- 1Department of General Surgery, Fuyang Hospital Affiliated to Anhui Medical Universitsy, Fuyang, China
- 2The First Clinical Medical College, Guangxi Medical University, Nanning, China
- 3Department of General Surgery, Hospital of Traditional Chinese Medicine, Baise, China
- 4College of Pharmacy, Jiangsu Ocean University, Lianyungang, China
- 5Department of Burn and Plastic Surgery, The First Affiliated Hospital of Nanjing Medical University, Nanjing, China
Background: Hepatocellular carcinoma (HCC) is a solid tumor with high recurrence rate and high mortality. It is crucial to discover available biomarkers to achieve early diagnosis and improve the prognosis. The effect of LSM4 in HCC still remains unrevealed. Our study is dedicated to exploring the expression of LSM4 in HCC, demonstrating its clinical significance and potential molecular mechanisms.
Methods: Clinical information and LSM4 expression values of HCC were obtained from Gene Expression Omnibus (GEO) and The Cancer Genome Atlas (TCGA) databases. Survival analysis and receiver operating characteristic (ROC) curve analysis were applied to evaluate the prognostic and diagnostic significance of LSM4. Calculating pooled standardized mean difference (SMD) and performing summary receiver operating characteristic (sROC) curve analysis to further determine its expression status and diagnostic significance. LSM4-related co-expressed genes (CEGs) were obtained and explored their clinical significance in HCC. LSM4-associated pathways were identified through Gene set enrichment analysis (GSEA).
Results: Up-regulated LSM4 was detected in HCC tissues (SMD = 1.56, 95% CI: 1.29–1.84) and overexpressed LSM4 had excellent distinguishing ability (AUC = 0.91, 95% CI: 0.88–0.93). LSM4 was associated with clinical stage, tumor grade, and lymph node metastasis status (p < 0.05). Survival analysis showed that high LSM4 expression was related to poor overall survival (OS) of HCC patients. Cox regression analysis suggested that high LSM4 expression may be an independent risk factor for HCC. We obtained nine up-regulated CEGs of LSM4 in HCC tissues, and six CEGs had good prognostic and diagnostic significance. GSEA analysis showed that up-regulated LSM4 was closely related to the cell cycle, cell replication, focal adhesion, and several metabolism-associated pathways, including fatty acid metabolism.
Conclusion: Overexpressed LSM4 may serve as a promising diagnostic and prognostic biomarker of HCC. Besides, LSM4 may play a synergistic effect with CEGs in promoting the growth and metastasis of HCC cells via regulating crucial pathways such as cell cycle, focal adhesion, and metabolism-associated pathways.
Introduction
As one of the most prevalent solid malignancies, hepatocellular carcinoma (HCC) is a major cause of cancer-related deaths worldwide (Nordenstedt et al., 2010). Numerous considerable improvements have been accomplished in drug therapies and non-drug therapies for HCC treatment over the past decades. However, HCC was still ranked fourth in mortality, causing over 780,000 deaths per year based on the World Health Organization’s statistics in 2018 (Bray et al., 2018). To date, alpha-fetoprotein (AFP) is one of the most common serum biomarkers used for diagnosing HCC (Liu Y et al., 2021). Despite this, due to insufficient sensitivity and specificity, AFP detection remains far from achieving satisfactory role for early HCC. (Wang and Wei, 2020). Numerous liver diseases, such as acute hepatitis and cirrhosis, can also cause elevating serum AFP levels (Xie et al., 2018). Therefore, it is vital to discover the novel effective biomarkers, which are of great significance for the early diagnosis and improvement of prognosis of HCC patients.
Smith-like (LSM) proteins are called the RNA binding protein family, with 13 members (LSM1 ∼ LSM14B). These members are closely related to tumorigenesis and metastasis of multiple tumor types, such as breast cancer (BRCA), mesothelioma and lung squamous cell carcinoma (LUSC) (Streicher et al., 2007; Watson et al., 2008; Li et al., 2020; Ta et al., 2021). Nevertheless, the biological functions of LSM gene family members in tumors are not fully understood. The small nuclear ribonucleoprotein Sm-like4 (LSM4) has been shown to be involved in the occurrence and malignant procession of BRCA, pancreatic cancer (PC), and ovarian cancer (OC) (Xue et al., 2018; Ta et al., 2021). For example, the expression of LSM4 in BRCA was significantly increased and was closely related to lymph node metastasis and tumor cell proliferation (Yin et al., 2021). In OC, LSM4 knockdown blocked cell migration and invasion of OC cells, and LSM4 overexpression reversed the inhibitory effect of circ_0,025,033 knockdown on the above process (Hou and Zhang, 2021). However, LSM4 has not been fully studied in HCC. The clinical application value and underlying molecular mechanism of LSM4 in HCC are still unclear. Therefore, we used multiple available online databases (TGCA, GEO, UALCAN, etc.) to evaluate the clinical value of LSM4 in HCC and explore its underlying mechanisms.
This study comprehensively analyzed the LSM4 expression in HCC by calculating the pooled SMD based on public datasets. Use ROC and sROC analysis to evaluate the diagnostic significance. Cox regression analysis and survival analysis were carried out to determine the correlation of LSM4 expression and the prognosis of HCC patients. GSEA analysis was used to identify LSM4-associated pathways.
Materials and Methods
Data Collection Through Online Databases
The HCC datasets used in this study were obtained from the TCGA (https://cancergenome.nih.gov/) and GEO databases (https://www.ncbi.nlm.nih.gov/geo/). The inclusion criteria we developed were as follows: 1) human origin, 2) HCC and non-HCC tissues were included, 3) the LSM4 expression data was included in the datasets. In addition, the exclusion criteria were as follows: 1) the sample size was insufficient (n < 6), 2) did not contain the expression data of LSM4, 3) the data could not be processed under the current conditions. Available datasets were collected strictly in accordance with the established inclusion and exclusion criteria.
LSM4 Expression Status in HCC and Non-HCC Tissues
R software (version 3.6.3) was used to perform an independent sample t-test on the LSM4 expression data included in our study to make the comparison on the LSM4 expression status between the non-HCC tissues and HCC tissues. The R package ggplot2 was used for visualization, and the results were presented in the form of box plots. Subsequently, based on the included GEO datasets, STATA 14.0 software was used to calculate the standard mean deviation (SMD) to detect the LSM4 expression level in HCC comprehensively. When datasets included had huge heterogeneity (I2 > 0.50), we selected the random-effects model for analysis. Publication bias was detected using Egger and Begg tests, and p-value ≥ 0.05 indicated no publication bias.
Genetic Alteration Analysis of LSM4
The online database cBioPortal for Cancer Genomics database (https://www.cbioportal.org/) could be used to explore the genetic alteration information in numerous cancers and provide visualization of the analysis results (Tang et al., 2020). We used a hepatocellular carcinoma (TCGA, PanCancer Atlas) study containing 372 samples to explore the genetic alteration and mutation types of LSM4 with this online tool.
Relationship Between Clinicopathological Characteristics and LSM4 Expression
In our current study, UALCAN (http://ualcan.path.uab.edu/) database was applied to explore the relationship between the clinicopathological characteristics and LSM4 expression in HCC.
Diagnostic Significance of LSM4 in HCC
For determining the capability of LSM4 in distinguishing between HCC tissues and non-HCC tissues, we carried out the receiver operating characteristic (ROC) curve analysis using the pROC package for analysis, and then the ROC curve was drawn with the application of the ggplot2 package (for visualization). In addition, the summary receiver operating characteristic (sROC) curve was also drawn by us to comprehensively determine the diagnostic value of LSM4 and calculated the specificity and sensitivity of LSM4 through STATA 14.0. Meanwhile, we also carried out Deeks’ test to further detect the publication bias of the study.
Prognostic Significance of LSM4 in HCC
The clinical data of HCC in the TCGA database was used to determine the relationship between the patient’s prognosis and LSM4 expression. In order to evaluate the prognosis, HCC samples with survival time and survival status are collected. Incorporate LSM4 expression data and corresponding clinical information were included in the univariate Cox regression analysis, and then incorporate the prognostic-related features selected in the above step into the multivariate Cox regression analysis to further assess the association between the patient’s prognosis and LSM4 expression. Besides, using the TCGA dataset and the GEPIA database (http://gepia.cancer-pku.cn/), the Kaplan-Meier tests were applied to compare the difference in Disease-Free Survival (DFS) and overall survival (OS) of HCC patients with different LSM4 expressions.
Identification Co-expressed Genes of LSM4 and Its Clinical Value
The cBioPortal and the GEPIA databases were used to obtain the co-expressed genes (CEGs) of LSM4 in HCC. Significantly CEGs should meet the following two criteria: 1) correlation coefficient≥0.60, 2) p-value <0.05. Finally, the intersection genes of LSM4 positively correlated CEGs obtained from the two databases were used for further analysis. Briefly, the expressions level of CEGs in HCC tissues and matched non-HCC tissues were also explored in our study. Furtherly, the survival analysis and ROC curve analysis were carried out to detect the clinical significance of CEGs in HCC.
Gene Set Enrichment Analysis
To identify the signaling pathways related to LSM4 expression in HCC, Gene Set Enrichment Analysis (GSEA) was utilized. When meeting the criteria of the false discovery rate (FDR) was less than 0.25, and the p-value was less than 0.05, the genes were considered significantly enriched in this pathway. Our current study used the clusterProfiler package and the enrichplot package on the R platform for analysis and visualization, respectively.
Result
Included Datasets and Information
We collected a total of 18 eligible datasets from GEO and TCGA databases. All specimens were liver tissues collected from HCC individuals and matched non-HCC individuals. Table 1 showed the basic information of included datasets. The mean (Mean) and standard deviation (SD) of LSM4 expression value in the HCC tissues and the matched non-HCC tissues contained in included datasets were displayed.
Up-Regulated LSM4 Expression in HCC
The LSM4 expression levels in HCC tissues and non-HCC tissues were presented in Figure 1. Compared with non-HCC tissues, LSM4 showed a significant up-regulation trend in 17 datasets (all p-value <0.05; Figure 1A–E, G–R). It was worth noting that the only dataset GSE33006 showed a significant down-regulation of LSM4 expression (p-value <0.05; Figure 1F). The heterogeneity test results showed that I2 = 68.6% (p-value <0.0001), indicating significant heterogeneity. Therefore, based on 17 GEO datasets, random-effects analysis was applied to calculate the SMD. We observed that the HCC tissues had significantly higher LSM4 expression levels than the matched non-HCC tissues (Figure 2A, SMD = 1.56, 95% CI: 1.29–1.84). The p-values of Begg and Egger tests were 0.070 and 0.424, respectively, indicating no publication bias (Figures 2B,C).
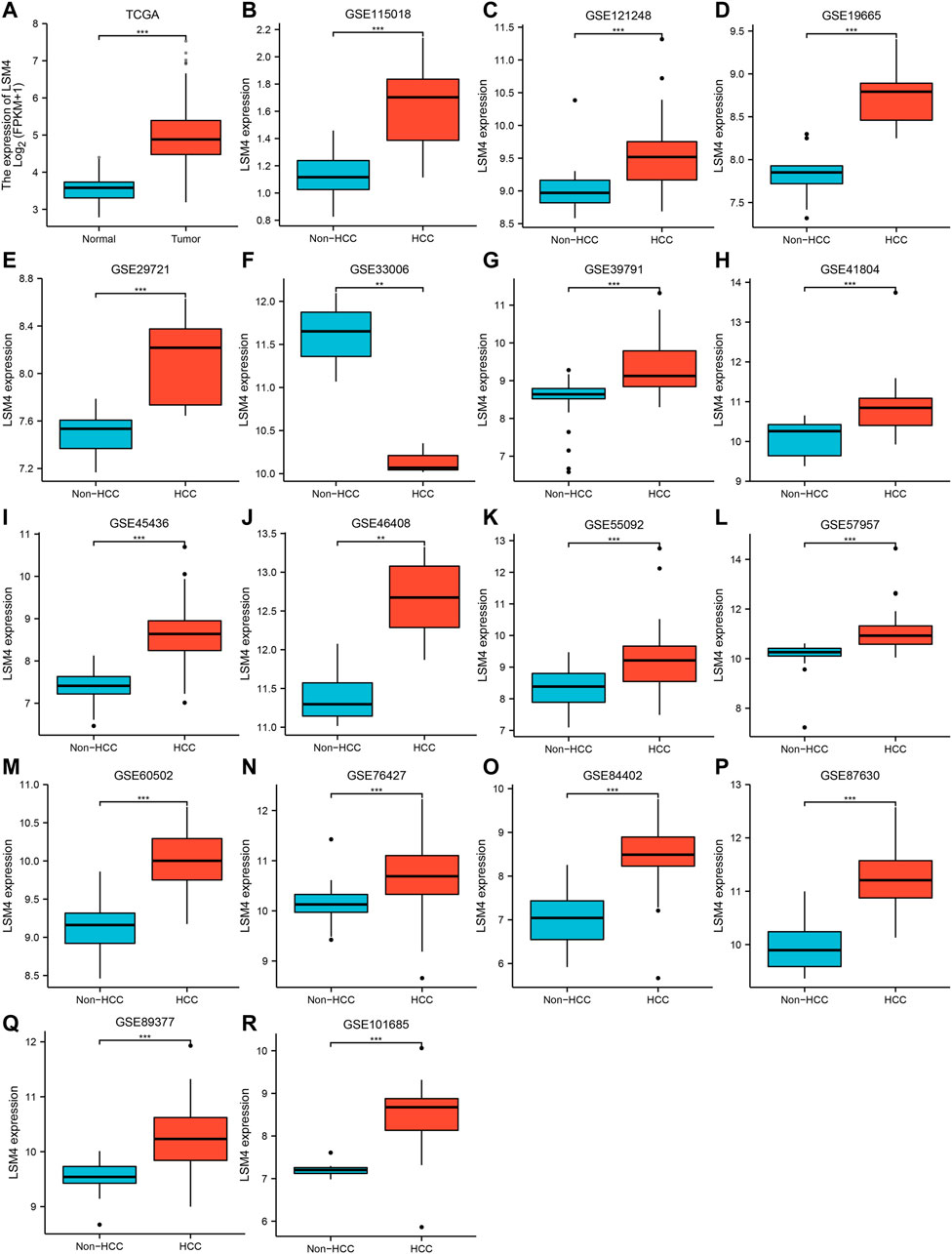
FIGURE 1. LSM4 expression in hepatocellular carcinoma. LSM4 expression between non-HCC tissues and HCC tissues based on GEO and TCGA datasets (*p < 0.05, **p < 0.01, ***p < 0.001). (A) TCGA. (B) GSE115018. (C) GSE121248. (D) GSE19665. (E) GSE29721. (F) GSE33006. (G) GSE39791. (H) GSE41804. (I) GSE45436. (J) GSE46408. (K) GSE55092. (L) GSE57957. (M) GSE60502. (N) GSE76427. (O) GSE84402. (P) GSE87630. (Q) GSE8937. (R) GSE101685.
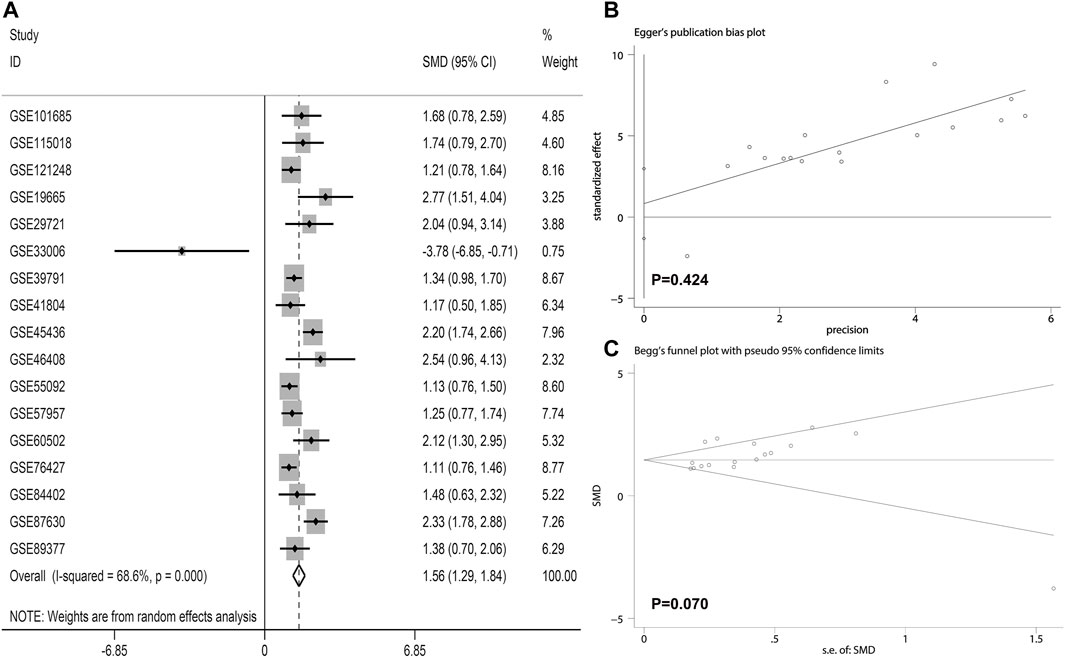
FIGURE 2. The integrated expression of LSM4 evaluated through calculating pooled standardized mean difference (SMD) (A); the Egger and Begg tests to evaluate publication bias (B–C).
Genetic Alteration Analysis of LSM4
The liver hepatocellular carcinoma (TCGA, PanCancer Atlas) was applied to analyze the genetic alterations via the cBioPortal database. The results revealed that the genetic alterations of LSM4 were detected in 26 (7%) of the 372 patients. The genetic alterations of LSM4 were mainly deep deletion, high mRNA expression, and amplification (Figure 3A). Our further study found that amplification was the most major mutation type (Figure 3B).
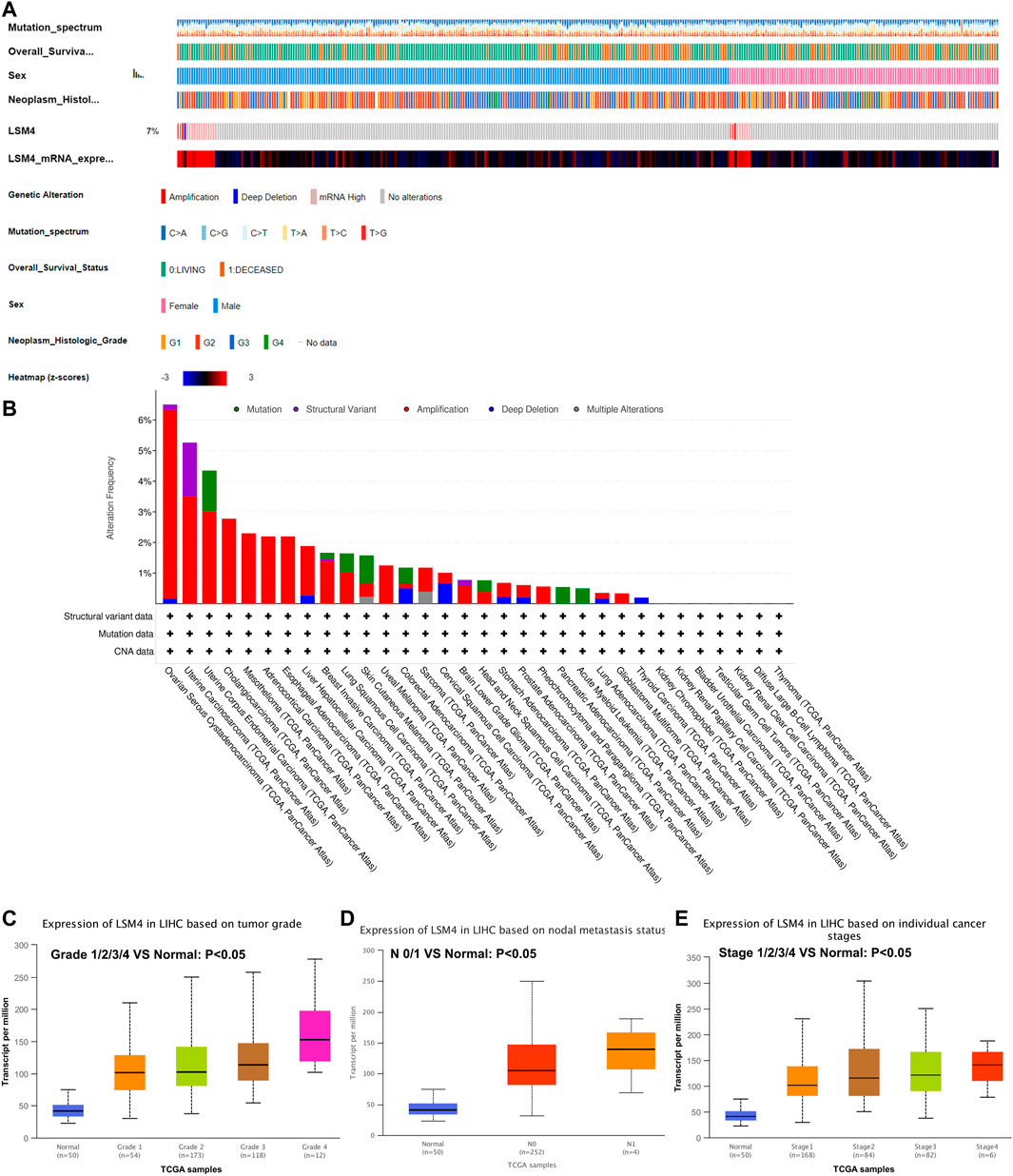
FIGURE 3. LSM4 genetic alteration status based on cBioPortal and the relationship between LSM4 expression and clinical characteristics of hepatocellular carcinoma based on UALCAN databases. LSM4 was altered in 26 (7%) of the queried 372 hepatocellular carcinoma patients (A–B). The expression of LSM4 in hepatocellular carcinoma based on tumor grade, and node metastasis status, and individual cancer stages (C–E).
Relationship Between LSM4 Expression and the Clinical Characteristics
The relationship between LSM4 expression and the clinical characteristics was analyzed based on the UALCAN database. As shown in Figures 3C–E, we explored the expression of LSM4 based on cancer stage and found that LSM4 expression was higher in advanced clinical stages (Figure 3E). After analyzing the expression of LSM4 based on lymph node metastasis status and tumor grade, the same upward trend was also observed in our study (Figures 3C,D). In summary, our study shows that LSM4 expression is related to cancer stage, tumor grade, and lymph node metastasis status.
Promising Diagnostic Value of LSM4 Overexpression in HCC
Current research results showed that LSM4 had a significant distinguish ability for HCC and the corresponding non-HCC tissues (All AUC> 0.77; Figure 4A–R). Regarding the sROC curve analysis, the AUC, sensitivity, and specificity were 0.91 (95% CI: 0.88–0.93), 0.84 (95% CI: 0.76–0.90), and 0.90 (95% CI: 0.74–0.96), respectively (Figures 5A, 6A). Besides, the positive diagnostic likelihood ratio (DLRP) of 8.11 (95% CI: 3.00–21.94) and negative diagnostic likelihood ratio (DLRN) of 0.18 (95% CI: 0.12–0.27) were observed (Figure 6B). According to the Deeks’ funnel test, no publication bias was detected in our study (p = 0.83, Figure 5B). In the Fagan chart, when the pre-test probability was 20%, the post-test probability of HCC using LSM4 for a positive test was 67%, and the probability of a negative test was 4%, indicating that LSM4 had the potential to be an effective biomarker for HCC (Figure 5C). The above results showed that LSM4 had excellent discrimination ability and had promising diagnostic value for HCC.
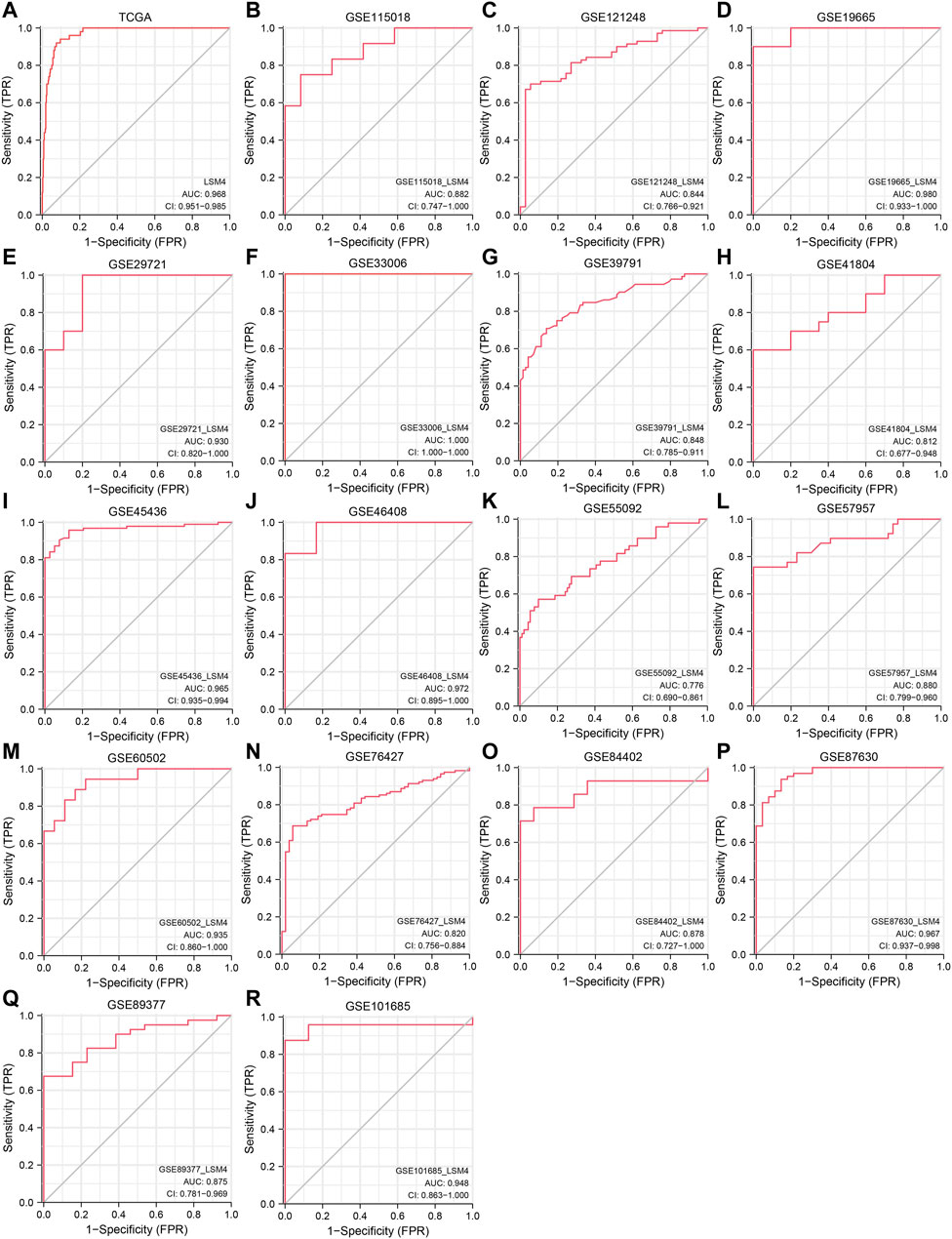
FIGURE 4. Diagnosis value of LSM4. The receiver operating characteristic (ROC) curve of non-HCC tissues and HCC tissues. tissues.(A) TCGA. (B) GSE115018. (C) GSE121248. (D) GSE19665. (E) GSE29721. (F) GSE33006. (G) GSE39791. (H) GSE41804. (I) GSE45436. (J) GSE46408. (K) GSE55092. (L) GSE57957. (M) GSE60502. (N) GSE76427. (O) GSE84402. (P) GSE87630. (Q) GSE8937. (R) GSE101685.
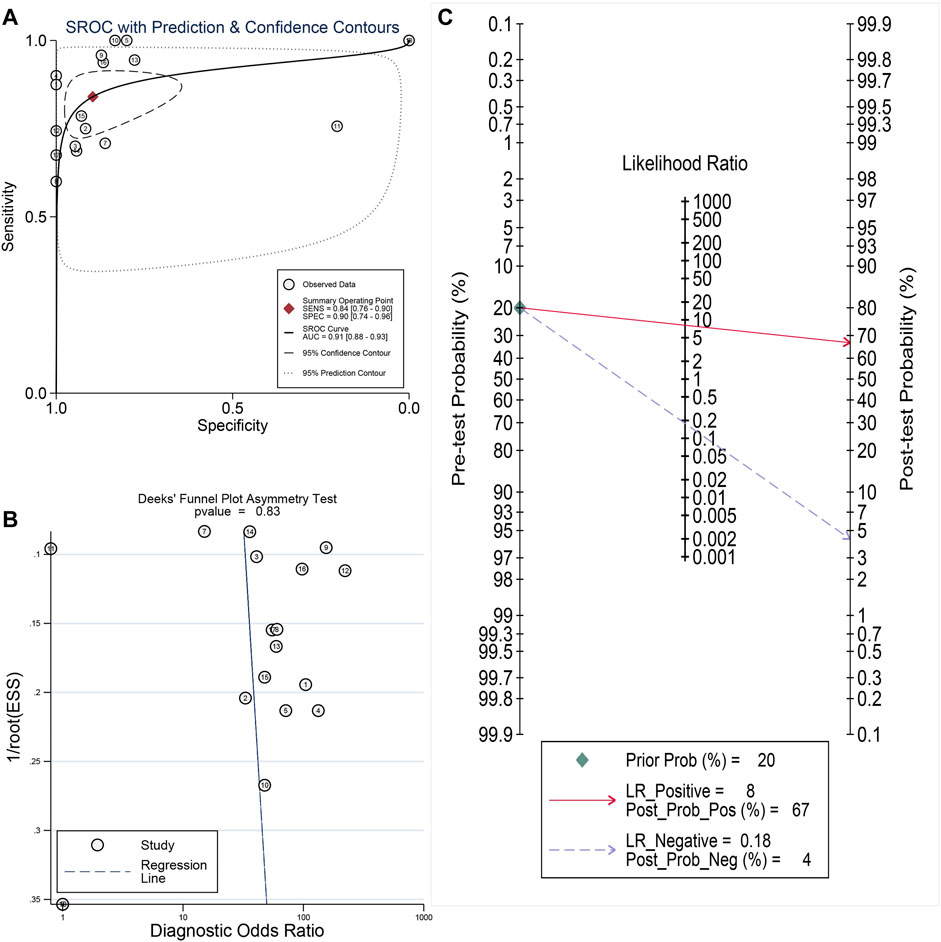
FIGURE 5. Comprehensive analysis of the diagnostic significance of LSM4 in hepatocellular carcinoma. The summary receiver operating characteristic (sROC) curve exhibited a tremendous distinguish capacity of LSM4 in HCC and non-HCC tissues (A). Deeks’ Funnel Plot, p-value >0.50, indicating no publication bias (B). Fagan’s plot also addressed the diagnostic value of LSM4 for HCC patients (C).
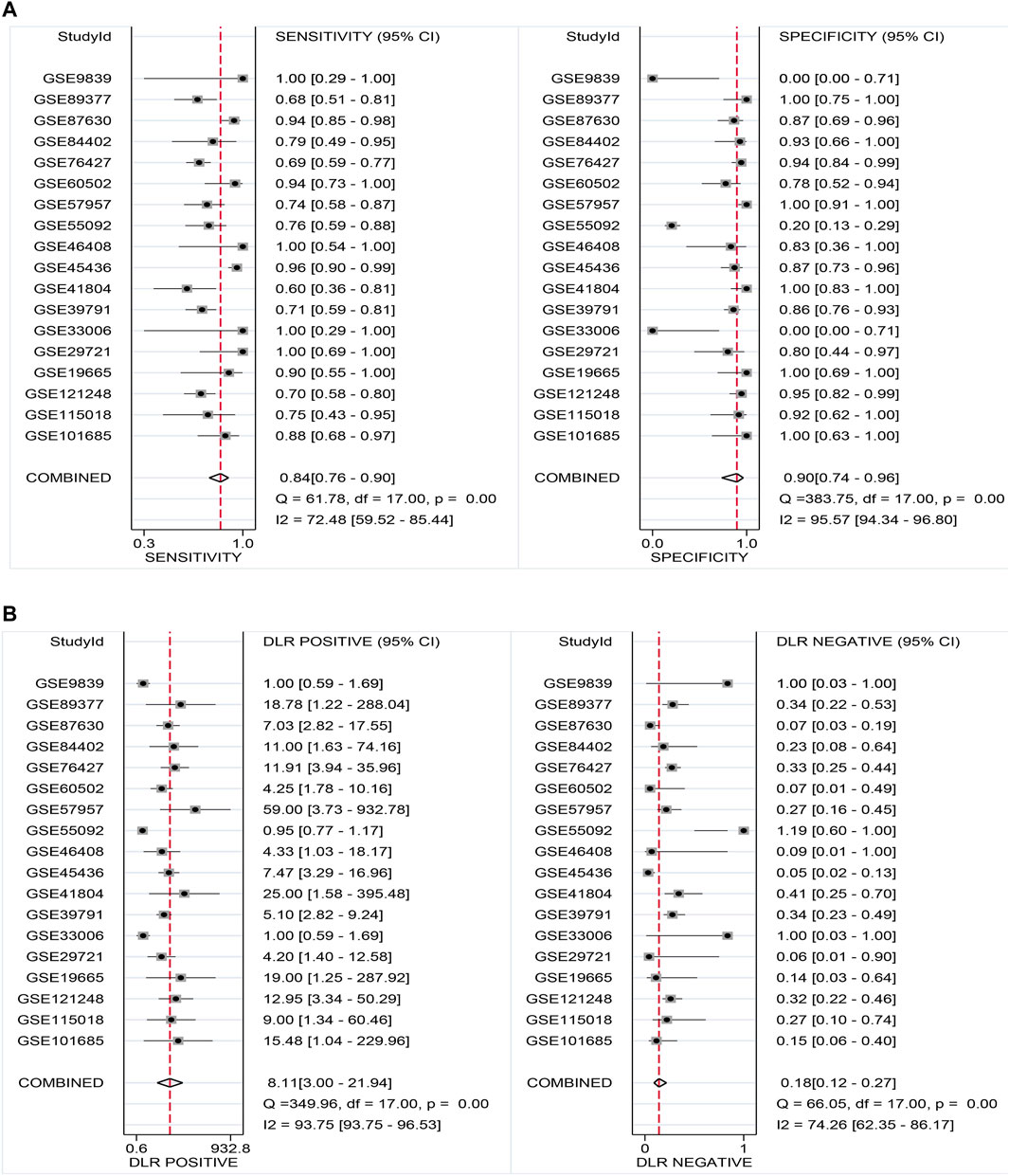
FIGURE 6. Sensitivity and specificity as well as DLR positive and DLR negative. (A) Sensitivity and specificity values of included studies. (B) DLR positive and DLR negative of included studies.
LSM4 Overexpression Predicted Poor Survival
To determine the prognostic significance of LSM4 expression in patients with HCC, we used the GEPIA database to detect the correlation of DFS, OS and LSM4 expression. We observed that LSM4 overexpression was significantly associated with shorter DFS (HR = 1.4, p = 0.035, Figure 7A) and OS (HR = 1.7, p = 0.0036, Figure 7B). In addition, based on the TCGA dataset, we divided patients into two groups (high and low groups) based on the median expression level of LSM4 and then constructed a Kaplan-Meier chart for survival analysis. We also observed a significant association between the high expression of LSM4 and poor OS (HR = 1.57, p = 0.011, Figure 7C). Furthermore, univariate and multivariate Cox regression analyses were performed on LSM4 expression (high and low expression were divided according to the median of LSM4 expression value) and other clinicopathological characteristics (such as AFP level, age, gender, stage, histologic grade, Fibrosis ishak score). Interestingly, the result of high LSM4 expression was associated with poor OS was observed in both univariate and multivariate Cox regression analysis (Table 2). This suggested that overexpressed LSM4 was independently associated with poor OS of patients with HCC.
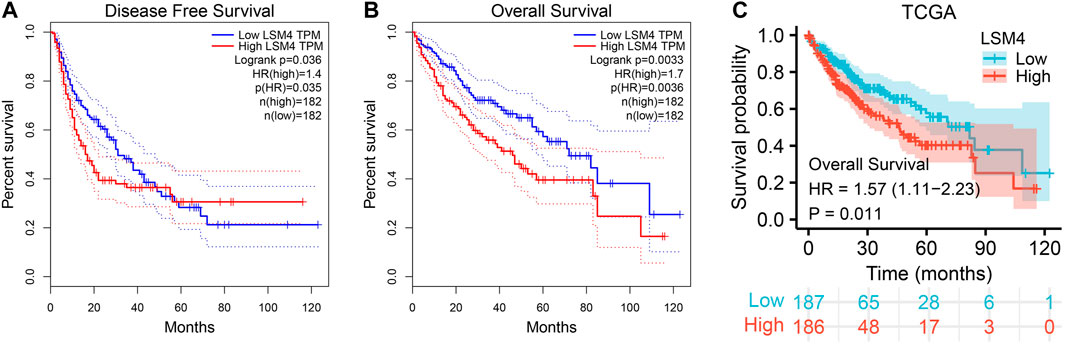
FIGURE 7. Survival curves of overall survival (OS) and disease-free survival (DFS) in hepatocellular carcinoma. Kaplan–Meier curves of hepatocellular carcinoma patients OS and DFS in patients with different LSM4 expression based on GEPIA database (A–B). Kaplan–Meier curves of OS grouped by LSM4 expression in hepatocellular carcinoma validated by TCGA datasets (C).
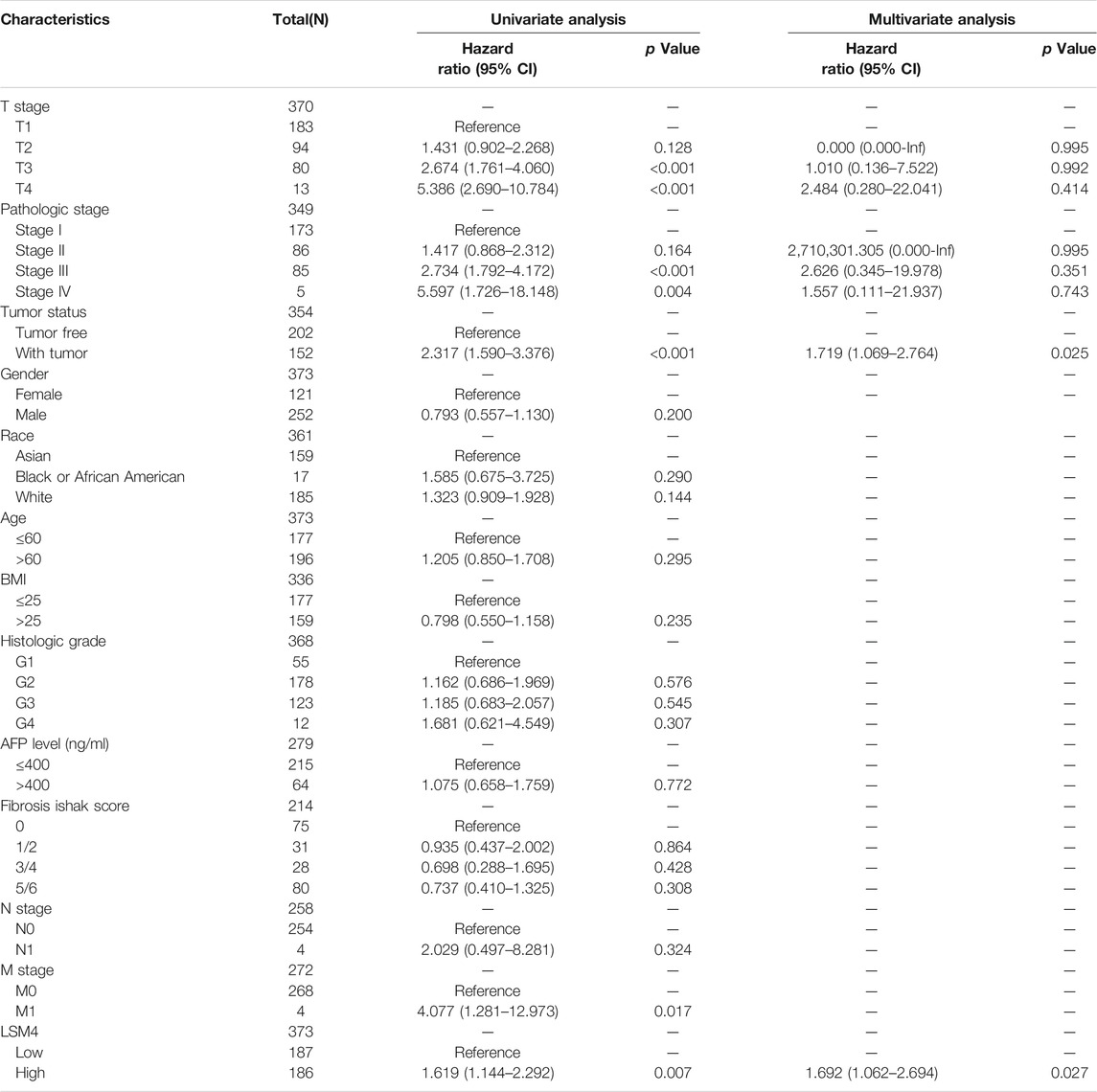
TABLE 2. Univariate and multivariate cox analysis of hepatocellular carcinoma patients’ overall survival.
Identification of CEGs and Their Clinical Value in HCC
The correlation coefficients of LSM4 and other genes were obtained through the GEPIA and cBioPortal databases. Subsequently, CEGs meeting the criteria of correlation coefficient ≥0.60 with p-value <0.05 were obtained for further analysis. In the GEPIA and cBioPortal databases, 22 and 50 LSM4 positively correlated CEGs were identified, respectively, and a total of nine crossing CEGs were obtained (Figure 8A). The scatter plot shows the significant positive correlation between LSM4 and nine CEGs (Figures 8B–J). In addition, our research based on the TCGA dataset found that the expression level of nine CEGs was significantly up-regulated in HCC tissues (Figure 9A). Next, we also explored the clinical significance of nine CEGs expressions in HCC. Our results show that the above genes had excellent recognition ability for HCC tissues and non-HCC tissues, showing the promising diagnostic value (2 CEGs AUC>0.7, seven CEGs AUC>0.90, Figure 9B). Subsequent survival analysis also showed that six CEGs were closely associated with the poor OS of patients with HCC, while the other three CEGs showed no significant relation to the OS of HCC patients (Figures 9C–K). In summary, six CEGs, including CCDC124, NR2C2AP, SLC25A39, DDX49, KXD1, TXNL4A, showed promising diagnostic and prognostic significance (AUC>0.90; HR > 1.40, p < 0.05), which may indicate showing its attractive research value in HCC.
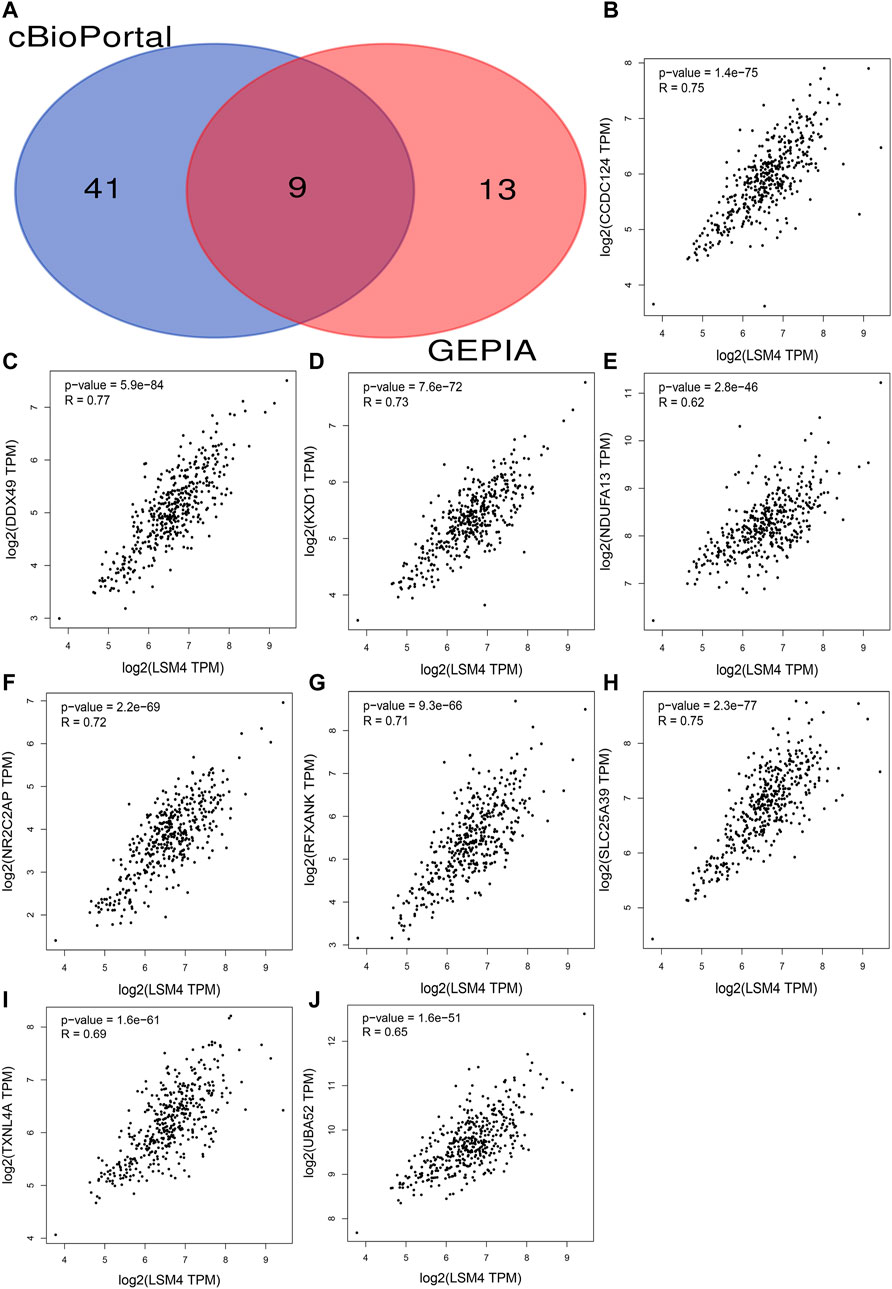
FIGURE 8. Identification co-expressed genes (CEGs) of LSM4 in hepatocellular carcinoma. Venn diagram for the nine intersection genes of LSM4 positively related CEGs identified by the cBioPortal and the GEPIA databases (A). The scatter plot shows the significant positive correlation between LSM4 and nine CEGs (B–J).
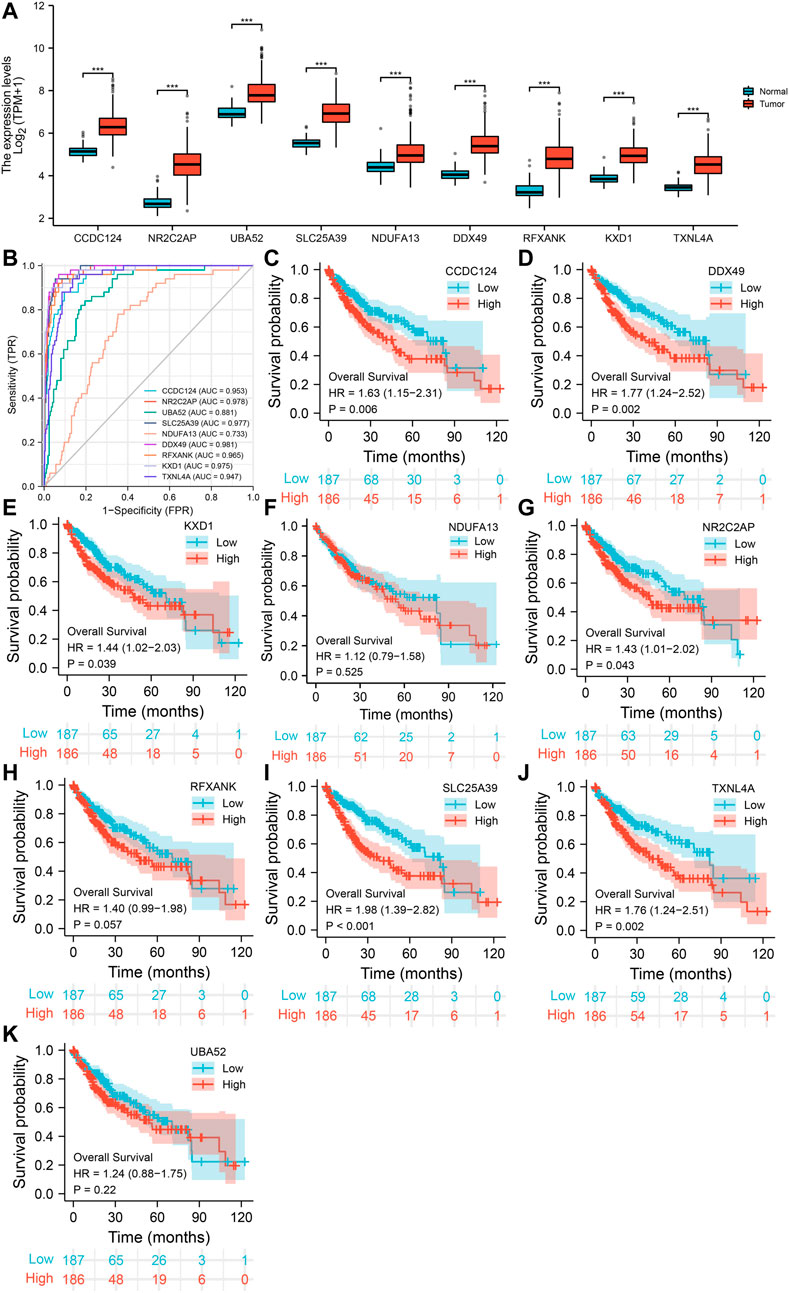
FIGURE 9. The expression of nine co-expressed genes (CEGs) in hepatocellular carcinoma and their diagnostic and prognostic significance based on TCGA dataset. The elevated expression of nine CEGs in HCC tissues (A). The receiver operating characteristic (ROC) curve of LSM4 in non-HCC tissues and HCC tissues (B). Kaplan–Meier curves of overall survival (OS) grouped by LSM4 expression in hepatocellular carcinoma (C–K).
LSM4-Associated Signal Pathways in HCC
In order to determine the cancer-related signaling pathways related to LSM4, GSEA analysis was performed using the LSM4 expression data contained in the TCGA dataset. The results showed that the cell cycle and DNA replication pathways were significantly activated in patients with LSM4 overexpression (Figures 10A,B). On the contrary, the LSM4 overexpression was negatively correlated with the focal adhesion pathway (Figure 10C). Besides, LSM4 overexpression was also significantly negatively correlated with multiple metabolism-associated pathways, such as fatty acid metabolism, propanoate acid metabolism, retinol metabolism, and tryptophan metabolism (Figures 10D–G).
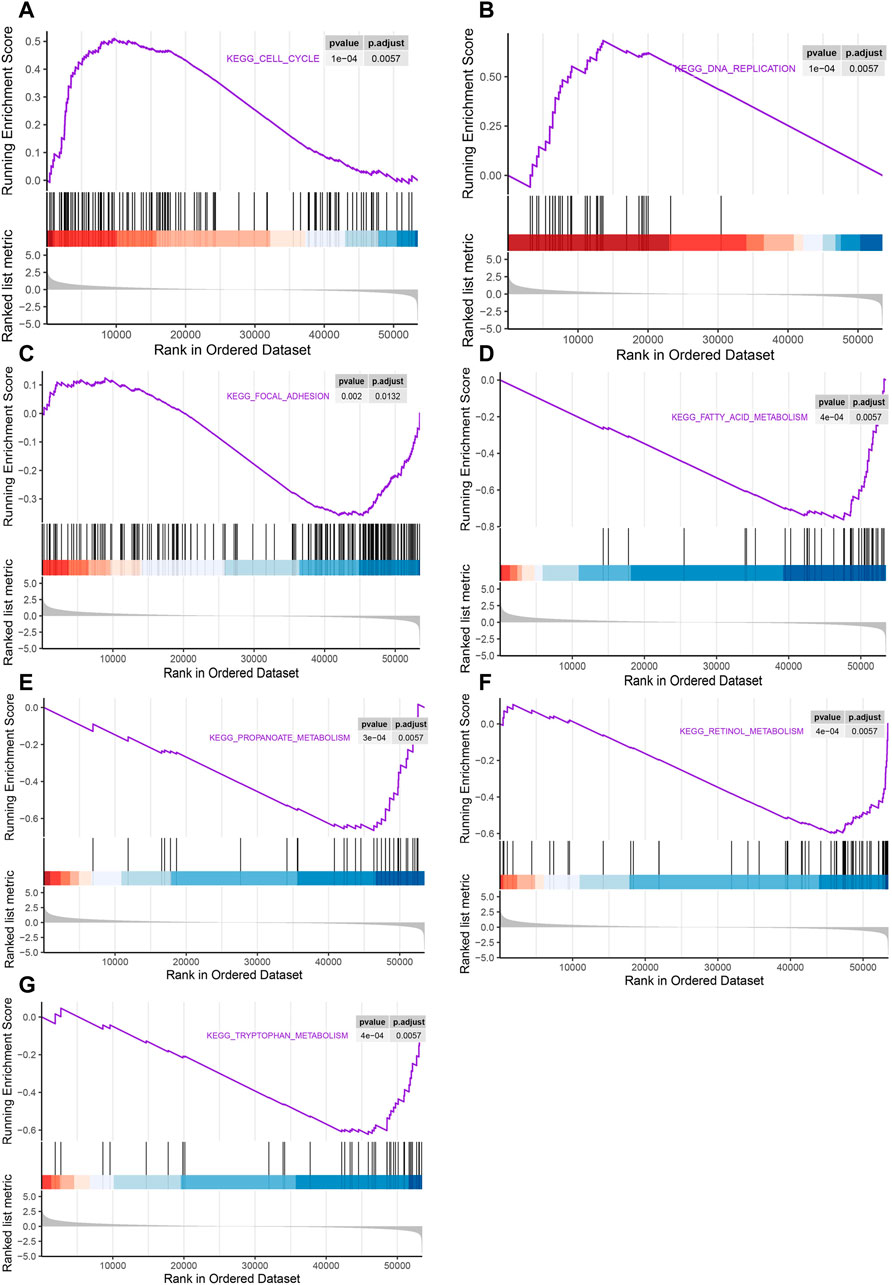
FIGURE 10. Gene set enrichment analysis (GSEA) of pathways associated with LSM4 expression. The LSM4 expression was significantly correlated with the cell cycle, DNA replication, focal adhesion and multiple metabolism-associated pathways (A–G).
Discussion
As a highly malignant tumor with a poor prognosis, hepatocellular carcinoma (HCC) seriously threatens human health and causes a great economic burden to patients and society (Chen et al., 2016; Yan and Fan, 2019). Although plenty of studies in recent years have reported a variety of biomarkers for HCC, the currently available are still insufficient (Pang et al., 2020; Xing et al., 2020; Cai et al., 2021; Dolicka et al., 2021). Therefore, it is necessary to identify novel biomarkers for early diagnosis and prognostic monitoring of HCC. The main purpose of our current study is to identify the clinical significance of LSM4 in HCC and try to reveal its underlying molecular mechanism using bioinformatics methods.
First, the expression pattern and clinical significance of LSM4 in HCC were explored using the GEO and TCGA datasets. In our study, the combined SMD reached 1.56 (95% CI: 1.29–1.84, p < 0.001), and there was no significant publication bias observed in our study, suggesting a significant overexpression of LSM4 in HCC. Through ROC curve analysis, the excellent performance of LSM4 to distinguish HCC tissues from non-HCC liver tissues was observed (All AUC> 0.77). Subsequent sROC analysis also confirmed the above conclusion (AUC = 0.91, 95% CI: 0.88–0.93). In addition, its higher expression also appeared to be associated with higher tumor grade, lymph node metastasis status, pathological stage and poor patient prognosis. In addition, univariate and multivariate Cox regression analysis, including the main clinical features, showed that LSM4 expression was independently associated with the poor OS in patients with HCC (both HR > 1.6, p < 0.05). Summarily, the current evidence indicated that LSM4 might act as a cancer-promoting factor and participate in the occurrence and malignant progression of HCC, and it has the potential as the diagnostic biomarker of HCC and the predictor of poor prognosis.
Subsequently, we identified nine CEGs positively correlated with LSM4 in HCC to further analyze the mechanisms. Our study showed that all CEGs were significantly up-regulated in HCC. Further study found that six CEGs, including CCDC124, NR2C2AP, SLC25A39, DDX49, KXD1, TXNL4A, had good diagnostic value and were related to poor OS in HCC patients (all AUC>0.90; all HR > 1.40, p < 0.05). According to the previous reports, CCDC124 was significantly overexpressed in endometrial cancer (EC), hepatocellular carcinoma (HCC), colorectal cancer (CRC), and ovarian cancer (OC) was related to tumor cell division, tumor heterogeneity and drug resistance (Lu et al., 2020; Arslan et al., 2021). The expression of DDX49 was significantly up-regulated in HCC, and knocking it down could suppress the growth and metastasis of HCC tumors, while overexpression of DDX49 could enhance the above procession (Dai et al., 2021). In addition, DDX49 participated in forming the SNHG20/miR-342/DDX49 axis, thereby participating in the positive regulation of lung adenocarcinoma cell proliferation, invasion and apoptosis (Wang et al., 2020). Another study proved that DDX49 participated in the promotion of the growth of NSCLC cells and lymph node metastasis via increasing the Akt/β-catenin pathway (Lian et al., 2020). In NSCLC, KXD1 overexpression was positively correlated with cancer invasion and metastasis and negatively correlated with non-surgical anti-cancer treatment resistance (Wang et al., 2021). NR2C2AP, which was highly expressed in NSCLC, also known as TRA16, could promote cancer cell growth by enhancing the ERβ signaling pathway and was associated with lymph node metastasis and poor OS (Fang et al., 2013). Since that TXNL4A and SLC25A39 were rarely reported in cancer, we did not discuss them in-depth. Although not all six CEGs have been reported in HCC, combined with our study and previous reports, the above genes seemed closely related to tumor growth and metastasis. Given the significant positive correlation between LSM4 and the above CEGs in HCC, we speculate that LSM4 may cooperate with the six positively correlated CEGs in the promotion of the growth and metastasis of HCC cells.
The results from the GSEA analysis showed that the LSM4 overexpression in HCC was strongly associated with the activation of cell proliferation-related pathways, including DNA replication and cell cycle pathways. Cell cycle disorder was one of the hallmarks of cancer (Stewart et al., 2003). Previous studies demonstrated that cell cycle disorders could lead to uncontrolled cell proliferation, leading to cancer development (Yang et al., 2017; Zhou et al., 2018). Disorders of cell proliferation and metastasis were important causes of cancer development (Yang et al., 2019). In addition, our study also showed that there was a significant correlation between the focal adhesion pathway and LSM4 overexpression. Numerous studies have reported that the focal adhesion pathway had a crucial effect on cancer metastasis and invasive behavior. Among the genes represented in this pathway was FAK, which may have an essential role in promoting tumorigenesis and metastasis (Luo and Guan, 2010; Ocak et al., 2010; Zhang et al., 2021). Another study showed that activation of focal adhesion pathway mediated the survival, invasion, proliferation and drug resistance of HCC cells. Besides, the results from GSEA analysis also indicated that LSM4 overexpression was related to multiple metabolic pathways, such as fatty acid metabolism, propionic acid metabolism, retinol metabolism, and tryptophan metabolism. In recent years, tumor metabolism has gradually become one of the research hotspots (Liu C et al., 2021). It played a key role in the malignant biological behavior of cancer and was beneficial to the survival, proliferation, invasion and metastasis of cancer cells (Ma and Zong, 2020). For example, fatty acid metabolism played an essential role in the progression and metastasis of various cancers, including PC and HCC (Sunami et al., 2021). In general, the results of GSEA analysis supported the previous speculation that overexpression of LSM4 seemed to be more closely related to the promotion of growth and metastasis of HCC cells. Besides, it may be through the regulation of crucial pathways, such as cell cycle, focal adhesion and metabolism-related pathways, to play the above role.
This study has some obvious advantages. Based on the integrated 18 datasets from the TCGA and GEO databases, we comprehensively identified the overexpression of LSM4 in HCC and its promising diagnostic and prognostic value. Datasets from multiple sources and large sample size ensured the credibility of our research. However, the limitations of this study also could not be ignored. First, although the random-effects model was applied, the influence of higher heterogeneity research could not be completely eliminated; secondly, our study was limited in vitro studies, and more in vivo and vitro studies were still needed in the future to demonstrate our conclusions and clarify the specific mechanism of LSM4 in the growth and metastasis of HCC.
Conclusions
In conclusion, overexpressed LSM4 may serve as a promising diagnostic and prognostic biomarker for HCC. Besides, LSM4 may play a synergistic effect with CEGs in promoting the growth and metastasis of HCC cells via regulating crucial pathways such as cell cycle, focal adhesion, and metabolism-associated pathways.
Abbreviations
AFP, alpha-fetoprotein; BRCA, breast cancer; CEGs, co-expressed genes; CRC, colorectal cancer; DFS, Disease-Free Survival; DLRN, negative diagnostic likelihood ratio; EC, endometrial cancer; FDR, false discovery rate; GEO, Gene Expression Omnibus; GSEA, Gene set enrichment analysis; HCC, Hepatocellular carcinoma; LSM, Smith-like; LUSC, lung squamous cell carcinoma; OS, overall survival; OC, ovarian cancer; PC, pancreatic cancer; ROC, receiver operating characteristic; SMD, standardized mean difference; sROC, summary receiver operating characteristic; TCGA, The Cancer Genome Atlas.
Data Availability Statement
The original contributions presented in the study are included in the article/supplementary material, further inquiries can be directed to the corresponding authors.
Author Contributions
All authors listed have made a substantial, direct, and intellectual contribution to the work and approved it for publication.
Conflict of Interest
The authors declare that the research was conducted in the absence of any commercial or financial relationships that could be construed as a potential conflict of interest.
Publisher’s Note
All claims expressed in this article are solely those of the authors and do not necessarily represent those of their affiliated organizations, or those of the publisher, the editors and the reviewers. Any product that may be evaluated in this article, or claim that may be made by its manufacturer, is not guaranteed or endorsed by the publisher.
Acknowledgments
We acknowledge TCGA and GEO databases for providing their platforms and contributors for uploading their meaningful datasets.
References
Arslan, Ö., Soylu, N. K., Akillilar, P. T., and Tazebay, U. H. (2021). Coiled-coil Domain-Containing Protein-124 (Ccdc124) Is a Novel RNA Binding Factor Up-Regulated in Endometrial, Ovarian, and Urinary Bladder Cancers. Cancer Biomark. 31, 149–164. doi:10.3233/cbm-200802
Bray, F., Ferlay, J., Soerjomataram, I., Siegel, R. L., Torre, L. A., and Jemal, A. (2018). Global Cancer Statistics 2018: GLOBOCAN Estimates of Incidence and Mortality Worldwide for 36 Cancers in 185 Countries. CA: A Cancer J. Clin. 68, 394–424. doi:10.3322/caac.21492
Cai, C., Zhang, Y., Hu, X., Hu, W., Yang, S., Qiu, H., et al. (2021). CDT1 Is a Novel Prognostic and Predictive Biomarkers for Hepatocellular Carcinoma. Front. Oncol. 11, 721644. doi:10.3389/fonc.2021.721644
Chen, M., Luo, F., Yu, J., Xiang, G., Jiang, D., and Pu, X. (2016). Common Functional Polymorphism within miR-146a and miR-196a-2 as Susceptibility Loci for Hepatocellular Carcinoma: An Updated Meta-Analysis. Meta Gene. 7, 40–47. doi:10.1016/j.mgene.2015.11.002
Dai, H., Feng, J., Nan, Z., Wei, L., Lin, F., Jin, R., et al. (2021). Morphine May Act via DDX49 to Inhibit Hepatocellular Carcinoma Cell Growth. Aging 13, 12766–12779. doi:10.18632/aging.202946
Dolicka, D., Sobolewski, C., Gjorgjieva, M., Correia de Sousa, M., Berthou, F., De Vito, C., et al. (2021). Tristetraprolin Promotes Hepatic Inflammation and Tumor Initiation but Restrains Cancer Progression to Malignancy. Cell Mol. Gastroenterol. Hepatol. 11, 597–621. doi:10.1016/j.jcmgh.2020.09.012
Fang, F., Zheng, Q., Zhang, J., Dong, B., Zhu, S., Huang, X., et al. (2013). Testicular Orphan Nuclear Receptor 4-associated Protein 16 Promotes Non-small Cell Lung Carcinoma by Activating Estrogen Receptor β and Blocking Testicular Orphan Nuclear Receptor 2. Oncol. Rep. 29, 297–305. doi:10.3892/or.2012.2107
Hou, W., and Zhang, Y. (2021). Circ_0025033 Promotes the Progression of Ovarian Cancer by Activating the Expression of LSM4 via Targeting miR-184. Pathol. Res. Pract. 217, 153275. doi:10.1016/j.prp.2020.153275
Li, W., Li, X., Gao, L.-N., and You, C.-G. (2020). Integrated Analysis of the Functions and Prognostic Values of RNA Binding Proteins in Lung Squamous Cell Carcinoma. Front. Genet. 11, 185. doi:10.3389/fgene.2020.00185
Lian, X., Xiang, D., Peng, C., Chen, J., Liao, M., Sun, G., et al. (2020). DDX49 Is a Novel Biomarker and Therapeutic Target for Lung Cancer Metastases. J. Cel Mol. Med. 24, 1141–1145. doi:10.1111/jcmm.14734
Liu C, C., Jin, Y., and Fan, Z. (2021). The Mechanism of Warburg Effect-Induced Chemoresistance in Cancer. Front. Oncol. 11, 698023. doi:10.3389/fonc.2021.698023
Liu Y, Y., Veeraraghavan, V., Pinkerton, M., Fu, J., Douglas, M. W., George, J., et al. (2021). Viral Biomarkers for Hepatitis B Virus-Related Hepatocellular Carcinoma Occurrence and Recurrence. Front. Microbiol. 12, 665201. doi:10.3389/fmicb.2021.665201
Lu, W., Fu, D., Kong, X., Huang, Z., Hwang, M., Zhu, Y., et al. (2020). FOLFOX Treatment Response Prediction in Metastatic or Recurrent Colorectal Cancer Patients via Machine Learning Algorithms. Cancer Med. 9, 1419–1429. doi:10.1002/cam4.2786
Luo, M., and Guan, J.-L. (2010). Focal Adhesion Kinase: a Prominent Determinant in Breast Cancer Initiation, Progression and Metastasis. Cancer Lett. 289, 127–139. doi:10.1016/j.canlet.2009.07.005
Ma, L., and Zong, X. (2020). Metabolic Symbiosis in Chemoresistance: Refocusing the Role of Aerobic Glycolysis. Front. Oncol. 10, 5. doi:10.3389/fonc.2020.00005
Nordenstedt, H., White, D. L., and El-Serag, H. B. (2010). The Changing Pattern of Epidemiology in Hepatocellular Carcinoma. Dig. Liver Dis. 42, S206–S214. doi:10.1016/s1590-8658(10)60507-5
Ocak, S., Yamashita, H., Udyavar, A. R., Miller, A. N., Gonzalez, A. L., Zou, Y., et al. (2010). DNA Copy Number Aberrations in Small-Cell Lung Cancer Reveal Activation of the Focal Adhesion Pathway. Oncogene 29, 6331–6342. doi:10.1038/onc.2010.362
Pang, Y. Y., Li, J. D., Gao, L., Yang, X., Dang, Y. W., Lai, Z. F., et al. (2020). The Clinical Value and Potential Molecular Mechanism of the Downregulation of MAOA in Hepatocellular Carcinoma Tissues. Cancer Med. 9, 8004–8019. doi:10.1002/cam4.3434
Stewart, Z. A., Westfall, M. D., and Pietenpol, J. A. (2003). Cell-cycle Dysregulation and Anticancer Therapy. Trends Pharmacol. Sci. 24, 139–145. doi:10.1016/s0165-6147(03)00026-9
Streicher, K. L., Yang, Z. Q., Draghici, S., and Ethier, S. P. (2007). Transforming Function of the LSM1 Oncogene in Human Breast Cancers with the 8p11-12 Amplicon. Oncogene 26, 2104–2114. doi:10.1038/sj.onc.1210002
Sunami, Y., Rebelo, A., and Kleeff, J. (2021). Lipid Droplet-Associated Factors, PNPLA3, TM6SF2, and HSD17B Proteins in Hepatopancreatobiliary Cancer. Cancers (Basel) 13 (17), 4391. doi:10.3390/cancers13174391
Ta, H. D. K., Wang, W. J., Phan, N. N., An Ton, N. T., Anuraga, G., Ku, S. C., et al. (2021). Potential Therapeutic and Prognostic Values of LSM Family Genes in Breast Cancer. Cancers (Basel) 13 (19), 4902. doi:10.3390/cancers13194902
Tang, W., Li, J., Chang, X., Jia, L., Tang, Q., Wang, Y., et al. (2020). Construction of a Novel Prognostic-Predicting Model Correlated to Ovarian Cancer. Biosci. Rep. 40 (8), BSR20201261. doi:10.1042/BSR20201261
Wang, W., and Wei, C. (2020). Advances in the Early Diagnosis of Hepatocellular Carcinoma. Genes Dis. 7, 308–319. doi:10.1016/j.gendis.2020.01.014
Wang, X., Gu, G., Zhu, H., Lu, S., Abuduwaili, K., and Liu, C. (2020). LncRNA SNHG20 Promoted Proliferation, Invasion and Inhibited Cell Apoptosis of Lung Adenocarcinoma via Sponging miR ‐342 and Upregulating DDX49. Thorac. Cancer 11, 3510–3520. doi:10.1111/1759-7714.13693
Wang, S., Ma, H., Li, H., Liu, Q., Huang, S., Huang, L., et al. (2021). Alternatively Expressed Transcripts Analysis of Non-small Cell Lung Cancer Cells under Different Hypoxic Microenvironment. J. Oncol. 2021, 5558304. doi:10.1155/2021/5558304
Watson, P. M., Miller, S. W., Fraig, M., Cole, D. J., Watson, D. K., and Boylan, A. M. (2008). CaSm (LSm-1) Overexpression in Lung Cancer and Mesothelioma Is Required for Transformed Phenotypes. Am. J. Respir. Cel Mol. Biol. 38, 671–678. doi:10.1165/rcmb.2007-0205oc
Xie, Z., Zhou, F., Yang, Y., Li, L., Lei, Y., Lin, X., et al. (2018). Lnc-PCDH9-13:1 Is a Hypersensitive and Specific Biomarker for Early Hepatocellular Carcinoma. EBioMedicine 33, 57–67. doi:10.1016/j.ebiom.2018.06.026
Xing, J., Tian, Y., Ji, W., and Wang, X. (2020). Comprehensive Evaluation of SPATS2 Expression and its Prognostic Potential in Liver Cancer. Medicine 99, e19230. doi:10.1097/md.0000000000019230
Xue, R., Hua, L., Xu, W., Gao, Y., Pang, Y., and Hao, J. (2018). Derivation and Validation of the Potential Core Genes in Pancreatic Cancer for Tumor-Stroma Crosstalk. Biomed. Res. Int. 2018, 4283673. doi:10.1155/2018/4283673
Yan, S. Y., and Fan, J. G. (2019). Advances in the Study of Non-infectious Liver Diseases and Precancerous Lesions of Hepatocellular Carcinoma. Zhonghua Gan Zang Bing Za Zhi 27, 487–490. doi:10.3760/cma.j.issn.1007-3418.2019.07.003
Yang, C.-Y., Hsieh, C.-C., Lin, C.-K., Lin, C.-S., Peng, B., Lin, G.-J., et al. (2017). Danshen Extract Circumvents Drug Resistance and Represses Cell Growth in Human Oral Cancer Cells. BMC Complement. Altern. Med. 17, 555. doi:10.1186/s12906-017-2063-y
Yang, Y., Chen, D., Liu, H., and Yang, K. (2019). Increased Expression of lncRNA CASC9 Promotes Tumor Progression by Suppressing Autophagy-Mediated Cell Apoptosis via the AKT/mTOR Pathway in Oral Squamous Cell Carcinoma. Cell Death Dis. 10, 41. doi:10.1038/s41419-018-1280-8
Yin, J., Lin, C., Jiang, M., Tang, X., Xie, D., Chen, J., et al. (2021). CENPL, ISG20L2, LSM4, MRPL3 Are Four Novel Hub Genes and May Serve as Diagnostic and Prognostic Markers in Breast Cancer. Sci. Rep. 11, 15610. doi:10.1038/s41598-021-95068-6
Zhang, Z., Yu, Y., Zhang, P., Ma, G., Zhang, M., Liang, Y., et al. (2021). Identification of NTRK3 as a Potential Prognostic Biomarker Associated with Tumor Mutation burden and Immune Infiltration in Bladder Cancer. BMC Cancer 21, 458. doi:10.1186/s12885-021-08229-1
Keywords: HCC, LSM4, prognosis, diagnostic, gene set enrichment analysis, co-expressed gene
Citation: Chen L, Lin Y-h, Liu G-q, Huang J-e, Wei W, Yang Z-h, Hu Y-m, Xie J-h and Yu H-z (2022) Clinical Significance and Potential Role of LSM4 Overexpression in Hepatocellular Carcinoma: An Integrated Analysis Based on Multiple Databases. Front. Genet. 12:804916. doi: 10.3389/fgene.2021.804916
Received: 29 October 2021; Accepted: 27 December 2021;
Published: 13 January 2022.
Edited by:
Yi Zhao, Institute of Computing Technology (CAS), ChinaReviewed by:
Tuba Denkçeken, Sanko University, TurkeyLiang-Ting Lin, Hong Kong Polytechnic University, Hong Kong SAR, China
Copyright © 2022 Chen, Lin, Liu, Huang, Wei, Yang, Hu, Xie and Yu. This is an open-access article distributed under the terms of the Creative Commons Attribution License (CC BY). The use, distribution or reproduction in other forums is permitted, provided the original author(s) and the copyright owner(s) are credited and that the original publication in this journal is cited, in accordance with accepted academic practice. No use, distribution or reproduction is permitted which does not comply with these terms.
*Correspondence: Jia-heng Xie, eGllamlhaGVuZ0Buam11LmVkdS5jbg==; Hong-zhu Yu, aG9uZ3podS42MjA5MjlAYWxpeXVuLmNvbQ==
†These authors have contributed equally to this work and share the first authorship