- 1Smart Health Big Data Analysis and Location Services Engineering Lab of Jiangsu Province, Department of Bioinformatics, School of Geographic and Biologic Information, Nanjing University of Posts and Telecommunications, Nanjing, China
- 2School of Pharmacy, Macau University of Science and Technology, Macau, China
Thyroid nodules are present in upto 50% of the population worldwide, and thyroid malignancy occurs in only 5–15% of nodules. Until now, fine-needle biopsy with cytologic evaluation remains the diagnostic choice to determine the risk of malignancy, yet it fails to discriminate as benign or malignant in one-third of cases. In order to improve the diagnostic accuracy and reliability, molecular testing based on transcriptomic data has developed rapidly. However, gene signatures of thyroid nodules identified in a plenty of transcriptomic studies are highly inconsistent and extremely difficult to be applied in clinical application. Therefore, it is highly necessary to identify consistent signatures to discriminate benign or malignant thyroid nodules. In this study, five independent transcriptomic studies were combined to discover the gene signature between benign and malignant thyroid nodules. This combined dataset comprises 150 malignant and 93 benign thyroid samples. Then, there were 279 differentially expressed genes (DEGs) discovered by the feature selection method (Student’s t test and fold change). And the weighted gene co-expression network analysis (WGCNA) was performed to identify the modules of highly co-expressed genes, and 454 genes in the gray module were discovered as the hub genes. The intersection between DEGs by the feature selection method and hub genes in the WGCNA model was identified as the key genes for thyroid nodules. Finally, four key genes (ST3GAL5, NRCAM, MT1F, and PROS1) participated in the pathogenesis of malignant thyroid nodules were validated using an independent dataset. Moreover, a high-performance classification model for discriminating thyroid nodules was constructed using these key genes. All in all, this study might provide a new insight into the key differentiation of benign and malignant thyroid nodules.
Introduction
Thyroid nodules are regarded as common clinical problems worldwide, and nearly 50% of the population harbor thyroid nodules (Burman and Wartofsky, 2015; Jasim et al., 2020). For benign thyroid nodules, there is no need to perform any medical treatment if it does not keep growing or cause other problems (Durante et al., 2015). Indeed, less than 10% of patients’ thyroid nodules demonstrate disease progression after a median follow-up of 6 years (Ito et al., 2014). But the thyroid malignancy occurring in only 5–15% of thyroid nodules needed to be treated surgically (Wong et al., 2018). Therefore, to improve treatment efficiency, the main challenge is on how to differentiate the malignant nodules from the majority of benign ones reliably using the diagnostic methods (Cho et al., 2020; Singh Ospina et al., 2020).
Until now, to determine the risk of malignancy, fine-needle aspiration (FNA) with cytologic evaluation remains the diagnostic choice for ≥1.0 cm nodules (Heider et al., 2020). But one-third of thyroid nodules could not be discriminated as benign or malignant correctly (Cibas and Ali, 2009). Over the past decade, molecular testing has developed rapidly to improve the diagnostic accuracy as well as minimize cost and unnecessary testing for indeterminate cases (Roth et al., 2018). Moreover, transcript profiling is a widely used technique to discover the molecular changes. Transcriptomics could obtain information simultaneously based on the abundance of multiple mRNA transcripts for the biological sample (Knyazeva et al., 2020; Moncada et al., 2020). So, the gene signatures based on transcriptomic data could be used to distinguish benign from malignant thyroid nodules efficiently.
Recently, there have been a lot of transcriptomic studies to identify the gene signatures associated with thyroid nodules. For example, Giordano et al. found the three genes (PPARG, AQP7, and ENO3) implicated for the neoplastic mechanism of thyroid follicular carcinomas (Giordano et al., 2006). Wojtas et al. confirmed differential expression of seven genes (CPQ, PLVAP, TFF3, ACVRL1, ZFYVE21, FAM189A2, and CLEC3B) between malignant and benign follicular thyroid tumors (Wojtas et al., 2017). Schulten et al. revealed 55 transcripts (GABBR2, NRCAM, ECM1, HS6ST2, RXRG, etc.) differentially expressed between follicular variant of papillary thyroid carcinomas and follicular adenomas of the thyroid (Schulten et al., 2015). Hinsch et al. detected that QPRT was a potential marker for the immunohistochemical screening of follicular thyroid nodules (Hinsch et al., 2009). Although there were various signatures identified in different studies, it was reported that they were difficult to be applied in clinical diagnosis because of the inconsistency and unreliability (Singh Ospina et al., 2020).
The inconsistency among gene signatures from different studies might result from many sources, such as limited number of samples (Schwalbe et al., 2017; Osborn et al., 2018). It is understood that these transcriptomic studies were performed using dozens of samples of thyroid nodules. If the multiple independent studies could be combined as one comprehensive dataset, the sample size could be enlarged and the stability of the gene signatures could be enhanced significantly (Mistry et al., 2013). Moreover, weighted gene co-expression network analysis (WGCNA) could be used to identify the modules of co-expressed genes highly associated with the biological mechanism (He et al., 2019). WGCNA has been widely used to explore biomarkers and therapeutic targets of various diseases (Niemira et al., 2019; Chen et al., 2020). Therefore, it was highly needed to identify key genes between malignant and benign thyroid nodules by WGCNA from a comprehensive dataset.
In this work, five independent transcriptomic studies comprising 150 malignant and 93 benign thyroid nodule samples were combined to discover the gene signatures of thyroid nodules. First, 279 differentially expressed genes (DEGs) were identified by the feature selection method (Student’s t test and fold change) after data preprocessing and batch effect removal. And various biological process terms (such as hormone metabolic process, platelet degranulation, and thyroid hormone generation) were enriched using these DEGs. Second, the WGCNA model was constructed to identify significant modules of highly co-expressed genes, and 454 hub genes in the gray module were identified. Third, the intersection between DEGs identified by the feature selection method and the hub genes using the WGCNA model was discovered as the key genes. In order to perform the systematic validation, four key genes participated in the pathogenesis of malignant thyroid nodules were validated by an independent dataset. Finally, a high-performance classification model for discriminating benign and malignant thyroid nodules was constructed using these key genes. All in all, this study might provide a useful classification model for discriminating benign and malignant thyroid nodules.
Materials and Methods
Collection of Transcriptomic Data From Multiple Studies
A variety of microarray studies based on thyroid tissue were collected by searching the key word “thyroid nodules” in the Gene Expression Omnibus (GEO) database (Barrett et al., 2013). These collected datasets should meet the following criteria (Yang et al., 2020b): 1) the gene expression profiling was conducted using cDNA microarray for “Homo Sapiens”; 2) the tissues analyzed were thyroid nodules; 3) raw data could be available for further analysis; and 4) the collected datasets should consist of one group of malignant samples and another group of benign ones. As a result, five independent transcriptomic datasets were collected, and each comprised both benign and malignant thyroid nodules. The detailed information of these five collected datasets is provided in Table 1, including dataset ID, number of samples, microarray platform, and tissue indicated in the original publication and references.
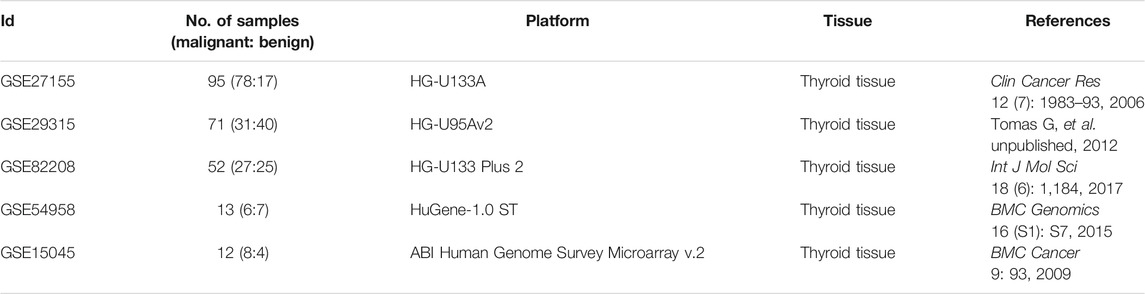
TABLE 1. Datasets collected from five independent microarray studies of thyroid nodules (sorted by sample size). Each dataset contained one cohort of malignant and another cohort of another group of benign samples.
Data Preprocessing and Batch Effect Removal
To enhance the consistency and classification capacity, all datasets in this study (Table 1) were combined to discover the key genes of thyroid nodules. The combination of multiple datasets was carried out in R environment (v3.4.3, http://www.r-project.org) (Sepulveda, 2020). The raw data (CEL file) of all datasets were read, log-transformed, and normalized using the corresponding R package, and all parameters were set as default. All probe sets were then mapped to their corresponding gene names using Bioconductor (Tippmann, 2015). The average expression value was retained if one gene was mapped to multiple probes (Yang et al., 2020c). To remove batch effects among five independent datasets, Z-score transformation was used to adjust the gene expression levels in each dataset (Yang Q et al., 2019b; Yang et al., 2020a). Z-score transformation for each gene could be computed by subtracting the mean of all genes and dividing the difference by the standard deviation of all genes in one experiment. After data transformation, the mean value for each experiment became zero with standard deviation equaling one.
Differentially Expressed Genes Discovered Between Benign and Malignant Thyroid Nodules
In this study, there were five collected datasets integrated as a comprehensive dataset for discovering signatures. This comprehensive dataset consisted of 150 malignant and 93 benign samples of thyroid nodules. To the best of one’s knowledge, this integrated dataset was the largest transcriptomic dataset in the analysis of thyroid nodules. Based on this comprehensive dataset, the DEGs were discovered using feature selection methods including Student’s t test and fold change (FC). For Student’s t test, multtest package of R language was applied, and the adjusted p-value < 0.05 was selected as the cutoff (Yan et al., 2019). The fold change was used to compare the mean expression of each gene between malignant and benign thyroid nodules (Yu et al., 2020). The cutoff level of FC was set to logFC >0.58 (FC > 1.5) or logFC < -0.58 (FC < 0.67). The equation of FC was shown below (as shown in Eq. (1)).
The volcano plot was applied to visualize and demonstrate the DEGs using ggplot2 package. Then the analysis of gene ontology (GO) enrichment was performed to identify the key biological processes for thyroid nodules (Yang et al., 2019a). Moreover, GOplot and clusterProfiler packages were used for visualizing the biological processes (BP) of GO enrichment (Yu et al., 2012; Yang et al., 2021). The raw p-value < 0.05 of GO terms was considered statistically significant.
Hub Genes Identified Using Weighted Gene Co-Expression Network Analysis
The WGCNA package was applied to establish the scale-free weight gene co-expression networks for thyroid nodules (Langfelder and Horvath, 2008). The unqualified genes were screened out, and the matrix of genes’ similarity by Pearson’s correlation analysis was created. Appropriate soft threshold power (β) was applied to strengthen this matrix to a scale-free co-expression network (Yang et al., 2020b). The lowest power was chosen, so the scale-free topology fit index curve flattened out upon reaching a high value. The highly correlated genes were assigned into the same module. As a result, the intersection was obtained between DEGs identified by the feature selection method and hub genes in a key module using the WGCNA model. These genes in the intersection were regarded as the key genes for further validation.
Validation of the Key Genes Based on the Independent Dataset
A systematic validation was conducted by evaluating the upregulated and downregulated genes based on the independent dataset (GSE34289) (Alexander et al., 2012). This validation dataset consisted of two independent datasets from two different platforms. The first independent dataset was detected based on GPL5175 platform (Affymetrix Human Exon 1.0 ST Array). In this dataset, there were 23 malignant and 26 benign thyroid nodules. The second independent dataset was detected based on GPL14961 platform (Afirma-T Human Custom Array). There were 120 malignant and 198 benign samples in this second independent dataset. In this study, the boxplot was used to demonstrate the differential expression of these key genes between malignant and benign thyroid nodules.
Construction of the High-Performance Classification Model Using the Key Genes
To construct a classification model for thyroid nodules, four powerful classifiers, namely, support vector machine, linear discriminate analysis, partial least squares, and random forest algorithm, were applied in this study (Orru et al., 2012). The key genes between malignant and benign thyroid nodules were used to discriminate different samples. In the first step, the five-fold cross validation of the comprehensive dataset (Table 1) was performed to validate the performance of this classification model. The accuracy of five-fold cross validation could reflect the quality of the model. In the second step, the comprehensive dataset was set as the training set, and the two independent datasets from GSE34289 were set as the test sets. The performance of the independent test set could accurately reflect the classification ability of the model. This high-performance classification model based on machine learning was constructed for discriminating benign and malignant thyroid nodules.
Results and Discussion
Collection of Multiple Transcriptomic Data for Thyroid Nodules
A variety of microarray studies based on thyroid tissue were collected by searching the key word “thyroid nodules” in the GEO database. As a result, five independent transcriptomic studies were obtained, and each comprised a cohort of malignant samples and another cohort of benign samples. The detailed information of these independent datasets is provided in Table 1. Among these studies, the five datasets including 150 malignant and 93 benign thyroid nodules were combined as a comprehensive dataset. The boxplots of five datasets before and after batch effect removal are shown in Supplementary Figure S1. The intensity of all samples before batch effect removal was distributed in the range of 4–15 and fluctuated greatly. After batch effect removal, the intensity of all samples was roughly distributed in the range of -1–1. The stable distribution indicated that the batch effects were well removed in the combined dataset by Z-score transformation. After data preprocessing and batch effect removal, the comprehensive dataset with 7,265 genes from five independent studies was applied to discover the key genes of thyroid nodules.
DEGs of Thyroid Nodules Identified Using the Combined Dataset
Based on this comprehensive dataset, the DEGs were discovered using feature selection methods (both Student’s t test and fold change). The volcano plot (as shown in Figure 1) illuminated the variation of DEGs in malignant versus benign thyroid nodules. The horizon line was the cutoff (adjusted p-value < 0.05) of Student’s t test. The cutoff levels for the vertical line were set to logFC >0.58 (FC > 1.5) or logFC < -0.58 (FC < 0.67) of fold change. The blue and red dots were used to indicate the upregulated (logFC >0.58) and downregulated (logFC < -0.58) genes, respectively. In this study, 279 DEGs were finally identified by both Student’s t test and fold change. The total number of upregulated genes (172 genes) was larger than that of the downregulated ones (107 genes). The top 25 upregulated and downregulated DEGs are shown in Table 2, including the information of entrez ID, gene symbol, adjusted p-value, and fold change for each gene. The information of all DEGs is shown in Supplementary Table S1.
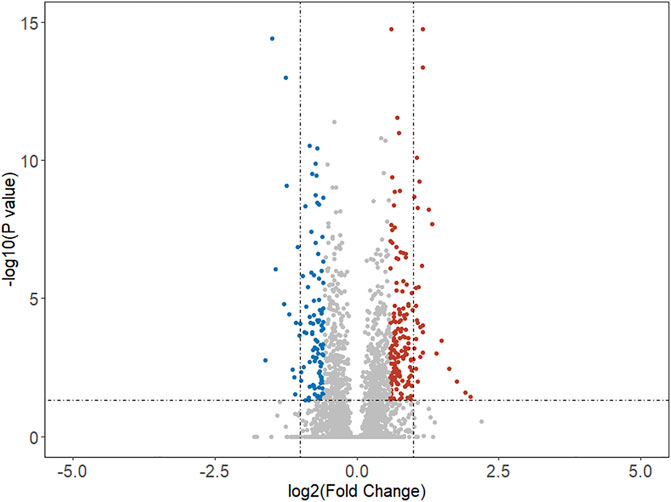
FIGURE 1. Volcano map of differentially expressed genes in malignant samples compared with benign samples. The horizon line was the cutoff (adjusted p-value < 0.05) of Student’s t test. The vertical line was the cutoff (logFC >0.58 or logFC < -0.58) of the fold change method. The blue and red dots indicated the downregulated and upregulated genes, respectively.
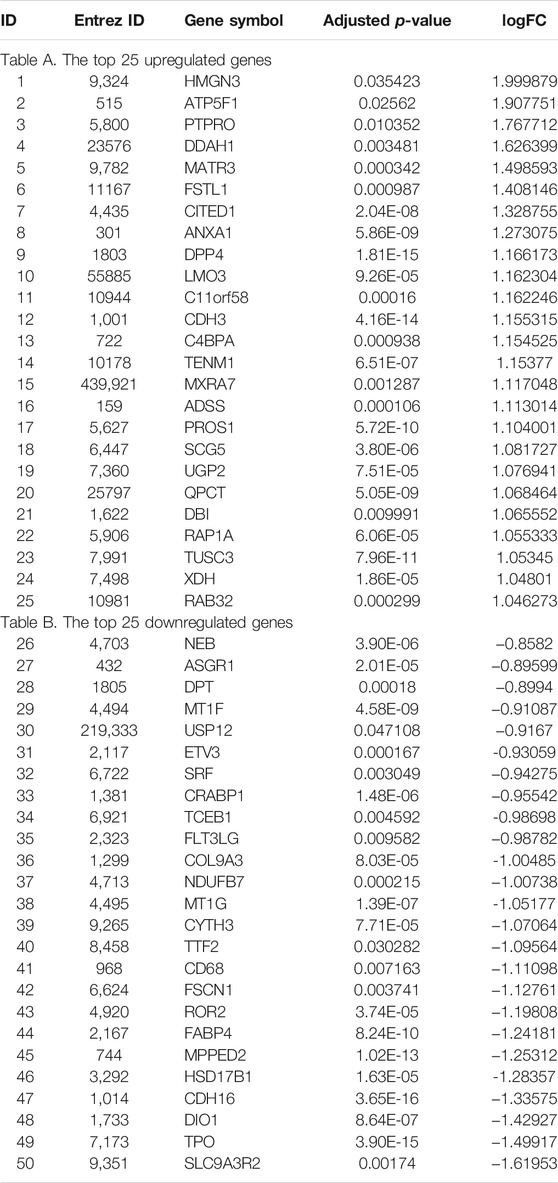
TABLE 2. Top 25 up- and downregulated DEGs identified by Student’s t test and fold change method (logFC >0.58 or logFC < -0.58 and adjusted p-value < 0.05) combining all five datasets in Table 1.
GO Enrichment Analysis Using DEGs of Thyroid Nodules
GO enrichment analysis is ubiquitously used for interpreting high throughput molecular data and underlying biological phenomena of experiments (Tomczak et al., 2018). For a set of genes, an enrichment analysis will find which GO terms are overrepresented using annotations for the gene set. GO enrichment analysis for the DEGs was performed in this study. Using the DEGs between malignant and benign thyroid nodules, the enrichment analysis included the BP (biological process), MF (molecular function), and CC (cell component) terms. The detailed information of GO ID, description, p-value, name, and the number of genes is shown in Supplementary Table S2.
Particularly, multiple biological processes were enriched to interpret the biological mechanism of malignant thyroid nodules. The chord diagram of BP enrichment (as interpreted in Figure 2) was applied to explain the relationship between DEGs and BP terms. It was reported that these BP terms were associated with the biological mechanism of thyroid nodules. For example, there were 15 DEGs enriched in the hormone metabolic process, and the association with thyroid cancer has been reported (Han et al., 2018). The platelet degranulation enriched by 10 DEGs was discovered in papillary thyroid carcinoma using the biomarkers (Wu et al., 2018). The concentration of the vascular endothelial growth factor was increased and stimulated endothelial cell proliferation in the cyst fluid of enlarging and recurrent thyroid nodules (Sato et al., 1997). It was reported that patients with spotty skin pigmentation had a predisposition toward the development of thyroid abnormalities (Courcoutsakis et al., 2009). It was found that low thyroid hormones might have implications for reproductive health, so the reproductive structure development and reproductive system development might be affected in thyroid nodules (Medda et al., 2017). The thyroid hormone generation reported that the significant biologic process was involved in thyroid cancers (Durante et al., 2018).
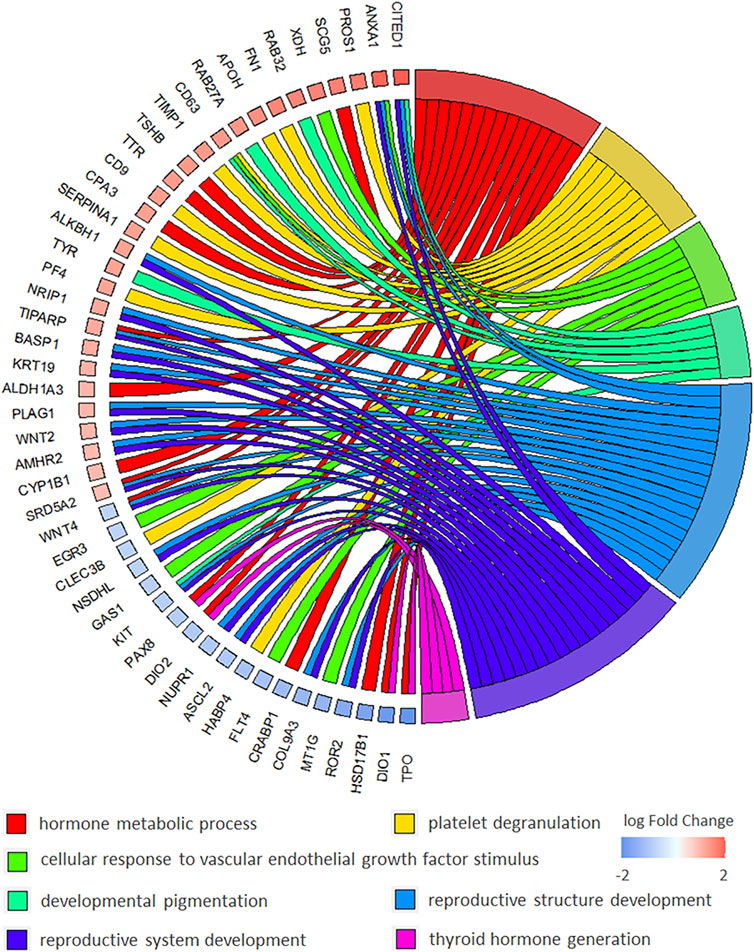
FIGURE 2. Chord diagram of BP (biological process) of GO enrichment to explain the relationship between BP terms and DEGs in malignant versus benign thyroid nodules.
Construction of the WGCNA Network and Identification of the Gene Co-Expression Module
The WGCNA network was constructed to identify the gene co-expression module (as shown in Figure 3). The value of power (10) was selected as the soft-threshold power to ensure scale-free (R2 = 0.8) networks using the WGCNA package (Figure 3A) because it reached the plateau at power 10 from the scale-free topology plot and mean connectivity plot. Genes with similar expression patterns were clustered into co-expression modules. Different modules were shown in different colors, and 13 modules were identified totally (Figure 3B). The heatmap of module–trait relationships was applied for depicting correlations between module eigengenes and phenotypic traits (the label of malignant and benign thyroid nodules). As shown in Figure 3C, the numbers correspond to the correlation, and the p-values were set in parentheses. Moreover, the degree of correlation was illustrated with the color legend. Here, the gray module was the most correlated one with malignant thyroid nodules (R = 0.32, p-value = 2 × 10–5). Hence, the gray module was used for the identification of the hub genes. Hub genes in the co-expression network were characterized by high intra-modular connectivity measured by the value of gene significance and module membership. The scatterplot of module eigengenes related to malignant thyroid nodules in the gray co-expression module (R = 0.29, p-value = 3 × 10–10) is shown in Figure 3D. As a result, 454 genes in the gray module highly correlated with gene significance were identified as hub genes using WGCNA.
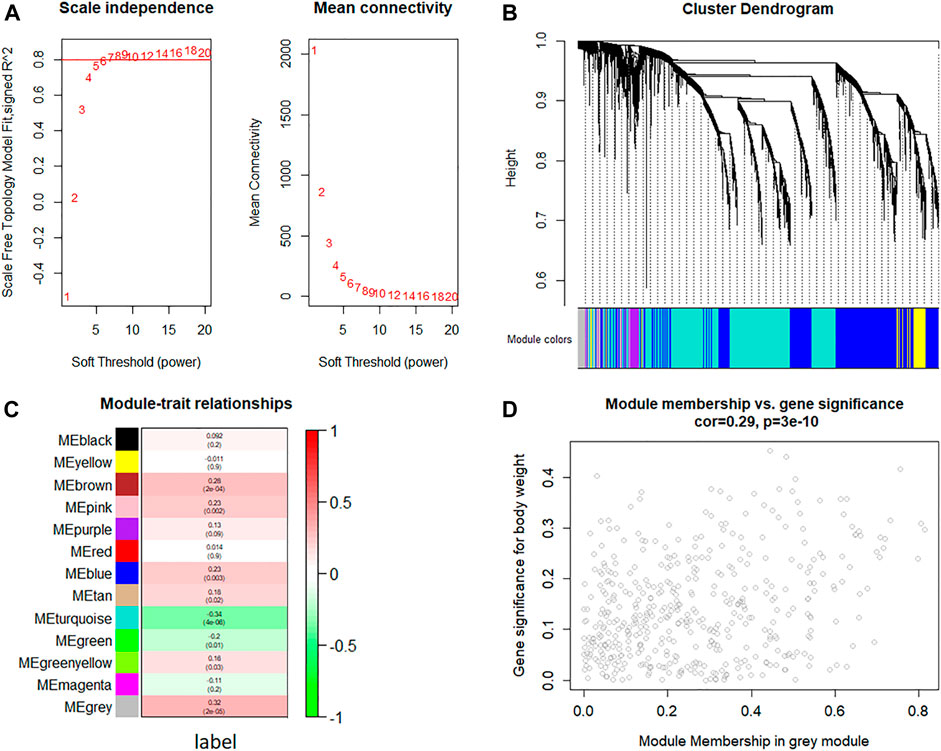
FIGURE 3. Weighted gene co-expression network analysis of gene expression between malignant and benign thyroid nodules. (A) Analysis of the scale-free topology fit index and the mean connectivity for various soft threshold powers (β) for the genes, (B) dendrogram of all expressed genes clustered based on a dissimilarity measure, (C) heatmap of module–trait relationships depicting correlations between module eigengenes and phenotypic traits (the label of malignant and benign thyroid nodules). Numbers correspond to the correlation and the p-value in parentheses. The degree of correlation is illustrated with the color legend, and (D) identification of hub genes using the scatterplot of module eigengenes in the gray co-expression module.
Validation of the Key Genes Using the Independent Datasets
In this study, there were 19 overlapping genes in the intersection between 279 DEGs identified by the feature selection method and 454 hub genes in the gray module totally. To validate these overlapping genes, two independent datasets from GSE34289 were applied to perform the systematic validation (Alexander et al., 2012). In this validation dataset, there were 23 malignant with 26 benign samples and 120 malignant with 198 benign samples form GPL5175 and GPL14961 platforms, respectively. The boxplots (as shown in Figure 4) were used to demonstrate the key genes between malignant and benign thyroid nodules. Among the 19 overlapping genes, there were four key genes expressed in the independent dataset, and the dysregulation of these key genes was validated. As shown in Figure 4, the significant differences of three upregulated genes (ST3GAL5, NRCAM, and MT1F) and one downregulated gene (PROS1) were indicated in these boxplots obviously for the independent data detected from GPL5175 (Figure 4A) and GPL14961 platforms (Figure 4B), respectively.
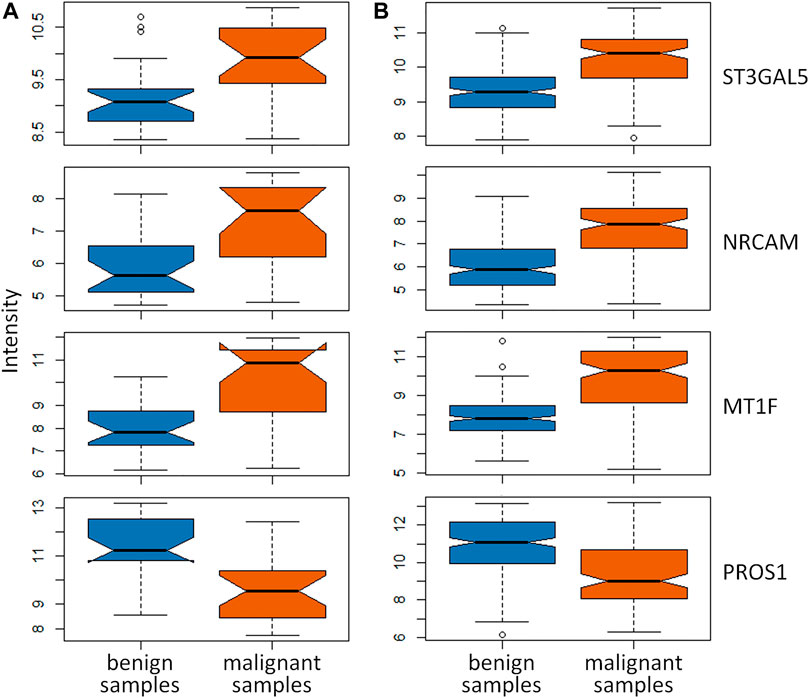
FIGURE 4. Validation of key genes identified by both DEGs identified by the feature selection method and hub genes in the gray module using WGCNA. The boxplots of these key genes between malignant and benign thyroid nodules were validated in the independent dataset detected by (A) GPL5175 and (B) GPL14961 platforms.
As a result, these four key genes were effectively validated as the important ones participated in the pathogenesis of thyroid nodules. It was reported that the specific genetic variants of ST3GAL5 in patients with thyroid-associated ophthalmopathy were discovered (Park et al., 2017). Górka et al. provided the first evidence that NRCAM is overexpressed in papillary thyroid carcinomas, and the upregulation of NRCAM was implicated in the pathogenesis and behavior of papillary thyroid cancers (Gorka et al., 2007). It was reported that MT1F might contribute to thyroid carcinogenesis and potentially serve as a diagnostic marker in distinguishing benign from malignant lesions (Kim et al., 2010; Wojtczak et al., 2017). In the previous studies, PROS1 was reported as the biomarker significantly related to thyroid nodules’ malignancy (Griffith et al., 2006; Wu et al., 2020). In this study, these four key genes (ST3GAL5, NRCAM, MT1F, and PROS1) were discovered for distinguishing malignant from benign thyroid nodules.
Construction of the High-Performance Classification Model Using the Key Genes
To distinguish malignant from benign thyroid nodules, four popular machine learning methods were applied to construct the classification model in this study. These methods included support vector machine, linear discriminate analysis, partial least squares, and random forest algorithm. The key genes between benign and malignant thyroid nodules were used to discriminate different samples. For the comprehensive dataset in Table 1, the five-fold cross validation was first performed to validate the performance of this classification model. As shown in Figure 5A1, 5B1, 5C1, and 5D1, the values of area under the ROC curve (AUC) were 0.83, 0.82, 0.82, and 0.78 for the five-fold cross validation using four different machine learning methods, respectively. Moreover, the high performance of the independent test sets could accurately reflect the ability of the classification model. The comprehensive dataset was set as the training set, and the test sets consisted of two parts detected by GPL5175 and GPL14961 platforms from the independent dataset (GSE34289). As displayed in Figure 5A2, 5B2, 5C2, and 5D2, the AUC values of the ROC curve for the first independent test set were 0.83, 0.67, 0.74, and 0.74 by four machine learning methods, respectively. As shown in Figure 5A3, 5B3, 5C3, and 5D3, the AUC values for the second independent test set were 0.81, 0.60, 0.69, and 0.77 by four machine learning methods, respectively.
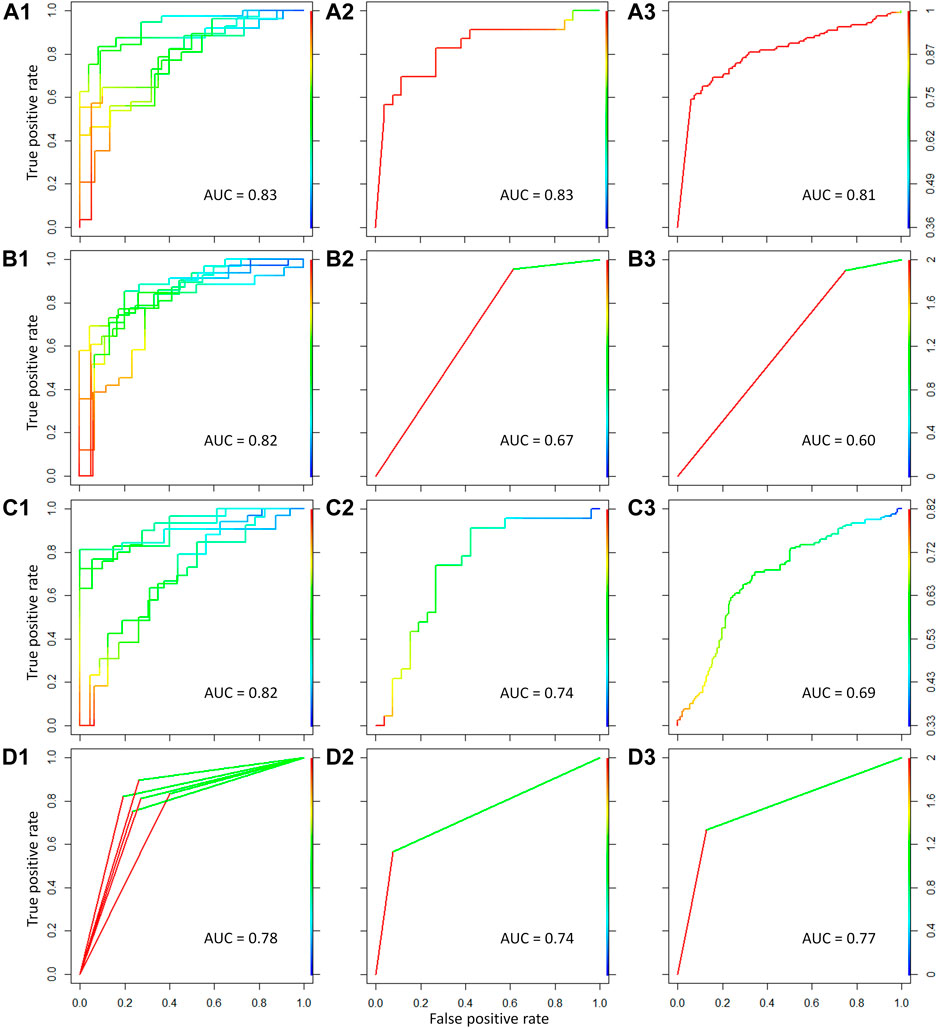
FIGURE 5. Classification model constructed for discriminating malignant from benign thyroid nodules using four different machine learning methods. The four methods referred to support vector machine, linear discriminate analysis, partial least squares, and random forest algorithm from top to bottom. The ROC curves and AUC values for the five-fold cross validation were shown in (A1–D1) for the comprehensive dataset using the four methods. The ROC curves and AUC values for the first independent test set were shown in (A2–D2). The ROC curves and AUC values for the second independent test set were shown in (A3–D3).
As shown in Figure 5, for the five-fold cross validation, the performances (AUC >0.8) of the classification model were outstanding using support vector machine, linear discriminate analysis, and partial least squares. However, the classification models of support vector machine and random forest (AUC >0.7) have shown more excellent performances than the other methods for the two independent test sets. Therefore, the high-performance classification model using support vector machine was recommended for discriminating malignant from benign thyroid nodules based on both five-fold cross validation and independent test.
Until now, it fails to discriminate as benign or malignant in one-third of thyroid nodules using FNA with cytologic evaluation. To save medical costs and improve the diagnostic accuracy, the high-performance classification model constructed in this study could be applied before FNA. For the thyroid nodule patients, the expression of four key genes could be detected. Then, this sample could be classified as benign or malignant thyroid nodules based on the classification model. If the patient was classified as a malignant thyroid sample, it was highly necessary to make a definite diagnosis using FNA with cytologic evaluation. If the patient was classified as a benign sample based on the classification model, the necessity of the FNA could be determined depending on the specific conditions. In the future, selection method, the high-performance classification model is expected to be applied for clinical diagnosis and management for malignant and benign thyroid nodules.
Conclusion
In this study, a comprehensive dataset including 150 malignant and 93 benign samples was collected to discover the gene signature of thyroid nodules. Then, 279 DEGs were identified by the feature selection method (Student’s t test and fold change). Then, the WGCNA network was performed to identify modules of highly co-expressed genes, and 454 genes were discovered as the hub genes. As a result, the intersection between the DEGs and the hub genes was identified as the key genes. Using the independent dataset, three upregulated genes (ST3GAL5, NRCAM, and MT1F) and one downregulated gene (PROS1) were effectively validated. Moreover, the high-performance classification model was constructed for discriminating malignant from benign thyroid nodules. However, certain limitations still exist in this study. The number of samples for identifying and validating key genes was still needed to be increased. In the future, the key genes and classification model could be further verified based on the experimental data.
Data Availability Statement
The original contributions presented in the study are included in the article/Supplementary Material; further inquiries can be directed to the corresponding author.
Author Contributions
YG designed research. QY performed research and wrote the scripts. QY and YG wrote the manuscript.
Funding
This work was funded by the National Natural Science Foundation of Jiangsu (BK20210597) and the NUPTSF (Grant No. NY220169).
Conflict of Interest
The authors declare that the research was conducted in the absence of any commercial or financial relationships that could be construed as a potential conflict of interest.
Publisher’s Note
All claims expressed in this article are solely those of the authors and do not necessarily represent those of their affiliated organizations, or those of the publisher, the editors, and the reviewers. Any product that may be evaluated in this article, or claim that may be made by its manufacturer, is not guaranteed or endorsed by the publisher.
Supplementary Material
The Supplementary Material for this article can be found online at: https://www.frontiersin.org/articles/10.3389/fgene.2021.791349/full#supplementary-material
References
Alexander, E. K., Kennedy, G. C., Baloch, Z. W., Cibas, E. S., Chudova, D., Diggans, J., et al. (2012). Preoperative Diagnosis of Benign Thyroid Nodules with Indeterminate Cytology. N. Engl. J. Med. 367, 705–715. doi:10.1056/NEJMoa1203208
Barrett, T., Wilhite, S. E., Ledoux, P., Evangelista, C., Kim, I. F., Tomashevsky, M., et al. (2013). NCBI GEO: Archive for Functional Genomics Data Sets-Update. Nucleic Acids Res. 41, D991–D995. doi:10.1093/nar/gks1193
Burman, K. D., and Wartofsky, L. (2015). Thyroid Nodules. N. Engl. J. Med. 373, 2347–2356. doi:10.1056/NEJMcp1415786
Chen, M., Yan, J., Han, Q., Luo, J., and Zhang, Q. (2020). Identification of Hub‐methylated Differentially Expressed Genes in Patients with Gestational Diabetes Mellitus by Multi‐omic WGCNA Basing Epigenome‐wide and Transcriptome‐wide Profiling. J. Cel Biochem. 121, 3173–3184. doi:10.1002/jcb.29584
Cho, Y. Y., Park, S. Y., Shin, J. H., Oh, Y. L., Choe, J.-H., Kim, J.-H., et al. (2020). Highly Sensitive and Specific Molecular Test for Mutations in the Diagnosis of Thyroid Nodules: a Prospective Study of BRAF-Prevalent Population. Ijms 21, 5629. doi:10.3390/ijms21165629
Cibas, E. S., and Ali, S. Z. (2009). The bethesda System for Reporting Thyroid Cytopathology. Thyroid 19, 1159–1165. doi:10.1089/thy.2009.0274
Courcoutsakis, N., Patronas, N., Filie, A. C., Carney, J. A., Moraitis, A., and Stratakis, C. A. (2009). Ectopic Thymus Presenting as a Thyroid Nodule in a Patient with the Carney Complex. Thyroid 19, 293–294. doi:10.1089/thy.2008.0404
Durante, C., Costante, G., Lucisano, G., Bruno, R., Meringolo, D., Paciaroni, A., et al. (2015). The Natural History of Benign Thyroid Nodules. JAMA 313, 926–935. doi:10.1001/jama.2015.0956
Durante, C., Grani, G., Lamartina, L., Filetti, S., Mandel, S. J., and Cooper, D. S. (2018). The Diagnosis and Management of Thyroid Nodules. JAMA 319, 914–924. doi:10.1001/jama.2018.0898
Giordano, T. J., Au, A. Y. M., Kuick, R., Thomas, D. G., Rhodes, D. R., Wilhelm, K. G., et al. (2006). Delineation, Functional Validation, and Bioinformatic Evaluation of Gene Expression in Thyroid Follicular Carcinomas with the PAX8-PPARG Translocation. Clin. Cancer Res. 12, 1983–1993. doi:10.1158/1078-0432.CCR-05-2039
Górka, B., Skubis-Zegadło, J., Mikula, M., Bardadin, K., Paliczka, E., and Czarnocka, B. (2007). NrCAM, a Neuronal System Cell-Adhesion Molecule, Is Induced in Papillary Thyroid Carcinomas. Br. J. Cancer 97, 531–538. doi:10.1038/sj.bjc.6603915
Griffith, O. L., Melck, A., Jones, S. J. M., and Wiseman, S. M. (2006). Meta-analysis and Meta-Review of Thyroid Cancer Gene Expression Profiling Studies Identifies Important Diagnostic Biomarkers. Jco 24, 5043–5051. doi:10.1200/JCO.2006.06.7330
Han, L.-o., Li, X.-y., Cao, M.-m., Cao, Y., and Zhou, L.-h. (2018). Development and Validation of an Individualized Diagnostic Signature in Thyroid Cancer. Cancer Med. 7, 1135–1140. doi:10.1002/cam4.1397
He, P., Mo, X.-B., Lei, S.-F., and Deng, F.-Y. (2019). Epigenetically Regulated Co-expression Network of Genes Significant for Rheumatoid Arthritis. Epigenomics 11, 1601–1612. doi:10.2217/epi-2019-0028
Heider, A., Arnold, S., and Jing, X. (2020). Bethesda System for Reporting Thyroid Cytopathology in Pediatric Thyroid Nodules: Experience of a Tertiary Care Referral center. Arch. Pathol. Lab. Med. 144, 473–477. doi:10.5858/arpa.2018-0596-OA
Hinsch, N., Frank, M., Döring, C., Vorländer, C., and Hansmann, M.-L. (2009). QPRT: a Potential Marker for Follicular Thyroid Carcinoma Including Minimal Invasive Variant; a Gene Expression, RNA and Immunohistochemical Study. BMC Cancer 9, 93. doi:10.1186/1471-2407-9-93
Ito, Y., Miyauchi, A., Kihara, M., Higashiyama, T., Kobayashi, K., and Miya, A. (2014). Patient Age Is Significantly Related to the Progression of Papillary Microcarcinoma of the Thyroid under Observation. Thyroid 24, 27–34. doi:10.1089/thy.2013.0367
Jasim, S., Baranski, T. J., Teefey, S. A., and Middleton, W. D. (2020). Investigating the Effect of Thyroid Nodule Location on the Risk of Thyroid Cancer. Thyroid 30, 401–407. doi:10.1089/thy.2019.0478
Kim, H. S., Kim, D. H., Kim, J. Y., Jeoung, N. H., Lee, I. K., Bong, J. G., et al. (2010). Microarray Analysis of Papillary Thyroid Cancers in Korean. Korean J. Intern. Med. 25, 399–407. doi:10.3904/kjim.2010.25.4.399
Knyazeva, M., Korobkina, E., Karizky, A., Sorokin, M., Buzdin, A., Vorobyev, S., et al. (2020). Reciprocal Dysregulation of MiR-146b and MiR-451 Contributes in Malignant Phenotype of Follicular Thyroid Tumor. Ijms 21, 5950. doi:10.3390/ijms21175950
Langfelder, P., and Horvath, S. (2008). WGCNA: an R Package for Weighted Correlation Network Analysis. BMC Bioinformatics 9, 559. doi:10.1186/1471-2105-9-559
Medda, E., Santini, F., De Angelis, S., Franzellin, F., Fiumalbi, C., Perico, A., et al. (2017). Iodine Nutritional Status and Thyroid Effects of Exposure to Ethylenebisdithiocarbamates. Environ. Res. 154, 152–159. doi:10.1016/j.envres.2016.12.019
Mistry, M., Gillis, J., and Pavlidis, P. (2013). Genome-wide Expression Profiling of Schizophrenia Using a Large Combined Cohort. Mol. Psychiatry 18, 215–225. doi:10.1038/mp.2011.172
Moncada, R., Barkley, D., Wagner, F., Chiodin, M., Devlin, J. C., Baron, M., et al. (2020). Integrating Microarray-Based Spatial Transcriptomics and Single-Cell RNA-Seq Reveals Tissue Architecture in Pancreatic Ductal Adenocarcinomas. Nat. Biotechnol. 38, 333–342. doi:10.1038/s41587-019-0392-8
Niemira, M., Collin, F., Szalkowska, A., Bielska, A., Chwialkowska, K., Reszec, J., et al. (2019). Molecular Signature of Subtypes of Non-small-cell Lung Cancer by Large-Scale Transcriptional Profiling: Identification of Key Modules and Genes by Weighted Gene Co-expression Network Analysis (WGCNA). Cancers 12, 37. doi:10.3390/cancers12010037
Orrù, G., Pettersson-Yeo, W., Marquand, A. F., Sartori, G., and Mechelli, A. (2012). Using Support Vector Machine to Identify Imaging Biomarkers of Neurological and Psychiatric Disease: a Critical Review. Neurosci. Biobehavioral Rev. 36, 1140–1152. doi:10.1016/j.neubiorev.2012.01.004
Osborn, D., Burton, A., Hunter, R., Marston, L., Atkins, L., Barnes, T., et al. (2018). Clinical and Cost-Effectiveness of an Intervention for Reducing Cholesterol and Cardiovascular Risk for People with Severe Mental Illness in English Primary Care: a Cluster Randomised Controlled Trial. The Lancet Psychiatry 5, 145–154. doi:10.1016/S2215-0366(18)30007-5
Park, H. J., Kim, J. H., Yoon, J. S., Choi, Y. J., Choi, Y.-H., Kook, K. H., et al. (2017). Identification and Functional Characterization of ST3GAL5 and ST8SIA1 Variants in Patients with Thyroid-Associated Ophthalmopathy. Yonsei. Med. J. 58, 1160–1169. doi:10.3349/ymj.2017.58.6.1160
Roth, M. Y., Witt, R. L., and Steward, D. L. (2018). Molecular Testing for Thyroid Nodules: Review and Current State. Cancer 124, 888–898. doi:10.1002/cncr.30708
Sato, K., Miyakawa, M., Onoda, N., Demura, H., Yamashita, T., Miura, M., et al. (1997). Increased Concentration of Vascular Endothelial Growth Factor/Vascular Permeability Factor in Cyst Fluid of Enlarging and Recurrent Thyroid Nodules1. J. Clin. Endocrinol. Metab. 82, 1968–1973. doi:10.1210/jcem.82.6.3989
Schulten, H.-J., Al-Mansouri, Z., Baghallab, I., Bagatian, N., Subhi, O., Karim, S., et al. (2015). Comparison of Microarray Expression Profiles between Follicular Variant of Papillary Thyroid Carcinomas and Follicular Adenomas of the Thyroid. BMC Genomics 16, S7. doi:10.1186/1471-2164-16-S1-S7
Schwalbe, E. C., Lindsey, J. C., Nakjang, S., Crosier, S., Smith, A. J., Hicks, D., et al. (2017). Novel Molecular Subgroups for Clinical Classification and Outcome Prediction in Childhood Medulloblastoma: a Cohort Study. Lancet Oncol. 18, 958–971. doi:10.1016/S1470-2045(17)30243-7
Sepulveda, J. L. (2020). Using R and Bioconductor in Clinical Genomics and Transcriptomics. J. Mol. Diagn. 22, 3–20. doi:10.1016/j.jmoldx.2019.08.006
Singh Ospina, N., Iñiguez-Ariza, N. M., and Castro, M. R. (2020). Thyroid Nodules: Diagnostic Evaluation Based on Thyroid Cancer Risk Assessment. BMJ 368, l6670. doi:10.1136/bmj.l6670
Tomczak, A., Mortensen, J. M., Winnenburg, R., Liu, C., Alessi, D. T., Swamy, V., et al. (2018). Interpretation of Biological Experiments Changes with Evolution of the Gene Ontology and its Annotations. Sci. Rep. 8, 5115. doi:10.1038/s41598-018-23395-2
Wojtas, B., Pfeifer, A., Oczko-Wojciechowska, M., Krajewska, J., Czarniecka, A., Kukulska, A., et al. (2017). Gene Expression (mRNA) Markers for Differentiating between Malignant and Benign Follicular Thyroid Tumours. Ijms 18, 1184. doi:10.3390/ijms18061184
Wojtczak, B., Pula, B., Gomulkiewicz, A., Olbromski, M., Podhorska-Okolow, M., Domoslawski, P., et al. (2017). Metallothionein Isoform Expression in Benign and Malignant Thyroid Lesions. Ar 37, 5179–5185. doi:10.21873/anticanres.11940
Wong, R., Farrell, S. G., and Grossmann, M. (2018). Thyroid Nodules: Diagnosis and Management. Med. J. Aust. 209, 92–98. doi:10.5694/mja17.01204
Wu, C.-C., Lin, J.-D., Chen, J.-T., Chang, C.-M., Weng, H.-F., Hsueh, C., et al. (2018). Integrated Analysis of fine-needle-aspiration Cystic Fluid Proteome, Cancer Cell Secretome, and Public Transcriptome Datasets for Papillary Thyroid Cancer Biomarker Discovery. Oncotarget 9, 12079–12100. doi:10.18632/oncotarget.23951
Wu, D., Hu, S., Hou, Y., He, Y., and Liu, S. (2020). Identification of Potential Novel Biomarkers to Differentiate Malignant Thyroid Nodules with Cytological Indeterminate. BMC Cancer 20, 199. doi:10.1186/s12885-020-6676-z
Yan, H., Zheng, G., Qu, J., Liu, Y., Huang, X., Zhang, E., et al. (2019). Identification of Key Candidate Genes and Pathways in Multiple Myeloma by Integrated Bioinformatics Analysis. J. Cel Physiol. 234, 23785–23797. doi:10.1002/jcp.28947
Yang, Q., Hong, J., Li, Y., Xue, W., Li, S., Yang, H., et al. (2020a). A Novel Bioinformatics Approach to Identify the Consistently Well-Performing Normalization Strategy for Current Metabolomic Studies. Brief. Bioinform. 21, 2142–2152. doi:10.1093/bib/bbz137
Yang, Q., Li, B., Chen, S., Tang, J., Li, Y., Li, Y., et al. (2021). MMEASE: Online Meta-Analysis of Metabolomic Data by Enhanced Metabolite Annotation, Marker Selection and Enrichment Analysis. J. Proteomics 232, 104023. doi:10.1016/j.jprot.2020.104023
Yang, Q., Li, B., Tang, J., Cui, X., Wang, Y., Li, X., et al. (2020b). Consistent Gene Signature of Schizophrenia Identified by a Novel Feature Selection Strategy from Comprehensive Sets of Transcriptomic Data. Brief. Bioinform. 21, 1058–1068. doi:10.1093/bib/bbz049
Yang, Q., Wang, Y., Zhang, S., Tang, J., Li, F., Yin, J., et al. (2019a). Biomarker Discovery for Immunotherapy of Pituitary Adenomas: Enhanced Robustness and Prediction Ability by Modern Computational Tools. Ijms 20, 151. doi:10.3390/ijms20010151
Yang, Q., Wang, Y., Zhang, Y., Li, F., Xia, W., Zhou, Y., et al. (2020c). NOREVA: Enhanced Normalization and Evaluation of Time-Course and Multi-Class Metabolomic Data. Nucleic Acids Res. 48, W436–W448. doi:10.1093/nar/gkaa258
Yang, Q. X., Wang, Y. X., Li, F. C., Zhang, S., Luo, Y. C., Li, Y., et al. (2019b). Identification of the Gene Signature Reflecting Schizophrenia's Etiology by Constructing Artificial Intelligence‐based Method of Enhanced Reproducibility. CNS Neurosci. Ther. 25, 1054–1063. doi:10.1111/cns.13196
Yu, G., Wang, L.-G., Han, Y., and He, Q.-Y. (2012). clusterProfiler: an R Package for Comparing Biological Themes Among Gene Clusters. OMICS: A J. Integr. Biol. 16, 284–287. doi:10.1089/omi.2011.0118
Keywords: classification model, key genes, transcriptomics, combined analysis, thyroid nodules
Citation: Yang Q and Gong Y (2022) Construction of the Classification Model Using Key Genes Identified Between Benign and Malignant Thyroid Nodules From Comprehensive Transcriptomic Data. Front. Genet. 12:791349. doi: 10.3389/fgene.2021.791349
Received: 08 October 2021; Accepted: 06 December 2021;
Published: 14 January 2022.
Edited by:
Fengfeng Zhou, Jilin University, ChinaReviewed by:
Ravi Pandey, Jackson Laboratory for Genomic Medicine, United StatesSumeet Gulati, International Clinical Research Center (FNUSA-ICRC), Czechia
Copyright © 2022 Yang and Gong. This is an open-access article distributed under the terms of the Creative Commons Attribution License (CC BY). The use, distribution or reproduction in other forums is permitted, provided the original author(s) and the copyright owner(s) are credited and that the original publication in this journal is cited, in accordance with accepted academic practice. No use, distribution or reproduction is permitted which does not comply with these terms.
*Correspondence: Yaguo Gong, Z29uZ3lnbGFiQGdtYWlsLmNvbQ==