- 1Department of Animal Science, University of Minnesota, Saint Paul, MN, United States
- 2Council on Dairy Cattle Breeding, Bowie, MD, United States
- 3Department of Animal Science, Michigan State University, East Lansing, MI, United States
- 4Department of Animal and Dairy Sciences, University of Wisconsin, Madison, WI, United States
- 5Department of Animal Science, Iowa State University, Ames, IA, United States
- 6Department of Animal Sciences, University of Florida, Gainesville, FL, United States
- 7Animal Genomics and Improvement Laboratory, ARS, USDA, Beltsville, MD, United States
The impact of genomic epistasis effects on the accuracy of predicting the phenotypic values of residual feed intake (RFI) in U.S. Holstein cows was evaluated using 6215 Holstein cows and 78,964 SNPs. Two SNP models and seven epistasis models were initially evaluated. Heritability estimates and the accuracy of predicting the RFI phenotypic values from 10-fold cross-validation studies identified the model with SNP additive effects and additive × additive (A×A) epistasis effects (A + A×A model) to be the best prediction model. Under the A + A×A model, additive heritability was 0.141, and A×A heritability was 0.263 that consisted of 0.260 inter-chromosome A×A heritability and 0.003 intra-chromosome A×A heritability, showing that inter-chromosome A×A effects were responsible for the accuracy increases due to A×A. Under the SNP additive model (A-only model), the additive heritability was 0.171. In the 10 validation populations, the average accuracy for predicting the RFI phenotypic values was 0.246 (with range 0.197–0.333) under A + A×A model and was 0.231 (with range of 0.188–0.319) under the A-only model. The average increase in the accuracy of predicting the RFI phenotypic values by the A + A×A model over the A-only model was 6.49% (with range of 3.02–14.29%). Results in this study showed A×A epistasis effects had a positive impact on the accuracy of predicting the RFI phenotypic values when combined with additive effects in the prediction model.
Introduction
Residual feed intake (RFI) is a measure of an animal’s feed efficiency (Koch et al., 1963; Kennedy et al., 1993; Pryce et al., 2014; Tempelman et al., 2015). In U.S. Holstein cattle, genome-wide association study (GWAS) and genomic prediction of RFI using single nucleotide polymorphism (SNP) markers have been reported (Yao et al., 2013; VanRaden et al., 2018; Li et al., 2019; Li et al., 2020). A national genomic evaluation has been implemented for feed-saved that is calculated based on RFI and body weight composite (Gaddis et al., 2021) using a SNP additive model. Additive effects are inheritable and genomic estimated breeding values (GEBV) based on additive effects are used for selecting breeding individuals. Additive effects also affect a cow’s lifetime performance. A selection index of GEBV’s of dairy traits named net merit is used as a measure of lifetime profit (VanRaden et al., 2014). Nonadditive effects including dominance and epistasis effects affect a cow’s lifetime performance if they exist but the inheritance of nonadditive effects is more complicated than that of additive effects and is discussed towards the end of this article. Given that approximately 1 million Holsteins in the U.S. were genotyped in 2020 and 2021 (CDCB, 2022) and the RFI phenotype is expensive to measure, an increase in the accuracy of predicting future RFI phenotypic performance may result in a reduction in the dairy expenses. In this study, we investigate the impact of epistasis effects on the genomic heritability of RFI and the accuracy of predicting the RFI phenotypic values of U.S. Holstein cows using prediction models with SNP additive, dominance and epistasis effects up to the third-order.
Materials and methods
Holstein population, SNP and phenotypic data
The Holstein population in this study had 6215 cows with RFI phenotypic observations and 78,964 imputed SNPs. The RFI phenotypic values were the phenotypic residuals after removing fixed non-genetic effects available from the December 2021 U.S. Holstein genomic evaluation data. The 6215 phenotypic values had a bell-shaped distribution with long tails. To evaluate the potential impact of the extreme phenotypic values, we evaluated the prediction accuracy after removing 17 phenotypic observations that were four standard deviations from the mean existed. The removal of 17 such extreme phenotypic values reduced the number of observations to 6198 with a similar bell-shaped distribution without the long tails of the original data (Supplementary Figure S1 and Table 1). However, the original dataset of 6215 cows had slightly higher accuracy (0.231) of predicting the phenotypic values than the dataset after removing the 17 extreme phenotypic values (0.227). Therefore, the original dataset with 6215 cows was used in this study.
Mixed model for GBLUP and GREML
Genomic best linear unbiased prediction (GBLUP) of genetic values was used for calculating the accuracy of predicting the RFI phenotypic values, and genomic restricted maximum likelihood estimation (GREML) was used for estimating the heritability of each type of genetic effects using mixed models. The effect types evaluated by GBLUP and GREML included SNP additive and dominance effects, pairwise and third-order epistasis effects, and intra- and inter-chromosome additive × additive (A × A) effects. Details of those effect types and the mixed models are described in Da et al., 2022. Since the prediction model with additive and A × A effects was identified as the best prediction model in this study, only these effects are included in the description of the mixed model for GBLUP and GREML here for simplicity of notations. For the phenotypic data in this study that already removed fixed non-genetic effects from the phenotypic values, the mixed model with additive and A × A epistasis genetic values as well as the variance-covariance matrices of the model can be described as:
where y = column vector of phenotypic observations, Z = incidence matrix allocating phenotypic observations to each individual = identity matrix for one observation per individual, µ = mean of the phenotypic values, X = column vector of 1’s as the model matrix for µ, a = column vector of genomic additive values, d = column vector of genomic dominance values,
Estimation of genomic heritability
The estimation of variance components used the method of genomic restricted maximum likelihood estimation (GREML) using a combination of EM-REML and AI-REML algorithms implemented by the EPIHAP computing package (Liang et al., 2021, Liang et al., 2022). GREML estimates of variance components were used for calculating heritability estimates from the entire sample of 6215 cows and from the 10 validation populations for calculating the observed standard deviation of each genomic heritability.
Evaluation of prediction accuracy using cross-validation
A 10-fold cross validation study was used to evaluate the accuracy of predicting the phenotypic values of each trait for each model. Individuals with phenotypic observations were randomly divided into 10 validation populations. The first nine validation populations had equal sample size of 621 cows each and the 10th population had 626 cows. In each validation population, phenotypic values were omitted when calculating GBLUP for training and validation individuals. Four measures of prediction accuracy were compared for the final prediction models, including three measures described previously (Legarra et al., 2008), denoted as
where
The
Results and Discussion
Genomic heritability estimates
Heritability estimates from the full model with additive, dominance and epistasis effects up to the third-order showed that only additive effects (A), A×A and A×A×A had nonzero heritability estimates of 0.141, 0.260 and 0.005 respectively; and SNP dominance effects (D) and dominance related epistasis effects (A×D, D×D, A×A×D, A×D×D and D×D×D) all had zero heritability (Supplementary Table S2). These results showed that only A, A×A and A×A×A could contribute to the accuracy of predicting the RFI phenotypic values. However, the A + A×A + A×A×A and A + A×A models had the same prediction accuracy that was higher than the accuracy of the A-only or A×A-only model (Supplementary Table S3), indicating that A×A×A effects had no contribution to the prediction accuracy. Therefore, the A + A×A model was identified as the best epistasis model with the smallest number of effect types among the models with the highest prediction accuracy. This model was compared with the A-only model for heritability estimates and prediction accuracy to determine whether A×A benefitted the prediction of RFI phenotypic values.
For the A-only and A + A×A models, two sets of heritability estimates were calculated, one set using the full population with 6215 cows and one set using the 10 training populations each with 5594 cows for the first nine training populations and 5589 cows for the 10th training population, and the results were similar (Table 1). Partitioning the A×A effects in the A + A×A model into intra-chromosome A×A effects (
Accuracy of predicting RFI phenotypic values in validation populations
Since the A + A×A model was identified as the best epistasis model, the evaluation of the impact of epistasis effects on the accuracy of predicting RFI phenotypic values was based on the comparison between the A-only and A + A×A models in validation populations. Results of the 10-fold validation study showed that the accuracy of predicting the RFI phenotypic values in the validation populations was 0.231 for the A-only model and was 0.246 for the best epistasis model (A + A×A), a 6.49% accuracy increase due to A×A effects over the accuracy of the A-only model (Table 2; Figure 1). In all ten validation populations, the A + A×A model was more accurate than the A-only model. The range of the prediction accuracy was 0.188–0.319 for the A-only model and was 0.197–0.333 for the A + A×A model, and the range of the increases in prediction accuracy of the A + A×A model over the A-only model was 3.02–14.29% (Figure 1). The standard deviation (SD) of the prediction accuracy was 0.045 for either model. The range of the prediction accuracy in terms of standard deviations from the mean was 0.96–1.96 SD for the A-only model and was 1.09–1.93 SD for the A + A×A model. These results showed that the variations of the two prediction models were all within the range of 1.09–1.96 SD or approximately −1.00 SD to 2.00 SD. Under the assumption of a normal distribution for the accuracy of predicting the RFI phenotypic values, the 95% interval of prediction accuracies from validations would be in the range of Mean ± 2.00 SD, and the observed ranges of 0.96–1.96 SD for the A-only model and 1.09–1.93 SD for the A + A×A model were all within the 95% interval. For the accuracy increase of the A + A×A model relative to the A-only model, the assumed 95% interval was −0.95% to 13.95%. The observed range of the accuracy increases in the ten validation populations was 3.02–14.29%, where the upper bound exceed that of the assumed 95% interval.
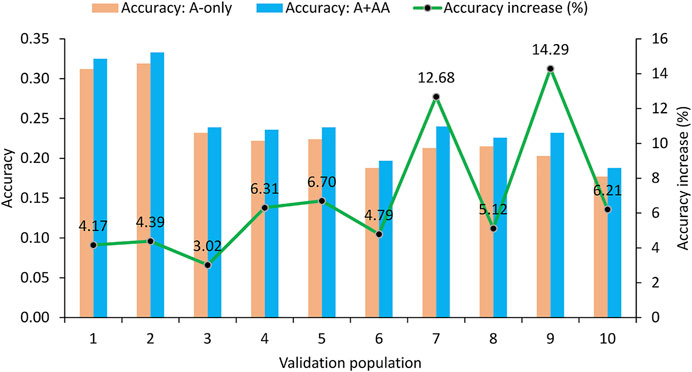
FIGURE 1. Accuracy of predicting RFI phenotypic values form 10-fold validation studies using 6215 Holstein cows.
Understanding of A×A heritability and prediction accuracy
The comparison of heritability estimates and prediction accuracy between the training and validation populations provided an understanding of the performance of A×A effects. The best prediction model for the training populations was the A×A-only model, with the highest prediction accuracy of 0.988 (Supplementary Table S3). This high prediction accuracy explained why the A×A-only model had the highest total heritability of 0.538, compared to the next highest total heritability of 0.406 from the full model with epistasis effects up to the third-order (Supplementary Table S3). This high prediction accuracy in the training populations likely was due to a mixture of model overfitting that accounted for some random residuals and true genetic effects. The evidence supporting the assumption of model overfitting was the high prediction accuracy of 0.988 in the training populations and the high heritability of 0.538 that substantially reduced the contribution of the random residuals to the phenotypic variance, although the exact amount of overfitting could not be determined. The evidence supporting the assumption of true A×A effects contributing to the 0.538 heritability estimate was the zero heritability of A×D that had twice as many effects as A×A, the zero heritability of D×D effects that had the same number of effects as the A×A, and the zero or nearly zero heritability of the third-order epistasis with many more effects than A×A (Supplementary Table S2). It is interesting to note that the integration of the A and A×A effects in the same model likely removed some inflation of the A×A heritability form the A×A-only model because the A×A heritability from the A + A×A model was 0.263, about half of the 0.538 heritability estimate from the A×A-only model. Since this 0.263 A×A heritability estimate still could be inflated for unknown reasons, this 0.263 estimate could be considered the upper bound of the true A×A heritability. The lower bound can be found based on the prediction efficiency of the additive and A×A effects. We define prediction efficiency as the ratio of the observed accuracy of predicting phenotypic values to the heritability estimate. Under this definition, the additive prediction efficiency of the A-only model was 0.231/0.171 = 1.35, and the A×A efficiency of the A×A-only model was 0.201/0.538 = 0.37. Therefore, the A×A efficiency was 27.41% of the additive efficiency. Requiring the additive efficiency for the A×A effects, the efficiency adjusted A×A heritability would be 0.201/1.35 = 0.155, which should be the lower bound of the A×A heritability because the main reason for the low A×A prediction efficiency likely was the low A×A genomic relationships between the validation and training individuals. Combining this result with the A×A heritability from the A + A×A model, the true A×A heritability should be in the range of 0.155–0.263.
The performance of the A×A-only model in the training and validation populations was drastically different. The A×A-only model had the highest prediction accuracy of 0.988 in the training populations and the lowest accuracy of 0.201 in the validation populations, an accuracy decrease of 12.99% relative the A-only model (Supplementary Table S3). The exact reasons for such a drastically different performance of the A×A-only model were unknown, but a likely reason was the low A×A genomic relationships between the training and validation populations relative to the additive genomic relationships, i.e.,
Accuracy of predicting RFI phenotypic values for different reliability levels
To study the effect of reliability levels on the accuracy of predicting phenotypic values, we compared the four measures of prediction accuracy defined by Eqs. 4–8 to provide an understanding of these measures in the 6215 Holstein cows, and then analyzed the accuracy of predicting the phenotypic values for cows with different reliability levels.
The A-only model had the highest average reliability of 0.339, followed by the 0.292 average additive reliability, 0.155 average reliability of the total genetic values, and 0.024 average reliability of A×A values under the A + A×A model (Table 3). The lower reliability of the A + A×A model was due to the larger genetic variance as the denominator of the reliability,
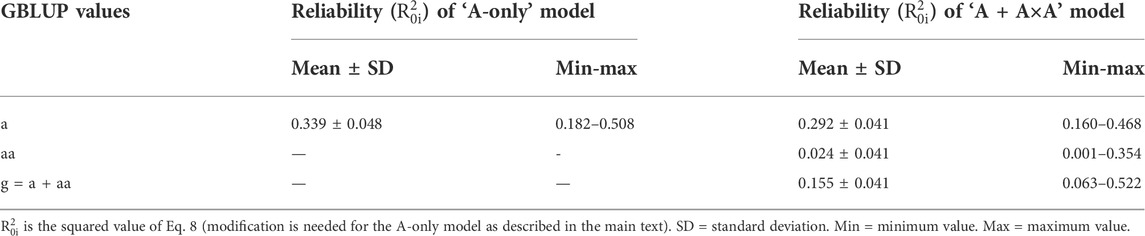
TABLE 3. Reliability estimates of validation cows for the A-only and A + A×A prediction models from 10 validation populations.
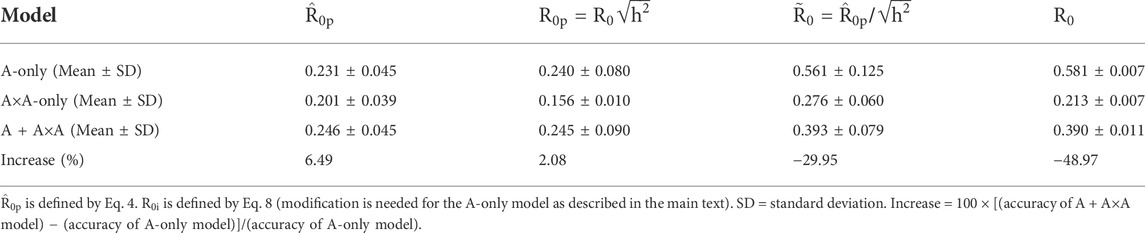
TABLE 4. Comparison of four measures of prediction accuracy in validation populations and differences in the four measures between the two prediction models.
The reliability levels were determined in two ways: Sorting reliability of predicting genetic values (
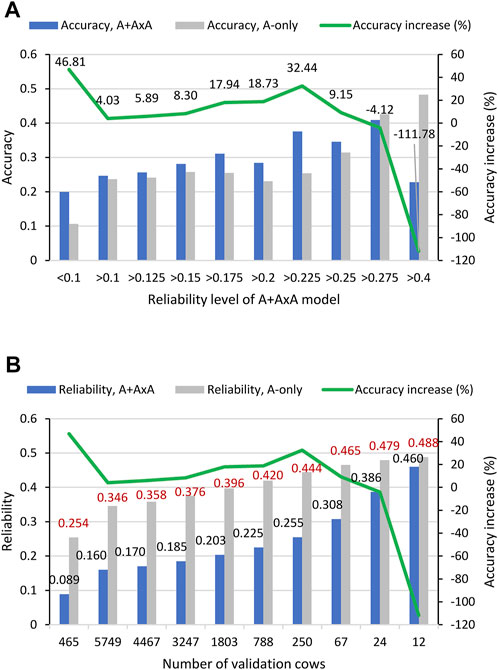
FIGURE 2. Accuracy of predicting RFI phenotypic values form 10-fold validation studies using 6215 Holstein cows for different reliability levels. (A) Accuracy of predicting RFI phenotypic values by the A + A×A and A-only models for different reliability levels of the GBLUP of total genetic values under the A + A×A model. The 6215 validation cows from the 10-fold validations were first sorted by the reliability of the GBLUP of the total genetic values under the A + A×A model, and the correlation between the GBLUP and phenotypic values of validation cows at each reliability level was calculated. Then the 6215 validation cows from the 10-fold validations were sorted by the reliability of the GBLUP of the additive values under the A-only model, and the correlation between the GBLUP and phenotypic values for the same number of validation cows at each reliability level of the GBLUP of total genetic values was calculated. The prediction accuracies of the two models were compared for the same number of cows. (B) Reliability of the GBLUP of total genetic values under the A + A×A model and additive reliability under the A-only model for the same numbers of cows. “Accuracy” is defined by Eq. 4. “Accuracy increase” = 100 × [(accuracy of A + A × A model)—(accuracy of A-only model)]/(accuracy of A-only model).
The results showed that the A + A×A model had higher accuracy of predicting the RFI phenotypic values than the A-only model for all reliability levels except the two highest reliability levels of >0.275 with 24 cows and >0.4 with 12 cows. The average reliability at each reliability of each model was 0.155–0.460 for the GBLUP of total genetic values of the A + A×A model and was 0.339–0.488 for additive reliability of the A-only model, and A-only reliability was higher than that of the A + A×A model at every reliability level (Figure 2B). For the highest reliability level with 12 cows, the A + A×A model had the worst performance relative to the A-only model, with prediction accuracy that was only 47.20% of the A-only accuracy and had lower accuracy for the second highest reliability level with 24 cows (Figure 2A). For the reliability level <0.09 with 465 cows, the A + A×A model had the highest accuracy increase relative to the A-only model, a 46.81% increase over the A-only model (Figures 2A,B). The fact that the inclusion of A×A epistasis effects in the prediction model improved the prediction accuracy over the A-only model for all cows except the 24 cows with the highest additive reliability under the A-only model indicated that the A + A×A model could be more beneficial than the A-only model for predicting the RFI phenotypic values for culling decisions, and that the A-only model could perform well for cows with the highest additive reliability. Given that RFI is expensive to measure and the growth of the RFI sample size is expected to be slow, the increase in reliability for about one million genomic evaluated cows every year is expected to be a slow process. This slow process should favor the A + A×A model for its better accuracy than the A-only model for all reliability levels except the 24 cows with the highest additive reliability.
Negative additive relationships leading to positive A×A relationships
Pedigree additive relationship between two individuals is positive, but a genomic additive relationship can be negative and genomic A×A relationship as the product of the additive relationship with itself is positive. The negative additive relationship could be interpreted as the two individuals involved being less related than two individuals with a positive genomic relationship, but the positive A×A relationship resulting from the negative additive relationship does not have a reasonable interpretation. Although this is a problem for interpreting the A×A relationship, the negative additive relationship and the positive A×A relationship resulting from the negative additive relationship are not problems for genomic prediction, because the additive and A×A relationships originated from the model matrix of additive effects (Da et al., 2022). Modifying the additive and A×A relationships is equivalent to modifying the additive and A×A model matrices. Therefore, for genomic prediction, negative additive relationships and positive A×A relationships resulting from the negative additive relationships should be left unchanged.
For this RFI dataset with 6215 cows, 55.15% of the 19,310,005 genomic additive relationships between two cows were negative, with an average value −0.022 (Supplementary Table S4). For the general formula of A×A relationship,
Inheritance of nonadditive effects
Additive effects are allelic effects and are inheritable because each parent transmits one allele of each locus to the offspring. This type of inheritance of transmitting the exact genetic material for a genetic effect from parents to offspring will be referred to as “direct inheritance”. The discussion of nonadditive inheritance uses the examples of dominance effects and A×A epistasis effects. The mating system is assumed a sire mating with many dams. With this mating system, the inheritance of dominance is indirect inheritance, whereas the inheritance of A×A effects involves both direct and indirect inheritance. We use “indirect inheritance” to refer to the inheritance where a parental allelic combination in daughters is the favorable genetic effect while each parental allele does not have a genetic effect.
Dominance effect is the difference between the heterozygous genotypic value and the average of the two homozygous genotypic values. A nonzero dominance effect is partial dominance if the heterozygous genotypic value is between two homozygous genotypic values, complete dominance if the heterozygous genotypic value is the same as one of the two homozygous genotypic values, or overdominance if the heterozygous genotypic value is more extreme than either of the two homozygous genotypic values. A genome-wide association study (GWAS) in Holstein cattle detected positive overdominance effects for the three yield traits and negative overdominance effects for fat and protein percentages (Jiang et al., 2019). The result of daughter genotypic arrays for given sire genotypes showed that the frequency of the offspring heterozygous genotype (Aa) was q, ½ and p for sire genotypes of AA, Aa and aa respectively, where p = frequency of the A allele and q = the frequency of the a allele in the population (Supplementary Table S3). Assuming p > q and q < ½, the aa sire genotype results in the highest heterozygosity in daughters, followed by Aa and AA. To quantify the impact of indirect inheritance, the difference between the heterozygosity of the daughters and the heterozygosity of the population will be termed as “daughter difference”, and the daughter difference relative to the population heterozygosity termed as ‘relative daughter difference’ (λ, Supplementary Table S5). Homozygous sire genotype of a rare allele (A or a) has the largest impact on increasing the heterozygosity in daughters. Based on this analysis, selection for sires with high dominance values in daughters theoretically could be selecting sires with the homozygous genotype of a rare allele and could dramatically increase the heterozygosity in future daughters and improve the dominance values of the future population, if the heterozygous genotype has a beneficial overdominance effect. As allele frequencies become closer to be equal, indirect inheritance diminishes, and dominance effect becomes completely noninheritable (Supplementary Figure S2).
An A×A effect is the interaction effect between the additive effects of two loci. Assuming two biallelic loci with alleles A and a at locus 1 and alleles B and b at locus 2, four allelic combinations between the two loci are possible, AB, Ab, aB, and ab; and nine two-locus genotypes are possible, AABB, AABb, AAbb, AaBB, AaBb, Aabb, aaBB, aaBb, and aabb. Assuming linkage equilibrium and the AB allelic combination to be the favorable combination and the other three combinations to be the unfavorable combinations, the inheritance of the favorable allelic combination (AB) is direct heritance for one sire genotype (AABB), is indirect inheritance for four sire genotypes (Aabb, AAbb, aaBB aaBb), and is a mixture of direct and indirect inheritance for two genotypes (AABb, AaBB), whereas the doubly heterozygous genotype (AaBb) does not change the gametic or genotypic frequencies of the daughters, and the inheritance of the ab combination is direct inheritance for the aabb sire genotype. This analysis shows that the inheritance of A×A effects involves both direct and indirect inheritance and is much more complex than that of additive effect. For this reason, the value of including A×A effect for sire selection should require further study. For predicting phenotypic values, the issue of A×A inheritance is not involved. Therefore, the utility of including A×A in the prediction model currently is for predicting phenotypic values that should benefit cow culling decision with respect to the cow’s phenotypic potential.
Conclusion
The inclusion of additive × additive effects along with additive effects in the prediction model improved the accuracy of predicting the RFI phenotypic values in U.S. Holstein cows over the accuracy of the additive-only model and is expected to have a positive economic impact on selecting the top 30% of cows with the best genomic evaluations. The nearly complete confounding between additive and intra-chromosome A×A effects and the nearly complete absence of confounding between additive and inter-chromosome A×A effects in terms of prediction accuracy and heritability estimates provided new evidence towards understanding the relationship between additive and A×A effects.
Data availability statement
Feed efficiency data are available to the public in summarized form, as predicted breeding values for residual feed intake and feed saved. Access to the original phenotypes for dry matter intake, secreted milk energy, metabolic body weight, body weight change, and residual feed intake may be requested, for research purposes, by contacting university partners in the multi-state feed efficiency collaborative. (Contact: Michael VandeHaar, mikevh@msu.edu).
Author contributions
YD conceived this study. ZL conducted the data analysis. DP contributed to the data work. MV, KW, JK, JS, HW, FP and RB contributed to the RFI data. RJT contributed to the RFI modeling. KG contributed to the RFI data organization. KW reviewed the manuscript. YD and ZL prepared the manuscript.
Funding
This research was supported by the National Institutes of Health’s National Human Genome Research Institute, grant R01HG012425 as part of the NSF/NIH Enabling Discovery through GEnomics (EDGE) Program; grant 2020-67015-31133 from the USDA National Institute of Food and Agriculture; and project MIN-16-124 of the Agricultural Experiment Station at the University of Minnesota.
Acknowledgments
Members of the Council on Dairy Cattle Breeding (CDCB) and the Cooperative Dairy DNA Repository (CDDR) are acknowledged for providing the dairy genomic evaluation data. The RFI data collection was partially supported by funding from the USDA National Institute of Food and Agriculture (Washington, DC; grant # 2011-68004-30340), the US Council on Dairy Cattle Breeding (Bowie, MD), and the US Foundation for Food and Agriculture Research (Washington, DC; grant # CA18-SS-0000000236). The Ceres and Atlas high performance computing systems of USDA-ARS were used for the data analysis and for the evaluation and testing of the EPIHAP computing package. Paul VanRaden and Steven Schroeder are acknowledged for help with the use of the CDCB data and USDA-ARS computing facilities. Paul VanRaden raised the issue of nonadditive inheritance. The use of the USDA-ARS computers by this research was supported by USDA-ARS projects 8042-31000-002-00-D and 8042-31000-001-00-D.
Conflict of interest
The authors declare that the research was conducted in the absence of any commercial or financial relationships that could be construed as a potential conflict of interest.
Publisher’s note
All claims expressed in this article are solely those of the authors and do not necessarily represent those of their affiliated organizations, or those of the publisher, the editors and the reviewers. Any product that may be evaluated in this article, or claim that may be made by its manufacturer, is not guaranteed or endorsed by the publisher.
Supplementary material
The Supplementary Material for this article can be found online at: https://www.frontiersin.org/articles/10.3389/fgene.2022.1017490/full#supplementary-material
References
CDCB (2022). Genotypes included in evaluations by breed, chip density, presence of phenotypes (old vs. young), and evaluation year-month (cumulative). Available at: https://queries.uscdcb.com/Genotype/cur_density.html.
Cockerham, C. C. (1954). An extension of the concept of partitioning hereditary variance for analysis of covariances among relatives when epistasis is present. Genetics 39 (6), 859–882. doi:10.1093/genetics/39.6.859
Da, Y., Liang, Z., and Prakapenka, D. (2022). Multifactorial methods integrating haplotype and epistasis effects for genomic estimation and prediction of quantitative traits. Front. Genet. 13, 922369. doi:10.3389/fgene.2022.922369
Gaddis, K., VanRaden, P., Tempelman, R., Weigel, K., White, H., Peñagaricano, F., et al. (2021). Implementation of feed saved evaluations in the US. Interbull Bull. 56, 147–152.
Henderson, C. (1985). Best linear unbiased prediction of nonadditive genetic merits in noninbred populations. J. Animal Sci. 60 (1), 111–117. doi:10.2527/jas1985.601111x
Jiang, J., Ma, L., Prakapenka, D., VanRaden, P. M., Cole, J. B., and Da, Y. (2019). A large-scale genome-wide association study in US Holstein cattle. Front. Genet. 10, 412. doi:10.3389/fgene.2019.00412
Jiang, Y., and Reif, J. C. (2020). Efficient algorithms for calculating epistatic genomic relationship matrices. Genetics 216 (3), 651–669. doi:10.1534/genetics.120.303459
Kennedy, B., Van der Werf, J., and Meuwissen, T. (1993). Genetic and statistical properties of residual feed intake. J. Anim. Sci. 71 (12), 3239–3250. doi:10.2527/1993.71123239x
Koch, R. M., Swiger, L. A., Chambers, D., and Gregory, K. E. (1963). Efficiency of feed use in beef cattle. J. Animal Sci. 22 (2), 486–494. doi:10.2527/jas1963.222486x
Legarra, A., Robert-Granié, C., Manfredi, E., and Elsen, J.-M. (2008). Performance of genomic selection in mice. Genetics 180 (1), 611–618. doi:10.1534/genetics.108.088575
Li, B., Fang, L., Null, D., Hutchison, J., Connor, E., VanRaden, P., et al. (2019). High-density genome-wide association study for residual feed intake in Holstein dairy cattle. J. Dairy Sci. 102 (12), 11067–11080. doi:10.3168/jds.2019-16645
Li, B., VanRaden, P., Guduk, E., O'Connell, J., Null, D., Connor, E., et al. (2020). Genomic prediction of residual feed intake in US Holstein dairy cattle. J. Dairy Sci. 103 (3), 2477–2486. doi:10.3168/jds.2019-17332
Liang, Z., Prakapenka, D., and Da, Y. (2022). Comparison of two methods of genomic epistasis relationship matrices using daughter pregnancy rate in U.S. Holstein cattle. Abstract 2466V, page 409 of ADSA2022 Abstracts. Available at: https://www.adsa.org/Portals/0/SiteContent/Docs/Meetings/2022ADSA/Abstracts_BOOK_2022.pdf?v=20220613.
Liang, Z., Prakapenka, D., and Da, Y. (2021). Epihap: A computing tool for genomic estimation and prediction using global epistasis effects and haplotype effects. Abstract P167, page 223 of ADSA2021 Abstracts, ADSA 2021 Virtual Annual Meeting. Available at: https://www.adsa.org/Portals/0/SiteContent/Docs/Meetings/2021ADSA/ADSA2021_Abstracts.pdf.
Martini, J. W., Toledo, F. H., and Crossa, J. (2020). On the approximation of interaction effect models by Hadamard powers of the additive genomic relationship. Theor. Popul. Biol. 132, 16–23. doi:10.1016/j.tpb.2020.01.004
Muñoz, P. R., Resende, M. F., Gezan, S. A., Resende, M. D. V., de los Campos, G., Kirst, M., et al. (2014). Unraveling additive from nonadditive effects using genomic relationship matrices. Genetics 198 (4), 1759–1768. doi:10.1534/genetics.114.171322
Prakapenka, D., Liang, Z., Jiang, J., Ma, L., and Da, Y. (2021). A Large-scale genome-wide association study of epistasis effects of production traits and daughter pregnancy rate in US Holstein cattle. Genes 12 (7), 1089. doi:10.3390/genes12071089
Pryce, J., Wales, W. d., De Haas, Y., Veerkamp, R., and Hayes, B. (2014). Genomic selection for feed efficiency in dairy cattle. Animal 8 (1), 1–10. doi:10.1017/S1751731113001687
Su, G., Christensen, O. F., Ostersen, T., Henryon, M., and Lund, M. S. (2012). Estimating additive and non-additive genetic variances and predicting genetic merits using genome-wide dense single nucleotide polymorphism markers. PloS One 7 (9), e45293. doi:10.1371/journal.pone.0045293
Tempelman, R., Spurlock, D., Coffey, M., Veerkamp, R., Armentano, L., Weigel, K., et al. (2015). Heterogeneity in genetic and nongenetic variation and energy sink relationships for residual feed intake across research stations and countries. J. Dairy Sci. 98 (3), 2013–2026. doi:10.3168/jds.2014.8510
VanRaden, P., Cole, J., and Gaddis, K. P. (2014). Net merit as a measure of lifetime profit: 2014 revision. AIP Res. Rep. 7, 5–18.
VanRaden, P., O’Connell, J., Connor, E., Vandehaar, M., Tempelman, R., and Weigel, K. (2018). “Including feed intake data from US Holsteins in genomic prediction,” in Proc. 11th world congress on genetics applied to Livestock production, vol. Biology–Feed intake and efficiency 1 (Auckland, New Zealand: World Congress on Genetics Applied to Livestock Production), 125.
Vitezica, Z. G., Legarra, A., Toro, M. A., and Varona, L. (2017). Orthogonal estimates of variances for additive, dominance, and epistatic effects in populations. Genetics 206 (3), 1297–1307. doi:10.1534/genetics.116.199406
Yao, C., Spurlock, D., Armentano, L., Page, C., VandeHaar, M., Bickhart, D., et al. (2013). Random Forests approach for identifying additive and epistatic single nucleotide polymorphisms associated with residual feed intake in dairy cattle. J. Dairy Sci. 96 (10), 6716–6729. doi:10.3168/jds.2012-6237
Keywords: RFI, holstein, epistasis, SNP, genomic prediction, heritability, GBLUP, GREML
Citation: Liang Z, Prakapenka D, Parker Gaddis KL, VandeHaar MJ, Weigel KA, Tempelman RJ, Koltes JE, Santos JEP, White HM, Peñagaricano F, Baldwin VI RL and Da Y (2022) Impact of epistasis effects on the accuracy of predicting phenotypic values of residual feed intake in U. S Holstein cows. Front. Genet. 13:1017490. doi: 10.3389/fgene.2022.1017490
Received: 12 August 2022; Accepted: 27 September 2022;
Published: 01 November 2022.
Edited by:
Wansheng Liu, The Pennsylvania State University (PSU), United StatesReviewed by:
Ning Gao, Hunan Agricultural University, ChinaChad Dechow, The Pennsylvania State University (PSU), United States
Copyright © 2022 Liang, Prakapenka, Parker Gaddis, VandeHaar, Weigel, Tempelman, Koltes, Santos, White, Peñagaricano, Baldwin VI and Da. This is an open-access article distributed under the terms of the Creative Commons Attribution License (CC BY). The use, distribution or reproduction in other forums is permitted, provided the original author(s) and the copyright owner(s) are credited and that the original publication in this journal is cited, in accordance with accepted academic practice. No use, distribution or reproduction is permitted which does not comply with these terms.
*Correspondence: Yang Da, yda@umn.edu