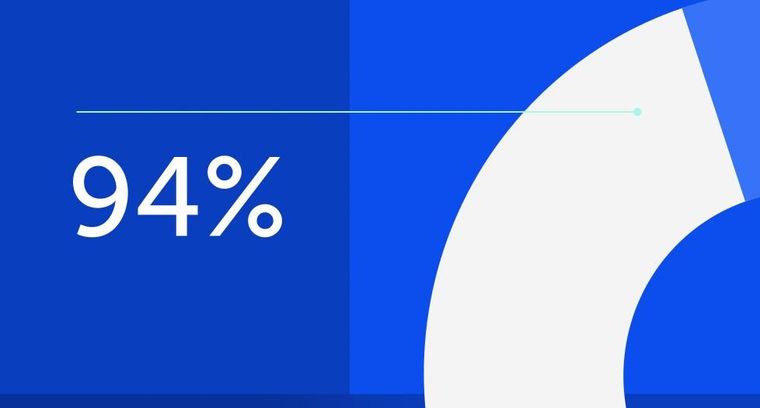
94% of researchers rate our articles as excellent or good
Learn more about the work of our research integrity team to safeguard the quality of each article we publish.
Find out more
ORIGINAL RESEARCH article
Front. Genet., 25 October 2022
Sec. Cancer Genetics and Oncogenomics
Volume 13 - 2022 | https://doi.org/10.3389/fgene.2022.1047435
This article is part of the Research TopicMulti-Omics Analysis in Tumor Microenvironment and Tumor HeterogeneityView all 37 articles
Background: Immunotherapy has been a promising approach option for lung cancer.
Method: All the open-accessed data was obtained from the Cancer Genome Atlas (TCGA) database. All the analysis was conducted using the R software analysis.
Results: Firstly, the genes differentially expressed in lung cancer immunotherapy responders and non-responders were identified. Then, the lung adenocarcinoma immunotherapy-related genes were determined by LASSO logistic regression and SVM-RFE, respectively. A total of 18 immunotherapy response-related genes were included in our investigation. Subsequently, we constructed the logistics score model. Patients with high logistics score had a better clinical effect on immunotherapy, with 63.2% of patients responding to immunotherapy, while only 12.1% of patients in the low logistics score group responded to immunotherapy. Moreover, we found that pathways related to immunotherapy were mainly enriched in metabolic pathways such as fatty acid metabolism, bile acid metabolism, oxidative phosphorylation, and carcinogenic pathways such as KRAS signaling. Logistics score was positively correlated with NK cells activated, Mast cells resting, Monocytes, Macrophages M2, dendritic cells resting, dendritic cells activated and eosinophils, while was negatively related to Tregs, macrophages M0, macrophages M1, and mast cells activated. In addition, ERVH48-1 was screened for single-cell exploration. The expression of ERVH48-1 increased in patients with distant metastasis, and ERVH48-1 was associated with pathways such as pancreas beta cells, spermatogenesis, G2M checkpoints and KRAS signaling. The result of quantitative real-time PCR showed that ERVH48-1 was upregulated in lung cancer cells.
Conclusion: Our study developed an effective signature to predict the immunotherapy response of lung cancer patients.
Deaths from lung cancer account for a significant percentage of all cancer-related deaths (Nguyen et al., 2022). There are many types of lung cancer, but the majority of them are non-small cell lung cancer (NSCLC) (Zhang et al., 2021). As cancer genomics has advanced in the past few decades, several mutations have been identified as lung adenocarcinoma (LUAD) driver genes, such as KRAS, epidermal growth factor receptors (EGFRs), c-METs, and so on (Chen et al., 2019; Wang et al., 2019; Sun et al., 2020; Wang et al., 2020). A variety of drugs have since been developed to target mutations in driver genes. Despite receiving targeted therapies, most patients eventually develop resistance to them, partly due to secondary mutations in the tumor (Foggetti et al., 2021; Tumbrink et al., 2021).
Biologically-based cancer immunotherapy provides new perspectives for cancer treatment (Denisenko et al., 2018). Generally, LUAD often has high tumor mutational burden (TMB) and immunogenicity characteristics (Skoulidis et al., 2018). Therefore, LUAD is an ideal immunotherapy indication (Zhang et al., 2020). Nowadays, immune therapy, like immune checkpoints (ICI), has shown encouraging results. ICI treatment, however, is relatively ineffective for LUAD patients (Santarpia et al., 2020). So far, a series of biomarkers that predict the treatment effectiveness of ICI have been verified, including immune cell status, PD-L1 expression levels, neoantigens, intestinal flora, and TMB (Peng et al., 2020). Transcriptome sequencing files have recently made it possible to estimate the immune status of cancer using the Tumor Immune Dysfunction and Exclusion (TIDE) algorithm. The core is to determine whether T cells are depleted in immune “hot” tumors, or whether there are three types of suppressive T cell infiltration in immune “cold “tumors (Connolly et al., 2021; Shao et al., 2021). There is an urgent need to build a model based on immunotherapy-related genes to indicate the clinical efficacy of ICI.
Cancer immune status is closely related to the prognosis of patients and can indicate the effect of immunotherapy (Ren et al., 2021; Yu et al., 2021). In our investigation, the lung cancer patients were divided into the corresponding immunotherapy responders and non-responders groups through the TIDE algorithm. The characteristic genes of immunotherapy were screened by LASSO logistic regression and the SVM-RFE algorithm. Logistics regression analysis was used to establish a logistics model based on the immunotherapy characteristic genes, and each patient was assigned a logistics score according to the identified formula. Furthermore, we investigated the underlying difference in patients with high and low logistic score. Finally, ERVH48-1 was identified as an underlying target to interfere with the response of immunotherapy in LUAD patients.
The transcriptomic data and clinical information of lung adenocarcinoma patients were obtained from the Cancer Genome Atlas database (TCGA-LUAD project). The format of transcriptomic data was STAR-counts and the format of clinical data was bcr-xml. All raw data was organized using the author’s R and Perl code. Before analysis, all the data were preprocessed, including probe annotation, missing value completion and Data standardization. The baseline information of enrolled patients was shown in Table 1.
Each patient’s response rate to immunotherapy was assessed using the Tumor Immune Dysfunction and Exclusion (TIDE) algorithm (Fu et al., 2020). Specifically, the cancer type was selected as NSCLC. Among them, patients with TIDE >0 were considered non-responders to immunotherapy, and patients with TIDE <0 were considered responders to immunotherapy.
The LASSO logistic regression and SVM-RFE algorithm were performed to optimize variable selection (Deo, 2015). The immunotherapy characteristic genes of LUAD were screened by LASSO logistic regression and SVM-RFE algorism. Logistics regression analysis was used to establish a logistics model based on the immunotherapy characteristic genes, and each patient was assigned a logistics score according to the identified formula.
The Gene Set Enrichment Analysis (GSEA) algorithm was utilized to explore the differences in biological pathways (Subramanian et al., 2005). The reference gene set was Hallmark and c2. cp.kegg.v2022.1. Hs.symbols.gmt file. Gene Ontology (GO) analysis was conducted using the “Clusterprofiler” package (Yu et al., 2012).
Immunocyte infiltration was quantified using the “CIBERSORT” algorithm (Chen et al., 2018).
TMB and microsatellite instability (MSI) were obtained directly from the TCGA database. Tumor stemness index mRNAsi and EREG-mRNAsi were obtained from previously published articles (Malta et al., 2018).
ERVH48-1 analysis at single-cell RNA-seq level was performed via the http://tisch.comp-genomics.org/home/website.
Normal BEAS-2B and lung cancer A549, H1299, and SPC-A1 cell lines were laboratory stocks. Total RNA was extracted using an RNA extraction kit following the protocol. SyBr Green PCR system was used for the qRT-PCR. The primers used were as follows: ERVH48-1, forward primer, 5′-CTCCGGGTTCCAACCAATG-3′, reverse primer, 5′-AGAGGCGACTAGAGGCTGAG-3’; GAPDH, forward primer, 5′- GGAGCGAGATCCCTCCAAAAT-3′, reverse primer, 5′- GGCTGTTGTCATACTTCTCATGG-3’.
An analysis of the data was conducted using R software, and a p value of 0.05 on both sides was considered statistically significant.
The whole flow chart of this study was shown in Supplementary Figure S1. To obtain immune response-related genes, we first divided patients into the immunotherapy responders group and the non-responders group. Figure 1A showed the TIDE score of each LUAD patient. The patients with TIDE score <0 were defined as the immunotherapy responder group, and those with TIDE score >0 were the non-responder group. The “limma” package was utilized to screen for differential expression analysis between the two specific groups, defined as immunotherapy-related candidate genes (Figure 1B). The LASSO logistic regression and SVM-RFE algorithm were used to optimize variable selection. The lung adenocarcinoma immunotherapy-related genes were screened by LASSO logistic regression and SVM-RFE, respectively. The LASSO logistic regression algorithm obtained a total of 18 immunotherapy characteristic genes (Figures 1C,D). The SVM-RFE algorithm screened 22 immunotherapy characteristic genes (Figure 1E). Lastly, the intersection of LASSO logistics regression and SVM-RFE algorithms identified 18 immunotherapy characteristic genes, including GABRA3, SST, APCDD1L, CEACAM8, GPR1, ERVH48-1, EPYC, IGF2, PLPP4, PADI3, MAGEA6, APELA, OBP2A, GAP43, MAGEA3, CYP4Z2P, MAGEA1, IMPA1P1 (Figures 1F,G).
FIGURE 1. Identification of the immunotherapy characteristic genes. Notes: (A) TIDE analysis was performed to evaluate the immunotherapy response of each patient. (B) Volcano plots depicted differentially expressed genes between immunotherapy responders and non-responders; (C,D): LASSO logistic regression analysis based on differentially expressed genes between immunotherapy responders and non-responders. (E) SVM-RFE analysis based on differentially expressed genes between immunotherapy responders and non-responders; (F,G): The intersection of LASSO logistics regression and SVM-RFE algorithms identified 18 immunotherapy characteristic genes.
Next, we found a different expression pattern in characteristic genes between the immunotherapy responders and non-responders groups. For example, GABRA3, EPYC, OBP2A, PAI3, SST, IGF2, GAP43, GPR1, MAGA6, MAGEA1, APCDD1L, PLPP4, MAGEA3, ERVH48-1, and APELA were highly expressed in the immunotherapy non-response group, while CEACAM8, CYP4Z2P, and IMPA1P1 were highly expressed in the immunotherapy responders group (Figure 2A). In addition, ROC curves were utilized to assess the predictive performance of 18 characteristic genes for immunotherapy of LUAD. The AUC values of all characteristic genes were >0.5 and the AUC value of APCDD1L was 0.766 (Figures 2B–S).
FIGURE 2. Evaluation of the prediction performance of immunotherapy characteristic genes. Notes: (A) Expression level of identified 18 characteristic genes in immunotherapy responders and non-responders; (B–S): Prediction performance of 18 characteristic genes in LUAD immunotherapy response.
To effectively evaluate the clinical efficacy of immunotherapy for each patient, we constructed the logistics model based on immunotherapy characteristic genes. Each patient was assigned a logistics score according to the formula: Logistics score = 1.14,241,470 + GABRA3 * -0.20857125 + EPYC * -0.28555130 + OBP2A * -0.23632246 + CEACAM8 * 0.20820226 + PADI3 * -0.04657940 + CYP4Z29 * 0.09164072 + SST * -0.03457475 + IGF2 * -0.92090211 + GAP43 * -0.24484709 + GPR1 * -0.32626723 + MAGEA6 * 0.36074564 + MAGEA1 * - 0.13692973 + APCDD1L * -0.3694431 + MAGEA3 * -0.29105109 + ERVH48-1 * -0.25158873 + APELA * -0.17219285 + IMPA1P1 * 0.75190815”.
Figure 3A displayed the logistics score of each patient. We found that the patients in the immunotherapy responder group had a higher logistics score (Figure 3B). The ROC curve indicated that the logistics score had excellent performance in predicting LUAD immunotherapy response (Figure 3C, AUC: 0.851). Among the patients with low logistics scores, only 12.1% responded to immunotherapy significantly, while 63.2% of those with high logistics scores responded significantly (Figure 3D). In addition, we found a significantly higher level of CTLA4 and PDCD1 in patients with low logistics score (Figure 3E).
FIGURE 3. Logistics regression model. Notes: (A) Logistic regression model was constructed based on the identified characteristic genes; (B) Differences of logistics score between immunotherapy responders and nonresponders; (C) ROC curve was utilized to assess the performance of Logistics score in predicting the response to immunotherapy in LUAD patients; (D) Proportion of immunotherapy responders and non-responders in patients with high and low logistics score; (E) The level of key immune checkpoints in patients with high and low logistics score.
Subsequently, we performed GSEA pathway analysis and GO analysis on patients with high and low logistics score subgroups. We found that pathways related to immunotherapy were mainly enriched in many metabolic pathways such as fatty acid metabolism, bile acid metabolism, oxidative phosphorylation, and carcinogenic pathways such as KRAS signaling up, MYC targets, epithelial-mesenchymal transition (Figure 4A). The loop diagram showed the enrichment of immunotherapy response-related genes in the pathway, such as GO: 0048568 and GO: 0048562 (Figure 4B). Kyoto Encyclopedia of Genes and Genomes (KEGG) based on GSEA analysis indicated that the terms of linoleic acid metabolism, arachidonic acid metabolism, ether lppid metabolism, alpha linolemic acid metabolism, fc epsilon ri signaling pathway, metabolism of xenobiotics by cytochrome P450, long term depression, glycerophospholipid metabolism, tyrosine metabolism were significantly enriched in the patients with high logistics score (Supplementary Figure S2).
FIGURE 4. Biological enrichment analysis. Notes: (A) GSEA analysis between high and low logistics score based on the Hallmark gene set; (B) GO analysis between high and low logistics score.
The quantified immune cell in the tumor microenvironment of each LUAD patient was shown in Figure 5A. Correlation analysis showed that logistics score was positively correlated with NK cells activated, monocytes, macrophages M2, dendritic cells activated, and eosinophils, while negatively related to T cells CD4 memory activated, Tregs and mast cells activated (Figure 5B). Subsequently, the infiltration level of 22 immune cells in the high and low logistics score subgroups were shown in Figure 5C. Also, correlation analysis showed that TMB index and EREG-mRNAsi scores were higher in the low logistics score group, while MSI scored was higher in the high logistics score patients (Figures 5D–G).
FIGURE 5. Immune infiltration analysis. Notes: (A) The CIBERSORT algorithm was utilized to quantify the immune infiltration in the LUAD tumor microenvironment; (B) Correlation of logistics score and quantified immune cells; (C) Infiltration level of quantified immune cells in patients with high and low logistics score; (D–G): Level of TMB, MSI, mRNAsi and EREG-mRNAsi in patients with high and low logistics score.
Univariate Cox regression analysis suggested that ERVH48-1 was significantly associated with the clinical performance of LUAD patients, suggesting that this gene could affect both the immune response and the progression of LUAD (Figure 6A). Kaplan-Meier survival curves also indicated the significant effect of ERVH48-1 on patients disease-specific survival and progression-free survival (Figures 6B,C). Subsequently, the expression of ERVH48-1 in patients with different clinical features was detected, and it was found that the expression of ERVH48-1 was increased in patients with distant metastasis (Figures 6D–I). We performed pathway enrichment analysis and found that ERVH48-1 mainly changed related pathways such as pancreas beta cells, spermatogenesis, G2M checkpoint and KRAS signaling (Figure 6J).
FIGURE 6. Further exploration of ERVH48-1. Notes: (A) Univariate Cox regression analysis of the characteristic genes; (B,C): Difference in disease-specific survival and progression-free survival in patients with high and low logistics score; (D–I): The expression of ERVH48-1 in populations with different clinical characteristics; (J) Pathway enrichment analysis of ERVH48-1.
Based on the online website, we evaluated the expression levels of ERVH48-1 in different cells. ERHV48-1 was mainly expressed in tumor cells and mast cells (Figures 7A,B). Moreover, we found that the expression of key immune checkpoints was decreased in the patients with high ERHV48-1 expression (Figure 7C). Results of qRT-PCR showed that ERVH48-1 was upregulated in lung cancer cells (Supplementary Figure S3).
FIGURE 7. Single-cell analysis of ERVH48-1. Notes: (A,B): The ERVH48-1 expression in different cell subgroups; (C): The level of key immune checkpoints in patients with high and low logistics score.
In our investigation, we collected the TCGA-LUAD dataset to construct prognostic immunotherapy response features. The signature consisted of 18 immune response-related genes. Fain et al. observed that the downstream promoter in tumor cells overlaps with the DNA methylation site, and then activates the hypermethylation of its long transcript, resulting in a similar regional DNA hypermethylation pattern, including tumor suppressor genes (Fain et al., 2021). Another investigation demonstrated that the expression of PLPP4 in lung cancer patients with a higher malignant degree was also increased (Zhang et al., 2017). In our paper, a model consisting of 18 immune response characteristic genes can efficiently predict the clinical response of LUAD patients to immunotherapy.
In general, previous prognostic stratification prediction models are based on the real characteristics of tumors, like clinical TNM stage, tumor texture, vascular growth distribution and nerve infiltration. Certain components of innate and adaptive immunity were also actively involved in the progression of cancer (Rolfo et al., 2021). An investigation has shown that the immunological profiles (type, location and number of tumor-infiltrating immune cells) were a better predictor of patient survival than traditional histopathology in colon cancer (Dejima et al., 2021). The “Immunoscore” was a quantitative tool for predicting tumor immunogenicity. In addition to the current histopathological staging system, it is undergoing clinical research on a variety of cancer types (Pfirschke et al., 2016; Della Corte et al., 2020). In addition to determining tumor immune microenvironment from RNA sequencing data, immune characteristics can be used to predict patient clinical performance. In addition to the survival rate of patients, this immune characteristic was also a predictor of response to ICI treatment. In our investigation, patients with low logistics score had poor responses to immunotherapy, suggesting that for the selection of patients before ICI treatment, these 18 immune response-related characteristic genes may be useful. In the process of selecting patients for ICI treatment, PD-L1 expression, TMB, mRNAsi, and EREG-mRNAsi have been measured (Li et al., 2021a). The predictive performance of this immune feature was not related to mRNAsi. On the contrary, we found a significant decrease in TMB in the high logistics score group. Due to logistics score being a complex model with multiple variables, we believe that other variables may help to improve the prediction effect of logistics score groups.
Based on the cancer-immune cycle hypothesis, the anti-tumor effect involved many gradual processes (Duhen et al., 2018). Cancer develops when some steps of the process are hindered, including an increase in immune checkpoint expression, impaired T-cell infiltration, and antigen regulation (Li et al., 2021b). As a result, patients may benefit little from ICI treatment when immune checkpoints are not the only rate-limiting step. In our investigation, patients with high logistics score had higher immune checkpoint molecules level. It can be inferred from the higher levels of immune checkpoint molecules that the high logistics score group already possessed T cell activation. Therefore, patients with high logistics scores may be more sensitive to ICI treatment. In the clinical, the application of our logistics model might contribute to the therapy section of the individual patient.
Although some positive results have been achieved, there were still some limitations. Firstly, this immune feature was constructed based on public data sets. The predictive ability needs to be further verified in randomized controlled cohorts. In addition, we used the logistics score to simulate the patients response to ICI treatment. But there are not enough immunotherapy cohorts to validate our model, so the logistics score still cannot completely replace the real treatment response.
Publicly available datasets were analyzed in this study. This data can be found here: https://portal.gdc.cancer.gov/.
XZ, SF, and XZ performed the analysis. JS designed this work. All authors participated in the article and agreed to contribute.
This study is supported by “The Effect of acupuncture on skeletal muscle depletion in advanced lung cancer cachexia and its effect on the expression of inflammatory factors (2020ZY3567)”.
The authors declare that the research was conducted in the absence of any commercial or financial relationships that could be construed as a potential conflict of interest.
All claims expressed in this article are solely those of the authors and do not necessarily represent those of their affiliated organizations, or those of the publisher, the editors and the reviewers. Any product that may be evaluated in this article, or claim that may be made by its manufacturer, is not guaranteed or endorsed by the publisher.
The Supplementary Material for this article can be found online at: https://www.frontiersin.org/articles/10.3389/fgene.2022.1047435/full#supplementary-material
Chen, B., Khodadoust, M. S., Liu, C. L., Newman, A. M., and Alizadeh, A. A. (2018). Profiling tumor infiltrating immune cells with cibersort. Methods Mol. Biol., 1711, 243–259. doi:10.1007/978-1-4939-7493-1_12
Chen, H., Carrot-Zhang, J., Zhao, Y., Hu, H., Freeman, S. S., and Yu, S., (2019). Genomic and immune profiling of pre-invasive lung adenocarcinoma. Nat. Commun. 10 (1), 5472. doi:10.1038/s41467-019-13460-3
Connolly, K. A., Kuchroo, M., Venkat, A., Khatun, A., Wang, J., and William, I., (2021). A reservoir of stem-like Cd8(+) T cells in the tumor-draining lymph node preserves the ongoing antitumor immune response. Sci. Immunol. 6 (64), eabg7836. Epub 2021/10/02. doi:10.1126/sciimmunol.abg7836
Dejima, H., Hu, X., Chen, R., Zhang, J., Fujimoto, J., and Parra, E. R., (2021). Immune evolution from preneoplasia to invasive lung adenocarcinomas and underlying molecular features. Nat. Commun. 12 (1), 2722. Epub 2021/05/13. doi:10.1038/s41467-021-22890-x
Della Corte, C. M., Sen, T., Gay, C. M., Ramkumar, K., Diao, L., and Cardnell, R. J., (2020). Sting pathway expression identifies nsclc with an immune-responsive phenotype. J. Thorac. Oncol. 15 (5), 777–791. Epub 2020/02/19. doi:10.1016/j.jtho.2020.01.009
Denisenko, T. V., Budkevich, I. N., and Zhivotovsky, B. (2018). Cell death-based treatment of lung adenocarcinoma. Cell. Death Dis. 9 (2), 117. Epub 2018/01/27. doi:10.1038/s41419-017-0063-y
Deo, R. C. (2015). Machine learning in medicine. Circulation 132 (20), 1920–1930. Epub 2015/11/18. doi:10.1161/circulationaha.115.001593
Duhen, T., Duhen, R., Montler, R., Moses, J., Moudgil, T., and de Miranda, N. F (2018). Co-expression of Cd39 and Cd103 identifies tumor-reactive Cd8 T cells in human solid tumors. Nat. Commun. 9 (1), 2724. Epub 2018/07/15. doi:10.1038/s41467-018-05072-0
Fain, J. S., Loriot, A., Diacofotaki, A., Van Tongelen, A., and De Smet, C. (2021). Transcriptional overlap links DNA hypomethylation with DNA hypermethylation at adjacent promoters in cancer. Sci. Rep. 11 (1), 17346. Epub 2021/09/01. doi:10.1038/s41598-021-96844-0
Foggetti, G., Li, C., Cai, H., Hellyer, J. A., Lin, W. Y., Ayeni, D., et al. (2021). Genetic determinants of egfr-driven lung cancer growth and therapeutic response in vivo. Cancer Discov. 11 (7), 1736–1753. Epub 2021/03/13. doi:10.1158/2159-8290.Cd-20-1385
Fu, J., Li, K., Zhang, W., Wan, C., Zhang, J., and Jiang, P., (2020). Large-scale public data reuse to model immunotherapy response and resistance. Genome Med. 12 (1), 21. Epub 2020/02/28. doi:10.1186/s13073-020-0721-z
Li, N., Li, Y., Zheng, P., and Zhan, X. (2021). Cancer stemness-based prognostic immune-related gene signatures in lung adenocarcinoma and lung squamous cell carcinoma. Front. Endocrinol. 12, 755805. Epub 2021/11/09. doi:10.3389/fendo.2021.755805
Li, Y., Shen, R., Wang, A., Zhao, J., Zhou, J., and Zhang, W., (2021). Construction of a prognostic immune-related lncrna risk model for lung adenocarcinoma. Front. Cell. Dev. Biol. 9, 648806. Epub 2021/04/20. doi:10.3389/fcell.2021.648806
Malta, T. M., Sokolov, A., Gentles, A. J., Burzykowski, T., Poisson, L., and Weinstein, J. N., (2018). Machine learning identifies stemness features associated with oncogenic dedifferentiation. Cell. 173 (2), 338–354. e15. Epub 2018/04/07. doi:10.1016/j.cell.2018.03.034
Nguyen, B., Fong, C., Luthra, A., Smith, S. A., DiNatale, R. G., and Nandakumar, S. (2022). Genomic characterization of metastatic patterns from prospective clinical sequencing of 25, 000 patients. Cell. 185, 563–575. doi:10.1016/j.cell.2022.01.003
Peng, D. H., Rodriguez, B. L., Diao, L., Chen, L., Wang, J., and Byers, L. A., (2020). Collagen promotes anti-Pd-1/Pd-L1 resistance in cancer through lair1-dependent Cd8(+) T cell exhaustion. Nat. Commun. 11 (1), 4520. Epub 2020/09/11. doi:10.1038/s41467-020-18298-8
Pfirschke, C., Engblom, C., Rickelt, S., Cortez-Retamozo, V., Garris, C., and Pucci, F., (2016). Immunogenic chemotherapy sensitizes tumors to checkpoint blockade therapy. Immunity 44 (2), 343–354. Epub 2016/02/14. doi:10.1016/j.immuni.2015.11.024
Ren, X., Chen, X., Zhang, X., Jiang, S., Zhang, T., and Li, G., (2021). Immune microenvironment and response in prostate cancer using large population cohorts. Front. Immunol. 12, 686809. Epub 2021/11/16. doi:10.3389/fimmu.2021.686809
Rolfo, C., Mack, P., Scagliotti, G. V., Aggarwal, C., Arcila, M. E., and Barlesi, F., (2021). Liquid biopsy for advanced nsclc: A consensus statement from the international association for the study of lung cancer. J. Thorac. Oncol. 16 (10), 1647–1662. Epub 2021/07/12. doi:10.1016/j.jtho.2021.06.017
Santarpia, M., Aguilar, A., Chaib, I., Cardona, A. F., Fancelli, S., and Laguia, F., (2020). Non-small-cell lung cancer signaling pathways, metabolism, and Pd-1/Pd-L1 antibodies. Cancers 12 (6), E1475. Epub 2020/06/11. doi:10.3390/cancers12061475
Shao, L., He, Q., Wang, J., He, F., Lin, S., and Wu, L., (2021). Microrna-326 attenuates immune escape and prevents metastasis in lung adenocarcinoma by targeting Pd-L1 and B7-H3. Cell. Death Discov. 7 (1), 145. Epub 2021/06/17. doi:10.1038/s41420-021-00527-8
Skoulidis, F., Goldberg, M. E., Greenawalt, D. M., Hellmann, M. D., Awad, M. M., and Gainor, J. F., (2018). Stk11/Lkb1 mutations and Pd-1 inhibitor resistance in kras-mutant lung adenocarcinoma. Cancer Discov. 8 (7), 822–835. Epub 2018/05/19. doi:10.1158/2159-8290.Cd-18-0099
Subramanian, A., Tamayo, P., Mootha, V. K., Mukherjee, S., Ebert, B. L., and Gillette, M. A., (2005). Gene set enrichment analysis: A knowledge-based approach for interpreting genome-wide expression profiles. Proc. Natl. Acad. Sci. U. S. A. 102 (43), 15545–15550. Epub 2005/10/04. doi:10.1073/pnas.0506580102
Sun, X., Li, C. W., Wang, W. J., Chen, M. K., Li, H., and Lai, Y. J., (2020). Inhibition of C-met upregulates Pd-L1 expression in lung adenocarcinoma. Am. J. Cancer Res. 10 (2), 564–571. Epub 2020/03/21.
Tumbrink, H. L., Heimsoeth, A., and Sos, M. L. (2021). The next tier of egfr resistance mutations in lung cancer. Oncogene 40 (1), 1–11. Epub 2020/10/17. doi:10.1038/s41388-020-01510-w
Wang, S., Shi, J., Ye, Z., Dong, D., Yu, D., and Zhou, M., (2019). Predicting egfr mutation status in lung adenocarcinoma on computed tomography image using deep learning. Eur. Respir. J. 53 (3), 1800986. Epub 2019/01/13. doi:10.1183/13993003.00986-2018
Wang, Y., Yang, N., Zhang, Y., Li, L., Han, R., and Zhu, M., (2020). Effective treatment of lung adenocarcinoma harboring egfr-activating mutation, T790m, and cis-C797s triple mutations by brigatinib and cetuximab combination therapy. J. Thorac. Oncol. 15 (8), 1369–1375. Epub 2020/05/01. doi:10.1016/j.jtho.2020.04.014
Yu, G., Wang, L. G., Han, Y., and He, Q. Y. (2012). Clusterprofiler: An R package for comparing biological themes among gene clusters. Omics a J. Integr. Biol. 16 (5), 284–287. Epub 2012/03/30. doi:10.1089/omi.2011.0118
Yu, Y., Wang, Z., Zheng, Q., and Li, J. (2021). Greb1l overexpression correlates with prognosis and immune cell infiltration in lung adenocarcinoma. Sci. Rep. 11 (1), 13281. Epub 2021/06/26. doi:10.1038/s41598-021-92695-x
Zhang, C., Zhang, Z., Sun, N., Zhang, Z., Zhang, G., and Wang, F., (2020). Identification of a costimulatory molecule-based signature for predicting prognosis risk and immunotherapy response in patients with lung adenocarcinoma. Oncoimmunology 9 (1), 1824641. Epub 2021/01/19. doi:10.1080/2162402x.2020.1824641
Zhang, W., Yao, S., Huang, H., Zhou, H., Zhou, H., and Wei, Q., (2021). Molecular subtypes based on ferroptosis-related genes and tumor microenvironment infiltration characterization in lung adenocarcinoma. Oncoimmunology 10 (1), 1959977. Epub 2021/09/17. doi:10.1080/2162402x.2021.1959977
Keywords: lung adenocarcinoma, immunotherapy response, ERVH48-1, tumor environment, predictive model
Citation: Zhan X, Feng S, Zhou X, Liao W, Zhao B, Yang Q, Tan Q and Shen J (2022) Immunotherapy response and microenvironment provide biomarkers of immunotherapy options for patients with lung adenocarcinoma. Front. Genet. 13:1047435. doi: 10.3389/fgene.2022.1047435
Received: 18 September 2022; Accepted: 17 October 2022;
Published: 25 October 2022.
Edited by:
Jie Mei, Wuxi People’s Hospital Affiliated to Nanjing Medical University, ChinaCopyright © 2022 Zhan, Feng, Zhou, Liao, Zhao, Yang, Tan and Shen. This is an open-access article distributed under the terms of the Creative Commons Attribution License (CC BY). The use, distribution or reproduction in other forums is permitted, provided the original author(s) and the copyright owner(s) are credited and that the original publication in this journal is cited, in accordance with accepted academic practice. No use, distribution or reproduction is permitted which does not comply with these terms.
*Correspondence: Jian Shen, c3dvcmRfU2hlbkAxNjMuY29t
†These authors have contributed equally to this work
Disclaimer: All claims expressed in this article are solely those of the authors and do not necessarily represent those of their affiliated organizations, or those of the publisher, the editors and the reviewers. Any product that may be evaluated in this article or claim that may be made by its manufacturer is not guaranteed or endorsed by the publisher.
Research integrity at Frontiers
Learn more about the work of our research integrity team to safeguard the quality of each article we publish.