- 1Departments of Orthopedics, Shanghai Eighth People’s Hospital, Shanghai, China
- 2Department of Neurology, The First Affiliated Hospital of Anhui Medical University, Hefei, China
- 3Departments of Orthopedics, Sixth People’s Hospital Affiliated to Shanghai Jiao Tong University, Shanghai, China
Osteosarcoma (OS) is a common bone cancer in children and adolescents, and metastasis and recurrence are the major causes of poor treatment outcomes. A better understanding of the tumor microenvironment is required to develop an effective treatment for OS. In this paper, a single-cell RNA sequencing dataset was taken to a systematic genetic analysis, and potential signaling pathways linked with osteosarcoma development were explored. Our findings revealed 25 clusters across 11 osteosarcoma tissues, with 11 cell types including “Chondroblastic cells”, “Osteoblastic cells”, “Myeloid cells”, “Pericytes”, “Fibroblasts”, “Proliferating osteoblastic cells”, “Osteoclasts”, “TILs”, “Endothelial cells”, “Mesenchymal stem cells”, and “Myoblasts”. The results of Cell communication analysis showed 17 potential cellular communication networks including “COLLAGEN signaling pathway network”, “CD99 signaling pathway network”, “PTN signaling pathway network”, “MIF signaling pathway network”, “SPP1 signaling pathway network”, “FN1 signaling pathway network”, “LAMININ signaling pathway network”, “FGF signaling pathway network”, “VEGF signaling pathway network”, “GALECTIN signaling pathway network”, “PERIOSTIN signaling pathway network”, “VISFATIN signaling pathway network”, “ITGB2 signaling pathway network”, “NOTCH signaling pathway network”, “IGF signaling pathway network”, “VWF signaling pathway network”, “PDGF signaling pathway network”. This research may provide novel insights into the pathophysiology of OS’s molecular processes.
Introduction
Osteosarcoma (OS) is a highly malignant solid bone tumor characterized by malignant mesenchymal cells producing pathological osteoid and/or bony matrix; it accounts for roughly 60% of all pediatric malignancies (Bousquet et al., 2016; Guo et al., 2022; Ho et al., 2017), and the incidence of OS in the overall population is two to three million per year (Shao et al., 2022). Clinical signs of OS affect the proximal tibia, proximal humerus, and distal femur, and consist predominantly of local discomfort, edema, and reduced joint movement (Rothzerg et al., 2021). Currently, this cancer is treated with surgical excision and chemotherapy with many agents. Unfortunately, the 5-years overall survival rate for osteosarcoma patients was just approximately 60% among patients with localized osteosarcoma but is only 20% among patients presenting with metastases or recurrent disease (Meltzer and Helman, 2021). The pathophysiology of OS is characterized by the substantial infiltration of complex cells, including malignant mesenchymal stem cells, proliferating osteoblastic cells, osteoblastic cells, immunological cells, and vascular networks, indicating the existence of a highly complex tumor microenvironment (TME) (Kansara et al., 2014). Nonetheless, the potential cellular communication networks of these cells are still not fully elucidated.
To understand cancer biology and immunology and to get the most out of tumor immunotherapy, it is important to figure out how this ecosystem’s cells work together and how they might talk to each other. The ultimate unit of biological activity is a single cell, where genetic processes interact with the cellular environment to determine the development and function of complex structures including tissues and organs. Understanding the biology of virtually all living phenomena in normal and disease states necessitates dissecting and characterization of their composition and characterization, as well as evaluating their interactions, dynamics, and function at the single-cell level (Ren et al., 2018). Technically, however, previous genomic, transcriptomic, and proteomic cancer investigations have been unable to comprehensively elaborate on TME due to its complexity.(Liu et al., 2021). The emergence of new technologies based on single-cell sequencing has enabled unparalleled resolution and scale in capturing diverse tumor stages and understanding tumor heterogeneity (Vegliante et al., 2022). Rapid advancements in single-cell technology provide us with a potent approach to examine the multiple allosteric states and potential cellular communication networks of the TME at the single cell level.
This study employed scRNA-seq to investigate potential cellular communication networks in the OS’s TME, as well as trajectory analysis and transcription factor enrichment analysis among mesenchymal stem cells, proliferating osteoblastic cells, and osteoblastic cells.
Materials and methods
Data source collection and processing
The 11 OS samples with scRNA-seq data based on the 10X Genomics platform were downloaded from GSE152048 via the Gene Expression Omnibus database (https://www.ncbi.nlm.nih.gov/geo/). The Seurat package (v4.1.1) was used to load the 10X genomics data for each individual sample into R software (v4.1.3). We excluded cells with identified genes <300 or a percentage of mitochondrial genes over 10% of total expressed genes. We eliminated low-quality cells with ≥7,500 detected genes, as well as genes detected in fewer than three cells. Furthermore, using the DoubletFinder package (v2.0.3), we eliminated any doublets that might have happened during encapsulation or as random pairings of cells that were not separated during sample preparation.
This research did not need ethical approval for our work because we used data from a publicly accessible database.
Identification of cell types
For each cell, gene expression was expressed as a fraction of the gene multiplied by 10,000, The log (x+1) method was used to perform natural log transformation. We identified, and scaled the top 2000 highly variable genes (HVGs) from the normalized expression matrix before doing principal component analysis (PCA) on these HVGs. Based on the top 50 PCA components identified, the batch effects were removed using the R Harmony package (version 1.0) (Zhou et al., 2020). On the basis of harmony-corrected data, k-nearest neighbors were estimated, and a shared nearest neighbor (SNN) graph was formed. The modular function was then adjusted to achieve cluster recognition based on the clustering algorithm. On the 2D map generated with the t-distributed stochastic neighbor embedding (tSNE) or uniform manifold approximation and projection for dimension reduction (UMAP) approach, the identified clusters were displayed.
Using the “FindAllMarkers” function, each cluster’s marker genes were identified according to the following criteria: logfc. threshold = 0.25, min. pct = 0.25, and min. diff.pct = 0.25. Using the “DotPlot” tool in Seurat, the expression pattern of each marker gene across clusters was shown. The cell groupings were annotated based on the DEGs and well-known cellular markers described in the scientific literature (Zhou et al., 2020).
Pseudotemporal ordering of single cells
The Monocle package (v2.22.0) was used to produce the single-cell pseudotime trajectories. Using pseudotemporal profiling of scRNA-seq data, Monocle aims to decipher cellular changes during differentiation. After inputting the scale of raw UMI counts into the “newCellDataSet” function with its clustering information, it was computed into a lower dimensional space using the discriminative dimensionality reduction with trees (DDRTree) method, a more recent manifold learning algorithm. Mesenchymal stem cells, proliferating osteoblastic cells, and osteoblastic cells were then ordered according to pseudotime. The plot pseudotime heatmap was used to compute and illustrate the genes whose expression varied in tandem with pseudotime.
Cell-cell communication
The CellChat package (version 1.4.0) predicted cell–cell communication across all cell types based on single-cell RNA sequencing data (Jin et al., 2021). Only the ligand–receptor interaction with a p-value 0.05 was utilized to predict cell–cell interaction in the various cell types.
SCENIC analysis
SCENIC is a technique that uses scRNA-seq data to rebuild gene regulation networks while also recognizing stable cell states. Transcription factor enrichment and regulon activity were assessed using SCENIC package (version 1.3.1) is introduced (Aibar et al., 2017). Based on co-expression and DNA motif analysis, the gene regulatory network was created, and the network activity in each cell was assessed to determine the cell state. For transcription factor regulatory network development, two gene-motif rankings (10 kb around the transcription start site or 500 bp upstream and 100 bp downstream of the transcription start site) were used as a guide to set the search space around the transcription start site. The gene-motif rankings for humans are obtained from https://resources.aertslab.org/cistarget/. The database used was Hallmark Gene Set from Molecular Signatures database (MsigDB) (Liberzon et al., 2015). In addition, Gene regulation was constructed using the R package GENIE3 (version 1.16.0), RcisTarget (version 1.14.0) and AUCell (version 1.16.0).
Results
Quality control and removal of batch effect
Eleven OS patients with scRNA-seq data enrolled in this research. Using the R Harmony package (version 1.0), batch effects between samples were eliminated based on the top 50 PCA components. After removal of batch effect, we used the t-SNE and UMAP techniques to decrease dimensionality, and then plotted the result as a 2D scatter plot (Figure 1A). In the process of quality control, we eliminated cells with fewer than 300 identified genes or a proportion of mitochondrial genes exceeding 10% of all expressed genes (Figure 1B). Dot plot of data quality control in scRNA-seq data were shown in Figure 1C.
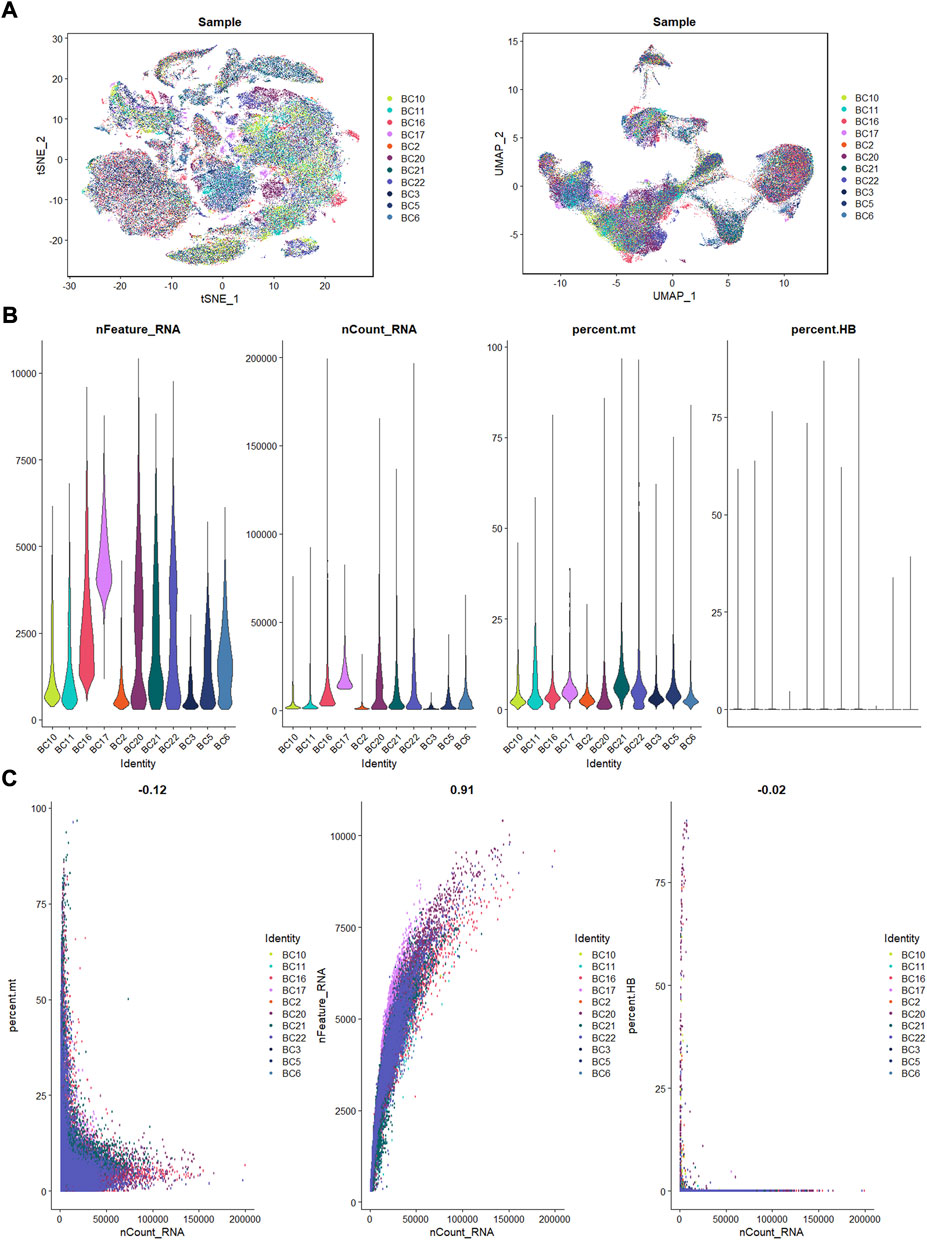
FIGURE 1. The process of quality control. (A): t-SNE and UMAP plots after harmony. (B): violin plots of feature, count, percent. mt, and percent.HB. (C): correlation plots for count and feature, percent. mt, percent. HB.
Identification of 25 cell clusters in osteosarcoma microenvironment using scRNA-seq data reveals high cell heterogeneity
Following the quality control standard, 110,042 cells were finally included in our analysis. These cells were clustered into 25 primary cell clusters (Figures 2A,B; Figures 3A,C). A value of adjusted p value <0.01 is displayed in red, whereas a value of adjusted p value ≥0.01 is displayed in black (Figure 2B). Analysis of differential gene expression revealing up- and down-regulated genes in all clusters. The cluster-specific markers were utilized to label cell types (Figures 3B,D,E): chondroblastic cells (Sox9, Acan, Pth1r), osteoblastic cells (Runx2, Col1a1, Cdh11, Ibsp), myeloid cells (Cd74, Cd14, Fcgr3a), pericytes (Rgs5, Acta2), fibroblasts (Dcn, Col1a1), proliferating osteoblastic cells (Mki67, Top2a, Pcna), osteoclasts (ACP5, Ctsk, Mmp9), TILs (IL7R, CD3D, NKG7), endothelial cells (Pecam1, Vwf), mesenchymal stem cells (Mme, Thy1, Cxcl12, Sfrp2), and myoblasts (Myl1, Mylpf).
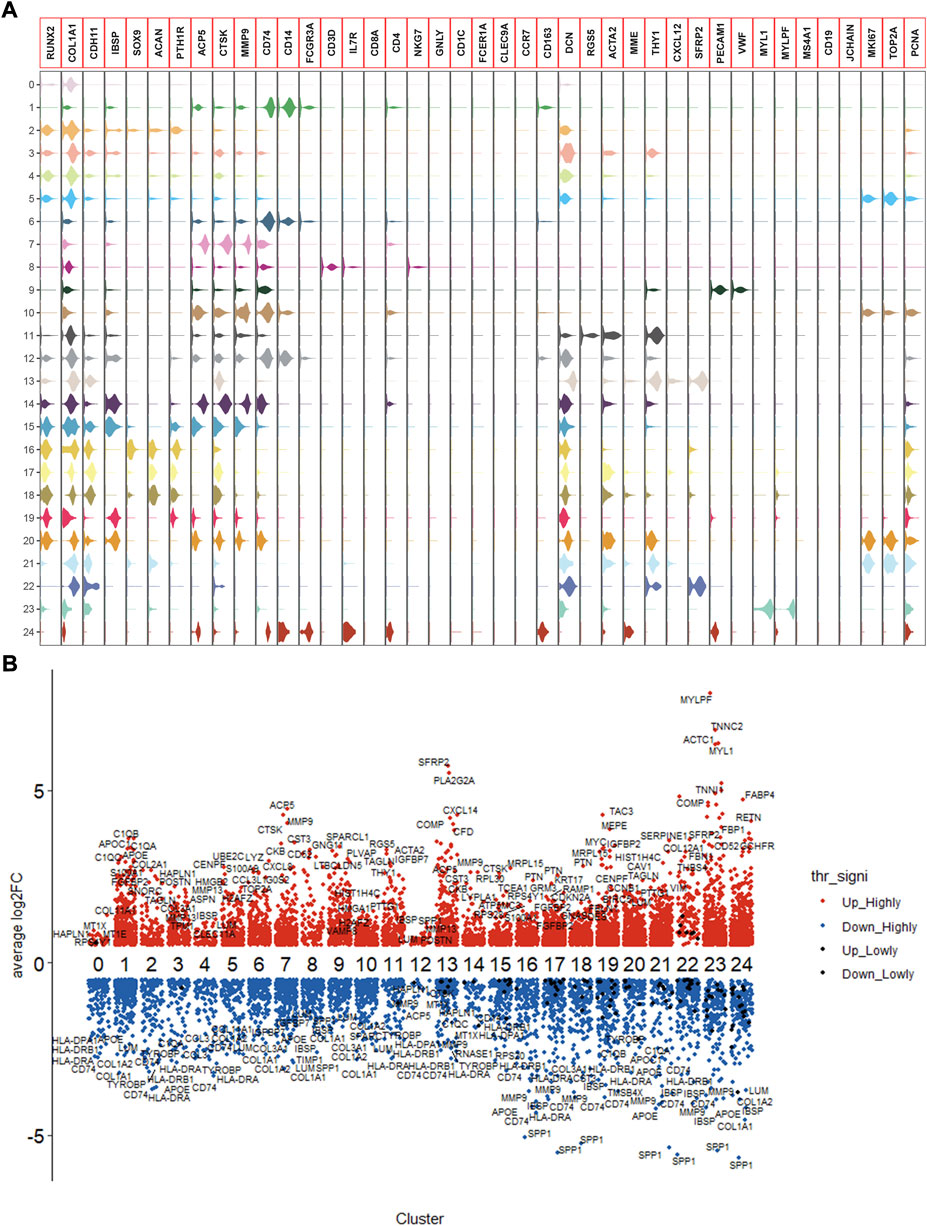
FIGURE 2. Expression of marker genes in the OS. (A): violin plot of marker genes. (B): columnar scatter plot of DEGs.
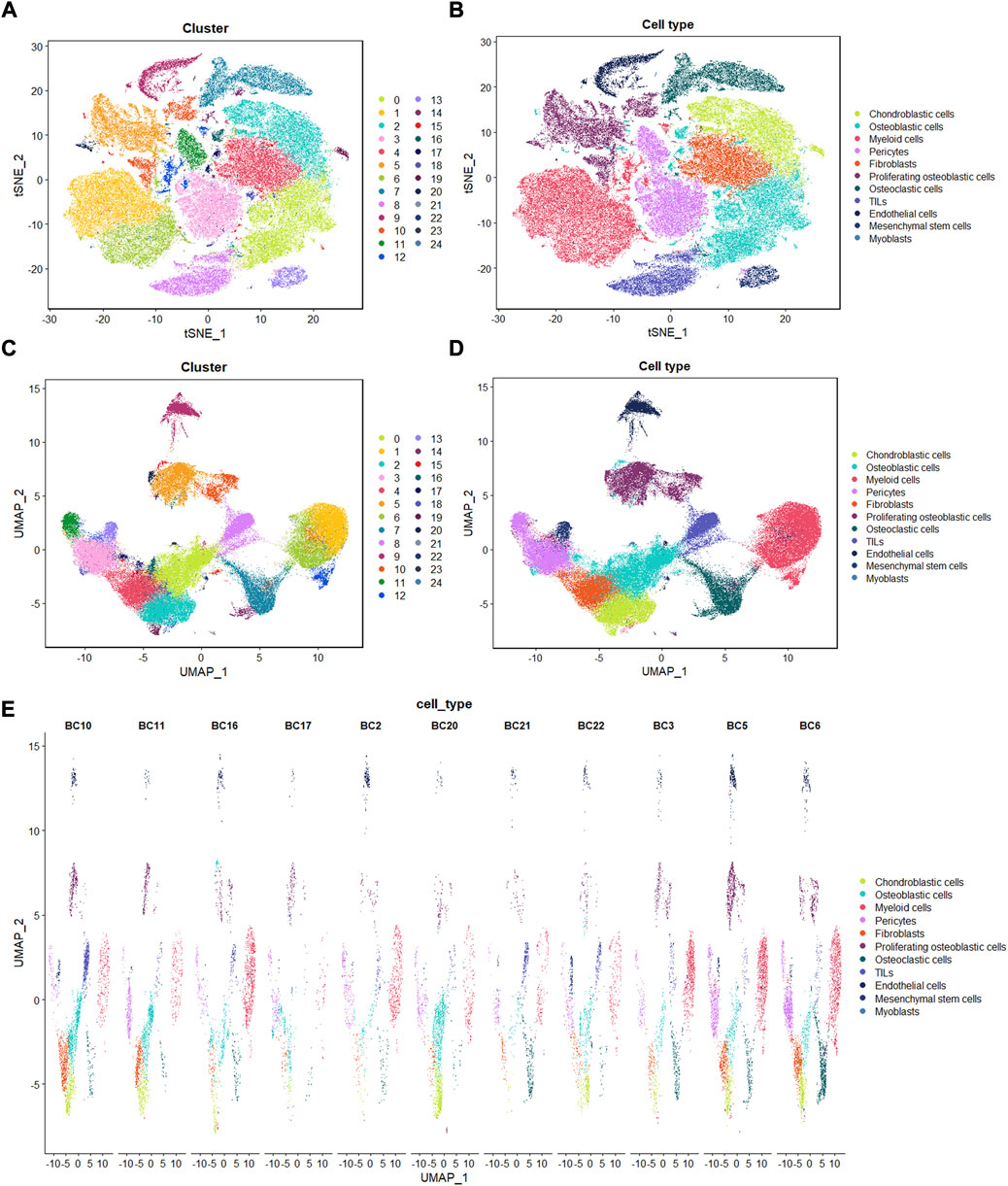
FIGURE 3. Single-cell transcriptomic analysis of OS lesions. (A,C): t-SNE and UMAP analysis showing the results of descending clustering. (B,D,E): t-SNE and UMAP analysis showing the results of annotation of cell subpopulations in cells of OS tissues.
Potential cellular communication networks in the osteosarcoma microenvironment
To identify the potential molecular connections between cells, CellChat package (version 1.4.0) of R was utilized to find the potential molecular interactions between ligand-receptor pairings and main cell types in order to build cellular communication networks. First, CellChat was used to analyze cellular communication among the chondroblastic cells, osteoblastic cells, myeloid cells, pericytes, fibroblasts, proliferating osteoblastic cells, osteoclasts, TILs, endothelial cells, mesenchymal stem cells, and myoblasts. The results of the CellChat analysis revealed the numbers and weights of ligand receptors among all cell types (Figures 4A,B). The outgoing and incoming signaling patterns were shown in (Figures 4C,D). The outgoing and incoming interaction strength were shown in (Figures 4B,C) (B: all signaling pathway networks identified; C: selected signaling pathway networks). In addition, all of their ligand-receptor interactions have been identified (Figure 5D).
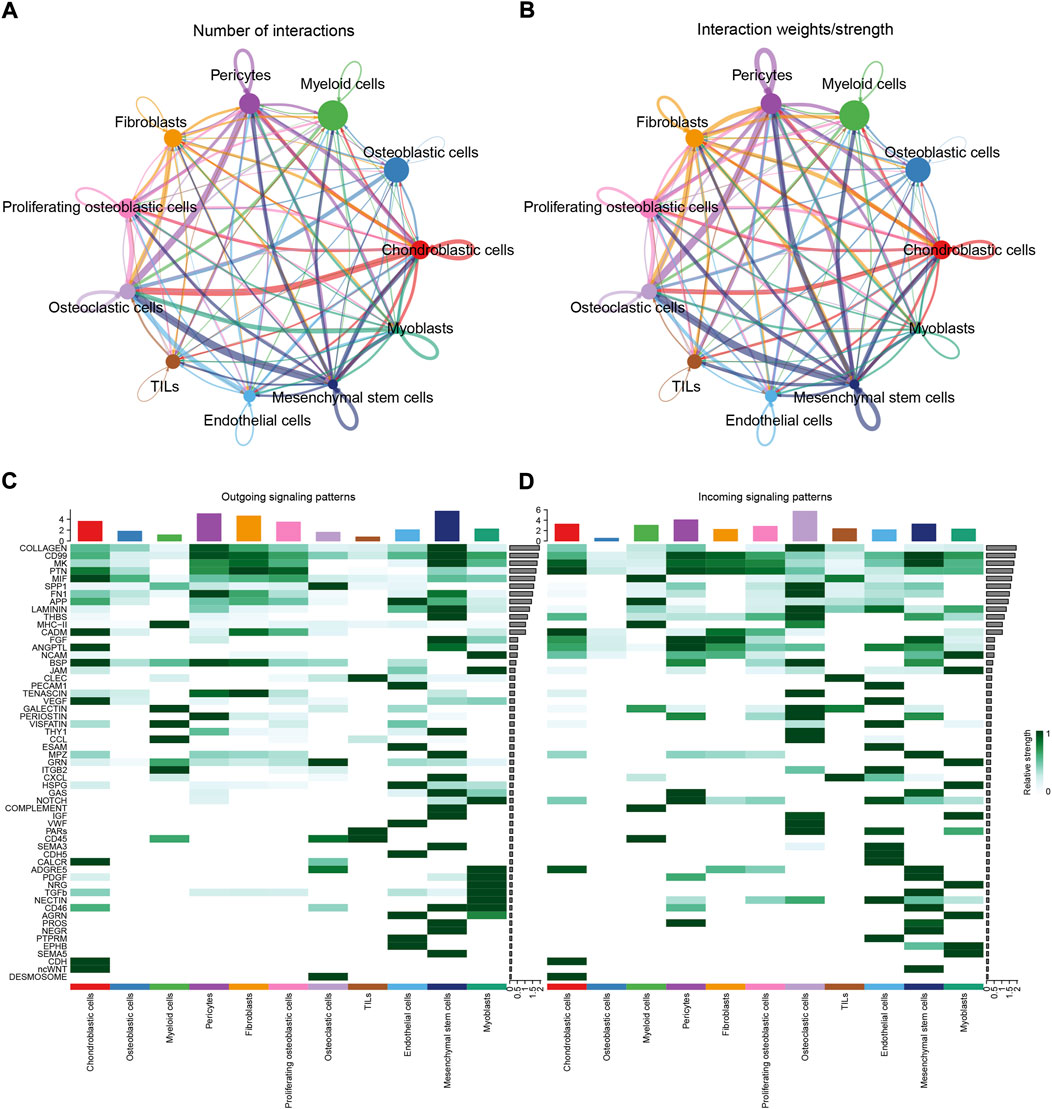
FIGURE 4. Cell–cell communication network among different cell types. (A,B): the numbers and weights of ligand receptors among all cell types. (C,D): the outgoing and incoming interaction strength among all cell types.
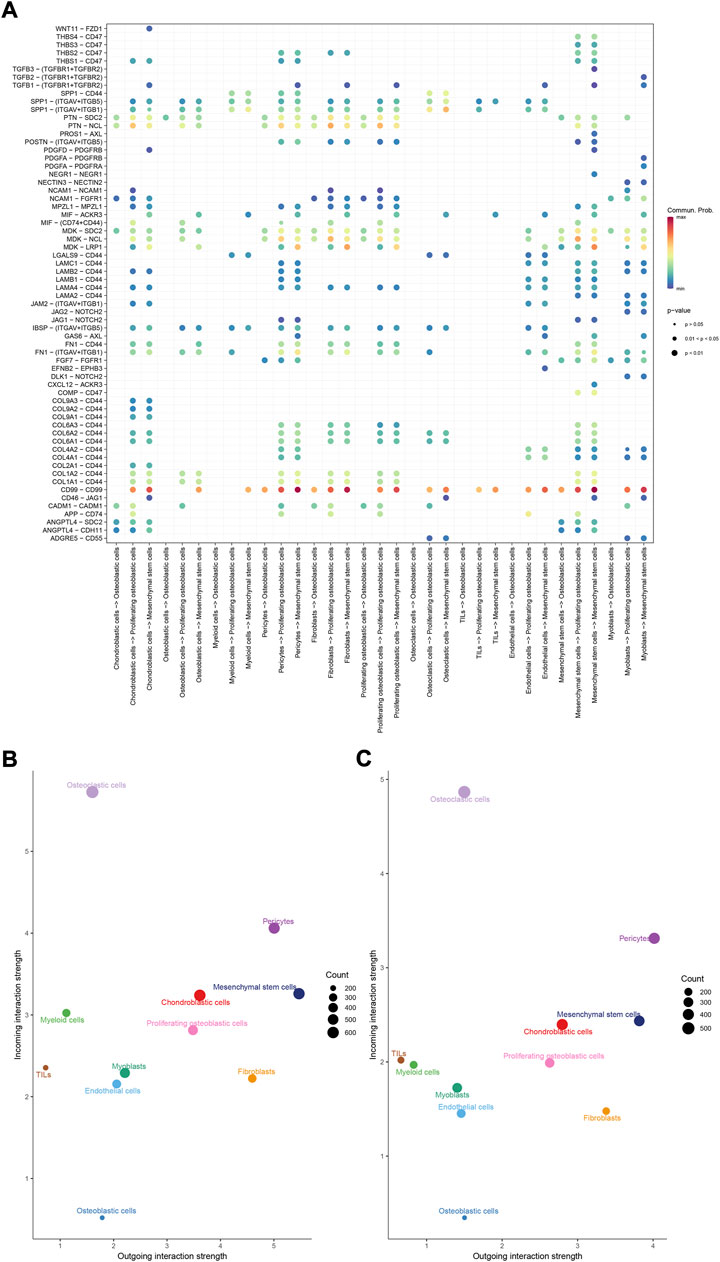
FIGURE 5. Overview of all ligand-receptor interactions of cells in OS. (A): all ligand-receptor interactions of cells in OS. (B,C): all signaling pathway networks identified, selected signaling pathway networks.
The details of all signaling pathway networks identified were also shown in Figures 6A-C (A: numbers of ligand receptors among all cell types; B: weights of ligand receptors among all cell types; C: chordal graph of ligand-receptor interactions among all cell types). Among the total of 57 signaling pathways, the following signaling pathways were related to osteosarcoma: COLLAGEN (Baumann and Hennet, 2016; Elenjord et al., 2009; Levinson et al., 2002; Yamaguchi et al., 2005), CD99 (Manara et al., 2006; Sciandra et al., 2014; Zucchini et al., 2014), PTN (He et al., 2019; Qin et al., 2022; Sun et al., 2020), MIF(Liu et al., 2014), SPP1(Dalla-Torre et al., 2006; Li et al., 2017), FN1(Saba et al., 2019; Zhou et al., 2019), LAMININ(Heino and Massague, 1989), FGF (Kurogi et al., 1996; Laulederkind et al., 2000; Li et al., 2019; Xu et al., 2010), VEGF (Ji et al., 2020; Lei et al., 2018; Oda et al., 2006; Tsai et al., 2017; Zhang et al., 2019), GALECTIN(Gomez-Brouchet et al., 2010; Miao et al., 2014; Park et al., 2015; Zhou et al., 2014), PERIOSTIN(Ma et al., 2020; Xu et al., 2022), VISFATIN(Cheng et al., 2015; Wang et al., 2019, 2016), ITGB2 (Dai et al., 2018), NOTCH(Jin et al., 2017; Mu et al., 2013; Ongaro et al., 2016; Tanaka et al., 2009; Zhang et al., 2010), IGF (Armakolas et al., 2016; Giatagana et al., 2022; Gvozdenovic et al., 2017; Molina et al., 2019; Tan et al., 2015), VWF(Wang et al., 2020), and PDGF (Chen et al., 2009; Egners et al., 2018; Heldin et al., 1986). The ligand-receptor interactions of these signaling pathways related to osteosarcoma were shown in Figure 6D. Furthermore, according to the results of this research, the potential communication of mesenchymal stem cells, proliferating osteoblastic cells, and osteoblastic cells mainly revolved around SPP1 (Figure 7), FGF (Figure 8), NOTCH (Figure 9).
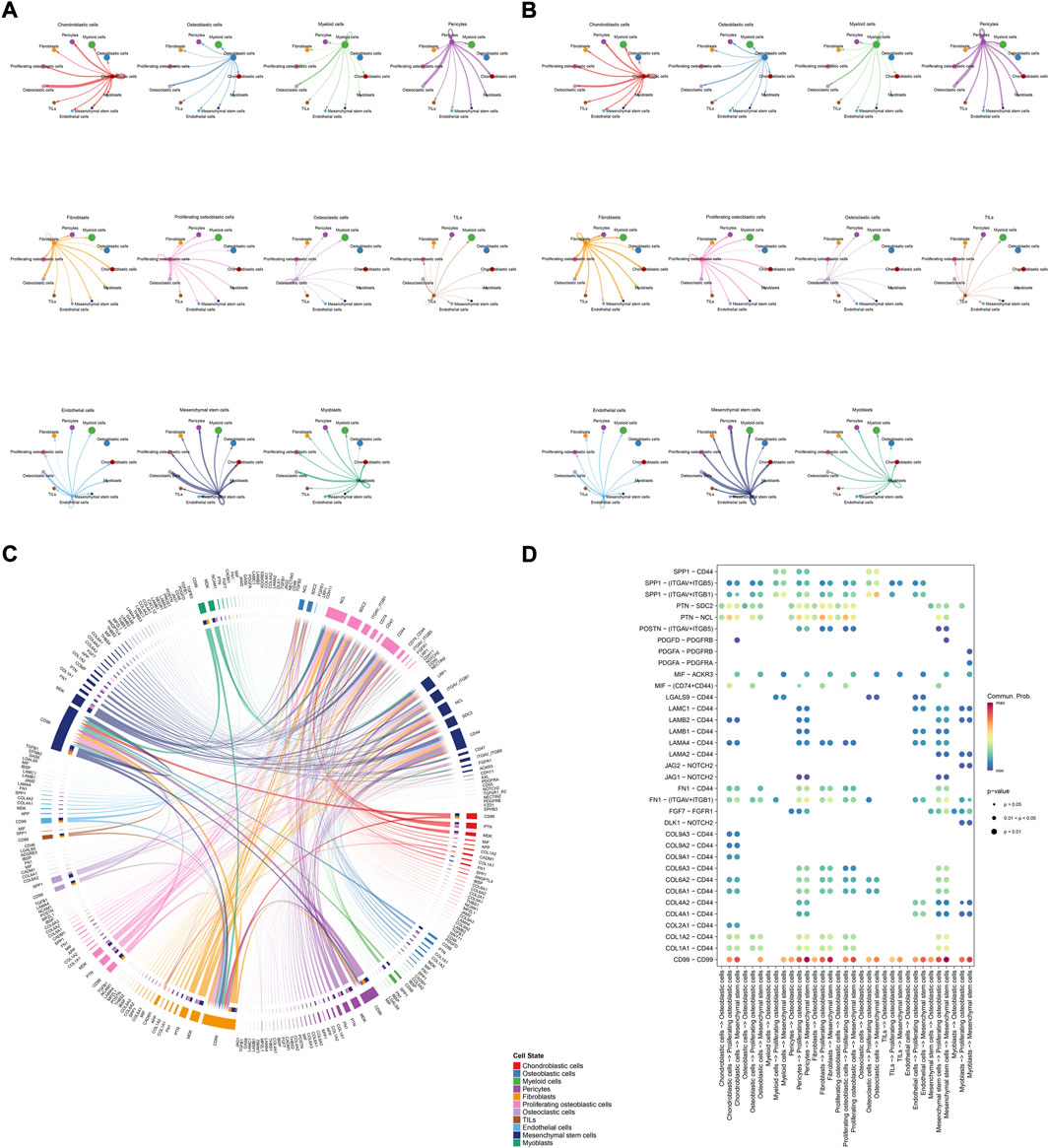
FIGURE 6. Detailed view of the ligands expressed by each major cell type. (A): a detailed perspective of the ligands expressed by each major cell type and the cells expressing the signal-receiving receptors is provided, the thickness of the lines indicated the numbers of ligand-receptor pairs for each intercellular link. (B): the thickness of the lines indicated the weights of ligand-receptor pairs for each intercellular link. (C): chordal graphs showing potential cellular crosstalk within the OS microenvironment. (D): the selected ligand-receptor interactions related to OS.
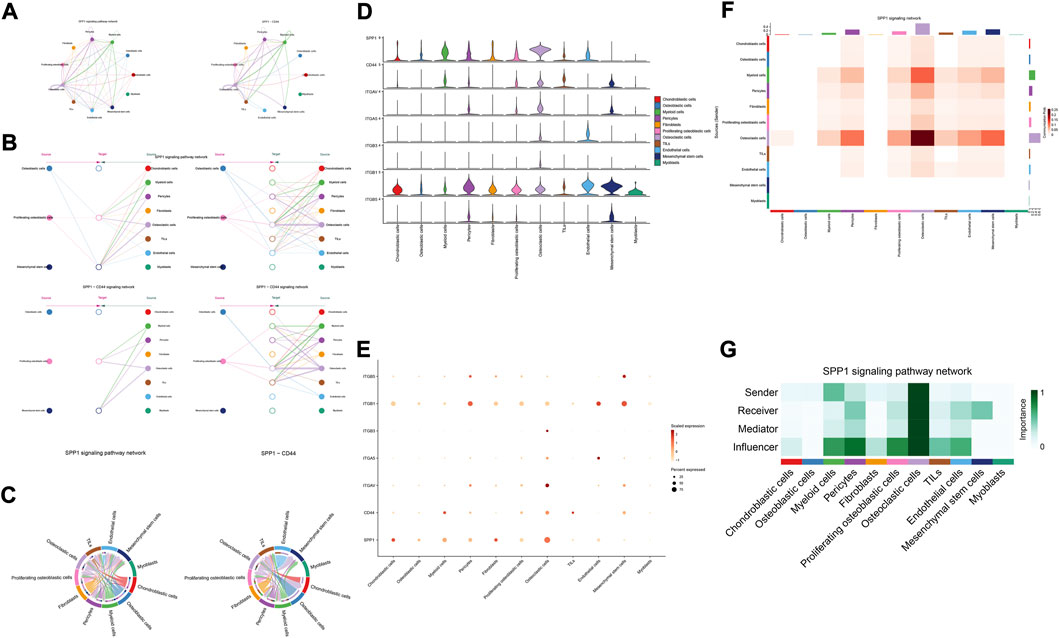
FIGURE 7. SPP1 signaling pathway. (A–C): ligand-receptor interactions of cells. (D,E): the ligand-receptors expressed by each major cell type. (F,G): the network of SPP1 signaling pathway.
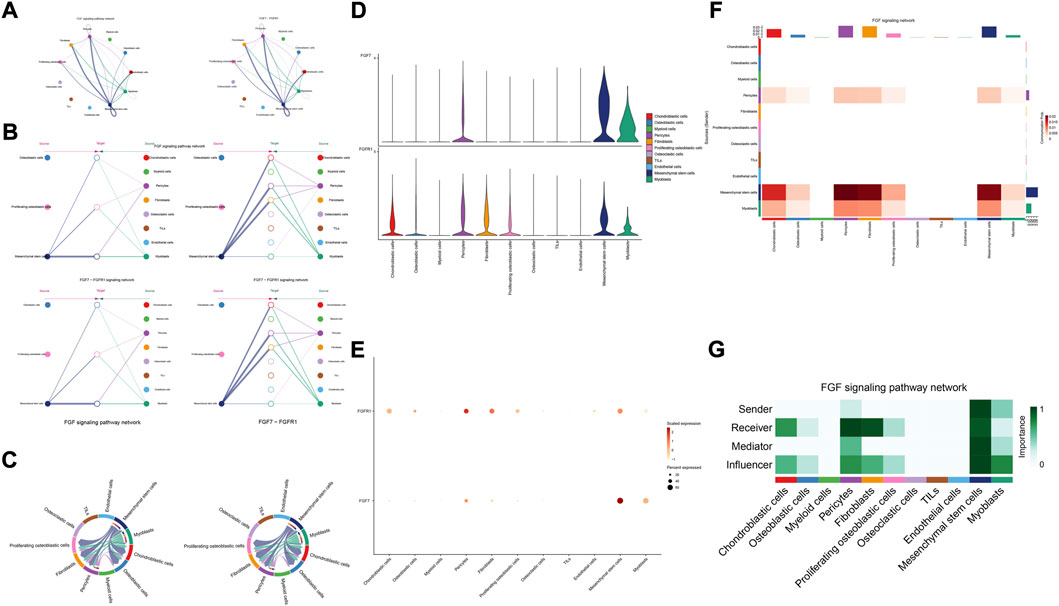
FIGURE 8. FGF signaling pathway. (A–C): ligand-receptor interactions of cells. (D,E): the ligand-receptors expressed by each major cell type. (F,G): the network of FGF signaling pathway.
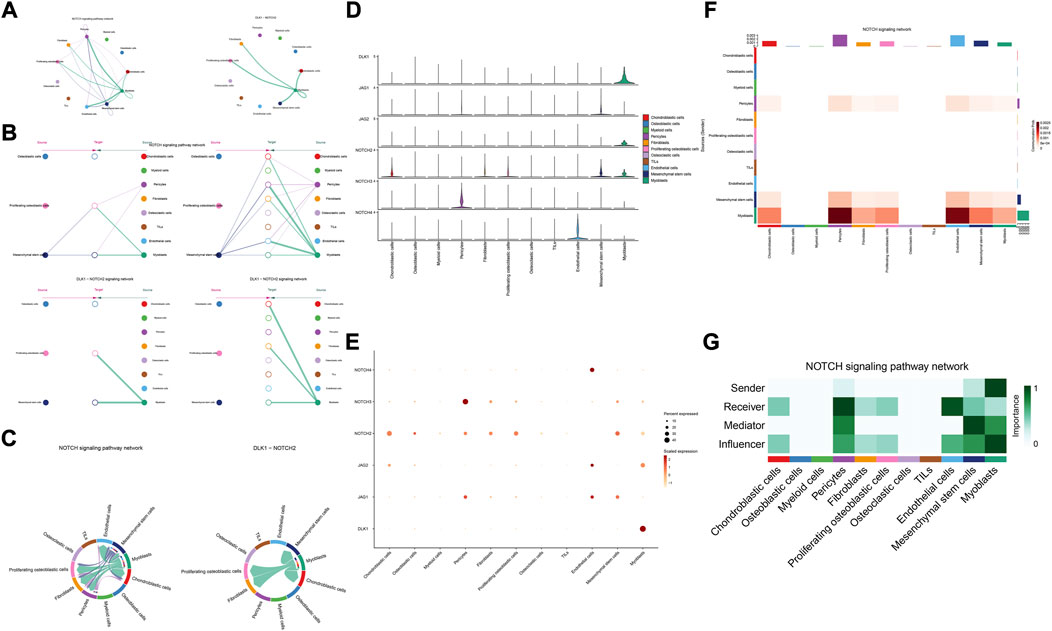
FIGURE 9. NOTCH signaling pathway. (A–C): ligand-receptor interactions of cells. (D,E): the ligand-receptors expressed by each major cell type. (F,G): the network of NOTCH signaling pathway.
Differentiation trajectory analysis of mesenchymal stem cells, proliferating osteoblastic cells, and osteoblastic cells
Cell state transmission was evaluated using pseudotime analysis based on the Monocle package. The mesenchymal stem cells, proliferating osteoblastic cells, and osteoblastic cells were subjected to differentiation trajectory analysis. We performed pseudotime analysis to explore the cell-state transitions among mesenchymal stem cells, proliferating osteoblastic cells, and osteoblastic cells (Figures 10A–E). Furthermore, we plotted the heatmap of the differentiation trajectory among these cells (Figure 10F). The results of trajectory analysis revealed that osteoblastic cells followed a differentiation trajectory that primarily began with clusters of mesenchymal stem cells and proliferating osteoblastic cells, from which they differentiated into osteoblastic cells.
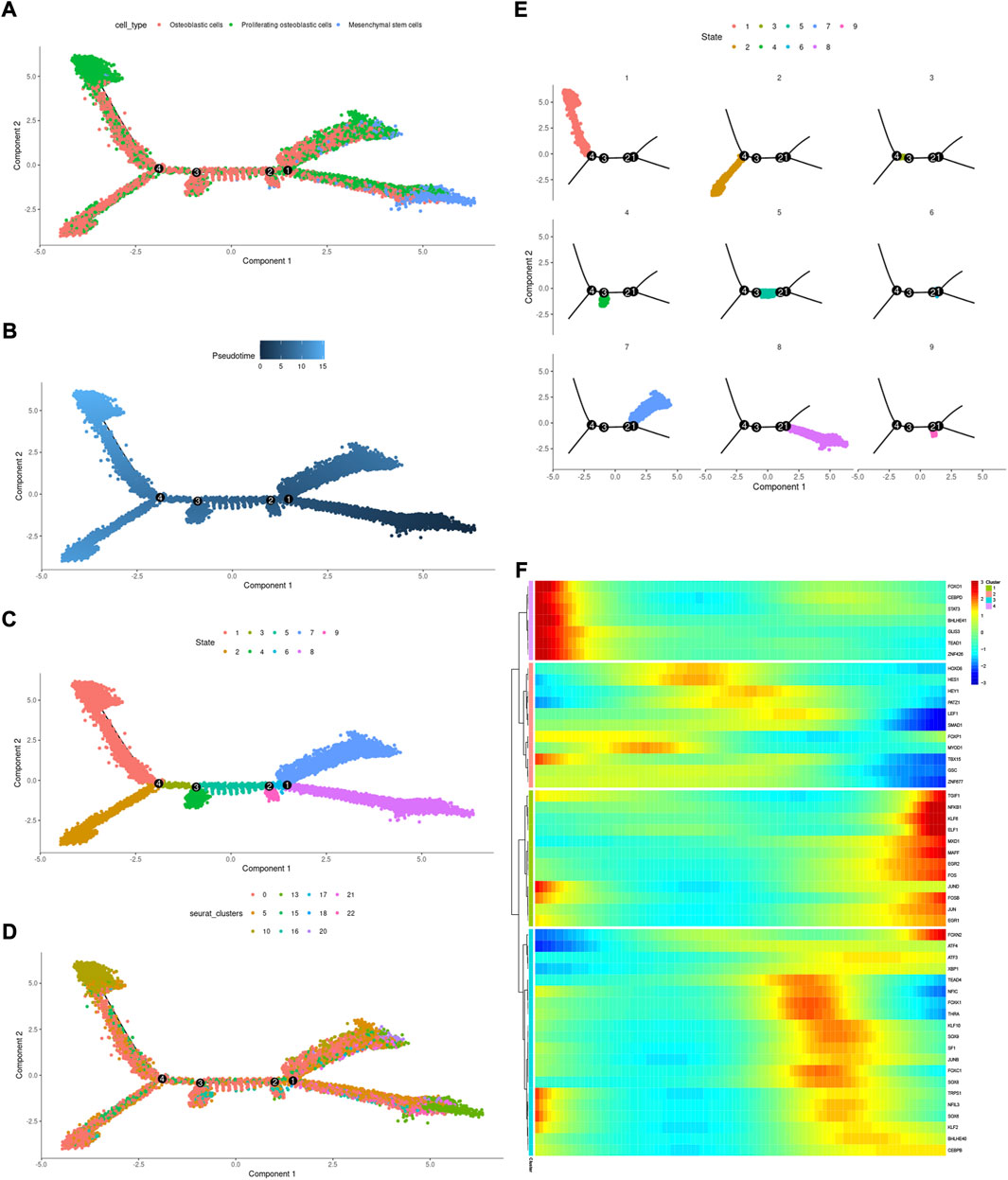
FIGURE 10. Trajectory analysis of mesenchymal stem cells, proliferating osteoblastic cells, and osteoblastic cells. (A–E): trajectory plots showing the differentiation of these cell types. (F): heatmap displaying the scaled expression of dynamic genes across time. The rows of the heatmap reflect genes exhibiting dynamic changes along the pseudotime, and these genes have been grouped into four categories based on their expression pattern over the pseudotime.
Single-cell regulatory network of mesenchymal stem cells, proliferating osteoblastic cells, and osteoblastic cells
A SCENIC analysis was conducted to detect the TFs of mesenchymal stem cells, proliferating osteoblastic cells, and osteoblastic cells. The genes of TFs (XBP1(Yang et al., 2015; Yu et al., 2022), ATF4 (Luo et al., 2017, 2019; Xian et al., 2017), and SOX9(Y. Chen S. et al., 2020; He et al., 2017; Wang et al., 2018)) were significantly activated in osteoblastic cells (Figures 11A–D), and were demonstrated to be expressed in osteosarcoma.
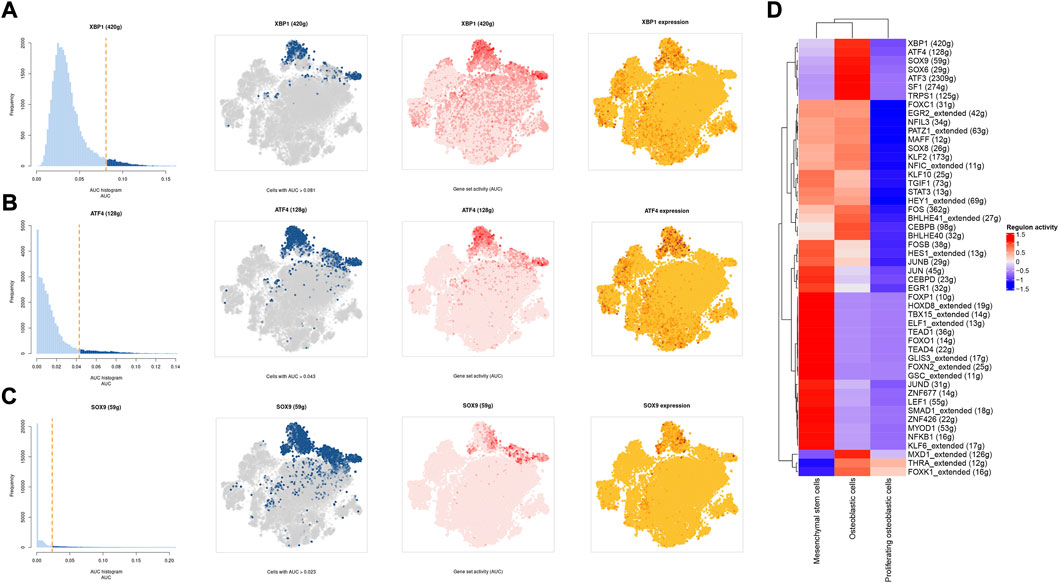
FIGURE 11. The SCENIC analysis predicted the TF. (A–C): tSNE plots and histograms show the top three TF activities in mesenchymal stem cells, proliferating osteoblastic cells, and osteoblastic cells. (D): Heatmap showing the top 50 TF in these cell types.
Discussion
Osteosarcoma is the most common malignant bone tumor in children, teens, and young adults with a median age of 16 years. It accounts for approximately 56% of bone sarcomas, and metastasis is the primary reason why treatment fails and the prognosis is poor (Chen et al., 2021). Despite previous molecular biology investigations having offered considerable information on the pathogenesis of osteosarcoma, the mechanisms that regulate its several oncogenic insults necessary for osteosarcoma start and development remain unknown (Isakoff et al., 2015; Kansara et al., 2014). It remains a serious concern due to poorly characterized carcinogenesis processes and restricted therapeutic options. So, it is essential to find important subpopulation driver mutations that promote diversity, expansion, invasion, and eventual colonization of other areas of the body. In addition, the potential cellular communication networks in osteosarcoma and the influence of tumor heterogeneity on cell aggregation are crucial.
Single-cell RNA sequencing (scRNA-seq) can show variation within cell populations. It could discriminate tumor cells from non-tumor cells and examine intercellular connections within the tumor microenvironment by analyzing transcripts inside individual cells. It is helpful to find unique cell types, look into tumor heterogeneity and potential networks of cell-to-cell communication, and show different developmental paths. This can give a theoretical foundation for future research into the molecular processes of OS growth and metastasis (Guo et al., 2022).
Mounting clinical and experimental data suggests that osteosarcoma stem cells, which originate from mesenchymal stem cells, may be the biological genesis of osteosarcomas and demonstrate osteoblastic differentiation, producing malignant osteoid (Brown et al., 2017; Xi et al., 2000). In addition, osteosarcoma is strongly connected with the osteoblastic lineage and displays osteogenic differentiation-related activities in proliferation, extracellular matrix secretion, and induction of ossification (Zeng et al., 2022). So, in this study, potential cellular communication networks among mesenchymal stem cells, proliferating osteoblastic cells, and osteoblastic cells were identified through comprehensive analysis of osteosarcoma single-cell RNA sequencing (scRNA-seq), illustrating the complex regulatory network in the advanced osteosarcoma microenvironment. Moreover, we performed transcription factor regulatory network analysis and trajectory analysis on these cells.
The results of cellular communication networks showed that mesenchymal stem cells, proliferating osteoblastic cells, and osteoblastic cells are mainly involved in SPP1, FGF, and NOTCH signaling pathways. The SPP1 gene (osteopontin, secreted phosphoprotein 1) encodes a protein with several activities, including bone remodeling, adhesion, tumor invasion, and metastasis (Dalla-Torre et al., 2006). It is generated by a variety of cell types, including osteoblasts, osteoclasts, and endothelial cells (Liu et al., 2013; Wang and Yang, 2015). SPP1 is now of interest in carcinogenesis, Lysosomal-associated membrane protein 3 (LAMP3) enhances osteosarcoma cell invasion via SPP1 signaling (Li et al., 2017). In colorectal cancer (CRC), SPP1 was highly upregulated and increased CRC metastasis by promoting epithelial-mesenchymal transition (EMT) (Xu et al., 2017). In addition, previous research found inhibition of the SPP1 gene may have therapeutic benefits for tongue cancer and may be a useful target for therapy (Zhang et al., 2020). Moreover, in pancreatic tumor microenvironment factors, the SPP1-CD44 axis can promote cancer stemness (Nallasamy et al., 2021). In head and neck squamous cell carcinoma (HNSCC), SPP1 overexpression is prognostic of worse survival results (Bie and Zhang, 2021). However, some scholars found that overexpression of SPP1 was correlated with improved overall survival, event-free survival, and relapse-free survival at diagnosis in osteosarcoma (Dalla-Torre et al., 2006). The results of our study revealed that through the SPP1-CD44 signaling pathway, myeloid cells, pericytes, and osteoclast cells can impact on mesenchymal stem cells and proliferating osteoblastic cells. Moreover, in these cellular communication networks, osteoclasts play a role as major senders, mediators, and influencers of the signal.
Fibroblast growth factor (FGF) signaling is essential for embryonic organ development and the progression of tumors (Brewer et al., 2016) and increases proliferation, invasion, and epithelial-to-mesenchymal transformation of tumor cells. (Bono et al., 2013). In the majority of malignancies, numerous FGFs are increased, and different FGF receptor (FGFR) subtypes are activated on tumor and stromal cells. (Turner and Grose, 2010). In addition, cancer, inflammation, and the resistance of tumor vascularization to VEGF inhibitor therapy have all been linked to FGFR signaling. (Beenken and Mohammadi, 2009; Casanovas et al., 2005; Fischer et al., 2007; Turner and Grose, 2010). Moreover, in the development of cancer, pathological FGF/FGFR signaling enhances cross-talk between oncogenic cells and its microenvironment, ultimately causing cancer cell proliferation, angiogenesis, and migration. (Li et al., 2018). For example, in the tumor microenvironment of esophageal cancer, NCAM- and FGF-2-mediated FGFR1 signaling modulates the survival and migration of tumor-associated macrophages and cancer cells (Takase et al., 2016). Additionally, FGFs activate myeloid cells, macrophages linked with tumors, cancer-related fibroblasts, and osteoclasts (Berardi et al., 1995; Collin-Osdoby et al., 2002; Itoh, 2007). Recent studies have found that in the evolution of osteosarcoma, FGF has emerged as a crucial regulator. According to previous research, LHX9 is critical for the proliferation, migration, invasion, and metastasis of OS cells via the FGF and TGF−/−catenin signaling pathways (Li et al., 2019). Some scholars have found that through the FRS2/TGF−/−catenin pathway, FGF-induced LHX9 controls osteosarcoma development and migration (Li et al., 2019).
Our research found, through the FGF7-FGFR1 signaling pathway, mesenchymal stem cells, pericytes, and myoblasts may influence mesenchymal stem cells, proliferating osteoblastic cells, and osteoblastic cells. High quantities of FGFR1 and FGF7 were detected in mesenchymal stem cells and pericytes. In addition, in these cellular communication networks, mesenchymal stem cells and pericytes serve as important signal senders, mediators, and influencers.
The Notch pathway regulates various mechanisms that control morphogenesis, lineage determination, apoptosis, and proliferation in some malignancies (Bray, 2006), and has been identified as both a tumor suppressor and an oncogene (Jin et al., 2017; Tanaka et al., 2009; Zhang et al., 2010). The Delta-Serrate-Lag (DSL) family of ligands (jagged 1/Jag1, Jag2, delta-like-1/DLL1, DLL3, and DLL4) on the surface of a cell connect with a membrane-bound Notch receptor (Notch1-4) on a different cell to start the Notch signaling pathway, a crucial step in normal bone growth that is also implicated as a critical mediator in a variety of different malignancies (Iso et al., 2003).
According to previous research, the notch pathway is strongly related to the development of osteosarcoma. Erk phosphorylation promotes osteosarcoma proliferation and migration in response to Notch stimulation (Qin et al., 2019). By activating cell division cycle 20, Notch-1 increases the evolution of osteosarcoma to a malignant state (Gao et al., 2020). The elevated expression of Jagged1 is intimately associated with osteosarcoma metastasis and recurrence. On the contrary, the knockdown of Jagged1 significantly reduced osteosarcoma cell proliferation, migration, and invasion (Zhang et al., 2021). Additionally, Notch signaling also regulates the immune system of the tumor microenvironment. Inhibiting the Notch signaling system enhances the polarization of TAM towards the M2 genotype, which in turn promotes the growth and spread of osteosarcoma (Ren et al., 2020). Our research found, through the DLK1- NOTCH2 signaling pathway, myoblasts may influence mesenchymal stem cells, proliferating osteoblastic cells. Additionally, myoblasts serve as important signal senders, mediators, and influencers.
One of the most prevalent issues in the development of human cancer is the dysregulation of transcription factors, which plays a role in the pathogenesis of the disease. The SCENIC analysis revealed that the regulon activity of XBP1, ATF4, and SOX9 were down-regulation in both mesenchymal stem cells and proliferating osteoblastic cells. X-box binding protein (XBP1) is a significant transcriptional regulator of the unfolded protein response. Lack of oxygen stimulated the transcription and translation of XBP1 mRNA, resulting in an increase in the activity of XBP1 protein (Romero-Ramirez et al., 2004). It was initially identified as a crucial regulator of major histocompatibility complex class II (MHC) gene expression in B cells (S. Chen Y. et al., 2020). High XBP1 levels were associated with advanced clinical stages, a high malignancy index, and a poor tumor necrosis rate in OS. XBP1 knockdown decreased OS cell growth and survival in culture (Yang et al., 2015). Recent studies have shown that XBP1 increases the susceptibility of HOS osteosarcoma cells to pyropheophorbide- α methyl ester-mediated photodynamic remedies (Yu et al., 2022). Activating transcription factor 4 (ATF4), a major regulator of the integrated stress response system, activates transcription of a group of transcriptional silencing genes that regulate cell survival and death (Ishizawa et al., 2016). In recent years, numerous investigations on the involvement of ATF4 in osteosarcoma have been reported. In human osteosarcoma, suppression of GRP78 increases ATF4-induced cell death via deubiquitination and stability of CHOP(Luo et al., 2017). Moreover, through endoplasmic reticulum (ER) stress-mediated PERK/eIF2/ATF4/CHOP activation and Wnt/β-catenin signal suppresses the development of human osteosarcoma (Zhao et al., 2020). ATF4 devastates RET by inhibiting nonclassical GRP78 to increase osteosarcoma chemosensitivity to bortezomib (Luo et al., 2019). Sex-determining region Y (SRY)- box 9 protein (SOX9) is a crucial transcription factor in a variety of illnesses, particularly in malignancies. Recent research has revealed that SOX9 plays an important function in the control of the tumor microenvironment (TME). Furthermore, SOX9 signaling or SOX9 controlled signaling pathways play an important role in cancer development and metastasis (Panda et al., 2021). Additionally, by means of a Sox9-Mediated positive feedback loop, MAFB contributes towards the progression of cancer stemness and tumorogenesis in osteosarcoma (Y. Chen S. et al., 2020). Moreover, previous study has found the cFOS-SOX9 axis of chondroblastic osteosarcoma reprograms bone marrow derived mesenchymal stem cells into chondroblastic cells (He et al., 2017).
In conclusion, this study uncovered the potential cellular communication networks between several cell types in advanced osteosarcoma. The SPP1, FGF, and NOTCH signaling pathways may play a crucial role in osteosarcoma TME regulation. This research may bring fresh insights into the pathophysiology of osteosarcoma’s molecular processes. However, this paper has the following limitations: no additional experiments were conducted to validate the data mining findings presented in this study; no further validation using the bulk RNA-seq database of osteosarcoma.
Data availability statement
The datasets presented in this study can be found in online repositories. The names of the repository/repositories and accession number(s) can be found in the article/Supplementary Material.
Ethics statement
Ethical review and approval was not required for the study on human participants in accordance with the local legislation and institutional requirements. Written informed consent for participation was not required for this study in accordance with the national legislation and the institutional requirements.
Author contributions
NX, XW, LW, YS, XZ, and HH conceived and designed the research. LW and YS downloaded and collected the data. NX, XW analyzed the data, XW and NX wrote the article. LW, YS, XZ, and HH conducted quality control on the articles and guided the submission. All authors read and approved the final manuscript.
Conflict of interest
The authors declare that the research was conducted in the absence of any commercial or financial relationships that could be construed as a potential conflict of interest.
Publisher’s note
All claims expressed in this article are solely those of the authors and do not necessarily represent those of their affiliated organizations, or those of the publisher, the editors and the reviewers. Any product that may be evaluated in this article, or claim that may be made by its manufacturer, is not guaranteed or endorsed by the publisher.
Supplementary material
The Supplementary Material for this article can be found online at: https://www.frontiersin.org/articles/10.3389/fgene.2022.1013737/full#supplementary-material
References
Aibar, S., Gonzalez-Blas, C. B., Moerman, T., Huynh-Thu, V. A., Imrichova, H., Hulselmans, G., et al. (2017). Scenic: Single-cell regulatory network inference and clustering. Nat. Methods 14 (11), 1083–1086. doi:10.1038/nmeth.4463
Armakolas, N., Armakolas, A., Antonopoulos, A., Dimakakos, A., Stathaki, M., and Koutsilieris, M. (2016). The role of the igf-1 ec in myoskeletal system and osteosarcoma pathophysiology. Crit. Rev. Oncol. Hematol. 108, 137–145. doi:10.1016/j.critrevonc.2016.11.004
Baumann, S., and Hennet, T. (2016). Collagen accumulation in osteosarcoma cells lacking glt25d1 collagen galactosyltransferase. J. Biol. Chem. 291 (35), 18514–18524. doi:10.1074/jbc.M116.723379
Beenken, A., and Mohammadi, M. (2009). The FGF family: Biology, pathophysiology and therapy.Research support. Rev. Drug Discov. 8 (3), 235–253. doi:10.1038/nrd2792
Berardi, A. C., Wang, A., Abraham, J., and Scadden, D. T. (1995). Basic fibroblast growth factor mediates its effects on committed myeloid progenitors by direct action and has no effect on hematopoietic stem cells. Blood 86 (6), 2123–2129. doi:10.1182/blood.v86.6.2123.bloodjournal8662123
Bie, T., and Zhang, X. (2021). Higher expression of spp1 predicts poorer survival outcomes in head and neck cancer. J. Immunol. Res. 2021, 8569575. doi:10.1155/2021/8569575
Bono, F., De Smet, F., Herbert, C., De Bock, K., Georgiadou, M., Fons, P., et al. (2013). Inhibition of tumor angiogenesis and growth by a small-molecule multi-fgf receptor blocker with allosteric properties. Cancer Cell 23 (4), 477–488. doi:10.1016/j.ccr.2013.02.019
Bousquet, M., Noirot, C., Accadbled, F., Sales, D. G. J., Castex, M. P., Brousset, P., et al. (2016). Whole-exome sequencing in osteosarcoma reveals important heterogeneity of genetic alterations. Ann. Oncol. 27 (4), 738–744. doi:10.1093/annonc/mdw009
Bray, S. J. (2006). Notch signalling: A simple pathway becomes complex. Nat. Rev. Mol. Cell Biol. 7 (9), 678–689. doi:10.1038/nrm2009
Brewer, J. R., Mazot, P., Soriano, P., and Extramural, N. I. H., (2016). Genetic insights into the mechanisms of Fgf signaling. Genes Dev. 30 (7), 751–771. doi:10.1101/gad.277137.115
Brown, H. K., Tellez-Gabriel, M., Heymann, D., and Gov't], Non-U. S. (2017). Review; Research Support, 386, 189–195. doi:10.1016/j.canlet.2016.11.019Cancer stem cells in osteosarcomaJ. Artic. Lett.
Casanovas, O., Hicklin, D. J., Bergers, G., and Hanahan, D. (2005). Drug resistance by evasion of antiangiogenic targeting of vegf signaling in late-stage pancreatic islet tumors. Cancer Cell 8 (4), 299–309. doi:10.1016/j.ccr.2005.09.005
Chen, C., Xie, L., Ren, T., Huang, Y., Xu, J., and Guo, W. (2021). Immunotherapy for osteosarcoma: Fundamental mechanism, rationale, and recent breakthroughs. Cancer Lett. 500, 1–10. doi:10.1016/j.canlet.2020.12.024
Chen, S., Chen, J., Hua, X., Sun, Y., Cui, R., Sha, J., et al. (2020a). The emerging role of xbp1 in cancer. Biomed. Pharmacother. 127, 110069. doi:10.1016/j.biopha.2020.110069
Chen, Y. C., Chang, C. N., Hsu, H. C., Chiou, S. J., Lee, L. T., and Hseu, T. H. (2009). Sennoside b inhibits pdgf receptor signaling and cell proliferation induced by pdgf-bb in human osteosarcoma cells. Life Sci. 84 (25-26), 915–922. doi:10.1016/j.lfs.2009.04.003
Chen, Y., Wang, T., Huang, M., Liu, Q., Hu, C., Wang, B., et al. (2020b). Mafb promotes cancer stemness and tumorigenesis in osteosarcoma through a sox9-mediated positive feedback loop. Cancer Res. 80 (12), 2472–2483. doi:10.1158/0008-5472.CAN-19-1764
Cheng, G., Liu, C., Sun, X., Zhang, L., Liu, L., Ouyang, J., et al. (2015). Visfatin promotes osteosarcoma cell migration and invasion via induction of epithelial-mesenchymal transition. Oncol. Rep. 34 (2), 987–994. doi:10.3892/or.2015.4053
Collin-Osdoby, P., Rothe, L., Bekker, S., Anderson, F., Huang, Y., and Osdoby, P. (2002). Basic fibroblast growth factor stimulates osteoclast recruitment, development, and bone pit resorption in association with angiogenesis in vivo on the chick chorioallantoic membrane and activates isolated avian osteoclast resorption in vitro. J. Bone Min. Res. 17 (10), 1859–1871. doi:10.1359/jbmr.2002.17.10.1859
Dai, J., Xu, L. J., Han, G. D., Jiang, H. T., Sun, H. L., Zhu, G. T., et al. (2018). Down-regulation of long non-coding RNA ITGB2-AS1 inhibits osteosarcoma proliferation and metastasis by repressing Wnt/β-catenin signalling and predicts favourable prognosis. Artif. Cells Nanomed. Biotechnol. 46, S783–S790. doi:10.1080/21691401.2018.1511576
Dalla-Torre, C. A., Yoshimoto, M., Lee, C. H., Joshua, A. M., de Toledo, S. R., Petrilli, A. S., et al. (2006). Effects of thbs3, sparc and spp1 expression on biological behavior and survival in patients with osteosarcoma. BMC Cancer 6, 237. doi:10.1186/1471-2407-6-237
Egners, A., Rezaei, M., Kuzmanov, A., Poitz, D. M., Streichert, D., Muller-Reichert, T., et al. (2018). Phd3 acts as tumor suppressor in mouse osteosarcoma and influences tumor vascularization via pdgf-c signaling. Cancers (Basel) 10 (12), E496. doi:10.3390/cancers10120496
Elenjord, R., Allen, J. B., Johansen, H. T., Kildalsen, H., Svineng, G., Maelandsmo, G. M., et al. (2009). Collagen i regulates matrix metalloproteinase-2 activation in osteosarcoma cells independent of s100a4. FEBS J. 276 (18), 5275–5286. doi:10.1111/j.1742-4658.2009.07223.x
Fischer, C., Jonckx, B., Mazzone, M., Zacchigna, S., Loges, S., Pattarini, L., et al. (2007). Anti-plgf inhibits growth of vegf(r)-inhibitor-resistant tumors without affecting healthy vessels. Cell 131 (3), 463–475. doi:10.1016/j.cell.2007.08.038
Gao, Y., Bai, L., and Shang, G. (2020). Notch-1 promotes the malignant progression of osteosarcoma through the activation of cell division cycle 20. Aging (Albany NY) 13 (2), 2668–2680. doi:10.18632/aging.202314
Giatagana, E. M., Berdiaki, A., Gaardlos, M., Samsonov, S. A., Tzanakakis, G. N., and Nikitovic, D. (2022). Biglycan interacts with type i insulin-like receptor (igf-ir) signaling pathway to regulate osteosarcoma cell growth and response to chemotherapy. Cancers (Basel) 14 (5), 1196. doi:10.3390/cancers14051196
Gomez-Brouchet, A., Mourcin, F., Gourraud, P. A., Bouvier, C., De Pinieux, G., Le Guelec, S., et al. (2010). Galectin-1 is a powerful marker to distinguish chondroblastic osteosarcoma and conventional chondrosarcoma. Hum. Pathol. 41 (9), 1220–1230. doi:10.1016/j.humpath.2009.10.028
Guo, J., Tang, H., Huang, P., Guo, J., Shi, Y., Yuan, C., et al. (2022). Single-cell profiling of tumor microenvironment heterogeneity in osteosarcoma identifies a highly invasive subcluster for predicting prognosis. Front. Oncol. 12, 732862. doi:10.3389/fonc.2022.732862
Gvozdenovic, A., Boro, A., Born, W., Muff, R., and Fuchs, B. (2017). A bispecific antibody targeting igf-ir and egfr has tumor and metastasis suppressive activity in an orthotopic xenograft osteosarcoma mouse model. Am. J. Cancer Res. 7 (7), 1435–1449.
He, M., Shen, P., Qiu, C., and Wang, J. (2019). Mir-627-3p inhibits osteosarcoma cell proliferation and metastasis by targeting ptn. Aging (Albany NY) 11 (15), 5744–5756. doi:10.18632/aging.102157
He, Y., Zhu, W., Shin, M. H., Gary, J., Liu, C., Dubois, W., et al. (2017). Cfos-sox9 axis reprograms bone marrow-derived mesenchymal stem cells into chondroblastic osteosarcoma. Stem Cell Rep. 8 (6), 1630–1644. doi:10.1016/j.stemcr.2017.04.029
Heino, J., and Massague, J. (1989). Transforming growth factor-beta switches the pattern of integrins expressed in mg-63 human osteosarcoma cells and causes a selective loss of cell adhesion to laminin. J. Biol. Chem. 264 (36), 21806–21811. doi:10.1016/s0021-9258(20)88255-6
Heldin, C. H., Johnsson, A., Wennergren, S., Wernstedt, C., Betsholtz, C., and Westermark, B. (1986). A human osteosarcoma cell line secretes a growth factor structurally related to a homodimer of pdgf a-chains. Nature 319 (6053), 511–514. doi:10.1038/319511a0
Ho, X. D., Nguyen, H. G., Trinh, L. H., Reimann, E., Prans, E., Koks, G., et al. (2017). Analysis of the expression of repetitive dna elements in osteosarcoma. Front. Genet. 8, 193. doi:10.3389/fgene.2017.00193
Isakoff, M. S., Bielack, S. S., Meltzer, P., and Gorlick, R. (2015). Osteosarcoma: Current treatment and a collaborative pathway to success. J. Clin. Oncol. 33 (27), 3029–3035. doi:10.1200/JCO.2014.59.4895
Ishizawa, J., Kojima, K., Chachad, D., Ruvolo, P., Ruvolo, V., Jacamo, R. O., et al. (2016). Atf4 induction through an atypical integrated stress response to onc201 triggers p53-independent apoptosis in hematological malignancies. Sci. Signal. 9 (415), a17. doi:10.1126/scisignal.aac4380
Iso, T., Kedes, L., and Hamamori, Y. (2003). Hes and herp families: Multiple effectors of the notch signaling pathway. J. Cell. Physiol. 194 (3), 237–255. doi:10.1002/jcp.10208
Itoh, N. (2007). The fgf families in humans, mice, and zebrafish: Their evolutional processes and roles in development, metabolism, and disease. Biol. Pharm. Bull. 30 (10), 1819–1825. doi:10.1248/bpb.30.1819
Ji, X., Shan, L., Shen, P., and He, M. (2020). Circular rna circ_001621 promotes osteosarcoma cells proliferation and migration by sponging mir-578 and regulating vegf expression. Cell Death Dis. 11 (1), 18. doi:10.1038/s41419-019-2204-y
Jin, H., Luo, S., Wang, Y., Liu, C., Piao, Z., Xu, M., et al. (2017). miR-135b stimulates osteosarcoma recurrence and lung metastasis via notch and wnt/β-catenin signaling. Mol. Ther. Nucleic Acids 8, 111–122. doi:10.1016/j.omtn.2017.06.008
Jin, S., Guerrero-Juarez, C. F., Zhang, L., Chang, I., Ramos, R., Kuan, C. H., et al. (2021). Inference and analysis of cell-cell communication using cellchat. Nat. Commun. 12 (1), 1088. doi:10.1038/s41467-021-21246-9
Kansara, M., Teng, M. W., Smyth, M. J., and Thomas, D. M. (2014). Translational biology of osteosarcoma. Nat. Rev. Cancer 14 (11), 722–735. doi:10.1038/nrc3838
Kurogi, T., Nabeshima, K., Kataoka, H., Okada, Y., and Koono, M. (1996). Stimulation of gelatinase b and tissue inhibitors of metalloproteinase (timp) production in co-culture of human osteosarcoma cells and human fibroblasts: Gelatinase b production was stimulated via up-regulation of fibroblast growth factor (fgf) receptor. Int. J. Cancer 66 (1), 82–90. doi:10.1002/(SICI)1097-0215(19960328)66:1<82::AID-IJC15>3.0.CO;2-D
Laulederkind, S. J., Kirtikara, K., Raghow, R., and Ballou, L. R. (2000). The regulation of pge(2) biosynthesis in mg-63 osteosarcoma cells by il-1 and fgf is cell density-dependent. Exp. Cell Res. 258 (2), 409–416. doi:10.1006/excr.2000.4961
Lei, Z., Duan, H., Zhao, T., Zhang, Y., Li, G., Meng, J., et al. (2018). Park2 inhibits osteosarcoma cell growth through the jak2/stat3/vegf signaling pathway. Cell Death Dis. 9 (3), 375. doi:10.1038/s41419-018-0401-8
Levinson, H., Hopper, J. E., and Ehrlich, H. P. (2002). Overexpression of integrin alphav promotes human osteosarcoma cell populated collagen lattice contraction and cell migration. J. Cell. Physiol. 193 (2), 219–224. doi:10.1002/jcp.10164
Li, Q., Alsaidan, O. A., Ma, Y., Kim, S., Liu, J., Albers, T., et al. (2018). Pharmacologically targeting the myristoylation of the scaffold protein FRS2α inhibits FGF/FGFR-mediated oncogenic signaling and tumor progression. J. Biol. Chem. 293 (17), 6434–6448. doi:10.1074/jbc.RA117.000940
Li, S. Q., Tu, C., Wan, L., Chen, R. Q., Duan, Z. X., Ren, X. L., et al. (2019). FGF-induced LHX9 regulates the progression and metastasis of osteosarcoma via FRS2/TGF-β/β-catenin pathway. Cell Div. 14, 13. doi:10.1186/s13008-019-0056-6
Li, Y., Du, W., Han, J., and Ge, J. (2017). Lamp3 promotes the invasion of osteosarcoma cells via spp1 signaling. Mol. Med. Rep. 16 (5), 5947–5953. doi:10.3892/mmr.2017.7349
Liberzon, A., Birger, C., Thorvaldsdottir, H., Ghandi, M., Mesirov, J. P., and Tamayo, P. (2015). The molecular signatures database (msigdb) hallmark gene set collection. Cell Syst. 1 (6), 417–425. doi:10.1016/j.cels.2015.12.004
Liu, J., Qu, S., Zhang, T., Gao, Y., Shi, H., Song, K., et al. (2021). Applications of single-cell omics in tumor immunology. Front. Immunol. 12, 697412. doi:10.3389/fimmu.2021.697412
Liu, L., Xu, Y., and Reiter, R. J. (2013). Melatonin inhibits the proliferation of human osteosarcoma cell line mg-63. Bone 55 (2), 432–438. doi:10.1016/j.bone.2013.02.021
Liu, Y., Zhao, L., Ju, Y., Li, W., Zhang, M., Jiao, Y., et al. (2014). A novel androstenedione derivative induces ros-mediated autophagy and attenuates drug resistance in osteosarcoma by inhibiting macrophage migration inhibitory factor (mif). Cell Death Dis. 5, e1361. doi:10.1038/cddis.2014.300
Luo, J., Xia, Y., Luo, J., Li, J., Zhang, C., Zhang, H., et al. (2017). Grp78 inhibition enhances atf4-induced cell death by the deubiquitination and stabilization of chop in human osteosarcoma. Cancer Lett. 410, 112–123. doi:10.1016/j.canlet.2017.09.021
Luo, J., Xia, Y., Yin, Y., Luo, J., Liu, M., Zhang, H., et al. (2019). Atf4 destabilizes ret through nonclassical grp78 inhibition to enhance chemosensitivity to bortezomib in human osteosarcoma. Theranostics 9 (21), 6334–6353. doi:10.7150/thno.36818
Ma, K., Zhang, C., and Li, W. (2020). Gamabufotalin suppressed osteosarcoma stem cells through the TGF-β/periostin/PI3K/AKT pathway. Chem. Biol. Interact. 331, 109275. doi:10.1016/j.cbi.2020.109275
Manara, M. C., Bernard, G., Lollini, P. L., Nanni, P., Zuntini, M., Landuzzi, L., et al. (2006). Cd99 acts as an oncosuppressor in osteosarcoma. Mol. Biol. Cell 17 (4), 1910–1921. doi:10.1091/mbc.e05-10-0971
Meltzer, P. S., and Helman, L. J. (2021). New horizons in the treatment of osteosarcoma. N. Engl. J. Med. 385 (22), 2066–2076. doi:10.1056/NEJMra2103423
Miao, J. H., Wang, S. Q., Zhang, M. H., Yu, F. B., Zhang, L., Yu, Z. X., et al. (2014). Knockdown of galectin-1 suppresses the growth and invasion of osteosarcoma cells through inhibition of the mapk/erk pathway. Oncol. Rep. 32 (4), 1497–1504. doi:10.3892/or.2014.3358
Molina, E. R., Chim, L. K., Salazar, M. C., Mehta, S. M., Menegaz, B. A., Lamhamedi-Cherradi, S. E., et al. (2019). Mechanically tunable coaxial electrospun models of yap/taz mechanoresponse and igf-1r activation in osteosarcoma. Acta Biomater. 100, 38–51. doi:10.1016/j.actbio.2019.09.029
Mu, X., Isaac, C., Greco, N., Huard, J., and Weiss, K. (2013). Notch signaling is associated with aldh activity and an aggressive metastatic phenotype in murine osteosarcoma cells. Front. Oncol. 3, 143. doi:10.3389/fonc.2013.00143
Nallasamy, P., Nimmakayala, R. K., Karmakar, S., Leon, F., Seshacharyulu, P., Lakshmanan, I., et al. (2021). Pancreatic tumor microenvironment factor promotes cancer stemness via spp1-cd44 axis. Gastroenterology 161 (6), 1998–2013.e7. doi:10.1053/j.gastro.2021.08.023
Oda, Y., Yamamoto, H., Tamiya, S., Matsuda, S., Tanaka, K., Yokoyama, R., et al. (2006). Cxcr4 and vegf expression in the primary site and the metastatic site of human osteosarcoma: Analysis within a group of patients, all of whom developed lung metastasis. Mod. Pathol. 19 (5), 738–745. doi:10.1038/modpathol.3800587
Ongaro, A., Pellati, A., Bagheri, L., Rizzo, P., Caliceti, C., Massari, L., et al. (2016). Characterization of notch signaling during osteogenic differentiation in human osteosarcoma cell line mg63. J. Cell. Physiol. 231 (12), 2652–2663. doi:10.1002/jcp.25366
Panda, M., Tripathi, S. K., and Biswal, B. K. (2021). Sox9: An emerging driving factor from cancer progression to drug resistance. Biochim. Biophys. Acta. Rev. Cancer 1875 (2), 188517. doi:10.1016/j.bbcan.2021.188517
Park, G. B., Kim, D. J., Kim, Y. S., Lee, H. K., Kim, C. W., and Hur, D. Y. (2015). Silencing of galectin-3 represses osteosarcoma cell migration and invasion through inhibition of fak/src/lyn activation and beta-catenin expression and increases susceptibility to chemotherapeutic agents. Int. J. Oncol. 46 (1), 185–194. doi:10.3892/ijo.2014.2721
Qin, J., Wang, R., Zhao, C., Wen, J., Dong, H., Wang, S., et al. (2019). Notch signaling regulates osteosarcoma proliferation and migration through erk phosphorylation. Tissue Cell 59, 51–61. doi:10.1016/j.tice.2019.07.002
Qin, T., Zhu, W., Kan, X., Li, L., and Wu, D. (2022). Luteolin attenuates the chemoresistance of osteosarcoma through inhibiting the PTN/β-catenin/MDR1 signaling axis by upregulating miR-384. J. Bone Oncol. 34, 100429. doi:10.1016/j.jbo.2022.100429
Ren, S., Zhang, X., Hu, Y., Wu, J., Ju, Y., Sun, X., et al. (2020). Blocking the notch signal transduction pathway promotes tumor growth in osteosarcoma by affecting polarization of tam to m2 phenotype. Ann. Transl. Med. 8 (17), 1057. doi:10.21037/atm-20-3881
Ren, X., Kang, B., and Zhang, Z. (2018). Understanding tumor ecosystems by single-cell sequencing: Promises and limitations. Genome Biol. 19 (1), 211. doi:10.1186/s13059-018-1593-z
Romero-Ramirez, L., Cao, H., Nelson, D., Hammond, E., Lee, A. H., Yoshida, H., et al. (2004). Xbp1 is essential for survival under hypoxic conditions and is required for tumor growth. Cancer Res. 64 (17), 5943–5947. doi:10.1158/0008-5472.CAN-04-1606
Rothzerg, E., Ho, X. D., Xu, J., Wood, D., Martson, A., and Koks, S. (2021). Upregulation of 15 antisense long non-coding rnas in osteosarcoma. Genes (Basel) 12 (8), 1132. doi:10.3390/genes12081132
Saba, K. H., Cornmark, L., Rissler, M., Fioretos, T., Astrom, K., Haglund, F., et al. (2019). Genetic profiling of a chondroblastoma-like osteosarcoma/malignant phosphaturic mesenchymal tumor of bone reveals a homozygous deletion of cdkn2a, intragenic deletion of dmd, and a targetable fn1-fgfr1 gene fusion. Genes Chromosom. Cancer 58 (10), 731–736. doi:10.1002/gcc.22764
Sciandra, M., Marino, M. T., Manara, M. C., Guerzoni, C., Grano, M., Oranger, A., et al. (2014). Cd99 drives terminal differentiation of osteosarcoma cells by acting as a spatial regulator of erk 1/2. J. Bone Min. Res. 29 (5), 1295–1309. doi:10.1002/jbmr.2141
Shao, H., Ge, M., Zhang, J., Zhao, T., and Zhang, S. (2022). Osteoclasts differential-related prognostic biomarker for osteosarcoma based on single cell, bulk cell and gene expression datasets. BMC Cancer 22 (1), 288. doi:10.1186/s12885-022-09380-z
Sun, X., Tian, C., Zhang, H., Han, K., Zhou, M., Gan, Z., et al. (2020). Long noncoding rna oip5-as1 mediates resistance to doxorubicin by regulating mir-137-3p/ptn axis in osteosarcoma. Biomed. Pharmacother. 128, 110201. doi:10.1016/j.biopha.2020.110201
Takase, N., Koma, Y., Urakawa, N., Nishio, M., Arai, N., Akiyama, H., et al. (2016). Ncam- and fgf-2-mediated fgfr1 signaling in the tumor microenvironment of esophageal cancer regulates the survival and migration of tumor-associated macrophages and cancer cells. Cancer Lett. 380 (1), 47–58. doi:10.1016/j.canlet.2016.06.009
Tan, X., Fan, S., Wu, W., and Zhang, Y. (2015). Microrna-26a inhibits osteosarcoma cell proliferation by targeting igf-1. Bone Res. 3, 15033. doi:10.1038/boneres.2015.33
Tanaka, M., Setoguchi, T., Hirotsu, M., Gao, H., Sasaki, H., Matsunoshita, Y., et al. (2009). Inhibition of notch pathway prevents osteosarcoma growth by cell cycle regulation. Br. J. Cancer 100 (12), 1957–1965. doi:10.1038/sj.bjc.6605060
Tsai, H. C., Tzeng, H. E., Huang, C. Y., Huang, Y. L., Tsai, C. H., Wang, S. W., et al. (2017). Wisp-1 positively regulates angiogenesis by controlling vegf-a expression in human osteosarcoma. Cell Death Dis. 8 (4), e2750. doi:10.1038/cddis.2016.421
Turner, N., and Grose, R. (2010). Fibroblast growth factor signalling: From development to cancer. Nat. Rev. Cancer 10 (2), 116–129. doi:10.1038/nrc2780
Vegliante, R., Pastushenko, I., and Blanpain, C. (2022). Deciphering functional tumor states at single-cell resolution. EMBO J. 41 (2), e109221. doi:10.15252/embj.2021109221
Wang, D., Qian, G., Wang, J., Wang, T., Zhang, L., Yang, P., et al. (2019). Visfatin is involved in the cisplatin resistance of osteosarcoma cells via upregulation of snail and zeb1. Cancer Biol. Ther. 20 (7), 999–1006. doi:10.1080/15384047.2019.1591675
Wang, G. J., Shen, N. J., Cheng, L., Yehan, F., Huang, H., and Li, K. H. (2016). Visfatin triggers the in vitro migration of osteosarcoma cells via activation of NF-κB/IL-6 signals. Eur. J. Pharmacol. 791, 322–330. doi:10.1016/j.ejphar.2016.08.029
Wang, Q., Liu, W., Fan, J., Guo, J., Shen, F., Ma, Z., et al. (2020). Von willebrand factor promotes platelet-induced metastasis of osteosarcoma through activation of the vwf-gpib axis. J. Bone Oncol. 25, 100325. doi:10.1016/j.jbo.2020.100325
Wang, W. T., Qi, Q., Zhao, P., Li, C. Y., Yin, X. Y., and Yan, R. B. (2018). Mir-590-3p is a novel microrna which suppresses osteosarcoma progression by targeting sox9. Biomed. Pharmacother. 107, 1763–1769. doi:10.1016/j.biopha.2018.06.124
Wang, Y. P., and Yang, Z. P. (2015). Effects of melatonin combined with cis-platinum or methotrexate on the proliferation of osteosarcoma cell line saos-2. Zhongguo Yi Xue Ke Xue Yuan Xue Bao. 37 (2), 215–220. doi:10.3881/j.issn.1000-503X.2015.02.013
Xi, S. C., Tam, P. C., Brown, G. M., Pang, S. F., and Shiu, S. Y. (2000). Potential involvement of mt1 receptor and attenuated sex steroid-induced calcium influx in the direct anti-proliferative action of melatonin on androgen-responsive lncap human prostate cancer cells. J. Pineal Res. 29 (3), 172–183. doi:10.1034/j.1600-079x.2000.d01-64.x
Xian, M., Cao, H., Cao, J., Shao, X., Zhu, D., Zhang, N., et al. (2017). Bortezomib sensitizes human osteosarcoma cells to adriamycin-induced apoptosis through ROS-dependent activation of p-eIF2α/ATF4/CHOP axis. Int. J. Cancer 141 (5), 1029–1041. doi:10.1002/ijc.30792
Xu, C. J., Song, J. F., Su, Y. X., and Liu, X. L. (2010). Expression of b-fgf and endostatin and their clinical significance in human osteosarcoma. Orthop. Surg. 2 (4), 291–298. doi:10.1111/j.1757-7861.2010.00102.x
Xu, C., Sun, L., Jiang, C., Zhou, H., Gu, L., Liu, Y., et al. (2017). Spp1, analyzed by bioinformatics methods, promotes the metastasis in colorectal cancer by activating emt pathway. 91, 1167–1177. doi:10.1016/j.biopha.2017.05.056
Xu, C., Wang, Z., Zhang, L., Feng, Y., Lv, J., Wu, Z., et al. (2022). Periostin promotes the proliferation and metastasis of osteosarcoma by increasing cell survival and activates the pi3k/akt pathway. Cancer Cell Int. 22 (1), 34. doi:10.1186/s12935-021-02441-6
Yamaguchi, K., Matsuo, N., Sumiyoshi, H., Fujimoto, N., Iyama, K. I., Yanagisawa, S., et al. (2005). Pro-alpha3(v) collagen chain is expressed in bone and its basic n-terminal peptide adheres to osteosarcoma cells. Matrix Biol. 24 (4), 283–294. doi:10.1016/j.matbio.2005.03.006
Yang, J., Cheng, D., Zhou, S., Zhu, B., Hu, T., and Yang, Q. (2015). Overexpression of x-box binding protein 1 (xbp1) correlates to poor prognosis and up-regulation of pi3k/mtor in human osteosarcoma. Int. J. Mol. Sci. 16 (12), 28635–28646. doi:10.3390/ijms161226123
Yu, H., Zhang, Y., Zuo, Q., Zhong, S., Chen, Y., Zhang, M., et al. (2022). Targeting x box-binding protein-1 (xbp1) enhances the sensitivity of hos osteosarcoma cells to pyropheophorbide- alpha methyl ester-mediated photodynamic therapy. Photodiagnosis Photodyn. Ther. 37, 102646. doi:10.1016/j.pdpdt.2021.102646
Zeng, Z., Li, W., Zhang, D., Zhang, C., Jiang, X., Guo, R., et al. (2022). Development of a chemoresistant risk scoring model for prechemotherapy osteosarcoma using single-cell sequencing. Front. Oncol. 12, 893282. doi:10.3389/fonc.2022.893282
Zhang, J., Li, N., Lu, S., Chen, Y., Shan, L., Zhao, X., et al. (2021). The role of notch ligand jagged1 in osteosarcoma proliferation, metastasis, and recurrence. J. Orthop. Surg. Res. 16 (1), 226. doi:10.1186/s13018-021-02372-y
Zhang, P., Yang, Y., Nolo, R., Zweidler-McKay, P. A., and Hughes, D. P. (2010). Regulation of notch signaling by reciprocal inhibition of hes1 and deltex 1 and its role in osteosarcoma invasiveness. Oncogene 29 (20), 2916–2926. doi:10.1038/onc.2010.62
Zhang, Q., Li, L., Lai, Y., and Zhao, T. (2020). Silencing of spp1 suppresses progression of tongue cancer by mediating the pi3k/akt signaling pathway. Technol. Cancer Res. Treat. 19, 1533033820971306. doi:10.1177/1533033820971306
Zhang, Y., Cheng, H., Li, W., Wu, H., and Yang, Y. (2019). Highly-expressed P2X7 receptor promotes growth and metastasis of human HOS/MNNG osteosarcoma cells via PI3K/Akt/GSK3β/β-catenin and mTOR/HIF1α/VEGF signaling. Int. J. Cancer 145 (4), 1068–1082. doi:10.1002/ijc.32207
Zhao, A., Zhang, Z., Zhou, Y., Li, X., Li, X., Ma, B., et al. (2020). β-Elemonic acid inhibits the growth of human Osteosarcoma through endoplasmic reticulum (ER) stress-mediated PERK/eIF2α/ATF4/CHOP activation and Wnt/β-catenin signal suppression. Phytomedicine 69, 153183. doi:10.1016/j.phymed.2020.153183
Zhou, X., Jing, J., Peng, J., Mao, W., Zheng, Y., Wang, D., et al. (2014). Expression and clinical significance of galectin-3 in osteosarcoma. Gene 546 (2), 403–407. doi:10.1016/j.gene.2014.04.066
Zhou, Y., Yang, D., Yang, Q., Lv, X., Huang, W., Zhou, Z., et al. (2020). Single-cell rna landscape of intratumoral heterogeneity and immunosuppressive microenvironment in advanced osteosarcoma. Nat. Commun. 11 (1), 6322. doi:10.1038/s41467-020-20059-6
Zhou, Y., Yin, L., Li, H., Liu, L. H., and Xiao, T. (2019). The lncrna linc00963 facilitates osteosarcoma proliferation and invasion by suppressing mir-204-3p/fn1 axis. Cancer Biol. Ther. 20 (8), 1141–1148. doi:10.1080/15384047.2019.1598766
Keywords: osteosarcoma, cell types, cellular communication networks, regulon activity, ScRNA-seq
Citation: Xu N, Wang X, Wang L, Song Y, Zheng X and Hu H (2022) Comprehensive analysis of potential cellular communication networks in advanced osteosarcoma using single-cell RNA sequencing data. Front. Genet. 13:1013737. doi: 10.3389/fgene.2022.1013737
Received: 07 August 2022; Accepted: 26 September 2022;
Published: 11 October 2022.
Edited by:
Qian Wang, Tai’an City Central Hospital, ChinaReviewed by:
Yiheng Ye, First Affiliated Hospital of Wenzhou Medical University, ChinaQiancheng Luo, Shanghai Pudong New Area Gongli Hospital, China
Xingju Zheng, Guizhou Provincial People’s Hospital, China
Copyright © 2022 Xu, Wang, Wang, Song, Zheng and Hu. This is an open-access article distributed under the terms of the Creative Commons Attribution License (CC BY). The use, distribution or reproduction in other forums is permitted, provided the original author(s) and the copyright owner(s) are credited and that the original publication in this journal is cited, in accordance with accepted academic practice. No use, distribution or reproduction is permitted which does not comply with these terms.
*Correspondence: Yuan Song, sypw@qq.com; Xianyou Zheng, zhengxianyou@126.com; Hai Hu, xmhuhai@hotmail.com
†These authors have contributed equally to this work