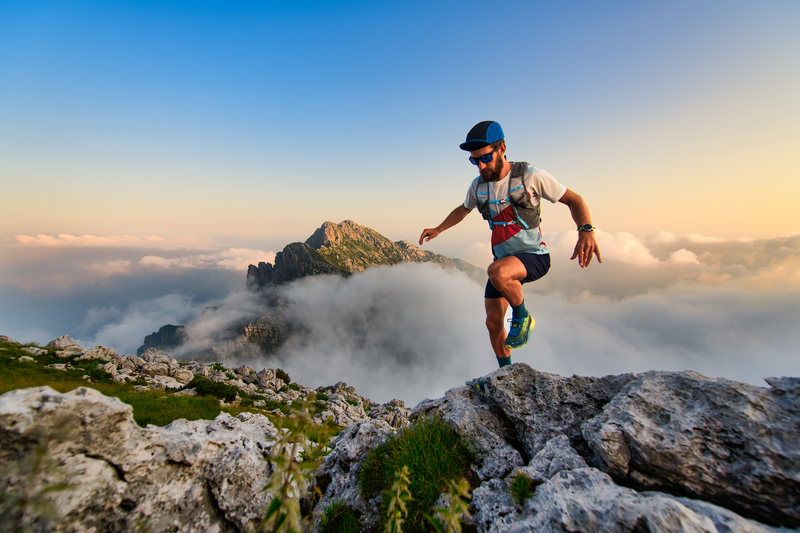
94% of researchers rate our articles as excellent or good
Learn more about the work of our research integrity team to safeguard the quality of each article we publish.
Find out more
PERSPECTIVE article
Front. Genet. , 01 March 2022
Sec. Neurogenomics
Volume 13 - 2022 | https://doi.org/10.3389/fgene.2022.781816
Parkinson’s disease is a neurodegenerative disorder with a heterogeneous genetic etiology. The advent of next-generation sequencing (NGS) technologies has aided novel gene discovery in several complex diseases, including PD. This Perspective article aimed to explore the use of NGS approaches to identify novel loci in familial PD, and to consider their current relevance. A total of 17 studies, spanning various populations (including Asian, Middle Eastern and European ancestry), were identified. All the studies used whole-exome sequencing (WES), with only one study incorporating both WES and whole-genome sequencing. It is worth noting how additional genetic analyses (including linkage analysis, haplotyping and homozygosity mapping) were incorporated to enhance the efficacy of some studies. Also, the use of consanguineous families and the specific search for de novo mutations appeared to facilitate the finding of causal mutations. Across the studies, similarities and differences in downstream analysis methods and the types of bioinformatic tools used, were observed. Although these studies serve as a practical guide for novel gene discovery in familial PD, these approaches have not significantly resolved the “missing heritability” of PD. We speculate that what is needed is the use of third-generation sequencing technologies to identify complex genomic rearrangements and new sequence variation, missed with existing methods. Additionally, the study of ancestrally diverse populations (in particular those of Black African ancestry), with the concomitant optimization and tailoring of sequencing and analytic workflows to these populations, are critical. Only then, will this pave the way for exciting new discoveries in the field.
Over the past almost 2 decades, next-generation sequencing (NGS) approaches, with their high-throughput and rapid output, have accelerated novel gene discovery for several human diseases. In this Perspective article, we summarize, analyze and highlight the studies that identified new loci for Parkinson’s disease (PD) using NGS strategies.
PD is a neurodegenerative disorder, typically presenting with bradykinesia, rigidity, resting tremor, postural instability, and various non-motor symptoms (Kalinderi et al., 2016). Approximately 90% of PD cases are considered sporadic; attributed to synergistic interactions between genetic, metabolic and environmental factors (Ball et al., 2019). The remaining 5–10% of cases are accounted for by familial PD, usually displaying a Mendelian mode of inheritance (Lesage and Brice, 2012; Hernandez et al., 2016). Positional cloning approaches have been used successfully to identify disease genes within large multi-incident PD kindreds (Hildebrandt and Omran, 1999). Linked regions of the genome that co-segregated with disease were then Sanger sequenced to identify the causal variant. PD genes identified using this approach have demonstrated autosomal dominant (AD-PD) (SNCA, LRRK2), autosomal recessive (AR-PD) (PRKN, PINK1, DJ1) and X-linked (RAB39B) inheritance patterns (Bras and Singleton., 2011; Gasser, 2013; Bandres-Ciga et al., 2020).
Later, development of high-throughput genotyping techniques allowed for the rapid screening of single-nucleotide variants (SNVs) - that occur with moderate to high allele frequencies - in large case/control cohorts (Shulskaya et al., 2018). This resulted in the rise of genome-wide association studies (GWAS), and adoption of the common-disease-common-variant hypothesis, which has been responsible for the discovery of many PD-susceptibility loci (Hemminki et al., 2008; Nalls et al., 2019). Yet, it has also been postulated that the gaping ‘missing heritability’ in complex disorders such as PD, may be attributed to larger penetrant effects of less common variants i.e., the rare-variant-common-disease hypothesis (Gasser et al., 2011; El-Fishawy, 2013; Germer et al., 2019).
NGS, in the form of whole-exome sequencing (WES), captures only the coding region; while whole-genome sequencing (WGS) sequences the entire genome including all non-coding regions (Fernandez-Marmiesse et al., 2017). When considering NGS for the study of genetic disorders, WES presents as the more suitable choice as most pathogenic mutations (80–85%), found to date, are exonic (Ku et al., 2016). WES is also cheaper, and less computationally intensive than WGS (Bonnefond et al., 2010; Chakravorty and Hegde, 2017). However, WES can result in skewed coverage due to hybridization biases and incomplete target enrichment, making detection of copy number variation (CNV) challenging (Belkadi et al., 2015). Since CNVs encompassing complete exons (in PRKN, PINK1 and DJ-1) or spanning multiple gene copies (SNCA) are a significant cause of PD, this is a notable limitation of WES in PD studies. Together, these factors indicate that WGS may be more effective for identification of novel or rare genetic variants, particularly in complex diseases like PD.
For our search, a comprehensive search string on NCBI’s PubMed Central database “((((((parkinson’s disease) AND NGS) AND familial) AND novel) AND candidate) AND gene)” was done on 13 May 2021. Abstracts were read to identify studies that specifically used NGS (either WES or WGS) approaches to identify potential novel genes in familial PD or parkinsonism. We did not exclude studies with a lack of evidence of pathogenicity, and this resulted in a total of 17 relevant studies. These studies and their approaches are summarized in Table 1 and are discussed in chronological order below.
TABLE 1. List of published studies that identified novel Parkinson’s disease loci using next-generation sequencing approaches.
In 2011, Vilariño-Güell and others published their WES findings on two first degree cousins from an AD PD-affected Swiss family, announcing the discovery of the p.Asp620Asn mutation in VPS35 (Vilariño-Güell et al., 2011). In a back-to-back publication, that same mutation in VPS35 was also identified in an Austrian family (Zimprich et al., 2011). Their study made use of haplotyping and linkage analysis in conjunction with WES, allowing for the simultaneous identification of linkage regions and the subsequent filtering of variants based on their distance to the linkage regions. Thus, postulating a time-and cost-effective approach to exome sequencing for AD-PD (Bras and Singleton, 2011; Gialluisi et al., 2020). Furthermore, the same mutation was found in six unrelated PD individuals of varying ethnicity and observed in a sporadic PD case (Zimprich et al., 2011). With these findings in several independent PD families, VPS35 is now considered a significant gene associated with AD-PD, though with still unresolved pathology. The successes observed in these two early studies sparked hope for the discovery of rare monogenic causal factors using NGS in PD families and subsequently, several similar studies ensued.
In 2012, the discovery of DNAJC6, linked to AR-juvenile parkinsonism in a consanguineous Palestinian family, was published (Edvardson et al., 2012). They performed SNP genotyping and homozygosity mapping (HM) analysis in conjunction with WES (Edvardson et al., 2012; Vahidnezhad et al., 2018). This approach potentially facilitates more rapid detection of a disease gene after WES (Kim et al., 2013). HM analysis allows for the identification of large, shared regions of homozygosity (where variants associated with AR disease genes are likely to be located) between affected family members (Wakeling et al., 2019). Therefore, HM could be beneficial for the identification of pathogenic mutations in AR-PD (Bras and Singleton, 2011). The following year, the same approach on a consanguineous Iranian family affected with early-onset PD (EO-PD) led to the discovery of a homozygous mutation in SYNJ1 (Krebs et al., 2013). Also in 2013, the finding of a heterozygous p.Ser657Asn mutation in PLXNA4 within a large German family, was published (Schulte et al., 2013).
Vilariño-Güell and others published their findings on identification of the p.Asn855Ser mutation in DNAJC13 in 2014 (Vilariño-Güell et al., 2014). WES was conducted on a large PD-affected Canadian-Mennonite family of Dutch German-Russian ancestry. The same mutation and disease-associated haplotype was found in two other families of Mennonite ancestry in the greater Canadian region (Vilariño-Güell et al., 2014). Remarkably, another group, studying the original Canadian-Mennonite family, published their findings in 2016, on a different genetic causal variant, p.Arg141Leu in TMEM230 (Deng et al., 2016). This difference in disease gene nominations in the same family may be due to differences in methodological approach, including the clinical phenotype used, genotyping approach and pathogenicity prediction scoring of mutations (Farrer, 2019). This highlights the importance of accurate clinical information, particularly in a disease like PD, where the phenotype may overlap with related neurological disorders.
Notably, in the discovery of CHCHD2 in 2015 in AD-PD, Funayama et al., performed both WES and WGS (Funayama et al., 2015). The authors state that WGS was done on one affected family member to correct for the regions that were inadequately covered during exome capture (Funayama et al., 2015). The use of WGS in combination with WES (particularly in the individual who has the variant of interest) is considered highly beneficial due to its increased coverage and enables screening for CNVs/SNVs in the regions of interest. However, WES continues to be the sequencing method of choice (and was the sole NGS approach used in 16/17 of the studies in Table 1), which could largely be attributed to the significant disparity in cost.
In 2016, Sudhaman and others nominated RIC3 (Sudhaman et al., 2016a) and PODXL (Sudhaman et al., 2016b) in South Indian and North Indian families, respectively. For RIC3, microsatellite markers were used, prior to WES, to rule out linkage to known AD-PD genes including SNCA, LRRK2 and VPS35 (Sudhaman et al., 2016a). A similar approach was used to discover PODXL. In 2017, a study using WES on a Spanish Basque family led to the discovery of CSMD1 as a potential disease-causing gene (Ruiz-Martínez et al., 2017). That same year, another study reported a homozygous loss-of-function mutation in DNAJC12, using a positional cloning approach in combination with WES (Straniero et al., 2017).
In 2018, two more novel PD genes were reported. In one study, SNP genotyping, linkage analysis, CNV analysis and WES was used in an Italian family to identify the Gly603Arg mutation in LRP10 (Quadri et al., 2018). In PD, de novo mutations may potentially account for several sporadic, EO-PD cases. In the second study, WES and subsequent analysis was performed on trios of Han Chinese ancestry with EO-PD and identified potential pathogenic de novo mutations in NUS1 (Guo et al., 2018). De novo mutations are typically rare, deleterious, and difficult to detect with traditional genotyping methods but were effectively identified using only WES in this study (Wang et al., 2019).
In 2019, the identification of UQCRC1 (a nuclear-encoded gene associated with mitochondrial metabolism) implicated in a Taiwanese PD family with parkinsonism and polyneuropathy, was published (Chen and Lin, 2020; Courtin et al., 2021). This study was the only one to make use of a comprehensive NGS gene panel to pre-screen ∼40 PD-associated genes (including SYNJ1, DNAJC13, DNAJC6, CHCHD2, VPS35) before performing WES. A study published in 2021 described the discovery of a novel PD gene (NRXN2) in a family from South Africa (Sebate et al., 2021). They analyzed WES data from 3 affected individuals from an Afrikaner family, an ethnic group consisting of Dutch, German and French ancestry that are native to South Africa. Most recently, a study examining six families from Australia used WES to narrow down two novel potential disease-causing genes in two families - SIPA1L1 and KCNJ15 (Bentley et al., 2021).
It should be noted that true monogenic PD is rare and establishing a familial PD candidate gene as pathogenic can have a degree of uncertainty due to the following factors: isolated findings in familial studies, presence of disease variants in healthy controls, erroneous gene-disease associations or possession of complex phenotypes that may skew towards other, diverse parkinsonisms (Day and Mullin., 2021). Of the candidate genes outlined in this article, VPS35, otherwise referred to as PARK 17, is firmly associated with classical PD. However, DNAJC6 (PARK 19), DNAJC13 (PARK 21), SYNJ1 (PARK 20), VPS13C (PARK 23), and CHCHD2 (PARK 22) are also considered pathogenic and viewed as rare genetic contributors to PD disease (Olgiati et al., 2016; Puschmann, 2017; Schormair et al., 2018; Correia Guedes et al., 2020; Day and Mullin., 2021; Li B et al., 2021). The remaining candidate genes require further study before being categorized as definite PD genes. “Proof of pathogenicity” of novel disease genes require that multiple mutations in the same gene co-segregate with disease in independent families, are absent in large collections of healthy controls or found to be significantly associated with sporadic PD cases (MaCarthur et al., 2014; Farrer, 2019). These criteria seem to necessitate a move away from small family studies and into population-based NGS studies for rare variant discovery - once again relying on large cohorts of individuals. This is also supported by the reasoning that many PD loci may be population-specific and therefore difficult to identify in small studies The (International Parkinson Disease Genomics Consortium, 2020). However, confirmation of these putative mutations through functional studies or by utilizing model organisms remains a challenge due to the novelty and the large number of variants being identified through NGS.
Consequently, it is clear that there is still a need for NGS studies on PD-affected families for its ability to nominate potentially pathogenic novel genes, even if not seen in other individuals, as this may provide mechanistic insight into PD pathobiology. As seen with the discovery of NUS1, where knockout RNAi experiments on Drosophila revealed PD phenotypes, lab-based functional analysis of candidate genes is useful to uncovering disease pathogenesis (Guo et al., 2018). However, many studies omit lab-based functional analysis due to the uncertainty as to whether the gene is disease-causing (Rodenburg, 2018). Alternatively, candidate genes can be further associated with a disease of interest through phenotypic associations, determining gene or protein interaction networks or establishing functional similarity with known PD genes using computational methods (Chen et al., 2021). Increasingly, a number of machine learning methods that incorporate information from known databases that provide functional annotations (e.g. Gene Ontology), tissue expression data (e.g., Human Protein Atlas) and metabolic/signaling pathways (e.g., Kyoto Encyclopaedia of Genes and Genomes) in order to determine protein or gene interactions between putative and established disease genes (Piro and Di Cunto, 2012). According to a recent study outlining a comprehensive PD gene database (GENE4PD), a functional correlation network was simulated between “high confidence” and “suggestive” PD-associated genes in PD pathways resulting in significant associations, including those seen with RIC3 and CHCHD2, with the latter significantly linked to SNCA, PINK1, LRRK2, PARK7, and VPS35 - a likely potential for expanding our knowledge on PD pathway architecture and future annotations (Li B et al., 2021). Furthermore, it is difficult to characterize a gene as being only PD-associated due to the inter-lapping of disease pathways across various parkinsonism disorders (Erratum, 2019; Li W et al., 2021).
Analysis of the tools used in the 17 studies, revealed several similarities and differences (Table 1; Figure 1).
FIGURE 1. Summary of tools used to analyze next-generation sequencing data in the 17 studies that identified novel Parkinson’s disease genes.
Burrows-Wheeler Aligner (http://bio-bwa.sourceforge.net/), specifically the BWA-MEM algorithm, was the software of choice (11/17 studies) for the alignment of the NGS reads to the human reference genome [Figure 1]. The studies reviewed here made use of both the hg18/GRCh36 and hg19/GRCh37 reference genomes. According to one study, SNV detection in WGS data resulted in enhanced genome coverage and a higher number of SNV calls when using GRCh38, as opposed to GRCh37, thereby necessitating the use of the latest reference genome available for NGS analysis (Pan et al., 2019). They conclude that the selection of the aligner in NGS is not as important as the reference genome selection (Pan et al., 2019). The UnifiedGenotyper was used for variant calling in 7 of the 9 studies using the Genome Analysis Toolkit (GATk). This was until the more recent studies, including NUS1, NRXN2 and KCNJ15, made use of GATk’s HaplotypeCaller for variant calling (Guo et al., 2018). The HaplotypeCaller is now considered best practice for variant calling through GATk’s Best Practices Workflows (https://gatk.broadinstitute.org) as it allows for SNP/inDEL detection via de novo haplotype assembly (Odumpatta & Mohanapriya. 2020). However, a combination of variant callers may be the most efficient method to prioritize variants (Kumaran et al., 2019; Zhao et al., 2020).
Annovar (https://annovar.openbioinformatics.org/) and AnnTools (http://an-ntools.sourceforge.net/) were the annotation tools used most frequently in 7/17 and 2/17 studies, respectively (Figure 1). These tools are capable of annotating variants using either gene-based, region-based or filtering-based approaches. A typical exome will produce ∼20,000 variants with ∼10% of these being novel (Belkadi et al., 2015). Thus, the variant filtering tools and exclusion/inclusion criteria must be sufficiently sensitive to identify the most likely causal factors from the ‘background noise’ (Kalinderi et al., 2016). In these PD studies, variants were searched against specific databases to determine allele frequencies. As seen in Figure 1, the three most frequently used databases are dbSNP (14/17), the 1000-Genomes-Project (11/17) and the NHLBI - Exome Sequencing Project (7/17), which are currently still considered the most widely used databases for NGS analysis. It was noted that GnomAD, the largest open-source population database, was only mentioned in 4/17 studies and highlights the need to prioritize the use of the larger databases (including the newly released UK BioBank database (https://www.ukbiobank.ac.uk/) as it may affect minor allele frequency (MAF) scores used in downstream variant filtering. Several criteria exist to prioritize possible disease-causing variants (Karczewski et al., 2020). Variants are excluded if they are synonymous as they are typically considered to be evolutionary neutral and are likely to have no functional impact on the protein. Variants are also excluded if found to appear in public databases with a MAF >0.01 indicating that the alternate allele is present in more than 1% of the population and is therefore a polymorphism. However, for inclusion, variants must possess PhRED scores >30 (indicating a base call accuracy of 99.9%), be exonic (at present, variants of interest are localized to protein-coding regions as disease-causing variants are likely to impact protein function), have either heterozygous or homozygous genotypes specific to the Mendelian inheritance pattern observed in the family, and also be validated through Sanger sequencing (Vilariño-Güell et al., 2011).
Notably, several caveats need to be considered in the case of PD. Homozygous variants may be disease-causing but may commonly appear in databases such as dbSNP and the 1,000 Genomes Project in heterozygous form, and therefore may be filtered out before variant prioritization (Bras & Singleton., 2011). Furthermore, there are instances in which not all PD affected family members carry the same pathogenic mutation and present as phenocopies (whereby two affected PD individuals with matching phenotypes in a family have different genotypes possibly due to an environmental risk factor). This phenomenon can easily be confused with intrafamilial heterogeneity (where one affected individual has a different mutation to the family mutation but where this difference may be due to de novo mutations, epigenetic changes, or pleiotropy or, in another instance, where multiple rare variants contribute to individual disease risk as seen in oligogenic inheritance (Klein et al., 2011; Farlow et al., 2016; Bentley et al., 2021). True phenocopies in a family may also lead to incorrect conclusions regarding the inheritance pattern within the family (Klein et al., 2011). These confounding factors are relevant in PD, thus requiring adaptation of inclusion criteria in bioinformatic tools going forward.
Popular tools used in these studies to predict the pathogenicity of variants included SIFT (https://sift.bii.a-star.edu.sg/) (5/17) and PolyPhen-2 (http://genetics.bwh.har-vard.edu/pph-2/) (8/15) (Flanagan et al., 2010). SIFT determines the effect of amino acid substitution on the protein function whereas PolyPhen-2 predicts the structural and functional impact non-synonymous SNPs have on the protein based on phylogenetic analysis (Odumpatta and Mohanapriya. 2020). Furthermore, many of the other pathogenicity prediction tools in Figure 1 were aimed at identifying variants with splice site effects. Subsequent performance assessment of pathogenicity assessment tools identified other options that outperform PolyPhen-2 and SIFT (Niroula and Vihinen, 2019). Recently, it has been noted that deep neural network models, in conjunction with general pathogenicity predictors such as CADD, are capable of improved variant prioritization as opposed to using the tool alone (Rentzsch et al., 2021). This may open the door to novel machine learning approaches, tailored to the disease of interest, in identifying or confirming disease-causing genes. Many of these newer tools, including RENOVO (Favalli et al., 2021) and DeepPVP (Boudellioua et al., 2019), typically make use of phenotypes to identify gene-disease associations by employing the use of publically available databases including ClinVar.
Also, there is a push to validate the functionality of these novel genes with wet-laboratory-based methods. However, the development of bioinformatic tools to aid the functional analysis of candidate variants may be useful in the interim. VS-CNV (Fortier et al., 2018), dudeML (Hill and Unckless, 2019), CNV-MEANN (Huang et al., 2021) are examples of newer computational software developed to detect and call CNVs in NGS data (including both exome and gene panel data) with CNVnator (Abyzov et al., 2011), Control-FREEC (Boeva et al., 2012) and LUMPY (Layer et al., 2014)) still widely used to replace standard multiplex ligation-dependent probe amplification (MLPA), fluorescence in situ hybridization (FISH) or microarray CNV detection (Zhang et al., 2019). In the discovery of NRXN2, computational protein modelling was performed using the Swissmodel webserver to simulate the potentially disruptive effect of the mutation on protein structure (Sebate et al., 2021).
As observed for LRRK2 p.G2019S, some PD-causing mutations may be population-specific (Correia Guedes et al., 2010). Therefore, given the significant differences in ancestral origins, it is likely that the genetic etiology of sub-Saharan populations may be different to that of European and Asian populations (Bope et al., 2019). Mutation screening of Sub-Saharan African individuals with PD has revealed a low frequency in the known PD-causing genes, thus fueling this hypothesis (Williams et al., 2018; Dekker et al., 2020). Additionally, a recent study, using commercial MLPA kits to detect CNVs in individuals with PD from South Africa and Nigeria, observed false-positive deletions due to the presence of SNPs, highlighting the need for data from diverse populations when designing genomic assays for detecting PD mutations (Müller-Nedebock et al., 2021).
The current human reference genome build (GRCh38) is derived from a small sample size, with ∼70% of the build derived from a single donor of European ancestry, thereby lacking genetic diversity and therefore inadequate in the context of genetic research in Africa (Wong et al., 2020). Attempts to bridge this fundamental gap in African genomics are currently underway. An example is the South African Human Genome Project initiative to develop a local reference genome based on 24 African ancestry individuals (https://sahgp.sanbi.ac.za/). Another initiative is the H3Africa Consortium which aims to develop a pan-African bioinformatics network (H3ABionet) and infrastructure to enhance African genomics research on the continent (Mulder et al., 2017). Additionally, South African researchers have developed a secondary data analysis pipeline to overcome the lack of African allele frequency data in population databases (Schoonen et al., 2019). Their software incorporates Ensembls Variant Effect Predictor (https://www.ensembl.org/info/docs/tools/vep) to annotate variants and GEnome MINIng (GEMINI v0.20) (https://gemini.readthedocs.io/) to effectively filter variants according to African allele frequencies, resulting in higher quality output (Schoonen et al., 2019). Furthermore, international efforts in PD are underway to bring underrepresented populations to the fore, through standardized NGS data storage and analysis, as seen with the Global Parkinson’s Genetics Program (Global Parkinson’s Genetics Program., 2021) that aims to sequence and analyze PD-affected, at-risk and control individuals from diverse populations to bridge the gap in the ‘missing heritability’ witnessed in PD.
Recently, the exponential increase in large genomic datasets has necessitated the use of cloud-based systems for the ease of storage, analysis and data-sharing (Navale and Bourne, 2018). However, cloud-based systems can be expensive and require careful consideration of the data use policies to adhere to security in the cloud. Another glaring issue in computational biology is inconsistencies regarding the reproducibility of genomic data analysis and reuseability of open-source analytic software (Russell et al., 2018). A review examining the state of Github repositories of popular bioinformatic tools found that nearly half (46%) of all public repositories had no opensource license and nearly 12% had no version control (Russell et al., 2018). They suggested that software need to be vetted for consistent maintenance by a developer team. Thus, it is important to check the credibility of analysis software before use in a research or clinical setting, and a need for journals to insist on providing datasets and code to reproduce analyses.
The initial studies that discovered VPS35, created excitement about the subsequent elucidation of the genetic etiology of PD. However, that initial hope has not been realized with most of the genes identified through NGS, only being found in a single family. This may be due to the complexity of PD etiology, with either, each family having its own rare genetic cause, or that the more common genetic causes underlying PD have not yet been identified. This leads us to question the future direction of NGS approaches in PD.
Third-Generation Sequencing or long-read sequencing are newly-developed approaches that aim to overcome the limitations of existing NGS methods. They produce long-reads that are far more expansive, reducing the complexity of detecting read overlaps—thus increasing the quality of the sequencing data and improving CNV detection (Giani et al., 2019). Approximately 15% of the genome is assumed to be inaccessible due to atypical GC content and repeat elements including trinucleotide repeat expansions which are disease-causing in several neurological disorders, including PD (Keogh and Chinnery., 2013). These mutable regions may harbor pathogenic mutations, particularly compound heterozygous mutations that may only be discovered with long-read sequencing (Mantere et al., 2019). Another limitation of short-read lengths produced by traditional NGS, is potential misalignment of GBA (a common genetic risk factor for PD) to its pseudogene which is ∼96% identical, resulting in false-positive mutations (Bras and Singleton., 2011). Furthermore, a study that explored the use of targeted-capture long-read sequencing of SNCA transcripts, detected previously-undiscovered isoforms capable of translating novel proteins (Tseng et al., 2019). Therefore, in the near future, long-read sequencing may be viewed as the more favorable sequencing alternative for disorders such as PD.
In conclusion, determining the complex genetic architecture underlying PD, particularly in underrepresented populations, is critical to provide insight into PD molecular mechanisms, detection of PD biomarkers, and elucidation of novel drug targets. Thus, this knowledge will change the course of future clinical diagnoses and therapeutic modalities for this currently incurable disorder. The aim of this article was to explore the use of NGS approaches to identify novel candidate genes in familial PD to consider not only their current relevance in research, but also their future potential in unraveling PD genetics. From our analysis, we recommend the use of third-generation sequencing technologies to identify complex genomic rearrangements and new sequence variation, in combination with current sequencing techniques, to propel future PD genetics research. Furthermore, we recommend that NGS researchers optimize and adjust their sequencing and analytic workflows according to the genetic background of their study participants with PD, and the constant evolution of bioinformatic tools. NGS approaches have revolutionized novel disease gene discovery, however, best practice guidelines need to be developed; taking into account diverse populations and ancestral origins, since it is apparent that a “one-size-fits-all” approach will have significant limitations.
The original contributions presented in the study are included in the article/Supplementary Material, further inquiries can be directed to the corresponding author.
NSP searched the literature, compiled the table and figure, and wrote the first draft of the manuscript. OAR, AC, and SB wrote sections and edited the manuscript. All authors approved the final version.
NSP is supported by South African Research Chairs Initiative of the Department of Science and Technology and National Research Foundation of South Africa (award number UID 64751); OAR is supported by grants from NIH/NINDS (U54-NS100693, UG3-NS104095, U54-NS110435), Department of Defense (DOD) (W81XWH-17-1-0249), The Michael J. Fox Foundation and American Parkinson disease Association Center for Advanced Research; AC is supported by the South African Research Chairs Initiative of the Department of Science and Technology and National Research Foundation of South Africa (award number UID 64751); SB is supported by grants from the South African Medical Research Council (Self-Initiated Research Grant) and the National Research Foundation of South Africa (Grant Numbers: 106052 and 129249).
The authors declare that the research was conducted in the absence of any commercial or financial relationships that could be construed as a potential conflict of interest.
All claims expressed in this article are solely those of the authors and do not necessarily represent those of their affiliated organizations, or those of the publisher, the editors and the reviewers. Any product that may be evaluated in this article, or claim that may be made by its manufacturer, is not guaranteed or endorsed by the publisher.
We acknowledge the support of the DST-NRF Centre of Excellence for Biomedical Tuberculosis Research, South African Medical Research Council Centre for Tuberculosis Research, Division of Molecular Biology and Human Genetics, Faculty of Medicine and Health Sciences, Stellenbosch University, Cape Town, South Africa.
Abyzov, A., Urban, A. E., Snyder, M., and Gerstein, M. (2011). CNVnator: An Approach to Discover, Genotype, and Characterize Typical and Atypical CNVs from Family and Population Genome Sequencing. Genome Res. 21, 974–984. pmid:21324876. doi:10.1101/gr.114876.110
Ball, N., Teo, W.-P., Chandra, S., and Chapman, J. (2019). Parkinson's Disease and the Environment. Front. Neurol. 10, 218. doi:10.3389/fneur.2019.00218
Bandres-Ciga, S., Diez-Fairen, M., Kim, J. J., and Singleton, A. B. (2020). Genetics of Parkinson's Disease: An Introspection of its Journey towards Precision Medicine. Neurobiol. Dis. 137 (January), 104782. doi:10.1016/j.nbd.2020.104782
Belkadi, A., Bolze, A., Itan, Y., Cobat, A., Vincent, Q. B., Antipenko, A., et al. (2015). Whole-genome Sequencing Is More Powerful Than Whole-Exome Sequencing for Detecting Exome Variants. Proc. Natl. Acad. Sci. USA 112 (17), 5473–5478. doi:10.1073/pnas.1418631112
Bentley, S. R., Guella, I., Sherman, H. E., Neuendorf, H. M., Sykes, A. M., Fowdar, J. Y., et al. (2021). Hunting for Familial Parkinson's Disease Mutations in the Post Genome Era. Genes 12 (3), 430. doi:10.3390/genes12030430
Boeva, V., Popova, T., Bleakley, K., Chiche, P., Cappo, J., Schleiermacher, G., et al. (2012). Control-FREEC: a Tool for Assessing Copy Number and Allelic Content Using Next-Generation Sequencing Data. Bioinformatics (Oxford, England) 28 (3), 423–425. doi:10.1093/bioinformatics/btr670
Bonnefond, A., Durand, E., Sand, O., De Graeve, F., Gallina, S., Busiah, K., et al. (2010). Molecular Diagnosis of Neonatal Diabetes Mellitus Using Next-Generation Sequencing of the Whole Exome. PLOS ONE 5 (10), e13630. doi:10.1371/journal.pone.0013630
Bope, C. D., Chimusa, E. R., Nembaware, V., Mazandu, G. K., de Vries, J., and Wonkam, A. (2019). Dissecting In Silico Mutation Prediction of Variants in African Genomes: Challenges and Perspectives. Front. Genet. 10, 601. doi:10.3389/fgene.2019.00601
Boudellioua, I., Kulmanov, M., Schofield, P. N., Gkoutos, G. V., and Hoehndorf, R. (2019). DeepPVP: Phenotype-Based Prioritization of Causative Variants Using Deep Learning. BMC Bioinformatics 20, 65. doi:10.1186/s12859-019-2633-8
Bras, J., and Singleton, A. (2011). Exome Sequencing in Parkinson's Disease. Clin. Genet. 80 (2), 104–109. doi:10.1111/j.1399-0004.2011.01722.x
Chakravorty, S., and Hegde, M. (2017). Gene and Variant Annotation for Mendelian Disorders in the Era of Advanced Sequencing Technologies. Annu. Rev. Genom. Hum. Genet. 18, 229–256. doi:10.1146/annurev-genom-083115-022545
Chen, J., Althagafi, A., and Hoehndorf, R. (2021). Predicting Candidate Genes from Phenotypes, Functions and Anatomical Site of Expression. Bioinformatics (Oxford, England) 37 (6), 853–860. doi:10.1093/bioinformatics/btaa879
Chen, S., and Lin, X. (2020). Analysis in Case-Control Sequencing Association Studies with Different Sequencing Depths. Biostatistics (Oxford, England) 21 (3), 577–593. doi:10.1093/biostatistics/kxy073
Correia Guedes, L., Ferreira, J. J., Rosa, M. M., Coelho, M., Bonifati, V., and Sampaio, C. (2010). Worldwide Frequency of G2019S LRRK2 Mutation in Parkinson's Disease: a Systematic Review. Parkinsonism Relat. Disord. 16 (4), 237–242. doi:10.1016/j.parkreldis.2009.11.004
Correia Guedes, L., Mestre, T., Outeiro, T. F., and Ferreira, J. J. (2020). Are Genetic and Idiopathic Forms of Parkinson's Disease the Same Disease. J. Neurochem. 152, 515–522. doi:10.1111/jnc.14902
Courtin, T., Tesson, C., Corvol, J.-C., Lesage, S., and Brice, A. (2021). Lack of Evidence for Association of UQCRC1 with Autosomal Dominant Parkinson's Disease in Caucasian Families. Neurogenetics 22, 365–366. doi:10.1007/s10048-021-00647-4
Day, J. O., and Mullin, S. (2021). The Genetics of Parkinson's Disease and Implications for Clinical Practice. Genes 12 (7), 1006. doi:10.3390/genes12071006
Dekker, M. C. J., Coulibaly, T., Bardien, S., Ross, O. A., Carr, J., and Komolafe, M. (2020). Parkinson's Disease Research on the African Continent: Obstacles and Opportunities. Front. Neurol. 11 (June). doi:10.3389/fneur.2020.00512
Deng, H.-X., Shi, Y., Yang, Y., Ahmeti, K. B., Miller, N., Huang, C., et al. (2016). Identification of TMEM230 Mutations in Familial Parkinson's Disease. Nat. Genet. 48 (7), 733–739. doi:10.1038/ng.3589
Edvardson, S., Cinnamon, Y., Ta-Shma, A., Shaag, A., Yim, Y.-I., Zenvirt, S., et al. (2012). A Deleterious Mutation in DNAJC6 Encoding the Neuronal-specific Clathrin-Uncoating Co-chaperone Auxilin, Is Associated with Juvenile Parkinsonism. PLoS ONE 7 (5), e36458–8. doi:10.1371/journal.pone.0036458
El-Fishawy, P. (2013). “Common Disease-Rare Variant Hypothesis,” in Encyclopedia of Autism Spectrum Disorders (New York: Springer), 720–722. doi:10.1007/978-1-4419-1698-3_1997
Erratum, (2019). Genetic Risk of Parkinson Disease and Progression: An Analysis of 13 Longitudinal Cohorts. Neurol. Genet. 5 (4), e354. doi:10.1212/NXG.0000000000000354
Farlow, J. L., Robak, L. A., Hetrick, K., Bowling, K., Boerwinkle, E., Coban-Akdemir, Z. H., et al. (2016). Whole-Exome Sequencing in Familial Parkinson Disease. JAMA Neurol. 73 (1), 68–75. doi:10.1001/jamaneurol.2015.3266
Farrer, M. J. (2019). Doubts about TMEM230 as a Gene for Parkinsonism. Nat. Genet. 51 (3), 367–368. doi:10.1038/s41588-019-0354-6
Favalli, V., Tini, G., Bonetti, E., Vozza, G., Guida, A., Gandini, S., et al. (2021). Machine Learning-Based Reclassification of Germline Variants of Unknown Significance: The RENOVO Algorithm. Am. J. Hum. Genet. 108 (4), 682–695. doi:10.1016/j.ajhg.2021.03.010
Fernandez-Marmiesse, A., Gouveia, S., and Couce, M. L. (2018). NGS Technologies as a Turning Point in Rare Disease Research , Diagnosis and Treatment. Cmc 25 (3), 404–432. doi:10.2174/0929867324666170718101946
Flanagan, S. E., Patch, A.-M., and Ellard, S. (2010). Using SIFT and PolyPhen to Predict Loss-Of-Function and Gain-Of-Function Mutations. Genet. Test. Mol. biomarkers 14 (4), 533–537. doi:10.1089/gtmb.2010.0036
Fortier, N., Rudy, G., and Scherer, A. (2018). Detection of CNVs in NGS Data Using VS-CNV. Methods Mol. Biol. (Clifton, N.J.) 1833, 115–127. doi:10.1007/978-1-4939-8666-8_9
Funayama, M., Ohe, K., Amo, T., Furuya, N., YamaguchiSaiki, J., Saiki, S., et al. (2015). CHCHD2 Mutations in Autosomal Dominant Late-Onset Parkinson's Disease: a Genome-wide Linkage and Sequencing Study. Lancet Neurol. 14 (3), 274–282. doi:10.1016/S1474-4422(14)70266-2
Gasser, T., Hardy, J., and Mizuno, Y. (2011). Milestones in PD Genetics. Mov. Disord. 26 (6), 1042–1048. doi:10.1002/mds.23637
Gasser, T. (2015). Usefulness of Genetic Testing in PD and PD Trials: A Balanced Review. Jpd 5 (2), 209–215. doi:10.3233/JPD-140507
Germer, E. L., Imhoff, S., Vilariño-Güell, C., Kasten, M., Seibler, P., Brüggemann, N., Klein, C., and Trinh, J.International Parkinson’s Disease Genomics Consortium (2019). The Role of Rare Coding Variants in Parkinson's Disease GWAS Loci. Front. Neurol. 10, 1284. doi:10.3389/fneur.2019.01284
Gialluisi, A., Reccia, M. G., Tirozzi, A., Nutile, T., Lombardi, A., De Sanctis, C., et al. (2020). Whole Exome Sequencing Study of Parkinson Disease and Related Endophenotypes in the Italian Population. Front. Neurol. 10, 1362. doi:10.3389/fneur.2019.01362
Giani, A. M., Gallo, G. R., Gianfranceschi, L., and Formenti, G. (2019). Long Walk to Genomics: History and Current Approaches to Genome Sequencing and Assembly. Comput. Struct. Biotechnol. J. doi:10.1016/j.csbj.2019.11.002
Global Parkinson's Genetics Program (2021). GP2: The Global Parkinson's Genetics Program. Move. Disord. : official J. Move. Disord. Soc. 36 (4), 842–851. doi:10.1002/mds.28494
Guo, J.-f., Zhang, L., Li, K., Mei, J.-p., Xue, J., Chen, J., et al. (2018). Coding Mutations inNUS1contribute to Parkinson's Disease. Proc. Natl. Acad. Sci. USA 115 (45), 11567–11572. doi:10.1073/pnas.1809969115
Hemminki, K., Försti, A., and Bermejo, J. L. (2008). The 'common Disease-Common Variant' Hypothesis and Familial Risks. PloS one 3 (6), e2504. doi:10.1371/journal.pone.0002504
Hernandez, D. G., Reed, X., and Singleton, A. B. (2016)., 139. Blackwell Publishing Ltd, 59–74. doi:10.1111/jnc.13593Genetics in Parkinson Disease: Mendelian versus Non-mendelian InheritanceJ. Neurochem.
Hildebrandt, F., and Omran, H. (1999). “Positional Cloning and Linkage Analysis,” in Techniques in Molecular Medicine (Springer Berlin Heidelberg), 352–363. doi:10.1007/978-3-642-59811-1_23
Hill, T., and Unckless, R. L. (2019). A Deep Learning Approach for Detecting Copy Number Variation in Next-Generation Sequencing Data. G3 (Bethesda, Md. 9 (11), 3575–3582. doi:10.1534/g3.119.400596
Huang, T., Li, J., Jia, B., and Sang, H. (2021). CNV-MEANN: A Neural Network and Mind Evolutionary Algorithm-Based Detection of Copy Number Variations from Next-Generation Sequencing Data. Front. Genet. 12, 700874. doi:10.3389/fgene.2021.700874
International Parkinson Disease Genomics Consortium (IPDGC) (2020). Ten Years of the International Parkinson Disease Genomics Consortium: Progress and Next Steps. J. Parkinsons Dis. 10 (1), 19–30. doi:10.3233/JPD-191854
Kalinderi, K., Bostantjopoulou, S., and Fidani, L. (2016). The Genetic Background of Parkinson's Disease: Current Progress and Future Prospects. Acta Neurol. Scand. 134 (5), 314–326. doi:10.1111/ane.12563
Karczewski, K. J., Francioli, L. C., Tiao, G., Cummings, B. B., Alföldi, J., Wang, Q., et al. (2020). The Mutational Constraint Spectrum Quantified from Variation in 141,456 Humans. Nature 581 (7809), 434–443. doi:10.1038/s41586-020-2308-7
Keogh, M. J., and Chinnery, P. F. (2013). Next Generation Sequencing for Neurological Diseases: New hope or New Hype. Clin. Neurol. Neurosurg. 115 (7), 948–953. doi:10.1016/j.clineuro.2012.09.030
Kim, H.-J., Won, H.-H., Park, K.-J., Hong, S. H., Ki, C.-S., Cho, S. S., et al. (2013). SNP Linkage Analysis and Whole Exome Sequencing Identify a Novel POU4F3 Mutation in Autosomal Dominant Late-Onset Nonsyndromic Hearing Loss (DFNA15). PloS one 8 (11), e79063. doi:10.1371/journal.pone.0079063
Klein, C., Chuang, R., Marras, C., and Lang, A. E. (2011). The Curious Case of Phenocopies in Families with Genetic Parkinson's Disease. Mov. Disord. 26 (10), 1793–1802. doi:10.1002/mds.23853
Krebs, C. E., Karkheiran, S., Powell, J. C., Cao, M., Makarov, V., Darvish, H., et al. (2013). The Sac1 Domain of SYNJ 1 Identified Mutated in a Family with Early‐Onset Progressive P Arkinsonism with Generalized Seizures. Hum. Mutat. 34 (9), 1200–1207. doi:10.1002/humu.22372
Ku, C.-S., Cooper, D. N., and Patrinos, G. P. (2016). The Rise and Rise of Exome Sequencing. Public health genomics 19 (6), 315–324. doi:10.1159/000450991
Kumaran, M., Subramanian, U., and Devarajan, B. (2019). Performance Assessment of Variant Calling Pipelines Using Human Whole Exome Sequencing and Simulated Data. BMC Bioinformatics 20, 342. doi:10.1186/s12859-019-2928-9
Layer, R. M., Chiang, C., Quinlan, A. R., and Hall, I. M. (2014). LUMPY: a Probabilistic Framework for Structural Variant Discovery. Genome Biol. 15 (6), R84. doi:10.1186/gb-2014-15-6-r84
Lesage, S., and Brice, A. (2012). Role of Mendelian Genes in "sporadic" Parkinson's Disease. Parkinsonism Relat. Disord. 18 (Suppl. 1), S66–S70. doi:10.1016/s1353-8020(11)70022-0
Li, B., Zhao, G., Zhou, Q., Xie, Y., Wang, Z., Fang, Z., et al. (2021). Gene4PD: A Comprehensive Genetic Database of Parkinson's Disease. Front. Neurosci. 15, 679568. doi:10.3389/fnins.2021.679568
Li, W., Fu, Y., Halliday, G. M., and Sue, C. M. (2021). PARK Genes Link Mitochondrial Dysfunction and Alpha-Synuclein Pathology in Sporadic Parkinson's Disease. Front. Cel Dev. Biol. 9, 612476. doi:10.3389/fcell.2021.612476
MacArthur, D. G., Manolio, T. A., Dimmock, D. P., Rehm, H. L., Shendure, J., Abecasis, G. R., et al. (2014). Guidelines for Investigating Causality of Sequence Variants in Human Disease. Nature 508 (7497), 469–476. doi:10.1038/nature13127
Mantere, T., Kersten, S., and Hoischen, A. (2019). Long-read Sequencing Emerging in Medical Genetics. Front. Genet. 10 (MAY), 1–14. doi:10.3389/fgene.2019.00426
Mulder, N. J., Adebiyi, E., Adebiyi, M., Adeyemi, S., Ahmed, A., Ahmed, R., et al. (2017). Development of Bioinformatics Infrastructure for Genomics Research. gh 12 (2), 91–98. doi:10.1016/j.gheart.2017.01.005
Müller‐Nedebock, A. C., Komolafe, M. A., Fawale, M. B., Carr, J. A., Westhuizen, F. H., Ross, O. A., et al. (2021). Copy Number Variation in Parkinson's Disease: An Update from Sub‐Saharan Africa. Mov Disord. 36, 2442–2444. doi:10.1002/MDS.28710
Nalls, M. A., Blauwendraat, C., Vallerga, C. L., Heilbron, K., Bandres-Ciga, S., Chang, D., et al. (2019). International Parkinson's Disease Genomics ConsortiumIdentification of Novel Risk Loci, Causal Insights, and Heritable Risk for Parkinson's Disease: a Meta-Analysis of Genome-wide Association Studies. The Lancet. Neurology 18 (12), 1091–1102. doi:10.1016/S1474-4422(19)30320-5
Navale, V., and Bourne, P. E. (2018). Cloud Computing Applications for Biomedical Science: A Perspective. Plos Comput. Biol. 14 (6), e1006144. doi:10.1371/journal.pcbi.1006144
Niroula, A., and Vihinen, M. (2019). How Good Are Pathogenicity Predictors in Detecting Benign Variants. Plos Comput. Biol. 15 (2), e1006481. doi:10.1371/journal.pcbi.1006481
Odumpatta, R., and Mohanapriya, A. (2020). Next Generation Sequencing Exome Data Analysis Aids in the Discovery of SNP and INDEL Patterns in Parkinson's Disease. Genomics 112 (5), 3722–3728. doi:10.1016/j.ygeno.2020.04.025
Olgiati, S., Quadri, M., Fang, M., Rood, J. P. M. A., Saute, J. A., Chien, H. F., Bouwkamp, C. G., Graafland, J., Minneboo, M., Breedveld, G. J., Zhang, J., Verheijen, F. W., Boon, A. J. W., Kievit, A. J. A., Jardim, L. B., Mandemakers, W., Barbosa, E. R., Rieder, C. R. M., Leenders, K. L., Wang, J., and Bonifati, V.International Parkinsonism Genetics Network (2016). D NAJC 6 Mutations Associated with Early-Onset Parkinson's Disease. Ann. Neurol. 79 (2), 244–256. doi:10.1002/ana.24553
Pan, B., Kusko, R., Xiao, W., Zheng, Y., Liu, Z., Xiao, C., et al. (2019). Similarities and Differences between Variants Called with Human Reference Genome HG19 or HG38. BMC Bioinformatics 20, 101. doi:10.1186/s12859-019-2620-0
Piro, R. M., and Di Cunto, F. (2012). Computational Approaches to Disease-Gene Prediction: Rationale, Classification and Successes. FEBS J. 279 (5), 678–696. doi:10.1111/j.1742-4658.2012.08471.x
Puschmann, A. (2017). New Genes Causing Hereditary Parkinson's Disease or Parkinsonism. Curr. Neurol. Neurosci. Rep. 17, 66. doi:10.1007/s11910-017-0780-8
Quadri, M., Mandemakers, W., Grochowska, M. M., Masius, R., Geut, H., Fabrizio, E., et al. (2018). LRP10 Genetic Variants in Familial Parkinson's Disease and Dementia with Lewy Bodies: a Genome-wide Linkage and Sequencing Study. Lancet Neurol. 17 (7), 597–608. doi:10.1016/S1474-4422(18)30179-0
Rentzsch, P., Schubach, M., Shendure, J., and Kircher, M. (2021). CADD-Splice-improving Genome-wide Variant Effect Prediction Using Deep Learning-Derived Splice Scores. Genome Med. 13 (1), 1–12. doi:10.1186/s13073-021-00835-9
Rodenburg, R. J. (2018). The Functional Genomics Laboratory: Functional Validation of Genetic Variants. J. Inherit. Metab. Dis. 41 (3), 297–307. doi:10.1007/s10545-018-0146-7
Ruiz-Martínez, J., Azcona, L. J., Bergareche, A., Martí-Massó, J. F., and Paisán-Ruiz, C. (2017). Whole-exome Sequencing Associates Novel CSMD1 Gene Mutations with Familial Parkinson Disease. Neurol. Genet. 3 (5), e177. doi:10.1212/NXG.0000000000000177
Russell, P. H., Johnson, R. L., Ananthan, S., Harnke, B., and Carlson, N. E. (2018). A Large-Scale Analysis of Bioinformatics Code on GitHub. PloS one 13 (10), e0205898. doi:10.1371/journal.pone.0205898
Schoonen, M., Seyffert, A. S., van der Westhuizen, F. H., and Smuts, I. (2019). A Bioinformatics Pipeline for Rare Genetic Diseases in South African Patients. S. Afr. J. Sci. 115 (3-4), 1–3. doi:10.17159/sajs.2019/4876
Schormair, B., Kemlink, D., Mollenhauer, B., Fiala, O., Machetanz, G., Roth, J., et al. (2018). Diagnostic Exome Sequencing in Early-Onset Parkinson's Disease confirmsVPS13Cas a Rare Cause of Autosomal-Recessive Parkinson's Disease. Clin. Genet. 93 (3), 603–612. doi:10.1111/cge.13124
Schulte, E. C., Stahl, I., Czamara, D., Ellwanger, D. C., Eck, S., Graf, E., et al. (2013). Rare Variants in PLXNA4 and Parkinson's Disease. PloS one 8 (11), e79145. doi:10.1371/journal.pone.0079145
Sebate, B., Cuttler, K., Cloete, R., Britz, M., Christoffels, A., Williams, M., et al. (2021). Prioritization of Candidate Genes for a South African Family with Parkinson's Disease Using In-Silico Tools. PloS one 16 (3), e0249324. doi:10.1371/journal.pone.0249324
Shulskaya, M. V., Alieva, A. K., Vlasov, I. N., Zyrin, V. V., Fedotova, E. Y., Abramycheva, N. Y., et al. (2018). Whole-Exome Sequencing in Searching for New Variants Associated with the Development of Parkinson's Disease. Front. Aging Neurosci. 10 (MAY), 1–8. doi:10.3389/fnagi.2018.00136
Straniero, L., Guella, I., Cilia, R., Parkkinen, L., Rimoldi, V., Young, A., et al. (2017). DNAJC12 and Dopa-Responsive Nonprogressive Parkinsonism. Ann. Neurol. 82 (4), 640–646. doi:10.1002/ana.25048
Sudhaman, S., Muthane, U. B., Behari, M., Govindappa, S. T., Juyal, R. C., and Thelma, B. K. (2016b). Evidence of Mutations inRIC3acetylcholine Receptor Chaperone as a Novel Cause of Autosomal-Dominant Parkinson's Disease with Non-motor Phenotypes. J. Med. Genet. 53 (8), 559–566. doi:10.1136/jmedgenet-2015-103616
Sudhaman, S., Prasad, K., Behari, M., Muthane, U. B., Juyal, R. C., and Thelma, B. (2016a). Discovery of a Frameshift Mutation in Podocalyxin-like (PODXL) Gene, Coding for a Neural Adhesion Molecule, as Causal for Autosomal-Recessive Juvenile Parkinsonism. J. Med. Genet. 53 (7), 450–456. doi:10.1136/jmedgenet-2015-103459
Tseng, E., Rowell, W. J., Glenn, O.-C., Hon, T., Barrera, J., Kujawa, S., et al. (2019). The Landscape of SNCA Transcripts across Synucleinopathies: New Insights from Long Reads Sequencing Analysis. Front. Genet. 10 (JUL). doi:10.3389/fgene.2019.00584
Vahidnezhad, H., Youssefian, L., Jazayeri, A., and Uitto, J. (2018). Research Techniques Made Simple: Genome-wide Homozygosity/Autozygosity Mapping Is a Powerful Tool for Identifying Candidate Genes in Autosomal Recessive Genetic Diseases. J. Invest. Dermatolelsevier B.V 138 (Issue 9), 1893–1900. doi:10.1016/j.jid.2018.06.170
Vilariño-Güell, C., Rajput, A., Milnerwood, A. J., Shah, B., Szu-Tu, C., Trinh, J., et al. (2014). DNAJC13 Mutations in Parkinson Disease. Hum. Mol. Genet. 23 (7), 1794–1801. doi:10.1093/hmg/ddt570
Vilariño-Güell, C., Wider, C., Ross, O. A., Dachsel, J. C., Kachergus, J. M., Lincoln, S. J., et al. (2011). VPS35 Mutations in Parkinson Disease. Am. J. Hum. Genet. 89 (1), 162–167. doi:10.1016/j.ajhg.2011.06.001
Wakeling, M. N., Laver, T. W., Wright, C. F., De Franco, E., Stals, K. L., Patch, A.-M., et al. (2019). Homozygosity Mapping Provides Supporting Evidence of Pathogenicity in Recessive Mendelian Disease. Genet. Med. 21 (4), 982–986. doi:10.1038/s41436-018-0281-4
Wang, W., Corominas, R., and Lin, G. N. (2019). De Novo Mutations from Whole Exome Sequencing in Neurodevelopmental and Psychiatric Disorders: From Discovery to Application. Front. Genet. 10 (APR). doi:10.3389/fgene.2019.00258
Williams, U., Bandmann, O., and Walker, R. (2018). Parkinson's Disease in Sub-saharan Africa: A Review of Epidemiology, Genetics and Access to Care. Jmd 11 (2), 53–64. doi:10.14802/jmd.17028
Wong, K. H. Y., Ma, W., Wei, C.-Y., Yeh, E.-C., Lin, W.-J., Wang, E. H. F., et al. (2020). Towards a Reference Genome that Captures Global Genetic Diversity. Nat. Commun. 11, 5482. doi:10.1038/s41467-020-19311-w
Zhang, L., Bai, W., Yuan, N., and Du, Z. (2019). Correction: Comprehensively Benchmarking Applications for Detecting Copy Number Variation. Plos Comput. Biol. 15 (9), e1007367. doi:10.1371/journal.pcbi.1007367
Zhao, S., Agafonov, O., Azab, A., Stokowy, T., and Hovig, E. (2020). Accuracy and Efficiency of Germline Variant Calling Pipelines for Human Genome Data. Sci. Rep. 10 (1), 20222. doi:10.1038/s41598-020-77218-4
Keywords: Parkinson’s disease, next-generation sequencing, whole-exome sequencing, familial PD, african ancestry, bioinformatic pipelines, third-generation sequencing, diverse populations
Citation: Pillay NS, Ross OA, Christoffels A and Bardien S (2022) Current Status of Next-Generation Sequencing Approaches for Candidate Gene Discovery in Familial Parkinson´s Disease. Front. Genet. 13:781816. doi: 10.3389/fgene.2022.781816
Received: 23 September 2021; Accepted: 12 January 2022;
Published: 01 March 2022.
Edited by:
Amir Hossein Saeidian, Thomas Jefferson University, United StatesReviewed by:
Rachita Yadav, Massachusetts General Hospital and Harvard Medical School, United StatesCopyright © 2022 Pillay, Ross, Christoffels and Bardien. This is an open-access article distributed under the terms of the Creative Commons Attribution License (CC BY). The use, distribution or reproduction in other forums is permitted, provided the original author(s) and the copyright owner(s) are credited and that the original publication in this journal is cited, in accordance with accepted academic practice. No use, distribution or reproduction is permitted which does not comply with these terms.
*Correspondence: Soraya Bardien, c2JhcmRpZW5Ac3VuLmFjLnph
Disclaimer: All claims expressed in this article are solely those of the authors and do not necessarily represent those of their affiliated organizations, or those of the publisher, the editors and the reviewers. Any product that may be evaluated in this article or claim that may be made by its manufacturer is not guaranteed or endorsed by the publisher.
Research integrity at Frontiers
Learn more about the work of our research integrity team to safeguard the quality of each article we publish.