- 1Hubei Engineering Research Center for Protection and Utilization of Special Biological Resources in the Hanjiang River Basin, Jianghan University, Wuhan, China
- 2Gansu Key Laboratory of Cold Water Fishes Germplasm Resources and Genetics Breeding, Gansu Fishers Research Institute, Lanzhou, China
- 3Hubei Key Laboratory of Environmental and Health Effects of Persistent Toxic Substances, Jianghan University, Wuhan, China
T. yarkandensis is a common species of Triplophysa, and it is distributed in Shule river of Hexi Corridor, of Gansu province in China. In order to enrich gene database resources and explore the environment adaptation of T. yarkandensis, fifteen tissues were collected from three adult T. yarkandensis for transcriptome sequencing and de novo assembly. Nine major international gene annotation databases (NR, COG, egg_NOG, TrEMBL, Pfam, KOG, Swiss prot, KEGG and Gene Ontology) were utilized to annotate unigenes. A detailed study was conducted to explore the gene expression and the differentially expressed genes among five tissues (brain, heart, kidney, liver and spleen). In addition, the current study showed that candidate genes involved in salinity-alkalinity and low temperature adaptation were differentially expressed in tissues of T. yarkandensis. Precisely, mapk1, abcc1, gpx1, gpx4, cat and aqp1 genes participated in the regulation process of salinity-alkalinity adaptation, and elovl4, acaca, fasn, acaa2, acox1 and acox3 genes were involved in fatty acid metabolism and closely associated with low temperature adaptation. On the one hand, it was found that the expression of these genes varied among different tissues, and the important pathways involved in these genes were mapped. Furthermore, we analyzed mapk1 and acox1 genes in depth to obtain the predicted gene structure and important amino acid sites. The transcriptome information in this study will be conducive to provide further understanding for the molecular level research and exploration of the environmental adaptation of T. yarkandensis.
Introduction
T. yarkandensis belongs to the Triplophysa genus within the Nemacheilidae family in the order Cypriniformes (Chen et al., 2017), and it is the most respresentative fish species of the fish population in the adjacent Qinghai-Tibetan Plateau and one of the high-altitude fishes (Chen et al., 2010). T. yarkandensis has a length and weight of about 30.0 cm and 305 g respectively (Chen et al., 2017), it possess the biological characteristics of benthic life, with periodic migration, selective spawning, and fierce feeding (Chen and Yao, 2008). The optimal physiological function for T. yarkandensis are encountered at a temperature of 6°–7°C, which means that this fish species has a strong low temperature adaptability (Xiang and Fan, 2014). Shule river is one of the three major inland rivers in Gansu province of China. It is located at the westernmost end of Hexi Corridor, with temperature of 6.98°C–9.82°C (Wang et al., 2022a). Shule river mainly came from melting snow and ice, atmospheric precipitation and groundwater (Guo et al., 2015). In line with the research conducted on the hydrochemical characteristics of Shule river basin, the main ions were Ca2+, Mg2+, Na+, HCO3−, SO42-, Cl−. The river shows a bicarbonate alkalinity with a pH of 7–8, which indicates a weak alkaline (Zhou et al., 2004; Yang et al., 2021).
In recent years, in view of the sharp decline of natural resources of T. yarkandensis, researchers have started to focus on the characteristics of T. yarkandensis population (Chen et al., 2011) and reproduction (Chen et al., 2013). Additionally, the complete mitochondrial genome of T. yarkandensis has been determined (Chen et al., 2016). Besides, a research to develop microsatellite markers of the populations of T. yarkandensis showed that the microsatellite markers of dibasic repeat was significantly in T. yarkandensis (Wang et al., 2020). Furthermore, the assessment of the otolith morphology was applied for the first time to classify and identify the population of T. yarkandensis. This analysis found that the relationship between otolith morphology and fish body growth greatly reflected the adaptability of the individuals’ development to their habitat (Wang et al., 2022b). Also, a study on genetic diversity of different geographical populations based on mitochondrial Cytb and D-loop sequences indicated that T. yarkandensis populations in Tarim river basin were relatively stable and no obvious population expansion occurred recently (Zhao et al., 2022).
In recent years, RNA sequencing (RNA-Seq) has been prevalently used in fundamental research as an effective tool and standard for transcriptome analysis (Dewey and Li, 2011). Transcriptome studies could comprehensively elucidate the functions and structures of genes and the molecular mechanisms of biological processes (Guan et al., 2020). As revealed by the transcriptome analysis of Leuciscus waleckii, genes involved in ion transportation was crucial in the acid-base homeostasis of fish under alkaline stress (Chang et al., 2014). Transcriptomic data identified several immune-related genes in Betta splendens (Amparyup et al., 2020). Transcriptome analysis of Triplophysa dalaica identified genes that were involved in hypoxia response (Wang et al., 2015). Liver transcriptome analysis not only identified that genes were involved in the responses of turbot to different salinity conditions, but also demonstrated that low salinity exposure disorders turbot lipid metabolism (Liu et al., 2020). Transcriptomic and metabolomic analyses indicated that when compared with diploid Apostichopus japonicus, triploid A. japonicus had more advantages in immunity, growth and development, stress resistance and adaptability to a harsh living environment (Xie et al., 2022). Based on RNA-seq, comparative transcriptome analysis of different tissues in Lota dalliance provided a reference for enriching gene resource (Yang et al., 2020). At present, studies have used the gill tissue of T. yarkandensis to analyze the comparative transcriptome of T. yarkandensis in response to salinity and alkalinity stress (Chen et al., 2020). However, there are no related studies that have been reported on multi-tissue transcriptome analysis and environmental adaptation of T. yarkandensis.
In the current study, the samples of brain, heart, kidney, liver and spleen of T. yarkandensis were extracted to provide a more comprehensive transcriptome reference. In addition, candidate genes involved in salinity-alkalinity and low temperature adaptation from the transcriptome of T. yarkandensis were selected. The results from the current study will provide additional knowledge on the biology of T. yarkandensis which can be used to improve conservation and breeding practices of this fish species.
Materials and methods
Sample collection, RNA extraction and sequencing
Three adults of T. yarkandensis were collected from the reach of Shule river basin (96.71 E, 40.57 N) at an altitude of 1,300 m. A total of fifteen tissues were collected from the three fish specimens including heart, liver, brain, spleen and kidney. These tissues were stored in ultra-low temperature liquid nitrogen at −80°C for standby. Liquid nitrogen was added to grind the tissues into powder, which allowed to extracted total RNA from the mixed tissues using the standard Trizol kit (Promega SV Total RNA Isolation system), and detect the quality of total RNA using agarose gel electrophoresis. This process allowed, the detection of the degradation degree, purity, quality, concentration and integrity of total RNA by Nanodrop spectrophotometer (Thermo Fisher Scientific, MA, United State), Qubit fluorescence quantitative analyzer (Thermo Fisher Scientific, MA, Unite State) and Agilent 2,100 biological analyzer (Agilent, CA, United State), to ensure that the samples met the requirements for transcriptome sequencing. After detection, the mRNA within the total RNA was broken into specific short fragments, and the double-terminal cDNA library of the transcriptome was amplified by RT-PCR. Based on sequencing by synthesis (SBS) technology, the cDNA library was sequenced by Illumina Hiseq high-throughput sequencing platform (Illumina, CA, United State), which could produce a large number of high-quality reads (raw data). After detecting quality and filtering, clean reads were produced.
De novo assembly and functional annotation
The clean reads were employed for de novo assembly using Trinity (v2.5.1) (Grabherr et al., 2011). The CD-HIT-EST program was utilized to remove the redundant sequences, and the parameters were set as the similarity of 0.95 and the sequence length of 10 bases (Li and Godzik, 2006). Annotation information were finally obtained by selecting BLAST (Altschul et al., 1997) parameter E-value ≤ 1e-5 and Hmmer (Finn et al., 2014) parameter E-value ≤ 1e-10. The annotation information of unigenes were derived from nine databases, including NCBI non-redundant protein sequence (NR) (Deng et al., 2006), Swiss prot (Rolf et al., 2004), Clusters of Orthologous Groups (COG) (Tatusov et al., 2000), EuKaryotic Orthologous Groups (KOG) (Koonin et al., 2004), Evolutionary genealogy of genes: Non-supervised Orthologous Groups (eggNOG) (Jaime et al., 2016), Gene Ontology (GO) (Ashburner et al., 2000), Kyoto Encyclopedia of Genes and Genomes (KEGG) (Minoru et al., 2004), Protein family (Pfam) (Finn et al., 2014), and Translation of EMBL (TrEMBL) (Amos and Rolf, 2000).
Gene expression and differential expression genes (DEGs) analysis
Bowtie (Langmead, 2009) was applied to compare the sequenced reads with the unigene library, and the expression level was estimated in combination with RSEM (Dewey and Li, 2011). The expression abundance of corresponding unigene was expressed by FPKM (Fragments Per Kilobase of transcript per Million mapped reads) value (Trapnell et al., 2010). Differential expression analysis was performed using DeSeq2 (v1.6.3) (Love et al., 2014). Differential expression results were obtained with FDR (False Discovery Rate) > .01 and the difference multiple FC (fold change) ≥ 2 were considered as the screening standards. The differentially expressed genes of five tissues were analyzed by GO functional annotation and KEGG pathway enrichment analysis.
Scanning for candidate genes in the salinity-alkalinity adaptation
In Shule river, the cations were predominantly Ca2+, Mg2+, Na+ and the anions were mainly HCO3−, SO42-, Cl−, and the pH was 7–8 (Zhou et al., 2004). The water was weakly saline and alkaline. Studies have indicated that T. yarkandensis had a certain tolerance under precondition of salinity and alkalinity stress (Yao et al., 2018). In the current study, six genes were identified which participated in the regulation process of salinity-alkalinity adaptation, these genes were mapk1 (mitogen-activated protein kinase 1), abcc1 (ATP-binding cassette sub-family C member 1), gpx1 (glutathione peroxidase 1), gpx4 (glutathione peroxidase 4), cat (catalase) and aqp1 (aquaporin-1) (Li et al., 2016; Chen et al., 2020; Zhang, 2020; Wang et al., 2022c). From the differential genes obtained in this study, the six genes were searched and the differences between tissues were analyzed. Additionally, according to the results of KEGG enrichment, the pathway map involving these genes was obtained. Finally, mapk1 gene was selected for bioinformatics analysis, including sequence characteristics and important amino acid sites. Firstly, FGENESH (http://www.softberry.com/berry.phtml?topic=fgenesh&group = programsandsubgroup = gfind) was used to analyze its sequence characteristics. Moreover, a search at the BLAST tool at NCBI was conducted for similar sequences of mapk1 gene, and important amino acid sites were compared by using Clustal Omega (https://www.ebi.ac.uk/Tools/msa/clustalo/).
Scanning for candidate genes in fatty acid metabolism related to low temperature adaptation
Water temperature is one of the crucial environmental factors not only affecting almost all life activities of fish, but also affecting the physiological and biochemical reactions of fish. Fish can gradually adapt low temperature environment by regulating the concentration of enzymes in different metabolic pathways. A previous report not only indicated that low temperature heightened lipid synthesis in the body of Trilophysa bleekeri, but also showed the content of polyunsaturated fatty acids in the body was up-regulated in the low temperature group (Yuan, 2021). Through fatty acid metabolism, the content of long-chain unsaturated fatty acids in phospholipid of cell membrane increased and the cell membrane would maintain stability and fluidity. In the current study, six genes was identified, which are involved in fatty acid metabolism pathway, these genes were acox1 (acyl-CoA oxidase 1, palmitoyl), acox3 (acyl-CoA oxidase 3, pristanoyl), acaa2 (acetyl-CoA acyltransferase 2), elovl4 (elongation of very long chain fatty acids elongase gene 4), acaca (acetyl-CoA carboxylases alpha) and fasn (fatty acid synthase) (Xu et al., 2020; Yang et al., 2020; Wang et al., 2022d; Liu et al., 2022). From the differential genes obtained in this study, the six genes were searched and the differences among tissues were analyzed. Moreover, according to the results of KEGG enrichment, the pathway map involving these genes was obtained. Finally, acox1 gene was selected for bioinformatics analysis, including sequence characteristics and important amino acid sites. Firstly, FGENESH (http://www.softberry.com/berry.phtml ?topic = fgeneshandgroup = programsandsubgroup = gfind) was used to analyze its sequence characteristics. Moreover, a search at the BLAST tool at NCBI was conducted for similar sequences of acox1 gene, and important amino acid sites were compared by using Clustal Omega (https://www.ebi.ac.uk/Tools/msa/clustalo/).
Results
Sequencing, de novo assembly and functional annotation
The cDNA library was sequenced using Illumina Hiseq high-throughput sequencing platform. After sequencing quality control, a total of 102.11 Gb clean data were obtained. Overall, the percentage of Q30 bases of all products exceeded 91.28%, and the GC content exceeded 44.90%. The sample sequencing data evaluation statistics information was shown in the Supplementary Table S1. A total of 100,112 unigenes were obtained by assembly, the N50 of transcript and unigene were 3,938 bp and 2,579 bp, with high assembly integrity (Supplementary Table S2, Supplementary Figure S1).
In the current study, 23,008 unigenes were annotated to nine databases, including 5,207, 19,535, 19,637, 15,404, 18,696, 13,941, 19,259, 18,421 and 19,270 unigenes in COG, GO, KEGG, KOG, Pfam, Swiss prot, TrEMBL, eggNOG and NR databases, respectively (Supplementary Table S3). Based on the BLASTx similarity analysis against the NR database, T. yarkandensis unigenes had the highest number of hits compared to other fish species such as to Carassius auratus (45.37%), followed by Anabarilius grahami (18.62%), Danio rerio (13.12%) (Figure 1A). A total of 19,637 assembled unigenes were assigned to 48 terms for GO classification in the biological process (25 terms), cellular component (6 terms), and molecular function (17 terms) categories (Figure 1B). Cellular anatomical entity (15,116 unigenes) showed the most annotated unigenes, followed by cellular process (13,879 unigenes), binding (12,320 unigenes), and intracellular (10,866 unigenes). In KOG database (Figure 1C), unigenes have been divided into 25 functional categories, and among them, the largest was signal transduction mechanisms, with 3,151 unigenes, accounting for 20.46%, followed by nothing but general function prediction (2,858 unigenes, 18.55%), posttranslational modification, protein turnover, chaperones (1,435 unigenes, 9.32%). No unknown genes were found from the KOG database annotation.
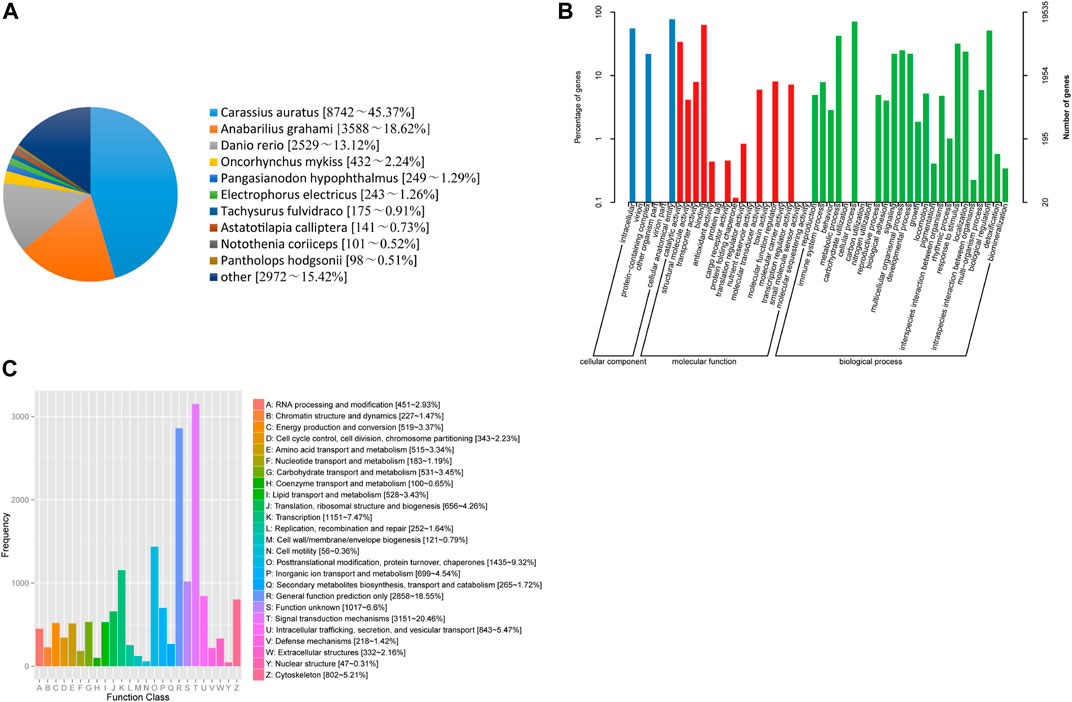
FIGURE 1. (A) Species distribution results of a similarity search of unigenes against Nr databases. (B) Functional classification of assembled unique sequences based on GO terms. (C) Functional classification of assembled unigenes based on KOG.
Analysis of differentially expressed genes (DEGs) among five tissues
We compared DEGs in five tissues: brain, heart, liver, kidney and spleen. Results of differentially expressed genes between each two tissues were shown in Supplementary Table S4. TYbrain_vs_TYliver screened the largest differentially expressed genes number, with a 9,814 DEGs, of which 3,818 genes were up-regulated and 5,996 genes were down-regulated (Figure 2A). Hierarchical clustering analysis was performed on the selected differentially expressed genes between brain and liver, and the genes with the same or similar expression behavior were clustered (Figure 2B). GO annotation results and KEGG enrichment of DEGs between brain and liver were shown in Figures 2C, D. The three biological duplicate samples of the same tissue could cluster, indicating that the biological duplicate of the samples used in this study was favorable. The GO annotation results of ten groups were similar. Most of the terms included “cellular process”, “single-organism process”, “biological regulation”, “metabolic process”, “cell”, “cell part”, “binding” and “catalytic activity”. In KEGG pathway enrichment analysis, DEGs were enriched including MAPK signaling pathway, calcium signaling pathway and neuroaction ligand-receptor interaction.
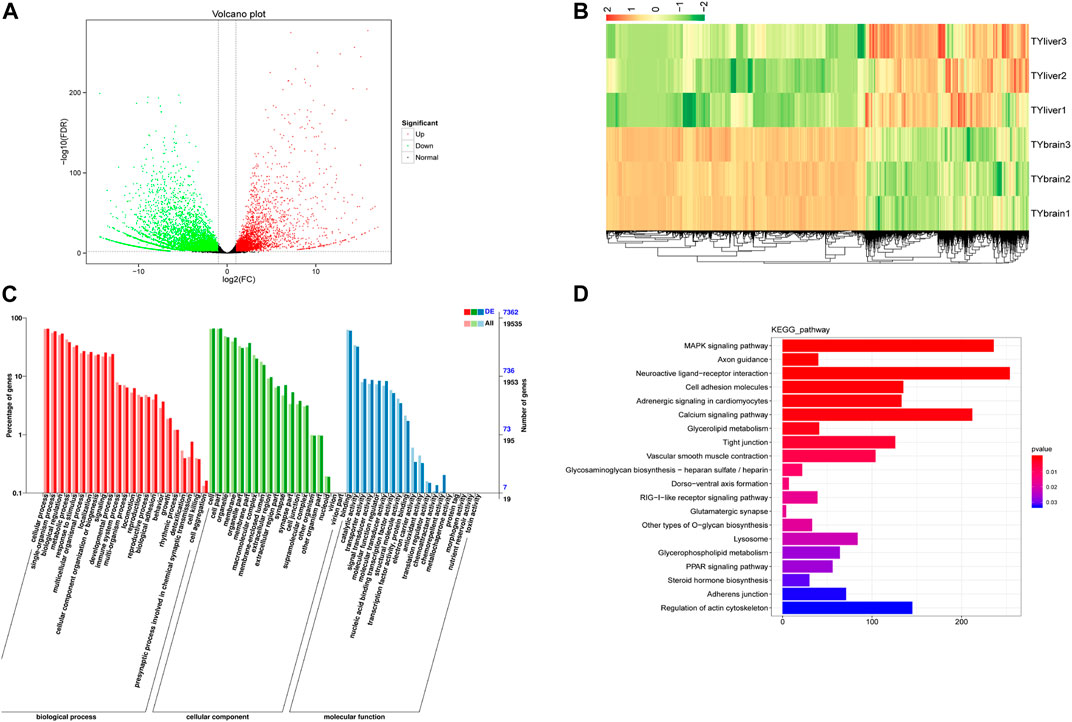
FIGURE 2. Differentially expressed genes (DEGs) analysis between brain and liver of T. yarkandensis. (A) Volcano plot of DEGs between brain and liver. Each point represents a gene. The green and red dots in the figure represent significantly differentially expressed genes, with green representing down-regulated gene expression, red representing up-regulated gene expression, and black dots represents a gene with no significant difference in expression. (B) Hierarchical clustering analysis was performed on the DEGs between brain and liver. The color represents log2(FC). (C) GO classification of DEGs between brain and liver. (D) KEGG enrichment of DEGs between brain and liver.
Signal pathway analysis was performed by KEGG
19,637 unigenes in KEGG database correspond to 330 pathways, and the top 20 pathways with the largest number of sequences were shown in the Supplementary Table S5. Unigenes with the largest three pathways annotations (neuroactive ligand-receptor interaction, calcium signaling pathway and MAPK signaling pathway) were associated with environmental information processing. In addition, eight of the top 20 pathways were correlated with signaling molecules and interaction, signal transduction. A previous study showed that different selenium sources could regulate the adaptation of yellow catfish to low temperature stress, neuroactive ligand-receptor interaction pathway significant enrichment (Hu et al., 2021). In the current study, the number of unigenes annotated in the neuroactive ligand-receptor interaction pathway was the largest, which was speculated to be associated with the low temperature environment adaptation of T. yarkandensis.
Candidate genes involved with salinity-alkalinity adaptation
In the current study, six candidate genes in the salinity-alkalinity adaptation: mapk1, abcc1, cat, gpx1, gpx4 and aqp1 were screened, and the expression differences of six genes among five tissues of T. yarkandensis were analyzed. The experimental results indicated that these genes were differentially expressed among the five tissues. As shown in Table 1, the expression of cat, abcc1 and gpx4 genes in liver was higher than that in brain, heart, kidney and spleen, while the expression of gpx1 and aqp1 genes in liver, heart, kidney and spleen was higher than that in brain. In addition, the expression of mapk1 genes in liver and kidney was higher. A previous research revealed that salinity stress could affect the changes of metabolic pathways (Liu et al., 2020). The six genes involved in the pathway were shown in Figure 3. Six genes in five tissues of T. yarkandensis were differentially expressed and all were highly expressed in the liver, which it was suspected to be related to the metabolism. Finally, mapk1 gene for bioinformatics analysis were selected, and the result showed that the sequence length was 2,424 bp, and there was one coding region of the predicted gene structure. Besides, divergent amino acid changes (Val-Ile at 321, 325) by comparing amino acid sequences with seven other fish were found (Figure 4A).
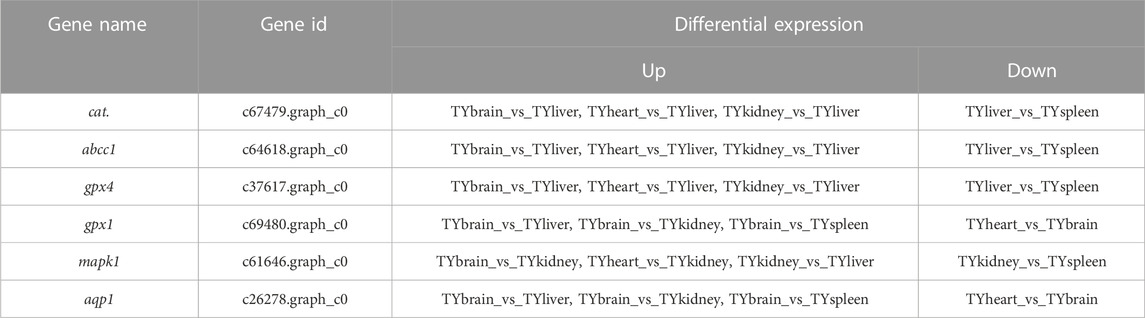
TABLE 1. Differential expression in tissues of six genes involved with salinity-alkalinity adaptation.
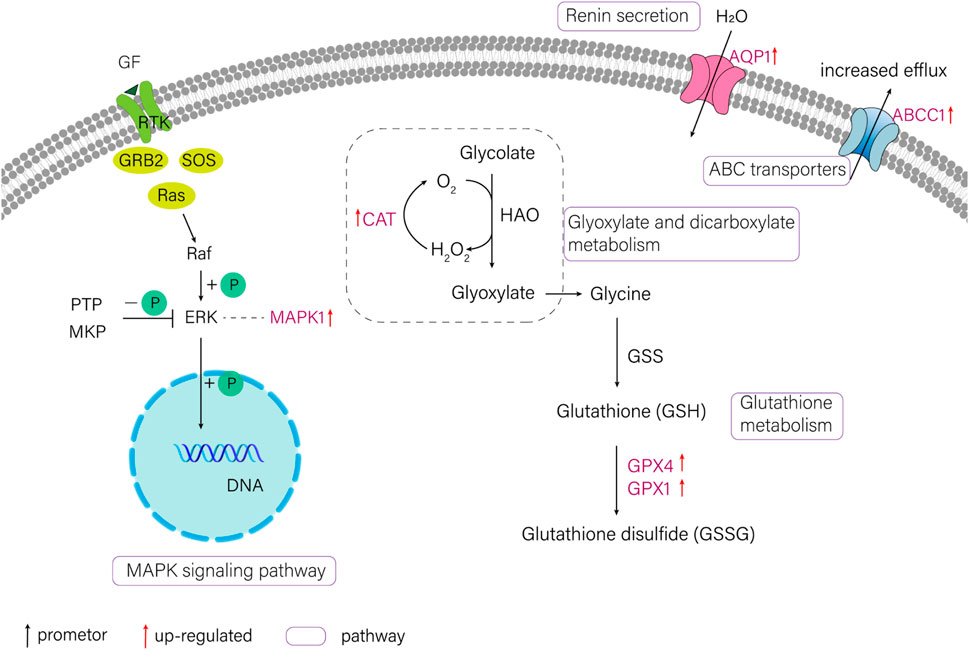
FIGURE 3. The schematic diagram of several pathways about salinity-alkalinity adaptation of T. yarkandensis. CAT (catalase), GPX1 (glutathione peroxidase 1), GPX4 (glutathione peroxidase 4), MAPK1 (mitogen-activated protein kinase 1), AQP1 (aquaporin-1), ABCC1 (ATP-binding cassette, subfamily C (CFTR/MRP), member 1).
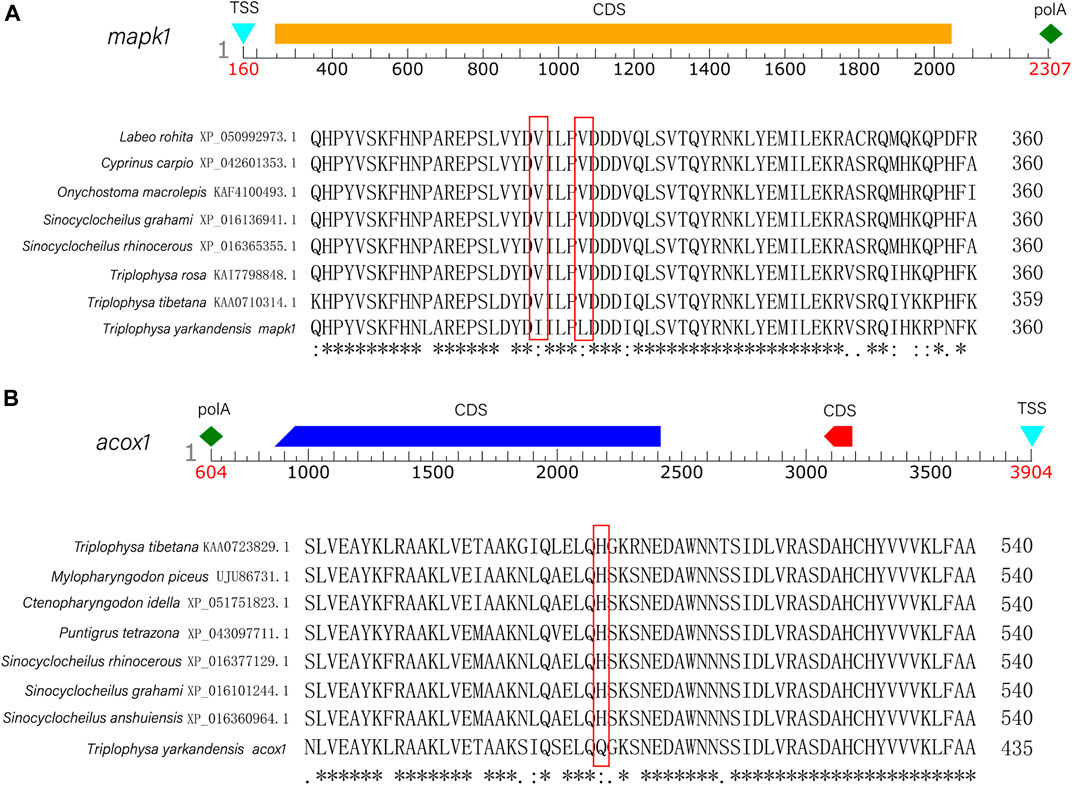
FIGURE 4. (A) The predicted sequence structure and a comparison of homologous the amino acid sequences with seven other fish (Triplophysa rosa, Triplophysa tibetana, Sinocyclocheilus grahami, Sinocyclocheilus rhinocerous, Cyprinus carpio, Labeo rohita, Onychostoma macrolepis) of mapk1 gene. (B) The predicted sequence structure and a comparison of homologous the amino acid sequences with seven other fish (Triplophysa tibetana, Mylopharyngodon piceus, Ctenopharyngodon idella, Puntigrus tetrazona, Sinocyclocheilus rhinocerous, Sinocyclocheilus grahami, Sinocyclocheilus anshuiensis) of acox1 gene.
Candidate genes involved with low temperature adaptation
In this study, six genes involved in fatty acid metabolism pathway, elovl4, acaca, fasn, acaa2, acox1, acox3, were selected to analyze the expression differences of six genes among liver and other tissues of T. yarkandensis. The results demonstrated that the expression of elovl4, acaca, fasn, acox1 and acox3 in liver were higher than that in brain, heart, kidney and spleen. The expression of acaa2 gene in liver were higher than that in brain, heart and spleen. The expression of six genes in fatty acid metabolism pathway differed among different tissues (Table 2). These genes involved in fatty acid metabolism pathway were shown in Figure 5. The expression of six genes in liver were higher than that in other tissues, indicating that T. yarkandensis had relatively fast fatty acid metabolism, which might be related to its low temperature adaptation. Finally, acox1 gene for bioinformatics analysis were selected, and the result showed that the sequence length was 5,277 bp, and there were two coding regions of the predicted gene structure. Besides, divergent amino acid changes (His-Gln at 402) by comparing amino acid sequences with seven other fish were found (Figure 4B).
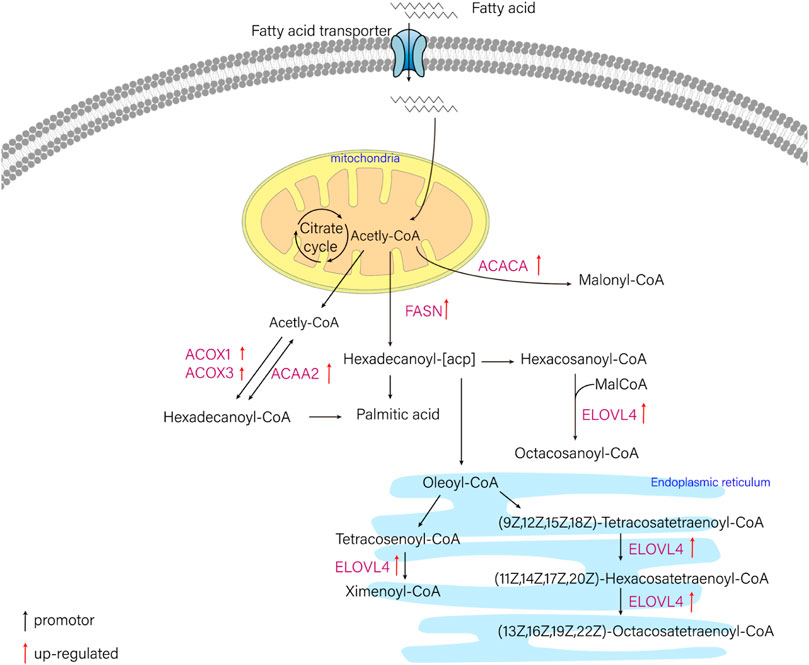
FIGURE 5. The schematic diagram of fatty acid metabolism pathway. ELOVL4 (elongation of very long chain fatty acids protein 4), ACACA (acetyl-CoA carboxylase/biotin carboxylase 1), FASN (fatty acid synthase, animal type), ACAA2 (acetyl-CoA acyltransferase 2), ACOX1 (acyl-CoA oxidase 1), ACOX3 (acyl-CoA oxidase 3).
Discussion
Transcriptomics is a laboratory technique that explores gene transcription and its regulation in specific cells or tissues at the RNA level (Wang et al., 2009). Transcriptome analysis might be able to obtain almost all transcriptional information of a specific tissue or organ. With the development of modern molecular biology and instruments, RNA-Seq technology developed rapidly and has become an indispensable tool for analyzing differential gene expression at the transcriptome level. In recent years, it has been extensively used in fish research (Wang et al., 2009; Palstra et al., 2012; Petzold et al., 2013; Min et al., 2018). The current study attained 102.11 Gb transcriptome clean data and 100,112 unigenes by sequencing, assembling and annotating transcripts. In addition, several pathways were associated with signaling molecules and interaction, signal transduction in the top 20 signaling pathways by KEGG enrichment pathway analysis.
The expression of transcriptome usually varies in different species, tissues and organs. For instance, analyzing the gill and liver transcriptome of Oryzias melastigma, the sensitivity of gill and liver to salinity hypotonic was strikingly different (Pl et al., 2021). In this study, the gene expression and differentially expressed genes in tissues were analysised. The GO annotation of differentially expressed genes in five tissues had resemblance in molecular function, cell components and biological processes. In addition, the KEGG pathway enrichment analysis showed that the DEGs in five tissues is noticeably enriched including glycerolipid metabolism, MAPK signaling pathway, calcium signaling pathway, adrenergic signaling in cardiomyocytes and neuroaction ligand-receptor interaction. Ultimately, both liver and kidney were associated with metabolism as indicated by the enrichment of these pathways. Furthermore, cells in the brain were involved in regulation and signal transduction, while the heart had the most cardiomyocytes, which were bound up with cell adhesion, tight junction and cardiomyopathy. In addition, the spleen was a lymphocyte with hematopoietic and immune functions (Huth and Place, 2013; Li et al., 2015; Wang et al., 2022e; Huang et al., 2022).
In the previous researches, mapk1 gene was an important gene in cell signal transduction process, and under the condition of high saline alkali concentration, the expression of mapk1 gene in T. yarkandensis would increase remarkably (Chen et al., 2020). Abcc1 gene was involved in the key regulatory processes of salinity and alkalinity adaptation, including ion transport and acid-base balance (Wang et al., 2022a). In the transcriptome study of Luciobarbus capito, gpx1, gpx4 and cat genes participated in the regulation process of salinity-alkalinity adaptation in liver and kidney (Zhang, 2020). The role of aqp1 gene in osmotic pressure regulation was closely related to salinity (Li et al., 2016). In the current study, mapk1, abcc1, cat, gpx1, gpx4 and aqp1 candidate genes involved in salinity-alkalinity adaptation were identified and in five tissues were differentially expressed in T. yarkandensis. Previous studies have found that the metabolism of unsaturated fatty acids in fish was sensitive in cold. Fatty acid metabolism was important for fish to survive at low temperatures, such as Larimichthy crocea, Cyprinus carpio, Chanos, Ctenopharyngodon Idella (Farkas et al., 1980; Hsieh et al., 2003; Hsieh and Kuo, 2005; Qian and Xue, 2016). Tissue distribution of transcription for 29 lipid metabolism-related genes in Takifugu rubripes demonstrated that in fatty acid β-oxidation, acox1 and acox3 gene expression levels were higher in liver than in other tissues (Xu et al., 2020). The expression levels of acaa2 gene, related to fatty acid metabolism, would change when affected by certain environment (Yang et al., 2020). Elovl4 gene in fish was significant in fatty acid synthesis and low-temperature stress adaptation (Liu et al., 2022), while acaca gene and fasn gene in liver were related to cold stress resistance in zebrafish (Wang et al., 2022b). In the current research, the expression levels of elovl4, acaca, fasn, acaa2, acox1 and acox3 genes in liver were higher. This not only showed that fatty acid metabolism of T. yarkandensis was relatively fast, but also indicated that the stability and fluidity were maintained by cell membranes, which might be related to its adaptation to low temperature environment. This study also provided a molecular basis for exploring salinity-alkalinity and low temperature adaptation of T. yarkandensis.
Conclusion
Overall, the sequencing results of this study add new information on the gene sequence data of T. yarkandensis. In fact, data of the gene expression and differential expression genes analysis among five tissues were obtained. Furthermore, our findings indicated that candidate genes respectively involved in the salinity-alkalinity and low temperature adaptation were differentially expressed in diffierent tissues of T. yarkandensis, including mapk1, abcc1, cat, gpx1, gpx4, aqp1, elovl4, acaca, fasn, acaa2, acox1 and acox3. Our study also showed that these genes were significant in salinity-alkalinity and low temperature adaptation. These transcriptome data can also be conducive to comprehensively study the population adaptive evolution and genetic diversity of Triplophysa family, as well as the development, protection and utilization of T. yarkandensis.
Data availability statement
The datasets presented in this study can be found in online repositories. The names of the repository/repositories and accession number(s) can be found below: https://www.ncbi.nlm.nih.gov/bioproject/PRJNA894312.
Author contributions
YW and FX conceived and designed the research. TW collected fish samples. ZX, DZ, and LH did experiments. ZX performed the computational analyses and wrote the manuscript. YW revised the manuscript. We are grateful for Sara Platto for English.
Funding
This research was funded by the grant from the National Natural Science Foundation of China (31702016) to YW.
Conflict of interest
The authors declare that the research was conducted in the absence of any commercial or financial relationships that could be construed as a potential conflict of interest.
Publisher’s note
All claims expressed in this article are solely those of the authors and do not necessarily represent those of their affiliated organizations, or those of the publisher, the editors and the reviewers. Any product that may be evaluated in this article, or claim that may be made by its manufacturer, is not guaranteed or endorsed by the publisher.
Supplementary material
The Supplementary Material for this article can be found online at: https://www.frontiersin.org/articles/10.3389/fgene.2022.1089274/full#supplementary-material
References
Altschul, S. F., Madden, T. L., Schaffer, A. A., Zhang, J., Zhang, Z., Miller, W., et al. (1997). Gapped blast and psi-blast: A new generation of protein database search programs. Nucleic Acids Res. 25 (17), 3389–3402. doi:10.1093/nar/25.17.3389
Amos, B., Rolf, A., and Bairoch, A. (2000). The SWISS-PROT protein sequence database and its supplement TrEMBL in 2000. Nucleic Acids Res. 28 (1), 45–48. doi:10.1093/nar/28.1.45
Amparyup, P., Charoensapsri, W., Samaluka, N., Chumtong, P., and Imjongjirak, C. (2020). Transcriptome analysis identifies immune-related genes and antimicrobial peptides in Siamese fighting fish (Betta splendens). Fish. Shellfish Immunol. 99, 403–413. doi:10.1016/j.fsi.2020.02.030
Ashburner, M., Ball, C. A., Blake, J. A., Botstein, D., Butler, H., Cherry, J. M., et al. (2000). Gene Ontology: Tool for the unification of biology. The gene Ontology consortium. Nat. Genet. 25, 25–29. doi:10.1038/75556
Chang, Y. M., Tang, R., Dou, X. J., Tao, R., Sun, X. W., and Liang, L. Q. (2014). Transcriptome and expression profiling analysis of Leuciscus waleckii: An exploration of the alkali-adapted mechanisms of a freshwater teleost. Mol. Biol. 10 (3), 491–504. doi:10.1039/c3mb70318e
Chen, S. A., Chun-Hui, M. A., Ding, H. P., Zhou, X. J., and Xie, C. X. (2013). The reproductive biology of Triplophysa (Hedinichthys) yarkandensis (Day) in Tarim river. Acta Hydrobiol. Sin. 37 (5), 810–816. doi:10.7541/2013.103
Chen, S. A., Hou, J., Yao, N., Xie, C., and Li, D. (2020). Comparative transcriptome analysis of Triplophysa yarkandensis in response to salinity and alkalinity stress. Comp. Biochem. Physiol. Part D. Genomics Proteomics 33, 100629–100639. doi:10.1016/j.cbd.2019.100629
Chen, S. A., Wang, Z. C., Song, Y., Cheng, Y., and Lei, M. H. (2010). Ecological research about Triplophysa (hedinichthgs) yarkandensis (day) in Tarim river. J. Northeast Agric. Univ. 41 (4), 90–94. doi:10.19720/j.cnki.issn.1005-9369.2010.04.019
Chen, S. A., Xie, C. X., Wang, Z. C., Chen, Y., and Sun, H. X. (2011). Population characteristics of Triplophysa (hedinichthys) yarkandensis (day) in Tarim river of alar section. J. Hydroecol 32 (2), 143–145. doi:10.15928/j.1674-3075.2011.02.026
Chen, S. A., and Yao, N. (2008). Research on biological characteristic of the Triplophysa (hedinichthys) yarkandensis (day) in Tarim river. J. Hydroecol 29 (5), 100–102. doi:10.15928/j.1674-3075.2008.05.033
Chen, S., Xie, C., Li, D., Yao, N., Ding, H., and Zhang, Z. (2017). Length-weight relationships of five Triplophysa species from the northwest of China. J. Appl. Ichthyol. 33 (6), 1234–1236. doi:10.1111/jai.13432
Chen, S., Ya, N., Xie, C., Wang, S., and Ren, D. (2016). Complete mitochondrial genome of the Triplophysa (Hedinichthys) yarkandensis (Day). Mitochondrial DNA B Resour. 1 (1), 235–236. doi:10.1080/23802359.2016.1156489
Deng, Y., Jianqi, L. I., Songfeng, W. U., Zhu, Y., Chen, Y., and Fuchu, H. E. (2006). Integrated nr database in protein annotation system and its localization. Comput. Eng. 32 (5), 71–72. doi:10.1109/INFOCOM.2006.241
Dewey, C. N., and Li, B. (2011). RSEM: Accurate transcript quantification from RNA-seq data with or without a reference genome. BMC Bioinforma. 12 (1), 323. doi:10.1186/1471-2105-12-323
Farkas, T., Csengeri, I., Majoros, F., and Oláh, J. (1980). Metabolism of fatty acids in fish: III. Combined effect of environmental temperature and diet on formation and deposition of fatty acids in the carp, Cyprinus carpio linnaeus 1758. Aquaculture 20 (1), 29–40. doi:10.1016/0044-8486(80)90059-9
Finn, R. D., Alex, B., Jody, C., Penelope, C., Eberhardt, R. Y., Eddy, S. R., et al. (2014). Pfam: The protein families database. Nucleic Acids Res. 42 (D1), D222–D230. doi:10.1093/nar/gkt1223
Grabherr, M. G., Haas, B. J., Yassour, M., Levin, J. Z., Thompson, D. A., Amit, I., et al. (2011). Full-length transcriptome assembly from RNA-Seq data without a reference genome. Nat. Biotechnol. 29 (7), 644–652. doi:10.1038/nbt.1883
Guan, W. Z., Qiu, G. F., and Liu, F (2020). Transcriptome analysis of the growth performance of hybrid Mandarin fish after food conversion. PLoS ONE 15 (10), e0240308. doi:10.1371/journal.pone.0240308
Guo, X., Feng, Q., Liu, W., Li, Z., Wen, X., Si, J., et al. (2015). Stable isotopic and geochemical identification of groundwater evolution and recharge sources in the arid Shule River Basin of Northwestern China. Hydrol. Process 29 (22), 4703–4718. doi:10.1002/hyp.10495
Hsieh, S. L., Chen, Y. N., and Kuo, C. M. (2003). Physiological responses, desaturase activity, and fatty acid composition in milkfish (Chanos chanos) under cold acclimation. Aquaculture 220 (1-4), 903–918. doi:10.1016/S0044-8486(02)00579-3
Hsieh, S. L., and Kuo, C. M. (2005). Stearoyl–CoA desaturase expression and fatty acid composition in milkfish (Chanos chanos) and grass carp (Ctenopharyngodon idella) during cold acclimation. Com. Biochem. Physiol. B Biochem. Mol. Biol. 141 (1), 95–101. doi:10.1016/j.cbpc.2005.02.001
Hu, J. R., Yuan, X. C., Huang, W., Li, G. L., Zhang, Y. F., Wang, G. X., et al. (2021). Analysis of different selenium sources on regulation of signal pathways and key genes of Pelteobrus fulvidraco under low temperature stress based on transcriptome. Chin. J. Anim. Nutr. 33 (8), 4662–4674. doi:10.3969/j.issn.1006267x.2021.08.045
Huang, Z., Guo, X., Wang, Q., Ma, A., Zhao, T., Qiao, X., et al. (2022). Digital RNA-seq analysis of the cardiac transcriptome response to thermal stress in turbot Scophthalmus maximus. J. Therm. Biol. 104, 103141. doi:10.1016/j.jtherbio.2021.103141
Huth, T. J., and Place, S. P. (2013). De novo assembly and characterization of tissue specific transcriptomes in the emerald notothen, Trematomus bernacchii. BMC Genomics 14, 805. doi:10.1186/1471-2164-14-805
Jaime, H. C., Damian, S., Kristoffer, F., Helen, C., Davide, H., Walter, M. C., et al. (2016). eggNOG 4.5: a hierarchical orthology framework with improved functional annotations for eukaryotic, prokaryotic and viral sequences. Nucleic Acids Res. 44 (D1), D286–D293. doi:10.1093/nar/gkv1248
Koonin, E. V., Fedorova, N. D., Jackson, J. D., Ar, J., Krylov, D. M., Makarova, K. S., et al. (2004). A comprehensive evolutionary classification of proteins encoded in complete eukaryotic genomes. Genome Biol. 5 (2), R7. doi:10.1186/gb-2004-5-2-r7
Langmead, B., Trapnell, C., Pop, M., and Salzberg, S. L. (2009). Ultrafast and memory-efficient alignment of short DNA sequences to the human genome. Genome Biol. 10 (3), R25. doi:10.1186/gb-2009-10-3-r25
Li, A., and Godzik, A (2006). Cd-Hit: A fast program for clustering and comparing large sets of protein or nucleotide sequences. Bioinformatics 22 (13), 1658–1659. doi:10.1093/bioinformatics/btl158
Li, G. X., Zhao, Y. L., Liu, Z. H., Gao, C. S., Yan, F. B., Liu, B. Z., et al. (2015). De novo assembly and characterization of the spleen transcriptome of common carp (Cyprinus carpio) using Illumina paired-end sequencing. Fish. Shellfish Immunol. 44 (2), 420–429. doi:10.1016/j.fsi.2015.03.014
Li, Q., Zhu, H., Lu, M., Liu, Z., Gao, F., and Ke, X. (2016). Effects of salt stress on aquaporin 1(AQP1) gene expression in Tilapia Oreochromis mossambicus. Genomics Appl. Biol. 35 (11), 2996–3006. doi:10.13417/j.gab.035.002996
Liu, S. J., Li, X. H., Qi, D. L., Qi, H. F., Zhao, K., and Tian, F. (2022). Genome-wide characterization of the Elovl gene family in Gymnocypris przewalskii and their potential roles in adaptation to cold temperature. Com. Biochem. Physiol. B Biochem. Mol. Biol. 262, 110759. doi:10.1016/j.cbpb.2022.110759
Liu, Z., Ma, A., Yuan, C., Zhao, T., and Zhang, J. (2020). Transcriptome analysis of liver lipid metabolism disorders of the turbot Scophthalmus maximus in response to low salinity stress. Aquaculture 534, 736273. doi:10.1016/j.aquaculture.2020.736273
Love, M. I., Huber, W., and Anders, S. (2014). Moderated estimation of fold change and dispersion for RNA-seq data with DESeq2. Genome Biol. 15 (12), 550–570. doi:10.1186/s13059-014-0550-8
Min, Z., Lu, J., and Zhao, D. (2018). Toxicity and transcriptome sequencing (RNA-seq) analyses of adult zebrafish in response to exposure carboxymethyl cellulose stabilized iron sulfide nanoparticles. Sci. Rep. 8 (1), 8083–8093. doi:10.1038/s41598-018-26499-x
Minoru, K., Susumu, G., Shuichi, K., Yasushi, O., and Masahiro, H. (2004). The KEGG resource for deciphering the genome. Nucleic Acids Res. 32, D277–D280. doi:10.1093/nar/gkh063
Palstra, A. P., Beltran, S., Burgerhout, E., Brittijn, S. A., Planas, J. V., Henkel, C. V., et al. (2012). Deep RNA sequencing of the skeletal muscle transcriptome in swimming fish. PLoS ONE 8 (1), e53171. doi:10.1371/journal.pone.0053171
Petzold, A., Reichwald, K., Groth, M., Taudien, S., Hartmann, N., Priebe, S., et al. (2013). The transcript catalogue of the short-lived fish Nothobranchius furzeri provides insights into age-dependent changes of mRNA levels. BMC Genomics 14 (1), 185. doi:10.1186/1471-2164-14-185
Pl, A., Hsas, B., Zl, A., Rz, A., Yq, A., Yx, A., et al. (2021). RNA-seq analyses of Marine Medaka (Oryzias melastigma) reveals salinity responsive transcriptomes in the gills and livers. Aquat. Toxicol. 240, 105970. doi:10.1016/j.aquatox.2021.105970
Qian, B. Y., and Xue, L. Y. (2016). Liver transcriptome sequencing and de novo annotation of the large yellow croaker (Larimichthy crocea) under heat and cold stress. Mar. Genomics 25, 95–102. doi:10.1016/j.margen.2015.12.001
Rolf, A., Amos, B., Wu, C. H., Barker, W. C., Brigitte, B., Serenella, F., et al. (2004). UniProt: The universal protein knowledgebase. Nucleic Acids Res. 32, D115–D119. doi:10.1093/nar/gkh131
Tatusov, R. L., Galperin, M. Y., Natale, D. A., and Koonin, E. V. (2000). The COG database: A tool for genome-scale analysis of protein functions and evolution. Nucleic Acids Res. 28 (1), 33–36. doi:10.1093/nar/28.1.33
Trapnell, C., Williams, B. A., Pertea, G., Mortazavi, A., Kwan, G., Baren, M. J. V., et al. (2010). Transcript assembly and quantification by RNA-Seq reveals unannotated transcripts and isoform switching during cell differentiation. Nat. Biotechnol. 28, 511–515. doi:10.1038/nbt.1621
Wang, J. X., Song, Y., Wang, X. Y., Chen, S. A., and Ren, D. Q. (2020). Microsatellite screening and characterization analysis of Triplophysa Yarkandensis based on the whole genome de novo sequencing. J. Tarim Univ. 32 (02), 25–32. doi:10.3969/j.issn.1009-0568.2020.02.004
Wang, S. Y., Liang, L. Q., Chang, Y. M., and Sun, B. (2022a). Mining and analysis of InDels in response to alkali-saline stress in Amur ide (Leuciscus waleckii). J. Fish. Sci. China 29 (2), 184–199. doi:10.12264/JFSC2021-0251
Wang, X., Chen, D., Lv, Z., Zhao, X., Ding, C., Liu, Y., et al. (2022b). Transcriptomics analysis provides new insights into the fish antiviral mechanism and identification of interferon-stimulated genes in grass carp (Ctenopharyngodon idella). Mol. Immunol. 148, 81–90. doi:10.1016/j.molimm.2022.05.120
Wang, X. Y., Chen, S. A., Wang, C. X., Zi, F. Z., Chang, D. S., Xu, H., et al. (2022c). Otolith morphology and population discrimination of Triplophysa yarkandensis. Prog. Fish. Sci. 43. doi:10.19663/j.issn2095-9869.20220228002
Wang, Y. F., Zhao, C. Z., Zeng, H. X., Kang, M. P., Zhao, T. T., and Tang, Y. R. (2022d). Spatial-temporal evolution of wetland landscape patterns and its influencing factors in the middle reaches of the Shule River. Arid Zone Res. 39 (1), 282–291. doi:10.13866/j.azr.2022.01.27
Wang, Y. M., Sun, S. X., He, J. X., Yang, G., and Gao, J. (2022e). Effects of elovl8 deletion on survival and lipid metabolism under cold stress of zebrafish. Acta Hydrobiol. Sin. 46 (3), 1–13. doi:10.7541/2022.2021.063
Wang, Y., Yang, L. D., Wu, B., Song, Z. B., and He, S. P. (2015). Transcriptome analysis of the plateau fish (Triplophysa dalaica): Implications for adaptation to hypoxia in fishes. Gene 565 (2), 211–220. doi:10.1016/j.gene.2015.04.023
Wang, Z., Gerstein, M., and Snyder, M. (2009). RNA-seq: A revolutionary tool for transcriptomics. Nat. Rev. Genet. 10 (1), 57–63. doi:10.1038/nrg2484
Xiang, W., and Fan, Z. M. (2014). Studies of Triplophysa(Hedinichthgs)yarkandensis(Day)on the oxygenconsumption rate and suffocation point. J. Aquac. 35 (4), 46–48. doi:10.3969/j.issn.1004-2091.2014.04.010
Xie, J. H., Sun, Y., Cao, Y., Han, L. H., Li, Y. X., Ding, B. H., et al. (2022). Transcriptomic and metabolomic analyses provide insights into the growth and development advantages of triploid Apostichopus japonicus. Mar. Biotechnol. 24 (1), 151–162. doi:10.1007/s10126-022-10093-4
Xu, H. G., Meng, X. X., Jia, L. L., Wei, Y. L., Sun, B., and Liang, M. Q. (2020). Tissue distribution of transcription for 29 lipid metabolism-related genes in Takifugu rubripes, a marine teleost storing lipid predominantly in liver. Fish. Physiol. Biochem. 46 (4), 1603–1619. doi:10.1007/s10695-020-00815-7
Yang, Q., Han, T. D., Li, X. Y., Qin, J., Cheng, P., and Pu, H. Z. (2021). Hydrochemical characteristics and controlling factors in the source region of Shule River. J. Glaciol. Geocryol. 43 (2), 568–579. doi:10.7522/j.issn.1000-0240.2021.0034
Yang, T. Y., Jiang, Y. L., Han, Z. Q., and Meng, W. (2020). Comparative transcriptome analysis of different tissues in burbot (Lota lota) based on RNA-seq. J. Agric. Biotechnol. 28 (02), 291–301. doi:10.3969/j.issn.1674-7968.2020.02.011
Yang, Y. J., Wang, W. X., Liu, X. L., Song, X. L., and Chai, L. H. (2020). Probing the effects of hexavalent chromium exposure on histology and fatty acid metabolism in liver of Bufo gargarizans tadpoles. Chemosphere 243, 125437. doi:10.1016/j.chemosphere.2019.125437
Yao, N., Song, Y., Wang, S., Chen, S. A., Adak, , Liu, J. Y., et al. (2018). Toxicity of salinity and alkalinity to Triplophysa (hedinichthys) yarkandensis (day) of Tarim river. Southwest China j.agric.sci. 31 (2), 423–428. doi:10.16213/j.cnki.scjas.2018.2.034
Yuan, D. Y. (2021). The genetic basis of high-elevation adaption andthe cold tolerance mechanism of Triplophysa bleekeri. Chongqing, China: Southwest University. [dissertation]. doi:10.27684/d.cnki.gxndx.2021.000013
Zhao, W. H., Yi, S. K., Su, J. X., Zhou, Q., Shen, J. Z., Li, D. P., et al. (2022). Genetic diversity of Triplophysa Yarkandensis populations in Tarim River Basin in Xinjiang. Acta. Hydrobiol. Sin. 46 (3), 364–374. doi:10.7541/2022.2021-006
Zhang, Y. T. (2020). Study of immunological reaction of Luciobarbus capito under saline-alkali stress based on transcriptome sequencing technology. Shanghai, China: Shanghai Ocean University. [dissertation]. doi:10.27314/d.cnki.gsscu.2020.000593
Keywords: T. yarkandensis, transcriptome, gene annotation, salinity-alkalinity adaptation, low temperature adaptation
Citation: Zhang X, Wang T, Zhai D, Liu H, Xiong F and Wang Y (2023) Transcriptome analysis and gene expression analysis related to salinity-alkalinity and low temperature adaptation of Triplophysa yarkandensis. Front. Genet. 13:1089274. doi: 10.3389/fgene.2022.1089274
Received: 04 November 2022; Accepted: 28 December 2022;
Published: 12 January 2023.
Edited by:
Chao Tong, University of Pennsylvania, United StatesReviewed by:
Hongtuo Fu, Chinese Academy of Fishery Sciences, ChinaBaocheng Guo, Institute of Zoology (CAS), China
Xue Kong, Jiangsu Ocean University, China
Copyright © 2023 Zhang, Wang, Zhai, Liu, Xiong and Wang. This is an open-access article distributed under the terms of the Creative Commons Attribution License (CC BY). The use, distribution or reproduction in other forums is permitted, provided the original author(s) and the copyright owner(s) are credited and that the original publication in this journal is cited, in accordance with accepted academic practice. No use, distribution or reproduction is permitted which does not comply with these terms.
*Correspondence: Fei Xiong, xiongfei@jhun.edu.cn; Ying Wang, xinyuanwangying@163.com