- 1University of Montreal Hospital Research Centre (CRCHUM), Montreal, QC, Canada
- 2Faculty of Medicine, University of Kikwit, Kikwit, Democratic Republic of the Congo
- 3Department of Social and Preventive Medicine, University of Montreal Public Health School (ESPUM), Montreal, QC, Canada
Pleiotropy assessment is critical for the validity of Mendelian randomization (MR) analyses, and its management remains a challenging task for researchers. This review examines how the authors of MR studies address bias due to pleiotropy in practice. We reviewed Pubmed, Medline, Embase and Web of Science for MR studies published before 21 May 2020 that used at least one single-nucleotide polymorphism (SNP) in the fat mass and obesity-associated (FTO) gene as instrumental variable (IV) for body mass index, irrespective of the outcome. We reviewed: 1) the approaches used to prevent pleiotropy, 2) the methods cited to detect or control the independence or the exclusion restriction assumption highlighting whether pleiotropy assessment was explicitly stated to justify the use of these methods, and 3) the discussion of findings related to pleiotropy. We included 128 studies, of which thirty-three reported one approach to prevent pleiotropy, such as the use of multiple (independent) SNPs combined in a genetic risk score as IVs. One hundred and twenty studies cited at least one method to detect or account for pleiotropy, including robust and other IV estimation methods (n = 70), methods for detection of heterogeneity between estimated causal effects across IVs (n = 72), methods to detect or account associations between IV and outcome outside thought the exposure (n = 85), and other methods (n = 5). Twenty-one studies suspected IV invalidity, of which 16 explicitly referred to pleiotropy, and six incriminating FTO SNPs. Most reviewed MR studies have cited methods to prevent or to detect or control bias due to pleiotropy. These methods are heterogeneous, their triangulation should increase the reliability of causal inference.
Introduction
Mendelian randomization (MR) is an instrumental variables (IVs) approach that exploits genetic variants (mostly single-nucleotide polymorphisms (SNPs)) as IVs of a non-genetic exposure to infer a causal relationship between this exposure and an outcome in observational studies (Lawlor et al., 2008). The validity of MR is based on three key assumptions: 1) the IV is associated with the exposure, 2) the association between the IV and the outcome is unconfounded, and 3) the IV only affects the outcome via the exposure, known as the exclusion restriction criterion (Labrecque and Swanson, 2018; Brumpton et al., 2020). Horizontal pleiotropy, the phenomenon whereby a genetic variant affects the exposure and the outcome through independent pathways and without being mediated by another (Davey Smith and Hemani, 2014; Hemani et al., 2018a; Jordan et al., 2019), is a primary cause of violation of the exclusion restriction criterion (Dixon et al., 2020). It may lead to biased causal effect estimates, reduced statistical power, and/or increased type I error (Bowden et al., 2016; Verbanck et al., 2018). We thereafter refer to “horizontal pleiotropy” as “pleiotropy” for the sake of brevity.
The increasing use of MR (Sekula et al., 2016) has prompted both subject-specific (Pingault et al., 2016; Frayling and Stoneman, 2018; Goodarzi, 2018; Lor et al., 2019; Meng et al., 2019; Guo et al., 2021) and general reviews (Bochud and Rousson, 2010; Davies et al., 2013; Boef et al., 2015) of MR studies summarizing the state of practice of MR in the last decade. These suggest that the exclusion restriction criterion is not systematically assessed or discussed. For example, in their meta-epidemiological overview on the approaches used in MR, Boef et al. (2015) noted that only 111 of 178 studies (62.4%) reported on the plausibility of the exclusion restriction criterion. However, no review thus far has focused on how authors prevent or minimize bias related to pleiotropy in MR studies. Such examination is important because while evidence suggests that pleiotropy is ubiquitous in the human genome (Boyle et al., 2017; Chesmore et al., 2018), the absence of pleiotropy in a MR study cannot be empirically proven (Glymour et al., 2012). While several approaches to detect pleiotropy and/or provide robust MR estimates have been recently proposed and compared (Bowden et al., 2015; Hartwig et al., 2017; Thompson et al., 2017; Hemani et al., 2018a; Verbanck et al., 2018; Rees et al., 2019; Burgess et al., 2020b; Zhao Q. et al., 2020; Minelli et al., 2021), their use in practice, including in sensitivity analyses and triangulation, has not been documented across studies. In light of the recent MR guidelines (Davey Smith et al., 2019; Burgess et al., 2020a) that recommend assessing the robustness of MR results, we examine how potential bias due to pleiotropy is considered in the literature. More specifically we summarize 1) the approaches used to avoid selecting pleiotropic genetic variants, 2) the methods used to detect and account for pleiotropy in the estimation of the causal effect, and 3) how researchers discuss the exclusion restriction criterion considering their assessment of pleiotropy, including the impact on the results when pleiotropy is suspected.
Reviewing the entire body of published MR studies would not be practical. Instead, we limited our investigation to MR studies that use SNPs in the fat mass and obesity-associated (FTO) gene as IV to investigate the causal effect of adiposity on diverse outcomes. We expected studies that used FTO as an IV to provide a rich discussion of pleiotropy for several reasons. First, several SNPs in FTO have large and robust associations with body mass index (BMI) (Gill et al., 2019) and thus are considered strong IVs for adiposity and commonly used in MR studies. Second, unlike IVs such as variants on the C-reactive protein gene which encodes C-reactive protein (Burgess et al., 2017; Reactive Protein Coronary Heart Disease Genetics Collaboration et al., 2011), the exact biological pathways through which FTO affect adiposity are not fully understood, which complexifies the assessment of pleiotropy. Third, some FTO SNPs are suspected of pleiotropy with reported effects on a wide array of health issues ranging from cardiometabolic outcomes to cancer or mental health (Pausova et al., 2009; Delahanty et al., 2011; Hertel et al., 2011; Kivimäki et al., 2011; Li et al., 2012; Iles et al., 2013; Liu et al., 2013; Cronin et al., 2014; Aijala et al., 2015).
Methods
Search Strategy and Inclusion/Exclusion Criteria
We searched Pubmed, Medline, Embase and Web of Science to identify articles published before 21 May 2020 that met the following three criteria: 1) the primary analysis was MR, 2) the primary exposure was adiposity assessed by BMI, and 3) the IV(s) included at least one SNP in the FTO gene. We excluded studies in which MR was a secondary analysis to ensure that the study provided a detailed assessment of MR assumptions. We placed no restrictions on the outcome of interest. The search strategy and the specific exclusion criteria are provided in Supplementary Methods S1, S2, respectively.
Data Extraction and Analysis
For each study we recorded the use of a one-sample or two-sample MR design. We considered as one-sample both 1) studies that performed MR using individual level data on the SNPs, exposure and outcome and 2) studies that used summary statistics on SNP-exposure and SNP-outcome associations from the same sample (Hemani et al., 2018a). Two-sample data were defined as the use of summary statistics on SNP-exposure and SNP-outcome associations both from two distinct samples (Hemani et al., 2018a). We documented three types of IVs including 1) single IV, 2) genetic risk scores (GRS) that aggregate several SNPs into a single variable that corresponds to a weighted or unweighted sum of risk alleles (Burgess and Thompson, 2013; Burgess et al., 2016) and 3) multiple IVs. We defined multiple IVs as the use of ≥2 SNPs as separate IVs in a single model or to the combination of estimated effects from ≥2 single SNPs into one summary causal effect using meta-analytic techniques (Burgess et al., 2016). We recorded the specific FTO SNP(s) used in each of the types of IVs described above.
We organized the data analysis around three themes that described how pleiotropy was handled in the analytical process including 1) the selection and combinations of IVs; 2) the methods used to detect and account for pleiotropy in the estimation of causal effects, and 3) the discussion of findings considering the assessment of pleiotropy. We documented the methods as they were explicitly stated in each article irrespective of their applicability or relevance. A critical appraisal of the use of some of the methods is provided in the Discussion.
Approaches to Prevent Pleiotropy (Selection and Combination of IVs)
According to MR guidelines (Burgess et al., 2020a), SNPs can either be selected from gene regions that specifically encodes the exposure (biological approach) or on the basis of their statistical association with the exposure of interest (statistical approach). SNPs known or suspected of pleiotropy may be excluded from the initial selection before performing the main analysis (Burgess et al., 2020a). We reported the use of the biological and or statistical approach to SNP selection. We also documented the use of multiple independent SNPs as IVs as a method to attenuate the effect of pleiotropy under the assumption that the pleiotropic effects of SNPs would be balanced and thus cancel each other out (Davey Smith, 2011). Finally, we recorded any other strategy explicitly presented as pertaining to the selection of IVs to minimize the presence of pleiotropy.
Approaches to Detect and/or Account for Pleiotropy in the Estimation of the Causal Effect
MR guidelines require authors to report on the methods used to evaluate MR assumptions, which includes investigating bias due to pleiotropy (Davey Smith et al., 2019; Burgess et al., 2020a). We recorded the methods used to evaluate the independence and the exclusion restriction assumptions with the exception of those used for population stratification, highlighting whether pleiotropy assessment was explicitly stated to justify the use of these methods. We organized methods into four categories including 1) robust (e.g., MR-Egger) and other IV estimation methods (e.g., multivariable MR), 2) methods to detect heterogeneity of estimated causal effects across IVs (e.g., statistical tests of heterogeneity between the estimated SNP-specific causal effects), 3) methods to detect or account for associations between the IVs and the outcome that arise through pathways outside of the exposure (e.g., mediation analysis), and 4) other methods. Robust methods provide causal effect estimates under a weaker set of assumptions than conventional methods (Burgess and Thompson, 2017; Burgess et al., 2020a). A summary description of the methods, including their main assumptions and limitations is presented in Supplementary Table S6.
Discussion of Findings Considering the Assessment of Pleiotropy
We verified whether the authors discussed the independence and the exclusion restriction assumptions, distinguishing studies that explicitly referred to the term “pleiotropy” from those that did not. When studies suspected IV invalidity, we further verified whether the authors report the impact of IV invalidity on MR results, and if any FTO SNPs were incriminated.
The data were tabulated using Microsoft Excel® 2016 and described using Stata/IC version 14.2 software (StataCorp, College Station, Texas, United States).
Results
Study Selection and Characteristics of Studies
Our search identified 2,985 publications, of which 128 articles were included upon completion of the screening process (Figure 1). The 128 articles are listed in Supplementary Table S1. Included articles were published between June 2008 and May 2020, and mostly comprised one-sample MR analyses (n = 98, 76.6%; Table 1 and Supplementary Table S3). A total of 31 FTO SNPs were selected as IVs with rs1558902 being the most frequently used (n = 64, 50%; Supplementary Table S2). While 74 studies (57.8%) used a GRS to represent the IVs (Table 1 and Supplementary Table S3), Figure 2 suggests a recent decline in the use of GRS in favor of multiple IVs.
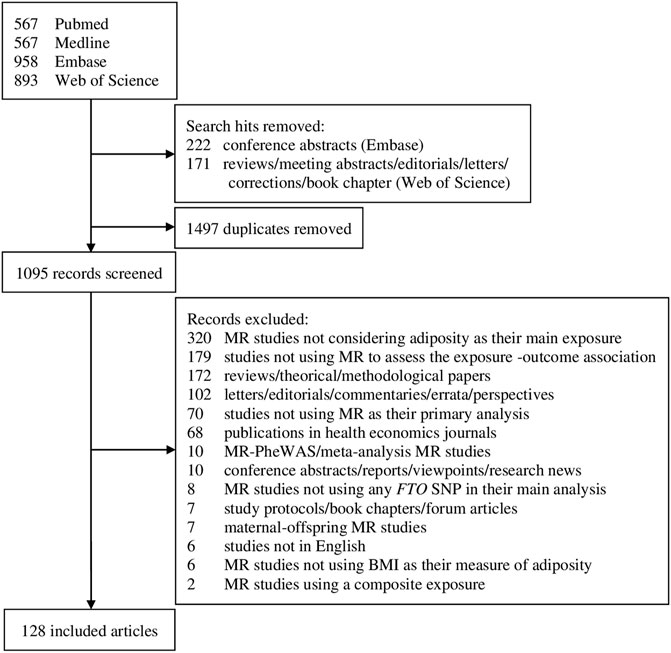
FIGURE 1. Flowchart of selection of studies includes in the review. MR, Mendelian randomization; PheWAS, phenome-wide association study; FTO, fat mass and obesity-associated; SNP, single-nucleotide polymorphism; BMI, body mass index.
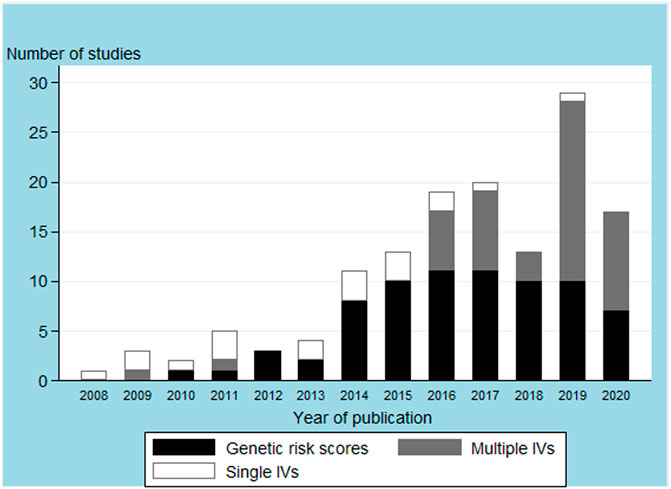
FIGURE 2. Body mass index instrumental variables (IVs) used in the main analysis by article publication year.
Approaches to Prevent Pleiotropy (Selection and Combination of IVs)
While all of the 128 studies selected IVs on the basis of statistical association between SNPs and BMI in the literature, 33 studies (25.8%) proceeded further in their attempt to prevent the effect of pleiotropy in the selection of the IVs (Table 2 and Supplementary Table S4). Of the 33 studies, 12 excluded previously selected SNPs known or suspected of pleiotropy. Ten articles respectively cited the use of a GRS and of multiple (independent) IVs (even if six of these ten in fact used a GRS as IVs) to prevent the effect of pleiotropy, a single study justified the use of a single SNP as IV as a method to prevent pleiotropy, although these strategies do not guarantee that bias due to pleiotropy is prevented or reduced (Burgess and Thompson, 2013).
Approaches to Detect and Account for Pleiotropy in the Estimation of the Causal Effect
Overall, 120 of 128 included studies assessed the plausibility of the independence and/or exclusion restriction assumptions (Table 3 and Supplementary Table S5). Of the 120 studies, 78 reported using more than one category of methods within our classification (robust and other IV estimation methods (n = 70), heterogeneity (n = 72), alternative pathways (n = 85) and others (n = 5, including the use of positive or negative control outcomes, colocalization, and verifying the concordance of MR results with those from other studies). A total of 95 studies explicitly cited pleiotropy to justify the use of such methods. MR-Egger was the most frequently reported method to assess pleiotropy (n = 68). Of the 68 studies, 66 used the intercept p-value as a test of the validity of IVs and 29 studies (Gao et al., 2016; Hartwig et al., 2016; Kemp et al., 2016; Mokry et al., 2016; Tyrrell et al., 2016; Censin et al., 2017; Dale et al., 2017; Lindstrom et al., 2017; Lyall et al., 2017; Noyce et al., 2017; Xu et al., 2017; Larsson et al., 2018; van den Broek et al., 2018; Wang T. et al., 2018; Bae and Lee, 2019; Brower et al., 2019; Censin et al., 2019; Gharahkhani et al., 2019; Howe et al., 2019; Taylor et al., 2019; Tyrrell et al., 2019; Xu et al., 2019; Dusingize et al., 2020; Kurz and Laxy, 2020; Larsson et al., 2020; Liu et al., 2020; Winter-Jensen et al., 2020; Zhang et al., 2020; Zhao Y. et al., 2020) further acknowledged that MR-Egger estimates or tests may be underpowered. Two studies (Tyrrell et al., 2016; Fan et al., 2018) compared the MR-Egger slope and the conventional MR causal effect estimate while the last study (Chen et al., 2019) did not specify how the MR-Egger results were used. Forty-six studies assessed pleiotropy by evaluating the heterogeneity of estimated causal effects across IVs, whether by graphical assessment (n = 20) or statistical testing (n = 7), or by comparing estimated causal effects from GRS or multiple IVs before vs. after exclusion of suspected pleiotropic SNP(s) (n = 20). A total of 30 studies attempted to detect pleiotropy by estimating pathways through which the IVs were associated with the outcomes outside of that implicating the exposure. Such studies mostly reported the estimation of associations between the IVs and measured risk factors of the outcome (n = 19), but also adjusted the IV-outcome or IV-confounders associations for exposure (n = 7) or documented the associations between the IV and risk factors for the outcome in the literature (n = 5).
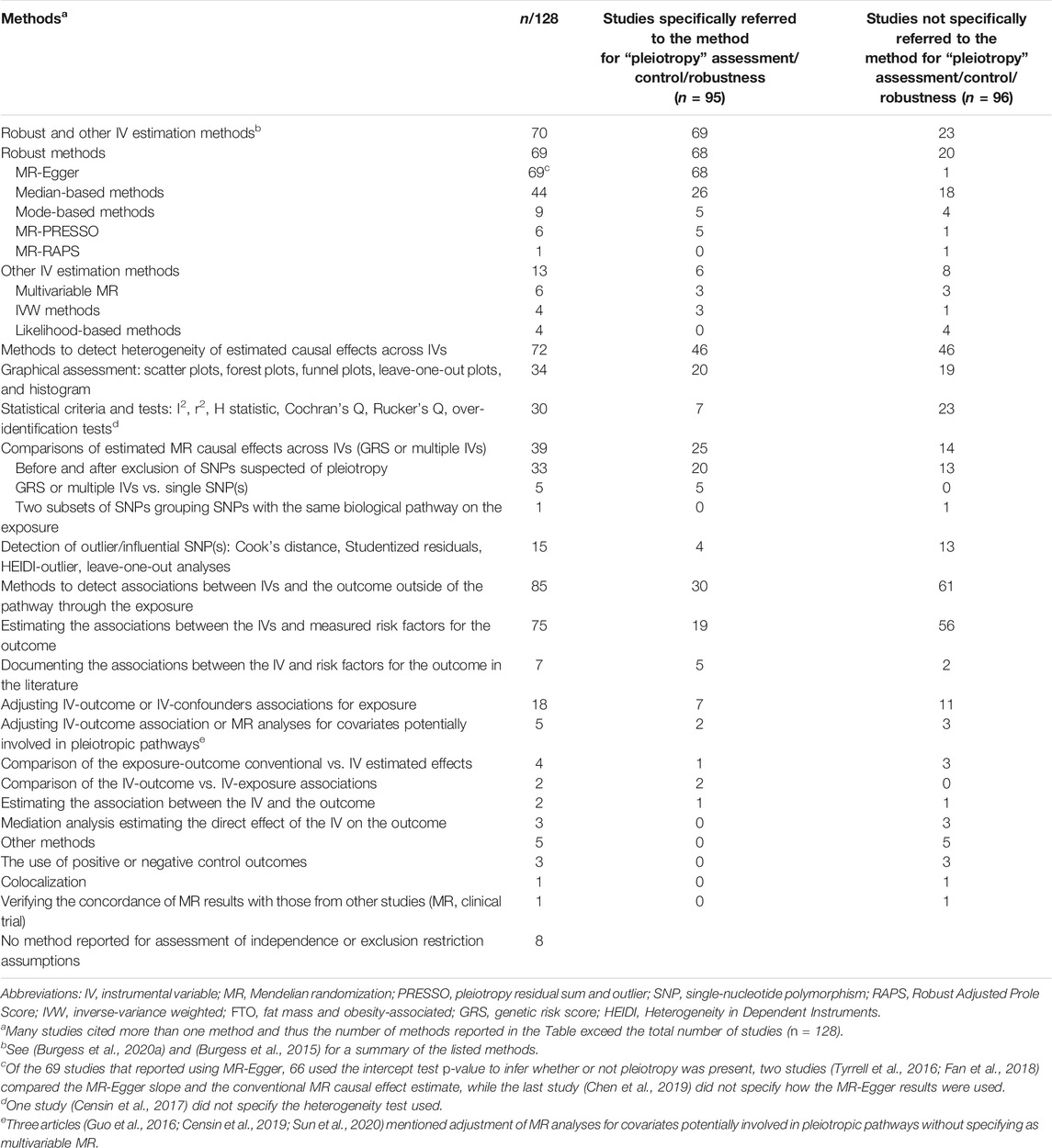
TABLE 3. Methods cited for detection or accounting the violation of the independence and/or restriction exclusion assumptions.
Discussion of Findings Considering the Assessment of Pleiotropy
Of 128 included articles, 108 discussed the plausibility of the independence and/or exclusion restriction assumptions of which 89 studies made an explicit reference to pleiotropy (Table 4 and Supplementary Table S7). Invalid IVs were suspected in 21 studies (Supplementary Table S8), 16 of which cited pleiotropy as a potential source of invalidity. Eight of the 21 studies concluded that the MR results were possibly invalid, while nine studies reported that the results were robust. The remaining four studies did not discuss the impact of IV invalidity on the results. Six studies suggested that at least one FTO SNP (rs1558902, rs1421085, and rs17817449) was suspected of pleiotropy on the basis of sensitivity analyses (Table 4, Supplementary Tables S7, S8).
Discussion
Pleiotropy is considered widespread in humans (Boyle et al., 2017) and thus presents a major challenge to the validity of MR studies, especially considering the limited knowledge of the biological function of many of the SNPs used as IVs (Danchin and Fang, 2016; Swerdlow et al., 2016). We reviewed studies that used SNP(s) in the FTO gene as IV(s) in MR to examine the strategies employed by authors to prevent, detect or control, and discuss biases due to the use of pleiotropic IVs. Our review extends the overview of statistical approaches used in MR published by Boef et al. (2015) by focusing on pleiotropy and by including the recent developments such as two-sample MR and the use of MR-Egger. We observed that the vast majority of studies addressed pleiotropy by using several methods that operate under different assumptions (Lawlor et al., 2016; Hemani et al., 2018b). While most authors invoked pleiotropy at the analytical stage to justify the use of detection tools and robust methods, explicit attention was also given to the prevention of pleiotropy in the selection of IVs in a fourth of the articles reviewed and pleiotropy was mentioned in the discussion in 70% of articles.
Our review highlighted three observations that merit attention for future MR studies. First, we documented an increasing use of multiple IVs over time, in addition to the exclusive use of statistical criteria to select IVs from the literature. This is largely explained by our focus on BMI as the exposure, since BMI is a polygenetic trait without a specific proximal coding gene from which to select SNPs as it is common with protein-like exposures (Swerdlow et al., 2016; Burgess and Thompson, 2017). The increasing use of multiple SNPs is also motivated by attempts to increase the strength of the instruments, the availability of large-scale genome-wide association studies to select instruments from, and by the development of MR methods that require multiple IVs to provide robust MR estimates under a less stringent set of assumptions (Burgess and Thompson, 2017; Burgess et al., 2020a). However, selecting SNPs exclusively by statistical approach increases the likelihood of including pleiotropic SNPs which may lead to biased MR results (Hartwig et al., 2017; Bowden and Holmes, 2019). Advantages of using multiple IVs independently in a MR study include the use of robust methods, such as MR-Egger or median or mode-based methods (Slob and Burgess, 2020). On the other hand, the use of GRS, which was the most frequent method to combine SNPs in the studies that we reviewed, is convenient because it leads to a single IV (Burgess et al., 2016). Further, weighted GRS with independently-derived weights lead to MR studies with similar statistical power than those using multiple independent IVs (Palmer et al., 2012). While several studies justified the use of a GRS as IV as a method to prevent bias due to pleiotropy, they have to rely on the restrictive assumption that the pleiotropic effects of SNPs cancel each other (Davey Smith, 2011), which is difficult to verify in practice. Using a GRS as IV further requires ensuring that each SNP in the GRS is itself a valid IV (Burgess and Thompson, 2013; Skaaby et al., 2018), which is limited by the low statistical power available for each SNP. Simulation studies have demonstrated that even including a small number of pleiotropic SNPs into a GRS can lead to biased MR estimates (Burgess and Thompson, 2013). We thus recommend that robust methods be used on the SNPs that form the GRS.
Second, our review suggests that the MR-Egger intercept test from is the most frequently reported method for pleiotropy assessment. The validity of MR-Egger estimates and the interpretation of the intercept as the average pleiotropic effect of IVs require the InSIDE (Instrument Strength Independent of Direct Effect) assumption to be satisfied (Burgess and Thompson, 2017). InSIDE is not required for the use of the p-value associated with the intercept test of the validity of IVs (Burgess and Thompson, 2017). InSIDE states that the effects of the IVs on the exposure must be uncorrelated with the direct effects of the IVs on the outcome (Bowden et al., 2015; Burgess et al., 2017), which is likely to be violated in a one-sample setting (Slob et al., 2017; Minelli et al., 2021) because parameters are estimated in the same subjects. Violations of the InSIDE assumption results in increased type I error rates (Hartwig and Davies, 2016; Burgess et al., 2017) and biased estimates in the direction of the observational associations (Minelli et al., 2021). Because testing the plausibility of InSIDE assumption is still a challenge to date (Bowden, 2017), researchers should restrict the interpretation of MR-Egger estimates in two sample settings where the lack of correlation between SNPs-exposure and SNPs-outcome associations is more plausible. Thus, when using summarized data in a one-sample setting, the MR-Egger intercept test can be used to assess the invalidity of the IVs, but other robust methods such as the median (the second robust method widely reported in this review)- and the mode-based methods should be preferred when estimating the robust causal estimates because they do not depend on the InSIDE assumption (Bowden, 2017). Further, their causal estimates are consistent in one-sample context, unlike Egger’s estimates which are biased in the direction of the observational association, as shown in simulations (Minelli et al., 2021). Burgess and Thompson offer a careful discussion of the use of MR-Egger (Burgess and Thompson, 2017), while Burgess et al. (2018) show that the statistical power for the intercept test is low in most settings.
Third, using statistical approaches to detect pleiotropic IVs is challenging because apparent manifestations of pleiotropy may be confused with other phenomena, some of which not invalidating MR or requiring different approaches than pleiotropy. For example, the assessment of heterogeneity in the estimated causal effects across different IVs is based on the principle that if IVs are valid, the variation in their corresponding MR estimates should be due to chance (Greco et al., 2015). Large variations in MR IV-specific estimates are often considered as indicative of pleiotropy, but can be due to other causes such as the non-collapsibility of odds ratios in case of MR analysis with binary outcome (Vansteelandt et al., 2011; Hemani et al., 2018a), heterogeneity in the distribution of confounders of IV-exposure or IV-outcome associations in two-sample settings (Hemani et al., 2018a; Zhao Q. et al., 2020), or differential complier causal effects, i.e., association between the IVs and the exposure that vary importantly across individuals (Baiocchia et al., 2014; Sainani, 2018). Similarly, reasons other than pleiotropy may explain non-null associations between the IVs and the outcome that may be considered indicative of pleiotropic IVs. For example, population stratification which can be addressed by restricting the sample to homogeneous ancestry or by applying correction methods (e.g., adjustment of MR models for principal components) (Davey Smith and Hemani, 2014). Additional causes of violation of the exclusion restriction that can be confused with pleiotropy have been proposed, including an exposure that varies over time, the presence of gene-environment interactions implicating IVs, and linkage disequilibrium between at least one of the IVs and a SNP that also affects the outcome (VanderWeele et al., 2014).
Our review also allows a few observations pertaining to the use of SNPs in the FTO gene as IVs for BMI. Four of the six studies that suspected that FTO SNPs used as IVs might be pleiotropic involved mental health phenotypes [e.g., subjective well-being (van den Broek et al., 2018) or phobic anxiety symptoms (Walter et al., 2015a), or common mental disorders (Kivimäki et al., 2011), including depression (Walter et al., 2015b)]. This suggests that FTO may be associated with mental health through pathways that do not involve BMI, a hypothesis that is supported by animal studies (Hess et al., 2013; Sun et al., 2019). For example, FTO regulates the activity of the dopaminergic signaling pathways related to the regulation of learning, reward behavior, motor functions, and feeding in mice (Hess et al., 2013). Furthermore, other work on FTO-deficient mice suggested that FTO could influence anxiety- and depression-like behaviours via alterations in gut microbiota (Sun et al., 2019). Caution is required regarding the use of FTO as an IV for BMI implicating mental health phenotypes.
Two limitations of the current review should be noted. First, we do not present an exhaustive list of the methods to prevent, detect or control, and discuss biases due to the use of pleiotropic IVs. Rather we focus on the methods reported in the 128 studies that we review. While we captured most of the methods that are currently available, newer methods such as the Causal Analysis Using Summary Effect estimates (CAUSE) (Morrison et al., 2020) and MR analysis using mixture-model (MRMix) (Qi and Chatterjee, 2019) are not reported. Second, the methods reported in this review include common and validated methods, as well as methods or strategies that may be less efficient/optimal for detecting or accounting bias due to pleiotropy in MR studies. Users must exert caution in selecting the best method(s) for the data at hand.
Pleiotropy is a ubiquitous phenomenon that poses a threat to the validity of MR results and that is difficult to assess. MR-related methodological development is thriving, and users are encouraged to use more than one method to assess pleiotropy, heeding the assumptions required for each.
Author Contributions
FM and M-PS designed the study. FM carried out the literature search and data extraction and analysis. All authors participated to the interpretation of the data. FM drafted the manuscript under the supervision of M-PS and TD translated it in English. M-PS and TD critically reviewed the manuscript. All authors read and approved the final manuscript.
Funding
This work was supported by a grant from the Canadian Institutes of Health Research (grant number 364471). FM was supported by a PhD scholarship from the Fonds de recherche du Québec–Santé (FRQS) (grant number 36057). M-PS was supported by a Chercheur-Boursier Junior II career award from the Fonds de recherche du Québec–Santé (FRQS) (grant number 296647).
Conflict of Interest
The authors declare that the research was conducted in the absence of any commercial or financial relationships that could be construed as a potential conflict of interest.
Publisher’s Note
All claims expressed in this article are solely those of the authors and do not necessarily represent those of their affiliated organizations, or those of the publisher, the editors and the reviewers. Any product that may be evaluated in this article, or claim that may be made by its manufacturer, is not guaranteed or endorsed by the publisher.
Supplementary Material
The Supplementary Material for this article can be found online at: https://www.frontiersin.org/articles/10.3389/fgene.2022.803238/full#supplementary-material
References
Aijala, M., Ronkainen, J., Huusko, T., Malo, E., Savolainen, E.-R., Savolainen, M. J., et al. (2015). The Fat Mass and Obesity-Associated (FTO) Gene Variant Rs9939609 Predicts Long-Term Incidence of Cardiovascular Disease and Related Death Independent of the Traditional Risk Factors. Ann. Med. 47, 655–663. doi:10.3109/07853890.2015.1091088
Bae, S. C., and Lee, Y. H. (2019). Causal Association between Body Mass index and Risk of Rheumatoid Arthritis: A Mendelian Randomization Study. Eur. J. Clin. Invest. 49, e13076. doi:10.1111/eci.13076
Baiocchi, M., Cheng, J., and Small, D. S. (2014). Instrumental Variable Methods for Causal Inference. Statist. Med. 33, 2297–2340. doi:10.1002/sim.6128
Bochud, M., and Rousson, V. (2010). Usefulness of Mendelian Randomization in Observational Epidemiology. Ijerph 7, 711–728. doi:10.3390/ijerph7030711
Boef, A. G. C., Dekkers, O. M., and le Cessie, S. (2015). Mendelian Randomization Studies: a Review of the Approaches Used and the Quality of Reporting. Int. J. Epidemiol. 44, 496–511. doi:10.1093/ije/dyv071
Bowden, J., Davey Smith, G., and Burgess, S. (2015). Mendelian Randomization with Invalid Instruments: Effect Estimation and Bias Detection through Egger Regression. Int. J. Epidemiol. 44, 512–525. doi:10.1093/ije/dyv080
Bowden, J., Del Greco , M. F., Minelli, C., Davey Smith, G., Sheehan, N. A., and Thompson, J. (2016). Assessing the Suitability of Summary data for Two-Sample Mendelian Randomization Analyses Using MR-Egger Regression: The Role of the I 2 Statistic. Int. J. Epidemiol. 45, 1961–1974. doi:10.1093/ije/dyw220
Bowden, J., and Holmes, M. V. (2019). Meta‐analysis and Mendelian Randomization: A Review. Res. Syn Meth 10, 486–496. doi:10.1002/jrsm.1346
Bowden, J. (2017). Misconceptions on the Use of MR-Egger Regression and the Evaluation of the InSIDE assumption. Int. J. Epidemiol. 46, 2097–2099. doi:10.1093/ije/dyx192
Boyle, E. A., Li, Y. I., and Pritchard, J. K. (2017). An Expanded View of Complex Traits: from Polygenic to Omnigenic. Cell 169, 1177–1186. doi:10.1016/j.cell.2017.05.038
Brower, M. A., Hai, Y., Jones, M. R., Guo, X., Chen, Y.-D. I., Rotter, J. I., et al. (2019). Bidirectional Mendelian Randomization to Explore the Causal Relationships between Body Mass index and Polycystic Ovary Syndrome. Hum. Reprod. 34, 127–136. doi:10.1093/humrep/dey343
Brumpton, B., Sanderson, E., Heilbron, K., Hartwig, F. P., Harrison, S., Vie, G. A., et al. (2020). Avoiding Dynastic, Assortative Mating, and Population Stratification Biases in Mendelian Randomization through Within-Family Analyses. Nat. Commun. 11, 3519. doi:10.1038/s41467-020-17117-4
Burgess, S., Bowden, J., Dudbridge, F., and Thompson, S. G. (2018). Assessing the Effectiveness of Robust Instrumental Variable Methods Using Multiple Candidate Instruments with Application to Mendelian Randomization. arXiv, 1606.03729v03722.
Burgess, S., Bowden, J., Fall, T., Ingelsson, E., and Thompson, S. G. (2017). Sensitivity Analyses for Robust Causal Inference from Mendelian Randomization Analyses with Multiple Genetic Variants. Epidemiology 28, 30–42. doi:10.1097/EDE.0000000000000559
Burgess, S., Davey Smith, G., Davies, N. M., Dudbridge, F., Gill, D., Glymour, M. M., et al. (2020a). Guidelines for Performing Mendelian Randomization Investigations. Wellcome Open Res. 4, 186. doi:10.12688/wellcomeopenres.15555.2
Burgess, S., Dudbridge, F., and Thompson, S. G. (2016). Combining Information on Multiple Instrumental Variables in Mendelian Randomization: Comparison of Allele Score and Summarized Data Methods. Statist. Med. 35, 1880–1906. doi:10.1002/sim.6835
Burgess, S., Foley, C. N., Allara, E., Staley, J. R., and Howson, J. M. M. (2020b). A Robust and Efficient Method for Mendelian Randomization with Hundreds of Genetic Variants. Nat. Commun. 11, 376. doi:10.1038/s41467-019-14156-4
Burgess, S., Scott, R. A., Scott, R. A., Timpson, N. J., Davey Smith, G., and Thompson, S. G.Epic- InterAct Consortium (2015). Using Published Data in Mendelian Randomization: a Blueprint for Efficient Identification of Causal Risk Factors. Eur. J. Epidemiol. 30, 543–552. doi:10.1007/s10654-015-0011-z
Burgess, S., and Thompson, S. G. (2017). Interpreting Findings from Mendelian Randomization Using the MR-Egger Method. Eur. J. Epidemiol. 32, 377–389. doi:10.1007/s10654-017-0255-x
Burgess, S., and Thompson, S. G. (2013). Use of Allele Scores as Instrumental Variables for Mendelian Randomization. Int. J. Epidemiol. 42, 1134–1144. doi:10.1093/ije/dyt093
Censin, J. C., Nowak, C., Cooper, N., Bergsten, P., Todd, J. A., and Fall, T. (2017). Childhood Adiposity and Risk of Type 1 Diabetes: A Mendelian Randomization Study. Plos Med. 14, e1002362. doi:10.1371/journal.pmed.1002362
Censin, J. C., Peters, S. A. E., Bovijn, J., Ferreira, T., Pulit, S. L., Mägi, R., et al. (2019). Causal Relationships between Obesity and the Leading Causes of Death in Women and Men. Plos Genet. 15, e1008405. doi:10.1371/journal.pgen.1008405
Chen, Y.-C., Fan, H.-Y., Yang, C., Hsieh, R.-H., Pan, W.-H., and Lee, Y. L. (2019). Assessing Causality between Childhood Adiposity and Early Puberty: A Bidirectional Mendelian Randomization and Longitudinal Study. Metabolism 100, 153961. doi:10.1016/j.metabol.2019.153961
Chesmore, K., Bartlett, J., and Williams, S. M. (2018). The Ubiquity of Pleiotropy in Human Disease. Hum. Genet. 137, 39–44. doi:10.1007/s00439-017-1854-z
Cronin, R. M., Field, J. R., Bradford, Y., Shaffer, C. M., Carroll, R. J., Mosley, J. D., et al. (2014). Phenome-wide Association Studies Demonstrating Pleiotropy of Genetic Variants within FTO with and without Adjustment for Body Mass index. Front. Genet. 5, 250. doi:10.3389/fgene.2014.00250
Dale, C. E., Fatemifar, G., Palmer, T. M., White, J., Prieto-Merino, D., Zabaneh, D., et al. (2017). Causal Associations of Adiposity and Body Fat Distribution with Coronary Heart Disease, Stroke Subtypes, and Type 2 Diabetes Mellitus. Circulation 135, 2373–2388. doi:10.1161/CIRCULATIONAHA.116.026560
Danchin, A., and Fang, G. (2016). Unknown Unknowns: Essential Genes in Quest for Function. Microb. Biotechnol. 9, 530–540. doi:10.1111/1751-7915.12384
Davey Smith, G., Davies, N. M., Dimou, N., Egger, M., Gallo, V., Golub, R., et al. (2019). STROBE-MR: Guidelines for Strengthening the Reporting of Mendelian Randomization Studies. PeerJ Preprints 7, e27857v27851. doi:10.7287/peerj.preprints.27857v1
Davey Smith, G., and Hemani, G. (2014). Mendelian Randomization: Genetic Anchors for Causal Inference in Epidemiological Studies. Hum. Mol. Genet. 23, R89–R98. doi:10.1093/hmg/ddu328
Davey Smith, G. (2011). Random Allocation in Observational Data. Epidemiology 22, 460–463. doi:10.1097/EDE.0b013e31821d0426
Davies, N. M., Smith, G. D., Windmeijer, F., and Martin, R. M. (2013). Issues in the Reporting and Conduct of Instrumental Variable Studies. Epidemiology 24, 363–369. doi:10.1097/EDE.0b013e31828abafb
Delahanty, R. J., Beeghly-Fadiel, A., Xiang, Y.-B., Long, J., Cai, Q., Wen, W., et al. (2011). Association of Obesity-Related Genetic Variants with Endometrial Cancer Risk: a Report from the Shanghai Endometrial Cancer Genetics Study. Am. J. Epidemiol. 174, 1115–1126. doi:10.1093/aje/kwr233
Dixon, P., Hollingworth, W., Harrison, S., Davies, N. M., and Davey Smith, G. (2020). Mendelian Randomization analysis of the causal effect of adiposity on hospital costs. J. Health Econ. 70, 102300. doi:10.1016/j.jhealeco.2020.102300
Dusingize, J. C., Olsen, C. M., An, J., Pandeya, N., Law, M. H., Thompson, B. S., et al. (2020). Body Mass index and Height and Risk of Cutaneous Melanoma: Mendelian Randomization Analyses. Int. J. Epidemiol. 49, 1236–1245. doi:10.1093/ije/dyaa009
Fan, H.-Y., Huang, Y.-T., Hsieh, R.-H., Chao, J. C.-J., Tung, Y.-C., Lee, Y. L., et al. (2018). Birthweight, Time-Varying Adiposity Growth and Early Menarche in Girls: A Mendelian Randomisation and Mediation Analysis. Obes. Res. Clin. Pract. 12, 445–451. doi:10.1016/j.orcp.2018.07.008
Frayling, T. M., and Stoneman, C. E. (2018). Mendelian Randomisation in Type 2 Diabetes and Coronary Artery Disease. Curr. Opin. Genet. Development 50, 111–120. doi:10.1016/j.gde.2018.05.010
Gao, C., Patel, C. J., Michailidou, K., Peters, U., Gong, J., Schildkraut, J., et al. (2016). Mendelian Randomization Study of Adiposity-Related Traits and Risk of Breast, Ovarian, Prostate, Lung and Colorectal Cancer. Int. J. Epidemiol. 45, 896–908. doi:10.1093/ije/dyw129
Gharahkhani, P., Ong, J.-S., An, J., Law, M. H., Whiteman, D. C., Neale, R. E., et al. (2019). Effect of Increased Body Mass index on Risk of Diagnosis or Death from Cancer. Br. J. Cancer 120, 565–570. doi:10.1038/s41416-019-0386-9
Gianfrancesco, M. A., Glymour, M. M., Walter, S., Rhead, B., Shao, X., Shen, L., et al. (2017). Causal Effect of Genetic Variants Associated with Body Mass Index on Multiple Sclerosis Susceptibility. Am. J. Epidemiol. 185, 162–171. doi:10.1093/aje/kww120
Gill, R., Stratigopoulos, G., Lee, J. H., and Leibel, R. L. (2019). Functional Genomic Characterization of the FTO Locus in African Americans. Physiol. Genomics 51, 517–528. doi:10.1152/physiolgenomics.00057.2019
Glymour, M. M., Tchetgen Tchetgen, E. J., and Robins, J. M. (2012). Credible Mendelian Randomization Studies: Approaches for Evaluating the Instrumental Variable Assumptions. Am. J. Epidemiol. 175, 332–339. doi:10.1093/aje/kwr323
Goodarzi, M. O. (2018). Genetics of Obesity: what Genetic Association Studies Have Taught Us about the Biology of Obesity and its Complications. Lancet Diabetes Endocrinol. 6, 223–236. doi:10.1016/S2213-8587(17)30200-0
Greco M, F. D., Minelli, C., Sheehan, N. A., and Thompson, J. R. (2015). Detecting Pleiotropy in Mendelian Randomisation Studies with Summary Data and a Continuous Outcome. Statist. Med. 34, 2926–2940. doi:10.1002/sim.6522
Guo, J.-Z., Xiao, Q., Gao, S., Li, X.-Q., Wu, Q.-J., and Gong, T.-T. (2021). Review of Mendelian Randomization Studies on Ovarian Cancer. Front. Oncol. 11, 681396. doi:10.3389/fonc.2021.681396
Guo, Y., Warren Andersen, S., Shu, X. O., Michailidou, K., Bolla, M. K., Wang, Q., et al. (2016). Genetically Predicted Body Mass Index and Breast Cancer Risk: Mendelian Randomization Analyses of Data from 145,000 Women of European Descent. Plos Med. 13, e1002105. doi:10.1371/journal.pmed.1002105
Hartwig, F. P., Bowden, J., Loret de Mola, C., Tovo-Rodrigues, L., Davey Smith, G., and Horta, B. L. (2016). Body Mass index and Psychiatric Disorders: a Mendelian Randomization Study. Sci. Rep. 6, 32730. doi:10.1038/srep32730
Hartwig, F. P., Davey Smith, G., and Bowden, J. (2017). Robust Inference in Summary Data Mendelian Randomization via the Zero Modal Pleiotropy assumption. Int. J. Epidemiol. 46, 1985–1998. doi:10.1093/ije/dyx102
Hartwig, F. P., and Davies, N. M. (2016). Why Internal Weights Should Be Avoided (Not Only) in MR-Egger Regression. Int. J. Epidemiol. 45, 1676–1678. doi:10.1093/ije/dyw240
Hemani, G., Zheng, J., Elsworth, B., Wade, K. H., Haberland, V., Baird, D., et al. (2018b). The MR-Base Platform Supports Systematic Causal Inference across the Human Phenome. ELife 7, e34408. doi:10.7554/eLife.34408.001
Hemani, G., Bowden, J., and Davey Smith, G. (2018a). Evaluating the Potential Role of Pleiotropy in Mendelian Randomization Studies. Hum. Mol. Genet. 27, R195–R208. doi:10.1093/hmg/ddy163
Hertel, J. K., Johansson, S., Sonestedt, E., Jonsson, A., Lie, R. T., Platou, C. G. P., et al. (2011). FTO, Type 2 Diabetes, and Weight Gain throughout Adult Life. Diabetes 60, 1637–1644. doi:10.2337/db10-1340
Hess, M. E., Hess, S., Meyer, K. D., Verhagen, L. A. W., Koch, L., Brönneke, H. S., et al. (2013). The Fat Mass and Obesity Associated Gene (Fto) Regulates Activity of the Dopaminergic Midbrain Circuitry. Nat. Neurosci. 16, 1042–1048. doi:10.1038/nn.3449
Howe, L. D., Kanayalal, R., Harrison, S., Beaumont, R. N., Davies, A. R., Frayling, T. M., et al. (2019). Effects of Body Mass index on Relationship Status, Social Contact and Socio-Economic Position: Mendelian Randomization and Within-Sibling Study in UK Biobank. Int. J. Epidemiol. 49, 1173–1184. doi:10.1093/ije/dyz240
Iles, M. M., Law, M. H., Stacey, S. N., Han, J., Fang, S., Pfeiffer, R., et al. (2013). A Variant in FTO Shows Association with Melanoma Risk Not Due to BMI. Nat. Genet. 45, 428–432. doi:10.1038/ng.2571
Jordan, D. M., Verbanck, M., and Do, R. (2019). HOPS: a Quantitative Score Reveals Pervasive Horizontal Pleiotropy in Human Genetic Variation Is Driven by Extreme Polygenicity of Human Traits and Diseases. Genome Biol. 20, 222. doi:10.1186/s13059-019-1844-7
Kemp, J. P., Sayers, A., Smith, G. D., Tobias, J. H., and Evans, D. M. (2016). Using Mendelian Randomization to Investigate a Possible Causal Relationship between Adiposity and Increased Bone mineral Density at Different Skeletal Sites in Children. Int. J. Epidemiol. 45, 1560–1572. doi:10.1093/ije/dyw079
Kivimäki, M., Jokela, M., Hamer, M., Geddes, J., Ebmeier, K., Kumari, M., et al. (2011). Examining Overweight and Obesity as Risk Factors for Common Mental Disorders Using Fat Mass and Obesity-Associated (FTO) Genotype-Instrumented Analysis: The Whitehall II Study, 1985-2004. Am. J. Epidemiol. 173, 421–429. doi:10.1093/aje/kwq444
Kurz, C. F., and Laxy, M. (2020). Application of Mendelian Randomization to Investigate the Association of Body Mass Index with Health Care Costs. Med. Decis. Making 40, 156–169. doi:10.1177/0272989X20905809
Labrecque, J., and Swanson, S. A. (2018). Understanding the Assumptions Underlying Instrumental Variable Analyses: a Brief Review of Falsification Strategies and Related Tools. Curr. Epidemiol. Rep. 5, 214–220. doi:10.1007/s40471-018-0152-1
Larsson, S. C., Bäck, M., Rees, J. M. B., Mason, A. M., and Burgess, S. (2020). Body Mass index and Body Composition in Relation to 14 Cardiovascular Conditions in UK Biobank: a Mendelian Randomization Study. Eur. Heart J. 41, 221–226. doi:10.1093/eurheartj/ehz388
Larsson, S. C., Burgess, S., and Michaëlsson, K. (2018). Genetic Association between Adiposity and Gout: a Mendelian Randomization Study. Rheumatology 57, 2145–2148. doi:10.1093/rheumatology/key229
Lawlor, D. A., Harbord, R. M., Sterne, J. A. C., Timpson, N., and Davey Smith, G. (2008). Mendelian Randomization: Using Genes as Instruments for Making Causal Inferences in Epidemiology. Statist. Med. 27, 1133–1163. doi:10.1002/sim.3034
Lawlor, D. A., Tilling, K., and Davey Smith, G. (2016). Triangulation in Aetiological Epidemiology. Int. J. Epidemiol. 45, dyw314–1886. doi:10.1093/ije/dyw314
Li, H., Kilpeläinen, T. O., Liu, C., Zhu, J., Liu, Y., Hu, C., et al. (2012). Association of Genetic Variation in FTO with Risk of Obesity and Type 2 Diabetes with Data from 96,551 East and South Asians. Diabetologia 55, 981–995. doi:10.1007/s00125-011-2370-7
Lindström, S., Germain, M., Germain, M., Crous-Bou, M., Smith, E. N., Morange, P.-E., et al. (2017). Assessing the Causal Relationship between Obesity and Venous Thromboembolism through a Mendelian Randomization Study. Hum. Genet. 136, 897–902. doi:10.1007/s00439-017-1811-x
Liu, C., Mou, S., and Pan, C. (2013). The FTO Gene Rs9939609 Polymorphism Predicts Risk of Cardiovascular Disease: a Systematic Review and Meta-Analysis. PLoS One 8, e71901. doi:10.1371/journal.pone.0071901
Liu, Q., Pan, J., Berzuini, C., Rutter, M. K., and Guo, H. (2020). Integrative Analysis of Mendelian Randomization and Bayesian Colocalization Highlights Four Genes with Putative BMI-Mediated Causal Pathways to Diabetes. Sci. Rep. 10, 7476. doi:10.1038/s41598-020-64493-4
Lor, G. C. Y., Risch, H. A., Fung, W. T., Au Yeung, S. L., Wong, I. O. L., Zheng, W., et al. (2019). Reporting and Guidelines for Mendelian Randomization Analysis: A Systematic Review of Oncological Studies. Cancer Epidemiol. 62, 101577. doi:10.1016/j.canep.2019.101577
Lyall, D. M., Celis-Morales, C., Ward, J., Iliodromiti, S., Anderson, J. J., Gill, J. M. R., et al. (2017). Association of Body Mass Index with Cardiometabolic Disease in the UK Biobank. JAMA Cardiol. 2, 882–889. doi:10.1001/jamacardio.2016.5804
Meng, X., Li, X., Timofeeva, M. N., He, Y., Spiliopoulou, A., Wei, W.-Q., et al. (2019). Phenome-wide Mendelian-Randomization Study of Genetically Determined Vitamin D on Multiple Health Outcomes Using the UK Biobank Study. Int. J. Epidemiol. 48, 1425–1434. doi:10.1093/ije/dyz182
Minelli, C., Del Greco M., F., van der Plaat, D. A., Bowden, J., Sheehan, N. A., and Thompson, J. (2021). The Use of Two-Sample Methods for Mendelian Randomization Analyses on Single Large Datasets. Int. J. Epidemiol. 50, 1651–1659. doi:10.1093/ije/dyab084
Mokry, L. E., Ross, S., Timpson, N. J., Sawcer, S., Davey Smith, G., and Richards, J. B. (2016). Obesity and Multiple Sclerosis: A Mendelian Randomization Study. Plos Med. 13, e1002053. doi:10.1371/journal.pmed.1002053
Morrison, J., Knoblauch, N., Marcus, J. H., Stephens, M., and He, X. (2020). Mendelian Randomization Accounting for Correlated and Uncorrelated Pleiotropic Effects Using Genome-wide Summary Statistics. Nat. Genet. 52, 740–747. doi:10.1038/s41588-020-0631-4
Noyce, A. J., Kia, D. A., Hemani, G., Nicolas, A., Price, T. R., De Pablo-Fernandez, E., et al. (2017). Estimating the Causal Influence of Body Mass index on Risk of Parkinson Disease: A Mendelian Randomisation Study. Plos Med. 14, e1002314. doi:10.1371/journal.pmed.1002314
Palmer, T. M., Lawlor, D. A., Harbord, R. M., Sheehan, N. A., Tobias, J. H., Timpson, N. J., et al. (2012). Using Multiple Genetic Variants as Instrumental Variables for Modifiable Risk Factors. Stat. Methods Med. Res. 21, 223–242. doi:10.1177/0962280210394459
Pausova, Z., Syme, C., Abrahamowicz, M., Xiao, Y., Leonard, G. T., Perron, M., et al. (2009). A Common Variant of the FTO Gene Is Associated with Not Only Increased Adiposity but Also Elevated Blood Pressure in French Canadians. Circ. Cardiovasc. Genet. 2, 260–269. doi:10.1161/CIRCGENETICS.109.857359
Pingault, J.-B., Cecil, C. A. M., Murray, J., Munafò, M. R., and Viding, E. (2016). Causal Inference in Psychopathology: A Systematic Review of Mendelian Randomisation Studies Aiming to Identify Environmental Risk Factors for Psychopathology. Psychopathology Rev. a4, 4–25. doi:10.5127/pr.038115
Qi, G., and Chatterjee, N. (2019). Mendelian Randomization Analysis Using Mixture Models for Robust and Efficient Estimation of Causal Effects. Nat. Commun. 10, 1941. doi:10.1038/s41467-019-09432-2
Reactive Protein Coronary Heart Disease Genetics Collaboration (Ccgc), C., Wensley, F., Gao, P., Burgess, S., Kaptoge, S., Di Angelantonio, E., et al. (2011). Association between C Reactive Protein and Coronary Heart Disease: Mendelian Randomisation Analysis Based on Individual Participant Data. BMJ 342, d548. doi:10.1136/bmj.d548
Rees, J. M. B., Wood, A. M., Dudbridge, F., and Burgess, S. (2019). Robust Methods in Mendelian Randomization via Penalization of Heterogeneous Causal Estimates. PLoS One 14, e0222362. doi:10.1371/journal.pone.0222362
Sainani, K. L. (2018). Instrumental Variables: Uses and Limitations. PM&R 10, 303–308. doi:10.1016/j.pmrj.2018.02.002
Sekula, P., Del Greco M, F., Pattaro, C., and Köttgen, A. (2016). Mendelian Randomization as an Approach to Assess Causality Using Observational Data. Jasn 27, 3253–3265. doi:10.1681/ASN.2016010098
Skaaby, T., Taylor, A. E., Thuesen, B. H., Jacobsen, R. K., Friedrich, N., Møllehave, L. T., et al. (2018). Estimating the Causal Effect of Body Mass index on hay Fever, Asthma and Lung Function Using Mendelian Randomization. Allergy 73, 153–164. doi:10.1111/all.13242
Slob, E. A., Groenen, P. J., Thurik, A. R., and Rietveld, C. A. (2017). A Note on the Use of Egger Regression in Mendelian Randomization Studies. Int. J. Epidemiol. 46, 2094–2097. doi:10.1093/ije/dyx191
Slob, E. A. W., and Burgess, S. (2020). A Comparison of Robust Mendelian Randomization Methods Using Summary Data. Genet. Epidemiol. 44, 313–329. doi:10.1002/gepi.22295
Sun, L., Ma, L., Zhang, H., Cao, Y., Wang, C., Hou, N., et al. (2019). Fto Deficiency Reduces Anxiety- and Depression-like Behaviors in Mice via Alterations in Gut Microbiota. Theranostics 9, 721–733. doi:10.7150/thno.31562
Sun, Y.-Q., Brumpton, B. M., Langhammer, A., Chen, Y., Kvaløy, K., and Mai, X.-M. (2020). Adiposity and Asthma in Adults: a Bidirectional Mendelian Randomisation Analysis of the HUNT Study. Thorax 75, 202–208. doi:10.1136/thoraxjnl-2019-213678
Swerdlow, D. I., Kuchenbaecker, K. B., Shah, S., Sofat, R., Holmes, M. V., White, J., et al. (2016). Selecting Instruments for Mendelian Randomization in the Wake of Genome-wide Association Studies. Int. J. Epidemiol. 45, 1600–1616. doi:10.1093/ije/dyw088
Taylor, A. E., Richmond, R. C., Palviainen, T., Loukola, A., Wootton, R. E., Kaprio, J., et al. (2019). The Effect of Body Mass index on Smoking Behaviour and Nicotine Metabolism: a Mendelian Randomization Study. Hum. Mol. Genet. 28, 1322–1330. doi:10.1093/hmg/ddy434
Thompson, J. R., Minelli, C., Bowden, J., Del Greco, F. M., Gill, D., Jones, E. M., et al. (2017). Mendelian Randomization Incorporating Uncertainty about Pleiotropy. Stat. Med. 36, 4627–4645. doi:10.1002/sim.7442
Tyrrell, J., Jones, S. E., Beaumont, R., Astley, C. M., Lovell, R., Yaghootkar, H., et al. (2016). Height, Body Mass index, and Socioeconomic Status: Mendelian Randomisation Study in UK Biobank. Bmj 352, i582. doi:10.1136/bmj.i582
Tyrrell, J., Mulugeta, A., Wood, A. R., Zhou, A., Beaumont, R. N., Tuke, M. A., et al. (2019). Using Genetics to Understand the Causal Influence of Higher BMI on Depression. Int. J. Epidemiol. 48, 834–848. doi:10.1093/ije/dyy223
van den Broek, N., Treur, J. L., Larsen, J. K., Verhagen, M., Verweij, K. J. H., and Vink, J. M. (2018). Causal Associations between Body Mass index and Mental Health: a Mendelian Randomisation Study. J. Epidemiol. Community Health 72, 708–710. doi:10.1136/jech-2017-210000
VanderWeele, T. J., Tchetgen Tchetgen, E. J., Cornelis, M., and Kraft, P. (2014). Methodological Challenges in Mendelian Randomization. Epidemiology 25, 427–435. doi:10.1097/ede.0000000000000081
Vansteelandt, S., Bowden, J., Babanezhad, M., and Goetghebeur, E. (2011). On Instrumental Variables Estimation of Causal Odds Ratios. Statist. Sci. 26, 403–422. doi:10.1214/11-STS360
Verbanck, M., Chen, C.-Y., Neale, B., and Do, R. (2018). Detection of Widespread Horizontal Pleiotropy in Causal Relationships Inferred from Mendelian Randomization between Complex Traits and Diseases. Nat. Genet. 50, 693–698. doi:10.1038/s41588-018-0099-7
Walter, S., Glymour, M. M., Koenen, K., Liang, L., Tchetgen Tchetgen, E. J., Cornelis, M., et al. (2015a). Do genetic Risk Scores for Body Mass index Predict Risk of Phobic Anxiety? Evidence for a Shared Genetic Risk Factor. Psychol. Med. 45, 181–191. doi:10.1017/S0033291714001226
Walter, S., Kubzansky, L. D., Koenen, K. C., Liang, L., Tchetgen Tchetgen, E. J., Cornelis, M. C., et al. (2015b). Revisiting Mendelian Randomization Studies of the Effect of Body Mass index on Depression. Am. J. Med. Genet. 168, 108–115. doi:10.1002/ajmg.b.32286
Wang, N., Cheng, J., Ning, Z., Chen, Y., Han, B., Li, Q., et al. (2018). Type 2 Diabetes and Adiposity Induce Different Lipid Profile Disorders: A Mendelian Randomization Analysis. J. Clin. Endocrinol. Metab. 103, 2016–2025. doi:10.1210/jc.2017-02789
Wang, T., Zhang, R., Ma, X., Wang, S., He, Z., Huang, Y., et al. (2018). Causal Association of Overall Obesity and Abdominal Obesity with Type 2 Diabetes: A Mendelian Randomization Analysis. Obesity 26, 934–942. doi:10.1002/oby.22167
Winter-Jensen, M., Afzal, S., Jess, T., Nordestgaard, B. G., and Allin, K. H. (2020). Body Mass index and Risk of Infections: a Mendelian Randomization Study of 101,447 Individuals. Eur. J. Epidemiol. 35, 347–354. doi:10.1007/s10654-020-00630-7
Xu, L., Borges, M. C., Hemani, G., and Lawlor, D. A. (2017). The Role of Glycaemic and Lipid Risk Factors in Mediating the Effect of BMI on Coronary Heart Disease: a Two-step, Two-Sample Mendelian Randomisation Study. Diabetologia 60, 2210–2220. doi:10.1007/s00125-017-4396-y
Xu, S., Gilliland, F. D., and Conti, D. V. (2019). Elucidation of Causal Direction between Asthma and Obesity: a Bi-directional Mendelian Randomization Study. Int. J. Epidemiol. 48, 899–907. doi:10.1093/ije/dyz070
Zhang, L., Tang, L., Huang, T., and Fan, D. (2020). Life Course Adiposity and Amyotrophic Lateral Sclerosis: A Mendelian Randomization Study. Ann. Neurol. 87, 434–441. doi:10.1002/ana.25671
Zhao, Q., Wang, J., Hemani, G., Bowden, J., and Small, D. S. (2020a). Statistical Inference in Two-Sample Summary-Data Mendelian Randomization Using Robust Adjusted Profile Score. Ann. Statist. 48, 1742–1769. doi:10.1214/19-AOS1866
Keywords: Mendelian randomization, instrumental variable, pleiotropy, FTO, adiposity, body mass index, genetic risk score
Citation: Mbutiwi FIN, Dessy T and Sylvestre M-P (2022) Mendelian Randomization: A Review of Methods for the Prevention, Assessment, and Discussion of Pleiotropy in Studies Using the Fat Mass and Obesity-Associated Gene as an Instrument for Adiposity. Front. Genet. 13:803238. doi: 10.3389/fgene.2022.803238
Received: 27 October 2021; Accepted: 14 January 2022;
Published: 04 February 2022.
Edited by:
Ni Zhao, Johns Hopkins University, United StatesReviewed by:
Haoyu Zhang, Harvard University, United StatesJeremy Labrecque, Erasmus Medical Center, Netherlands
Copyright © 2022 Mbutiwi, Dessy and Sylvestre. This is an open-access article distributed under the terms of the Creative Commons Attribution License (CC BY). The use, distribution or reproduction in other forums is permitted, provided the original author(s) and the copyright owner(s) are credited and that the original publication in this journal is cited, in accordance with accepted academic practice. No use, distribution or reproduction is permitted which does not comply with these terms.
*Correspondence: Marie-Pierre Sylvestre, bWFyaWUtcGllcnJlLnN5bHZlc3RyZUB1bW9udHJlYWwuY2E=