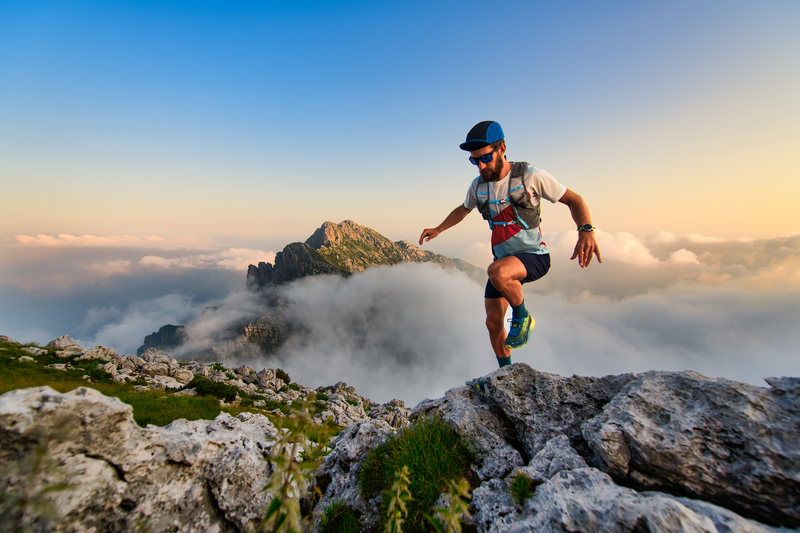
95% of researchers rate our articles as excellent or good
Learn more about the work of our research integrity team to safeguard the quality of each article we publish.
Find out more
ORIGINAL RESEARCH article
Front. Genet. , 16 November 2022
Sec. Cancer Genetics and Oncogenomics
Volume 13 - 2022 | https://doi.org/10.3389/fgene.2022.1056224
Prostate cancer (PCa) is the most common malignancy. New biomarkers are in demand to facilitate the management. The role of the pinin protein (encoded by PNN gene) in PCa has not been thoroughly explored yet. Using The Cancer Genome Atlas (TCGA-PCa) dataset validated with Gene Expression Omnibus (GEO) and protein expression data retrieved from the Human Protein Atlas, the prognostic and diagnostic values of PNN were studied. Highly co-expressed genes with PNN (HCEG) were constructed for pathway enrichment analysis and drug prediction. A prognostic signature based on methylation status using HCEG was constructed. Gene set enrichment analysis (GSEA) and the TISIDB database were utilised to analyse the associations between PNN and tumour-infiltrating immune cells. The upregulated PNN expression in PCa at both transcription and protein levels suggests its potential as an independent prognostic factor of PCa. Analyses of the PNN’s co-expression network indicated that PNN plays a role in RNA splicing and spliceosomes. The prognostic methylation signature demonstrated good performance for progression-free survival. Finally, our results showed that the PNN gene was involved in splicing-related pathways in PCa and identified as a potential biomarker for PCa.
Prostate Cancer (PCa) is the third most common cancer overall (Pan et al., 2017) and the most common malignant tumour in the male genitourinary system (Ren et al., 2017; Caggiano et al., 2019; Jambor et al., 2019). Its prevalence and mortality vary greatly depending on race and geographic location (Lindberg et al., 2013). At present, PCa is usually screened and diagnosed through digital rectal examination (DRE), prostate-specific antigen (PSA) value, Gleason score by prostate biopsy, and magnetic resonance imaging (MRI) of the prostate (Patil and Gaitonde, 2016). New biomarkers used with techniques such as liquid biopsy and imaging have also been used for clinical diagnosis (Kim et al., 2016; Li et al., 2018; Law et al., 2020). In fact, metastatic PCa remains incurable despite promising advances in biomedical research. Therefore, patients’ good prognosis is currently dependent on early detection. Conventional non-surgical options for PCa therapy include androgen deprivation therapy (ADT), radiotherapy (RT), ablation therapy, chemotherapy, and emerging immunotherapy. However, the effectiveness of the drugs including abiraterone and enzalutamide, are limited and temporary, but has been established clinically.
New biomarkers for diagnosis and treatment need to explore the mechanism deeply. In the past two decades, several mechanisms of PCa have been continuously reported, including novel associations of androgen signalling (Caggiano et al., 2019; Cioni et al., 2020), TP53 signalling (Ecke et al., 2010; Liu et al., 2021), and the Wnt signalling pathway (Murillo-Garzón et al., 2018; Datta et al., 2020) with the disease. In fact, it is now believed that various cytokines and intercellular signals regulate PCa during its development (Cucchiara et al., 2017). Thus, many potential mechanisms of PCa remain to be explored, which may lead to new diagnostic techniques or therapeutic strategies, especially for metastatic PCa.
The pinin protein, reported as a desmosome-associated protein encoded by the PNN gene, is a phosphoprotein rich in serine and arginine with a molecular size of 140 kDa. Recently, it has been suggested that pinin is associated with cell adhesion (Tang et al., 2020; Yao and Ma, 2020). It serves as a putative tumour promoter by reversing the expression of E-cadherin (Simon et al., 2015). The upregulation of pininhas been reported to enhance metastasis in colorectal cancer (Wei et al., 2016), triple-negative breast cancer cells (Kang et al., 2020), pancreatic cancer (Yao and Ma, 2020), and nasopharyngeal carcinoma cells (Tang et al., 2020). As an oncogenic factor, PNN can protect hepatocellular carcinoma cells from apoptosis (Yang et al., 2016) and promote cell adhesion in ovarian cancer (Zhang et al., 2016), as well as renal cell carcinoma (Jin et al., 2021). These studies indicate the critical role of PNN in metastasis; thus, it could be a potential biomarker for some tumours. However, the role of pininin PCa progression has not been thoroughly studied yet. Since the tumour microenvironment (TME) has emerged as a critical factor in metastasis (Yin et al., 2019; Yuan et al., 2022), there may also be a functional linkage between TME and PNN in PCa, but this hypothesis remains to be investigated.
Since the PNN gene has not been comprehensively deciphered in PCa, we conducted a series of studies on its roles in patients’ survival and prognosis, as well as in immune infiltration in PCa through various bioinformatic approaches. We explored the expression pattern of the PNN gene and its potential prognostic value for PCa. We also investigated the relationship between PNN and the tumour immune microenvironment (TIME), which could facilitate understanding the mechanism of immunotherapy for PCa and lead to the discovery of a prognosis signature or novel therapeutic targets.
To illustrate the function of PNN in PCa, we conducted a comprehensive bioinformatic analysis using multiple datasets. The whole analysis pipeline performed here is displayed in Figure 1.
The transcriptome data [the level 3 mRNA expression data (FPKM), normalized using
The Human Protein Atlas (HPA) provides the protein expression of pinin in normal prostate (via https://www.proteinatlas.org/ENSG00000100941-PNN/tissue/prostate) and tumour tissues (via https://www.proteinatlas.org/ENSG00000100941-PNN/pathology/prostate+cancer) (Uhlén et al., 2015). All images of tissues in HPA database are stained by immunohistochemistry. We extracted the immunohistochemistry images directly from the HPA database.
Correlation analysis of PNN expression and clinicopathological characteristics was performed. The expression of PNN between the subgroups was compared based on the following clinicopathological features: age (<60 or ≥60 years old), N stage (N0, N1), M stage (M0, M1), T stage (T2, T3, T4), surgical margin (R0, R1, R2, RX), prostate-specific antigen (PSA) level (<10 or ≥10 years), and Gleason score (6, 7, 8, 9, 10). Univariate and multivariate Cox regression analyses were implemented to identify independent predictors of survival in the TCGA-PRAD and GSE116918 datasets.
We downloaded GSE38241 (Aryee et al., 2013) and GSE25136 (Sun and Goodison, 2009) datasets (the authors processed normalisation) from GEO. For the merging of these datasets, we used the method of COMBAT (Johnson et al., 2007), implemented in the R package inSilicoMerging (Taminau et al., 2012) to obtain the expression matrix. Finally, the PNN expression was compared using the Kruskal-Wallis test.
We calculated the Pearson correlation of all genes (RNA-seq) in the TCGA dataset with PNN using the Linkomics database (http://www.linkedomics.org/) and selected the genes with correlation coefficients > 0.8 and p < 0.05 as PNN co-expressed genes.
The “clusterProfiler” R package was utilised to conduct Gene Ontology (GO) and Kyoto Encyclopedia of Genes and Genomes (KEGG) analysis (Yu et al., 2012). GO enrichment analysis mainly described the biological processes (BP), cellular components (CC), and molecular functions (MF) correlated with genes. The threshold for significant enrichment was set as a p-value < 0.05 or FDR < 0.05, as stated. Single sample gene set enrichment analysis (ssGSEA) enrichment scores were calculated in each sample using the “GSVA” package of R (Hänzelmann et al., 2013).
In this research, potential drug (or molecules) was predicted using the Drug Signatures database (DSigDB) via Enrichr (https://maayanlab.cloud/Enrichr/) based on the PNN gene as well as the positively co-expressed gene with PNN (correlation coefficient > 0.8 and p < 0.05) (Chen et al., 2013; Kuleshov et al., 2016; Xie et al., 2021).
The CpG sites in the promoter of PNN and PNN’s co-expressed genes were obtained from the MEXPRESS database (Koch et al., 2015; Koch et al., 2019). A univariate Cox analysis in R was used to determine the association between methylation levels at each CpG site and progression-free survival (PFS) for each patient, and p < 0.01 was considered statistically significant. Candidate prognostic CpG sites were selected using the Least Absolute Shrinkage and Selection Operator (LASSO) algorithm. Based on the candidate CpG sites generated from the above algorithm, a multivariate Cox regression model was used to construct a prognostic signature. The RiskScore of each recipient was calculated using the following formula:
In which
Patients were divided into the high-risk (
To inspect the different signalling pathways between the PNN low- and high-expression groups in the TCGA-PRAD dataset, Gene Set Enrichment Analysis (GSEA) was conducted by the “clusterProfiler” package in R software (Subramanian et al., 2005). Pathways with a p-value < 0.05 were considered significantly enriched.
The Tumor and Immune System Interaction Database (TISIDB) (http://cis.hku.hk/TISIDB) database was utilised to analyse the associations between PNN and tumour-infiltrating lymphocytes (TIL), immunosuppressors, and chemokines (Ru et al., 2019).
Statistical analysis was performed using the R software package (version 3.6.1). The differential mRNA expression of PNN between tumour tissues and normal controls was compared using Student’s t-test. The expression of PNN among the clinicopathological parameters groups was compared using Student’s t-test and ANOVA. The area under the curve (AUC) of receiver operating characteristic (ROC) was utilised to determine the diagnostic ability of PNN and was calculated using the “pROC” R package (Malone et al., 2015). KM curves of disease-free survival (DFS or PFS) of the patients were performed by setting the median expression of PNN as the cut-off in the ‘survival’ R package. The log-rank test was used to assess statistical differences, and a cut-off p-value < 0.05 was deemed statistically significant.
The expression levels of PNN between PCa and control samples were compared in the TCGA-PRAD, and the PNN expression level was validated with GSE29079 and GSE6956 datasets. As shown in the violin plots, the mRNA expression level of PNN was significantly higher in the PCa group in all datasets (Figures 2A–C). Next, we used the same datasets to evaluate the diagnostic value of the PNN gene. The accuracy of the diagnostic model was evaluated by ROC curve analysis (Figure 2D). As a result, the AUC of the PNN diagnostic model was greater than 0.7 in all three datasets, indicating that the PNN gene can be used to discriminate cancer from normal tissues. Moreover, we also observed that the abundance of pinin protein was higher in PCa tissue than in normal tissue (Figures 2E,F).
FIGURE 2. PNN expression profile and its diagnostic value in Prostate Cancer (PCa). (A–C) Comparison of PNN expression levels in the TCGA-PRAD, GSE29079, and GSE6956 datasets. (D) The diagnostic value of PNN as evaluated by ROC curve. (E,F) Immunohistochemistry results of normal (two cases) and PCa tissue (four cases) from the HPA database.
To explore the relationship between PNN expression and the clinicopathological characteristics in PCa, we compared the PNN expression levels according to sample clinical information. The high PNN expression was found in the advanced stage of PCa (Figure 3B), and the Gleason scores were strongly correlated with the PNN expression levels in PCa patients in both TCGA-PRAD datasets (p =
FIGURE 3. Comparison of PNN expression and clinical information in TCGA (A) Age, (B) N stage, (C) T stage, (D) Surgical margin, and (E) Gleason score. Comparison of PNN expression and clinical information of GSE116918 (F) Age, (G) PSA level, (H) T stage, and (I) Gleason score. The t-test was used to evaluate the difference between two groups, and analysis of variance (ANOVA) was used to compare data divided into more than two groups. (J) Comparision of the PNN gene expression between primary and metastatic PCa using GSE38241 and GSE25136 datasets following batch effects removal.
Univariate and multivariate Cox analyses were conducted to investigate the independent prognostic factors in TCGA-PRAD and validated with GSE116918 datasets. The univariate analysis in the TCGA-PRAD dataset indicated that the surgical margin, T stage, N stage, Gleason score, and PNN expression were associated with the prognosis of PCa patients (Figure 4A). In contrast, multivariate Cox regression analyses in the same dataset demonstrated that only the Gleason score could be used independently to predict the prognosis of patients (Figure 4B). Similarly, the PSA levels, Gleason score, T stage, and PNN expression were found to be significant risk factors by univariate Cox analysis in the GSE116918 dataset (Figure 4C). In the same dataset, multivariate Cox regression analyses demonstrated that T stage and PNN expression could be used independently to predict the prognosis of patients (Figure 4D). We then validated these findings by analysing the DFS curves of the PNN high- and low-expression groups, which showed that the PNN high-expression group had remarkably worse survival rates than the low-expression group in both the TCGA-PRAD and the GSE116918 datasets (Figures 4E,F). The hazard ratio of PNN was greater than 1 in both datasets. Taken together, it suggested that PNN was a risk factor in the prognosis of PCa. However, the independent prognostic value of PNN needed further investigation and confirmation.
FIGURE 4. PNN prognostic value in the TCGA-PRAD and the GSE116918 cohorts. Forest plots of univariate and multivariate Cox regression analysis for the TCGA cohort (A) univariate, (B) multivariate and the GSE116918 cohort (C) univariate, (D) multivariate. (E,F) DFS curves plotted according to the KM method for the TCGA-PRAD and GSE116918 cohorts using the log-rank test.
To identify pharmaceutical molecules with DsigDB database and further uncover the biological processes PNN participated, the co-expression pattern of PNN in PCa was explored. All co-expressed genes are listed in Supplementary Table S2.
BioGrid hosted 243 proteins interacting with pinin extracted from published literature. A total of 368 genes were co-expressed with pinin following the criteria of r > 0.6 and p < 0.05, of them, twenty-five genes overlapped with 243 interactive proteins of pinin (25UC for short). Those 25UC genes were enriched in RNA splicing and RNA/mRNA processing based on GO enriched analysis (Figure 5A) and enriched in the spliceosome, mRNA surveillance pathway, and RNA transport based on KEGG enrichment analysis (Figure 5B). These results suggest that PNN is mainly linked to the RNA process and RNA transport in PCa. PNISR, RBM39, DDX39B, SF3B1, SRSF11, CPSF6, CLK2, and SNRPB2 have the function of splicing or process of RNA; ACIN1 and NKTR participate in cell apoptosis and immune response. The protein-protein interaction network can be found in Figure 5C.
FIGURE 5. Co-expressed network with PNN. Enrichment results filtered with FDR < 0.05 based on 25 uniquely interacted and co-expressed genes with PNN with (A) GO and (B) KEGG. (C) Protein-Protein-interaction (PPI) network constructed using Cytoscape 3.8.2 based on PNN and 25UC.
To explore the potential therapeutic targets in PCa, we focused on those genes that strongly positively (r > 0.8 and p < 0.05) correlated with upregulated PNN, including FNBP4, TCERG1, RBM39, DDX39B and DMTF1. Ten possible pharmaceutical molecules were identified using the Enrichr package from the DsigDB database, based on their p-value. Table 1 lists the effective drugs from the DsigDB database for PCa.
After excluding missing values, a total of 180 CpG sites in the PNN and its co-expressed genes FNBP4, TCERG1, RBM39, DDX39B and DMTF1 (r > 0.8 and p < 0.05) promoter regions were extracted from TCGA-PRAD methylation data. Univariate Cox regression analysis showed that 25 CpG sites were significantly correlated with PFS. Following the LASSO algorithm, 16 CpG sites were selected (lambda = 0.009914324, Figures 6A,B). A model was then constructed with multivariate Cox regression. We constructed a risk score system Eq. 1 with seven CpG sites.
FIGURE 6. Analysis of DNA methylation levels concerning the PNN expression. (A) LASSO model tuning parameters (
The areas under the ROC curves (AUC) of 1-, 2-, and 3-year PFS were 0.80, 0.74 and 0.71, respectively (Figure 6C), indicating the good performance of the risk score signature. We noticed that this risk score was linked to the PFS status of the PCa patients (Figure 6D), indicating that this risk score could be used to predict the progression of PCa. Multivariate Cox regression confirmed that the risk scores could also be an independent prognostic factor (Figures 6E,F). In addition, the expression level of 47 immune checkpoint genes (ICG) proposed by Danilova et al. (2019) were compared between high and low-risk groups with the Wilcoxon test based on the signature constructed above. As a result, CTLA4, CD276, CD80, NRP1, TNFRSF18, TNFSF18 and TNFRSF14 were found to be significantly higher in the high-risk group, while the expression levels of BTNL2, CD160, CD200, CD244, CD274, CD40, CD44, LAG3, TMIGD2, TNFSF15, VSIR and VTCN1 were reduced significantly in the high-risk group (Figure 6G). Then, a ssGSEA was performed using the KEGG database to explore different molecular mechanisms between the high- and low-risk groups. Among significantly enriched pathways (p < 0.05), the top 10 were compared between high and low-risk groups. Between the two risk groups, the splicing factors genes, such as SF3B1, SRSF11 and SRSF7, are significantly higher in high risk group based on the median (Figure 6H). The expression of SRSF10 (p = 0.05) and SRSF4 (p = 0.07) were marginally higher. Moreover, the splicing isoforms expression was also significantly increased in the highly risky group based on the median. Besides prostate cancer and other cancer pathways, the risk model also found significant different enrichment scores in spliceosome and biogenesis and degradation pathways (Figure 6I). The correlation between the pathways and the PNN expression is illustrated in Figure 6J.
To infer the pathways by which PNN genes were involved in the development of PCa, GSEA enrichment was performed on the PNN high- and low-expression groups. Among the enriched pathways (adjusted p < 0.05) (Supplementary Table S1), we noted that immune-related pathways were enriched, including the IL-17 signalling pathway, the T cell receptor signalling pathway, Th1 and Th2 cell differentiation, Th17 cell differentiation, and the TNF signalling pathway. In addition, cancer-related pathways, such as the cell cycle, choline metabolism in cancer, PD-L1 expression and PD-1 checkpoint pathway in cancer, and proteoglycans in cancer were also enriched in PCa (Figure 7A). We calculated the expression difference between normal and tumour samples in each tumour, and observed significant upregulation in 14 tumours (Figure 7B). Subsequently, the correlation between PNN and immune infiltration was executed to broaden the cognition of the correlation between PNN and TIL, immune inhibitors, and chemokines in PCa. As to TIL, PNN expression was negatively correlated with iDC, monocyte, NK cell, and Tgd in Figure 7C (rho < −0.3 and p < 0.05). Figure 7D showed the correlations between PNN expression and chemokines, of which CCL14 was negatively correlated with PNN (r < −0.3 and p < 0.05).
FIGURE 7. Immune infiltration. (A) GSEA results are based on PNN high- and low-expression groups. (B) PNN expression profile in pan-cancers. Unpaired Wilcoxon Rank Sum and Signed Rank Tests for significance of differences analysis. Analysis of the correlation between PNN expression and (C) tumor-infiltrating lymphocytes (TIL) and (D) chemokines. The left figure shows the correlation between PNN expression in TCGA pan-cancer and TIL, immunosuppressants, and chemokines, and the Scatter plot (right) clearly shows correlations with PNN expression in PCa patients.
Prostate cancer remains one of the most common cancers, with a poor patient prognosis upon transition to metastasis (Phillips et al., 2020). It is urgent to continually identify proteins or hormones related to this disease for potential novel treatments or as potential biomarkers for early detection. It has been suggested that PNN promotes the epithelial-mesenchymal transition in tumours, which is the preliminary stage of metastasis (Vandamme et al., 2020; Dai et al., 2021; Zhang et al., 2022). The gene encodes a transcriptional activator binding to the E-box 1 core sequence of the E-cadherin promoter and upregulates E-cadherin expression implicated in tumour metastasis as a promoter of growth and metastasis (Na et al., 2020). In this study, we comprehensively analysed TCGA and GEO PCa datasets with bioinformatics approaches, which provided relations of PNN with PCa on the molecular pathway level. Similarly, these results indicated that the PNN gene could be a useful prognosis feature for PCa patients in clinical practice related to tumour progression and poor prognosis (Wei et al., 2016; Yang et al., 2016; Tang et al., 2020; Jin et al., 2021).
We identified several FDA-approved drugs potentially useful for PCa patients. Valproic acid (VPA) is a neuroprotective agent used for disease relating to neurological diseases (Kale et al., 2021). It is reported that through autophagy and apoptosis, VPA synergistically enhances anticancer effects with Arsenic trioxide in lung cancer cells (Park et al., 2020) and advanced patients in clinical Phase I (Atmaca et al., 2007). Another identified drug is Vorinostat which is applied to cutaneous T cell lymphoma (Dumont et al., 2014). In fact, some research has applied this drug to PCa patients as a sensitiser in therapy for PCa (Stiborova et al., 2012; Jonsson et al., 2016). Trichostatin A comes from the same family as Vorinostat; it inhibits histone deacetylases (HDAC) and is a broad spectral drug for various cancers. Additionally, the natural products and anti-proliferative agents, Camptothecin (and its derivative) (Zhang et al., 2000; Chiu et al., 2020) and Fisetin (Kashyap et al., 2019), are able to induce apoptosis and interfere with the cell cycle. Cephaeline inhibits cancer cells’ growth, migration and proliferation (Silva et al., 2021). The progression of the PCa tumours can be hindered by the medication of those drugs or molecules. The drug sensitivity data were integrated to identify those drugs with variant sensitivity in different subgroups. By proposing promising therapy candidates for targeted treatment for PCa patients, our results provide an additional selection of the clinical practice for treating PCa patients. By contrast, due to the ambiguous role of Vitamin E (Yang et al., 2020) and Glibenclamide potentially causing death (Monami et al., 2006), these two drugs are not suitable for clinical application. Doxorubicin, a highly effective anticancer drug, induces many cardiotoxic effects (Ferreira et al., 2019); hence, it is not recommended so far.
Several studies elucidate the critical role of RNA splicing in cancer pathogenesis (Inoue et al., 2019; Shuai et al., 2019; Suzuki et al., 2019; Wang et al., 2020). With GO and KEGG enrichment analysis, we found that the gene PNN, together with its 25UC gene set, is involved in RNA splicing. We could conclude that PNN might play a role in RNA splicing by participating in spliceosomes. It has been suggested that tumour pathogenesis is influenced by splicing resulting from abnormal splicing that is widespread in cancer, such as dysregulation of splicing and aberrant splicing patterns (Ryan et al., 2015; Seiler et al., 2018; Wang et al., 2020). Thus, we believe that dysfunction of the PNN gene will affect the normal function of the spliceosome, which will result in many aberrant mRNAs because of abnormal splicing.
The GSEA based on PNN high- and low-expression groups offered possible pathways related to immune infiltration. Nevertheless, we observed that plenty of genes were co-expressed with PNN gene; hence, it is hard to state that PNN was related to immune infiltration. The tumours with increased PNN expression shared a similar correlation pattern with TIL and chemokines. Thus, PNN could be a indictor for TIL. We also performed ssGSEA to explore the potential mechanism of PNN in RNA splicing with the high- and low-risk groups of PCa. Among all the differently enriched pathways, it has been found that the high-risk group showed higher enriched scores in the spliceosome pathway and PNN expression also positively correlated with this pathway. The hypomethylation status of CpG sites in the PNN gene promoter probably resulted in an increased PNN expression and then potentially contributes to the progression of PCa. The significantly increased expression of several splicing factor genes, such as SF3B1, SRSF11 and SRSF7 (Figure 6H), in the high-risk group suggested that abnormal splicing was associated with an increased risk for PCa, such as progression.
We attempted to comprehensively determine the potential underlying mechanisms of PNN on PCa progression. Therefore, we also explored the role of epigenetic markers in PCa. DNA methylation is an epigenetic marker that is essential in regulating gene expression. DNA methylation of CGIs is essential for gene expression and tissue-specific processes. Previous reports indicate that DNA methylation at promoters negatively correlates with gene expression (Chen et al., 2016; Keller et al., 2016; Neri et al., 2017). Aberrant methylation of PNN CGIs was correlated with changed PNN expression (Akin et al., 2016). Using the methylation status of CpG sites in the PNN gene and its co-expressed genes, we constructed a prognostic signature. This signature suggested that the methylation status of CpG sites may play a role in the prognostic prediction, while the combined methylation signature might provide better potential for achieving more sensitive and specific prognostic value in PCa patients. The prognostic value of these DNA methylation signatures has not been intensively explored yet. We have found that the high- and low-risk groups could respond differently to the immune therapies, suggesting the classification of PCa is meaningful (Xia et al., 2021). Dividing patients based on their risk scores would be a direction of precision therapeutics, which will be facilitated by classifying PCa (Wu et al., 2021). Therefore, the present study provides a new insight that a combination of epigenetic biomarkers may improve risk stratification and survival prediction in PCa patients.
Based on those results, the pinin protein should participate in the biological activity of spliceosomes or splicing (Kim et al., 2017). Pinin is an exon junction component (EJC), which is a member of the spliceosome complexes (Akin et al., 2016). Although the mechanism underlying PNN promoting tumourigenesis is rarely reported, SF3B1, a well-known spliceosome-associated gene and co-expressed with PNN (r > 0.8), is linked to a variety of solid tumours, including PCa (Rahman et al., 2020; Yang et al., 2022). Therefore, as a member of the spliceosome, mutations in PNN could rewire its interactions with other proteins in the spliceosome, including the SF3B1 gene, which will lead to splicesome dysfunction, and enhance the activation of the NF-
Through bioinformatics analyses, we have explored the differential expression pattern of PNN between normal and PCa patients, its independent prognostic value, the potential regulatory mechanisms, the relationship with immune infiltration, and the co-expression genes. We validated our results to prove our results using external datasets. Since our results solely come from data analysis, experimental verification will need to support further the rationale of the molecular mechanisms underlying PCa progression. In conclusion, PNN is a potentially valuable biomarker for PCa diagnosis and patient management. Furthermore, we have identified the potential new drugs as well as the ICGs that could be utilised in immune therapy for PCa treatment for PCa patients with high expression of PNN.
The original contributions presented in the study are included in the article/Supplementary Material, further inquiries can be directed to the corresponding authors.
Conceptualisation, ML; Data curation, RW, ZQ, and HL; Formal analysis, RW and MP; Funding acquisition, PY; Investigation, RW; Methodology, MP; Project administration, PY; Resources, RW; Software, MP; Supervision, PY and TS; Validation, ZQ and HL; Visualization, RW; Writing—original draft, RW; Writing—review and editing, RW and TS. All authors have read and agreed to the published version of the manuscript.
This research was funded by the Hunan University of Arts and Science PhD Boosting Project, grant number 20BSQD08 and The APC was funded by Changde Research Centre for Artificial Intelligence and Biomedicine.
We acknowledge TCGA and GEO databases for providing their platforms and contributors for uploading their meaningful datasets. The authors also acknowledge Sangerbox, an online bioinformatics platform, for visualising results of comparisons between high and low-risk groups and GO and KEGG enrichment analysis.
The authors declare that the research was conducted in the absence of any commercial or financial relationships that could be construed as a potential conflict of interest.
All claims expressed in this article are solely those of the authors and do not necessarily represent those of their affiliated organizations, or those of the publisher, the editors and the reviewers. Any product that may be evaluated in this article, or claim that may be made by its manufacturer, is not guaranteed or endorsed by the publisher.
The Supplementary Material for this article can be found online at: https://www.frontiersin.org/articles/10.3389/fgene.2022.1056224/full#supplementary-material
Akin, D., Newman, J. R., Mcintyre, L. M., and Sugrue, S. P. (2016). RNA-seq analysis of impact of PNN on gene expression and alternative splicing in corneal epithelial cells. Mol. Vis. 22, 40–60.
Aryee, M. J., Liu, W., Engelmann, J. C., Nuhn, P., Gurel, M., Haffner, M. C., et al. (2013). DNA methylation alterations exhibit intraindividual stability and interindividual heterogeneity in prostate cancer metastases. Sci. Transl. Med. 5, 169ra10. doi:10.1126/scitranslmed.3005211
Atmaca, A., Al-Batran, S. E., Maurer, A., Neumann, A., Heinzel, T., Hentsch, B., et al. (2007). Valproic acid (VPA) in patients with refractory advanced cancer: A dose escalating phase I clinical trial. Br. J. Cancer 97, 177–182. doi:10.1038/sj.bjc.6603851
Berman, H. M., Westbrook, J., Feng, Z., Gilliland, G., Bhat, T. N., Weissig, H., et al. (2000). The protein data bank. Nucleic Acids Res. 28, 235–242. doi:10.1093/nar/28.1.235
BöRNO, S. T., Fischer, A., Kerick, M., FäLTH, M., Laible, M., Brase, J. C., et al. (2012). Genome-wide DNA methylation events in TMPRSS2-ERG fusion-negative prostate cancers implicate an EZH2-dependent mechanism with miR-26a hypermethylation. Cancer Discov. 2, 1024–1035. doi:10.1158/2159-8290.CD-12-0041
Caggiano, C., Pieraccioli, M., Panzeri, V., Sette, C., and Bielli, P. (2019). c-MYC empowers transcription and productive splicing of the oncogenic splicing factor Sam68 in cancer. Nucleic Acids Res. 47, 6160–6171. doi:10.1093/nar/gkz344
Chen, E. Y., Tan, C. M., Kou, Y., Duan, Q., Wang, Z., Meirelles, G. V., et al. (2013). Enrichr: Interactive and collaborative HTML5 gene list enrichment analysis tool. BMC Bioinforma. 14, 128. doi:10.1186/1471-2105-14-128
Chen, K., Zhang, J., Guo, Z., Ma, Q., Xu, Z., Zhou, Y., et al. (2016). Loss of 5-hydroxymethylcytosine is linked to gene body hypermethylation in kidney cancer. Cell. Res. 26, 103–118. doi:10.1038/cr.2015.150
Chen, X., Ji, Z. L., and Chen, Y. Z. (2002). Ttd: Therapeutic target database. Nucleic Acids Res. 30, 412–415. doi:10.1093/nar/30.1.412
Chiu, C.-F., Lin, Y.-Q., Park, J. M., Chen, Y.-C., Hung, S.-W., Chiu, C.-C., et al. (2020). The novel camptothecin derivative, CPT211, induces cell cycle arrest and apoptosis in models of human breast cancer. Biomed. Pharmacother. 128, 110309. doi:10.1016/j.biopha.2020.110309
Cioni, B., Zaalberg, A., van Beijnum, J. R., Melis, M. H. M., van Burgsteden, J., Muraro, M. J., et al. (2020). Androgen receptor signalling in macrophages promotes TREM-1-mediated prostate cancer cell line migration and invasion. Nat. Commun. 11, 4498. doi:10.1038/s41467-020-18313-y
Cucchiara, V., Cooperberg, M. R., Dall’Era, M., Lin, D. W., Montorsi, F., Schalken, J. A., et al. (2017). Genomic markers in prostate cancer decision making. Eur. Urol. 73, 572–582. S0302283817309752. doi:10.1016/j.eururo.2017.10.036
Dai, X., Xin, Y., Xu, W., Tian, X., Wei, X., and Zhang, H. (2021). CBP-mediated Slug acetylation stabilizes Slug and promotes EMT and migration of breast cancer cells. Sci. China. Life Sci. 64, 563–574. doi:10.1007/s11427-020-1736-5
Danilova, L., Ho, W. J., Zhu, Q., Vithayathil, T., de Jesus-Acosta, A., Azad, N. S., et al. (2019). Programmed cell death ligand-1 (PD-L1) and CD8 expression profiling identify an immunologic subtype of pancreatic ductal adenocarcinomas with favorable survival. Cancer Immunol. Res. 7, 886–895. doi:10.1158/2326-6066.CIR-18-0822
Datta, L. P., Manchineella, S., and Govindaraju, T. (2020). Biomolecules-derived biomaterials. Biomaterials 230, 119633. doi:10.1016/j.biomaterials.2019.119633
Dumont, S. N., Yang, D., Dumont, A. G., Reynoso, D., Blay, J.-Y., and Trent, J. C. (2014). Targeted polytherapy in small cell sarcoma and its association with doxorubicin. Mol. Oncol. 8, 1458–1468. doi:10.1016/j.molonc.2014.05.016
Ecke, T. H., Schlechte, H. H., Schiemenz, K., Sachs, M., Loening, S. A., Rudolph, B. D., et al. (2010). TP53 gene mutations in prostate cancer progression. Anticancer Res. 30, 1579–1586.
Ferreira, L. L., Oliveira, P. J., and Cunha-Oliveira, T. (2019). “Chapter 33 - epigenetics in doxorubicin cardiotoxicity,” in Pharmacoepigenetics. Editor R. CACABELOS (Massachusetts, United States: Academic Press).
HäNZELMANN, S., Castelo, R., and Guinney, J. (2013). Gsva: Gene set variation analysis for microarray and RNA-seq data. BMC Bioinforma. 14, 7. doi:10.1186/1471-2105-14-7
Inoue, D., Chew, G. L., Liu, B., Michel, B. C., Pangallo, J., D'Avino, A. R., et al. (2019). Spliceosomal disruption of the non-canonical BAF complex in cancer. Nature 574, 432–436. doi:10.1038/s41586-019-1646-9
Jain, S., Lyons, C. A., Walker, S. M., Mcquaid, S., Hynes, S. O., Mitchell, D. M., et al. (2018). Validation of a Metastatic Assay using biopsies to improve risk stratification in patients with prostate cancer treated with radical radiation therapy. Ann. Oncol. 29, 215–222. doi:10.1093/annonc/mdx637
Jambor, I., Verho, J., Ettala, O., Knaapila, J., Taimen, P., SyväNEN, K. T., et al. (2019). Validation of improd biparametric MRI in men with clinically suspected prostate cancer: A prospective multi-institutional trial. PLoS Med. 16, e1002813. doi:10.1371/journal.pmed.1002813
Jin, M., Li, D., Liu, W., Wang, P., Xiang, Z., and Liu, K. (2021). Pinin acts as a poor prognostic indicator for renal cell carcinoma by reducing apoptosis and promoting cell migration and invasion. J. Cell. Mol. Med. 25, 4340–4348. doi:10.1111/jcmm.16495
Johnson, W. E., Li, C., and Rabinovic, A. (2007). Adjusting batch effects in microarray expression data using empirical Bayes methods. Biostatistics 8, 118–127. doi:10.1093/biostatistics/kxj037
Jonsson, M., Ragnum, H. B., Julin, C. H., Yeramian, A., Clancy, T., Frikstad, K.-A. M., et al. (2016). Hypoxia-independent gene expression signature associated with radiosensitisation of prostate cancer cell lines by histone deacetylase inhibition. Br. J. Cancer 115, 929–939. doi:10.1038/bjc.2016.278
Kale, V. P., Habib, H., Chitren, R., Patel, M., Pramanik, K. C., Jonnalagadda, S. C., et al. (2021). Old drugs, new uses: Drug repurposing in hematological malignancies. Semin. Cancer Biol. 68, 242–248. doi:10.1016/j.semcancer.2020.03.005
Kanai, H., Sawa, A., Chen, R. W., Leeds, P., and Chuang, D. M. (2004). Valproic acid inhibits histone deacetylase activity and suppresses excitotoxicity-induced GAPDH nuclear accumulation and apoptotic death in neurons. Pharmacogenomics J. 4, 336–344. doi:10.1038/sj.tpj.6500269
Kang, G. J., Park, M. K., Byun, H. J., Kim, H. J., Kim, E. J., Yu, L., et al. (2020). SARNP, a participant in mRNA splicing and export, negatively regulates E-cadherin expression via interaction with pinin. J. Cell. Physiol. 235, 1543–1555. doi:10.1002/jcp.29073
Kashyap, D., Garg, V. K., Tuli, H. S., Yerer, M. B., Sak, K., Sharma, A. K., et al. (2019). Fisetin and quercetin: Promising flavonoids with chemopreventive potential. Biomolecules 9, 174. doi:10.3390/biom9050174
Keller, T. E., Han, P., and Yi, S. V. (2016). Evolutionary transition of promoter and gene body DNA methylation across invertebrate-vertebrate boundary. Mol. Biol. Evol. 33, 1019–1028. doi:10.1093/molbev/msv345
Kim, Y. D., Lee, J., Kim, H. S., Lee, M. O., Son, M. Y., Yoo, C. H., et al. (2017). The unique spliceosome signature of human pluripotent stem cells is mediated by SNRPA1, SNRPD1, and PNN. Stem Cell. Res. 22, 43–53. doi:10.1016/j.scr.2017.05.010
Kim, Y., Jeon, J., Mejia, S., Yao, C. Q., Ignatchenko, V., Nyalwidhe, J. O., et al. (2016). Targeted proteomics identifies liquid-biopsy signatures for extracapsular prostate cancer. Nat. Commun. 7, 11906. doi:10.1038/ncomms11906
Koch, A., de Meyer, T., Jeschke, J., and van Criekinge, W. (2015). Mexpress: Visualizing expression, DNA methylation and clinical TCGA data. BMC Genomics 16, 636. doi:10.1186/s12864-015-1847-z
Koch, A., Jeschke, J., van Criekinge, W., van Engeland, M., and de Meyer, T. (2019). MEXPRESS update 2019. Nucleic Acids Res. 47, W561–W565. doi:10.1093/nar/gkz445
Komata, T., Kanzawa, T., Nashimoto, T., Aoki, H., Endo, S., Kon, T., et al. (2005). Histone deacetylase inhibitors, N-butyric acid and trichostatin A, induce caspase-8- but not caspase-9-dependent apoptosis in human malignant glioma cells. Int. J. Oncol. 26, 1345–1352. doi:10.3892/ijo.26.5.1345
Kuleshov, M. V., Jones, M. R., Rouillard, A. D., Fernandez, N. F., Duan, Q., Wang, Z., et al. (2016). Enrichr: A comprehensive gene set enrichment analysis web server 2016 update. Nucleic Acids Res. 44, W90–W97. doi:10.1093/nar/gkw377
Law, K. W., Nguyen, D. D., Barkin, J., and Zorn, K. C. (2020). Diagnosis of prostate cancer: The implications and proper utilization of PSA and its variants; indications and use of MRI and biomarkers. Can. J. Urol. 27, 3–10.
Li, D., Hao, X., and Song, Y. (2018). Identification of the key MicroRNAs and the miRNA-mRNA regulatory pathways in prostate cancer by bioinformatics methods. Biomed. Res. Int. 2018, 6204128–6204210. doi:10.1155/2018/6204128
Lindberg, J., Mills, I. G., Klevebring, D., Liu, W., Neiman, M., Xu, J., et al. (2013). The mitochondrial and autosomal mutation landscapes of prostate cancer. Eur. Urol. 63, 702–708. doi:10.1016/j.eururo.2012.11.053
Liu, X., Zhang, L., Thu, P. M., Min, W., Yang, P., Li, J., et al. (2021). Sodium cantharidinate, a novel anti-pancreatic cancer agent that activates functional p53. Sci. China. Life Sci. 64, 1295–1310. doi:10.1007/s11427-019-1753-3
Malone, C. D., Olson, E. S., Mattrey, R. F., Jiang, T., Tsien, R. Y., and Nguyen, Q. T. (2015). Tumor detection at 3 tesla with an activatable cell penetrating peptide dendrimer (ACPPD-Gd), a T1 magnetic resonance (MR) molecular imaging agent. Plos One 10, e0137104. doi:10.1371/journal.pone.0137104
Menendez, J. A., Vellon, L., and Lupu, R. (2006). DNA topoisomerase IIalpha (TOP2A) inhibitors up-regulate fatty acid synthase gene expression in SK-Br3 breast cancer cells: In vitro evidence for a 'functional amplicon' involving FAS, her-2/neu and TOP2A genes. Int. J. Mol. Med. 18, 1081–1087.
Monami, M., Luzzi, C., Lamanna, C., Chiasserini, V., Addante, F., Desideri, C. M., et al. (2006). Three-year mortality in diabetic patients treated with different combinations of insulin secretagogues and metformin. Diabetes. Metab. Res. Rev. 22, 477–482. doi:10.1002/dmrr.642
Murillo-GarzóN, V., GorroñO-Etxebarria, I., Åkerfelt, M., Puustinen, M. C., Sistonen, L., Nees, M., et al. (2018). Frizzled-8 integrates Wnt-11 and transforming growth factor-β signaling in prostate cancer. Nat. Commun. 9, 1747. doi:10.1038/s41467-018-04042-w
Na, T.-Y., Schecterson, L., Mendonsa, A. M., and Gumbiner, B. M. (2020). The functional activity of E-cadherin controls tumor cell metastasis at multiple steps. Proc. Natl. Acad. Sci. U. S. A. 117, 5931–5937. doi:10.1073/pnas.1918167117
Neri, F., Rapelli, S., Krepelova, A., Incarnato, D., Parlato, C., Basile, G., et al. (2017). Intragenic DNA methylation prevents spurious transcription initiation. Nature 543, 72–77. doi:10.1038/nature21373
Oughtred, R., Rust, J., Chang, C., Breitkreutz, B. J., Stark, C., Willems, A., et al. (2021). The BioGRID database: A comprehensive biomedical resource of curated protein, genetic, and chemical interactions. Protein Sci. 30, 187–200. doi:10.1002/pro.3978
Pan, L.-H., Pang, S.-T., Fang, P.-Y., Chuang, C.-K., and Yang, H.-W. (2017). Label-free biochips for accurate detection of prostate cancer in the clinic: Dual biomarkers and circulating tumor cells. Theranostics 7, 4289–4300. doi:10.7150/thno.21092
Park, H. K., Han, B. R., and Park, W. H. (2020). Combination of arsenic trioxide and valproic acid efficiently inhibits growth of lung cancer cells via G2/M-phase Arrest and apoptotic cell death. Int. J. Mol. Sci. 21, 2649. doi:10.3390/ijms21072649
Patil, N., and Gaitonde, K. (2016). Clinical perspective of prostate cancer. Top. Magnetic Reson. Imaging 25, 103–108. doi:10.1097/rmr.0000000000000091
Phillips, R., Shi, W. Y., Deek, M., Radwan, N., Lim, S. J., Antonarakis, E. S., et al. (2020). Outcomes of observation vs stereotactic ablative radiation for oligometastatic prostate cancer: The ORIOLE phase 2 randomized clinical trial. JAMA Oncol. 6, 650–659. doi:10.1001/jamaoncol.2020.0147
Pollyea, D. A., Harris, C., Rabe, J. L., Hedin, B. R., de Arras, L., Katz, S., et al. (2019). Myelodysplastic syndrome-associated spliceosome gene mutations enhance innate immune signaling. Haematologica 104, e388–e392. doi:10.3324/haematol.2018.214155
Rahman, M. A., Krainer, A. R., and Abdel-Wahab, O. (2020). SnapShot: Splicing alterations in cancer. Cell. 180, 208–208.e1. doi:10.1016/j.cell.2019.12.011
Ren, D., Yang, Q., Dai, Y., Guo, W., Du, H., Song, L., et al. (2017). Oncogenic miR-210-3p promotes prostate cancer cell EMT and bone metastasis via NF-κB signaling pathway. Mol. Cancer 16, 117. doi:10.1186/s12943-017-0688-6
Ru, B., Wong, C. N., Tong, Y., Zhong, J. Y., Zhong, S. S. W., Wu, W. C., et al. (2019). Tisidb: An integrated repository portal for tumor-immune system interactions. Bioinformatics 35, 4200–4202. doi:10.1093/bioinformatics/btz210
Ryan, M., Wong, W. C., Brown, R., Akbani, R., Su, X., Broom, B., et al. (2015). TCGASpliceSeq a compendium of alternative mRNA splicing in cancer. Nucleic Acids Res. 44, D1018–D1022. doi:10.1093/nar/gkv1288
SchmöLZ, L., Birringer, M., Lorkowski, S., and Wallert, M. (2016). Complexity of vitamin E metabolism. World J. Biol. Chem. 7, 14–43. doi:10.4331/wjbc.v7.i1.14
Seiler, M., Peng, S., Agrawal, A. A., Palacino, J., Teng, T., Zhu, P., et al. (2018). Somatic mutational landscape of splicing factor genes and their functional consequences across 33 cancer types. Cell. Rep. 23, 282–296. e4. doi:10.1016/j.celrep.2018.01.088
Serrano-MartíN, X., Payares, G., and Mendoza-LeóN, A. (2006). Glibenclamide, a blocker of K+(ATP) channels, shows antileishmanial activity in experimental murine cutaneous leishmaniasis. Antimicrob. Agents Chemother. 50, 4214–4216. doi:10.1128/AAC.00617-06
Shuai, S., Suzuki, H., Diaz-Navarro, A., Nadeu, F., Kumar, S. A., Gutierrez-Fernandez, A., et al. (2019). The U1 spliceosomal RNA is recurrently mutated in multiple cancers. Nature 574, 712–716. doi:10.1038/s41586-019-1651-z
Silva, L. C., Borgato, G. B., Wagner, V. P., Martins, M. D., Rocha, G. Z., Lopes, M. A., et al. (2021). Cephaeline is an inductor of histone H3 acetylation and inhibitor of mucoepidermoid carcinoma cancer stem cells. J. Oral Pathol. Med. 51, 553–562. n/a. doi:10.1111/jop.13252
Simon, L., Hollenbach, A. D., Zabaleta, J., and Molina, P. E. (2015). Chronic binge alcohol administration dysregulates global regulatory gene networks associated with skeletal muscle wasting in simian immunodeficiency virus-infected macaques. BMC genomics 16, 1097. doi:10.1186/s12864-015-2329-z
Stiborova, M., Eckschlager, T., Poljakova, J., Hrabeta, J., Adam, V., Kizek, R., et al. (2012). The synergistic effects of DNA-targeted chemotherapeutics and histone deacetylase inhibitors as therapeutic strategies for cancer treatment. Curr. Med. Chem. 19, 4218–4238. doi:10.2174/092986712802884286
Subramanian, A., Tamayo, P., Mootha, V. K., Mukherjee, S., Ebert, B. L., Gillette, M. A., et al. (2005). Gene set enrichment analysis: A knowledge-based approach for interpreting genome-wide expression profiles. Proc. Natl. Acad. Sci. U. S. A. 102, 15545–15550. doi:10.1073/pnas.0506580102
Sun, W., Duan, T., Ye, P., Chen, K., Zhang, G., Lai, M., et al. (2018). TSVdb: A web-tool for TCGA splicing variants analysis. BMC Genomics 19, 405. doi:10.1186/s12864-018-4775-x
Sun, Y., and Goodison, S. (2009). Optimizing molecular signatures for predicting prostate cancer recurrence. Prostate 69, 1119–1127. doi:10.1002/pros.20961
Suzuki, H., Kumar, S. A., Shuai, S., Diaz-Navarro, A., Gutierrez-Fernandez, A., de Antonellis, P., et al. (2019). Recurrent noncoding U1 snRNA mutations drive cryptic splicing in SHH medulloblastoma. Nature 574, 707–711. doi:10.1038/s41586-019-1650-0
Taminau, J., Meganck, S., Lazar, C., Steenhoff, D., Coletta, A., Molter, C., et al. (2012). Unlocking the potential of publicly available microarray data using inSilicoDb and inSilicoMerging R/Bioconductor packages. BMC Bioinforma. 13, 335. doi:10.1186/1471-2105-13-335
Tang, T., Yang, L., Cao, Y., Wang, M., Zhang, S., Gong, Z., et al. (2020). LncRNA AATBC regulates Pinin to promote metastasis in nasopharyngeal carcinoma. Mol. Oncol. 14, 2251–2270. doi:10.1002/1878-0261.12703
Ueda, K., Komine, J., Matsuo, M., Seino, S., and Amachi, T. (1999). Cooperative binding of ATP and MgADP in the sulfonylurea receptor is modulated by glibenclamide. Proc. Natl. Acad. Sci. U. S. A. 96, 1268–1272. doi:10.1073/pnas.96.4.1268
UhléN, M., Fagerberg, L., Hallstrm, B. M., Lindskog, C., PontéN, F., Mardinoglu, A., et al. (2015). Proteomics. Tissue-based map of the human proteome. Science 347, 1260419. doi:10.1126/science.1260419
Vandamme, N., Denecker, G., Bruneel, K., Blancke, G., Akay, Ö., Taminau, J., et al. (2020). The EMT transcription factor ZEB2 promotes proliferation of primary and metastatic melanoma while suppressing an invasive, mesenchymal-like phenotype. Cancer Res. 80, 2983–2995. doi:10.1158/0008-5472.CAN-19-2373
Wallace, T. A., Prueitt, R. L., Yi, M., Howe, T. M., Gillespie, J. W., Yfantis, H. G., et al. (2008). Tumor immunobiological differences in prostate cancer between African-American and European-American men. Cancer Res. 68, 927–936. doi:10.1158/0008-5472.CAN-07-2608
Wang, Y., Bao, Y., Zhang, S., and Wang, Z. (2020). Splicing dysregulation in cancer: From mechanistic understanding to a new class of therapeutic targets. Sci. China. Life Sci. 63, 469–484. doi:10.1007/s11427-019-1605-0
Wei, Z., Ma, W., Qi, X., Zhu, X., Wang, Y., Xu, Z., et al. (2016). Pinin facilitated proliferation and metastasis of colorectal cancer through activating EGFR/ERK signaling pathway. Oncotarget 7, 29429–29439. doi:10.18632/oncotarget.8738
Wu, S. Y., Wang, H., Shao, Z. M., and Jiang, Y. Z. (2021). Triple-negative breast cancer: New treatment strategies in the era of precision medicine. Sci. China. Life Sci. 64, 372–388. doi:10.1007/s11427-020-1714-8
Xia, L., Wang, H., Sun, M., Yang, Y., Yao, C., He, S., et al. (2021). Peripheral CD4(+) T cell signatures in predicting the responses to anti-PD-1/PD-L1 monotherapy for Chinese advanced non-small cell lung cancer. Sci. China. Life Sci. 64, 1590–1601. doi:10.1007/s11427-020-1861-5
Xie, Z., Bailey, A., Kuleshov, M. V., Clarke, D. J. B., Evangelista, J. E., Jenkins, S. L., et al. (2021). Gene set knowledge discovery with Enrichr. Curr. Protoc. 1, e90. doi:10.1002/cpz1.90
Xu, W. S., Parmigiani, R. B., and Marks, P. A. (2007). Histone deacetylase inhibitors: Molecular mechanisms of action. Oncogene 26, 5541–5552. doi:10.1038/sj.onc.1210620
Yang, C. S., Luo, P., Zeng, Z., Wang, H., Malafa, M., and Suh, N. (2020). Vitamin E and cancer prevention: Studies with different forms of tocopherols and tocotrienols. Mol. Carcinog. 59, 365–389. doi:10.1002/mc.23160
Yang, H., Beutler, B., and Zhang, D. (2022). Emerging roles of spliceosome in cancer and immunity. Protein Cell. 13, 559–579. doi:10.1007/s13238-021-00856-5
Yang, X., Sun, D., Dong, C., Tian, Y., Gao, Z., and Wang, L. (2016). Pinin associates with prognosis of hepatocellular carcinoma through promoting cell proliferation and suppressing glucose deprivation-induced apoptosis. Oncotarget 7, 39694–39704. doi:10.18632/oncotarget.9233
Yao, F., and Ma, L. (2020). piRNA-unbound PIWIL1 promotes metastasis. Nat. Cell. Biol. 22, 359–360. doi:10.1038/s41556-020-0502-3
Yin, Q., Han, T., Fang, B., Zhang, G., Zhang, C., Roberts, E. R., et al. (2019). K27-linked ubiquitination of BRAF by ITCH engages cytokine response to maintain MEK-ERK signaling. Nat. Commun. 10, 1870. doi:10.1038/s41467-019-09844-0
Yu, G., Wang, L. G., Han, Y., and He, Q. Y. (2012). clusterProfiler: an R package for comparing biological themes among gene clusters. Omics-a J. Integr. Biol. 16, 284–287. doi:10.1089/omi.2011.0118
Yuan, Y., Li, H., Pu, W., Chen, L., Guo, D., Jiang, H., et al. (2022). Cancer metabolism and tumor microenvironment: Fostering each other? Sci. China. Life Sci. 65, 236–279. doi:10.1007/s11427-021-1999-2
Zhang, Q., Lin, F., Huang, J., and Xiong, C. (2022). Mechanical transmission enables EMT cancer cells to drive epithelial cancer cell migration to guide tumor spheroid disaggregation. Sci. China. Life Sci. 65, 2031–2049. doi:10.1007/s11427-021-2054-3
Zhang, Y., Kwok, J. S.-L., Choi, P.-W., Liu, M., Yang, J., Singh, M., et al. (2016). Pinin interacts with C-terminal binding proteins for RNA alternative splicing and epithelial cell identity of human ovarian cancer cells. Oncotarget 7, 11397–11411. doi:10.18632/oncotarget.7242
Keywords: prognosis signature, PNN, immune infiltration, drug prediction, methylation status, prostate cancer
Citation: Wang R, Qin Z, Luo H, Pan M, Liu M, Yang P and Shi T (2022) Prognostic value of PNN in prostate cancer and its correlation with therapeutic significance. Front. Genet. 13:1056224. doi: 10.3389/fgene.2022.1056224
Received: 28 September 2022; Accepted: 31 October 2022;
Published: 16 November 2022.
Edited by:
Youping Deng, Rush University Medical Center, United StatesCopyright © 2022 Wang, Qin, Luo, Pan, Liu, Yang and Shi. This is an open-access article distributed under the terms of the Creative Commons Attribution License (CC BY). The use, distribution or reproduction in other forums is permitted, provided the original author(s) and the copyright owner(s) are credited and that the original publication in this journal is cited, in accordance with accepted academic practice. No use, distribution or reproduction is permitted which does not comply with these terms.
*Correspondence: Pinhong Yang,cGluaG9uZ195YW5nQDE2My5jb20=Tieliu Shi,dGllbGl1c2hpQHlhaG9vLmNvbQ==
Disclaimer: All claims expressed in this article are solely those of the authors and do not necessarily represent those of their affiliated organizations, or those of the publisher, the editors and the reviewers. Any product that may be evaluated in this article or claim that may be made by its manufacturer is not guaranteed or endorsed by the publisher.
Research integrity at Frontiers
Learn more about the work of our research integrity team to safeguard the quality of each article we publish.