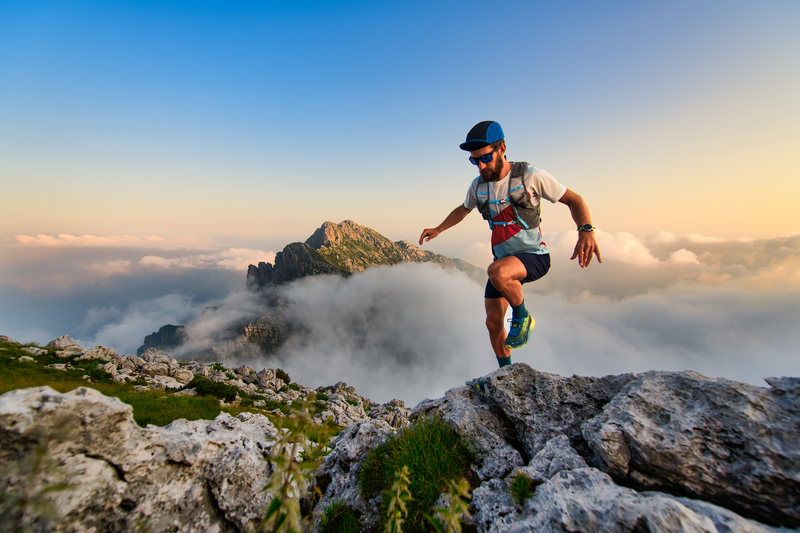
95% of researchers rate our articles as excellent or good
Learn more about the work of our research integrity team to safeguard the quality of each article we publish.
Find out more
ORIGINAL RESEARCH article
Front. Genet. , 11 November 2022
Sec. Genomics of Plants and the Phytoecosystem
Volume 13 - 2022 | https://doi.org/10.3389/fgene.2022.1004211
This article is part of the Research Topic Accelerating Genetic Gain for Key Traits Using Genome-Wide Association Studies and Genomic Selection: Promising Breeding Tools for Sustainable Agriculture View all 11 articles
Leaf angle (LA) is a key component of maize plant architecture that can simultaneously govern planting density and improve final yield. However, the genetic mechanisms underlying LA have not been fully addressed. To broaden our understanding of its genetic basis, we scored three LA-related traits on upper, middle, and low leaves of 492 maize inbred lines in five environments. Phenotypic data revealed that the three LA-related traits were normally distributed, and significant variation was observed among environments and genotypes. A genome-wide association study (GWAS) was then performed to dissect the genetic factors that control natural variation in maize LA. In total, 85 significant SNPs (involving 32 non-redundant QTLs) were detected (p ≤ 2.04 × 10–6), and individual QTL explained 4.80%–24.09% of the phenotypic variation. Five co-located QTL were detected in at least two environments, and two QTLs were co-located with multiple LA-related traits. Forty-seven meta-QTLs were identified based on meta-analysis combing 294 LA-related QTLs extracted from 18 previously published studies, 816 genes were identified within these meta-QTLs, and seven co-located QTLs were jointly identified by both GWAS and meta-analysis. ZmULA1 was located in one of the co-located QTLs, qLA7, and its haplotypes, hap1 and hap2, differed significantly in LA-related traits. Interestingly, the temperate materials with hap2 had smallest LA. Finally, we also performed haplotype analysis using the reported genes that regulate LA, and identified a lot of maize germplasms that aggregated favorable haplotypes. These results will be helpful for elucidating the genetic basis of LA and breeding new maize varieties with ideal plant architecture.
Leaf angle (LA) is one of the key traits of maize plant architecture. Upright leaves can make maize plants more compact, enable them to adapt to high planting density, and reduce effects of shading between plants, thereby increasing photosynthetic efficiency and final grain yield (Stewart et al., 2003). Maize yield in the United States has increased eight-fold in the past 90 years. During this period, changes in maize LA have altered plant architecture, resulting in new varieties that are tolerant of high planting densities, allowing for more efficient light capture as planting density has increased (Tian et al., 2011). Compact plant architecture with upright leaves can increase the ability of crops to capture light energy and increase final yield (Saitoh et al., 2002).
LA is one of the important indicators used to describe maize canopy structure (Arkebauer et al., 2009) and is a quantitative trait. Thirty QTLs related to upper leaf angle were identified in a Nested Association Mapping Population (NAM) composed of 25 recombinant inbred line (RIL) families, including 4,892 lines (Tian et al., 2011). A maize BC2S3 population was constructed from teosinte and the maize inbred line W22, 12 QTLs underlying LA were identified. UPA1-NILW22 (Near Isogenic Line) has smaller LA (Upper, Middle and Low LA) than UPA1-NIL8759. On the contrary, UPA2-NILW22 has larger LA (Upper, Middle and Low LA) than UPA2-NIL8759. These results indicated that UPA1 and UPA2 have opposite regulatory patterns, and with the increase of planting density, UPA2-NIL8759 has higher grain yield (kg/ha) than UPA2-NILW22 (Tian et al., 2019). Several genes within the quantitative trait loci (QTL) that govern LA have been cloned. ZmILI1 was located within the qLA2, which was identified in a maize F2:3 population constructed from the compact inbred line Yu82 and the expanded inbred line Yu87-1. ZmILI1 (Zm00001d002121) binds to the promoter of CYP90D1 (Zm00001d039453) and inhibits expression of CYP90D1 through a CYP90D1-mediated cytochrome P450-catalyzed reaction, Brassinosteroids (BRs) can be delivered to the BR receptor BAK1, which binds to the nuclear transcription factors BZR1, BZR2 and BES1, resulting in a smaller LA (Upper LA) (Tang et al., 2010; Ren et al., 2020). Li et al. found that the rice gene oslazy1 regulates LA by affecting gravitropism (Li et al., 2007), its maize homolog ZmCLA4 regulates LA by changing mRNA accumulation, leading to changes in gravitational properties and cell development (Zhang et al., 2014). Gao et al. used a Gmilpa1 mutant with increased soybean petiole angle and isolated the gene GmILPA1, which encodes the APC8 protein. It is expressed in leaf primordium cells and can increase petiole angle by promoting the growth and division of leaf occipital cells (Gao et al., 2017).
Genome-wide association study (GWAS) has been widely used in plant genetics research and has proven to be a powerful tool for mining QTLs or causal genes for complex quantitative traits. The first GWAS report in plants was published in 2008. Single nucleotide polymorphism (SNP) haplotypes at 8,590 loci across 10 maize chromosomes were tested for association with kernel oleic acid content in 553 maize inbred lines, and a putative gene responsible for the target trait (fad2) was identified (Belo et al., 2008). Recently, Wang et al. performed whole-genome sequencing on 350 elite maize inbred lines representing multiple eras of germplasms from China and the United States and measured 15 agronomic traits for GWAS. A number of key candidate genes, such as ZmNAC16, ZmSBP18, ZmPIF4, and ZmPIF3.3, that regulate maize density tolerance and ideal plant architecture were cloned, providing an important foundation for future genomics-enabled maize breeding (Wang et al., 2020). Besides, meta-analysis is an objective method for statistically re-analyzing existing empirical literature, enabling a more unbiased evaluation of the evidence than that provided by traditional narrative commentary (Egger et al., 1997). It has been widely used to summarize and further explore complex biological mechanisms (Makinde et al., 2021), and it has also been applied in genetic studies of crop heterosis, grain yield, and stress tolerance (Li et al., 2011; Thiemann et al., 2014; Sharma et al., 2018; Wang et al., 2021). As an important plant architectural trait, LA affects the ability of the maize canopy to capture light and the light energy utilization efficiency of the population, understanding natural variation in LA and identifying its key genes are very important for breeding maize with high photosynthetic efficiency (Tao et al., 2002).
Here, 492 diverse maize inbred lines were used to investigate LA-related traits at different leaf positions in multiple environments. A GWAS was performed with 1.25 M SNPs to explore natural allelic variations that influence LA; in addition, a large amount of LA QTL data from previously published studies was used for meta-analysis. The main purposes were: 1) to explore the phenotypic variation of LA 2) to identify natural variation of SNPs/loci and candidate genes significantly associated with LA 3) to select germplasms with the favorable haplotypes of LA to improve maize plant architecture. The results will enrich our understanding of the genetic basis of LA and enhance ideotype-based maize breeding.
The association mapping panel (AMP) used in this study consists of 492 maize inbred lines, including 225 tropical/subtropical germplasms and 267 temperate germplasms, which is a subset of 527 inbred lines (Yang et al., 2011). In 2020, all 492 maize inbred lines were planted in Yuanyang Modern Agricultural Science and Technology Park of Henan Agricultural University (Yuanyang, N35°N, E113°E) in the end of April (defined as YYC) and in early July (defined as YYX). In addition, 269 inbreds (73 tropical/subtropical and 196 temperate) were randomly selected from the AMP and planted at the XunXian Experimental Station of Hebi Academy of Agricultural Sciences in Henan province (Hebi, N35°N, E114°E, defined as HB), the Cotton Seed Farm in Yongcheng, Henan (YongCheng, N33°, E116°, defined as YC) and the Yuanyang Modern Agricultural Science and Technology Park of Henan Agricultural University (Yuanyang, N35°, E113°, defined as YY) in early June 2020. All inbred lines were planted in a randomized complete block design with two replications, a single row length of 3 m, a row spacing of 0.67 m, and a final planting density of 45,000 plants/ha in all environments.
LA data from the AMP were collected using a digital angle ruler (digital display 360° angle ruler 0–200 mm, Wenzhou Weidu Electronics Co., Ltd.). To be consistent, we investigated the flowering date of each line, and LA were investigated at 15 days after pollination for each line at all environments. Three Leaf Angle (LA-related traits) was scored at three positions: the upper leaf (first leaf below the flag leaf, ULA), the middle leaf (first leaf above the first ear, MLA), and the lower leaf (second leaf below the first ear, LLA). Only ULA was investigated at YYC and YYX, whereas all three LA-related traits were recorded at HB, YC, and YY. The average values of ULA, MLA, and LLA were calculated from five uniformly growing plants in each row. The average of each LA-related traits for the two replications in each environment was calculated. Overall, ULA at all the five environments (YYC, YYX, YY, HB, and YC), MLA, and LLA at the three environments (YY, HB, and YC) were collected. These phenotypic data was used for general statistical analysis, Pearson correlation analysis, two-way ANOVA, broad-sense heritability calculation, Best Linear Unbiased Prediction (BLUP) and GWAS.
General statistical analyses (e.g. mean, variation range, standard deviation, kurtosis, skewness) were finished in SPSS Statistics V17.0 after removal of outliers. For the five values measured for one trait of a genotype, if a value is not within the range of the mean plus or minus 1.5 times of standard deviation, it will be regarded as an outlier. A repeated-measures two-way ANOVA, using the following formula: V = G + E + G × E + e, here, V is total variance, G is variance of genotype, E is environmental variance, G × E is variance of genotype-environment interaction, e is error. It was also performed in SPSS Statistics V17.0. The mixed linear model of the lme4 package in R (version 4.1.1, R Foundation for Statistical Computing, http://www.r-project.org/) was used to calculate the Best Linear Unbiased Prediction (BLUP) value for each trait in the five environments (Eugster et al., 2011). In addition, the Best Linear Unbiased Prediction (BLUP) was calculated for GWAS, which can estimate the random effects (Coram et al., 2017). For MLA and LLA, broad-sense heritability was computed using the following formula: H2 = [δG2 /δG2 +(δGE2/n)+δe2/(nr)], where δG2 is the genotypic variance, δGE2 is the genotype × environment variance, δe2 is the error variance, r is the number of replications, and n is the number of environments. Considering the unbalanced data for ULA, the harmonic mean (h), h = (492 + 269)/(492/2 + 269/3), was used to instead of the number of environments (n) for calculating the H2 (Nyquist and Baker, 1991; Schmidt et al., 2019). Pearson correlation coefficients between paired traits were calculated using the corr function in R (version 4.1.1).
The genotype data used in this study was downloaded from the Maizego website (http://www.maizego.org/Resources.html) and have been deposited in the European Variation Archive (EVA) at EMBL-EBI under accession number PRJEB56161 (https://www.ebi.ac.uk/eva/?eva-study=PRJEB56161). This genotype data was inferred from the Illumina MaizeSNP50 array, reduced-representation genome sequencing (genotyping-by-sequencing, GBS), the high density Affymetrix Axiom Maize 600K array (600K), and previous deep RNA-sequencing data of whole kernels at 15 days after pollination obtained by Liu et al. (Liu et al., 2016). The genotype data consisted of 1.25 M SNPs (B73_RefGen_v2) covering the whole maize genome with a minimum allele frequency (MAF) ≥ 0.05 (Liu et al., 2016). Here, the GLM approach controlling population structure (Q) was adopted after comparing the performances of three linear models, that is, GLM (GLM + Q, only control population structure), MLM (GLM + K, only control relative kinship) and MLM (GLM + Q + K, correcting for population structure and relative kinship) models (Li et al., 2013) implemented in TASSEL 3.0 (Bradbury et al., 2007) was therefore used to perform GWAS. For GLM model, y = Xα + Zβ + e. or y = SNP + Q + e. For MLM model, y = Xα+ Zβ + Wμ+ e. or y = SNP + Q + Kinship + e. where, y is the trait value, Xα (population structure or Q matrix) is fixed effect, Zβ (SNP or marker effect) is fixed effect, Wμ (Kinship matrix) is random effect and e is residual (Yu et al., 2006). In addition, to control the type I (false positive) and type II (false negative) error rates, Quantile-Quantile (QQ) plots of the three statistical models for each LA-related trait were compared, if a model that has a distribution closer to the diagonal line indicates a better control for type I and II errors (Zhang et al., 2010). Thus, the more appropriate model was selected to interpret the GWAS results of LA-related traits.
Taking into account the linkage disequilibrium (LD) among SNP markers, the effective marker number (En) for the genotypic dataset was 490 548, as previously calculated using GEC software (Deng et al., 2017). The suggested p-value of 2.04 × 10–6 (1/En) was used as the genome-wide threshold for significant SNP–trait associations, as commonly used in plant genome-wide association studies.
Based on a published review (Cao et al., 2022), the QTL information have been reported for LA-related traits (Lu et al., 2007; Ku et al., 2010; Tian et al., 2011; Ku et al., 2012; Chen et al., 2015; Ding et al., 2015; Hu et al., 2015; Li et al., 2015; Yang et al., 2015; Ku et al., 2016; Pan et al., 2017; Shi et al., 2017; Wang et al., 2017; Dzievit et al., 2019; Liu et al., 2019; Zhang et al., 2019; Zhang et al., 2020; Tang et al., 2021). The QTL information (Chromosome, LOD, R2, Confidence Interval and so on) were summarized from eighteen studies published in the lasted 15 years. For QTLs whose confidence interval (CI) was unknown, the following formulas were used to calculate CI:
where CI is the confidence interval, N is the number of materials in the mapping population, and R2 is the phenotypic variation. Eq. 1 was used for Backcross and F2 mapping populations, and Eq. 2 was used for recombinant inbred line (RIL) mapping populations (Darvasi and Soller, 1997). If the LOD value was unknown, it was calculated using R2 = 1–10(−2LOD/N) (Nagelkerke, 1991; Liu, 1997). QTLs without R2 information were discarded.
IBM2 2008 Neighbors (MaizeGDB, https://www.maizegdb.org/) (Sharopova et al., 2002) was used as a reference genetic map, and 19 051 high-density markers (SSR, RFLP, RAPD, and SNP) covering the whole maize genome were obtained. The markers were combined with the collected QTLs and genetic map information, and a meta-analysis was performed to analyze all QTLs and markers in the genome and obtain the most suitable number of QTLs (Veyrieras et al., 2007). Five different models (1-, 2-, 3-, 4-, or N-QTL) with different Akaike information criterion (AIC) values were proposed and used in BioMercator V4.2.3, a genetic map compilation and meta-analysis software to integrate QTL data with genome structural and functional annotation. The model with the lowest AIC-value was considered optimal (Arcade et al., 2004). Finally, the QTLs presented by the optimum model were regarded as the meta-QTLs, we named these meta-QTLs using the format M-q-trait-chromosome number-sequence number [for example, MqLA1-1, MqLA (Leaf Angle) 1 (chromosome number) -1 (sequence number)].
The previously estimated decay distance of LD in this AMP (∼30 kb, R2 = 0.1) (Liu et al., 2016) was used to define a 60-kb QTL interval, i.e., the 30 kb upstream and downstream of each SNP. In each environment and for each trait, all QTLs with overlapping QTL intervals were categorized as non-redundant QTLs. If a non-redundant QTL was detected by different LA-related traits, in different environments, from previously published LA QTLs, or physical distance of less than 10 Mb by GWAS and meta-QTL analysis, it will be defined as a co-located QTL. All potential candidate genes within all non-redundant QTLs or co-located QTLs were identified based on the filtered working gene list from the reference genome of the maize inbred line B73 (RefGen_v2). These genes were downloaded from MaizeGDB and annotated using InterProScan (http://www.ebi.ac.uk/interpro/scan.html). Phenotypic variation explained (PVE) by each QTL was estimated based on the R2 value of the most significant SNPs within the QTL. Candidate genes within the CIs of meta-QTLs were also obtained. In each non-redundant QTL or co-located QTL, the most likely candidate gene was selected based on its annotation or because it contained the peak SNP (the most significant SNP). If there was no gene in the interval, the neighboring gene of the peak SNP was considered to be the most likely candidate gene.
Gene Ontology (GO) enrichment analysis was performed using OmicShare tools (https://www.omicshare.com/tools) (Ding et al., 2019). Specifically, the candidate genes were mapped to the various sets of the GO database (http://www.geneontology.org/), the number of genes in each set was counted, and the list of genes with a specific GO function and the number of genes were obtained. The top 20 GO terms with minimum p values were selected for analysis and plotting (Lv et al., 2019).
Linkage disequilibrium (LD) was estimated by the squared correlation of the paired SNPs, which was calculated with TASSEL 3.0 software. An LD plot was generated using the ‘genetics’ and ‘LDheatmap’ package in R (version 4.1.1).
All SNPs (MAF ≥0.05) in target genes (ZmULA1, ZmCLA4, lg1, lg2 and ZmTAC1) were used for haplotype analysis by using 1.25M SNPs genotype data (Liu et al., 2016). The BLUP values of LA-related traits in 492 inbred lines were used as phenotypic data. Haplotypes contained in more than 10 inbred lines were used for comparative analysis.
LA at three positions, ULA, MLA and LLA (Supplementary Figure S1), were investigated in five environments. The LA-related traits showed the greatest variation in YY and lowest variation in YC. The maximum angle was 8.7-fold higher than the minimum angle (9.90°–86.50°) for ULA, 4.5-fold higher for MLA (14.05°–63.34°), and 3.8-fold higher for LLA (19.48°–74.00°) (Table 1). Thus, the panel exhibited rich genetic diversity in LA, which could be ranked LLA > MLA > ULA (Table 1 and Supplementary Figure S2). All phenotypes were typical quantitative traits, in that they were continuous variables that exhibited a normal distribution (Supplementary Figure S3).
TABLE 1. Descriptive statistics for leaf angle-related traits of maize in the association mapping panel in different environments.
Two-way ANOVA indicated that there were significant genetic (G) and environmental (E) effects on LA-related traits, but the effect of their interaction (G × E) was not significant (Supplementary Table S1). The results also showed that genetics had a greater effect on LA than environment. There were positive correlations between LA-related traits in all environments (Figure 1). The broad-sense heritabilities of ULA, MLA, and LLA were 0.59, 0.71, and 0.69, respectively (Table 1), again indicating that they were mainly affected by genetic factors.
FIGURE 1. Pearson correlation coefficients for LA-related traits of maize in the association mapping panel in different environments. (a‒f): HB, YC, YY, YYC, YYX, and BLUP, respectively; (1–3): ULA, MLA, and LLA, respectively.
LA was less sensitive to the K model than other two models, and this model could better control type I and type II errors (Supplementary Figure S4). In addition, we examined the distribution of the three LA traits in each sub-population across different locations, the results shown that population structure has a small effect on the leaf angle (Supplementary Figure S5). Therefore, the GWAS under the K model was analyzed further (Supplementary Figure S6).
In total, 85 significant SNPs were detected by ULA, MLA and LLA in all environments, and involving 32 non-redundant QTLs. The QTLs were distributed on all chromosomes except chromosome 5 and 9, and there was a QTL hot spot at chromosome 1 and 3 (Figure 2). Twenty-one major-effect QTLs explained more than 10% of the phenotypic variation (R2 = 10.01–24.09%). Twenty-six QTLs were identified for ULA, with a mean R2 of 9.34% (4.80%–15.16%), and seven QTLs were detected for MLA, with a mean R2 of 15.14% (9.48%–24.09%). Only one QTL was associated with LLA, and it explained 11.63% of the phenotypic variation.
FIGURE 2. Distribution of significant loci detected by GWAS on maize chromosomes. Red arrows indicate co-located QTLs, for the same trait in different environments.
Fourteen non-redundant QTLs were detected in HB and explained 9.08%–21.93% of the phenotypic variation. Ten non-redundant QTLs were detected in YY (10.05%–15.16%), nine in BLUP (4.80%–24.09%), and only four and two in YYC and YYX, respectively. No significant QTLs were detected in YC (Figure 2).
Five QTLs were identified in multiple environments (Figure 2). For ULA, qLA2 was detected in YYC, YYX, and BLUP, and qLA3 and qLA27 were detected in YYC and BLUP. For MLA, qLA23 was identified in HB and BLUP, and qLA29 was detected in BLUP and YY. These loci can be stably inherited in different environments and play an important role in the regulation of plant architecture. QTLs detected for different traits may have pleiotropic effects. qLA1 was identified by MLA and ULA and may therefore regulate the size of both ULA and MLA. In addition, qLA21 was also detected in different traits (ULA and MLA), suggesting that qLA21 may have a similar role in the regulation of LA at different positions. Detailed information on the GWAS results, including the physical position, p-value, and R2 for each QTL is provided in Supplementary Table S3.
Based on the B73 RefGen_v2 reference genome (https://www.maizegdb.org/), 82 genes were found within 32 non-redundant QTLs. For example, GRMZM2G049159 within qLA7 was identified for ULA in HB and encodes a GRAS family transcription factor; its homolog OsGRAS23 induces downstream stress-responsive genes and positively regulates drought tolerance in rice (Xu et al., 2015), and it may be involved in the regulation of rosette leaf development in Arabidopsis (Schmid et al., 2005). qLA18 was also identified for ULA in HB, and four genes are located in this QTL. Among them, GRMZM2G071705 encodes an F-box protein; this type of protein may be involved in the strigolactone (SL) biosynthetic pathway and further regulate plant architectural traits such as tiller number and LA (Ishikawa et al., 2005; Dong et al., 2016).
Twenty genes were identified in co-located QTLs and may be involved in protein ubiquitination (GRMZM2G134176), carbon metabolism (GRMZM2G134256), and the citric acid cycle (GRMZM2G158378) and play important roles in the regulation of plant growth and stress tolerance. GRMZM2G311328 is located in qLA21, which was identified for MLA and ULA in HB. This gene may be pleiotropic; its homolog encodes a vesicle auxin mediated transporter, and regulation of auxin polar transport is important for development and architecture of Arabidopsis (Kitakura et al., 2011). GRMZM2G134073 in qLA29 was simultaneously identified for MLA in BLUP and YY; it encodes a NAC family transcription factor that can promote resistance to heat damage (Li H et al., 2020). Its homolog OsNAC10 plays a key role in rice drought and disease resistance (Jeong et al., 2010), and it may also participate in regulating plant architecture and abiotic stress tolerance.
Among the 82 genes, only GRMZM2G071790 has been reported, it encodes a beta-6 tubulin and plays an important role in maize tolerance to Ustilago maydis (Ruan et al., 2021), as well as catalyzing auxin transport in Arabidopsis (Terasaka et al., 2005). Although most of these genes screened by GWAS have unknown functions, their homologous genes can provide valuable information. Interestingly, some genes may have pleiotropic effects, that may play important roles at both LA and stress resistance. Thus, our results provide new and valuable information for understanding the genetic mechanisms that underlie LA.
A total of 294 QTLs related to LA were obtained from studies published in the last 15 years (Supplementary Table S4) (Cao et al., 2022). 47 meta-QTLs were obtained by the meta-QTL analysis, containing 816 genes. These 47 QTLs were distributed on all chromosomes except chromosome 6 (Table 2 and Figure 3). The largest number of meta-QTLs (9) were located on chromosome 8, and only one was located on chromosome 2. Seven co-located QTLs were identified by both GWAS and meta-analysis (Table 3). Based on the GWAS results, 17 genes within the seven co-located QTLs were examined, but none had been characterized previously. Gene Ontology enrichment was then performed with the 17 genes, and they were mainly involved in the synthesis of intracellular parts (cellular component, GO: 0044424), transcriptional regulatory activity (molecular function, GO:0140110) and organic substance biosynthetic process (biological process, GO:1901576) (Figure 4). Interestingly, LA-related genes identified to date encode transcription factors or participate in hormonal signal transduction (Cao et al., 2022), and plant hormones are natural organic compounds (Adam 1999). These results suggest that the 17 genes in the co-localized QTLs may regulate LA by encoding transcription factors and/or participating in the synthesis of organic compounds, including plant hormones.
FIGURE 4. GO enrichment analysis of seventeen genes within seven co-localized QTLs. Three GO Ontologies are shown (A) Cellular Component; (B) Molecular Function, and (C) Biological Process.
qLA7, which was related to ULA, was located on chromosome 1 and had an extremely strong association signal (Figure 5A and Figure 5B). There was only one gene (GRMZM2G049159) within this QTL, and it encodes a GRAS family transcription factor, involved in the synthesis of organic (GO:1901576) (Figure 4), plant hormones such as CK and BR, as natural organic compounds, play an important role in regulating LA. Therefore, ZmULA1 may be involved in the metabolic process of plant hormones to regulate maize LA. Additionally, its homolog may be involved in the development of rosette leaves in Arabidopsis (Schmid et al., 2005). We speculate that it may affect the formation of LA in maize, and we named it ZmULA1.
FIGURE 5. ZmULA1 affected LA-related traits. (A) Manhattan plot of ULA at HB. The line represents the threshold −log10(P) ≥ 5.69 (p ≤ 2.04 × 10–6). (B) Enlarged Manhattan plot of the lead SNP and 85 SNPs associated with ZmULA1; red diamonds represent the lead SNP. (C) R2 values of significant SNPs associated with ZmULA1; the lead SNP was located in 2.64 kb downstream of ZmULA1.
To analyze the haplotype of ZmULA1, we extracted all polymorphic sites within one LD decay distance near the lead SNP (chr1.S_40141659, p = 7.57e−08) to perform LD analysis, there was a strong linkage relationship (the average pairwise r2 value was 0.45) between the lead SNP and polymorphic sites (Figure 5C). Subsequent analysis of 17 SNPs in ZmULA1 using BLUP values of 492 lines identified two haplotypes. All maize inbred lines belonged to hap1 (412) or hap2 (80), and the mean value of hap2 was smaller than that of hap1 for the three LA-related traits (Supplementary Table S5). Specifically, ULA (p = 7.20e−10), MLA (p = 3.80e−08), and LLA (p = 1.40e−05) showed extremely significant differences between hap1 and hap2 (Figure 6). Temperate materials had smaller values of LA-related traits (ULA, MLA and LLA) than tropical lines, and the temperate materials with hap2 had the smallest LAs (Figure 7). Furthermore, 65% (52/80) of the maize inbred lines derived from China belonged to hap2 (Table 4), and these elite inbred lines can be used to improve the plant architecture of maize cultivars. In summary, these results suggest that natural variation in ZmULA1 may affect the three LA-related traits, which may be influenced by constant selection during maize breeding. In addition, we conducted haplotype analysis of four known genes (ZmCLA4, lg1, lg2 and ZmTAC1) that regulate LA in previous studies (Harper and Freeling, 1996; Moreno et al., 1997; Yu et al., 2007; Zhang et al., 2014), favorable haplotype for each gene which have smallest LA was identified, that are hap4 for ZmCLA4, hap4 for lg1, hap2 for lg2 and hap2 for ZmTAC1 (Figure 8). The germplasms with favorable haplotype combinations of 492 inbred lines were identified, and a trend was found that the more favorable haplotypes, the smaller the leaf angle (Supplementary Table S6).
FIGURE 6. Differences in LA-related traits between Hap1 and Hap2. (A) ULA. (B) MLA. (C) (LLA). *: p < 0.05, **: p < 0.01, ***: p < 0.001, ****: p < 0.0001.
FIGURE 7. Differences in LA-related traits between Hap1 (temperate), Hap1 (tropical), Hap2 (temperate), and Hap2 (tropical). (A) ULA. (B) MLA. (C) (LLA). The significance level was obtained by comparing others haplotypes with Hap1(temperate), respectively. *: p < 0.05, **: p < 0.01, ***: p < 0.001, ****: p < 0.0001, not marked stands for not significant.
TABLE 4. The number of haplotypes for ZmULA1 in 492 maize inbred lines classified by origin and source, respectively.
FIGURE 8. Differences of haplotypes in LA-related traits within four cloned genes that regulate maize LA. (A–D), ULA, of ZmCLA4, lg1, lg2, ZmTAC1, respectively; (E–H), MLA, of ZmCLA4, lg1, lg2, ZmTAC1, respectively; (I–L), LLA, of ZmCLA4, lg1, lg2, ZmTAC1, respectively. The significance level was obtained by comparing others haplotype with hap1, respectively. *: p < 0.05, **: p < 0.01, ***: p < 0.001, ****: p < 0.0001, not marked stands for not significant.
Increasing planting density is an important way to improve maize yield, and LA is one of the key traits that determine whether maize can tolerate high planting density. Several genes that regulate maize LA have been cloned in previous studies. For example, lg1 (liguleless1) encodes a protein containing an SBP domain, and its mutation causes the loss of tongues and ears, making plants more compact (Moreno et al., 1997). lg2 (liguleless2) encodes a basic leucine zipper (bZIP) transcription factor. These two genes are located in the same developmental pathway, in which lg2 plays an earlier role than lg1 (Harper and Freeling, 1996; Walsh et al., 1998). ZmTAC1 is also considered to regulate LA in maize. It encodes a protein composed of 263 amino acids that is most highly expressed in the maize leaf sheath, and the difference in LA traits between the compact leaf inbred line Yu82 and the extended leaf inbred line Shen137 is caused by nucleotide variation in its non-coding region (Ku et al., 2011). Recently, Li et al. cloned ZmACS7 from the Sdw3 mutant; its overexpression caused lower plant height and higher LA, and multi-omics analysis showed that it alters plant architecture by promoting growth of the auricle and inhibiting elongation of the internode cells (Li Z et al., 2020). The genetic and molecular mechanisms of LA have also been widely dissected in other crops. For example, OsTAC1 in rice is a homolog of maize ZmTAC1 and has an intron sequence AGGA in the 3′ non-coding region. A single SNP variation (A/G) that changes ‘AGGA’ to ‘GGGA’ can lead to reduced expression of ZmTAC1 and an LA close to zero, making the rice plant more compact (Yu et al., 2007).
In this study, 85 significant SNPs in 32 QTLs were identified by GWAS and included 82 genes. Seven QTLs could be co-located in different environments or different LA-related traits (Figure 2), they are considered as genomic hot regions and worth to mining for valuable information. qLA1 and qLA21 were co-located by ULA and MLA and they explained 16.96% and 9.48% of phenotypic variation, respectively. Only one gene (GRMZM2G158378) was found within qLA1, and its rice homolog has been shown to promote the transport and absorption of silicon (Mitani et al., 2009). Five genes were located in qLA21, one of which encoded a glycosyltransferase (GRMZM2G014770) and another a vesicle-mediated substance transporter (GRMZM2G311328). Five co-located QTLs (qLA2, qLA3, qLA23, qLA27, and qLA29), explaining 6.13%–18.89% of phenotypic variation, were identified in at least two environments, indicating that they are genetically stable and less affected by the environment. Most QTLs (∼84%) were detected in only one environment (27/32); a possible explanation is that LA is a complex quantitative trait regulated by a large number of minor genes that are greatly affected by the environment, leading QTL effects to vary in different environments. It is worth mentioning that 21 of the 32 QTLs (∼66%) were major QTLs with R2 values greater than 10%. These results provide new information for understanding the genetic basis of the natural variation in LA.
In the current study, we collected QTLs for LA published over the past 15 years. In total, 294 QTLs were obtained and mapped to the IBM2 2008 Neighbors genetic map; 47 meta-QTLs were then identified by meta-analysis (Figure 3 and Table 2). Meta-analysis can effectively narrow the confidence interval of QTL and improve the prediction accuracy of candidate genes (Goffinet and Gerber, 2000; Arcade et al., 2004). Moreover, 816 genes were examined in 47 meta-QTLs; fifteen had been reported previously, and five had been functionally characterized. Two genes (GRMZM2G005066, c1 and GRMZM2G089713, sh1) were involved in kernel colored aleurone and endosperm development (Tohge et al., 2017; Zhou et al., 2021). GRMZM2G141399 (du1) encoded starch synthase III, which makes kernels glassy and inhibits glycogen accumulation (Cao et al., 1999). Two additional genes (GRMZM2G051637, cr4 and GRMZM2G419806, oy1) were associated with plant height and senescence of maize seedlings (Peiffer et al., 2014; Khangura et al., 2020). Notably, AC195340.3_FG001 (tua1), located in MqLA5-6, is associated with plant architecture at different planting densities and encodes an alpha tubulin family protein; it has been annotated but not functionally characterized (Incognito et al., 2020). These results suggested that meta-analysis can provide more valuable information.
Seven QTLs were jointly identified by GWAS and meta-analysis (Table 3), and two, qLA3 and qLA7, had significant association signals at chromosome one by GWAS (Figure 5A). Three genes (AC205725.3_FG010, GRMZM2G471253, and GRMZM2G171073) were located in qLA3 and were expressed in leaves, potentially affecting leaf development. GRMZM2G171073 encodes a C2H2-like zinc finger protein, and its homolog IDD1 is involved in gibberellin (GA) signal transduction and transport in Arabidopsis (Fukazawa et al., 2014). GA affects plant structure and interacts with BR, causing variation in LA (Tang et al., 2018). ZmULA1 was located in qLA7, and hap1 and hap2 for this gene showed highly significant differences in LA-related traits (Figure 6). Hap2 was considered as a favorable haplotype because the hap2 had smaller LA (ULA, MLA and LLA) than other haplotypes, and temperate germplasms with hap2 had the smallest LA (Figure 7). About 80% (52/65) of the elite temperate germplasms from China (Table 4). Four genes (ZmCLA4, lg1, lg2 and ZmTAC1) have been shown to regulate LA, and a lot of maize inbred lines with favorable haplotypes were selected by haplotype analysis (Figure 8). The results indicated that there was a trend that the more favorable alleles, the smaller the leaf angle. The germplasms carrying favorable haplotypes can be used to improve maize plant architecture to increase planting density and increase maize yield.
Although GWAS has been recognized as a powerful method for understanding the genetic basis of complex quantitative traits, meta-analysis is complementary to GWAS and can increase precision and accuracy of detected QTLs. Therefore, combining GWAS and meta-analysis, we screened a lot of potential targets/loci regulating LA in maize, and the candidate gene, ZmULA1, was predicted to play important roles in the regulation of LA. These results will provide reference for improving maize plant architecture.
The datasets presented in this study can be found in online repositories. The names of the repository/repositories and accession number(s) can be found below: https://www.ebi.ac.uk/eva/, PRJEB56161.
XZ and JT designed the research. XZ supervised the research. HD, YS, XX, LS, JG, NL, JZ, JC, and ZF performed the experiment. HD, JL, and WL analyzed the data. HD and XZ wrote the paper. All authors read and approved the final manuscript.
This research was supported by the National Natural Science Foundation of China (32171980), a project funded by the China Postdoctoral Science Foundation (2020M682295), a first-class postdoctoral research grant in Henan Province (202001032), the Research Start-up Fund for Youth Talents of Henan Agricultural University (30500563), the Open Project Funding of the State Key Laboratory of Crop Stress Adaptation and Improvement (2021KF07) and the HAU grant for collaborative crop science research (CCSR2022-1).
We thank the research groups of Prof. Jianbing Yan at Huazhong Agricultural University and Prof. Xiaohong Yang at China Agricultural University for providing the maize association mapping panel and genotypic data.
The authors declare that the research was conducted in the absence of any commercial or financial relationships that could be construed as a potential conflict of interest.
All claims expressed in this article are solely those of the authors and do not necessarily represent those of their affiliated organizations, or those of the publisher, the editors and the reviewers. Any product that may be evaluated in this article, or claim that may be made by its manufacturer, is not guaranteed or endorsed by the publisher.
The Supplementary Material for this article can be found online at: https://www.frontiersin.org/articles/10.3389/fgene.2022.1004211/full#supplementary-material.
Adam, C. (1999). Fatty acid composition of Mesorhizobium huakuii lipopolysaccharides. Identification of 27-oxooctacosanoic acid. FEMS Microbiology Letters. doi:10.1016/S0378-1097(99)00321-3
Arcade, A., Labourdette, A., Falque, M., Mangin, B., Chardon, F., Charcosset, A., et al. (2004). BioMercator: integrating genetic maps and QTL towards discovery of candidate genes. Bioinformatics 20, 2324–2326. doi:10.1093/bioinformatics/bth230
Arkebauer, T. J., Walter-Shea, E. A., Mesarch, M. A., Suyker, A. E., and Verma, S. B. (2009). Scaling up of Co2 fluxes from leaf to canopy in maize-based agroecosystems. Agric. For. Meteorol. 149, 2110–2119. doi:10.1016/j.agrformet.2009.04.013
Beló, A., Zheng, P., Luck, S., Shen, B., Meyer, D. J., Li, B., et al. (2008). Whole genome scan detects an allelic variant of fad2 associated with increased oleic acid levels in maize. Mol. Genet. Genomics 279, 1–10. doi:10.1007/s00438-007-0289-y
Bradbury, P. J., Zhang, Z., Kroon, D. E., Casstevens, T. M., Ramdoss, Y., and Buckler, E. S. (2007). TASSEL: software for association mapping of complex traits in diverse samples. Bioinformatics 23, 2633–2635. doi:10.1093/bioinformatics/btm308
Cao, H., Imparl-Radosevich, J., Guan, H., Keeling, P. L., James, M. G., and Myers, A. M. (1999). Identification of the soluble starch synthase activities of maize endosperm. Plant Physiol. 120, 205–216. doi:10.1104/pp.120.1.205
Cao, Y., Zhong, Z., Wang, H., and Shen, R. (2022). Leaf angle: a target of genetic improvement in cereal crops tailored for high-density planting. Plant Biotechnol. J. 20, 426–436. doi:10.1111/pbi.13780
Chen, X., Xu, D., Liu, Z., Yu, T., Mei, X., and Cai, Y. (2015). Identification of qtl for leaf angle and leaf space above ear position across different environments and generations in maize (Zea mays L). Euphytica 204, 395–405. doi:10.1007/s10681-015-1351-1
Coram, M. A., Fang, H., Candille, S. I., Assimes, T. L., and Tang, H. (2017). Leveraging multi-ethnic evidence for risk assessment of quantitative traits in minority populations. Am. J. Hum. Genet. 101, 218–226. doi:10.1016/j.ajhg.2017.06.015
Darvasi, A., and Soller, M. (1997). A simple method to calculate resolving power and confidence interval of QTL map location. Behav. Genet. 27, 125–132. doi:10.1023/a:1025685324830
Deng, M., Li, D., Luo, J., Xiao, Y., Liu, H., Pan, Q., et al. (2017). The genetic architecture of amino acids dissection by association and linkage analysis in maize. Plant Biotechnol. J. 10, 1250–1263. doi:10.1111/pbi.12712
Ding, J., Zhang, L., Chen, J., Li, X., Li, Y., Cheng, H., et al. (2015). Genomic dissection of leaf angle in maize (Zea mays L.) using a four-way cross mapping population. PLoS One 10, e0141619. doi:10.1371/journal.pone.0141619
Ding, L., Zhao, K., Zhang, X., Song, A., Su, J., Hu, Y., et al. (2019). Comprehensive characterization of a floral mutant reveals the mechanism of hooked petal morphogenesis in Chrysanthemum morifolium. Plant Biotechnol. J. 17, 2325–2340. doi:10.1111/pbi.13143
Dong, H., Zhao, H., Xie, W., Han, Z., Li, G., Yao, W., et al. (2016). A novel tiller angle gene, TAC3, together with TAC1 and D2 largely determine the natural variation of tiller angle in rice cultivars. PLoS Genet. 12, e1006412. doi:10.1371/journal.pgen.1006412
Dzievit, M. J., Li, X., and Yu, J. (2019). Dissection of leaf angle variation in maize through genetic mapping and meta-analysis. Plant Genome 12, 180024. doi:10.3835/plantgenome2018.05.0024
Egger, M., Smith, G. D., and Phillips, A. N. (1997). Meta-analysis: principles and procedures. BMJ 315, 1533–1537. doi:10.1136/bmj.315.7121.1533
Eugster, M., Knaus, J., Porzelius, C., Schmidberger, M., and Vicedo, E. (2011). Hands-on tutorial for parallel computing with R. Comput. Stat. 26, 219–239. doi:10.1007/s00180-010-0206-4
Fukazawa, J., Teramura, H., Murakoshi, S., Nasuno, K., Nishida, N., Ito, T., et al. (2014). DELLAs function as coactivators of GAI-ASSOCIATED FACTOR1 in regulation of gibberellin homeostasis and signaling in Arabidopsis. Plant Cell 26, 2920–2938. doi:10.1105/tpc.114.125690
Gao, J., Yang, S., Cheng, W., Fu, Y., Leng, J., Yuan, X., et al. (2017). GmILPA1, encoding an APC8-like protein, controls leaf petiole angle in soybean. Plant Physiol. 174, 1167–1176. doi:10.1104/pp.16.00074
Goffinet, B., and Gerber, S. (2000). Quantitative trait loci: a meta-analysis. Genetics 155, 463–473. doi:10.1093/genetics/155.1.463
Harper, L., and Freeling, M. (1996). Interactions of liguleless1 and liguleless2 function during ligule induction in maize. Genetics 144, 1871–1882. doi:10.1093/genetics/144.4.1871
Hu, Y., Gu, Y., Xiao, Q., Hou, X., Zhang, X., et al. (2015). Genetic analysis for canopy architecture in an F2:3 population derived from two-type foundation parents across multi-environments. Euphytica 205, 421–440. doi:10.1007/s10681-015-1401-8
Incognito, S., Maddonni, G., and López, C. (2020). Genetic control of maize plant architecture traits under contrasting plant densities. Euphytica 216, 20. doi:10.1007/s10681-019-2552-9
Ishikawa, S., Maekawa, M., Arite, T., Onishi, K., Takamure, I., and Kyozuka, J. (2005). Suppression of tiller bud activity in tillering dwarf mutants of rice. Plant Cell Physiol. 46, 79–86. doi:10.1093/pcp/pci022
Jeong, J. S., Kim, Y. S., Baek, K. H., Jung, H., Ha, S., Do Choi, Y., et al. (2010). Root-specific expression of OsNAC10 improves drought tolerance and grain yield in rice under field drought conditions. Plant Physiol. 153, 185–197. doi:10.1104/pp.110.154773
Khangura, R. S., Johal, G. S., and Dilkes, B. P. (2020). Variation in maize chlorophyll biosynthesis alters plant architecture. Plant Physiol. 184, 300–315. doi:10.1104/pp.20.00306
Kitakura, S., Vanneste, S., Robert, S., Löfke, C., Teichmann, T., Tanaka, H., et al. (2011). Clathrin mediates endocytosis and polar distribution of PIN auxin transporters in Arabidopsis. Plant Cell 23, 1920–1931. doi:10.1105/tpc.111.083030
Ku, L., Ren, Z., Chen, X., Shi, Y., Qi, J., Su, H., et al. (2016). Genetic analysis of leaf morphology underlying the plant density response by QTL mapping in maize (Zea mays L). Mol. Breed. 36, 63–16. doi:10.1007/s11032-016-0483-x
Ku, L., Wei, X., Zhang, S., Zhang, J., Guo, S., and Chen, Y. (2011). Cloning and characterization of a putative TAC1 ortholog associated with leaf angle in maize (Zea mays L). PLoS One 6, e20621. doi:10.1371/journal.pone.0020621
Ku, L., Zhang, J., Guo, S., Liu, H., Zhao, R., and Chen, Y. (2012). Integrated multiple population analysis of leaf architecture traits in maize (Zea mays L). J. Exp. Bot. 63, 261–274. doi:10.1093/jxb/err277
Ku, L., Zhao, W., Zhang, J., Wu, L., Wang, C., Wang, P., et al. (2010). Quantitative trait loci mapping of leaf angle and leaf orientation value in maize (Zea mays L). Theor. Appl. Genet. 121, 951–959. doi:10.1007/s00122-010-1364-z
Li, C., Li, Y., Shi, Y., Song, Y., Zhang, D., Buckler, E. S., et al. (2015). Genetic control of the leaf angle and leaf orientation value as revealed by ultra-high density maps in three connected maize populations. PLoS One 10, e0121624. doi:10.1371/journal.pone.0121624
Li, H., Peng, Z., Yang, X., Wang, W., Fu, J., Wang, J., et al. (2013). Genome-wide association study dissects the genetic architecture of oil biosynthesis in maize kernels. Nat. Genet. 45, 43–50. doi:10.1038/ng.2484
Li, H., Wang, L., Liu, M., Dong, Z., Li, Q., Fei, S., et al. (2020). Maize plant architecture is regulated by the ethylene biosynthetic gene ZmACS7. Plant Physiol. 183, 1184–1199. doi:10.1104/pp.19.01421
Li, J., Zhang, Z., Li, Y., Wang, Q., and Zhou, Y. (2011). QTL consistency and meta-analysis for grain yield components in three generations in maize. Theor. Appl. Genet. 122, 771–782. doi:10.1007/s00122-010-1485-4
Li, P., Wang, Y., Qian, Q., Fu, Z., Wang, M., Zeng, D., et al. (2007). LAZY1 controls rice shoot gravitropism through regulating polar auxin transport. Cell Res. 17, 402–410. doi:10.1038/cr.2007.38
Li, Z., Tang, J., Srivastava, R., Bassham, D. C., and Howell, S. H. (2020). The transcription factor bZIP60 links the unfolded protein response to the heat stress response in maize. Plant Cell 32, 3559–3575. doi:10.1105/tpc.20.00260
Liu, H., Luo, X., Niu, L., Xiao, Y., Chen, L., Liu, J., et al. (2016). Distant eQTLs and non-coding sequences play critical roles in regulating gene expression and quantitative trait variation in maize. Mol. Plant 10, 414–426. doi:10.1016/j.molp.2016.06.016
Liu, X., Hao, L., Kou, S., Su, E., Zhou, Y., and Wang, R. (2019). The combined effects of omitting confounders and measurement error on statistical inference of mediation and a new tool for sensitivity analysis. Multivar. Behav. Res. 39, 137–138. doi:10.1080/00273171.2019.1694478
Lu, M., Zhou, F., Xie, C., Li, M., Xu, Y., Marilyn, W., et al. (2007). Construction of a SSR linkage map and mapping of quantitative trait loci (QTL) for leaf angle and leaf orientation with an elite maize hybrid. Yi Chuan 29, 1131–1138. doi:10.1360/yc-007-1131
Lv, R., Li, Z., Li, M., Dogra, V., Lv, S., Liu, R., et al. (2019). Uncoupled expression of nuclear and plastid photosynthesis-associated genes contributes to cell death in a lesion mimic mutant. Plant Cell 31, 210–230. doi:10.1105/tpc.18.00813
Makinde, F. L., Tchamga, M. S. S., Jafali, J., Fatumo, S., Chimusa, E. R., Mulder, N., et al. (2021). Reviewing and assessing existing meta-analysis models and tools. Brief. Bioinform. 22, bbab324. doi:10.1093/bib/bbab324
Mitani, N., Chiba, Y., Yamaji, N., and Ma, J. (2009). Identification and characterization of maize and barley Lsi2-like silicon efflux transporters reveals a distinct silicon uptake system from that in rice. Plant Cell 21, 2133–2142. doi:10.1105/tpc.109.067884
Moreno, M. A., Harper, L. C., Krueger, R. W., Dellaporta, S. L., and Freeling, M. (1997). liguleless1 encodes a nuclear-localized protein required for induction of ligules and auricles during maize leaf organogenesis. Genes Dev. 11, 616–628. doi:10.1101/gad.11.5.616
Nagelkerke, N. (1991). A note on a general definition of the coefficient of determination. Biometrika 78, 691–692. doi:10.1093/biomet/78.3.691
Nyquist, E., and Baker, J. (1991). Estimation of heritability and prediction of selection response in Plant-populations. Crit. Rev. Plant Sci. 10, 235–322. doi:10.1080/07352689109382313
Pan, Q., Xu, Y., Li, K., Peng, Y., Zhan, W., Li, W., et al. (2017). The genetic basis of plant architecture in 10 maize recombinant inbred line populations. Plant Physiol. 175, 858–873. doi:10.1104/pp.17.00709
Peiffer, J. A., Romay, M. C., Gore, M. A., Flint-Garcia, S. A., Zhang, Z., Millard, M. J., et al. (2014). The genetic architecture of maize height. Genetics 196, 1337–1356. doi:10.1534/genetics.113.159152
Ren, Z., Wu, L., Ku, L., Wang, H., Zeng, H., Su, H., et al. (2020). ZmILI1 regulates leaf angle by directly affecting liguleless1 expression in maize. Plant Biotechnol. J. 18, 881–883. doi:10.1111/pbi.13255
Ruan, X., Ma, L., Zhang, Y., Wang, Q., and Gao, X. (2021). Dissection of the complex transcription and metabolism regulation networks associated with maize resistance to Ustilago maydis. Genes (Basel) 12, 1789. doi:10.3390/genes12111789
Saitoh, K., Yonetani, K., Murota, T., and Kuroda, T. (2002). Effects of flag leaves and panicles on light interception and canopy photosynthesis in high-yielding rice cultivars. Plant Prod. Sci. 5, 275–280. doi:10.1626/pps.5.275
Schmid, M., Davison, T. S., Henz, S. R., Pape, U. J., Demar, M., Vingron, M., et al. (2005). A gene expression map of Arabidopsis thaliana development. Nat. Genet. 37, 501–506. doi:10.1038/ng1543
Schmidt, P., Hartung, J., Rath, J., and Piepho, H. (2019). Estimating broad-sense heritability with unbalanced data from agricultural cultivar trials. Crop Sci. 59, 525–536. doi:10.2135/cropsci2018.06.0376
Sharma, R., Singh, G., Bhattacharya, S., and Singh, A. (2018). Comparative transcriptome meta-analysis of Arabidopsis thaliana under drought and cold stress. PLoS One 13, e0203266. doi:10.1371/journal.pone.0203266
Sharopova, N., McMullen, M. D., Schultz, L., Schroeder, S., Sanchez-Villeda, H., Gardiner, J., et al. (2002). Development and mapping of SSR markers for maize. Plant Mol. Biol. 48, 463–481. doi:10.1023/a:1014868625533
Shi, Y., Wang, X., Guo, S., Ren, Z., Ku, L., Zhu, Y., et al. (2017). Detection of epistatic and environmental interaction qtls for leaf orientation‐related traits in maize. Plant Breed. 136, 33–40. doi:10.1111/pbr.12431
Stewart, D. W., Costa, C., Dwyer, L. M., Smith, D. L., Hamilton, R. I., and Ma, B. L. (2003). Canopy structure, light interception, and photosynthesis in maize. Agron. J. 95, 1465–1474. doi:10.2134/agronj2003.1465
Tang, D., Chen, Z., Ni, J., Jiang, Q., Liu, J., Wang, L., et al. (2021). Identification of qtl for leaf angle at canopy-wide levels in maize. Euphytica 217, 75. doi:10.1007/s10681-021-02781-4
Tang, W., Deng, Z., and Wang, Z. (2010). Proteomics shed light on the brassinosteroid signaling mechanisms. Curr. Opin. Plant Biol. 13, 27–33. doi:10.1016/j.pbi.2009.10.007
Tang, Y., Liu, H., Guo, S., Wang, B., Li, Z., Chong, K., et al. (2018). OsmiR396d affects gibberellin and brassinosteroid signaling to regulate plant architecture in rice. Plant Physiol. 176, 946–959. doi:10.1104/pp.17.00964
Tao, S., Chu, J., Liu, X., Zhang, R., Zhang, Z., and Luo, Z. (2002). High-resolution gene mapping using admixture linkage disequilibrium. Chin. Sci. Bull. 47, 1717–1719. doi:10.1007/bf03183315
Terasaka, K., Blakeslee, J. J., Titapiwatanakun, B., Peer, W. A., Bandyopadhyay, A., Makam, S. N., et al. (2005). PGP4, an ATP binding cassette P-glycoprotein, catalyzes auxin transport in Arabidopsis thaliana roots. Plant Cell 17, 2922–2939. doi:10.1105/tpc.105.035816
Thiemann, A., Fu, J., Seifert, F., Grant-Downton, R. T., Schrag, T. A., Pospisil, H., et al. (2014). Genome-wide meta-analysis of maize heterosis reveals the potential role of additive gene expression at pericentromeric loci. BMC Plant Biol. 14, 88. doi:10.1186/1471-2229-14-88
Tian, F., Bradbury, P. J., Brown, P. J., Hung, H., Sun, Q., Flint-Garcia, S., et al. (2011). Genome-wide association study of leaf architecture in the maize nested association mapping population. Nat. Genet. 43, 159–162. doi:10.1038/ng.746
Tian, J., Wang, C., Xia, J., Wu, L., Xu, G., Wu, W., et al. (2019). Teosinte ligule allele narrows plant architecture and enhances high-density maize yields. Science 365, 658–664. doi:10.1126/science.aax5482
Tohge, T., de Souza, L. P., and Fernie, A. R. (2017). Current understanding of the pathways of flavonoid biosynthesis in model and crop plants. J. Exp. Bot. 68, 4013–4028. doi:10.1093/jxb/erx177
Veyrieras, J. B., Goffinet, B., and Charcosset, A. (2007). MetaQTL: a package of new computational methods for the meta-analysis of QTL mapping experiments. BMC Bioinforma. 8, 49. doi:10.1186/1471-2105-8-49
Walsh, J., Waters, C. A., and Freeling, M. (1998). The maize gene liguleless2 encodes a basic leucine zipper protein involved in the establishment of the leaf blade-sheath boundary. Genes Dev. 12, 208–218. doi:10.1101/gad.12.2.208
Wang, B., Lin, Z., Li, X., Zhao, Y., Zhao, B., Wu, G., et al. (2020). Genome-wide selection and genetic improvement during modern maize breeding. Nat. Genet. 52, 565–571. doi:10.1038/s41588-020-0616-3
Wang, H., Liang, K., Li, X., Hu, Y., Wu, H., Wang, Z., et al. (2017). QTL analysis of ear leaf traits in maize (Zea mays L.) under different planting densities. Crop J. 5, 387–395. doi:10.1016/j.cj.2017.05.001
Wang, J., Yan, C., Shi, D., Zhao, X., Yuan, C., Sun, Q., et al. (2021). The genetic base for peanut height-related traits revealed by a meta-analysis. Plants (Basel) 10, 1058. doi:10.3390/plants10061058
Xu, K., Chen, S., Li, T., Ma, X., Liang, X., Ding, X., et al. (2015). OsGRAS23, a rice GRAS transcription factor gene, is involved in drought stress response through regulating expression of stress-responsive genes. BMC Plant Biol. 15, 141. doi:10.1186/s12870-015-0532-3
Yang, G., Dong, Y., Li, Y., Wang, Q., Shi, Q., Zhou, Q., et al. (2015). Integrative detection and verification of QTL for plant traits in two connected RIL populations of high-oil maize. Euphytica 206, 203–223. doi:10.1007/s10681-015-1502-4
Yang, X., Gao, S., Xu, S., Zhang, Z., Prasanna, B. M., Li, L., et al. (2011). Characterization of a global germplasm collection and its potential utilization for analysis of complex quantitative traits in maize. Mol. Breed. 28, 511–526. doi:10.1007/s11032-010-9500-7
Yu, B., Lin, Z., Li, H., Li, X., Li, J., Wang, Y., et al. (2007). TAC1, a major quantitative trait locus controlling tiller angle in rice. Plant J. 52, 891–898. doi:10.1111/j.1365-313X.2007.03284.x
Yu, J., Pressoir, G., Briggs, W. H., Vroh Bi, I., Yamasaki, M., Doebley, J. F., et al. (2006). A unified mixed-model method for association mapping that accounts for multiple levels of relatedness. Nat. Genet. 8, 203–208. doi:10.1038/ng1702
Zhang, J., Ku, L., Han, Z., Guo, S., Liu, H., Zhang, Z., et al. (2014). The ZmCLA4 gene in the qLA4-1 QTL controls leaf angle in maize (Zea mays L). J. Exp. Bot. 65, 5063–5076. doi:10.1093/jxb/eru271
Zhang, K., Lv, F., Li, J., Wang, H., Yu, J., Li, W., et al. (2019). Genetic mapping of quantitative trait locus for the leaf morphological traits in a recombinant inbred line population by ultra‐high–density maps across multi‐environments of maize (Zea mays). Plant Breed. 139, 107–118. doi:10.1111/pbr.12749
Zhang, M., Jin, Y., Ma, Y., Zhang, Q., Wang, P., Jiang, N., et al. (2020). Identification of QTLs and candidate genes associated with leaf angle and leaf orientation value in maize (zea mays L.) based on GBS. Trop. Plant Biol. 1, 34–49. doi:10.1007/s12042-020-09270-3
Zhang, Z., Ersoz, E., Lai, C., Todhunter, R., Tiwari, H., Gore, M., et al. (2010). Mixed linear model approach adapted for genome-wide association studies. Nat. Genet. 42, 355–360. doi:10.1038/ng.546
Keywords: maize, leaf angle, genome-wide association study, meta-analysis, ideal plant architecture
Citation: Duan H, Li J, Sun Y, Xiong X, Sun L, Li W, Gao J, Li N, Zhang J, Cui J, Fu Z, Zhang X and Tang J (2022) Candidate loci for leaf angle in maize revealed by a combination of genome-wide association study and meta-analysis. Front. Genet. 13:1004211. doi: 10.3389/fgene.2022.1004211
Received: 27 July 2022; Accepted: 28 October 2022;
Published: 11 November 2022.
Edited by:
Dwijesh Chandra Mishra, Indian Agricultural Statistics Research Institute (ICAR) IndiaReviewed by:
Xiaoli Fan, Chengdu Institute of Biology (CAS), ChinaCopyright © 2022 Duan, Li, Sun, Xiong, Sun, Li, Gao, Li, Zhang, Cui, Fu, Zhang and Tang. This is an open-access article distributed under the terms of the Creative Commons Attribution License (CC BY). The use, distribution or reproduction in other forums is permitted, provided the original author(s) and the copyright owner(s) are credited and that the original publication in this journal is cited, in accordance with accepted academic practice. No use, distribution or reproduction is permitted which does not comply with these terms.
*Correspondence: Xuehai Zhang, eHVlaGFpODVAMTI2LmNvbQ==; Jihua Tang, dGFuZ2ppaHVhMUAxNjMuY29t
Disclaimer: All claims expressed in this article are solely those of the authors and do not necessarily represent those of their affiliated organizations, or those of the publisher, the editors and the reviewers. Any product that may be evaluated in this article or claim that may be made by its manufacturer is not guaranteed or endorsed by the publisher.
Research integrity at Frontiers
Learn more about the work of our research integrity team to safeguard the quality of each article we publish.