- 1Department of Pharmacy, Sichuan Academy of Medical Science and Sichuan Provincial People’s Hospital, School of Medicine, University of Electronic Science and Technology of China, Chengdu, China
- 2Personalized Drug Therapy Key Laboratory of Sichuan Province, Sichuan Academy of Medical Sciences and Sichuan Provincial People’s Hospital, Chengdu, China
- 3Department of Pharmacy, Sichuan Academy of Medical Sciences and Sichuan Provincial People’s Hospital, Chengdu, China
- 4Department of Pharmacy, Gansu Provincial Hospital of TCM, Lanzhou, China
- 5Central Sterile Supply Department, Sichuan Academy of Medical Sciences and Sichuan Provincial People’s Hospital, Chengdu, China
Background: Dolichyl-diphosphooligosaccharide–protein glycosyltransferase non-catalytic subunit (DDOST) is an important enzyme in the process of high-mannose oligosaccharide transferring in cells. Increasing DDOST expression is associated with impairing liver function and the increase of hepatic fibrosis degrees, hence exacerbating the liver injury. However, the relation between DDOST and hepatocellular carcinoma (HCC) has not been revealed yet.
Method: In this study, we evaluated the prognostic value of DDOST in HCC based on data from The Cancer Genome Atlas (TCGA) database. The relationship between DDOST expression and clinical-pathologic features was evaluated by logistic regression, the Wilcoxon signed-rank test, and Kruskal–Wallis test. Prognosis-related factors of HCC including DDOST were evaluated by univariate and multivariate Cox regression and the Kaplan–Meier method. DDOST-related key pathways were identified by gene set enrichment analysis (GSEA). The correlations between DDOST and cancer immune infiltrates were investigated by the single-sample gene set enrichment analysis (ssGSEA) of TCGA data.
Results: High DDOST expression was associated with poorer overall survival and disease-specific survival of HCC patients. GSEA suggested that DDOST is closely correlated with cell cycle and immune response via the PPAR signaling pathway. ssGSEA indicated that DDOST expression was positively correlated with the infiltrating levels of Th2 cells and negatively correlated with the infiltration levels of cytotoxic cells.
Conclusion: All those findings indicated that DDOST was correlated with prognosis and immune infiltration in HCC.
Introduction
As the principal histologic type of liver cancer, hepatocellular carcinoma (HCC) ranks third among the leading cause of cancer-related mortalities (Forner et al., 2018). It has been reported that the highest incidence rates of HCC cases occur in Asia and Africa (Petrick et al., 2020), where the exposure to chronic hepatitis B is the main risk factor (Howell et al., 2021). Therapeutic options for the treatment of HCC have substantially evolved over the past 10 years. Nowadays, patients diagnosed with HCC at any stage of the disease can benefit from effective treatment, which greatly improves their survival rate. However, there are still several areas that need urgent improvement. The molecular mechanisms underlying tumorigenesis and the progression of HCC remain poorly understood (Zhao et al., 2019). At present, serum alpha-fetoprotein (AFP), ultrasonography, and CT scanning are still important means for the early diagnosis of HCC; however, the misdiagnosis rate is high (Kelley et al., 2020; Singal et al., 2020). Despite intensive research, the 5-year survival rate of HCC is still as low as <12% due to the lack of early detection strategy and effective therapy (Hlady et al., 2019). As a result, the investigation of effective prognostic biomarkers is a pivotal area among several considerations within the research of HCC.
DDOST encodes dolichyl-diphosphooligosaccharide–protein glycosyltransferase non-catalytic subunit that forms oligosaccharyltransferase (OST) complex, which catalyzes high-mannose oligosaccharides transferring to asparagine residues on nascent polypeptides in the lumen of the rough endoplasmic reticulum (ER) (Yamagata et al., 1997). A short cytosolic tail of DDOST has a functional ER-retention di-lysine motif, serving as a mechanism for retaining OST in the ER (Fu and Kreibich, 2000). DDOST also played a role in the processing of advanced glycation end products (AGEs), which are formed from non-enzymatic reactions between lipids or protein and sugars and are associated with aging and many diseases including the congenital disorders of glycosylation (Jones et al., 2012; Zhuang et al., 2017). AGEs and their receptor had been proven to be upregulated in liver fibrosis, and the silencing receptor of AGEs reduced collagen deposition and the tumor growth of HCC (Hollenbach, 2017). A previous study also revealed that the increased expression of DDOST was significantly associated with poorer clinical outcomes in cutaneous squamous cell carcinoma (Shapanis et al., 2021). Based on the those research, DDOST may play an important role in HCC. However, the prognostic potential of DDOST for HCC has not been reported.
Materials and Methods
Data Acquisition and Preprocessing
We utilized The Cancer Genome Atlas (TCGA) database (https://portal.gdc.cancer.gov/) for liver hepatocellular carcinoma (LIHC) to obtain the RNA-Seq data of 374 HCC patients accompanied with 50 normal tissues on gene expression, immune system infiltrates, and related patients’ clinical information (Blum et al., 2018). Then, we transferred RNAseq data in FPKM format to TPM format, retained clinical data and RNAseq data, and further analyzed all data in accordance with the publication guidelines provided by TCGA (https://www.cancer.gov/about-nci/organization/ccg/research/structural-genomics/tcga/using-tcga).
Differentially Expressed Gene Analysis
The expression data (HTseq-Counts) were divided into high and low expression groups according to the median DDOST expression level and was then further analyzed by unpaired Student’s t-test within the DESeq2 R package (3.6.3) (Love et al., 2014). Adjusted p <0.05 and |log2-fold change (FC)| >1.5 were considered as thresholds for the DEGs.
Enrichment Analysis
Gene ontology (GO) functional enrichment analysis and gene set enrichment analysis (GSEA) were all performed by ClusterProfiler package in R (3.6.3) (Yu et al., 2012). The DEGs between the high and low expression levels of DDOST were selected to be analyzed. GO analysis includes cellular component (CC), molecular function (MF), and biological process (BP). GSEA is a computational method to determine whether an a priori defined set of genes has statistical significance and concordant differences in two biological states. Additionally, the normalized enrichment score (NES) and adjusted p-value were utilized to sort the enriched pathways in each phenotype (Subramanian et al., 2005). C2. Cp.v7.2. symbols.gmt [Curated] was selected as the reference gene set of the KEGG pathway, C5. All.v7.2. symbols.gmt [Gene ontology] was selected as the reference gene set of GO term. Gene sets with a false discovery rate (FDR) <0.25 and adjusted p <0.05 were considered significantly enriched.
Immune Infiltration Analysis
ssGSEA was realized by the GSVA package (Hänzelmann et al., 2013) in R to investigate the correlation between DDOST and the signature genes of 24 types of immune cells and then systematically analyzed the immune infiltrates of DDOST in the published literature (Bindea et al., 2013). The infiltration of immunocytes between the DDOST high and low expression group was analyzed by Spearman correlation and the Wilcoxon rank-sum test.
Protein–Protein Interaction Network
The protein–protein interaction (PPI) network of co-regulated DEGs and the functional interaction between proteins were analyzed by the Search Tool for the Retrieval of Interacting Genes database (http://string-db.org) (Szklarczyk et al., 2019) and visualized by Cytoscape software (version 3.7.2). The combined score threshold of interaction in our study was 0.7. The database has a comprehensive score for each pair of protein relationships distributed between 0 and 1; the higher the total score, the more reliable the PPI relationship.
Validation Analysis
The different DDOST expressions between HCC and non-tumor tissue was also analyzed in three RNAseq datasets (GSE87630, GSE101685, and GSE60502), which were downloaded from the GEO database (http://www.ncbi.nlm.nih.gov/geo).
The Kaplan–Meier (K-M) plotter is capable to assess the effect of 54 k genes on survival in 21 cancer types (http://kmplot.com/analysis/index.php?p=service&cancer=liver_rnaseq). The sources for the databases include GEO, EGA, and TCGA. The primary purpose of the tool is a meta-analysis-based discovery and validation of survival biomarkers (Menyhárt et al., 2018). DDOST was inputted in the K-M plotter to analyze the relationship between the expression of DDOST and the survival days of HCC patients, which were visualized in K-M survival plots. The log rank p-value <0.05 was considered statistically significant.
Statistical Analysis
The statistical data acquired from TCGA were processed by R 3.6.3. The expression levels of DDOST between HCC and the normal group were compared by Wilcoxon rank-sum test and Wilcoxon signed-rank test. The correlation between DDOST expression and the grade of clinicopathological factors was analyzed by Welch one-way ANOVA, followed by the Bonferroni correction or t-test. The effect of the clinicopathological factors on DDOST expression was analyzed by univariate logistic regression, the Fisher exact test, and normal and adjusted Pearson κ2 tests. Moreover, we combined univariate Cox regression analysis and multivariate Cox regression analysis to evaluate the prognostic value of DDOST expression and other clinicopathological factors on overall survival (OS). All variables in the univariate analysis were put into the multivariate analysis. The K-M curve was drawn to evaluate the prognostic value of DDOST. The hazard risk (HR) of the individual for OS and disease-specific survival (DSS) were estimated by univariate Cox proportional hazard regressions. The HR of individual factors was estimated by measuring the HR with a 95% confidence interval (CI).
The receiver operating characteristic (ROC) analysis of DDOST was realized by the pROC package (Robin et al., 2011). The calculated area under the curve (AUC) value ranges, which were from 0.5 to 1.0, indicated the discrimination ability of 50%–100%. The time-dependent analysis of the ROC curve was constructed to evaluate DDOST for predicting the HCC outcome at 1, 3, and 5 years. All statistical tests were considered significant when two-tailed p ≤0.05.
Result
Clinical Characteristics
The clinical data of 374 HCC patients included the patients’ age, gender, T stage, N stage, M stage, pathologic stage, gender, age, histologic grade, vascular invasion, OS event, BMI, and AFP (ng/ml) (Table 1). A total of 253 males and 121 females were analyzed in the present study. The Fisher’s exact test result showed that DDOST was significantly correlated with OS event (p = 0.013); the chi-square test result revealed that DDOST had a trend of correlation with T stage (p = 0.074), histological grade (p = 0.077), and vascular invasion (p = 0.072). The Wilcoxon rank-sum test showed that DDOST was significantly correlated with AFP (ng/ml) (p < 0.001) and BMI (p = 0.031). DDOST expression was not significantly correlated with other clinicopathologic features.
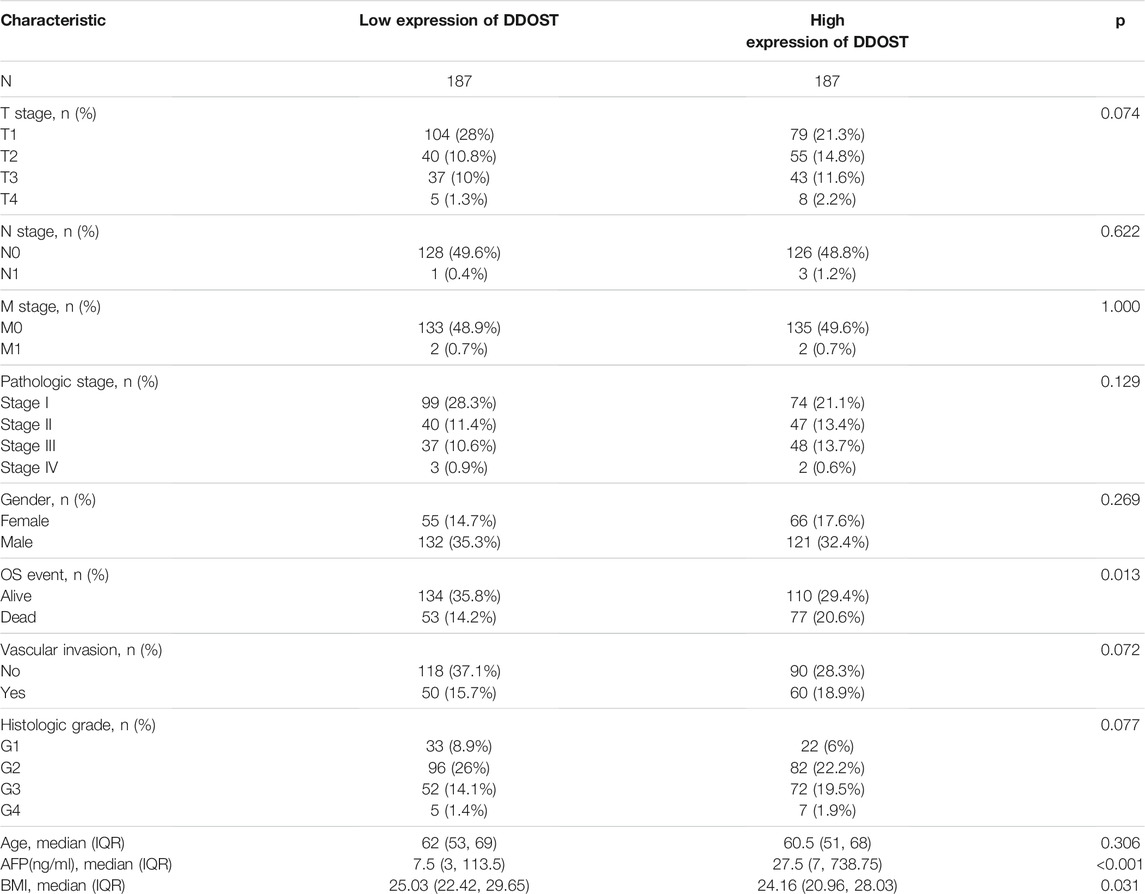
TABLE 1. Demographic and clinicopathological parameters of high and low DDOST expression group patients with hepatocellular carcinoma in TCGA-LIHC.
Differential Expression Analysis of DDOST in HCC
With |logFC| <1.5 and adjusted p < 0.05 set as the cut-off criteria, a total of 951 DEGs were identified (857 upregulated and 94 downregulated) by analyzing the HTSeq-Counts data of DDOST-related genes from TCGA. DEGs expressions were visualized in a volcano plot (Figure 1A). The unpaired and paired differential expression analyses between normal and HCC groups indicated that DDOST was expressed significantly higher in tumors compared to normal tissue (Figures 1B,C). The correlation between DDOST and 25 genes was demonstrated in a heat map (Figure 1D).
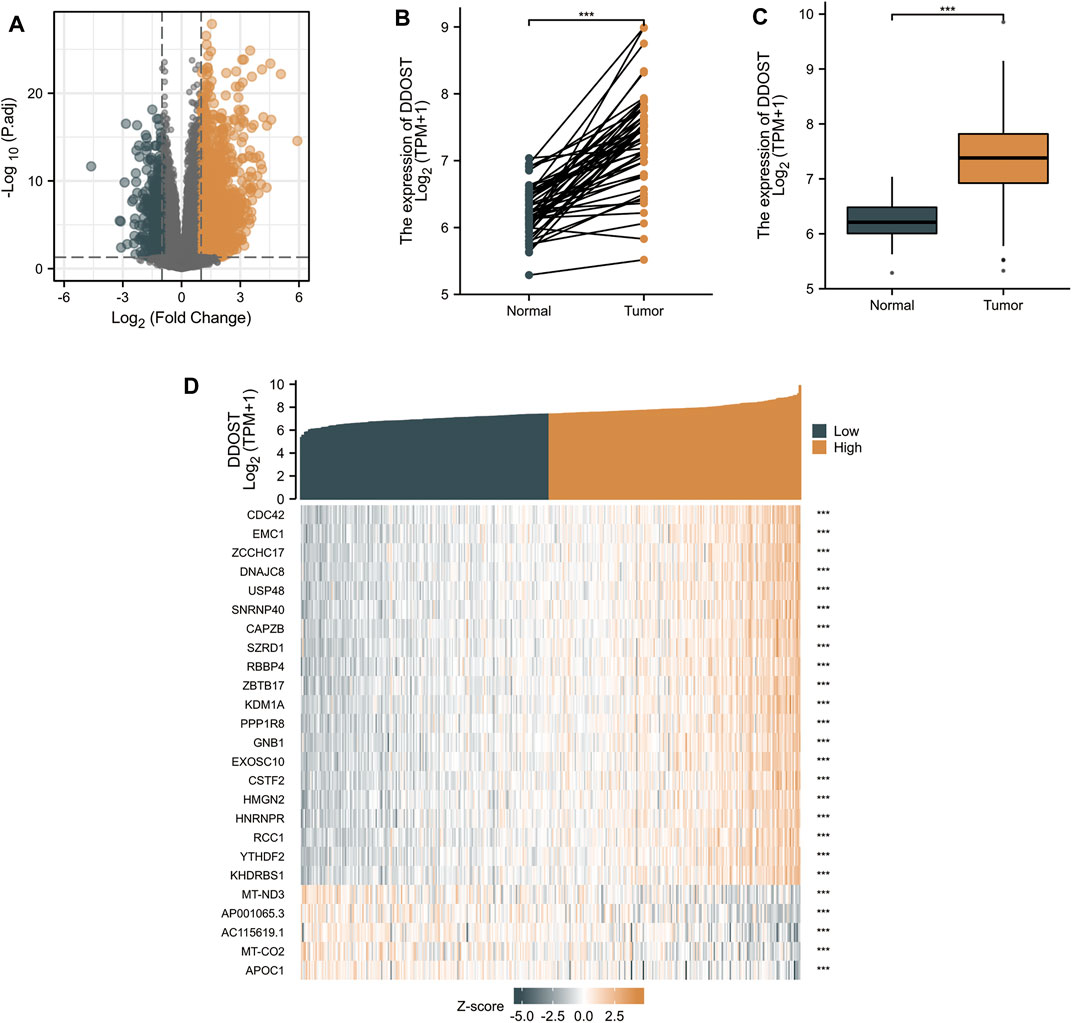
FIGURE 1. The results of differentially expressed gene (DEG) analysis. (A) The volcano plot of differentially expressed RNAs. (B,C) The different expressions of DDOST between HCC and the normal group. (D) The heat map of the 25 genes correlated to DDOST.
Functional Enrichment Analysis of DEGs
GO analysis indicated that DEG-related DDOST had significant regulation on epidermis development, skin development, epidermal cell differentiation, keratinocyte differentiation, channel activity, substrate-specific channel activity, inorganic anion transmembrane transporter activity, serine-type endopeptidase inhibitor activity, the anchored component of membrane, and cornified envelope (Figure 2A). The network of DDOST and its potential co-expression genes in DDOST-related DEGs are shown in Figure 2B.
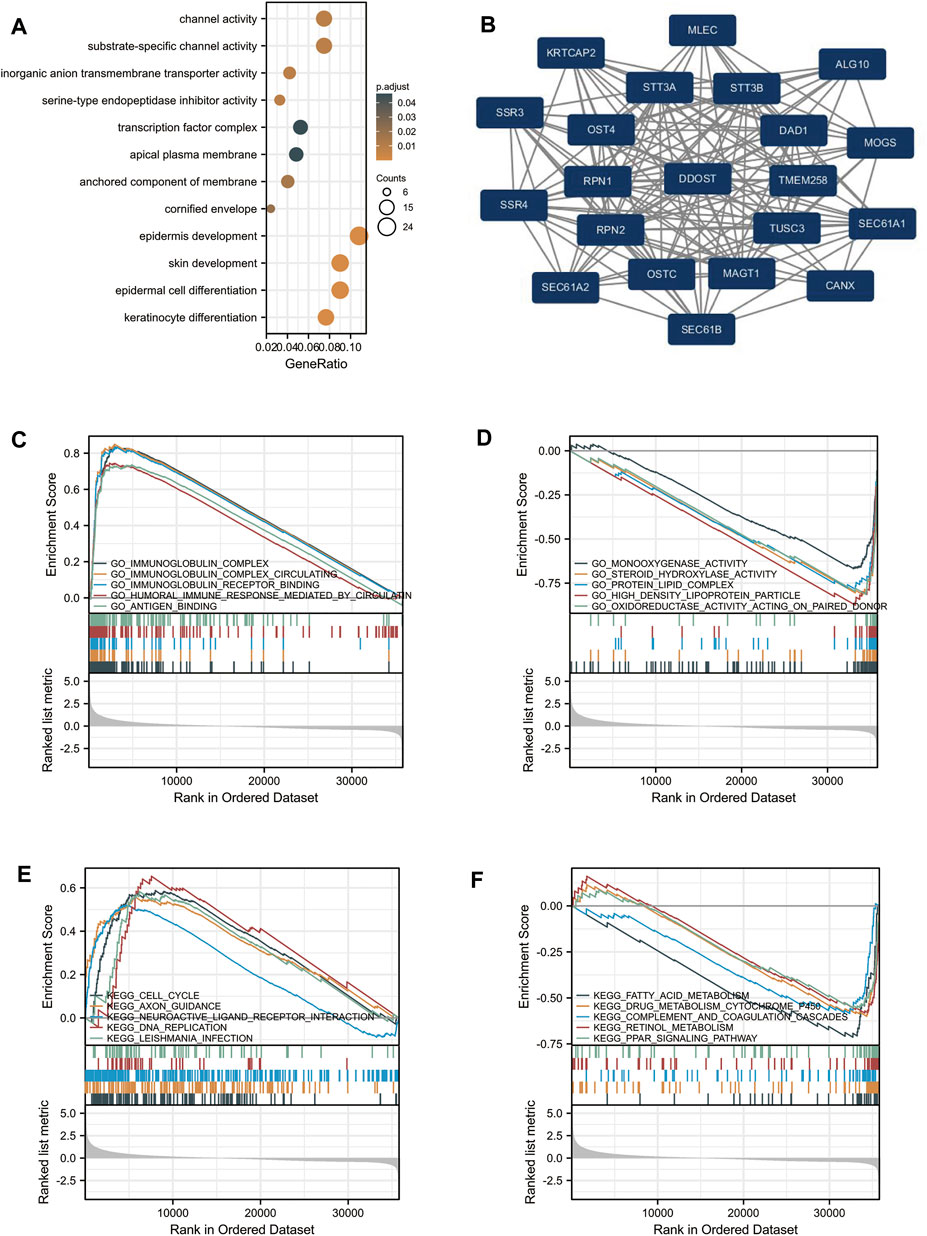
FIGURE 2. Enrichment analysis of DDOST in HCC. (A) Biological process enrichment related to DDOST-related genes. (B) A network of DDOST and its 20 potential co-interaction proteins. (C–F) The results of enrichment analysis from GSEA.
To further identify the biological function of DDOST, the GSEA of differences between low and high DDOST expression data sets were performed to identify the GO term and KEGG pathway associated with DDOST. A total of 476 pathways showed significant differences (FDR <0.05, adjusted p < 0.05) in the enrichment of GO terms and KEGG pathways in samples with a high expression of DDOST. The most significantly enriched GO term and KEGG pathway based on their NES are shown in Table 2. The GSEA analysis in GO term revealed that immunoglobulin complex, immunoglobulin complex circulating, immunoglobulin complex receptor binding, humoral immune response mediated by circulating immunoglobulin, and antigen-binding were positively correlated with high levels of DDOST (Figure 2C); monooxygenase activity, steroid hydroxylase activity, protein–lipid complex, high-density lipoprotein particle, and oxidoreductase activity on paired donors were negatively correlated with high levels of DDOST (Figure 2D). The GSEA analysis in the KEGG pathway revealed that cell cycle, axon guidance, neuroactive ligand–receptor interaction, DNA replication, and Leishmania infection were positively correlated with high levels of DDOST (Figure 2E); Fatty acid metabolism, drug metabolism cytochrome P450, complement and coagulation cascades, retinol metabolism, and the PPAR signaling pathway were negatively correlated with high levels of DDOST (Figure 2F). These results indicate that the pathways regulating immunoglobulin complex, cell cycle control, and DNA replication were strongly associated with DDOST expression.
Relationship Between DDOST Expression and Immune Infiltration
Spearman correlation was employed to study the correlation between the DDOST expression level in TPM format and the immune cell infiltration level quantified as the ssGSEA score. The Th2 cells’ infiltration level displays a significantly positive correlation with DDOST expression (Spearman R = 0.390, p < 0.001) (Figure 3A) and was significantly higher in the DDOST high-expression group (p < 0.001) (Figure 3B). On the other hand, the cytotoxic cells’ infiltration level showed a significantly negative correlation with DDOST expression (Spearman R = -0.232, p < 0.001) (Figure 3C) and was significantly lower in the DDOST high-expression group (p < 0.001) (Figure 3D). T helper cells, NK CD56bright cells, TFH, aDC, and macrophages have also shown a positive relation with DDOST. pDC, DC, CD8 T cells, Th17 cells, Tgd, neutrophils, and NK cells have shown a negative correlation with DDOST (Figure 3E). These results indicated the vital role of DDOST in the immune infiltration in HCC. Different degrees of correlation between the ratios of 24 types of different tumor-infiltrating immune cells’ subpopulations were assessed and visualized by a heat map (Figure 3F).
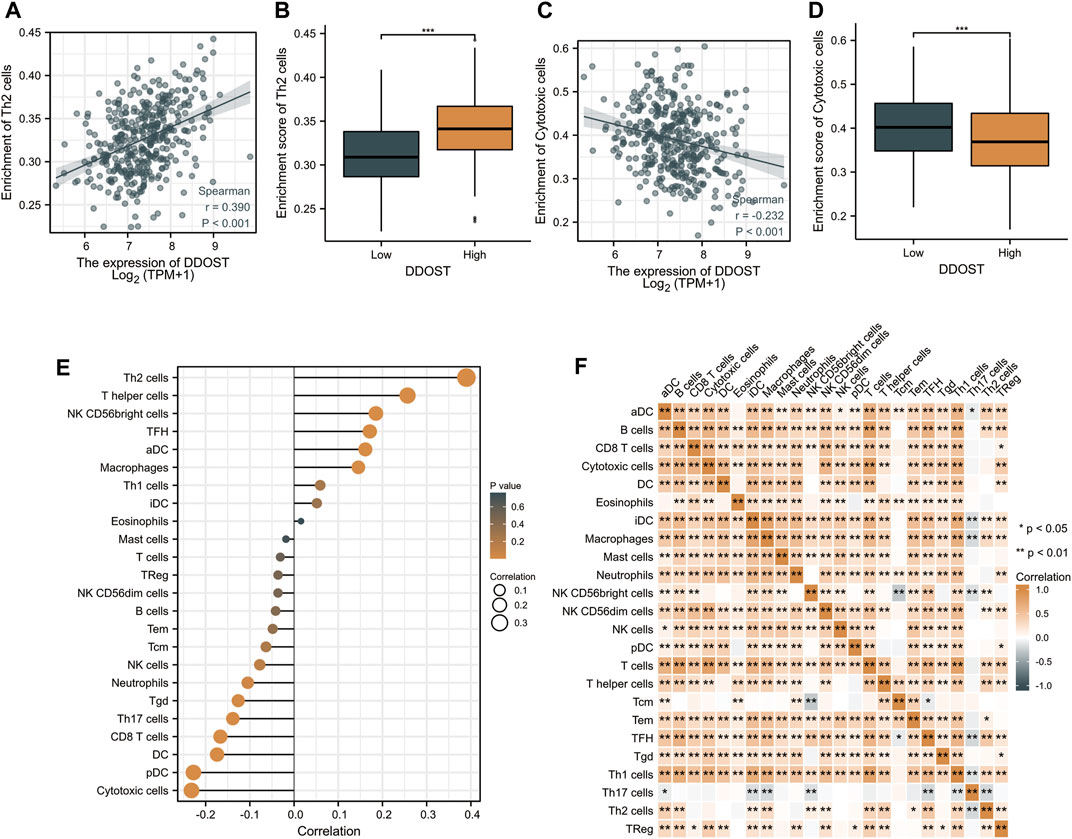
FIGURE 3. The results of analysis between DDOST expression and immune infiltration. (A) The positive correlation between DDOST expression and Th2 cells. (B) Th2 cells’ infiltration level in different DDOST expression groups. (C) The negative correlation between DDOST expression and cytotoxic cells. (D) Cytotoxic cells’ infiltration level in different DDOST expression groups. (E) Correlation between DDOST expression level and the relative abundances of 24 immune cells. (F) Heat map of 24 immune infiltration cells in HCC.
Associations Between DDOST Expression and Clinicopathologic Variables
Welch one-way ANOVA followed by the Bonferroni correction proved that the expression of DDOST was significantly correlated with the pathologic stage and T stage (Figures 4A,B). The t-test revealed that the expression of DDOST was significantly correlated with the histologic grade, vascular invasion, and OS event (Figures 4C–E). Logistic regression analysis showed that DDOST was significantly correlated with the T stage (p = 0.011) and histologic grade (p < 0.013) and had a trend of correlation with vascular invasion (p = 0.056) (Table 3).
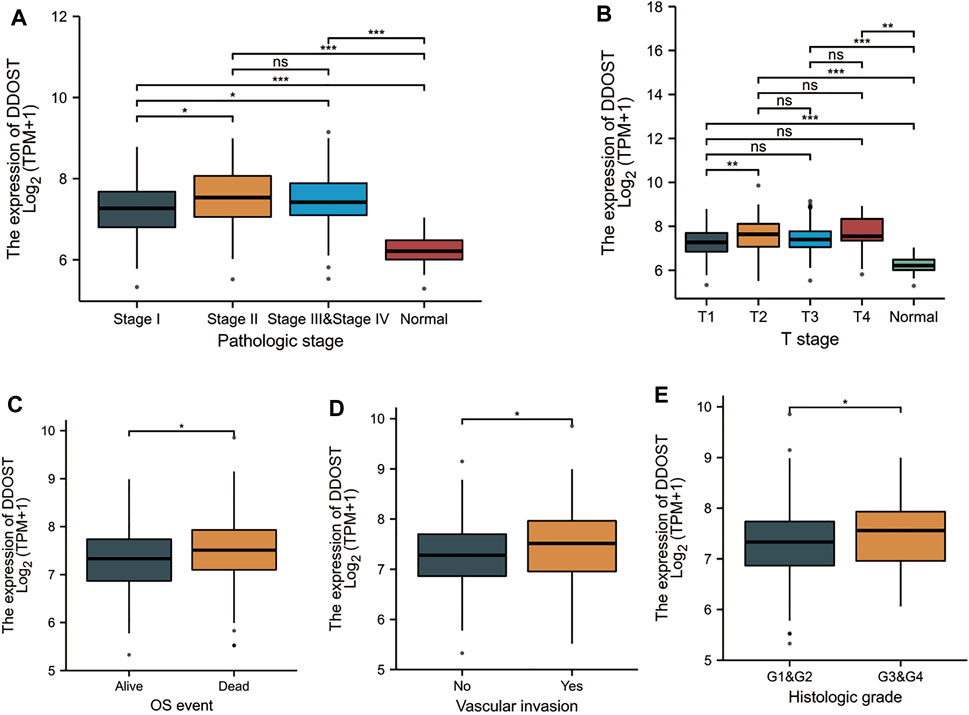
FIGURE 4. Association between the DDOST expression and different clinicopathologic characteristics. (A) Association between the DDOST expression and the pathologic stage of HCC, (B) T stage, (C) OS event, (D) vascular invasion, and (E) histologic grade.
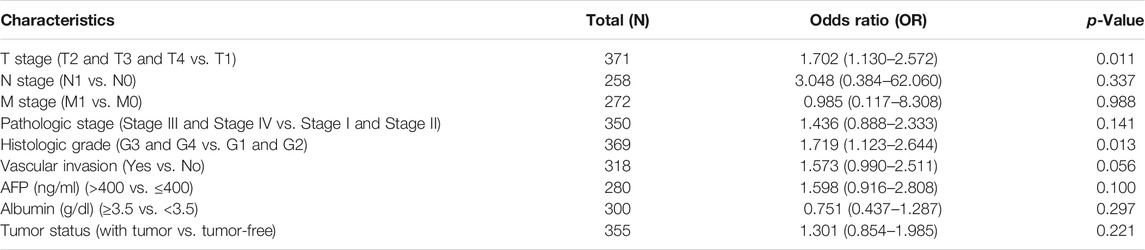
TABLE 3. DDOST expression correlated with clinicopathological characteristics analyzed by logistic regression.
In the Cox regression model, univariate Cox regression indicates that the T stage (p < 0.001), M stage (p = 0.017), pathologic stage (p < 0.001), and DDOST (p < 0.001) were correlated with the bad prognosis of HCC (Table 4). All variables in univariate Cox regression were included in multivariate Cox regression. Multivariate Cox regression showed that T stage (p = 0.017) and DDOST (p = 0.038) were independent prognostic factors for OS (Figure 5A).
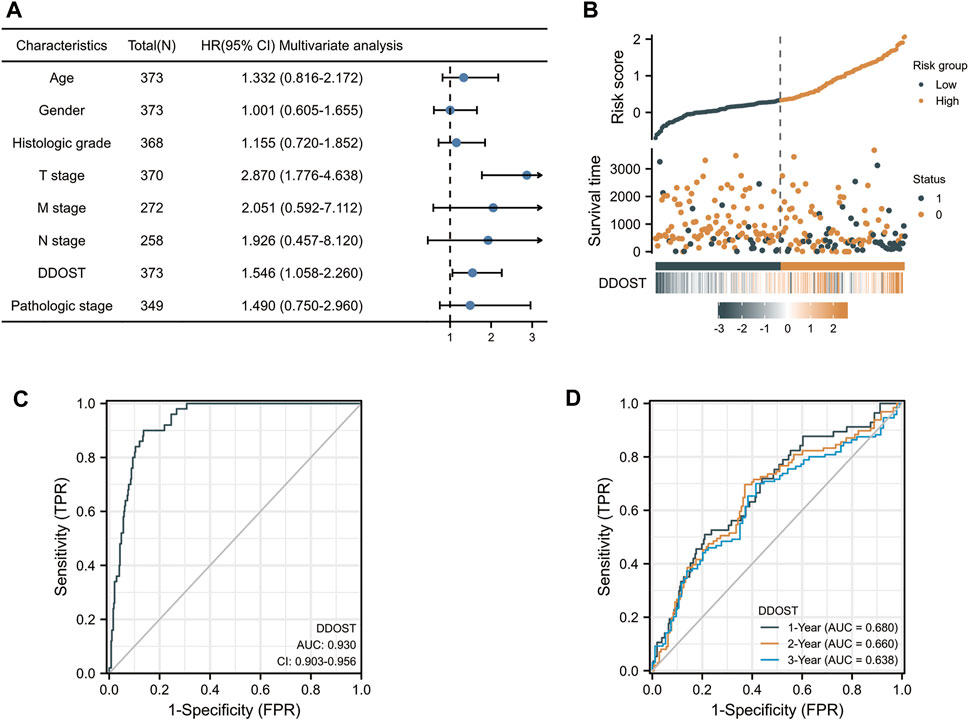
FIGURE 5. The prognostic value of DDOST in LIHC. (A) Multivariate Cox regression visualized in the forest plot (B) DDOST expression distribution and survival status. 0: dead, 1: alive. (C) Diagnostic ROC curve of DDOST. (D) Time-dependent ROC curve of DDOST.
The distribution of DDOST expression, survival status of HCC patients, and expression profiles of DDOST are shown in Figure 5B. The blue dots represent the surviving HCC patients, and the orange dots represent the dead HCC patients. The upper line represents the median of risk score. The left side of the upper line represents the low-risk score group with a low expression of DDOST, and the right side of the dotted line represents the high-risk score group with a high expression of DDOST. With the increase of risk score in HCC patients, the number of orange dots increased gradually, and the number of dead HCC patients increased. It shows that the patients in the high-risk group have poorer survival and a higher risk of death.
The ROC analysis of DDOST supported the diagnostic accuracy of the score (AUC = 0.93, 95% CI: 0.903–0.956) (Figure 5C). The time-dependent accuracy of DDOST in predicting OS in 1, 2, and 3 years was also assessed through a time-dependent ROC analysis (Figure 5D).
The K-M survival curve drawn by survminer package in R was used to evaluate the prognostic value of DDOST in OS of HCC. HCC patients were divided into high and low expression groups based on the DDOST expression median value. The high expression group has a strong correlation with worse OS (HR = 1.96 (1.38–2.79), p < 0.001) and DSS (HR = 1.97 (1.25–3.09), p = 0.003) (Figures 6A,B).
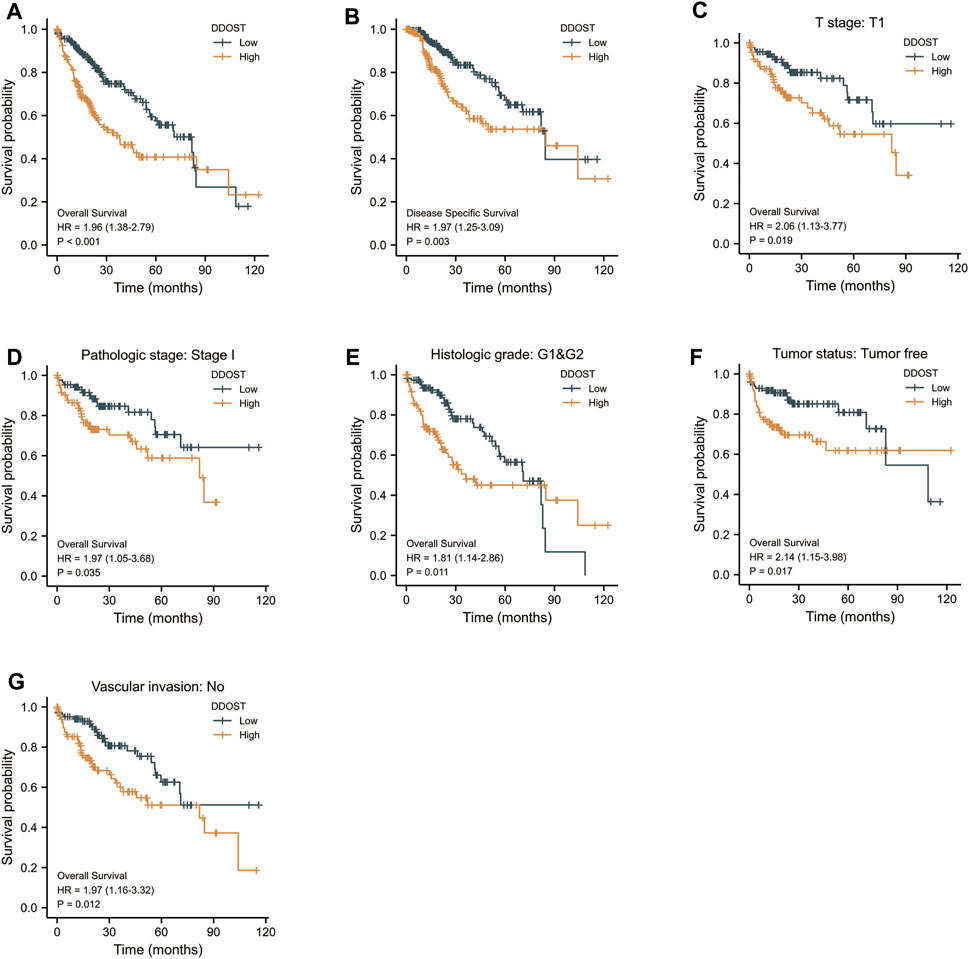
FIGURE 6. The prognostic value of DDOST in the different subgroups. (A,B) The prognostic value of DDOST in OS and DSS of HCC. (C–G) High expression of DDOST was associated with worse OS in different subgroups.
The high expression of DDOST was also associated with worse OS in the T1 subgroup of T stage (HR = 2.06 (1.13–3.77), p = 0.019), stage I subgroup of pathologic stage (HR = 1.97 (1.05–3.68), p = 0.035), G1 and G2 subgroup of histologic grade [HR = 1.81 (1.14–2.86), p = 0.011], tumor- free subgroup of tumor status (HR = 2.14 (1.15–3.98), p = 0.017), and no vascular invasion subgroup of vascular invasion (HR = 1.97 (1.16–3.32), p = 0.012) (Figures 6C–F).
Data Validation
In all three GEO datasets, DDOST mRNA expression exhibited a significant increase in HCC when compared to the normal group (p-value < 0.01, Supplement Figures 1A–C). K-M survival plots also showed the group with high DDOST expression having poor OS rates (log rank p-value = 0.010, Supplement Figure 1D).
Discussion
As far as we know, the majority of the membrane and secretory proteins synthesized in the ER are modified with N-glycans in eukaryotes. The N-glycosylation reaction catalyzed by OST had been implicated in cell-to-cell communication, signal transduction, trafficking, folding, and the degradation of proteins (Ohtsubo and Marth, 2006; Harada et al., 2015; Mikolajczyk et al., 2020) and was involved in the mechanism of tumor immune escape in the tumor microenvironment (Hsu et al., 2018). Thus, OST can be a potential therapeutic target for cancer treatment (Harada et al., 2019). RPN2, TUSC, as well as DDOST are the subunits of OST. The expression of RPN2 is positively correlated with the progression of breast cancers (Ono et al., 2015), non-small cell lung (Fujita et al., 2015), gastric (Fujimoto et al., 2018), esophageal (Li et al., 2019), and colorectal cancers (Bi and Jiang, 2018), whereas TUSC3 was reported as a candidate tumor suppressor (Vašíčková et al., 2018). DDOST, which also acted as advanced glycation end product-receptor 1 (Li et al., 1996), had been reported in regulating AGE, which increased oxidative stress and inflammation and may be involved in liver injury and subsequent carcinogenesis (Moy et al., 2013). In this research, we analyzed the sequencing data on liver cancer patients from TCGA to study the potential function and evaluate the prognostic value of DDOST.
DDOST is highly expressed in HCC patients and correlated with several advanced clinical features (pathological stage, T stage histologic grade, vascular invasion, OS event), which suggested that DDOST is a potential prognostic and diagnostic marker deserving further clinical validation. The function of DDOST in HCC was further investigated in GSEA using TCGA data.
The PPI network indicated that DDOST can interact with SSR3, SSR4, SEC61A1, SEC61A2, SEC61B, CANX, MOGS, ALG10, and MLEC other than the subunit protein of OST. All those proteins were closely associated with the N-linked oligosaccharide processing pathway, which had long been considered directly associated with the metastatic potential of malignant tumor cells (Dennis, 1991). GSEA showed that positively enriched GO terms including immunoglobulin complex, immunoglobulin complex circulating, immunoglobulin complex receptor binding, humoral immune response mediated by circulating immunoglobulin, and antigen-binding were pivotal in immune complex formation. It is indicated that DDOST might participate in the immune response in the tumorigenesis of HCCs. On the other hand, the KEGG pathway analysis indicated that cancer-related pathways including cell cycle and DNA replication were positively enriched when DDOST was highly expressed. Furthermore, GSEA analysis revealed the vital role of DDOST in the metabolism of protein and fatty acids, and in the downregulation of the PPAR signaling pathway, which plays a vital role in protecting the liver from oxidation, inflammation, fibrosis, and tumors (Wu et al., 2020). Based on the results, we can presume that as a crucial molecular in regulating protein and lipid metabolism, the high DDOST expression may induce an immune response and regulate cell cycles in HCC by suppressing the PPAR pathway.
ssGSEA combined with Spearman correlation was adopted to investigate the relationship between DDOST expression and immune infiltration levels in HCC. Our results demonstrate that DDOST expression has a significantly positive correlation with Th2 cells and a strong-to-moderate correlation with T helper cells, NK CD56 bright cells, Tfh, aDC, macrophages, and Th1 cells. Our results indicate that a shift of Th1/Th2 balance toward Th2, which plays a vital role in HCC metastasis (Budhu and Wang, 2006), may be caused by the DDOST high expression. Th2 cell is one type of T helper cell that can induce the polarization of M1 macrophages into immunosuppressive M2 macrophages (DeNardo et al., 2009), and lead to the inhibition of the host immune system, hence contributing to tumorigenesis. IL-4 produced by Th2 cells can result in the activation of several cancer-related pathways (Zhao et al., 2015; Dey et al., 2020). Tfh cells can differentiate into Th1 and Th2 cells and regulate humoral immune response (Rezende et al., 2018). Based on previous research, we can conclude that overexpression of DDOST may induce immune infiltration in HCC genesis and progression.
There is an inverse correlation between cytotoxic cells, pDC, DC, CD8 T cells, Th17 cells, Tgd, neutrophils, NK cells, and DDOST. Cytotoxic cells including NK cells play a vital role in anti-tumor immunity. NK cells are important in innate immune surveillance against cancer (Lanier, 2005). CD8+ T cells exhibit a cytotoxic ability against tumor cells through differentiating cytotoxic T cells (Iwahori, 2020). DCs including pDC were essential contributors to immune defenses against cancer. IFN-I produced by pDC shows good anti-tumor activity (Saulep-Easton et al., 2014). Th17 cells were closely related to neutrophils (Amicarella et al., 2017), and they are critical in tumor immunity and predict a poor prognosis in HCC (Wang et al., 2020). The downregulation of those types of immune cells may facilitate the progression of HCC. All findings according to ssGSEA exhibited the important role of DDOST in regulating immune infiltration in HCC.
As a traditional serological marker, AFP has been adopted in the diagnosis of HCC for decades (Wong et al., 2014). However, AFP was not significant in all HCC cases. It is reported that only 60%–70% of total HCC patients have elevated AFP levels, and nonspecific increases are also observed in non-HCC diseases such as chronic hepatitis or liver cirrhosis (Akeyama et al., 1972; Di Bisceglie and Hoofnagle, 1989). More importantly, AFP levels are usually normal in early HCC (Chen et al., 1984). On the contrary, DDOST is highly expressed in early-stage HCC. Furthermore, compared with the DDOST low-level expression group, HCC patients with DDOST highly expressed the result in poor OS and DSS. The Cox HR model also suggested that DDOST was strongly associated with the OS in HCC. The relation between DDOST and those prognostic indicators suggested that DDOST was a powerful prognostic biomarker in HCC.
Overall, the important role of DDOST in HCC was revealed through our study. Our work demonstrated that increased expression of DDOST is associated with poor OS in HCC patients. GSEA showed that pathways including DNA replication, cell cycle, immune response in cancer, the PPAR signaling pathway, and lipid acid metabolism were associated with DDOST expression. Moreover, the connection between DDOST and tumor-infiltrating immune cells was identified. The work presented here provides a detailed analysis of the role of DDOST in HCC development, which will aid in the understanding of the mechanisms underlying HCC. Combined with a previous study, our research indicates that DDOST can affect protein and lipid metabolism by joining in nascent polypeptide processing in ER and regulating the AGE level; then, it may lead to PPAR pathway suppression and play an important role in cell cycle regulation and immune infiltration in HCC.
Although the vital role of DDOST in the regulation of the cell cycle and immune response in the tumorigenesis of HCC had been proven, in vitro and in vivo experiments are still needed to verify the correlation between DDOST expression and HCC development and then to illustrate the biological mechanism of DDOST in HCC progression. Clinical researches are required to evaluate the relationship between DDOST expression and clinical features including the HCC stage, and prognosis value, which might facilitate the identification of new markers for assessing the tumor progression, promoting drug development, and improving treatment strategy.
Data Availability Statement
The datasets presented in this study can be found in online repositories. The names of the repository/repositories and accession number(s) can be found in the article/Supplementary Material.
Author Contributions
CZ and RT conceived and designed the study. CZ, XJ, and HX did the literature review and conceptualization. CZ and JG analyzed the data and wrote the original draft. All authors read and approved the final manuscript.
Funding
We are grateful for support of Key Research and Development Program of Science and Technology Department of Sichuan Province (2019YFS0514) and National Key R and D Program of China (2020YFC2005500).
Conflict of Interest
The authors declare that the research was conducted in the absence of any commercial or financial relationships that could be construed as a potential conflict of interest.
Publisher’s Note
All claims expressed in this article are solely those of the authors and do not necessarily represent those of their affiliated organizations, or those of the publisher, the editors and the reviewers. Any product that may be evaluated in this article, or claim that may be made by its manufacturer, is not guaranteed or endorsed by the publisher.
Supplementary Material
The Supplementary Material for this article can be found online at: https://www.frontiersin.org/articles/10.3389/fgene.2021.819520/full#supplementary-material
References
Akeyama, T., Koyama, T., and Kamada, T. (1972). Alpha-fetoprotein in Acute Viral Hepatitis. N. Engl. J. Med. 287, 989. doi:10.1056/nejm197211092871923
Amicarella, F., Muraro, M. G., Hirt, C., Cremonesi, E., Padovan, E., Mele, V., et al. (2017). Dual Role of Tumour-Infiltrating T Helper 17 Cells in Human Colorectal Cancer. Gut 66, 692–704. doi:10.1136/gutjnl-2015-310016
Bi, C., and Jiang, B. (2018). Downregulation of RPN2 Induces Apoptosis and Inhibits Migration and Invasion in colon Carcinoma. Oncol. Rep. 40, 283–293. doi:10.3892/or.2018.6434
Bindea, G., Mlecnik, B., Tosolini, M., Kirilovsky, A., Waldner, M., Obenauf, A. C., et al. (2013). Spatiotemporal Dynamics of Intratumoral Immune Cells Reveal the Immune Landscape in Human Cancer. Immunity 39, 782–795. doi:10.1016/j.immuni.2013.10.003
Blum, A., Wang, P., and Zenklusen, J. C. (2018). SnapShot: TCGA-Analyzed Tumors. Cell 173, 530. doi:10.1016/j.cell.2018.03.059
Budhu, A., and Wang, X. (2006). The Role of Cytokines in Hepatocellular Carcinoma. J. Leukoc. Biol. 80, 1197–1213. doi:10.1189/jlb.0506297
Chen, D.-S., Sung, J.-L., Shed, J.-C., Lai, M.-Y., How, S.-W., Hsu, H.-C., et al. (1984). Serum α-Fetoprotein in the Early Stage of Human Hepatocellular Carcinoma. Gastroenterology 86, 1404–1409. doi:10.1016/s0016-5085(84)80151-1
DeNardo, D. G., Barreto, J. B., Andreu, P., Vasquez, L., Tawfik, D., Kolhatkar, N., et al. (2009). CD4+ T Cells Regulate Pulmonary Metastasis of Mammary Carcinomas by Enhancing Protumor Properties of Macrophages. Cancer cell 16, 91–102. doi:10.1016/j.ccr.2009.06.018
Dennis, J. W. (1991). N-linked Oligosaccharide Processing and Tumor Cell Biology. Semin. Cancer Biol. 2, 411–420.
Dey, P., Li, J., Zhang, J., Chaurasiya, S., Strom, A., Wang, H., et al. (2020). Oncogenic KRAS-Driven Metabolic Reprogramming in Pancreatic Cancer Cells Utilizes Cytokines from the Tumor Microenvironment. Cancer Discov. 10, 608–625. doi:10.1158/2159-8290.CD-19-0297
Di Bisceglie, A. M., and Hoofnagle, J. H. (1989). Elevations in Serum Alpha-Fetoprotein Levels in Patients with Chronic Hepatitis B. Cancer 64, 2117–2120. doi:10.1002/1097-0142(19891115)64:10<2117:aid-cncr2820641024>3.0.co;2-7
Forner, A., Reig, M., and Bruix, J. (2018). Hepatocellular Carcinoma. Lancet 391, 1301–1314. doi:10.1016/S0140-6736(18)30010-2
Fu, J., and Kreibich, G. (2000). Retention of Subunits of the Oligosaccharyltransferase Complex in the Endoplasmic Reticulum. J. Biol. Chem. 275, 3984–3990. doi:10.1074/jbc.275.6.3984
Fujimoto, D., Goi, T., Koneri, K., and Hirono, Y. (2018). RPN2 Is Effective Biomarker to Predict the Outcome of Combined Chemotherapy Docetaxel and Cisplatin for Advanced Gastric Cancer. Oncotarget 9, 15208–15218. doi:10.18632/oncotarget.24622
Fujita, Y., Yagishita, S., Takeshita, F., Yamamoto, Y., Kuwano, K., and Ochiya, T. (2015). Prognostic and Therapeutic Impact of RPN2-Mediated Tumor Malignancy in Non-small-cell Lung Cancer. Oncotarget 6, 3335–3345. doi:10.18632/oncotarget.2793
Hänzelmann, S., Castelo, R., and Guinney, J. (2013). GSVA: Gene Set Variation Analysis for Microarray and RNA-Seq Data. BMC Bioinformatics 14, 7. doi:10.1186/1471-2105-14-7
Harada, Y., Hirayama, H., and Suzuki, T. (2015). Generation and Degradation of Free Asparagine-Linked Glycans. Cell. Mol. Life Sci. 72, 2509–2533. doi:10.1007/s00018-015-1881-7
Harada, Y., Ohkawa, Y., Kizuka, Y., and Taniguchi, N. (2019). Oligosaccharyltransferase: A Gatekeeper of Health and Tumor Progression. Int. J. Mol. Sci. 20, 6074. doi:10.3390/ijms20236074
Hlady, R. A., Sathyanarayan, A., Thompson, J. J., Zhou, D., Wu, Q., Pham, K., et al. (2019). Integrating the Epigenome to Identify Drivers of Hepatocellular Carcinoma. Hepatology 69, 639–652. doi:10.1002/hep.30211
Hollenbach, M. (2017). The Role of Glyoxalase-I (Glo-I), Advanced Glycation Endproducts (AGEs), and Their Receptor (RAGE) in Chronic Liver Disease and Hepatocellular Carcinoma (HCC). Int. J. Mol. Sci. 18, 2466. doi:10.3390/ijms18112466
Howell, J., Pedrana, A., Schroeder, S. E., Scott, N., Aufegger, L., Atun, R., et al. (2021). A Global Investment Framework for the Elimination of Hepatitis B. J. Hepatol. 74, 535–549. doi:10.1016/j.jhep.2020.09.013
Hsu, J.-M., Li, C.-W., Lai, Y.-J., and Hung, M.-C. (2018). Posttranslational Modifications of PD-L1 and Their Applications in Cancer Therapy. Cancer Res. 78, 6349–6353. doi:10.1158/0008-5472.CAN-18-1892
Iwahori, K. (2020). Cytotoxic CD8+ Lymphocytes in the Tumor Microenvironment. Adv. Exp. Med. Biol. 1224, 53–62. doi:10.1007/978-3-030-35723-8_4
Jones, M. A., Ng, B. G., Bhide, S., Chin, E., Rhodenizer, D., He, P., et al. (2012). DDOST Mutations Identified by Whole-Exome Sequencing Are Implicated in Congenital Disorders of Glycosylation. Am. J. Hum. Genet. 90, 363–368. doi:10.1016/j.ajhg.2011.12.024
Kelley, R. K., Meyer, T., Rimassa, L., Merle, P., Park, J.-W., Yau, T., et al. (2020). Serum Alpha-Fetoprotein Levels and Clinical Outcomes in the Phase III CELESTIAL Study of Cabozantinib versus Placebo in Patients with Advanced Hepatocellular Carcinoma. Clin. Cancer Res. 26, 4795–4804. doi:10.1158/1078-0432.CCR-19-3884
Lanier, L. L. (2005). NK Cell Recognition. Annu. Rev. Immunol. 23, 225–274. doi:10.1146/annurev.immunol.23.021704.115526
Li, Y., Huang, C., Bai, Q., and Yu, J. (2019). Ribophorin II Promotes Cell Proliferation, Migration, and Invasion in Esophageal Cancer Cells In Vitro and In Vivo. Biosci. Rep. 39, BSR20182448. doi:10.1042/BSR20182448
Li, Y. M., Mitsuhashi, T., Wojciechowicz, D., Shimizu, N., Li, J., Stitt, A., et al. (1996). Molecular Identity and Cellular Distribution of Advanced Glycation Endproduct Receptors: Relationship of P60 to OST-48 and P90 to 80K-H Membrane Proteins. Proc. Natl. Acad. Sci. 93, 11047–11052. doi:10.1073/pnas.93.20.11047
Love, M. I., Huber, W., and Anders, S. (2014). Moderated Estimation of Fold Change and Dispersion for RNA-Seq Data with DESeq2. Genome Biol. 15, 550. doi:10.1186/s13059-014-0550-8
Menyhárt, O., Nagy, Á., and Győrffy, B. (2018). Determining Consistent Prognostic Biomarkers of Overall Survival and Vascular Invasion in Hepatocellular Carcinoma. R. Soc. Open Sci. 5, 181006. doi:10.1098/rsos.181006
Mikolajczyk, K., Kaczmarek, R., and Czerwinski, M. (2020). How Glycosylation Affects Glycosylation: the Role of N-Glycans in Glycosyltransferase Activity. Glycobiology 30, 941–969. doi:10.1093/glycob/cwaa041
Moy, K. A., Jiao, L., Freedman, N. D., Weinstein, S. J., Sinha, R., Virtamo, J., et al. (2013). Soluble Receptor for Advanced Glycation End Products and Risk of Liver Cancer. Hepatology 57, 2338–2345. doi:10.1002/hep.26264
Ohtsubo, K., and Marth, J. D. (2006). Glycosylation in Cellular Mechanisms of Health and Disease. Cell 126, 855–867. doi:10.1016/j.cell.2006.08.019
Ono, M., Tsuda, H., Kobayashi, T., Takeshita, F., Takahashi, R.-U., Tamura, K., et al. (2015). The Expression and Clinical Significance of Ribophorin II (RPN2) in Human Breast Cancer. Pathol. Int. 65, 301–308. doi:10.1111/pin.12297
Petrick, J. L., Florio, A. A., Znaor, A., Ruggieri, D., Laversanne, M., Alvarez, C. S., et al. (2020). International Trends in Hepatocellular Carcinoma Incidence, 1978-2012. Int. J. Cancer 147, 317–330. doi:10.1002/ijc.32723
Rezende, R. M., Lanser, A. J., Rubino, S., Kuhn, C., Skillin, N., Moreira, T. G., et al. (2018). Γδ T Cells Control Humoral Immune Response by Inducing T Follicular Helper Cell Differentiation. Nat. Commun. 9, 3151. doi:10.1038/s41467-018-05487-9
Robin, X., Turck, N., Hainard, A., Tiberti, N., Lisacek, F., Sanchez, J.-C., et al. (2011). pROC: an Open-Source Package for R and S+ to Analyze and Compare ROC Curves. BMC Bioinformatics 12, 77. doi:10.1186/1471-2105-12-77
Saulep-Easton, D., Vincent, F. B., Le Page, M., Wei, A., Ting, S. B., Croce, C. M., et al. (2014). Cytokine-driven Loss of Plasmacytoid Dendritic Cell Function in Chronic Lymphocytic Leukemia. Leukemia 28, 2005–2015. doi:10.1038/leu.2014.105
Shapanis, A., Lai, C., Smith, S., Coltart, G., Sommerlad, M., Schofield, J., et al. (2021). Identification of Proteins Associated with Development of Metastasis from Cutaneous Squamous Cell Carcinomas (cSCCs) via Proteomic Analysis of Primary cSCCs*. Br. J. Dermatol. 184, 709–721. doi:10.1111/bjd.19485
Singal, A. G., Lampertico, P., and Nahon, P. (2020). Epidemiology and Surveillance for Hepatocellular Carcinoma: New Trends. J. Hepatol. 72, 250–261. doi:10.1016/j.jhep.2019.08.025
Subramanian, A., Tamayo, P., Mootha, V. K., Mukherjee, S., Ebert, B. L., Gillette, M. A., et al. (2005). Gene Set Enrichment Analysis: a Knowledge-Based Approach for Interpreting Genome-wide Expression Profiles. Proc. Natl. Acad. Sci. 102, 15545–15550. doi:10.1073/pnas.0506580102
Szklarczyk, D., Gable, A. L., Lyon, D., Junge, A., Wyder, S., Huerta-Cepas, J., et al. (2019). STRING V11: Protein-Protein Association Networks with Increased Coverage, Supporting Functional Discovery in Genome-wide Experimental Datasets. Nucleic Acids Res. 47, D607–D613. doi:10.1093/nar/gky1131
Vašíčková, K., Horak, P., and Vaňhara, P. (2018). TUSC3: Functional Duality of a Cancer Gene. Cel. Mol. Life Sci. 75, 849–857. doi:10.1007/s00018-017-2660-4
Wang, Y., Yao, R., Zhang, D., Chen, R., Ren, Z., and Zhang, L. (2020). Circulating Neutrophils Predict Poor Survival for HCC and Promote HCC Progression through P53 and STAT3 Signaling Pathway. J. Cancer 11, 3736–3744. doi:10.7150/jca.42953
Wong, G. L. H., Chan, H. L. Y., Tse, Y.-K., Chan, H.-Y., Tse, C.-H., Lo, A. O. S., et al. (2014). On-treatment Alpha-Fetoprotein Is a Specific Tumor Marker for Hepatocellular Carcinoma in Patients with Chronic Hepatitis B Receiving Entecavir. Hepatology 59, 986–995. doi:10.1002/hep.26739
Wu, L., Guo, C., and Wu, J. (2020). Therapeutic Potential of PPARγ Natural Agonists in Liver Diseases. J. Cel Mol. Med. 24, 2736–2748. doi:10.1111/jcmm.15028
Yamagata, T., Tsuru, T., Momoi, M. Y., Suwa, K., Nozaki, Y., Mukasa, T., et al. (1997). Genome Organization of Human 48-kDa Oligosaccharyltransferase (DDOST). Genomics 45, 535–540. doi:10.1006/geno.1997.4966
Yu, G., Wang, L.-G., Han, Y., and He, Q.-Y. (2012). clusterProfiler: an R Package for Comparing Biological Themes Among Gene Clusters. OMICS: A J. Integr. Biol. 16, 284–287. doi:10.1089/omi.2011.0118
Zhao, P., Bu, X., Wei, X., Sun, W., Xie, X., Li, C., et al. (2015). Dendritic Cell Immunotherapy Combined with Cytokine-Induced Killer Cells Promotes Skewing toward Th2 Cytokine Profile in Patients with Metastatic Non-small Cell Lung Cancer. Int. Immunopharmacol. 25, 450–456. doi:10.1016/j.intimp.2015.02.010
Zhao, X., Liu, J., Ge, S., Chen, C., Li, S., Wu, X., et al. (2019). Saikosaponin A Inhibits Breast Cancer by Regulating Th1/Th2 Balance. Front. Pharmacol. 10, 624. doi:10.3389/fphar.2019.00624
Keywords: DDOST, HCC, tumor-infiltration, prognosis, T helper cells
Citation: Zhu C, Xiao H, Jiang X, Tong R and Guan J (2022) Prognostic Biomarker DDOST and Its Correlation With Immune Infiltrates in Hepatocellular Carcinoma. Front. Genet. 12:819520. doi: 10.3389/fgene.2021.819520
Received: 21 November 2021; Accepted: 23 December 2021;
Published: 31 January 2022.
Edited by:
Luis Zapata, Institute of Cancer Research (ICR), United KingdomReviewed by:
Yi Shi, Shanghai Jiao Tong University, ChinaEleonora Lusito, San Raffaele Telethon Institute for Gene Therapy (SR-Tiget), Italy
Copyright © 2022 Zhu, Xiao, Jiang, Tong and Guan. This is an open-access article distributed under the terms of the Creative Commons Attribution License (CC BY). The use, distribution or reproduction in other forums is permitted, provided the original author(s) and the copyright owner(s) are credited and that the original publication in this journal is cited, in accordance with accepted academic practice. No use, distribution or reproduction is permitted which does not comply with these terms.
*Correspondence: Rongsheng Tong, tongrs@126.com; Jianmei Guan, 781067606@qq.com