- 1College of Medicine, Alfaisal University, Riyadh, Saudi Arabia
- 2Department of Molecular Oncology, King Faisal Specialist Hospital and Research Centre, Riyadh, Saudi Arabia
Background: Colorectal cancer (CRC) is the third most common cancer and third leading cause of cancer-associated deaths worldwide. Diagnosing CRC patients reliably at an early and curable stage is of utmost importance to reduce the risk of mortality.
Methods: We identified global differentially expressed genes with copy number alterations in patients with CRC. We then identified genes that are also expressed in blood, which resulted in a blood-based gene signature. We validated the gene signature’s diagnostic and prognostic potential using independent datasets of gene expression profiling from over 800 CRC patients with detailed clinical data. Functional enrichment, gene interaction networks and pathway analyses were also performed.
Results: The analysis revealed a 17-gene signature that is expressed in blood and demonstrated that it has diagnostic potential. The 17-gene SVM classifier displayed 99 percent accuracy in predicting the patients with CRC. Moreover, we developed a prognostic model and defined a risk-score using 17-gene and validated that high risk score is strongly associated with poor disease outcome. The 17-gene signature predicted disease outcome independent of other clinical factors in the multivariate analysis (HR = 2.7, 95% CI = 1.3–5.3, p = 0.005). In addition, our gene network and pathway analyses revealed alterations in oxidative stress, STAT3, ERK/MAPK, interleukin and cytokine signaling pathways as well as potentially important hub genes, including BCL2, MS4A1, SLC7A11, AURKA, IL6R, TP53, NUPR1, DICER1, DUSP5, SMAD3, and CCND1.
Conclusion: Our results revealed alterations in various genes and cancer-related pathways that may be essential for CRC transformation. Moreover, our study highlights diagnostic and prognostic value of our gene signature as well as its potential use as a blood biomarker as a non-invasive diagnostic method. Integrated analysis transcriptomic data coupled with copy number aberrations may provide a reliable method to identify key biological programs associated with CRC and lead to improved diagnosis and therapeutic options.
Introduction
Colorectal cancer (CRC) is the third most common cancer and the third highest cancer-related mortality worldwide (American Cancer Society, 2021; Sung et al., 2021). Despite all the advances in cancer therapies and raising awareness, colorectal cancer continues to be one of the deadliest cancers worldwide (Rawla et al., 2019; Xi and Xu, 2021). Diagnosing CRC patients during the early stages of the tumor development is essential, as that is when CRC is most curable. Therefore, it is of utmost importance to identify robust non-invasive diagnostic biomarkers for early detection of the cancer in order to achieve a better outcome. In addition, it is also essential to have biomarkers that would prognosticate patients with high-risk profiles to guide for personalized treatment.
Changes in gene expression and gene copy number are closely related to diseases such as cancer (Colak et al., 2010; Colak et al., 2013; Shao et al., 2019). Since tumorigenesis genes show associations with copy number variations (CNVs) and expression levels, it is possible to increase the diagnostic reliability as well as the predictive potential of prognosis by integrating CNV and gene expression data (Sheng et al., 2011; Miao et al., 2014; Shao et al., 2019; Kaya et al., 2022). Indeed, the previous studies, including our own, reported that the multi-omics approach may increase the robustness and reliability of biomarkers associated with complex diseases, including cancer (Miao et al., 2014; Aldosary et al., 2020; Das et al., 2020; Al-Harazi et al., 2021a; Baloni et al., 2021; Kaya et al., 2022; Ruan et al., 2022). Additionally, it has been reported that network-based approaches have high efficacy in identifying biomarkers for many complex diseases, including several different types of cancer (Wang et al., 2017; Chen et al., 2019; Liu et al., 2019; Uddin et al., 2019; Khan et al., 2020; Al-Harazi et al., 2021b). However, most biomarkers identified thus far require invasive procedures.
In this study, we identified a blood-based gene signature with diagnostic and prognostic potential for CRC by utilizing an integrated approach of transcriptomic analysis coupled with overlapping genes associated with the copy number alterations (CNA) in CRC. We then validated the gene signature’s classification performance as well as the prognostic potential using independent transcriptomics datasets from over 800 CRC patients with detailed clinical data. The identified gene signature may improve the diagnosis and prognosis of CRC and help to develop therapeutic strategies.
Materials and methods
Data collection and integrated analysis
Whole-genome gene expression dataset for patients with colorectal cancer (CRC) was gathered from GEO (GSE23878) (www.ncbi.nlm.nih.gov/geo). In addition, CNA regions associated with CRC in genomic data comprising thirty samples (15 tumor and 15 adjacent normal samples) from Saudi patients were identified as described previously in (Eldai et al., 2013). The gene expression dataset (GSE23878) contains samples from 35 colon tumors and 24 normal controls (Uddin et al., 2011). The samples were probed using Affymetrix Human Genome U133 Plus 2.0 Array. The differentially expressed genes (DEGs) were identified using independent two-sample t-test with adjusted p-value of <0.05 and absolute fold change (FC) ≥ 2.0 between CRC and normal samples. Multiple hypothesis testing was controlled by applying the Benjamini–Hochberg false discovery rate (FDR) correction (Benjamini and Hochberg, 1995). Genes expressed in blood are identified using data from GTEx portal (https://gtexportal.org/home/). We implemented Venn diagram approach in order to find the genes that have CNAs with concomitant gene expression changes and are also expressed in blood. Our methodology is shown in Figure 1.
Diagnostic validation of the gene signature
For validating the diagnostic and prognostic value of our gene signature, we used independently performed microarray and RNA sequencing datasets from The Cancer Genome Atlas (TCGA) database. The microarray data (TCGA data version 2016_01_28 for colorectal adenocarcinoma (COADREAD) included 244 samples (222 tumor and 22 normal samples) and the RNAseq data contained 675 samples (624 tumor and 51 normal). We used level 3 preprocessed and normalized gene expression data as described in detail by the TCGA workgroup (https://gdac.broadinstitute.org/). We performed unsupervised principal component analysis (PCA) and hierarchical clustering by Pearson correlation with average linkage clustering to validate the diagnostic performance of our gene signature. Moreover, transcription profiling datasets of blood samples from CRC patients (n = 100) and healthy controls (n = 100) were retrieved from ArrayExpress database (E-MTAB-1532) to test the gene signature expression levels in blood samples from patients with CRC as compared to those from normal controls.
Colorectal cancer classifier model and performance evaluation
We designed a 17-gene-CRC classifier using several machine learning algorithms, including Support Vector Machine (SVM), K-Nearest Neighbor (KNN), Linear Discriminant Analysis (LDA), and Nearest Centroid. First, we used the GSE23878 dataset for building the classification model, and then tested the classification performance on an indepedent dataset (TCGA dataset) to confirm if the 17-gene-classifier can distinguish patients from normal controls. We evaluated the performance of the classifier for its accuracy, specificity, sensitivity, and area under curve (AUC), as described previously (Al-Harazi et al., 2021a; Al-Harazi et al., 2021b). The analyses were performed using PARTEK Genomics Suite (Partek Inc., St. Lois, MO, United States).
Survival and multivariate analyses
We performed univariate and multivariate analyses using the Cox proportional hazard regression model to investigate the prognostic value of our gene signature along with other clinic-pathological variables. We defined a risk score for each patient in the TCGA dataset as a linear combination of expression level of 17 genes multiplied by the regression coefficient β) of each gene extracted from the Cox proportional hazards regression model, using the following formula: prognosis risk score = expression of gene1 × β1+ expression of gene2 × β2 + … expression of genen × βn. Patients are defined as high and low risk groups using the median score as the cutoff. We then used the Kaplan-Meier method to plot survival curves. Significance between survival curves was calculated by log-rank test. Univariate Cox regression analysis was performed to evaluate the prognostic value of the 17-gene signature and their relationships with overall survival of CRC patients. Moreover, multivariate Cox regression analysis was performed to examine the predictive ability of the 17-gene signature independent of other clinical factors, including gender, age, pathologic stage, and lymphatic invasion. A p-value < 0.05 was considered statistically significant.
Gene ontology enrichment, canonical pathway, and network analyses
Functional, pathway, gene ontology (GO) enrichment, and gene interaction network analyses of the identified gene signature were performed using QIAGEN’s Ingenuity Pathway Analysis (IPA) (QIAGEN Inc., https://www.qiagenbioinformatics.com/products/ingenuity-pathway-analysis), DAVID bioinformatics tools (Sherman et al., 2007), and PANTHER™ classification systems (Thomas et al., 2003). We performed gene interaction network and causal network analyses after mapping the identified gene signature to its corresponding gene object in the Ingenuity pathway knowledge base. A right-tailed Fisher’s exact test was used to calculate a p-value determining the probability that the biological function (or pathway) assigned to the data set is explained by chance alone (Colak et al., 2020).
Results
Identification of a gene signature associated with colorectal cancer
We first analyzed global mRNA expression profile of patients with CRC (n = 35) and normal controls (n = 24) using data from GSE23878 (Uddin et al., 2011). The analysis revealed 1,366 DEGs with adjusted p-value < 5% and absolute fold-change >2 in tumor compared to normal (Supplementary Table S1). Following that, we identified significantly dysregulated genes that have also copy number alterations (gains/losses) by mapping these dysregulated genes on the CNA regions in the genomic data from CRC patients and controls (Eldai et al., 2013). There were 144 genes in CNA regions of CRC patients. Of note, the patients in the transcriptomic and genomic data all belong to the same ethnicity (Arabs) (Eldai et al., 2013). Having ethnically matched cohorts in both types of omics measurements would limit the bias due to ethnicity and may reveal more biologically relevant results. Integrating with the genes in the CNA regions revealed that 30 of the significantly dysregulated genes have concomitant copy number alterations, 17 of which are also expressed in blood (Figure 2A; Table 1).
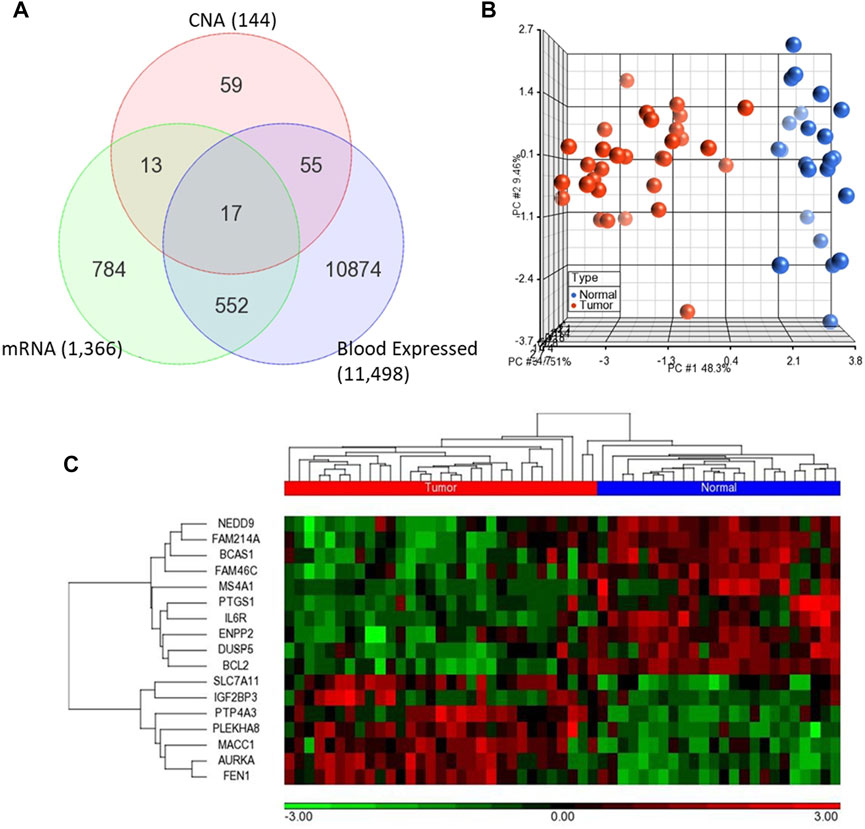
FIGURE 2. (A) Venn diagram representing the overlapping 17 genes among differentially expressed genes (mRNA) and CNA that are also expressed in blood (B–C) Unsupervised principal component analysis (PCA) and two-dimensional hierarchical clustering using 17-gene signature on the GSE23878. The red spheres refer to tumors and blue ones for normal controls. The hierarchical clustering resulted in two main clusters of tumors and controls. Samples are denoted in columns and genes are denoted in rows.
Validation of the 17-gene signature for diagnostic and prognostic potential
We validated diagnostic value of the 17-gene signature on GSE23878 (Figure 2) as well as on two independent datasets; TCGA microarray (n = 244 samples) and TCGA RNA-sequencing datasets (n = 675 samples) (Figures 3A–C, respectively). The unsupervised PCA and two-dimensional hierarchical clustering clearly distinguished patients as either CRC or normal controls in all datasets (Figures 2B, C, 3A–C). We also used early stage CRC data from TCGA (n = 47, Stage I tumor) to test the 17-gene signature’s diagnostic potential to discriminate the early stage CRC patients from normal controls. The analysis provided 100% accurate clustering of the two groups (Figures 3D, E). Moreover, we investigated our 17-gene signature’s expression level within blood samples obtained from CRC patients (n = 100) and healthy controls (n = 100) (E-MTAB-1532), which revealed that those with CRC have significantly higher expression levels than the controls (p-value <0.0001) (Supplementary Figure S1).
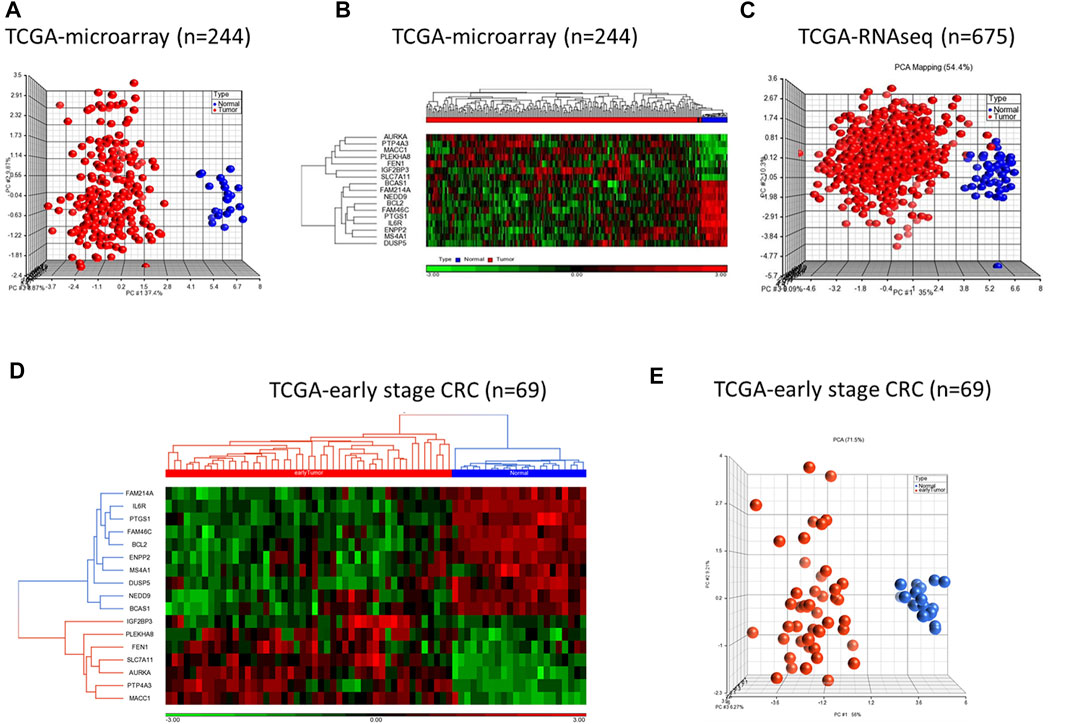
FIGURE 3. PCA and hierarchical clustering analyses using 17-gene signature on the TCGA microarray dataset (n = 244) (A–B) and TCGA RNA-sequencing dataset (n = 675) (C). The hierarchical clustering and PCA analyses using the 17-gene signature on early stage CRC data from TCGA (n = 69) (D–E). The analyses clearly distinguished patients as either CRC or normal controls on all datasets. Red and blue indicate tumor and normal samples, respectively.
To validate the prognostic significance of the 17-gene signature, we used the TCGA dataset with detailed clinical information and overall survival. We first calculated a prognostic risk score based on 17-gene signature, as described in the methods section and patients are classified as high or low risk using the median score as a cutoff. Our results demonstrated that a high 17-gene prognostic score is significantly associated with poor disease outcome (p-value = 0.006). Indeed, Kaplan–Meier survival analysis displayed that the high-risk group had significantly worse prognosis than the low-risk group (Figure 4A). Furthermore, the multivariate Cox regression analysis revealed that 17-gene signature prognosticated the CRC outcome independent of other clinical variables, including age, gender, pathologic stage and lymphatic invasion (HR = 2.61, 95% CI = 1.3–5.23; p = 0.0069) (Table 2).
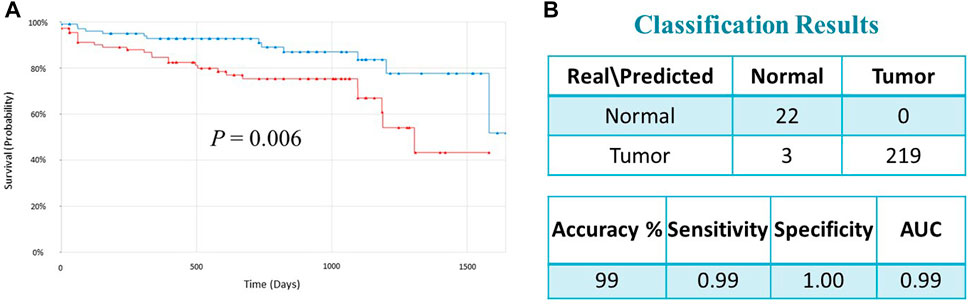
FIGURE 4. (A) Kaplan–Meier survival analysis of the TCGA dataset indicated that the high-risk group had significantly worse prognosis than the low-risk group (p = 0.006). Red and green curves indicate high and low-risk groups, respectively. (B) Classification performance of the 17-gene classifier modeled using SVM with linear kernel algorithm. The classification performance is evaluated on the TCGA dataset (n = 244).
Classification model and performance assessment
We designed a 17-gene CRC classifier using different classification algorithms, including Support Vector Machine (SVM), K-Nearest Neighbor (KNN), Linear Discriminant Analysis (LDA), and Nearest Centroid and estimated the classification performance. The GSE23878 dataset is used for building the classification model and the classification performance is tested on an indepedent dataset (TCGA dataset). We assessed the classifier’s performance in terms of accuracy, specificity, sensitivity, and area under the curve (AUC), as described previously (Al-Harazi et al., 2021a; Al-Harazi et al., 2021b). The SVM with linear kernel has outperformed other algorithms and the 17-gene classifier achieved a high accuracy of 99 percent, and sensitivity, specificity and AUC of 99%, 100% and 99%, respectively (Figure 4B), confirming the 17-gene signature’s ability to discriminate patients from normal controls.
Functional, pathway and gene interaction network analyses
Gene ontology enrichment and functional analyses revealed that the 17-gene signature is significantly associated with diseases and functions related to cancer, cellular movement, cellular growth and proliferation, cell death and survival (Figure 5A and Supplementary Table S2). Moreover, pathway analysis using several bioinformatics tools revealed alterations in STAT3, ERK/MAPK, oxidative stress, interleukin and cytokine signaling al pathways (Figures 5B,C). Gene interaction network analysis indicated hub genes that may have potentially important role in CRC transformation and progression, including BCL2, MS4A1, AURKA, IL6R, TP53, NUPR1, DUSP5, and CCND1 (Figure 5D). Furthermore, IPA causal network analyses revealed predicted activation of DICER1 and SMAD3 in CRC (Supplementary Figure S2).
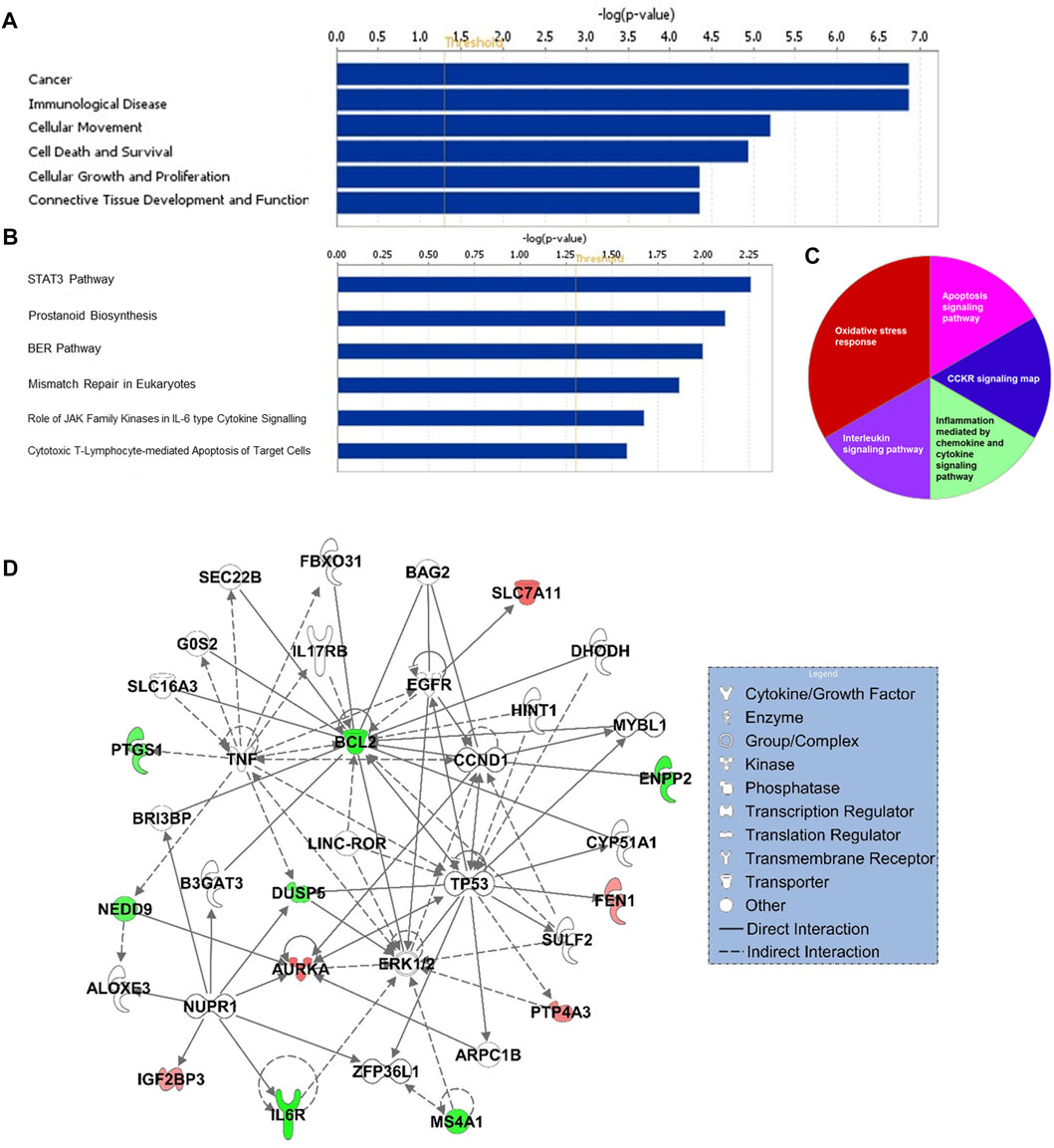
FIGURE 5. Functional (A) and canonical pathway (B) and PANTHER pathway (C) analyses of 17-gene signature. X-axis (in A and B) represents–log (p-value); the significance of the functional/pathway term. The threshold line indicates p-value of 0.05. (D) Gene interaction network analyses of 17-gene signature. Red/green indicates higher/lower expression in CRC in comparison to controls.
Discussion
In this study, we aimed to identify a robust gene signature that would detect the disease accurately and have prognostic significance that would differentiate the high-risk patients from the low-risk ones. Since appropriate management of choice and curative surgical resection success rate depend largely on staging of the cancer, convenient and non-invasive early detection biomarkers are still needed to ensure early diagnosis and good prognosis (Al Bandar and Kim, 2017; Feo et al., 2017).
We performed an integrated analysis of significantly dysregulated genes within the transcriptome of CRC patients with the genes that have copy number changes (gains/losses) in a patient cohort from an ethnically matched population, and identified a blood-based 17-gene signature. We then validated its diagnostic and prognostic potential on an independent large cohort of CRC patients. Previous studies have demonstrated that multi-omics analysis (using whole-genome gene expression profiling, copy number variations (CNVs), proteomics, metabolomics, and others) may lead to reliable biomarkers that are robust in disease classification and may also help identify cancer driver genes that are involved in tumor initiation and progression (Colak et al., 2010; Colak et al., 2013; Ohshima et al., 2017; Liu et al., 2021; Kaya et al., 2022; Ruan et al., 2022). Moreover, integrating omics data with the gene interaction networks has been shown to be a robust methodology that may lead to more reliable and accurate predictive biomarkers for human diseases (Al-Harazi et al., 2016; Ma et al., 2019; Khan et al., 2020; Seifert et al., 2020; Sinkala et al., 2020).
Our gene network analysis revealed several key hub genes that may have potentially important roles in CRC transformation and progression, including BCL2 (Lindner et al., 2017; Perini et al., 2018; Diaz-Flores et al., 2019), MS4A1 (Mudd et al., 2021; Li and Fang, 2022), AURKA (Wang et al., 2020; Mou et al., 2021; Kahl et al., 2022), IL6R (Mendez-Clemente et al., 2022), TP53 (Oner et al., 2018), NUPR1 (Martin et al., 2021; Xiao et al., 2022), DICER1 (Iliou et al., 2014; Luan et al., 2021), DUSP5, SMAD3 (De Mattia et al., 2021; Tang et al., 2022), and CCND1 (Shan et al., 2017; Chen et al., 2020). Some of the identified genes were reported to be associated with cancers, including colorectal cancer. For example, BCL2 family are central regulators of apoptosis, and up-regulation of BCL2 has been shown to lead to tumor development and progression as well as resistance to cancer therapy (Lindner et al., 2017; Perini et al., 2018; Diaz-Flores et al., 2019). MS4A1 encodes a B-lymphocyte surface molecule CD20 that has been reported to be associated with lipid metabolism and immune cell activation, and its expression is an independent predictor of cancer prognosis (Mudd et al., 2021; Li and Fang, 2022). Aurora kinases are involved in cell cycle regulation, G2/M transition, mitosis, and DNA replication functions. Recent reports have shown that aurora kinase A (AURKA), IL6R, NUPR1, and DICER1 play important role in the development, progression, and metastasis of a variety of cancers including colon cancer (Iliou et al., 2014; Wang et al., 2020; Luan et al., 2021; Martin et al., 2021; Mou et al., 2021; Kahl et al., 2022).
The causal network analysis indicated predicted activation of DICER1 and SMAD3 in CRC. Recent studies have shown that DICER1 is involved in the cancer initiation and development (Ma et al., 2020; Luan et al., 2021). Although the underlying mechanism is still unclear, transfer RNA-derived fragment biogenesis by DICER1 is directly associated with cancer development. A high expression of the enzyme is related to poor survival, independent of the patient’s other predisposing factors (Luan et al., 2021). SMAD3 has also been shown to be associated with tumor initiation and progression in earlier studies in several cancers (Colak et al., 2010; De Mattia et al., 2021; Tang et al., 2022). It has been also reported to have tumor promotor roles and directly involved in epithelium to mesenchyme transition (EMT), hence enhancing invasion, migration and metastasis (Millet and Zhang, 2007).
The pathway analyses indicated significant alterations in several cancer-related signaling pathways, such as oxidative stress, STAT3, ERK/MAPK, interleukin and cytokine signaling pathways. The ERK enzyme belongs to the MAPK family, which is involved in a various signaling cascades that regulate fundamental cellular processes such as cell growth, proliferation, differentiation, as well as stress responses. Our study as well as prior research findings have shown that there is a strong correlation with MAPK inhibition, especially ERK inhibition, and the development and advancement of most cancer types. ERK pathway dysfunction plays a major role in tumor invasion and metastasis, with varying level of different components of the cascade depending on the type of cancer. This makes it an abundant oncogenic factor that can also be used to identify CRC and differentiate it from other tumors (Guo et al., 2020).
In conclusion, the 17-gene signature that is identified in this study revealed genes and pathways that may be critical for CRC transformation and progression, and has the potential to detect the disease non-invasively as well as predict its outcome.
Data availability statement
The datasets used in this study can be found in online repositories, including The Cancer Genome Atlas (TCGA), ArrayExpress, and the NCBI Gene Expression Omnibus (GEO). The names of the repositories and accession numbers can be found in this article/materials and methods.
Ethics statement
The study is approved by King Faisal Specialist Hospital and Research Centre (KFSH&RC) (RAC#2110006). As the study uses publicly available datasets, it is exempt from any ethical approval procedures. Written informed consent for participation was not required for this study in accordance with the national legislation and the institutional requirements.
Author contributions
DC conception, design, and supervision. DC, OA, and IHK collected, analysed, interpreted the data, and drafted the manuscript. All authors read and approved the manuscript.
Funding
This study is funded by the Research Grant (RAC#2110006 to DC). The funder had no role in the study design and collection, analysis, and interpretation of the results.
Acknowledgments
We would like to thank King Faisal Specialist Hospital and Research Centre (KFSH&RC), RC Administration and Office of Research Affairs for their support of this research (RAC#2110006 to DC) and RCA-Logistics and Facilities Management Office, especially Mr. Marei Alenazi and his team, for their kind support in facilitating and expediting our requests. We also would like to thank Mr. Ibrahim Bin Olayan for IT support and Ms. Rawan AlShemali for administrative support. This work was under an institutionally approved King Faisal Specialist Hospital and Research Centre project (RAC# 2110006). The content of this paper in part is presented at the European Human Genetics Virtual Conference, 15–18 June 2019.
Conflict of interest
The authors declare that the research was conducted in the absence of any commercial or financial relationships that could be construed as a potential conflict of interest.
Publisher’s note
All claims expressed in this article are solely those of the authors and do not necessarily represent those of their affiliated organizations, or those of the publisher, the editors and the reviewers. Any product that may be evaluated in this article, or claim that may be made by its manufacturer, is not guaranteed or endorsed by the publisher.
Supplementary material
The Supplementary Material for this article can be found online at: https://www.frontiersin.org/articles/10.3389/fgene.2022.1031086/full#supplementary-material
References
Al Bandar, M. H., and Kim, N. K. (2017). Current status and future perspectives on treatment of liver metastasis in colorectal cancer (Review). Oncol. Rep. 37, 2553–2564. doi:10.3892/or.2017.5531
Al-Harazi, O., Al Insaif, S., Al-Ajlan, M. A., Kaya, N., Dzimiri, N., and Colak, D. (2016). Integrated genomic and network-based analyses of complex diseases and human disease network. J. Genet. Genomics 43, 349–367. doi:10.1016/j.jgg.2015.11.002
Al-Harazi, O., Kaya, I. H., Al-Eid, M., Alfantoukh, L., Al Zahrani, A. S., Al Sebayel, M., et al. (2021a). Identification of gene signature as diagnostic and prognostic blood biomarker for early hepatocellular carcinoma using integrated cross-species transcriptomic and network analyses. Front. Genet. 12, 710049. doi:10.3389/fgene.2021.710049
Al-Harazi, O., Kaya, I. H., El Allali, A., and Colak, D. (2021b). A network-based methodology to identify subnetwork markers for diagnosis and prognosis of colorectal cancer. Front. Genet. 12, 721949. doi:10.3389/fgene.2021.721949
Aldosary, M., Al-Bakheet, A., Al-Dhalaan, H., Almass, R., Alsagob, M., Al-Younes, B., et al. (2020). Rett syndrome, a neurodevelopmental disorder, whole-transcriptome, and mitochondrial genome multiomics analyses identify novel variations and disease pathways. OMICS 24, 160–171. doi:10.1089/omi.2019.0192
Alves Martins, B. A., De Bulhoes, G. F., Cavalcanti, I. N., Martins, M. M., De Oliveira, P. G., and Martins, A. M. A. (2019). Biomarkers in colorectal cancer: The role of translational proteomics research. Front. Oncol. 9, 1284. doi:10.3389/fonc.2019.01284
Baloni, P., Arnold, M., Moreno, H., Nho, K., Kastenmüller, G., Suhre, K., et al. (2021). Transcriptomics, metabolomics, lipidomics, metabolic flux and mGWAS analyses of sphingolipid pathway highlights novel drugs for Alzheimer’s disease. Alzheimer's. Dementia 17, e056152. doi:10.1002/alz.056152
Benjamini, Y., and Hochberg, Y. (1995). Controlling the false discovery rate: A practical and powerful approach to multiple testing. J. R. Stat. Soc. Ser. B 57, 289–300. doi:10.1111/j.2517-6161.1995.tb02031.x
Chen, L., Lu, D., Sun, K., Xu, Y., Hu, P., Li, X., et al. (2019). Identification of biomarkers associated with diagnosis and prognosis of colorectal cancer patients based on integrated bioinformatics analysis. Gene 692, 119–125. doi:10.1016/j.gene.2019.01.001
Chen, Y., Huang, Y., Gao, X., Li, Y., Lin, J., Chen, L., et al. (2020). CCND1 amplification contributes to immunosuppression and is associated with a poor prognosis to immune checkpoint inhibitors in solid tumors. Front. Immunol. 11, 1620. doi:10.3389/fimmu.2020.01620
Colak, D., Al-Harazi, O., Mustafa, O. M., Meng, F., Assiri, A. M., Dhar, D. K., et al. (2020). RNA-seq transcriptome profiling in three liver regeneration models in rats: Comparative analysis of partial hepatectomy, ALLPS, and PVL. Sci. Rep. 10, 5213. doi:10.1038/s41598-020-61826-1
Colak, D., Chishti, M. A., Al-Bakheet, A. B., Al-Qahtani, A., Shoukri, M. M., Goyns, M. H., et al. (2010). Integrative and comparative genomics analysis of early hepatocellular carcinoma differentiated from liver regeneration in young and old. Mol. Cancer 9, 146. doi:10.1186/1476-4598-9-146
Colak, D., Nofal, A., Albakheet, A., Nirmal, M., Jeprel, H., Eldali, A., et al. (2013). Age-specific gene expression signatures for breast tumors and cross-species conserved potential cancer progression markers in young women. PLoS One 8, e63204. doi:10.1371/journal.pone.0063204
Das, T., Andrieux, G., Ahmed, M., and Chakraborty, S. (2020). Integration of online omics-data resources for cancer research. Front. Genet. 11, 578345. doi:10.3389/fgene.2020.578345
De Mattia, E., Canzonieri, V., Polesel, J., Mezzalira, S., Dalle Fratte, C., Dreussi, E., et al. (2021). SMAD3 host and tumor profiling to identify locally advanced rectal cancer patients at high risk of poor response to neoadjuvant chemoradiotherapy. Front. Pharmacol. 12, 778781. doi:10.3389/fphar.2021.778781
Diaz-Flores, E., Comeaux, E. Q., Kim, K. L., Melnik, E., Beckman, K., Davis, K. L., et al. (2019). Bcl-2 is a therapeutic target for hypodiploid B-lineage acute lymphoblastic leukemia. Cancer Res. 79, 2339–2351. doi:10.1158/0008-5472.CAN-18-0236
Eldai, H., Periyasamy, S., Al Qarni, S., Al Rodayyan, M., Muhammed Mustafa, S., Deeb, A., et al. (2013). Novel genes associated with colorectal cancer are revealed by high resolution cytogenetic analysis in a patient specific manner. PLoS One 8, e76251. doi:10.1371/journal.pone.0076251
Feo, L., Polcino, M., and Nash, G. M. (2017). Resection of the primary tumor in stage IV colorectal cancer: When is it necessary? Surg. Clin. North Am. 97, 657–669. doi:10.1016/j.suc.2017.01.012
Guo, Y. J., Pan, W. W., Liu, S. B., Shen, Z. F., Xu, Y., and Hu, L. L. (2020). ERK/MAPK signalling pathway and tumorigenesis. Exp. Ther. Med. 19, 1997–2007. doi:10.3892/etm.2020.8454
Iliou, M. S., Da Silva-Diz, V., Carmona, F. J., Ramalho-Carvalho, J., Heyn, H., Villanueva, A., et al. (2014). Impaired DICER1 function promotes stemness and metastasis in colon cancer. Oncogene 33, 4003–4015. doi:10.1038/onc.2013.398
Kahl, I., Mense, J., Finke, C., Boller, A. L., Lorber, C., Gyorffy, B., et al. (2022). The cell cycle-related genes RHAMM, AURKA, TPX2, PLK1, and PLK4 are associated with the poor prognosis of breast cancer patients. J. Cell. Biochem. 123, 581–600. doi:10.1002/jcb.30205
Kaya, I. H., Al-Harazi, O., Kaya, M. T., and Colak, D. (2022). Integrated analysis of transcriptomic and genomic data reveals blood biomarkers with diagnostic and prognostic potential in non-small cell lung cancer. Front. Mol. Biosci. 9, 774738. doi:10.3389/fmolb.2022.774738
Khan, A., Rehman, Z., Hashmi, H. F., Khan, A. A., Junaid, M., Sayaf, A. M., et al. (2020). An integrated systems Biology and network-based approaches to identify novel biomarkers in breast cancer cell lines using gene expression data. Interdiscip. Sci. 12, 155–168. doi:10.1007/s12539-020-00360-0
Li, S., and Fang, Y. (2022). MS4A1 as a potential independent prognostic factor of breast cancer related to lipid metabolism and immune microenvironment based on TCGA database analysis. Med. Sci. Monit. 28, e934597. doi:10.12659/MSM.934597
Lindner, A. U., Salvucci, M., Morgan, C., Monsefi, N., Resler, A. J., Cremona, M., et al. (2017). BCL-2 system analysis identifies high-risk colorectal cancer patients. Gut 66, 2141–2148. doi:10.1136/gutjnl-2016-312287
Liu, N., Wu, Y., Cheng, W., Wu, Y., Wang, L., and Zhuang, L. (2021). Identification of novel prognostic biomarkers by integrating multi-omics data in gastric cancer. BMC Cancer 21, 460. doi:10.1186/s12885-021-08210-y
Liu, S., Zheng, B., Sheng, Y., Kong, Q., Jiang, Y., Yang, Y., et al. (2019). Identification of cancer dysfunctional subpathways by integrating DNA methylation, copy number variation, and gene-expression data. Front. Genet. 10, 441. doi:10.3389/fgene.2019.00441
Luan, N., Mu, Y., Mu, J., Chen, Y., Ye, X., Zhou, Q., et al. (2021). Dicer1 promotes colon cancer cell invasion and migration through modulation of tRF-20-MEJB5Y13 expression under hypoxia. Front. Genet. 12, 638244. doi:10.3389/fgene.2021.638244
Ma, C., Ma, N., Qin, L., Miao, C., Luo, M., and Liu, S. (2020). DICER1-AS1 promotes the malignant behaviors of colorectal cancer cells by regulating miR-296-5p/STAT3 Axis. Cancer Manag. Res. 12, 10035–10046. doi:10.2147/CMAR.S252786
Ma, J., Karnovsky, A., Afshinnia, F., Wigginton, J., Rader, D. J., Natarajan, L., et al. (2019). Differential network enrichment analysis reveals novel lipid pathways in chronic kidney disease. Bioinformatics 35, 3441–3452. doi:10.1093/bioinformatics/btz114
Martin, T. A., Li, A. X., Sanders, A. J., Ye, L., Frewer, K., Hargest, R., et al. (2021). NUPR1 and its potential role in cancer and pathological conditions (Review). Int. J. Oncol. 58, 21. doi:10.3892/ijo.2021.5201
Mendez-Clemente, A., Bravo-Cuellar, A., Gonzalez-Ochoa, S., Santiago-Mercado, M., Palafox-Mariscal, L., Jave-Suarez, L., et al. (2022). Dual STAT3 and IL6R inhibition with stattic and tocilizumab decreases migration, invasion and proliferation of prostate cancer cells by targeting the IL6/IL6R/STAT3 axis. Oncol. Rep. 48, 138. doi:10.3892/or.2022.8349
Miao, R., Luo, H., Zhou, H., Li, G., Bu, D., Yang, X., et al. (2014). Identification of prognostic biomarkers in Hepatitis B virus-related hepatocellular carcinoma and stratification by integrative multi-omics analysis. J. Hepatol. 61, 840–849. doi:10.1016/j.jhep.2014.05.025
Millet, C., and Zhang, Y. E. (2007). Roles of Smad3 in TGF-beta signaling during carcinogenesis. Crit. Rev. Eukaryot. Gene Expr. 17, 281–293. doi:10.1615/critreveukargeneexpr.v17.i4.30
Mou, P. K., Yang, E. J., Shi, C., Ren, G., Tao, S., and Shim, J. S. (2021). Aurora kinase A, a synthetic lethal target for precision cancer medicine. Exp. Mol. Med. 53, 835–847. doi:10.1038/s12276-021-00635-6
Mudd, T. W., Lu, C., Klement, J. D., and Liu, K. (2021). MS4A1 expression and function in T cells in the colorectal cancer tumor microenvironment. Cell. Immunol. 360, 104260. doi:10.1016/j.cellimm.2020.104260
Ohshima, K., Hatakeyama, K., Nagashima, T., Watanabe, Y., Kanto, K., Doi, Y., et al. (2017). Integrated analysis of gene expression and copy number identified potential cancer driver genes with amplification-dependent overexpression in 1, 454 solid tumors. Sci. Rep. 7, 641. doi:10.1038/s41598-017-00219-3
Oner, M. G., Rokavec, M., Kaller, M., Bouznad, N., Horst, D., Kirchner, T., et al. (2018). Combined inactivation of TP53 and MIR34A promotes colorectal cancer development and progression in mice via increasing levels of IL6R and PAI1. Gastroenterology 155, 1868–1882. doi:10.1053/j.gastro.2018.08.011
Perini, G. F., Ribeiro, G. N., Pinto Neto, J. V., Campos, L. T., and Hamerschlak, N. (2018). BCL-2 as therapeutic target for hematological malignancies. J. Hematol. Oncol. 11, 65. doi:10.1186/s13045-018-0608-2
Rawla, P., Sunkara, T., and Barsouk, A. (2019). Epidemiology of colorectal cancer: Incidence, mortality, survival, and risk factors. Prz. Gastroenterol. 14, 89–103. doi:10.5114/pg.2018.81072
Ruan, X., Ye, Y., Cheng, W., Xu, L., Huang, M., Chen, Y., et al. (2022). Multi-omics integrative analysis of lung adenocarcinoma: An in silico profiling for precise medicine. Front. Med. 9, 894338. doi:10.3389/fmed.2022.894338
Seifert, S., Gundlach, S., Junge, O., and Szymczak, S. (2020). Integrating biological knowledge and gene expression data using pathway-guided random forests: A benchmarking study. Bioinformatics 36, 4301–4308. doi:10.1093/bioinformatics/btaa483
Shan, Y. S., Hsu, H. P., Lai, M. D., Hung, Y. H., Wang, C. Y., Yen, M. C., et al. (2017). Cyclin D1 overexpression correlates with poor tumor differentiation and prognosis in gastric cancer. Oncol. Lett. 14, 4517–4526. doi:10.3892/ol.2017.6736
Shao, X., Lv, N., Liao, J., Long, J., Xue, R., Ai, N., et al. (2019). Copy number variation is highly correlated with differential gene expression: A pan-cancer study. BMC Med. Genet. 20, 175. doi:10.1186/s12881-019-0909-5
Sheng, J., Deng, H. W., Calhoun, V. D., and Wang, Y. P. (2011). Integrated analysis of gene expression and copy number data on gene shaving using independent component analysis. IEEE/ACM Trans. Comput. Biol. Bioinform. 8, 1568–1579. doi:10.1109/TCBB.2011.71
Sherman, B. T., Huang, D. W., Tan, Q., Guo, Y., Bour, S., Liu, D., et al. (2007). DAVID knowledgebase: A gene-centered database integrating heterogeneous gene annotation resources to facilitate high-throughput gene functional analysis. BMC Bioinforma. 8, 426. doi:10.1186/1471-2105-8-426
Siegel, R. L., Miller, K. D., Goding Sauer, A., Fedewa, S. A., Butterly, L. F., Anderson, J. C., et al. (2020). Colorectal cancer statistics, 2020. Ca. Cancer J. Clin. 70, 145–164. doi:10.3322/caac.21601
Sinkala, M., Mulder, N., and Martin, D. (2020). Machine learning and network analyses reveal disease subtypes of pancreatic cancer and their molecular characteristics. Sci. Rep. 10, 1212. doi:10.1038/s41598-020-58290-2
Sung, H., Ferlay, J., Siegel, R. L., Laversanne, M., Soerjomataram, I., Jemal, A., et al. (2021). Global cancer statistics 2020: GLOBOCAN estimates of incidence and mortality worldwide for 36 cancers in 185 countries. Ca. Cancer J. Clin. 71, 209–249. doi:10.3322/caac.21660
Tang, P. C., Chung, J. Y., Xue, V. W., Xiao, J., Meng, X. M., Huang, X. R., et al. (2022). Smad3 promotes cancer-associated fibroblasts generation via macrophage-myofibroblast transition. Adv. Sci. 9, e2101235. doi:10.1002/advs.202101235
Thomas, P. D., Kejariwal, A., Campbell, M. J., Mi, H., Diemer, K., Guo, N., et al. (2003). Panther: A browsable database of gene products organized by biological function, using curated protein family and subfamily classification. Nucleic Acids Res. 31, 334–341. doi:10.1093/nar/gkg115
Uddin, M. N., Li, M., and Wang, X. (2019). Identification of transcriptional markers and microRNA-mRNA regulatory networks in colon cancer by integrative analysis of mRNA and microRNA expression profiles in colon tumor stroma. Cells 8, 1054. doi:10.3390/cells8091054
Uddin, S., Ahmed, M., Hussain, A., Abubaker, J., Al-Sanea, N., Abduljabbar, A., et al. (2011). Genome-wide expression analysis of Middle Eastern colorectal cancer reveals FOXM1 as a novel target for cancer therapy. Am. J. Pathol. 178, 537–547. doi:10.1016/j.ajpath.2010.10.020
Wang, F., Wang, R., Li, Q., Qu, X., Hao, Y., Yang, J., et al. (2017). A transcriptome profile in hepatocellular carcinomas based on integrated analysis of microarray studies. Diagn. Pathol. 12, 4. doi:10.1186/s13000-016-0596-x
Wang, S., Qi, J., Zhu, M., Wang, M., and Nie, J. (2020). AURKA rs2273535 T>A polymorphism associated with cancer risk: A systematic review with meta-analysis. Front. Oncol. 10, 1040. doi:10.3389/fonc.2020.01040
Xi, Y., and Xu, P. (2021). Global colorectal cancer burden in 2020 and projections to 2040. Transl. Oncol. 14, 101174. doi:10.1016/j.tranon.2021.101174
Keywords: colorectal cancer, transcriptomic, genomic, biomarker, blood, gene signature, diagnosis, prognosis
Citation: Kaya IH, Al-Harazi O and Colak D (2023) Transcriptomic data analysis coupled with copy number aberrations reveals a blood-based 17-gene signature for diagnosis and prognosis of patients with colorectal cancer. Front. Genet. 13:1031086. doi: 10.3389/fgene.2022.1031086
Received: 29 August 2022; Accepted: 01 December 2022;
Published: 06 January 2023.
Edited by:
Marta Rusmini, Giannina Gaslini Institute (IRCCS), ItalyCopyright © 2023 Kaya, Al-Harazi and Colak. This is an open-access article distributed under the terms of the Creative Commons Attribution License (CC BY). The use, distribution or reproduction in other forums is permitted, provided the original author(s) and the copyright owner(s) are credited and that the original publication in this journal is cited, in accordance with accepted academic practice. No use, distribution or reproduction is permitted which does not comply with these terms.
*Correspondence: Dilek Colak, ZGtjb2xha0BnbWFpbC5jb20=