼57K Affymetrix Axiom array (DlabCHIP, Griot et al., 2021), and the position of markers was determined based on the genome build 1.0 of European sea bass (seabass_V1.0, Tine et al., 2014). Genotyping was performed at the Gentyane facility, Clermont-Ferrand, France.
The raw genotype data were quality-filtered by PLINK software (Purcell et al., 2007). SNP markers with the minor allele frequency (MAF) lower than 5%, missing rate higher than 10%, and those not passing the Hardy–Weinberg equilibrium exact test (p < 1.0 × 10−6) were excluded from the data. Filtering was also performed at the individual level with individuals removed based on the missing genotype rate (>10%) and poor heterozygosity (higher than ±3sd of population). The filtering step retained a total of 1,489 individuals (754 and 735 for YC2016 and YC2017, respectively) from 120 full-sib families of which 28 families represented YC2016 (15–30 sibs per family), and 92 families from YC2017 (4–14 sibs per family) genotyped with approximately 52K SNPs.
Statistical Analyses
Data description and statistics: The statistics for the recorded traits and the initial evaluation of models were obtained using the generalized linear model in statistical software “R”. With the full dataset (n = 4,851) year classes and the family effects were tested; year classes did not show significant effects (p = 0.106), while the family effect was highly significant with p < 0.001. Though the effect of year classes was not significant, it was still used in the model to avoid year class-specific deviations in estimates. The analysis and/or results presented below are mainly focused on using the combined dataset from both year classes (YC2016 and YC2017) unless otherwise specified.
Quantitative genetic parameter estimation: Estimation of genetic parameters was conducted for the binary survival trait recorded at the end of test(s) (1 = survival; 0 = dead). The analysis was conducted including either all data (nind = 4,851) or the genotyped subset of the data (nind = 1,489) summarized in Table 1. Two models (Model-1 and Model-2) were applied to estimate the variance component by ASReml software (Gilmour et al., 2015). Model-1 was the linear mixed animal model which can be written as follows:
where
Model-2 was a sire-dam model where the binary data (1 = survival; 0 = dead) were fitted to a threshold model using the “probit” link function. In matrix notation, the model can be written as follows:
where
From Model-1, heritability was estimated as
where
Genome-wide association analysis (GWAS): Genome-wide association analysis was performed using the linear mixed animal model implemented in the GCTA program with the “--mlma-loco” function (Yang et al., 2011a). The “--mlma-loco” function allows the estimation of an SNP effect by accounting the additive genetic variance expressed by all the markers distributed over all the linkage groups other than the linkage group which contains the SNP. The method of leaving one chromosome out (the chromosome carrying the marker in question for which the effect and association must be computed) increases the power of the association analysis. This removal of the marker in question and all the other linked markers from the chromosome avoids double-fitting/adjusting in the model, both as a fixed effect tested for association and as a random component by including in the genomic relationship matrix (Yang et al., 2014). The model used in GWAS was similar to Model-1 except that the first five eigenvectors computed from the genomic relationship matrix were included as covariates in the model, and the common environment effect was excluded. The eigenvectors as covariates in genome-wide association analysis are usually used to adjust for population stratification and control spurious genetic associations caused by false linkages of markers with the population structure instead of true marker trait associations (Price et al., 2006). The
The SNP markers were categorized as genome-wide significant when they surpass the Bonferroni threshold for multiple testing p-value of
Estimation of the SNP(s) variance: The proportion of the genetic variance explained by the top significant SNP(s) were computed using two methods. One is the direct method where genetic variances of SNPs were computed using allele frequencies and allele substitution effects as
Breeding value estimation: Breeding values for the survival (binary, 0/1 trait) against VNN were computed through model-1 without using random effects common to full-sibs (
Accuracy of prediction: For the evaluation of accuracy of predictions, two datasets were used, 1) genotyped individuals (n = 1,489) and 2) a full dataset (n = 4,851, genotyped and ungenotyped individuals). The comparison for the accuracy of predictions among the genomic and pedigree models was performed using genotyped individuals only, while PBLUP and HBLUP models were compared using the full dataset (n = 4,851).
To compare prediction accuracies, validation schemes were designed in two ways.
1) Within family masking: Under this scheme, 30% of individuals within each family were randomly masked, which produced 447 individuals as validation animals, and the remaining 1,042 individuals with available phenotypes were kept as the training set.
2) Random masking: Under this scheme, 30% of the individuals were randomly masked without giving any consideration on families. Hence, 1,042 individuals were used for training and 447 as validation animals.
The adopted schemes for the comparison of PBLUP vs. HBLUP were the same as 1) and 2), but the number for validation and training individuals ended up large with 1,455 validation and 3,396 training animals. The breeding values for the masked individuals were computed using pedigree, genomic, and the hybrid relationship matrices The mean accuracy of 20 replicates was computed as the correlation (
Results
Challenge Test
The challenge test resulted significant and very similar mortalities with 58.2 and 59.3% for YC2016 (Bakopoulos et al., 2018) and YC2017, respectively (Figure 1). Mortalities started to appear during the first and second day after infection in YC2016 and YC2017, respectively, reaching a peak during day 5 for both challenge tests. Mortalities ceased before termination of the trial approx. on day 20 after challenge. All mortalities were recorded, and the individuals who survived until day ∼20 from the start of the challenge were considered as survivors (alive). The distribution of mortalities in each full-sib family along with the distribution of full-sibs per family is given in Supplementary Figure S1.1.
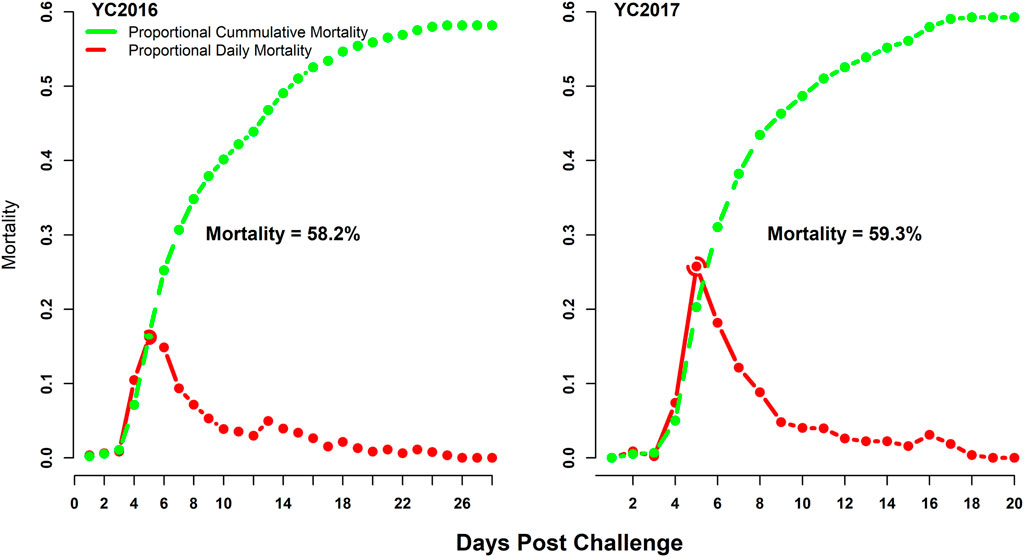
FIGURE 1. Distribution of mortalities in YC2016 and YC2017 during the challenge test against the nervous necrosis virus.
Data Statistics
The recorded data contained 4,851 observations (
Genetic Parameters
The observed and the liability scale heritability estimates obtained using genomic information were higher than the pedigree-based estimates (Table 2). The observed scale heritability estimates obtained using the large dataset (including YC2016 and YC2017) were 0.18 ± 0.03 and 0.25 ± 0.04 for pedigree and genomic information, respectively. The liability scale heritability estimates obtained using pedigree information were 0.27 ± 0.04 while the same scale estimates with genomic information were 0.40 ± 0.06, respectively. The estimates for the environment common to full sibs,
Genome-wide Association Analysis
The GWAS analysis from the combined dataset of both year classes (YC2016 and YC 2017) revealed a strong signal of QTL at LG12 with 72 SNPs crossing the genome-wide threshold (Figure 2). Moreover, the analyses on individual year class (YC2016 or YC2017) were also performed which showed a consistent strong peak of QTL at LG12 (Supplementary Figure S1.2). The details for the top ten highest significant SNPs with their position in genome and allele substitution effects, along with the genetic variances explained by the SNPs, are given in Table 3. The detected genome-wide significant SNPs (n = 72) cover a large region of 15 Mb (3,778,017 bp to 18,822,298 bp) with the top significant SNP AX-172280857 centered at 11,359,282 bp (Figure 3). However, when the most significant SNP (AX-172280857) was used as an additional fixed effect in the model (as explained under the indirect method for computation of SNP variance), none of the SNPs showed any chromosome and/or genome-wide significance, indicating that there is only one QTL in the region (Supplementary Figure S1.3). A quantile–quantile plot (QQ-plot) obtained using
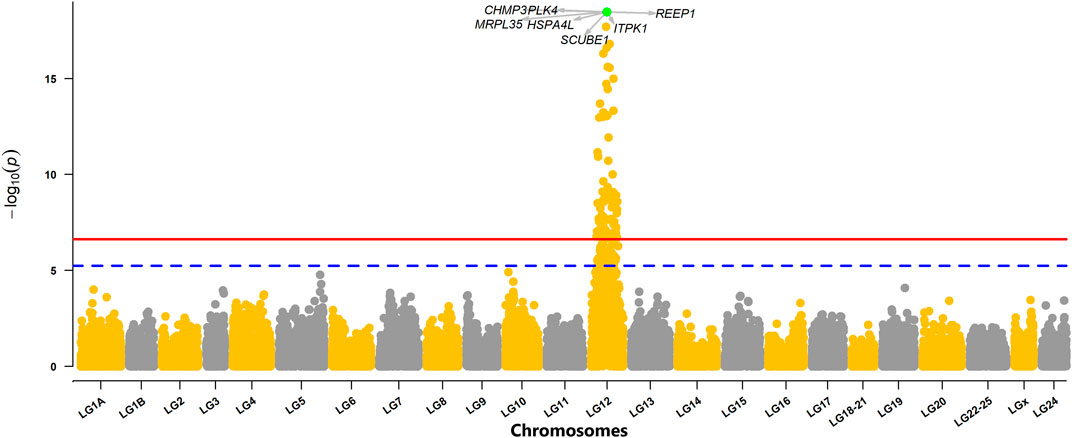
FIGURE 2. Manhattan plot with the distribution of
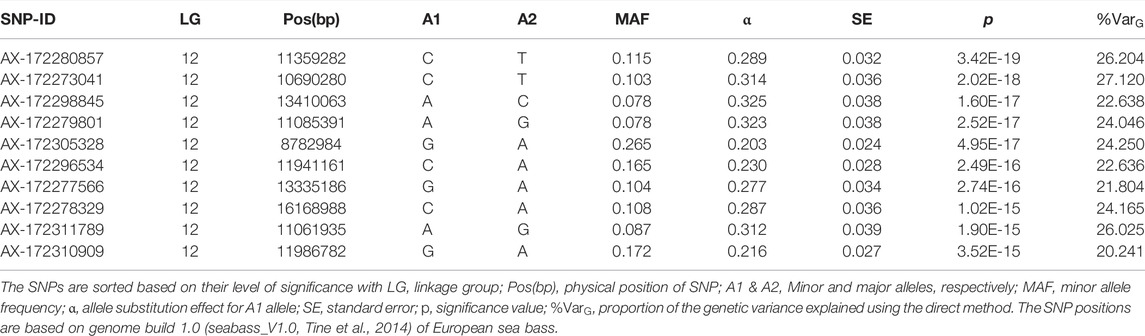
TABLE 3. The top 10 significant SNPs detected in GWAS analysis ranked with respect to the level of significance.
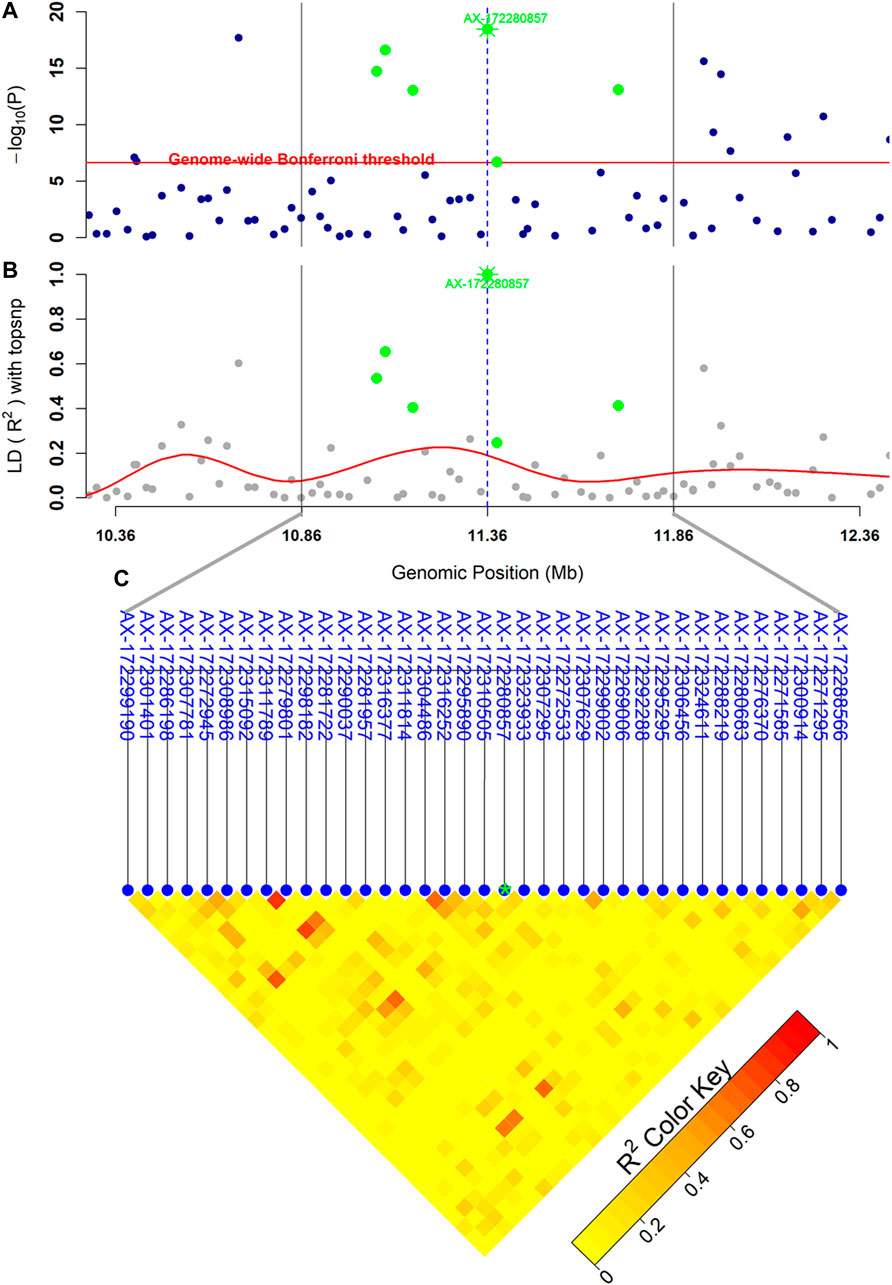
FIGURE 3. High-resolution depiction of the QTL region at LG12 with linkage disequilibrium information. (A) displays the distribution of
SNP variance: The proportion of genetic variance explained by the highest significant SNP using the direct method was ∼26.0% (Table 3). The application of the highest significant SNP as an additional fixed effect in the GWAS model caused reduction of genetic variance from 0.06 to 0.04 (Supplementary Table S1.1). Hence, the proportion of genetic variance explained by the highest significant SNP using the indirect method was 33.33%, which is a proportional reduction in the genetic variance with the application of the highest significant SNP as an additional fixed effect.
Accuracy of Predictions
Accuracies of prediction using pedigree vs. genomic information are plotted in Figure 4. Regardless of validation schemes applied, the mean accuracies obtained using genomic information were significantly higher than the pedigree information-based accuracies (Figure 4 and Supplementary Table S1.2). The mean accuracy achieved from the genomic models (GBLUP, BayesB, BayesC, and Bayesian Lasso) was 0.71, while the mean accuracy from the pedigree-based PBLUP model was 0.55 (Figure 4 and Supplementary Table S1.2). The mean accuracy of 0.70 and 0.72 was achieved with the genomic models using validation scheme “a” (within family masking) and validation scheme “b” (random masking), respectively. A similar trend was also seen using the PBLUP model with higher mean accuracy obtained with validation scheme “b” (
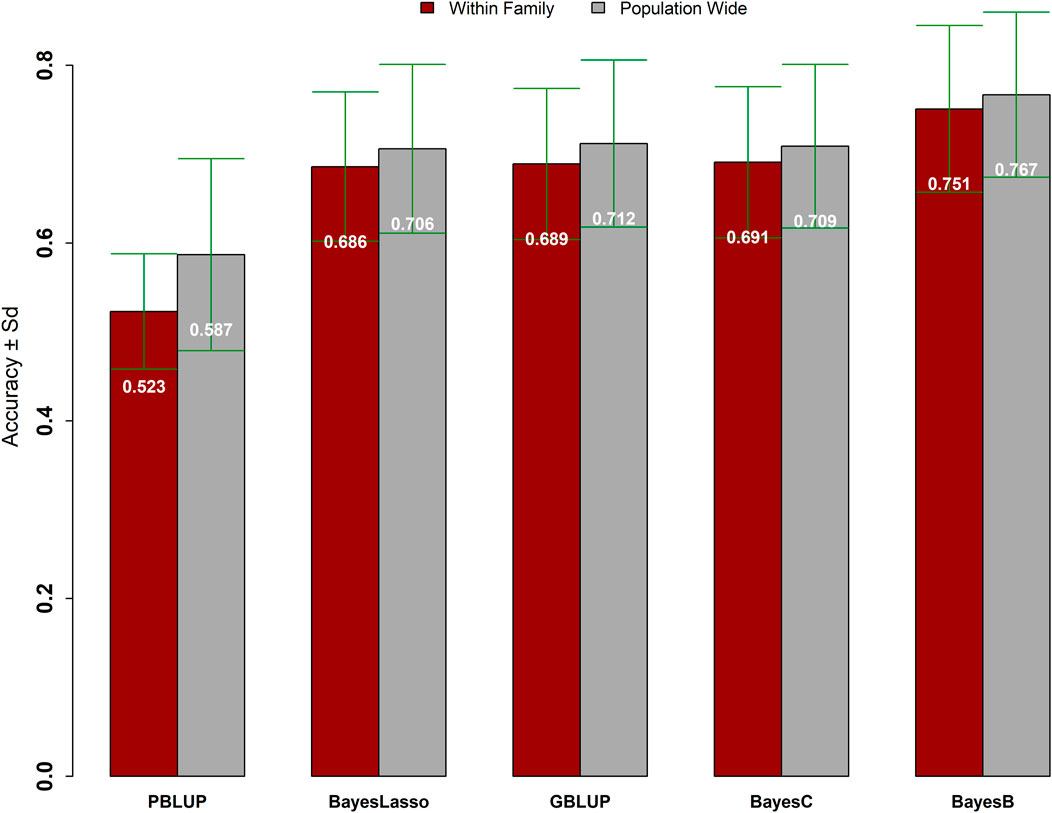
FIGURE 4. The accuracies of prediction for resistance against VNN using pedigree vs. genomic models.
The comparison among the genomic models with respect to the prediction accuracy for survival against VNN showed that the Bayesian models worked either better or equally well as GBLUP. The trend in the rank on performance of genomic models was the same within both validation schemes (“a” and “b”). The BayesB model displayed the highest accuracy with estimates of 0.77 ± 0.09 and 0.75 ± 0.09 for validation schemes “b” and “a”, respectively. The other genomic models (BayesC, Bayesian Lasso, and GBLUP) showed approximately similar accuracy of prediction with fractional differences with values 0.69 ± 0.09 and 0.71 ± 0.09 for validation schemes “a” and “b”, respectively (Figure 4 and Supplementary Table S1.2).
The comparison of the prediction accuracy for PBLUP vs. HBLUP models showed higher accuracies obtained with the HBLUP model, with estimates of 0.57 ± 0.05 and 0.58 ± 0.05 for validation schemes “a” and “b”, respectively (Supplementary Figure S1.6). The prediction accuracy for the PBLUP model was 0.52 ± 0.05 and 0.53 ± 0.05 with validation schemes “a” and “b”, respectively.
Discussion
Viral nervous necrosis is one of the highly contagious and deadliest diseases for many aquaculture species which also pose a serious threat for European sea bass producers, with fry and juveniles being highly susceptible. The outbreak of VNN may cause up to 100% mortalities at larval and around 20% mortalities at advanced juvenile stages (Le Breton et al., 1997; Munday et al., 2002). Moreover, the surviving fish present poor growth rate and ultimately high economic losses for the producers. In the current study, we explored the genetic basis of resistance against the RGNNV using large-scale challenge experimentation, phenotype recording, and high-density genotyping using SNP markers with ultimate intentions to improve fish health and welfare, leading to sustainable production.
The challenge test results from the current study presented steady/predictable survival/mortality curves, with a peak in mortality at day 5 after infection and sharp reduction of mortalities at day 11 after infection, which were similar to results reported by Doan et al. (2017) and Faggion et al. (2021) where individuals were infected via intraperitoneal and intramuscular injections, respectively. The reported trend from the studies of Doan et al., and Faggion et al., was followed as peak mortalities at days 6 and 4 after the infection and the sharp decrease in mortalities from days 7 and 9 after infection, respectively. Contrarily, Palaiokostas et al. (2018) reported smoother mortality curves with mortality peaks at days 8 and 15 post-infection where fish were infected by the immersion model. In another study conducted by Griot et al. (2021), the immersion-based challenge test that was performed showed variable mortality/survival curves (specific to each population) with survival ranging from 38 to 79% and peak mortalities at day 10 post-infection. Hence, the behavior of the mortality curve may slightly deviate depending on experimental population and the type of the infection model used. The level of peak mortalities reaches in the first week when applying injection-based infection models and may move to the second week of infection with immersion models. The shift/delay in reaching the peak mortality from day 4 or 5 [(Faggion et al., 2021); current study] to day 10 or 15 (Palaiokostas et al., 2018; Griot et al., 2021) and the duration of challenge tests (∼40–49 (Palaiokostas et al., 2018; Griot et al., 2021) vs. 20–28 days (Faggion et al., 2021); current study) meet the expectations because intramuscular injection-based models skip mucus and skin barriers which are also a part of the defense system in case of immersion-based models that will ultimately delay the time to infection and immune response of the host.
The current study revealed moderate heritability estimates for survival to VNN (Table 2) which are concordant with those reported in the literature (Palaiokostas et al., 2018; Faggion et al., 2021; Griot et al., 2021). However, a few extreme estimates of heritability as high as 0.59 (observed scale) and 0.84 (on underlying liability scale) were also reported by Griot et al. (2021) which were computed using data recorded on four backcross families. In general, the estimates of heritability for survival against VNN are quite consistent and robust probably because the history of breeding for European sea bass is not very long, and possibly, selection-based divergence has not taken place for this trait in farmed populations.
The GWAS analysis of the current study presented a strong signal of QTL at LG12 which was very consistent across datasets coming from two different year classes, though both year classes YC2016 and YC2017 were exposed to the virus in two independent challenge tests (Supplementary Figure S1.2). Moreover, the study from Griot et al. (2021) also reported very stable QTL detected at the same region of LG12 using composite interval mapping analysis. However, Griot et al. (2021) also detected multiple other putative QTLs at LG8, LG15, and LG19 with weaker signal crossing the chromosome-wide significance threshold. The putative QTLs of LG15 and LG19 in the study by Griot et al. (2021) were also consistent with our results when GWAS analysis was performed on YC2016 only (Supplementary Figure S1.2). The inconsistency of QTLs detected at LG15 and LG19, especially with the increased power by the added dataset from YC2017 perhaps indicates false signals possibly due to pseudo-linkages among markers which turn out to be insignificant with added datasets from relatively less-related individuals of YC2017. A study from Palaiokostas et al. (2018) also reported putative QTLs (signals crossing the chromosome-wide threshold) located at LG3, LG20, and the markers from unassigned scaffolds which were not detected either in our study or the study from Griot et al. (2021). However, a single QTL in the current study vs. multiple detected QTLs in both studies by Griot et al. (2021) and Palaiokostas et al. (2018) might be due to the genetic differences in populations and/or may reflect the differences in challenge tests (immersion vs. injection models) as infecting the fish by the immersion method will involve both specific and non-specific immune responses (through skin and mucus barriers) while infection through injection (this study) will skip the skin and mucus barriers and triggers specific immune responses.
The statistics of the top ten highest significant SNPs including minor allele frequencies, position in the genome, and allele substitution effects are detailed in Table 3. All the top 10 SNPs present low minor allele frequencies ranging from 0.078 to 0.265 with a similar magnitude of the allele substitution effect (ranging from 0.203 to 0.325) along with the same direction with minor alleles being favorable with the positive effect on survival (phenotype coded as 1 = survival and 0 = dead). These observations perhaps indicate that these top significant SNPs are in linkage disequilibrium (LD) and possibly are in the same phase with the causative mutation. The distribution of the survival percentage across the genotypes from the highest significant SNP (SNP-ID = AX-172280857) showed ∼118% higher survival for individuals carrying favorable genotypes (CC) than homozygous unfavorable (TT) genotypes (Supplementary Figure S1.7).
The highest significant SNP in our study explained up to 33.33% (Supplementary Table S1.1) of the genetic variance detected through the analysis of combined datasets from both year classes (YC2016 and YC2017). The proportion of the genetic variance explained by the highest significant SNP computed using the direct method (Hill and Mackay, 2004) was ∼26.20%, which is slightly lower than what is obtained using the indirect method, 33.33%. The variances computed using the direct method are largely influenced by allele frequencies and computed, considering SNPs/markers are independent genomic fragments which is normally not the case. Hence, variances of significant SNPs within the QTL region should not be summed to calculate the total genetic variance due to possible LD among the SNPs. The top ten genome-wide significant SNPs from our study showed a mean LD of 0.49 (ranging from 0.19 to 0.87, in Supplementary Figure S1.8) although the markers are distributed over a large distance of more than 7 Mb (Table 3). Moreover, the application of the highest significant SNP as a fixed effect in the indirect method causes shrinkage of p-values for all the other chromosomes and/or genome-wide significant SNPs at LG12 (Supplementary Figure S1.3) which further supports the argument on the existence of single QTL rather summing the variances from all the SNPs in the QTL region. The relatively large impact of single QTL (up to 33.33% of the genetic variance) does not necessarily mean that the tagged SNP (SNP-ID = AX-172280857) is a causative mutation, but this SNP explains an important part of QTL variation, either directly or through LD with the causative mutation.
The QTLs in the study from Griot et al. (2021) explained relatively small proportion (up to 9%) of total genetic variance which is approximately four times lower than what is found in the current study. These large differences might be very likely due to the differences in computational methods used (e.g., effects using Bayesian models (Griot et al., 2021) vs. effects computed using linear mixed models). The other factors which might also contribute include specific genetic variations among populations, challenge test methods (immersion vs. IM injection in our study), and/or family structures. The infection model using the immersion method is relatively less controlled which might have been influenced by the natural history of the disease such as involvement of natural external barriers of skin and mucus, random variations on pathogen loads, nonspecific immunity response, and stress among others (Oidtmann and Sitja-Bobadilla, 2017). Moreover, Griot et al. (2021) did not observe the putative QTL of LG12 when the progeny of the VNN-susceptible parent/line was challenge tested via IP injection, and the most of the detected QTLs were population-specific, suggesting the influence of challenge test methods and/or level of population differences. The consistency of detected QTLs of LG12 across different year classes of our study and the concordance with the results from the study by Griot et al. (2021) possibly validate the large effect of the QTL region on survival against VNN. Moreover, it also strengthens the argument that the effect of identified genomic regions at LG12 perhaps covers variation due to the specific immune-based defense mechanism of the host.
Genes underlying the QTL region i.e., the 50 Kb region covering ±25 Kb from the highest significant SNP (AX-172280857) position was searched using the European sea bass genome (seabass_V1.0, Tine et al., 2014). The QTL region contained seven genes including ITPK1, PLK4, HSPA4L, REEP1, CHMP2, MRPL35, and SCUBE1 (Supplementary Material S1, Supplementary Table S1.3, Supplementary Figure S1.9). The upstream genes to the highest significant SNP were ITPK1 (inositol tetrakisphosphate 1-kinase 1) and polo-like kinase 4 (PLK4). The ITPK1 gene is known to be engaged with cell physiological functions involving signaling molecules inositol phosphates (Desfougères et al., 2019), and PLK4 (polo-like kinase 4) has a role in cell replication by centriole duplication (Garvey et al., 2021). The highest significant SNP was annotated as the intronic SNP, that is, located within the intron of the HSPA4L (heat shock protein family A, member four like) gene which belongs to a family of heat shock proteins (HSPs), HSP70 and plays a role in response to harmful circumstances and protect the cell from stress (Liu et al., 2019). Moreover, HSPs from the family of HSP70 have also shown association nervous necrosis virus infection in Asian sea bass (Liu et al., 2016) and with immune system maintenance in humans (Chiricosta et al., 2020). The immediate next downstream gene after HSPA4L is REEP1 (receptor expression-enhancing protein 1) which is found in nerve cells (neurons), the brain, and the spinal cord (Hurt et al., 2014). The other downstream genes from the position of the highest significant SNP were CHMP2 (charged multivesicular body protein 2) and MRPL35 (mitochondrial ribosomal protein L35), which are known to be involved in the physiological function in cells while SCUBE1 (signal peptide-CUB-EGF (epidermal growth factor) domain-containing protein 1) seems to be localized in the endothelium with developmental functions.
Out of these seven genes, HSPA4L and REEP1 appear to have more relevant functions which might be playing a role for variation in survival against the RGNNV. The involvement of HSPA4L with managing stress and cell protection may assist individuals by coping with the pathological condition when the fish is infected with virus. The function of the REEP1 gene is very relevant to the disease as infected individuals present neurological symptoms with clinical signs characterized as rapid swimming, spiraling, whirling, and lying down at the bottom (Yoshikoshi and Inoue, 1990). The autopsy observations of the VNN disease include hemorrhages in the brain tissues and vacuolization in cells of the spinal cord, brain, and retina along with the high level of necrosis in the nervous cells (Munday et al., 2002; Yang et al., 2021). The findings seem to suggest that the RGNNV mainly targets the nerve cells although lesions can also be detected in the liver and spleen tissues (Yang et al., 2021). The mutations in regulatory regions of a gene may cause its under or overexpression and ultimately deviates in the availability of receptor expression-enhancing protein in the cells. The changes in the availability of protein (abundance or shortage than optimal level) under RGNNV infection might be playing a role for variation in survival against the virus possibly by coping or managing disease symptoms from the hosts. Further studies involving identification/validation of the causative mutation/gene through functional level assays (proteomics, gene editing etc.) would likely assist in unveiling the biological mechanism behind the host resistance against the RGNNV. The selection of individuals based on the actual causative mutation is highly likely to make a faster genetic progress in the desired direction due to increased accuracy of selection compared to when the selection is performed based on markers in LD with the causative mutation.
The accuracy of prediction using validation schemes, random vs. within-family masking did not show significant difference, which may be the result of genetic links across the population as accuracy of predictions using the GS is known to be sensitive to close the genetic relationship between training and candidate populations. Regardless of validation schemes, the average prediction accuracy for survival against VNN using pedigree information was 0.56 while the average accuracy using genomic information was 0.71, which is 29% higher than the pedigree information-based accuracy (Supplementary Table S1.2). The highest gain in accuracy using genomic over pedigree information was 44% which was obtained using the BayesB model (Supplementary Table S1.2). The use of genomic information for survival against VNN clearly outperformed the use of pedigree information-based predictions with a clear advantage. The advantage of genomic information over pedigree mainly contributed through the realized genomic-based relatedness among individuals which deviates from the pedigree information-based relationship coefficients. The realized relationships derived through variations contributed by mutations/variants at the QTL regions possibly become much more important when the QTL(s) have a relatively large effect on the trait. The effect of genomic relationship matrices designed using QTL-linked vs. -unlinked markers on prediction accuracy was recently tested which revealed three times increase in the prediction accuracy with QTL-linked markers compared to when the genomic relationship matrix designed using only unlinked markers (Ling et al., 2021) which highlights the importance of variants within the QTL region. The difference of the prediction accuracy between genomic vs. pedigree is likely to shrink with the availability of deep phenotypes and pedigree information with minimal pedigree errors. The availability of deep pedigree is not possible for the recent commercial populations in breeding, and hence, genomic information can play a very important role to improve traits effectively and efficiently in the desired direction.
The comparison for the accuracy of predictions within genomic models showed that Bayesian models worked better or equally well as GBLUP (Figure 4, Supplementary Table S1.2). The BayesB model outperformed all other genomic models with the highest mean accuracy of 0.76 across the validation schemes (Supplementary Table S1.2). The top ranked BayesB model was followed by BayesC, GBLUP, and lastly Bayesian Lasso with a mean accuracy of ∼0.70 across validation schemes. Hence, the genomic model BayesB showed ∼7% increase in the accuracy compared to other genomic models. The performance of genomic models is known to be affected by the genetic architecture of trait(s), and the Bayesian models are expected to perform better if the trait(s) are affected by a few QTLs (Daetwyler et al., 2012; Van Den Berg et al., 2015; Vallejo et al., 2017). Recently, Aslam et al. (2021) evaluated the accuracy of prediction for survival against the RGNNV using QTL markers (i.e., marker assisted selection, MAS) with the prediction accuracy obtained with the genomic and the pedigree-based models which showed that the gain in the accuracy of prediction using marker-assisted selection was ∼37% higher than pedigree-based selection but fractionally lower than the BayesB model (0.692 with MAS vs. 0.752 with BayesB). The GWAS analysis of our study detected a single genome-wide significant QTL with a large effect and hence validates the expectation on the accuracy of prediction as well supports that the survival against VNN is perhaps affected by a few QTLs.
The presence of a relatively simpler genetic architecture for survival against VNN with a single genome-wide detected QTL explaining a large proportion (though a large part is still missing) of the total genetic variance along with the obtained highest accuracy of prediction using BayesB is very convincing and complimenting results which present a strong potential for the application of efficient and economical marker-assisted selection. Increasing the resolution of a QTL region using more variants (SNPs, deletions, copy number variations, etc.) along with a high-quality phenotype might assist to uncover the missing genetic variance and detect the actual causative mutation affecting the trait.
Conclusion
The survival against the VNN disease showed low to moderate levels of genetic variation with a possibility of improvement in traits through selective breeding. The genetic architecture for host resistance to the RGNNV appears to be affected by a locus with a large effect and perhaps smaller contribution from the other loci. Multiple genes were identified within the QTL region with the REEP1 gene located immediately at the upstream of the highest significant SNP which seems to be more pronounced with functions involving the nervous system. The detected QTL explained ∼33% of genetic variance suggesting a strong potential of marker(s)-based efficient and economical selection for improving survival against VNN. The comparative results on the accuracy of predicting breeding values with genomic information were substantially higher (20–44%) than predictions using pedigree information which suggests a strong advantage of using genomics over pedigree-based selection to genetically improve host resistance against the RGNNV.
Data Availability Statement
The datasets presented in this study can be found in online repositories. The names of the repository/repositories and accession number(s) can be found below: European Variation Archive, accession number PRJEB50333.
Ethics Statement
The animal study was reviewed and approved by the Departmental Animal Care Committee following the three “Rs” principle, in accordance with Greek (PD 56/2013) and EU (directive 2010/63/EE, decision number “4053/14-3-2017”) legislation on the care and use of experimental animals. The protocol for experimental procedures has been approved by the decision number “5379/4-4-2017” of the competent regional veterinary authorities.
Author Contributions
The analysis of data and drafting of the manuscript was jointly performed by SV-A, IT, and MA. The other coauthors VB, KP, AD, PL, BG, and CT were involved in generation of the idea, the design of the study, and actively contributed to discussions. VB, KP, AD, EK, and PL assisted in arranging the population material, sampling, phenotype recording, and coordination at the testing facility. All authors read the manuscript, gave suggestions and comments for the improvement, and approved the final manuscript.
Funding
This work received funding from the framework of the 3rd Joint Transnational Call of the ERA-Net COFASP (Cooperation in Fisheries, Aquaculture and Seafood Processing) in collaboration with ERA-NET Marine Biotechnology under grant agreement n° 6153 (ROBUSTBASS; https://robustbass.hcmr.gr/). The funding body played no role in the design of the study and collection, analysis and interpretation of data, and in writing the manuscript.
Conflict of Interest
SV-A and IT were employed with Benchmark Genetics Norway AS. KP, AD, EK PL were employees at the company Nireus Aquaculture SA.
The remaining authors declare that the research was conducted in the absence of any commercial or financial relationships that could be construed as a potential conflict of interest.
Publisher’s Note
All claims expressed in this article are solely those of the authors and do not necessarily represent those of their affiliated organizations, or those of the publisher, the editors, and the reviewers. Any product that may be evaluated in this article, or claim that may be made by its manufacturer, is not guaranteed or endorsed by the publisher.
Acknowledgments
The authors acknowledge the 3rd Joint Transnational Call of the ERA-Net COFASP (Cooperation in Fisheries, Aquaculture and Seafood Processing) in collaboration with the ERA-NET in Marine Biotechnology for funding the “RobustBass” project. We also thank reviewers of this manuscript for their very useful suggestions which certainly assist in improving the quality of the manuscript.
Supplementary Material
The Supplementary Material for this article can be found online at: https://www.frontiersin.org/articles/10.3389/fgene.2022.804584/full#supplementary-material
Abbreviations
GBLUP, genomic-based best linear unbiased prediction; GS, genomic selection; GWAS, genome-wide association analysis; h2, heritability; HBLUP, hybrid information-based best linear unbiased prediction; LD, linkage disequilibrium; MAF, minor allele frequency; MAS, marker-assisted selection; PBLUP, pedigree-based best linear unbiased prediction; QTL, quantitative trait loci; RGNNV, red-spotted grouper nervous necrosis virus; SNPs, single nucleotide polymorphisms; VER, viral encephalopathy and retinopathy; VNN, viral nervous necrosis; YC, year class.
References
Aslam, M. L., Boison, S. A., Lillehammer, M., Norris, A., and Gjerde, B. (2020a). Genome-wide Association Mapping and Accuracy of Predictions for Amoebic Gill Disease in Atlantic salmon (Salmo salar). Sci. Rep. 10, 6435. doi:10.1038/s41598-020-63423-8
Aslam, M. L., Carraro, R., Bestin, A., Cariou, S., Sonesson, A. K., Bruant, J.-S., et al. (2018). Genetics of Resistance to Photobacteriosis in Gilthead Sea Bream (Sparus aurata) Using 2b-RAD Sequencing. BMC Genet. 19, 43. doi:10.1186/s12863-018-0631-x
Aslam, M. L., Carraro, R., Sonesson, A. K., Meuwissen, T., Tsigenopoulos, C. S., Rigos, G., et al. (2020b). Genetic Variation, GWAS and Accuracy of Prediction for Host Resistance to Sparicotyle Chrysophrii in Farmed Gilthead Sea Bream (Sparus aurata). Front. Genet. 11, 1–14. doi:10.3389/fgene.2020.594770
Aslam, M. L., Vela-Avitúa, S., Bakopoulos, V., Papanna, K., Kottaras, L., Dimitroglou, A., et al. (2021). “Marker Assisted Selection for Resistance against Viral Nervous Necrosis in European Seabass (Dicentrarchus labrax),” in Aquaculture Europe 2021. Aquaculture Europe: Maderia, Portugal.
Bakopoulos, V., Palaiologos, A., Kantham, P., Kottaras, L., Kyvelou, V., Dimitroglou, A., et al. (2018). “Selective Breeding and Disease Resistance of Sea Bass, Dicentrarchus labrax, against Viral Nervous Necrosis,” in 3rd International Congress on Applied Ichthyology & Aquatic Environment, Hydromedit, Volos, Greece, 8–11 November 2018.
Baranski, M., Moen, T., and Våge, D. I. (2010). Mapping of Quantitative Trait Loci for Flesh Colour and Growth Traits in Atlantic salmon (Salmo salar). Genet. Sel Evol. 42, 17. doi:10.1186/1297-9686-42-17
Barson, N. J., Aykanat, T., Hindar, K., Baranski, M., Bolstad, G. H., Fiske, P., et al. (2015). Sex-dependent Dominance at a Single Locus Maintains Variation in Age at Maturity in salmon. Nature 528, 405–408. doi:10.1038/nature16062
Boison, S., Ding, J., Leder, E., Gjerde, B., Bergtun, P. H., Norris, A., et al. (2019). QTLs Associated with Resistance to Cardiomyopathy Syndrome in Atlantic Salmon. J. Hered. 110, 727–737. doi:10.1093/jhered/esz042
Chatziplis, D., Batargias, C., Tsigenopoulos, C. S., Magoulas, A., Kollias, S., Kotoulas, G., et al. (2007). Mapping Quantitative Trait Loci in European Sea Bass (Dicentrarchus labrax): The BASSMAP Pilot Study. Aquaculture 272, S172–S182. doi:10.1016/j.aquaculture.2007.08.022
Chatziplis, D., Oikonomou, S., Loukovitis, D., Tsiokos, D., Samaras, A., Dimitroglou, A., et al. (2020). QTL for Stress and Disease Resistance in European Sea Bass, Dicentrarhus labrax L. Animals 10, 1668. doi:10.3390/ani10091668
Chiricosta, L., Gugliandolo, A., Bramanti, P., and Mazzon, E. (2020). Could the Heat Shock Proteins 70 Family Members Exacerbate the Immune Response in Multiple Sclerosis? an In Silico Study. Genes 11, 615. doi:10.3390/genes11060615
Correa, K., Lhorente, J. P., Bassini, L., López, M. E., Di Genova, A., Maass, A., et al. (2017). Genome Wide Association Study for Resistance to Caligus Rogercresseyi in Atlantic salmon ( Salmo salar L.) Using a 50K SNP Genotyping Array. Aquaculture 472, 61–65. doi:10.1016/j.aquaculture.2016.04.008
Costa, J. Z., and Thompson, K. D. (2016). Understanding the Interaction between Betanodavirus and its Host for the Development of Prophylactic Measures for Viral Encephalopathy and Retinopathy. Fish Shellfish Immunol. 53, 35–49. doi:10.1016/j.fsi.2016.03.033
Daetwyler, H. D., Swan, A. A., Van Der Werf, J. H., and Hayes, B. J. (2012). Accuracy of Pedigree and Genomic Predictions of Carcass and Novel Meat Quality Traits in Multi-Breed Sheep Data Assessed by Cross-Validation. Genet. Sel Evol. 44, 33. doi:10.1186/1297-9686-44-33
Dekkers, J. C. M. (2007). Prediction of Response to Marker-Assisted and Genomic Selection Using Selection index Theory. J. Anim. Breed. Genet. 124, 331–341. doi:10.1111/j.1439-0388.2007.00701.x
Desfougères, Y., Wilson, M. S. C., Laha, D., Miller, G. J., and Saiardi, A. (2019). ITPK1 Mediates the Lipid-independent Synthesis of Inositol Phosphates Controlled by Metabolism. Proc. Natl. Acad. Sci. USA 116, 24551–24561. doi:10.1073/pnas.1911431116
Doan, Q. K., Vandeputte, M., Chatain, B., Haffray, P., Vergnet, A., Breuil, G., et al. (2017). Genetic Variation Of Resistance To Viral Nervous Necrosis And Genetic Correlations With Production Traits In Wild Populations Of The European Sea Bass (Dicentrarchus Labrax). Aquaculture 478, 1–8.
Faggion, S., Bertotto, D., Babbucci, M., Dalla Rovere, G., Franch, R., Bovolenta, M., et al. (2021). Resistance to Viral Nervous Necrosis in European Sea Bass (Dicentrarchus labrax L.): Heritability and Relationships with Body Weight, Cortisol Concentration, and Antibody Titer. Genet. Sel Evol. 53, 32. doi:10.1186/s12711-021-00625-2
Garvey, D. R., Chhabra, G., Ndiaye, M. A., and Ahmad, N. (2021). Role of Polo-Like Kinase 4 (PLK4) in Epithelial Cancers and Recent Progress in its Small Molecule Targeting for Cancer Management. Mol. Cancer Ther. 20, 632–640. doi:10.1158/1535-7163.mct-20-0741
Gilmour, A. R., Gogel, B. J., Cullis, R. B., Welham, S. J., and Thompson, R. (2015). ASReml User Guide Release 4.1 Structural Specification. Hemel Hempstead, UK: VSN International Ltd.
Gjedrem, T., and Rye, M. (2018). Selection Response in Fish and Shellfish: a Review. Rev. Aquacult 10, 168–179. doi:10.1111/raq.12154
Gonzalez-Silvera, D., Guardiola, F. A., Espinosa, C., Chaves-Pozo, E., Esteban, M. Á., and Cuesta, A. (2019). Recombinant Nodavirus Vaccine Produced in Bacteria and Administered without Purification Elicits Humoral Immunity and Protects European Sea Bass against Infection. Fish Shellfish Immunol. 88, 458–463. doi:10.1016/j.fsi.2019.03.013
Griot, R., Allal, F., Phocas, F., Brard-Fudulea, S., Morvezen, R., Bestin, A., et al. (2021). Genome-wide Association Studies for Resistance to Viral Nervous Necrosis in Three Populations of European Sea Bass (Dicentrarchus labrax) Using a Novel 57k SNP Array DlabChip. Aquaculture 530, 735930. doi:10.1016/j.aquaculture.2020.735930
Habier, D., Fernando, R. L., Kizilkaya, K., and Garrick, D. J. (2011). Extension of the Bayesian Alphabet for Genomic Selection. BMC Bioinformatics 12, 186. doi:10.1186/1471-2105-12-186
Hazreen-Nita, M., Azila, A., Mukai, Y., Firdaus-Nawi, M., and Nur-Nazifah, M. (2019). A Review of Betanodavirus Vaccination as Preventive Strategy to Viral Nervous Necrosis (VNN) Disease in Grouper. Aquacult. Int. 27, 1565–1577. doi:10.1007/s10499-019-00410-5
Hill, W. G., and Mackay, T. F. C. (2004). D. S. Falconer and Introduction to Quantitative Genetics. Genetics 167, 1529–1536. doi:10.1093/genetics/167.4.1529
Hillestad, B., and Moghadam, H. K. (2019). Genome-Wide Association Study of Piscine Myocarditis Virus (PMCV) Resistance in Atlantic Salmon (Salmo salar). J. Hered. 110, 720–726. doi:10.1093/jhered/esz040
Houston, R. D., Gheyas, A., Hamilton, A., Guy, D. R., Tinch, A. E., Taggart, J. B., et al. (2008). Detection and Confirmation of a Major QTL Affecting Resistance to Infectious Pancreatic Necrosis (IPN) in Atlantic salmon (Salmo salar). Dev. Biol. (Basel) 132, 199–204. doi:10.1159/000317160
Hurt, C. M., Björk, S., Ho, V. K., Gilsbach, R., Hein, L., and Angelotti, T. (2014). REEP1 and REEP2 Proteins Are Preferentially Expressed in Neuronal and Neuronal-like Exocytotic Tissues. Brain Res. 1545, 12–22. doi:10.1016/j.brainres.2013.12.008
Le Breton, A., Grisez, L., Sweetman, J., and Ollevier, F. (1997). Viral Nervous Necrosis (VNN) Associated with Mass Mortalities in Cage-Reared Sea Bass,Dicentrarchus labrax (L.). J. Fish. Dis. 20, 145–151. doi:10.1046/j.1365-2761.1997.00284.x
Lee, S. H., Wray, N. R., Goddard, M. E., and Visscher, P. M. (2011). Estimating Missing Heritability for Disease from Genome-wide Association Studies. Am. J. Hum. Genet. 88, 294–305. doi:10.1016/j.ajhg.2011.02.002
Legarra, A., Aguilar, I., and Misztal, I. (2009). A Relationship Matrix Including Full Pedigree and Genomic Information. J. Dairy Sci. 92, 4656–4663. doi:10.3168/jds.2009-2061
Ling, A. S., Hay, E. H., Aggrey, S. E., and Rekaya, R. (2021). Dissection of the Impact of Prioritized QTL-Linked and -unlinked SNP Markers on the Accuracy of Genomic Selection1. BMC Genom Data 22, 26. doi:10.1186/s12863-021-00979-y
Liu, K., Hao, X., Wang, Q., Hou, J., Lai, X., Dong, Z., et al. (2019). Genome-wide Identification and Characterization of Heat Shock Protein Family 70 Provides Insight into its Divergent Functions on Immune Response and Development ofParalichthys Olivaceus. PeerJ 7, e7781. doi:10.7717/peerj.7781
Liu, P., Wang, L., Kwang, J., Yue, G. H., and Wong, S.-M. (2016). Transcriptome Analysis of Genes Responding to NNV Infection in Asian Seabass Epithelial Cells. Fish Shellfish Immunol. 54, 342–352. doi:10.1016/j.fsi.2016.04.029
Meuwissen, T. H. E., Hayes, B. J., and Goddard, M. E. (2001). Prediction of Total Genetic Value Using Genome-wide Dense Marker Maps. Genetics 157, 1819–1829. doi:10.1093/genetics/157.4.1819
Moen, T., Baranski, M., Sonesson, A. K., and Kjøglum, S. (2009). Confirmation and fine-mapping of a Major QTL for Resistance to Infectious Pancreatic Necrosis in Atlantic salmon (Salmo salar): Population-Level Associations between Markers and Trait. BMC Genomics 10, 368. doi:10.1186/1471-2164-10-368
Munday, B. L., Kwang, J., and Moody, N. (2002). Betanodavirus Infections of Teleost Fish: a Review. J. Fish. Dis. 25, 127–142. doi:10.1046/j.1365-2761.2002.00350.x
Nirea, K. G., Thorland, I., Refstie, T., Thodesen, J., Rye, M., Papharasis, L., et al. (2021). Highlights from Multigenerational Breeding Programs in the European Aquaculture Sector - Case Studies on Rainbow trout, Gilthead Seabream and European Seabass Aquaculture Europe.
Oidtmann, B., and Sitja-Bobadilla, A. (2017). Fish Diseases: Prevention and Control Strategies. Nikki Levy.
OIE (2019). “Viral encephalopathy and retinopathy,” in Manual of diagnostic tests for aquatic animals. Available at: https://www.oie.int/fileadmin/Home/eng/Health_standards/aahm/current/chapitre_viral_encephalopathy_retinopathy.pdf
Palaiokostas, C., Cariou, S., Bestin, A., Bruant, J.-S., Haffray, P., Morin, T., et al. (2018). Genome-wide Association and Genomic Prediction of Resistance to Viral Nervous Necrosis in European Sea Bass (Dicentrarchus labrax) Using RAD Sequencing. Genet. Sel Evol. 50, 30. doi:10.1186/s12711-018-0401-2
Park, T., and Casella, G. (2008). The Bayesian Lasso. J. Am. Stat. Assoc. 103, 681–686. doi:10.1198/016214508000000337
Peñaloza, C., Manousaki, T., Franch, R., Tsakogiannis, A., Sonesson, A. K., Aslam, M. L., et al. (2021). Development and Testing of a Combined Species SNP Array for the European Seabass (Dicentrarchus labrax) and Gilthead Seabream (Sparus aurata). Genomics 113, 2096–2107. doi:10.1016/j.ygeno.2021.04.038
Pérez, P., and De Los Campos, G. (2014). Genome-Wide Regression and Prediction with the BGLR Statistical Package. Genetics 198, 483–495. doi:10.1534/genetics.114.164442
Price, A. L., Patterson, N. J., Plenge, R. M., Weinblatt, M. E., Shadick, N. A., and Reich, D. (2006). Principal Components Analysis Corrects for Stratification in Genome-wide Association Studies. Nat. Genet. 38, 904–909. doi:10.1038/ng1847
Purcell, S., Neale, B., Todd-Brown, K., Thomas, L., Ferreira, M. A. R., Bender, D., et al. (2007). PLINK: A Tool Set for Whole-Genome Association and Population-Based Linkage Analyses. Am. J. Hum. Genet. 81, 559–575. doi:10.1086/519795
Stein, E. D., White, B. P., Mazor, R. D., Miller, P. E., and Pilgrim, E. M. (2013). Evaluating Ethanol-Based Sample Preservation to Facilitate Use of DNA Barcoding in Routine Freshwater Biomonitoring Programs Using Benthic Macroinvertebrates. PLoS One 8, e51273. doi:10.1371/journal.pone.0051273
Thorland, I., Kottaras, L., Refstie, T., Papanna, K., Papaharisis, L., and Rye, M. (2017). “Response to Selection for Harvest Weight in Sea Bass (Dicentrarchus labrax),” in Abstracts: Twelfth International Symposium on Genetics in Aquaculture 2015 472, 136–137. doi:10.1016/j.aquaculture.2017.03.032
Tine, M., Kuhl, H., Gagnaire, P.-A., Louro, B., Desmarais, E., Martins, R. S. T., et al. (2014). European Sea Bass Genome and its Variation Provide Insights into Adaptation to Euryhalinity and Speciation. Nat. Commun. 5, 5770. doi:10.1038/ncomms6770
Toffan, A., Pascoli, F., Pretto, T., Panzarin, V., Abbadi, M., Buratin, A., et al. (2017). Viral Nervous Necrosis in Gilthead Sea Bream (Sparus aurata) Caused by Reassortant Betanodavirus RGNNV/SJNNV: an Emerging Threat for Mediterranean Aquaculture. Sci. Rep. 7, 46755. doi:10.1038/srep46755
Tsai, H.-Y., Hamilton, A., Tinch, A. E., Guy, D. R., Bron, J. E., Taggart, J. B., et al. (2016). Genomic Prediction of Host Resistance to Sea Lice in Farmed Atlantic salmon Populations. Genet. Sel Evol. 48, 47. doi:10.1186/s12711-016-0226-9
Valero, Y., Awad, E., Buonocore, F., Arizcun, M., Esteban, M. Á., Meseguer, J., et al. (2016). An Oral Chitosan DNA Vaccine against Nodavirus Improves Transcription of Cell-Mediated Cytotoxicity and Interferon Genes in the European Sea Bass Juveniles Gut and Survival upon Infection. Dev. Comp. Immunol. 65, 64–72. doi:10.1016/j.dci.2016.06.021
Vallejo, R. L., Leeds, T. D., Gao, G., Parsons, J. E., Martin, K. E., Evenhuis, J. P., et al. (2017). Genomic Selection Models Double the Accuracy of Predicted Breeding Values for Bacterial Cold Water Disease Resistance Compared to a Traditional Pedigree-Based Model in Rainbow trout Aquaculture. Genet. Sel Evol. 49, 17. doi:10.1186/s12711-017-0293-6
Van Den Berg, S., Calus, M. P. L., Meuwissen, T. H. E., and Wientjes, Y. C. J. (2015). Across Population Genomic Prediction Scenarios in Which Bayesian Variable Selection Outperforms GBLUP. BMC Genet. 16, 146. doi:10.1186/s12863-015-0305-x
Vanraden, P. M. (2008). Efficient Methods to Compute Genomic Predictions. J. Dairy Sci. 91, 4414–4423. doi:10.3168/jds.2007-0980
Vendramin, N., Zrncic, S., Padrós, F., Oraic, D., Le Breton, A., Zarza, C., et al. (2016). Fish Health in Mediterranean Aquaculture, Past Mistakes and Future Challenges. Bull. Eur. Ass. Fish. Pathol. 36.
Yang, J., Lee, S. H., Goddard, M. E., and Visscher, P. M. (2011a). GCTA: A Tool for Genome-wide Complex Trait Analysis. Am. J. Hum. Genet. 88, 76–82. doi:10.1016/j.ajhg.2010.11.011
Yang, J., Weedon, M. N., Weedon, M. N., Purcell, S., Lettre, G., Estrada, K., et al. (2011b). Genomic Inflation Factors under Polygenic Inheritance. Eur. J. Hum. Genet. 19, 807–812. doi:10.1038/ejhg.2011.39
Yang, J., Zaitlen, N. A., Goddard, M. E., Visscher, P. M., and Price, A. L. (2014). Advantages and Pitfalls in the Application of Mixed-Model Association Methods. Nat. Genet. 46, 100–106. doi:10.1038/ng.2876
Yang, Z., Yue, G. H., and Wong, S.-M. (2021). VNN Disease and Status of Breeding for Resistance to NNV in Aquaculture. Singapore: Aquaculture and Fisheries.
Keywords: nervous necrosis virus, viral nervous necrosis, VNN, single nucleotide polymorphisms, genomic prediction, quantitative trait loci, genome-wide association analysis, heritability
Citation: Vela-Avitúa S, Thorland I, Bakopoulos V, Papanna K, Dimitroglou A, Kottaras E, Leonidas P, Guinand B, Tsigenopoulos CS and Aslam ML (2022) Genetic Basis for Resistance Against Viral Nervous Necrosis: GWAS and Potential of Genomic Prediction Explored in Farmed European Sea Bass (Dicentrarchus labrax). Front. Genet. 13:804584. doi: 10.3389/fgene.2022.804584
Received: 29 October 2021; Accepted: 22 February 2022;
Published: 25 March 2022.
Edited by:
José Manuel Yáñez, University of Chile, ChileReviewed by:
Antti Kause, Natural Resources Institute Finland (Luke), FinlandYniv Palti, Cool and Cold Water Aquaculture Research (USDA-ARS), United States
Copyright © 2022 Vela-Avitúa, Thorland, Bakopoulos, Papanna, Dimitroglou, Kottaras, Leonidas, Guinand, Tsigenopoulos and Aslam. This is an open-access article distributed under the terms of the Creative Commons Attribution License (CC BY). The use, distribution or reproduction in other forums is permitted, provided the original author(s) and the copyright owner(s) are credited and that the original publication in this journal is cited, in accordance with accepted academic practice. No use, distribution or reproduction is permitted which does not comply with these terms.
*Correspondence: Muhammad L. Aslam, bHVxbWFuLmFzbGFtQG5vZmltYS5ubw==