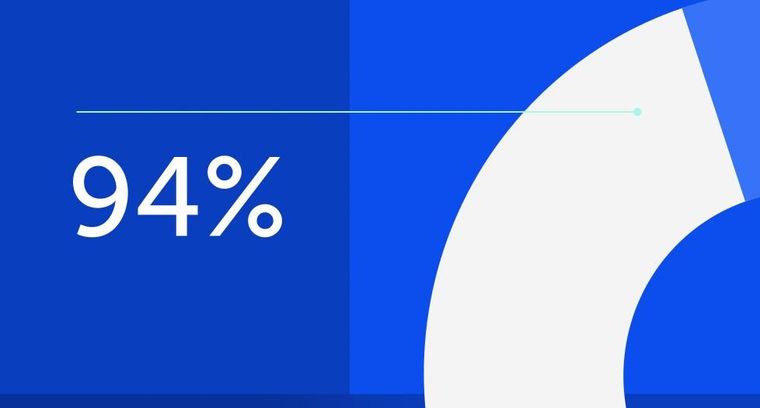
94% of researchers rate our articles as excellent or good
Learn more about the work of our research integrity team to safeguard the quality of each article we publish.
Find out more
ORIGINAL RESEARCH article
Front. Genet., 04 January 2023
Sec. Computational Genomics
Volume 13 - 2022 | https://doi.org/10.3389/fgene.2022.1032382
This article is part of the Research TopicSystematic Identification of Novel Diagnostic and Prognostic Tumor Biomarkers Based on Multi-Omics Data Analysis of Solid TumorsView all 22 articles
Background: IGSF10 is a member of the immunoglobulin superfamily. Over the previous decade, growing proof has validated definitive correlations between individuals of the immunoglobulin superfamily and human diseases. However, the function of IGSF10 in pan-cancer stays unclear. We aimed to analyze the immunological and prognostic value of IGSF10 in pan-cancer.
Methods: We utilized a vary of bioinformatic ways to inspect the function of IGSF10 in pan-cancer, including its correlation with prognosis, immune cell infiltration, tumor mutational burden (TMB), microsatellite instability (MSI), mismatch repair (MMR), DNA methyltransferases, genetic alteration, drug sensitivity, etc.
Results: We noticed low expression of IGSF10 in most cancer types. IGSF10 expression in tumor samples correlates with prognosis in most cancers. In most cancer types, IGSF10 expression was strongly related to immune cells infiltration, immune checkpoints, immune modulators, TMB, MSI, MMR, and DNA methyltransferases, among others. Functional enrichment analyses indicated that IGSF10 expression was involved in lymphocyte differentiation, cell molecules adhesion, etc. Furthermore, low IGSF10 expression could increase the drug sensitivity of many drugs.
Conclusion: IGSF10 could serve as a novel prognostic marker and attainable immunotherapy target for several malignancies.
Cancer has grown to be one of the principal causes of human death in every country around the world and a vital obstacle for countries in the world to increase human life expectancy (Sung et al., 2021). In 2020, greater than 19 million humans have been recognized with cancer, and almost 10 million human beings have died from cancer in countries around the world. Some researchers predict that by 2040, there will be about 28 million new instances of cancer and 16 million deaths due to cancer, respectively (Mao et al., 2022). Molecular profiling of tumor tissues from patients in various cancer types has been notably and intensively studied over the previous few years, and these studies have driven rapid advances in “personalized” or “precision” medicine (El-Deiry et al., 2019). As the attentions and efforts in precision care increase, there is a recognition not only of the importance of biomarkers, but also how they can be used for targeted therapies in clinical research is critical (Park et al., 2020).
Immunoglobulin superfamily (IgSF) proteins include many members, which are widely present in most types of cells, and this family of proteins is a class of cell surface proteins with multiple functions (Wojtowicz et al., 2020). IGSF10 is a member of IGSF with multiple biological functions, and its gene mutation affects the migration of gonadotropin-releasing hormone neuronal, which can lead to delayed puberty (Howard et al., 2016). To date, little has been reported about the role of IGSF10 in tumors. Previous study reported that significantly reduced expression of IGSF10 was detected in a radiation-induced rat osteosarcoma model (Daino et al., 2009). A recent study investigated that IGSF10 expression was considerably reduced in lung cancer, and knockdown of IGSF10 promoted malignant progression of lung cancer cells (Ling et al., 2020). Furthermore, another research determined that the expression of IGSF10 was down-regulated in breast cancer tissues, and IGSF10 expression was related to good prognosis (Wu et al., 2021). Although researchers have conducted multiple studies on IGSF10, until now, the role of IGSF10 in most cancers has remained largely unknown.
To this end, our research is the first to perform a pan-cancer analysis of IGSF10 based on multiple databases to comprehensively understand the function of IGSF10 in pan-cancer. We analyzed the IGSF10 expression in different tumor tissues and normal tissues. We further investigated the prognostic value of IGSF10 and the correlations between IGSF10 and immune cells infiltration, immune-related genes, TMB, MSI, MMR, and drug sensitivity. Furthermore, we performed functional enrichment analysis of IGSF10-related genes to expose the potential molecular pathogenesis of various cancers. Taken together, the results of our study suggest that IGSF10 can act as a novel prognostic marker and potential immunotherapy target for several malignancies.
Firstly, we downloaded gene expression information of different normal tissues from the GTEx database. We obtained gene expression information for various tumor cell lines in 29 tissues from the CCLE database. Gene expression information from 33 different tumor tissues in the TCGA database were analyzed using online software UALCAN. We obtained the RNA-seq information of different tumor and paired normal specimens of TCGA dataset uniformly processed by the UCSC XENA database through the Xian Tao academic tools (https://www.xiantao.love/). We also obtained the RNA-seq information of different tumor and normal specimens of TCGA and GTEx datasets uniformly processed by the UCSC XENA database through the Xian Tao academic tools. RNA-seq information in TPM format were log2 transformed and then analyzed. Data analysis was performed using R software (version 3.6.3), and the R package “ggplot2 (version 3.3.3)” was utilized for visualization. We analyzed the expression differences of IGSF10 gene in pan-cancer tissues of different cancer stages and normal tissues by using the online software UALCAN.
We acquired RNA-seq information from the TCGA database through Xian Tao academic tools. RNA-seq information in FPKM format was transformed to TPM format and log2 transformation was conducted, while retaining samples with clinical information. We selected overall survival (OS), disease-specific survival (DSS) and progression-free interval (PFI) to examine the relationships between IGSF10 expression and prognostic information in cancer patients. Survival analyses were performed for patients with different tumor types by utilizing univariate Cox regression analysis. Data were analyzed by utilizing R software (version 3.6.3), R package “survival (version 3.2–10)” was utilized for statistical analysis of survival data, and R package “survminer (version 0.4.9)” was utilized for visualization. Furthermore, we additionally analyzed the relationships between IGSF10 expression and OS in a vary of cancers using Kaplan-Meier online tool. Long-term Outcome and Gene Expression Profiling Database of pan-cancers (LOGpc) was used to study the relationships between IGSF10 expression and prognosis in GSE13507, GSE31684, GSE20685, GSE31448, GSE31210, GSE3141, GSE41271, GSE62254, GSE29623, and GSE40967.
We obtained data on the correlations between expression of IGSF10 and immune cell infiltration in pan-cancer from the TCGA database through Assistant for Clinical Bioinformatics platform. The R software package “immunedeconv” and the TIMER algorithm were used to estimate immune cell infiltration levels. The expression data of eight common immune checkpoint-related genes have been extracted, and correlations between IGSF10 expression and the expression of immune checkpoint-related genes have been noticed. R software program (version 4.0.3) was utilized to operate statistical analysis on obtained data. RNA-seq information for different tumors from the TCGA database have been downloaded through the Xian Tao academic tools. The stromal score, immune score and ESTIMATE score of multiple cancers have been obtained via R package “estimate (version 1.0.13)”.
In addition, we downloaded data on correlations between expression of IGSF10 and expression of immune-related genes from the TIMER 2.0 online website, particularly involving genes encoding immune stimulators, immune inhibitors, and major histocompatibility complex (MHC) molecules. The visualization results have been presented via R package “ggplot2 (version 3.3.3)”.
Finally, we downloaded data on correlations between expression of IGSF10 and TMB, MSI through Assistant for Clinical Bioinformatics platform. The correlations between expression of IGSF10 and TMB, MSI have been assessed by Spearman correlation analysis. The visualization results were presented by the R package “ggradar (version 0.2)” and “ggplot2 (version 3.3.3)”.
We downloaded the information on the correlations between IGSF10 expression and five DNA repair genes (MLH1, MSH2, MSH6, PMS2, EPCAM), four methyltransferases genes (DNMT1, DNMT3L, DNMT3A, DNMT3B) from the TIMER 2.0 online website. The correlations between IGSF10 expression and five DNA repair genes, four methyltransferases genes were assessed by way of Spearman correlation analysis. The visualization outcomes have been presented by R package “ggplot2 (version 3.3.3)”.
GSCA is an integrated platform, which integrates over 10,000 multi-dimensional genomic information across 33 kinds of tumors from TCGA. We used the GSCA online platform to analyze IGSF10 mutations in different cancers, such as Single Nucleotide Variation (SNV), Copy Number Variation (CNV), and Methylation. Moreover, we also analyzed the relationships between IGSF10 gene alterations and prognosis in different tumors using the GSCA.
We performed drug sensitivity analysis using the GSCA database, which integrated the gene expression profile and drug sensitivity information in Genomics of Drug Sensitivity in Cancer (GDSC) and The Cancer Therapeutics Response Portal (CTRP) for investigation. The correlations between IGSF10 expression and drug IC50 were assessed by Pearson correlation analysis.
We used the GeneMANIA database to analyze IGSF10 interacting proteins and obtained 20 IGSF10-binding proteins. Furthermore, we obtained the top 1000 IGSF10-related genes based on TCGA data through the GEPIA2 database. We utilized GEPIA2 to examine correlations between IGSF10 expression and the top five IGSF10-related genes, and correlations between IGSF10 expression and the top five IGSF10-related genes was assessed by Pearson correlation analysis. Two datasets were combined to operate GO enrichment and KEGG pathway analysis. The R package “clusterProfiler (version 3.14.3)” was utilized to operate GO enrichment and KEGG pathway analysis. The R package “org.Hs.eg.db (version 3.10.0)” was utilized to operate ID conversion.
To elucidate the physiological expression of IGSF10 in normal specimens, we explored expression levels of IGSF10 in different normal specimens using the GTEx database and ranked them from high to low. The results showed that IGSF10 expression was highest in ovarian tissue, and the lowest in whole blood (Figure 1A). Subsequently, we obtained and analyzed expression data for every tumor cell line from the CCLE database, and there have been variations in expression amongst the cell lines for 29 tumors (Figure 1B). Next, we analyzed IGSF10 expression in a range of tumor tissues from the TCGA database. IGSF10 was expressed differently in 33 tumor tissues, with the highest expression in Acute myeloid leukemia (LAML) (Figure 1C). Furthermore, we analyzed and compared the IGSF10 expression levels between different tumors and paired normal specimens. Significant variations in IGSF10 expression between tumors and paired normal specimens were detected in 14 tumors, except for these tumors without paired normal specimen data. Most tumor tissues had lower IGSF10 expression than paired normal tissues, such as Bladder urothelial carcinoma (BLCA), Breast invasive carcinoma (BRCA), Colon adenocarcinoma (COAD), Head and neck squamous cell carcinoma (HNSC), Kidney chromophobe (KICH), Kidney renal clear cell carcinoma (KIRC), Kidney renal papillary cell carcinoma (KIRP), Liver hepatocellular carcinoma (LIHC), Lung adenocarcinoma (LUAD), Lung squamous cell carcinoma (LUSC), Prostate adenocarcinoma (PRAD), Stomach adenocarcinoma (STAD), Thyroid carcinoma (THCA) and Uterine corpus endometrial carcinoma (UCEC). The Cholangiocarcinoma (CHOL), Esophageal carcinoma (ESCA), Pancreatic adenocarcinoma (PAAD) and Rectum adenocarcinoma (READ) cohorts had similar IGSF10 expression in contrast to paired normal specimens (Figure 1D).
FIGURE 1. Differential expression of IGSF10. (A) Expression of IGSF10 in normal specimens. (B) Expression of IGSF10 in a vary of cancer cell lines. (C) Expression of IGSF10 in 33 kinds of cancer. (D) Comparison of IGSF10 expression between tumor and paired normal specimens. (E) Comparison of IGSF10 expression between tumor and normal specimens. ns, p ≥ 0.05, *p < 0.05, **p < 0.01, ***p < 0.001.
Finally, we integrated RNA-seq information to analyze the IGSF10 expression in a vary of cancer and normal samples (Figure 1E). Thereinto, IGSF10 levels were downregulated in Adrenocortical carcinoma (ACC), BLCA, BRCA, Cervical squamous cell carcinoma and endocervical adenocarcinoma (CESC), COAD, ESCA, HNSC, KICH, KIRC, KIRP, LIHC, LUAD, LUSC, Ovarian serous cystadenocarcinoma (OV), PRAD, READ, Skin cutaneous melanoma (SKCM), STAD, Testicular germ cell tumors (TGCT), THCA, and UCEC. In contrast, IGSF10 had higher expression levels in tumors relative to normal samples in Lymphoid neoplasm diffuse large B-cell lymphoma (DLBC), Glioblastoma multiforme (GBM), LAML, Brain lower grade glioma (LGG), PAAD and Thymoma (THYM). However, there was no notable change in the expression level of IGSF10 between CHOL, Pheochromocytoma and paraganglioma (PCPG), Uterine carcinosarcoma (UCS), and non-tumor tissues.
To study IGSF10 expression levels across various tumor stages, we used the UALCAN online tool to compare IGSF10 expression in tumor samples from patients at various tumor stages. We observed that the IGSF10 was significantly reduced in the early tumor stages of 14 cancers (Figure 2), inclusive of BLCA, BRCA, COAD, HNSC, KICH, KIRC, KIRP, LIHC, LUAD, LUSC, READ, STAD, THCA, and UCEC, suggesting that IGSF10 may have vital guiding magnitude for the early diagnosis of sufferers with these kinds of tumors.
FIGURE 2. (Continued). Correlations between the IGSF10 expression and tumor stage. ns, p ≥ 0.05, *p < 0.05, **p < 0.01, ***p < 0.001.
To understand the prognostic value of IGSF10 in a vary of cancers, we investigated correlations between IGSF10 expression and OS of tumor sufferers by single variate Cox regression analysis. Our outcomes confirmed that IGSF10 expression were drastically associated with OS in BLCA, BRCA, LUAD, KICH, LAML, LGG, CESC, Osteosarcoma (OS), SARC, STAD, THCA, and UCEC. Furthermore, IGSF10 was a low-risk gene in BRCA, LUAD, and Osteosarcoma (OS), while it was a high-risk gene in BLCA, KICH, LAML, LGG, CESC, SARC, STAD, THCA, and UCEC (Figure 3).
FIGURE 3. Correlations between the IGSF10 expression and overall survival (OS). (A) Forest plot of results from the univariate survival analysis in pan-cancer for OS. (B–M) Kaplan-Meier survival curves showed associations between the IGSF10 expression and OS.
Furthermore, we additionally examined correlations between IGSF10 expression and DSS in a vary of cancers. Our findings confirmed that excessive IGSF10 expression was a perilous factor for DSS in BLCA, LGG, SARC, STAD and UCEC. Interestingly, it was a beneficial factor in BRCA, LUAD and LUSC (Figure 4).
FIGURE 4. Correlations between the IGSF10 expression and disease-specific survival (DSS). (A) Forest plot of results from the univariate survival analysis in pan-cancer for DSS. (B–I) Kaplan-Meier survival curves showed associations between the IGSF10 expression and DSS.
Next, correlations between IGSF10 expression and PFI were also analyzed by Cox regression analysis. Our findings confirmed that excessive IGSF10 expression was correlated with terrible PFI of cancer sufferers in BLCA, COAD and KICH, while low IGSF10 expression was correlated with bad PFI of cancer sufferers in BRCA, LUAD, LUSC, and TGCT (Figure 5).
FIGURE 5. Correlations between the IGSF10 expression and progression-free interval (PFI). (A) Forest plot of results from the univariate survival analysis in pan-cancer for PFI. (B–H) Kaplan-Meier survival curves showed associations between the IGSF10 expression and PFI.
According to the Kaplan-Meier Plotter database, we further analyzed the correlations of IGSF10 expression with OS. Our outcomes suggested that IGSF10 expression performed a detrimental function in six kinds of cancer including BLCA, CESC, SARC, STAD, THCA, and UCEC. On the contrary, IGSF10 expression owed a significant protective role in BRCA and LUAD (Figure 6). In addition, we also validated the relationships between IGSF10 expression and prognosis using the GEO dataset. We found that IGSF10 was a low-risk gene in BRCA, LUAD, and LUSC, while it was a high-risk gene in BLCA, Gastric cancer (GC), and Colorectal cancer (CRC) (Supplementary Figure S1).
FIGURE 6. Associations between IGSF10 expression and OS. (A–I) Correlations between the IGSF10 expression and OS based on Kaplan-Meier Plotter database.
Overall, the above consequences confirmed that IGSF10 could function as a prognostic predictor for several types of cancer.
Immunotherapy has become another effective cancer treatment method besides chemotherapy, surgery, radiation therapy and targeted drug therapy (Murciano-Goroff et al., 2020). Notably development has been made in the application of immune checkpoint blockade in cancer therapy in latest years (Morad et al., 2021), but research showed that the proportion of sufferers who responded to checkpoint inhibitor drugs was estimated at 0.14% in 2011, and the percentage was estimated at 12.46% in 2018 (Haslam and Prasad, 2019). Based on this status quo, the search for novel predictive biomarkers is crucial to increase the proportion of individual patients who respond to immune checkpoint inhibitor. Therefore, we investigated the correlations between IGSF10 expression and immune cell infiltration in a vary of cancers via the usage of the TIMER online database. The consequences confirmed that the IGSF10 expression was notably related to CD8+ T cell infiltration in 12 kinds of tumors, CD4+ T cell infiltration in 20 tumors, neutrophils infiltration in 19 tumors, myeloid dendritic cells infiltration in 17 tumors, macrophages infiltration in 22 tumors and B cells infiltration in 15 tumors (Figure 7A). As IGSF10 was found to show prognostic value in BLCA, BRCA, CESC, COAD, KICH, LAML, LGG, LUAD, LUSC, OS, SARC, STAD, TGCT, THCA, and UCEC, we examined stromal score, immune score and ESTIMATE score to evaluate the correlations between IGSF10 expression levels and immune infiltration in a vary of cancers. The consequences confirmed that IGSF10 expression was positively associated with the stromal score in BLCA, BRCA, COAD, KICH, LGG, LUAD, SARC, STAD, TGCT, and THCA. IGSF10 expression was negatively associated with the immune score in CESC, LUSC, and UCEC. However, it was positively associated with the immune score in BLCA, BRCA, LGG, LUAD, STAD, and THCA. Furthermore, the IGSF10 expression was notably negatively associated with ESTIMATE score in CESC, LUSC, and UCEC, while positively in BLCA, BRCA, COAD, KICH, LGG, LUAD, STAD, and THCA (Figure 7B).
FIGURE 7. IGSF10 expression was associated with cancer immunity. (A) Relationships between IGSF10 expression and immune cell infiltration in different cancers. *p < 0.05, **p < 0.01, ***p < 0.001. (B) The correlations of IGSF10 expression with the stromal score, immune score and ESTIMATE score in various cancers.
Furthermore, we analyzed correlations of IGSF10 expression with common immune checkpoint genes. Interestingly, from the heatmap (Figure 8A), we can observe that nearly all immune checkpoint genes have been related to IGSF10 in most cancer types, with the exception of CESC, LUSC, MESO, SARC, UCEC, and UVM, majority of immune checkpoint genes have been positively related to IGSF10 in all kinds of tumors.
FIGURE 8. Correlation analysis of IGSF10 and immune checkpoints, as well as immune modulators in pan-cancer. (A) The correlations of IGSF10 and common immune checkpoints in pan-cancer. (B) The correlations of IGSF10 and immune stimulators in pan-cancer. (C) The correlations of IGSF10 and immune inhibitors in pan-cancer. (D) The correlations of IGSF10 and MHC molecules in pan-cancer.
Besides, we investigated correlations between IGSF10 expression and immune-related genes encoding immune stimulators, immune inhibitors, and MHC molecules in all kinds of tumors. The outcomes of the heatmap showed that nearly all immune-related genes have been positively associated with IGSF10 in the vast majority of cancers (Figures 8B–D).
Altogether, the above data strongly support that IGSF10 performs a crucial function in tumor immunity.
TMB refers to the total quantity of genetic mutations assessed for a tumor specimen (Jardim et al., 2021). The conceptual definition of MSI is the hypermutator phenotype secondary to frequent polymorphism in short repetitive DNA sequences and single nucleotide substitution, resulting from MMR deficiency (Baretti and Le, 2018). In recent years, increasing evidence indicated that TMB and MSI function as biomarkers for predicting the immunotherapy response (Chan et al., 2019; Liu et al., 2019; Samstein et al., 2019; Yamamoto and Imai, 2019; Chen, 2022), so we examined correlations between IGSF10 and TMB, MSI. As proven in Figure 9A, IGSF10 expression was positively associated with TMB in THYM, and negatively associated in BRCA, CESC, ESCA, LUAD, STAD, THCA, and UCEC. The expression of IGSF10 was positively associated with MSI in LUSC and TGCT, however negatively associated in DLBC, PCPG, STAD, and UCEC (Figure 9B).
FIGURE 9. The correlations between IGSF10 expression and TMB, MSI. (A) Radar map of the correlations between IGSF10 expression and TMB in pan-cancer. (B) Radar map of the correlations between IGSF10 expression and MSI in pan-cancer. *p < 0.05, **p < 0.01, ***p < 0.001.
MMR is a security system in cells and an evolutionarily highly conserved biological process responsible for repairing base mismatches that occur during DNA replication (Hermans et al., 2016). Dysregulation of the MMR system can alter cellular biological functions, thereby promoting tumorigenesis or promoting the malignant progression of tumors (Germano et al., 2017). To explore the potential function of IGSF10 in tumorigenesis and development, we assessed associations of IGSF10 expression with mutation levels of five MMR genes. The outcomes confirmed that IGSF10 was noticeably positively associated with MMR genes in 27 kinds of cancers, besides CHOL, MESO, OV, STAD and TGCT (Figure 10A). We also examined relationships between IGSF10 and four DNA methyltransferases. The expression of IGSF10 was highly positively related to these four DNA methyltransferases in 24 cancers, except CHOL, KICH, MESO, OV, PCPG, STAD, TGCT, and UCS (Figure 10B). These consequences suggest that IGSF10 may affect tumorigenesis and development by means of regulating DNA repair and DNA methylation in cancers.
FIGURE 10. The associations of IGSF10 expression with MMR genes and DNA methyltransferases in pan-cancer. (A) Correlations between IGSF10 expression and fiveMMR genes (MLH1, MSH2, MSH6, PMS2, EPCAM) expression. The lower right triangle of each cell represents the correlation coefficient calculated by Spearman’s correlation test, and the upper left triangle represents the p-value. (B) Correlations between IGSF10 expression and four DNA methyltransferase genes. *p < 0.05, **p < 0.01
Firstly, the SNV information of 10,234 samples from all kinds of cancers have been gathered from TCGA database. As shown in Figure 11A, the percentage heatmap summarized the frequency of deleterious mutations in pan-cancer. SNV was found in 26 cancer types and mutations were most common in SKCM and UCEC. Furthermore, SNV of IGSF10 was related to a significantly beneficial prognosis for DFI in UCEC. SNV of IGSF10 indicated a significantly poor prognosis for DSS and OS in GBM, but the opposite trend in UCEC. The SNV of IGSF10 in PRAD indicated a significantly poor prognosis for PFS, whereas the opposite was found in UCEC (Figure 11B). We also analyzed the CNV of IGSF10 and the correlation of CNV with mRNA expression through the GSCA database (Figures 11C, D). CNV pie plot represented the proportion of different types of CNV of IGSF10 gene in each cancer. Bubble plot confirmed that CNV of IGSF10 was positively related to mRNA expression in eight cancers, including CESC, BLCA, OV, UCS, ACC, HNSC, LUSC, and ESCA. Likewise, we investigated the impact of CNV of IGSF10 on the prognosis of patients with various cancers. In UCEC, BLCA, COAD, PCPG, KIRP, LAML, LGG, PAAD, SARC, SKCM, THYM, UVM, and KIRC, the CNV of IGSF10 was associated with prognosis (Figure 11E).
FIGURE 11. (Continued). Genetic alterations of IGSF10 across different cancers from the GSCA database. (A) The profile of SNV of IGSF10 gene set in pan-cancer. (B) Bubble plot of the survival difference between mutant (deleterious) and wide type in pan-cancer. (C) Pie plot summarizes the CNV of IGSF10 gene in pan-cancer. (D) The correlations between CNV and IGSF10 mRNA expression in pan-cancer. (E) The difference of survival between CNV and wide type in pan-cancer.
Methylation of IGSF10 in a vary of cancers was analyzed via the usage of GSCA database. Bubble chart showed differences between IGSF10 gene methylation levels in various tumor and normal specimens. We found that IGSF10 was significantly hypermethylated in UCEC, LUSC, KIRP, BRCA, LUAD, and KIRC samples compared with that in normal samples (Figure 12A). Figure 12B showed the correlation results between IGSF10 mRNA expression and IGSF10 methylation levels in various tumors. Methylation of IGSF10 was negatively correlated with IGSF10 mRNA expression in PCPG, PRAD, PAAD, MESO, and UCS etc. The association between methylation of IGSF10 and prognosis in pan-cancer was further analyzed. As shown in Figure 12C, patients with hypermethylation of IGSF10 had a good prognosis in KIRP, LGG and PAAD, but the opposite trend in ACC.
FIGURE 12. DNA methylation aberration of IGSF10 across different cancers and correlation of IGSF10 expression with drug (top 30) sensitivity from the GSCA database. (A) The methylation difference between tumor and normal samples of IGSF10 gene in pan-cancer. (B) The profile of correlations between methylation and mRNA expression of IGSF10 gene in pan-cancer. (C) The overall survival difference between higher and lower methylation groups in pan-cancer. (D) The correlations between IGSF10 gene expression and the sensitivity of CTRP drugs (top 30) in pan-cancer. (E) The correlations between IGSF10 gene expression and the sensitivity of GDSC drugs (top 30) in pan-cancer.
To explore whether the IGSF10 expression was correlated with drug sensitivity, Pearson correlation analysis was used to detect correlations between IGSF10 expression and the drug sensitivity (IC50) of various anticancer drugs in the GDSC and CTRP databases. The drug sensitivity of almost all drugs shown in Figures 12D, E was negatively associated with IGSF10 mRNA expression. These data strongly advised that IGSF10 could act as a biomarker for predicting drug response.
To reveal molecular mechanism of IGSF10 in tumorigenesis and progression, we integrated IGSF10-related genes and IGSF10-binding proteins for enrichment analysis. Firstly, we obtained 20 IGSF10-binding proteins through the GeneMANIA database (Figure 13A). In addition, we got the top 1000 IGSF10 expression-related genes through GEPIA2 database. IGSF10 expression was positively related to the expression of the top five genes, including RP11-1000B6.7 (R = 0.56), MRPL42P6 (R = 0.54), AC026150.8 (R = 0.52), RP11-1000B6.3 (R = 0.48) and CHRFAM7A (R = 0.48) (Figures 13D–H). Furthermore, KEGG and GO enrichment analyses have been carried out on these IGSF10-binding proteins and IGSF10-related genes. The GO enrichment results showed that most of IGSF10-binding proteins and IGSF10-related genes were related to lymphocyte differentiation, Golgi organization, Golgi cis cisterna, and others (Figure 13B). The KEGG results further suggested that IGSF10 could adjust the occurrence and development of cancers via participating in the “cell molecules adhesion” signaling pathway (Figure 13C).
FIGURE 13. Enrichment analysis of IGSF10-interacted proteins and IGSF10-related genes. (A) IGSF10-interacted proteins from the GeneMANIA database. (B) GO enrichment analysis based on the IGSF10-interacted proteins and IGSF10-related genes. (C) KEGG enrichment analysis based on the IGSF10-interacted proteins and IGSF10-related genes. (D–H) Scatter plots of expression correlations between IGSF10 and RP11-1000B6.7, MRPL42P6, AC026150.8, RP11-1000B6.3, and CHRFAM7A in pan-cancer using the GEPIA2 database.
Cancer is caused by a variety of factors, mainly including exogenous and endogenous factors (Neveu et al., 2020). With the advent of genetic testing and the era of targeted therapy, molecular signatures have become increasingly critical, which can predict individual patients prognosis or predict individual patients response to specific treatments (Michiels et al., 2016). Hence, there is a pressing want to discover effective biomarkers to precisely assess the prognosis and effectively improve the treatment of tumor patients.
Our study aimed to comprehensively analyze function of IGSF10 in all kinds of tumors. We obtained expression data of IGSF10 in various cancers from multiple friendly public databases open to the world, which was helpful to discover variations in IGSF10 expression in a vary of cancers. Our findings indicated that IGSF10 expression were notably different between tumor and normal tissues across multiple cancer types. Among them, IGSF10 expression levels were downregulated in ACC, BLCA, BRCA, CESC, COAD, ESCA, HNSC, KICH, KIRC, KIRP, LIHC, LUAD, LUSC, OV, PRAD, READ, SKCM, STAD, TGCT, THCA, and UCEC, but the opposite trend in DLBC, GBM, LAML, LGG, PAAD, and THYM. Furthermore, we investigated the prognostic function of IGSF10 in multiple cancers via the usage of three prognostic indicators: OS, DSS and PFI. On the basis of our results, we found that IGSF10 played a beneficial role in five tumors including BRCA, LUAD, LUSC, Osteosarcoma (OS) and TGCT. In contrast, IGSF10 expression owed a significant detrimental role in BLCA, CESC, COAD, KICH, LAML, LGG, SARC, STAD, THCA, and UCEC.
Multiple researches have showed that infiltrating immune cells in tumor specimens influenced malignant tumor development (Fridman et al., 2012; Garnelo et al., 2017; Kalafati et al., 2020). More recently, a growing quantity of researches have revealed that tumor-infiltrating immune cells were closely correlated with the prognosis of tumor patients (Gooden et al., 2011; Loi et al., 2014; Schalper et al., 2015; Goode et al., 2017; Disis et al., 2019; Stewart et al., 2019). Our research showed that IGSF10 may perform a specific function in regulating tumor immunity. In our research, we provided evidence of the relationships between IGSF10 expression and immune infiltration, immune checkpoints, immune modulators, etc. in multiple tumors. We observed notably correlations between IGSF10 expression and infiltration levels of CD8+ T cells, CD4+ T cells, neutrophils, myeloid dendritic cells, macrophages and B cells in most cancer types. Furthermore, ESTIMATE was described as a method for estimating the proportion of stromal and immune cells in tumor specimens via the usage of gene expression signatures (Yoshihara et al., 2013). It is worth noting that IGSF10 expression was significantly negatively related to ESTIMATE score in CESC, LUSC, and UCEC, while positively in BLCA, BRCA, COAD, KICH, LGG, LUAD, STAD, and THCA. Our results also showed that IGSF10 was associated with almost all immune checkpoint genes in most types of tumors, IGSF10 was positively associated with majority of immune checkpoint genes in all kinds of tumors. Additionally, our study demonstrated that close to all immune-related genes were positively associated with IGSF10 expression in vast majority of tumors. These outcomes all confirmed that IGSF10 expression was closely correlated with the tumor-infiltrating immune cells, affected the prognosis of sufferers, and provided a novel target for the development of immunosuppressants.
TMB is an emerging good biomarker that has recently attracted widespread attention to predict response of individual patients to immune checkpoint inhibitor (ICI) (Samstein et al., 2019; Fumet et al., 2020; Sampson et al., 2020), which could lead immuno-oncology rapidly into the period of precision medicine (Steuer and Ramalingam, 2018). MSI is characterized by high-frequency frameshift mutations in microsatellite DNA, caused by MMR deficiency that fails to repair insertion or deletion mutations during DNA replication (Pal et al., 2008; Song et al., 2018; El-Deiry et al., 2019; McGrail et al., 2020). MMR deficiency causes both MSI and high TMB(Georgiadis et al., 2019; Willis et al., 2020). Further, MMR and MSI also may predict clinical response to ICI(Hodges et al., 2017; Le et al., 2017; Janjigian et al., 2018; Cortez, 2019). The outcomes of our research showed that IGSF10 expression was related to TMB in BRCA, CESC, ESCA, LUAD, STAD, THCA, THYM, and UCEC. Our findings also showed that IGSF10 expression was related to MSI in DLBC, LUSC, PCPG, STAD, TGCT, and UCEC. In addition, our research confirmed that IGSF10 was noticeably positively related to MMR genes in 27 kinds of tumors, besides CHOL, MESO, OV, STAD, and TGCT. Taken together, the above outcomes suggested that IGSF10 expression may affect the TMB, MSI and MMR of tumors, thereby exerting influence on the response of individual patients to ICI therapy. The above findings suggested that IGSF10 was expected to provide a valuable reference for predicting response of individual patients to immunotherapy. DNA methylation is a common, stably inherited epigenetic modification, and it can influence gene regulation (Catoni et al., 2018). Previous researches have proven that aberrant methylation is relate to tumorigenesis and immune evasion of tumors (Cheng et al., 2015; Jung et al., 2019; Zhao et al., 2020). The IGSF10 expression was noticeably positively correlated with these four DNA methyltransferases in 24 cancers, except CHOL, KICH, MESO, OV, PCPG, STAD, TGCT, and UCS. These outcomes propose that aberrant IGSF10 expression may play a critical function in tumorigenesis by regulating DNA methylation in cancers.
The genetic variations include CNVs and SNVs, which can be inherited or de novo (Mercati et al., 2017). The accumulation of SNV performs a very important function in tumorigenesis and tumor progression (Abelson et al., 2020). In addition to SNVs, DNA copy number alterations and chromosomal instability are a hallmark of cancer (Seifert et al., 2016). To date, few studies have reported on relationships between IGSF10 gene variations and cancers. In this study, we found that IGSF10 has different degrees of SNVs and CNVs in most cancer types, among which IGSF10 is most prone to SNVs in SKCM and UCEC. Furthermore, we found that IGSF10 mutations were significantly correlated with prognosis of patients in multiple tumor types.
In addition, we combined IGSF10-interacted proteins and IGSF10-related genes for enrichment analysis. Gene enrichment analysis revealed that IGSF10 may have an effect on the occurrence, progression or immunity of cancer via participating in lymphocyte differentiation and cell molecules adhesion.
In summary, our systemic pan-cancer analysis showed for the first time aberrant IGSF10 expression across different tumors. Furthermore, we found that IGSF10 can serve as a valuable prognostic biomarker for certain types of cancer. According to our findings, the level of IGSF10 is associated with cancer immunity, providing a new idea for individualized cancer immunotherapy, and is expected to be a potential immunological and prognostic biomarker.
The original contributions presented in the study are included in the article/Supplementary Material, further inquiries can be directed to the corresponding author.
YZ and MG contributed equally to this research. XW designed and supervised the research. YZ and MG performed the analysis and wrote the manuscript. YJ modified the article. All authors examined and accredited the final manuscript.
This work was funded by Tianjin Key Medical Discipline (Specialty) Construction Project (TJYXZDXK-009A).
We are very grateful to Tianjin Medical University Cancer Institute and Hospital for supporting our research.
The authors declare that the research was conducted in the absence of any commercial or financial relationships that could be construed as a potential conflict of interest.
All claims expressed in this article are solely those of the authors and do not necessarily represent those of their affiliated organizations, or those of the publisher, the editors and the reviewers. Any product that may be evaluated in this article, or claim that may be made by its manufacturer, is not guaranteed or endorsed by the publisher.
The Supplementary Material for this article can be found online at: https://www.frontiersin.org/articles/10.3389/fgene.2022.1032382/full#supplementary-material
SUPPLEMENTARY FIGURE S1 | Associations between IGSF10 expression and prognosis. (A–L) Correlations between the IGSF10 expression and prognosis based on the GEO dataset.
Abelson, S., Zeng, A. G. X., Nofech-Mozes, I., Wang, T. T., Ng, S. W. K., Minden, M. D., et al. (2020). Integration of intra-sample contextual error modeling for improved detection of somatic mutations from deep sequencing. Sci. Adv. 6 (50), eabe3722. doi:10.1126/sciadv.abe3722
Baretti, M., and Le, D. T. (2018). DNA mismatch repair in cancer. Pharmacol. Ther. 189, 45–62. doi:10.1016/j.pharmthera.2018.04.004
Catoni, M., Tsang, J. M., Greco, A. P., and Zabet, N. R. (2018). DMRcaller: A versatile R/bioconductor package for detection and visualization of differentially methylated regions in CpG and non-CpG contexts. Nucleic Acids Res. 46 (19), e114. doi:10.1093/nar/gky602
Chan, T. A., Yarchoan, M., Jaffee, E., Swanton, C., Quezada, S. A., Stenzinger, A., et al. (2019). Development of tumor mutation burden as an immunotherapy biomarker: Utility for the oncology clinic. Ann. Oncol. 30 (1), 44–56. doi:10.1093/annonc/mdy495
Chen, Y. (2022). Identification and validation of cuproptosis-related prognostic signature and associated regulatory Axis in uterine corpus endometrial carcinoma. Front. Genet. 13, 912037. doi:10.3389/fgene.2022.912037
Cheng, P. F., Shakhova, O., Widmer, D. S., Eichhoff, O. M., Zingg, D., Frommel, S. C., et al. (2015). Methylation-dependent SOX9 expression mediates invasion in human melanoma cells and is a negative prognostic factor in advanced melanoma. Genome Biol. 16 (1), 42. doi:10.1186/s13059-015-0594-4
Cortez, D. (2019). Replication-coupled DNA repair. Mol. Cell 74 (5), 866–876. doi:10.1016/j.molcel.2019.04.027
Daino, K., Ugolin, N., Altmeyer-Morel, S., Guilly, M. N., and Chevillard, S. (2009). Gene expression profiling of alpha-radiation-induced rat osteosarcomas: Identification of dysregulated genes involved in radiation-induced tumorigenesis of bone. Int. J. Cancer 125 (3), 612–620. doi:10.1002/ijc.24392
Disis, M. L., Taylor, M. H., Kelly, K., Beck, J. T., Gordon, M., Moore, K. M., et al. (2019). Efficacy and safety of avelumab for patients with recurrent or refractory ovarian cancer: Phase 1b results from the JAVELIN solid tumor trial. JAMA Oncol. 5 (3), 393–401. doi:10.1001/jamaoncol.2018.6258
El-Deiry, W. S., Goldberg, R. M., Lenz, H. J., Shields, A. F., Gibney, G. T., Tan, A. R., et al. (2019). The current state of molecular testing in the treatment of patients with solid tumors, 2019. Ca. Cancer J. Clin. 69 (4), 305–343. doi:10.3322/caac.21560
Fridman, W. H., Pagès, F., Sautès-Fridman, C., and Galon, J. (2012). The immune contexture in human tumours: Impact on clinical outcome. Nat. Rev. Cancer 12 (4), 298–306. doi:10.1038/nrc3245
Fumet, J. D., Truntzer, C., Yarchoan, M., and Ghiringhelli, F. (2020). Tumour mutational burden as a biomarker for immunotherapy: Current data and emerging concepts. Eur. J. Cancer 131, 40–50. doi:10.1016/j.ejca.2020.02.038
Garnelo, M., Tan, A., Her, Z., Yeong, J., Lim, C. J., Chen, J., et al. (2017). Interaction between tumour-infiltrating B cells and T cells controls the progression of hepatocellular carcinoma. Gut 66 (2), 342–351. doi:10.1136/gutjnl-2015-310814
Georgiadis, A., Durham, J. N., Keefer, L. A., Bartlett, B. R., Zielonka, M., Murphy, D., et al. (2019). Noninvasive detection of microsatellite instability and high tumor mutation burden in cancer patients treated with PD-1 blockade. Clin. Cancer Res. 25 (23), 7024–7034. doi:10.1158/1078-0432.Ccr-19-1372
Germano, G., Lamba, S., Rospo, G., Barault, L., Magrì, A., Maione, F., et al. (2017). Inactivation of DNA repair triggers neoantigen generation and impairs tumour growth. Nature 552 (7683), 116–120. doi:10.1038/nature24673
Goode, E. L., Block, M. S., Kalli, K. R., Vierkant, R. A., Chen, W., Fogarty, Z. C., et al. (2017). Dose-response association of CD8+ tumor-infiltrating lymphocytes and survival time in high-grade serous ovarian cancer. JAMA Oncol. 3 (12), e173290. doi:10.1001/jamaoncol.2017.3290
Gooden, M. J., de Bock, G. H., Leffers, N., Daemen, T., and Nijman, H. W. (2011). The prognostic influence of tumour-infiltrating lymphocytes in cancer: A systematic review with meta-analysis. Br. J. Cancer 105 (1), 93–103. doi:10.1038/bjc.2011.189
Haslam, A., and Prasad, V. (2019). Estimation of the percentage of US patients with cancer who are eligible for and respond to checkpoint inhibitor immunotherapy drugs. JAMA Netw. Open 2 (5), e192535. doi:10.1001/jamanetworkopen.2019.2535
Hermans, N., Laffeber, C., Cristovão, M., Artola-Borán, M., Mardenborough, Y., Ikpa, P., et al. (2016). Dual daughter strand incision is processive and increases the efficiency of DNA mismatch repair. Nucleic Acids Res. 44 (14), 6770–6786. doi:10.1093/nar/gkw411
Hodges, T. R., Ott, M., Xiu, J., Gatalica, Z., Swensen, J., Zhou, S., et al. (2017). Mutational burden, immune checkpoint expression, and mismatch repair in glioma: Implications for immune checkpoint immunotherapy. Neuro. Oncol. 19 (8), 1047–1057. doi:10.1093/neuonc/nox026
Howard, S. R., Guasti, L., Ruiz-Babot, G., Mancini, A., David, A., Storr, H. L., et al. (2016). IGSF10 mutations dysregulate gonadotropin-releasing hormone neuronal migration resulting in delayed puberty. EMBO Mol. Med. 8 (6), 626–642. doi:10.15252/emmm.201606250
Janjigian, Y. Y., Bendell, J., Calvo, E., Kim, J. W., Ascierto, P. A., Sharma, P., et al. (2018). CheckMate-032 study: Efficacy and safety of nivolumab and nivolumab plus ipilimumab in patients with metastatic esophagogastric cancer. J. Clin. Oncol. 36 (28), 2836–2844. doi:10.1200/jco.2017.76.6212
Jardim, D. L., Goodman, A., de Melo Gagliato, D., and Kurzrock, R. (2021). The challenges of tumor mutational burden as an immunotherapy biomarker. Cancer Cell 39 (2), 154–173. doi:10.1016/j.ccell.2020.10.001
Jung, H., Kim, H. S., Kim, J. Y., Sun, J. M., Ahn, J. S., Ahn, M. J., et al. (2019). DNA methylation loss promotes immune evasion of tumours with high mutation and copy number load. Nat. Commun. 10 (1), 4278. doi:10.1038/s41467-019-12159-9
Kalafati, L., Kourtzelis, I., Schulte-Schrepping, J., Li, X., Hatzioannou, A., Grinenko, T., et al. (2020). Innate immune training of granulopoiesis promotes anti-tumor activity. Cell 183 (3), 771–785. doi:10.1016/j.cell.2020.09.058
Le, D. T., Durham, J. N., Smith, K. N., Wang, H., Bartlett, B. R., Aulakh, L. K., et al. (2017). Mismatch repair deficiency predicts response of solid tumors to PD-1 blockade. Science 357 (6349), 409–413. doi:10.1126/science.aan6733
Ling, B., Liao, X., Huang, Y., Liang, L., Jiang, Y., Pang, Y., et al. (2020). Identification of prognostic markers of lung cancer through bioinformatics analysis and in vitro experiments. Int. J. Oncol. 56 (1), 193–205. doi:10.3892/ijo.2019.4926
Liu, L., Bai, X., Wang, J., Tang, X. R., Wu, D. H., Du, S. S., et al. (2019). Combination of TMB and CNA stratifies prognostic and predictive responses to immunotherapy across metastatic cancer. Clin. Cancer Res. 25 (24), 7413–7423. doi:10.1158/1078-0432.Ccr-19-0558
Loi, S., Michiels, S., Salgado, R., Sirtaine, N., Jose, V., Fumagalli, D., et al. (2014). Tumor infiltrating lymphocytes are prognostic in triple negative breast cancer and predictive for trastuzumab benefit in early breast cancer: Results from the FinHER trial. Ann. Oncol. 25 (8), 1544–1550. doi:10.1093/annonc/mdu112
Mao, J. J., Pillai, G. G., Andrade, C. J., Ligibel, J. A., Basu, P., Cohen, L., et al. (2022). Integrative oncology: Addressing the global challenges of cancer prevention and treatment. Ca. Cancer J. Clin. 72 (2), 144–164. doi:10.3322/caac.21706
McGrail, D. J., Garnett, J., Yin, J., Dai, H., Shih, D. J. H., Lam, T. N. A., et al. (2020). Proteome instability is a therapeutic vulnerability in mismatch repair-deficient cancer. Cancer Cell 37 (3), 371–386. doi:10.1016/j.ccell.2020.01.011
Mercati, O., Huguet, G., Danckaert, A., André-Leroux, G., Maruani, A., Bellinzoni, M., et al. (2017). CNTN6 mutations are risk factors for abnormal auditory sensory perception in autism spectrum disorders. Mol. Psychiatry 22 (4), 625–633. doi:10.1038/mp.2016.61
Michiels, S., Ternès, N., and Rotolo, F. (2016). Statistical controversies in clinical research: Prognostic gene signatures are not (yet) useful in clinical practice. Ann. Oncol. 27 (12), 2160–2167. doi:10.1093/annonc/mdw307
Morad, G., Helmink, B. A., Sharma, P., and Wargo, J. A. (2021). Hallmarks of response, resistance, and toxicity to immune checkpoint blockade. Cell 184 (21), 5309–5337. doi:10.1016/j.cell.2021.09.020
Murciano-Goroff, Y. R., Warner, A. B., and Wolchok, J. D. (2020). The future of cancer immunotherapy: Microenvironment-targeting combinations. Cell Res. 30 (6), 507–519. doi:10.1038/s41422-020-0337-2
Neveu, V., Nicolas, G., Salek, R. M., Wishart, D. S., and Scalbert, A. (2020). Exposome-explorer 2.0: An update incorporating candidate dietary biomarkers and dietary associations with cancer risk. Nucleic Acids Res. 48 (D1), D908–d912. doi:10.1093/nar/gkz1009
Pal, T., Permuth-Wey, J., Kumar, A., and Sellers, T. A. (2008). Systematic review and meta-analysis of ovarian cancers: Estimation of microsatellite-high frequency and characterization of mismatch repair deficient tumor histology. Clin. Cancer Res. 14 (21), 6847–6854. doi:10.1158/1078-0432.Ccr-08-1387
Park, J. J. H., Hsu, G., Siden, E. G., Thorlund, K., and Mills, E. J. (2020). An overview of precision oncology basket and umbrella trials for clinicians. Ca. Cancer J. Clin. 70 (2), 125–137. doi:10.3322/caac.21600
Sampson, J. H., Gunn, M. D., Fecci, P. E., and Ashley, D. M. (2020). Brain immunology and immunotherapy in brain tumours. Nat. Rev. Cancer 20 (1), 12–25. doi:10.1038/s41568-019-0224-7
Samstein, R. M., Lee, C. H., Shoushtari, A. N., Hellmann, M. D., Shen, R., Janjigian, Y. Y., et al. (2019). Tumor mutational load predicts survival after immunotherapy across multiple cancer types. Nat. Genet. 51 (2), 202–206. doi:10.1038/s41588-018-0312-8
Schalper, K. A., Brown, J., Carvajal-Hausdorf, D., McLaughlin, J., Velcheti, V., Syrigos, K. N., et al. (2015). Objective measurement and clinical significance of TILs in non-small cell lung cancer. J. Natl. Cancer Inst. 107 (3), dju435. doi:10.1093/jnci/dju435
Seifert, M., Friedrich, B., and Beyer, A. (2016). Importance of rare gene copy number alterations for personalized tumor characterization and survival analysis. Genome Biol. 17 (1), 204. doi:10.1186/s13059-016-1058-1
Song, W., Shen, L., Wang, Y., Liu, Q., Goodwin, T. J., Li, J., et al. (2018). Synergistic and low adverse effect cancer immunotherapy by immunogenic chemotherapy and locally expressed PD-L1 trap. Nat. Commun. 9 (1), 2237. doi:10.1038/s41467-018-04605-x
Steuer, C. E., and Ramalingam, S. S. (2018). Tumor mutation burden: Leading immunotherapy to the era of precision medicine? J. Clin. Oncol. 36 (7), 631–632. doi:10.1200/jco.2017.76.8770
Stewart, P. A., Welsh, E. A., Slebos, R. J. C., Fang, B., Izumi, V., Chambers, M., et al. (2019). Proteogenomic landscape of squamous cell lung cancer. Nat. Commun. 10 (1), 3578. doi:10.1038/s41467-019-11452-x
Sung, H., Ferlay, J., Siegel, R. L., Laversanne, M., Soerjomataram, I., Jemal, A., et al. (2021). Global cancer statistics 2020: GLOBOCAN estimates of incidence and mortality worldwide for 36 cancers in 185 countries. Ca. Cancer J. Clin. 71 (3), 209–249. doi:10.3322/caac.21660
Willis, J. A., Reyes-Uribe, L., Chang, K., Lipkin, S. M., and Vilar, E. (2020). Immune activation in mismatch repair-deficient Carcinogenesis: More than just mutational rate. Clin. Cancer Res. 26 (1), 11–17. doi:10.1158/1078-0432.Ccr-18-0856
Wojtowicz, W. M., Vielmetter, J., Fernandes, R. A., Siepe, D. H., Eastman, C. L., Chisholm, G. B., et al. (2020). A human IgSF cell-surface interactome reveals a complex network of protein-protein interactions. Cell 182 (4), 1027–1043. doi:10.1016/j.cell.2020.07.025
Wu, M., Li, Q., and Wang, H. (2021). Identification of novel biomarkers associated with the prognosis and potential pathogenesis of breast cancer via integrated bioinformatics analysis. Technol. Cancer Res. Treat. 20, 1533033821992081. doi:10.1177/1533033821992081
Yamamoto, H., and Imai, K. (2019). An updated review of microsatellite instability in the era of next-generation sequencing and precision medicine. Semin. Oncol. 46 (3), 261–270. doi:10.1053/j.seminoncol.2019.08.003
Yoshihara, K., Shahmoradgoli, M., Martínez, E., Vegesna, R., Kim, H., Torres-Garcia, W., et al. (2013). Inferring tumour purity and stromal and immune cell admixture from expression data. Nat. Commun. 4, 2612. doi:10.1038/ncomms3612
Keywords: IGSF10, pan-cancer, prognosis, immune infiltration, TMB, MSI, drug sensitivity
Citation: Zhou Y, Gao M, Jing Y and Wang X (2023) Pan-cancer analyses reveal IGSF10 as an immunological and prognostic biomarker. Front. Genet. 13:1032382. doi: 10.3389/fgene.2022.1032382
Received: 30 August 2022; Accepted: 29 November 2022;
Published: 04 January 2023.
Edited by:
Mingming Deng, Peking Union Medical College, ChinaReviewed by:
Lintao Bi, Jilin University, ChinaCopyright © 2023 Zhou, Gao, Jing and Wang. This is an open-access article distributed under the terms of the Creative Commons Attribution License (CC BY). The use, distribution or reproduction in other forums is permitted, provided the original author(s) and the copyright owner(s) are credited and that the original publication in this journal is cited, in accordance with accepted academic practice. No use, distribution or reproduction is permitted which does not comply with these terms.
*Correspondence: Xiaofang Wang, eGlhb2Zhbmd3YW5nMjAwNUAxNjMuY29t
†These authors have contributed equally to this work and share first authorship
Disclaimer: All claims expressed in this article are solely those of the authors and do not necessarily represent those of their affiliated organizations, or those of the publisher, the editors and the reviewers. Any product that may be evaluated in this article or claim that may be made by its manufacturer is not guaranteed or endorsed by the publisher.
Research integrity at Frontiers
Learn more about the work of our research integrity team to safeguard the quality of each article we publish.