- 1Dale Bumpers National Rice Research Center, United States Department of Agriculture—Agricultural Research Service, Stuttgart, AR, United States
- 2Plant Science Research Unit, United States Department of Agriculture—Agricultural Research Service, St. Paul, CO, United States
There is global concern that rice grains and foods can contain harmful amounts of arsenic (As), motivating breeders to produce cultivars that restrict As accumulation in grains to protect human health. Arsenic is also toxic to plants, with straighthead disorder (StHD), causing panicle sterility, being observed in rice. The genetic variation in StHD resistance suggests that plants have evolved mechanisms that reduce As toxicity, possibly via regulation of As uptake, transport, or detoxification/sequestration. Because these mechanisms could also underlie the wide (3- to 100-fold) differences in grain As concentration (grain-As) observed among diverse rice genotypes, it was hypothesized that some genes reduce both grain-As content and StHD susceptibility and may be detectable as co-located StDH and As quantitative trait loci (QTL). We used a machine-learning Bayesian network approach plus high-resolution genome-wide association study (GWAS) to identify QTL for grain-As and StHD resistance within the USDA Rice Minicore Collection (RMC). Arsenic enters roots through phosphorus (P) and silica (Si) transporters, As detoxification involves sulfur (S), and cell signaling to activate stress tolerance mechanisms is impacted by Si, calcium (Ca), and copper (Cu). Therefore, concentrations of Si, P, S, Ca, and Cu were included in this study to elucidate physiological mechanisms underlying grain-As and StHD QTL. Multiple QTL (from 9 to 33) were identified for each of the investigated As-associated traits. Although the QTL for StHD, Si, and grain-As did not overlap as heavily as our hypothesis predicted (4/33 StHD and 4/15 As QTL co-located), they do provide useful guidance to future research. Furthermore, these are the first StHD and Si QTL to be identified using high-density mapping, resulting in their being mapped to shorter, more precise genomic regions than previously reported QTL. The candidate genes identified provide guidance for future research, such as gene editing or mutation studies to further investigate the role of antioxidants and ROS scavenging to StHD resistance, as indicated by candidate genes around the commonly reported qStHD8-2 QTL. Other genes indicated for future study for improving grain-As and StHD include several multidrug and toxic compound extrusion (MATE) genes, F-box genes, and NIPs not documented to date to transport As.
1 Introduction
Arsenic (As) is naturally present in the environment, and trace amounts are found in all soil and groundwater, but it is toxic to animal and plant life and has been associated with various cancers, cardiovascular disease, and diabetes in humans (WHO/FAO Joint Expert Committee on Food Additives, 2010; FAO/WHO, 2013). The environmental occurrence of As concentrations differs geographically around the world due to different As contents of bedrock, and from manmade causes such as mining or use of arsenical solutions for tanning hides, for preserving wood, or as agricultural herbicides (Agency for Toxic Substances and Disease Registry, 2007). Plants acquire As through roots along with beneficial soil nutrients and accumulate it in vegetative tissues and seeds. While trace amounts of As may be expected in any plant product, concentrations of As in edible seeds is of higher concern in rice than other grains (Williams et al., 2007) primarily because rice is commonly grown in flooded paddies where the anaerobic soil conditions increase the availability of As (see reviews Abedi and Mojiri, 2020; Tang and Zhao, 2020b). Although rice produced in the United States has been shown safe for consumption by the general population, not all US Rice meets the more stringent requirements set for baby food (US Food and Drug Administration, 2016; US Food and Drug Administration, 2020). There is global interest in identifying and implementing strategies for reducing rice grain As concentrations (grain-As). In aerobic soils, As is predominantly present as arsenate where, like its chemical analogue phosphate, it is largely bound to metal oxides and unavailable for plant uptake. Under anaerobic (flooded) conditions, arsenate becomes chemically reduced to arsenite and is more available for plant uptake (Dixit and Hering, 2003). Because rice roots exude oxygen into the rhizosphere, some arsenate and phosphate remain available for plant uptake in flooded fields (Seyfferth et al., 2010). Arsenate enters plant roots through phosphate transporters (Meharg and Hartley-Whitaker, 2002; Cao et al., 2017; Ye et al., 2017), while arsenite travels into and through plants via Si transporters (Ma et al., 2008). Some soil microbes methylate inorganic As (i.e., arsenate and arsenite), converting it into less toxic organic As (oAs) forms such as dimethylarsinic acid (DMA), which also enters roots through the Lsi1 silicon transporter (Ma et al., 2008; Abedi and Mojiri, 2020; Tang and Zhao, 2020b). The predominant forms of As in rice grains are arsenite and DMA (Meharg et al., 2008; Meharg et al., 2009; Heitkemper et al., 2009).
One method proposed for reducing the amount of As in rice plants and grains is to produce the crop without a flood for part or all of its production period, production systems known in the United States as alternate wetting and drying (AWD) and furrow irrigation, respectively. Use of flooded paddies for rice production became preferred over millennia, however, because the flood water protects rice plants from weeds, insects, drought, temperature extremes, and some diseases, including rice blast. Because roots uptake As through phosphorus (P) and silica (Si) transporters, application of P (Begum et al., 2008; Choudhury et al., 2011) and Si fertilizers has also been evaluated as a mitigation strategy (Seyfferth and Fendorf, 2012; Matsumoto et al., 2015; Seyfferth et al., 2016, Seyfferth et al., 2018). Our research focuses instead on identifying rice genes and physiological factors that can reduce grain-As in a variety of production systems.
As exposure is known to reduce root and shoot growth (Heuschele et al., 2017; Kalita et al., 2018; Murugaiyan et al., 2019) and has long been associated with a physiological disorder in rice known as straighthead (StHD), so called because it is characterized by erect seed heads (panicles) upon maturity from poor seed set in often distorted spikelets. While direct evidence documenting the oAs DMA as the cause of StHD was only recently determined (Tang et al., 2020a), herbicides containing the synthetic oAs monosodium methanearsonate (MSMA) have been used for decades to induce StHD for the purpose of facilitating selection of StHD-resistant breeding progeny (Yan et al., 2005). Plant genes and mechanisms that reduce As uptake or enhance As detoxification by chelation and/or vacuolar sequestration would be expected to reduce both grain-As and the severity of As-induced plant stress, such as StHD.
Altering the Lsi1 transporter to reduce the root uptake of As may seem enticing, but alterations in this protein have been detrimental, substantially decreasing plant growth and grain yield as well as grain-As (Ma et al., 2008). Furthermore, of the quantitative trait loci (QTL) for grain-As reported to date (Norton et al., 2010; Norton et al., 2012a; Norton et al., 2014; Zhang et al., 2014; Yang et al., 2018; Frouin et al., 2019; Norton et al., 2019; Fernández-Baca et al., 2021; Liu et al., 2021), none have encompassed the Lsi1 locus, indicating that the wide natural variation observed for grain-As (3- to 100-fold differences reported by Norton et al. (2012b), Pinson et al. (2015), and Duan et al. (2017) is not caused by mutation in the Lsi1 gene. In contrast, grain-As QTL have co-located with the Lsi2 gene (Norton et al., 2019), which impacts the root-to-shoot transfer of Si and As (Ma et al., 2008), and to the ABCC1 gene (Norton et al., 2019) which reduces transport of As to grains by increasing vacuolar sequestration (Song et al., 2014). Heuschele et al. (2017) compared three rice cultivars with high grain-As with three low grain-As cultivars to see if their seedlings differed metabolically in response to arsenite. Data indicated that reduced grain-As concentrations were neither due to reduced root uptake (e.g., Lsi1) nor due to root-to-shoot transfer differences, but were instead associated with an increase in cysteine and glutathione (GSH) in the leaves, with cysteine being a key substrate for synthesis of GSH. With other studies showing that binding of As to either GSH or GSH-containing phytochelatins is required before As can be transported into vacuoles (Raab et al., 2004, Raab et al., 2005; Hossain et al., 2012; Song et al., 2014), there is a growing body of evidence on the importance of post-uptake metabolism in regulating grain-As. Because cysteine, GSH, and phytochelatins are sulfur (S)-based compounds, this further suggests that plants require sufficient sulfur to limit the accumulation of As in grains.
GSH is important not only for detoxification but also for stress tolerance. Like other causes of abiotic stress, As toxicity induces the production of reactive oxygen species (ROS) (Tripathi et al., 2013; Nath et al., 2014) which are themselves injurious to plants. The increased ability to scavenge ROS with antioxidants has been shown to reduce cell damage due to drought (Zhu et al., 2020; Panda et al., 2021) and would likely also decrease StHD severity in rice. GSH molecules protect cells by reducing accumulation of ROS (Larrainzar et al., 2014), and an increase in S was found to reduce Cd-induced toxicity in Brassica campestris (Anjum et al., 2008). It appears, then, that increasing S and GSH within a plant could reduce StHD severity in two ways, by increasing As-chelation and by increasing ROS scavenging. Other elements with known roles in induction of ROS scavenging include Si (Kim et al., 2017), Ca (Fichman and Mittler, 2021), and Cu (Ma et al., 2015).
The aim of the present study was to identify QTL and candidate genes that can enhance breeding efforts to limit the accumulation of As in rice grain (grain-As) and/or reduce the susceptibility of rice plants to As-induced stress, as reflected in StHD severity. Because genes that reduce the uptake or transport of As, as well as those that increase chelation and sequestration of As in vegetative tissues, could reduce both grain-As and StHD severity, our working hypothesis was that some QTL would be associated with reductions in both grain-As and StHD. Co-location with Si, P, S, Ca, or Cu QTL would direct candidate gene identification by revealing if the underlying gene altered upward As transport, sequestration, or ROS. While QTL for these individual traits have been identified in various populations, the present analysis of the traits together in one population improved the ability to evaluate trait-to-trait relationships and more precisely evaluate co-location among QTL to provide insight on underlying gene functions. The severity of the StHD disorder was used as a measure of susceptibility to As-induced stress. The concentration of Si in rice hulls (hull-Si) reflected differences in Si uptake and upward transport to panicles and grains, and grain concentrations of As, P, S, Ca, and Cu were used as a measure of element availability and upward transport.
2 Materials and Methods
2.1 Genetic Materials and Genotypic Data
Trait-to-trait relationships and QTL were identified using phenotypic and genotypic data on the USDA-ARS Rice Minicore Collection (RMC), a set of 202 pure line rice (Oryza sativa) accessions collected from 14 global rice-growing regions (Agrama et al., 2009). The RMC was previously used for GWAS using a marker map consisting of 156 PCR-based molecular markers distributed across the twelve rice chromosomes, including identification of hull-Si QTL (Bryant et al., 2011). Subsequent to that study, 173 RMC accessions were resequenced (Wang et al., 2016), and a marker map containing 3,200,320 (3.2 million) filtered, reliable SNPs was created and used for GWA mapping (Huggins et al., 2019). The present study accumulated phenotypic data on the subset of 167 RMC accessions having a high-density marker map listed in Supplementary Table S1 which includes accessions from six genetic subpopulations in rice, specifically 30 accessions classified as being from the aus (AUS) subpopulation, 54 being indica (IND), 29 temperate japonica (TEJ), 28 tropical japonica (TRJ), and 6 aromatic (ARO), with the remaining 20 accessions being admixtures of two or more subpopulation groups. With the AUS, IND, and AUS/IND admixtures being considered members of the indica subspecies (INDAUS), there were 89 INDAUS accessions. Similarly, the study set includes 66 accessions of the japonica subspecies, identified as TEJ, TRJ, or TEJ/TRJ admixtures.
2.2 Rice Minicore Data on Silica, Arsenic, Phosphorus, Sulfur, Calcium, and Copper From Prior Studies
Bryant et al. (2011) evaluated the hull silica concentrations of RMC accessions using rice harvested from 2 replications × 2 locations (Stuttgart, AR, and Beaumont, TX) then used least square means (LSMeans) along with a low-density marker map (164 DNA markers) to identify 12 putative hull-Si QTL. Raw sample data were obtained and reanalyzed for the present study. As a subset of the USDA Rice Core Collection, the RMC was evaluated by Pinson et al. (2015) for grain concentrations of 16 elements, including As, P, S, Ca, and Cu, using grains harvested from 2 replications × 2 years × 1 location (Beaumont, TX) from both flooded and unflooded fields. For the present GWA analyses, raw sample data from flooded plots were obtained for grain concentrations (mg kg−1) of As, P, S, Ca, and Cu, hereafter called grain-As, grain-P, and so forth. Details of sample production, separation of hulls and grains, sample digestions, and laboratory equipment and methods for determining element concentrations are in Bryant et al. (2011) and Pinson et al. (2015).
2.3 De Novo Evaluation for Straighthead Disorder Resistance
While some of the RMC accessions had been included in previous StHD QTL mapping studies (Li et al., 2016; Li et al., 2017), numerous RMC accessions had not yet been characterized for StHD. The RMC was evaluated for resistance to MSMA-induced StHD in the USDA-ARS Straighthead Nursery at Stuttgart, AR over 2 years, using two replications planted on May 5, 2015, and four replications planted on May 30, 2016. Growing rice accessions with red- or purple-colored bran in the Stuttgart, AR Straighthead Nursery is prohibited to prevent pollen outcrossing into nearby seed production fields. We therefore evaluated StHD on the 156 brown bran RMC accessions using 2-row plots drill-seeded with 3 g seed per 1.5 m row to ensure a dense plant stand, with 0.3 m between rows and plots. Plots were arranged in a randomized complete block design, with plots of a StHD-susceptible (“Cocodrie,” PI 606331, Linscombe et al., 2000) and resistant (“Zhe733,” PI 629016) cultivar inserted approximately every 10 plots in order to assure consistent StHD symptoms throughout the study area. The same RMC were similarly planted concurrently in an adjacent field area not treated with “MSMA,” hereafter called Native soil plots. Monosodium methanearsonate (MSMA) was applied on the day of planting at a rate of 6.7 kg ha−1 using Target® 6.6 (Luxembourg-Pamol, Inc., Houston, TX, United States) which corresponds with 1.6 kg As ha−1 and incorporated into the upper 15 cm of soil before planting. Fertilization of fields began a month prior to planting with incorporation of P (triple super phosphate, 20 kg P ha−1) and potassium (K) (muriate of potash, 56 kg K ha−1). Nitrogen fertilizer (112 kg N ha−1 as dry urea) was applied to the soil surface just before the permanent flood was established at the five-leaf stage. The flood was maintained throughout the growing season, until maturity of all plots, to ensure ideal conditions for StHD development. Weeds were controlled with 9.3 l ha−1 of propanil (3′,4′-dichloropropionanilide) mixed with 0.4 kg ha−1 of Quinclorac (3,7-dichloroquinoline-8-carboxylic acid; Facet®, BASF, Ludwigshafen, Germany) when the rice was at the four-leaf stage. The soil at the site is Dewitt silt loam (fine, smectitic, thermic, Typic Albaqualfs) (5% sand, 78% silt, 17% clay), and all fields in this study had been managed in a rice-soybean rotation for more than 20 years. With the Straighthead nursery area receiving a fresh application of MSMA prior to planting rice every other year, the total As (iAs + oAs) in the soil has increased over time to 13 ± 6 kg ha−1 (Maguffin et al., 2020) compared to 4 ± 2 kg As ha−1 in the Native soil field area (Norton et al., 2012b). Regardless of the increased soil As content, a fresh application of MSMA is required to ensure development of StHD disorder in the nursery plots.
The MSMA-treated and Native soil plots were rated for StHD at maturity, approximately 35–40 days after heading. Heading was defined as 50% of the plants per plot having at least 1 panicle at anthesis. Days to heading (DHD) is the number of days between planting and heading per plot, with DHDms denoting data from MSMA-treated plots, and DHDnt for data from Native soil plots. Straighthead severity was rated on a 0 to 9 scale based on a visual observation of floret sterility and panicle emergence from the flag leaf sheath, as described by Yan et al. (2005). The plant height (PHT) of three random plants per plot was measured also at maturity and averaged per plot. The PHT for this study was defined as the distance (cm) from the soil surface to the tip of the tallest leaf to accommodate the fact that panicles are often not fully exerted on MSMA-treated plants. As for DHD, PHTms denotes data from MSMA-treated plots, and PHTnt denotes data from the Native soil plots. The 2015 trial was planted using RMC accessions obtained from the USDA Genetic Stocks Oryza (GSOR) Collection (www.ars.usda.gov/GSOR); 2016 plantings used seed harvested from 2015 Native soil plots.
2.4 Statistical Analyses of Rice Minicore Collection Trait Data
Analyses of variance (ANOVAs) were conducted using the generalized linear mixed model (GLIMMIX) procedure in SAS version 9.4 (SAS Institute Inc., 2012). Least square means (LSmeans) were calculated using the GLIMMIX procedure, with replication considered as a random effect and the RMC accessions as fixed effects. For calculating LSmeans combined across environments (either locations or years), the statistical model considered environment and replications nested within the environment as random effects.
Trait distributions, summary statistics, and mean comparisons across the seven population panels were calculated in JMP 14 (SAS Institute Inc. 2018) using trait LSmeans. Best linear unbiased predictions (BLUPs) computed per trait from raw replication data were used for the Pearson correlations and Bayesian network analyses. After a review of the data distributions, it was decided to transform the grain element data to reduce the strong skewing of these trait datasets prior to GWA analyses. Raw element concentration data were log-transformed and new BLUPs calculated for GWAS.
2.5 Bayesian Network Analysis
Bayesian network learning is a multivariate probabilistic modeling which computes, through an iterative learning process, the relationship between random variables represented by “nodes” and probabilistic dependencies represented by “arrows” in a directed acyclic graph (DAG) (Pearl, 1988; Scutari, 2010). The DAG was computed using the R package “bnlearn” (Scutari, 2010), as described in more detail in Sharma et al. (2021). The Bayesian network (BN) model was trained with 10-fold cross validation to compute the DAG (Scutari, 2010). Each validation used a random 17 accessions as the validation set (VS = 17) with the rest of the RMC accessions serving as the training set (TS = 150). Trait BLUPs were computed anew using the lme4 R package (Bates et al., 2011), this time accounting for population structure by using a mixed-effect model with a simple nested family structure (Piepho and Williams, 2006) that considered subgroups in the RMC as blocks. The growth stage progression of the traits in the order of flowering (DHDnt), plant height (PHTnt), grain element compositions, and lastly StHD disease ratings (StHDms) was encoded in the BN model using blacklisted arcs to compute the DAG (Scutari 2010).
The “bnlearn” package learns by iteratively selecting and estimating the model. The procedure first uses feature selection to find “parents” and “children” relationships among traits in the Markov blanket by the Semi-Interleaved HITON PC algorithm. In our model, the dependence was analyzed using Student’s t-test for Pearson’s correlations with alpha at 0.1. The large values of alpha were used because it allows Markov blankets to initially involve traits that are weakly associated, so that they are not initially discarded. Next, the procedure uses structure learning to find the DAG by computing the conditional independence present in data with a score-based algorithm using a heuristic optimization technique. In each iteration, the candidate DAG is assigned a network score reflecting its goodness of fit, which the algorithm then attempts to maximize. Then across all iterations, the analysis selects the DAG that maximizes the Bayesian information criterion.
2.6 Rice Minicore Genome Wide Association Panels and Parameters
GWA was conducted using 7 different “panels” derived from the RMC data, including the full RMC population (“All”); four subpopulations AUS, IND, TEJ, and TRJ; and the two subspecies indica (INDAUS = IND + AUS + IND/AUS admixtures) and japonica (JAP = TEJ + TRJ + TEJ/TRJ admixtures). For each filtered panel, Tassel V.5 (Bradbury et al., 2007) was used to generate a centered identity by state (IBS) kinship matrix and to conduct principal component analysis (PCA). The number of principal components (PCs) included in the mixed linear model analysis of each panel was adjusted in the following manner to account for population structure within each panel. For GWAS of the full RMC, the first three PCs were used as covariates in the mixed linear model; two PCs were used with the INDAUS subspecies; 1 PC with the JAP subspecies and the IND subpopulation; and no PCs used for TEJ, TRJ, and AUS. Mixed linear models were performed in Tassel version 5 (Zhang et al., 2010) with the variance components estimated for each marker and no compression options.
2.7 Interpretation of Genome Wide Association Mapping Results
As described in Huggins et al. (2019), a script was used that identified associated chromosome regions from individual SNPs or groups of physically linked SNPs. Chromosome regions included 50 kb in both directions around each individual significant SNP and were extended to include nearby significant SNPs occurring within 200 kb. A “Peak SNP” was designated for each region, which corresponded to the SNP with the most significant p-value. The observed frequencies of the alleles present at the peak SNP as well as the allele effect value were outputted (Huggins et al., 2019). Quantile–quantile (QQ) and Manhattan plots were created using the R package qqman (Turner, 2014). SNPs with −log10(p) > 5 were considered significant, and increased stringency was applied during data interpretation as needed, such as when only one SNP in a region met the log10(p) = 5 threshold, or regions where all associated SNPs had rare alleles (in <6 accessions), in which cases QTL were not claimed in these results. Running GWA on multiple panels provides more opportunity to identify chromosomal regions containing QTL but also prevents identification of a single “most strongly associated” SNP per QTL since it is common for SNPs to not be polymorphic in all panels, causing panels to identify QTL via association with different SNPs. Following the long-held precedent of claiming the fewest number of genes fitting a genetic model, we declared a single QTL region rather than multiple QTL per trait when different panels identified different but closely linked SNPs, merging linked SNPs into a single QTL (or not) per the following criteria. When considering SNP peaks across panels, a single QTL was declared if the string of SNPs significantly associated with a trait in one or more panels and/or study environments did not have a gap >800 kb and the additive effects were consistent across the SNPs and panels (example, qStHD1-1 in Supplementary Table S2). A chromosomal region containing multiple associated SNPs was declared as two QTL when a gap >800 kb existed between associated SNPs (e.g., qStHD1-1 to qStHD1-2), or if there were no SNP gaps >800 kb, but the additive effect of the predominant allele changed from positive to negative within a string of associated SNPs (e.g., qSi10-2 to qSi10-3). QTL for different traits were considered to overlap if the SNPs significantly associated with the two traits were interspersed with each other or if the ends of the QTL regions were ≤500 kb apart.
2.8 Confirmation of Targeted SNPs Using Pivot Tables
The multiple SNPs identified by GWA analyses for each QTL region were further evaluated using pivot tables in Microsoft Excel to characterize their allele effects on additional traits, e.g., to evaluate the effect of Si QTL on StHD or grain-As. Most of the QTL identified in a subpopulation panel were also significant in the GWA of all RMC, allowing Table 2, discussion text, and the pivot analysis to focus on SNP peaks as identified in the GWAS of “All” RMC. Calcium was unusual among the traits with several QTL significant in one subpopulation and not also significant in the related subspecies or “All” panels.
2.9 Candidate Gene Identification
Annotated functions of genes within the QTL regions were evaluated to identify candidate genes underlying the QTL for traits central to this study. Gene annotations from multiple public databases were merged into one file for this effort (Huggins et al., 2019) and included the candidate genes for general biotic and abiotic stress response genes identified in Cohen and Leach (2019), merged with all gene annotations in the Os-Nipponbare-Reference-IRGSP-1.0 assembly (Ouyang et al., 2006; Kawahara et al., 2013), the Rice Annotation Project (RAP1; http://rapdb.dna.affrc.go.jp/, accessed 26 Sept. 2019) (Sakai et al., 2013), and Oryzabase (OrzbaseGeneListEn_20190424010057; https://shigen.nig.ac.jp/rice/oryzabase/download/gene, accessed 26 Sept. 2019), and in Cohen and Leach (2019), which examined general biotic and abiotic stress response genes in rice.
3 Results
3.1 Rice Minicore Collection Trait Summaries
The StHD ratings for the susceptible Cocodrie checks averaged 6.0 ± 0.9 in 2015 and 5.3 ± 0.7 in 2016 on the 0–9 rating scale (Yan et al., 2005). Zhe733 had notably less severe StHD symptoms with rating averages of 2.3 ± 0.5 in 2015, 2.1 ± 0.3 in 2016, and plot ratings ranging 2 to 3 in both years. Check plot ratings verified the production of MSMA-induced symptoms across the experimental areas in both years. Among the RMC, the average StHD 2-year LSmean in MSMA-treated plots was 6.7 ± 1.3 and ranged from 3.4 to 9 (Figure 1), with none of the accessions being as StHD resistant as Zhe733, the resistant check. The majority of the RMC (101 of 156 accessions) were as or more susceptible than Cocodrie, with LSmeans >6.0. None of the plots planted in the Native soil treatment received StHD ratings >3.
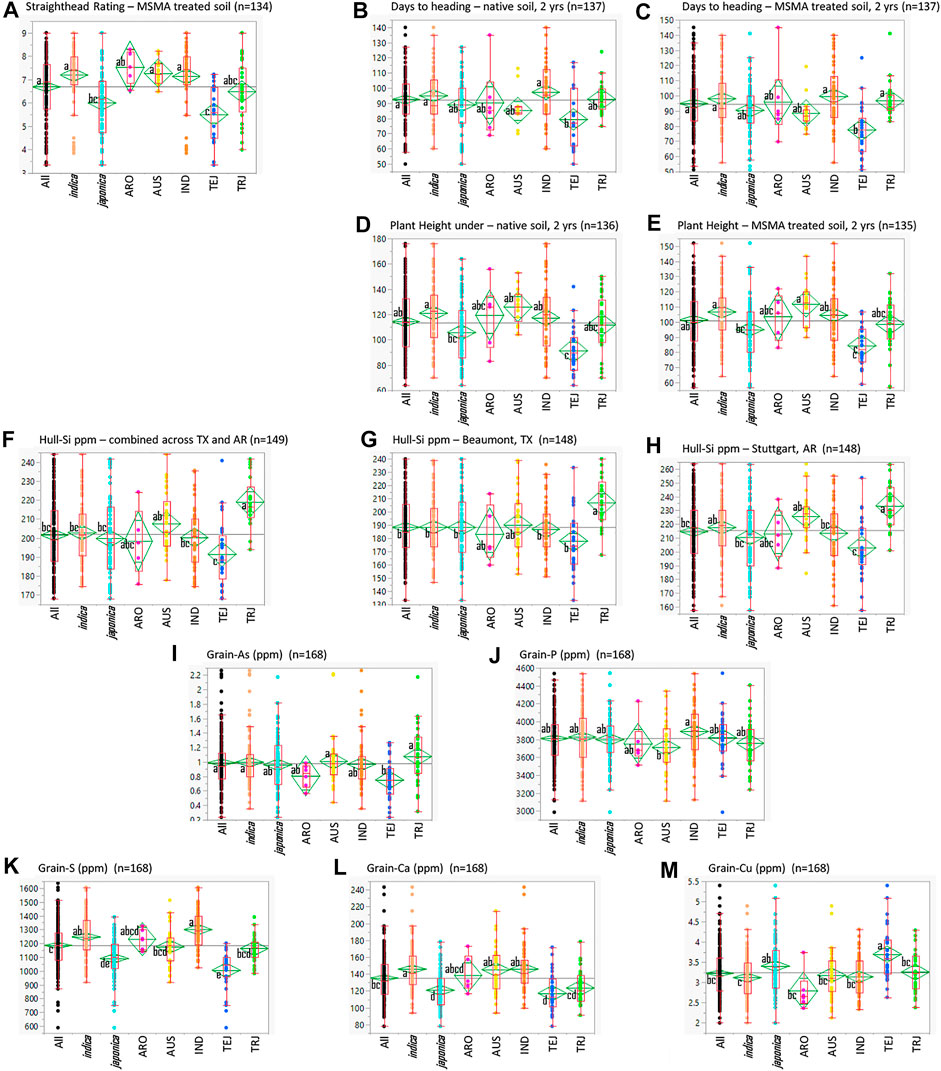
FIGURE 1. Quantile plots comparing the various traits in the entire USDA Rice Minicore (All) and the O. sativa subspecies (indica and japonica) and their subpopulations, aromatic (ARO), aus (AUS), indica (IND), temperate japonica (TEJ), and tropical japonica (TRJ). Lower and upper sides of boxes indicate the 25th and 75th percentiles, respectively; horizontal lines in boxes are medians, vertical lines indicate the 5th and 95th percentiles; and dots indicate full ranges of observed data. Difference among the small letters beside subpopulation means indicates differences among the means at α = 0.05.
Summaries of all traits observed across the entire RMC (“All”) as well as divided among the subspecies (indica and japonica) and among the subpopulations (ARO, AUS, IND, TEJ, TRJ) are presented in Figure 1. Table 1 presents Pearson correlation coefficients between the trait BLUPs for the whole RMC (“All”) and for the indica and japonica subspecies. Subpopulation correlations are not presented or discussed further because their smaller panels identified relatively few significant correlations and their correlation patterns were similar to those of the indica and japonica subspecies panels. On average, the As-induced stress in the MSMA plots delayed DHD by 2 days and decreased height by 13 cm compared to the Native soil plots, but both DHD and PHT were highly correlated between the MSMA and Native soil treatments (r = 0.90 and 0.79, respectively, Table 1). While hull-Si was lower in Beaumont, TX (avg. 188 mg kg−1), compared to Stuttgart, AR (avg. 215 mg kg−1), the hull-Si calculated across the two locations was highly correlated with the Si data from each individual location (r = 0.86 for both Beaumont, TX, and Stuttgart, AR, Table 1). A positive correlation was observed between PHT and DHD under both Native and MSMA conditions among all panels in this RMC study.
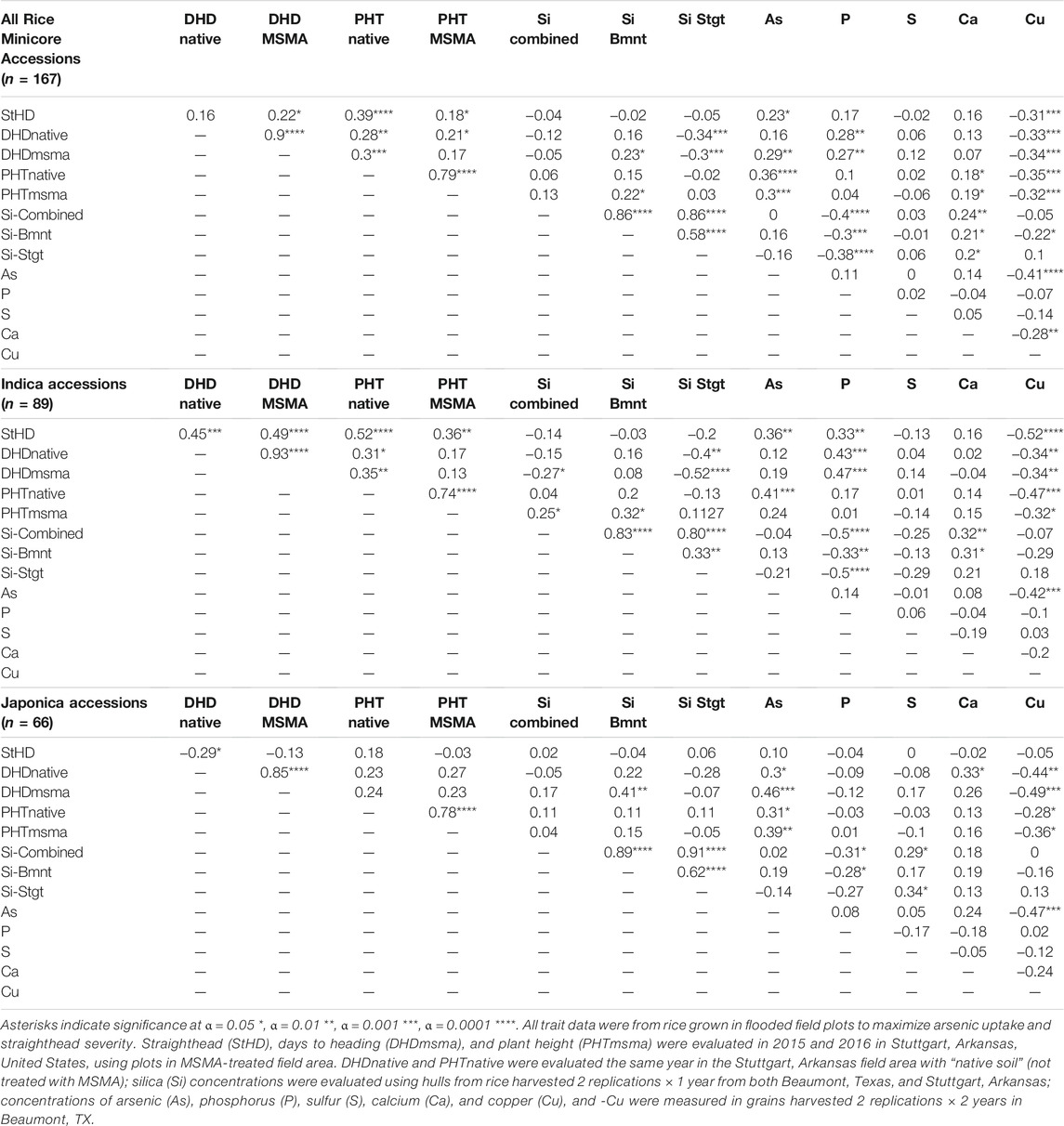
TABLE 1. Pearson correlations between traits across all the Rice Minicore accessions, and across the indica and japonica subspecies panels.
Among the subpopulations, the TEJ were notably more resistant to StHD, earlier, and shorter (Figure 1). The TEJ were also lower in hull-Si, grain-As, grain-S, and grain-Ca, but higher in grain-Cu. This exemplifies the need to account for population structure when conducting GWA analyses across multiple subpopulations, accomplished in this study by using PCs as covariates.
3.2 Relationships Among Rice Minicore Collection Traits Indicated by Pearson Correlations and Bayesian Network
StHD and grain-As were always positively correlated, although the correlations were significant in the entire population (r = 0.23, p = 0.011) and among the indica accessions (r = 0.36, p = 0.003), but not significant among the japonica accessions (r = 0.50, p = 0.10) (Table 1). Contrary to our working hypothesis, Si, P, S, and Ca were not associated with either StHD or grain-As. Copper was negatively correlated with StHD, as predicted if Cu reduces StHD severity by increasing ROS scavenging, but Cu was also negatively correlated with grain-As. In fact, the strongest correlations with StHD are PHT from Native soil (PHTnt) (r = 0.39, p < 0.0001) and Cu (r = −0.31, p < 0.001), followed by grain-As (r = 0.23, p < 0.05), and the traits most strongly correlated with grain-As are Cu (r = −0.41, p < 0.0001) and PHTnt (r = 0.36, p < 0.0001), suggesting that correlations with PHT might be underlying the correlations observed between Cu with either StHD or grain-As.
BN analysis is useful for predicting the likelihood that any one of several possible causes was the contributing factor to an observed event and presents the modeled predictions graphically using uni- or bidirectional arrows in the resulting DAG. Because of the high correlations (r > 0.75) observed between PHT and DHD in both Native and MSMA soils, and between hull-Si calculated across both locations with the hull-Si per individual location (r > 0.80), the BN analysis included only one version of these traits, namely, DHDnt, PHTnt, and hull-Si combined by BLUP across locations. Figure 2 presents the DAG determined by BN to best fit the phenotypic data from “All” RMC. The relationship between DHD and PHT was considered bidirectional (no arrow points on the connecting line), while all other relationships included in the BN model are unidirectional cause–effect relationships. The DAG indicates that the Pearson correlations observed between StHD, grain-As, and grain-Cu are likely caused by all three traits being affected by DHD, either directly or indirectly through PHT. The DAG also indicates no direct relationships between Si, S, Ca, and Cu with neither StHD nor grain-As. The DAG indicates a directional effect of As on P, a relationship which was positive but not significant in the Pearson correlations.
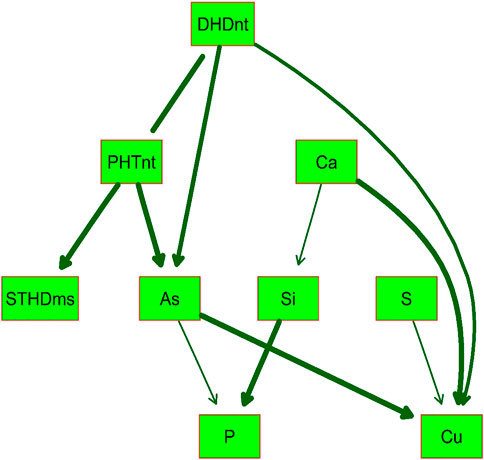
FIGURE 2. Directed acyclic graph (DAG) of the validated Bayesian network for nine arsenic-related traits determined in the USDA-ARS Rice Minicore (RMC) population with significance level of p = 0.001. The thickness of arrows between traits in green boxes represents the strength of the relationship. Straighthead disease severity was determined over 2 years using MSMA-treated soil (StHDms) in Stuttgart, AR. Days to heading (DHDnt) and plant height (PHTnt) were determined in the same 2 years and location using a native soil area (not treated with MSMA). Hull silica concentration (Si) was determined using rice grown in 1 year, two locations (Beaumont, TX and Stuttgart, AR), and two replications each. Grain concentrations of arsenic (As), phosphorus (P), sulfur (S), calcium (Ca), and copper (Cu) were determined in grains produced using 2 replications × 2 years at Beaumont, TX.
3.3 GWA-QTL Identified
Figure 3 presents the 195 GWA-QTL identified among the RMC for nine traits. Tables 2–4 present the QTL for Si (33 QTL), StHD (23), and As (15), respectively, while Supplementary Table S3 presents the QTL identified for the remaining As-related traits, P (11), S (18), Ca (19), Cu (9), DHD (39), and PHT (28). Manhattan and QQ plots are in Supplementary Figure S1. Because identification of a QTL in more than one population or environment not only validates that QTL but also demonstrates the reliability of the methods used to identify QTL in a particular study, when an RMC QTL was co-located with a previously reported QTL for the same or similar trait, this is noted in Tables 2–4 and Supplementary Table S3 by citing the previous QTL study or studies. Because the present study reevaluated the same data previously used to identify 12 hull-Si QTL (Bryant et al., 2011), we expected finding many of the same loci, and eight of the 33 Si RMC GWA-QTL did coincide with a QTL identified by Bryant et al. (2011) (Table 2). Seven additional Si RMC QTL coincided with QTL for hull, stem, or root Si concentrations reported by Dai et al. (2005) or Wu et al. (2006), and one encompassed the Lsi1 gene. These 16 validated Si QTL are now mapped more precisely in the present high-density GWA study. Of the four known Lsi transporter genes, only Lis1 on chr2 coincides with a RMC Si QTL (Figure 3; Table 2). Among the 23 StHD GWA-QTL (Table 3), seven were close (≤1.2 Mb distant) from a QTL previously reported for StHD (Agrama and Yan 2009; Pan et al., 2012; Li et al., 2016; Li et al., 2017), arsenite toxicity (Murugaiyan et al., 2019), or the chemical mimic, germanium toxicity (Talukdar et al., 2015), increasing confidence also in the 15 novel StHD RMC GWA-QTL. The most commonly identified StHD QTL was qStHD8-2, which co-located with QTL previously identified in four different populations (Pan et al., 2012; Li et al., 2016; Murugaiyan et al., 2019). When the RMC QTL for grain-As were compared with previously reported As QTL determined in various biparental mapping or GWA populations (Zhang et al., 2008; Norton et al., 2012a; Norton et al., 2014; Zhang et al., 2014; Norton et al., 2019; Liu et al., 2021), nine of the 15 RMC grain-As GWA-QTL coincided with a previously reported grain-As locus (Table 4). Four of the 11 RMC QTL for P, six of 18 for S, one of 19 for Ca, and 2 of 9 for Cu were validated by known transporter genes or previous QTL studies (Zhang et al., 2014; Liu et al., 2021) (Supplementary Table S3). Thirty-two percent (41/128) of the StHD and elemental QTL were validated by other studies, leaving 84 of the RMC QTL for As, Si, StHD, P, S, Ca, and Cu as novel. With the literature containing more than 200 reports of QTL affecting rice DHD and PHT, encompassing much of the rice genome, the present DHD and PHT GWA-QTL were compared only with genes confirmed to affect DHD or PHT. Seven of the 39 DHD QTL found among the RMC and 13 of the 28 PHT QTL encompassed a known DHD or PHT gene (Supplementary Table S3).
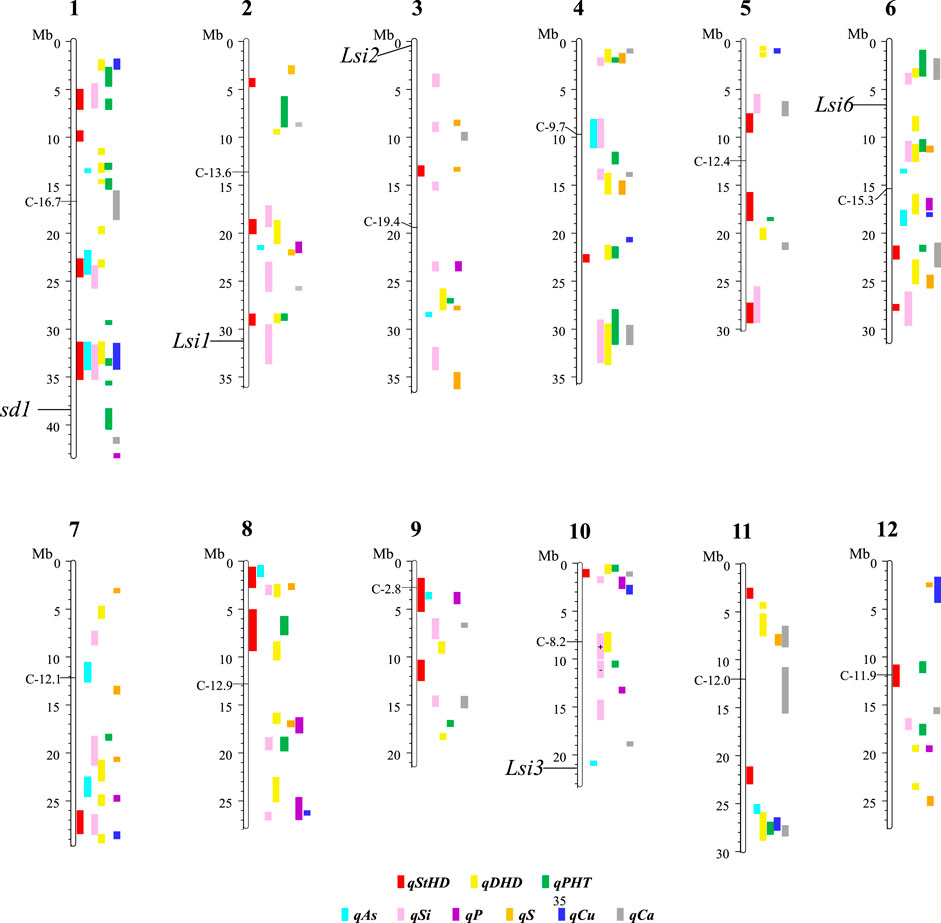
FIGURE 3. The physical position of the QTL for straighthead disease severity (qStHD), days to heading (qDHD), plant height (qPHT), hull silica concentration (qSi), and grain concentrations of arsenic (qAs), phosphorus (qP), sulfur (qS), calcium (qCa), and copper (qCu) identified by genome-wide association (GWA) mapping in the USDA Rice Minicore (RMC) with 3,200,320 SNP markers across the entire rice genome. Chromosome and megabase (Mb) positions of QTL and centromeres (C-Mb) are based on the Os-Nipponbare-Reference-IRGSP-1.0 assembly (Kawahara et al., 2013). The details of the QTL and their distinguishing SNPs are organized per trait with Si in Table 2, StHD in Table 3, As in Table 4, and the remaining traits in Supplementary Table S2.
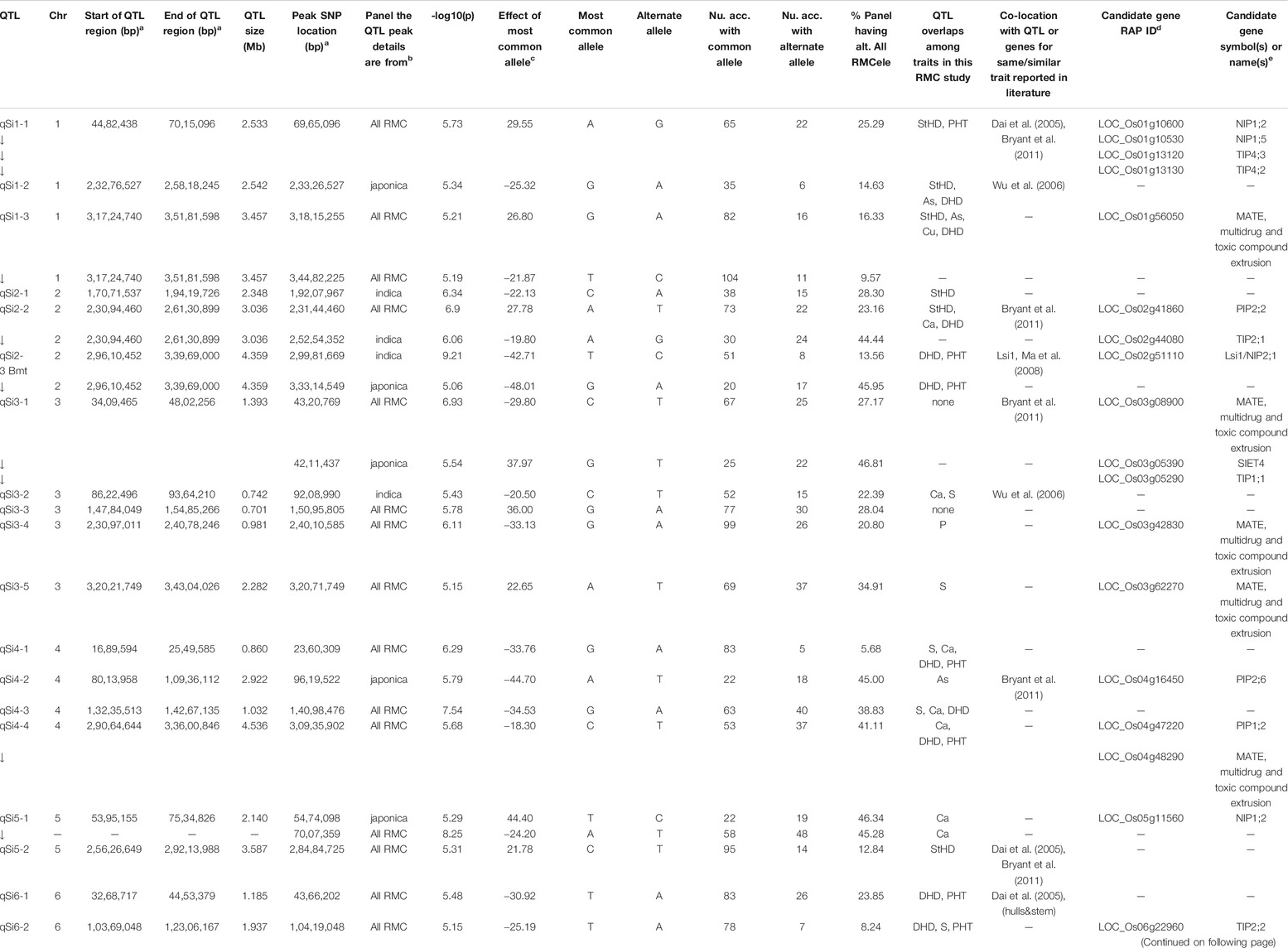
TABLE 2. QTL associated by GWA with hull-Si concentration (qSi) in the Rice Minicore Panel (RMC) arranged in chromosomal order.
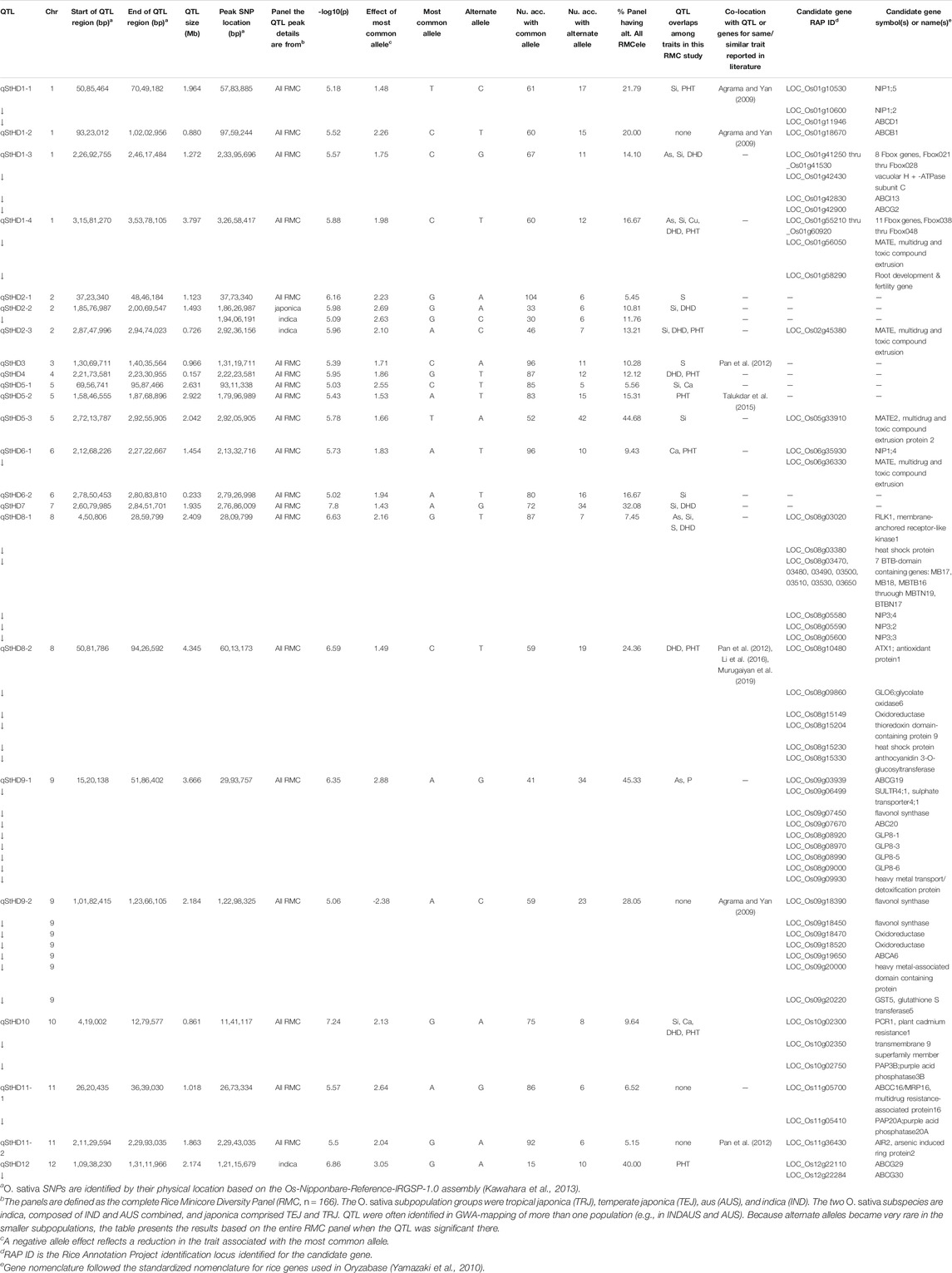
TABLE 3. QTL associated by GWA with straighthead disease response (qStHD) in the Rice Minicore Panel (RMC) arranged in chromosomal order. Overlaps with QTL for other traits within this study, and candidate genes are also noted. Panels for which QTL are listed include “all RMC” containing all RMC accessions, indica subspecies, japonica subspecies, IND subgroup, and AUS subgroup.
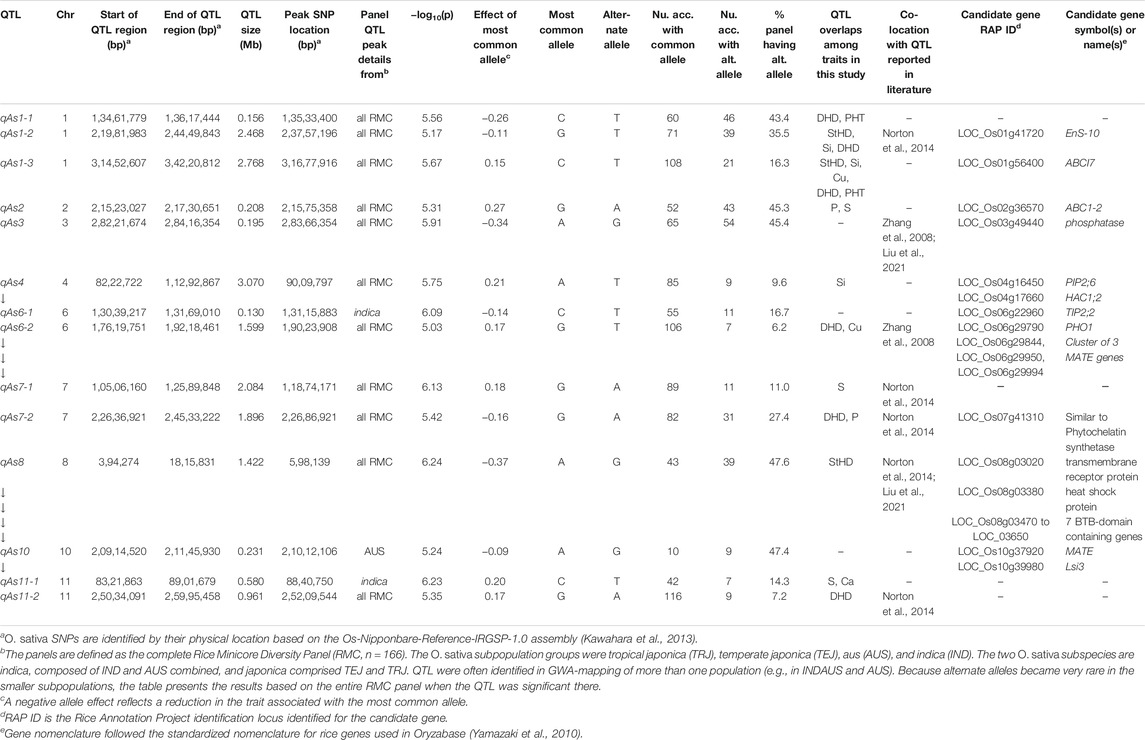
TABLE 4. QTL associated by GWA with grain arsenic concentration (qAs) in the Rice Minicore Panel (RMC) arranged in chromosomal order. Overlaps with QTL for other traits within this study, and candidate genes are also noted. Panels for which QTL are listed include “all RMC” containing all RMC accessions, indica subspecies, japonica subspecies, IND subgroup, and AUS subgroup.
3.4 Co-Location Among GWA-QTL for Different Arsenic-Related Traits
Among the 23 StHD QTL and 15 As QTL, four were co-located (Tables 3, 4; Figure 3). Eleven of the StHD QTL and four of the As QTL coincided with Si. Although less common, P, S, Cu, and Ca QTL were found co-located with some StHD and As QTL. In all four instances of coincidence between StHD and As QTL, a pivot table evaluation of allele effects showed that the allele that decreased StHD at qStHD1-3, qStHD1-4, and qStHD8-1 also decreased As and Si. Similarly, the qStHD9-1 allele that decreased StHD severity also decreased both As and P. Thus, we did find some QTL overlaps lending support to the hypothesis that decreased As-uptake, as evidenced by decreased grain-As, hull-Si, or grain-P concentrations, results in decreased StHD as well. Because the DAG (Figure 2) suggested that overlap of StHD and As QTL might instead be caused by mutual dependence on DHD, it must be noted that all three instances of coincidence between StHD, As, and Si QTL also coincide with DHD. The one instance of overlap between P, StHD, and As (at qStHD9-1) does appear to be independent of DHD and PHT, as well as all other traits in this study (Table 3; Figure 3).
In agreement with the DAG (Figure 2) showing a strong influence of DHD on StHD via PHT, 10 of the 23 StHD QTL co-located with a PHT QTL, 8 with DHD, for a total of 12 that coincide with DHD, PHT, or both (Figure 3; Table 3). The DAG did not indicate a close relationship between StHD and Si, nor between Si and DHD. Even so, co-location among StHD and Si QTL was high, with 12 of the 23 StHD loci overlapping or being within 1 Mb of a Si QTL. This suggests that Si concentrations may in fact affect StHD severity in a manner not detected in this data by BN. For example, Si might affect StHD but less strongly than DHD and PHT. Similarly, four As QTL (qAs2, qAs3, qAs7-1, and qAs8-1), and three StHD QTL (qStHD1-1, qStHD3, and qStHD8-1) were on or near S QTL (Tables 3, 4; Figure 3), suggesting that increased As-chelation and/or increased ROS scavenging by increased S might be affecting grain-As or StHD. Overlaps between StHD and Ca or Cu QTL were notably uncommon and always involved another trait such as DHD or Si (qStHD1-4 and qStHD7 with Cu QTL, and qStHD5-1, qStHD6-1, and qStHD10 with Ca QTL), indicating that increased ROS scavenging from increased Ca or Cu is not contributing significantly to the observed variance for StHD resistance.
There are four instances of co-location between As and P QTL (chr 2, 6, 7, and 9; Table 4 and Figure 3), with pivot table analyses showing the allele that increased As also increased P. The instance of overlap on chr9 was discussed above as independent from DHD, but the other three do coincide with a DHD QTL. While the DAG does not show a direct connection between DHD and P, it does show a DHD effect on P channeling through As (Figure 2), and DHD-P correlations were significant and positive among the entire RMC and indica subspecies, but the DHD-P correlations were near zero among japonica accessions (Table 1).
4 Discussion
Factors affecting Si concentrations are candidates for factors affecting As, which are in turn candidates for StHD. We will therefore discuss the traits in that order.
4.1 Candidate Genes for Si QTL
To explore candidate genes in the 33 Si RMC GWA-QTL (Table 2), we searched annotated genes to find those involved with transmembrane transport of silicon transport or metals and metalloids. The four known Low silicon transporters (Lsi1, Lsi2, Lsi3, and Lsi6) are of two types, with Lsi2 and Lsi3 being silicon efflux transporters (SIETs), and Lsi1 and Lsi6 being aquaporins of the Nodulin-26 like intrinsic protein (NIP) type. Only one of the four Lsi genes, specifically Lsi1, coincided with an RMC Si QTL (Table 2; Figure 3). NIPs are a subclass of membrane intrinsic transporters (MIP) which include also plasma membrane intrinsic proteins (PIPs) and tonoplast intrinsic proteins (TIPs). Overall, across the 33 Si RMC GWA-QTL regions (Table 2), we identified as candidate genes two SIETs, seven NIPs, three PIPs, five (TIPs), and 13 multidrug and toxic compound extrusion (MATE) genes. All these genes were located within 1 Mb of 17 of the 33 Si RMC GWA-QTL.
4.2 Candidate Genes for As QTL
To explore candidate genes in the 15 As RMC GWA-QTL (Table 4), we again looked in QTL regions for components of silicon and heavy metal uptake or transport and added to the search genes for signaling, and/or tolerance mechanisms (Abedi and Mojiri 2020) as well as chelation and detoxifying enzymes reported in the literature as affecting metalloid metabolism (Chen et al., 2017; Abedi and Mojiri, 2020). Overall, across the 15 As RMC GWA-QTL regions, we identified three NIPs, one SIET/Lsi3, four MATE genes, two ABC family proteins, one PIP, one TIP, one phosphate transporter, two transmembrane proteins (i.e., transmembrane receptor protein and EnS-10, endosperm-specific gene with metal ion transmembrane transporter activity), three metabolizing enzymes (phosphatase, arsenate reductase (HAC1;2), and one similar to phytochelatin synthetase), and one heat shock protein as noted in Table 4. These genes were located within 1 Mb of 10 of the 15 As RMC GWA-QTL.
The candidate gene identified by the overlapping qSi4-2/qAs4 QTL, PIP2;6, was initially reported to be involved in influx and efflux of boron transport (Mosa et al., 2016), but the heterologous expression of OsPIP2;6 in Xenopus laevis oocytes was also found to increase the uptake of arsenite (Mosa et al., 2012). Several NIP proteins have been shown to transport As, including the well-known Lsi1 and Lsi6 aquaporin transporters, NIP1;1 (Kamiya et al., 2009), and Arabidopsis NIP3;1 (Xu et al., 2015). The NIP3;2 protein (candidate for qSi8-1) has also been shown permeable to arsenite (Bienert et al., 2008; Ma et al., 2008). However, while this Si QTL overlapped with qStHD8-1, it did not overlap with the nearby qAs8. MATE genes play an important role in cellular detoxification processes. Using a transcriptomic study, Seth et al. (2020) identified nine MATE genes that were upregulated upon exposure to As. The constitutive expression of OsMATE2 in transgenic tobacco plants decreased root-to-shoot As transfer, and transgenic rice plants wherein RNAi was used to silence OsMATE2 produced grains with less grain-As than wild-type rice plants (Das et al., 2018). While MATE2 was not among the grain-As candidate genes, it is a candidate gene for qStHD5-3.
4.3 Candidate Genes for StHD QTL
Because StHD has been attributed to As toxicity (Tang et al., 2020a), to explore candidate genes underlying the 23 StHD RMC GWA-QTL (Table 4), we included in the search the same components of Si and heavy metal uptake, transport, signaling, and/or tolerance mechanisms, chelating and detoxifying enzymes included in the As candidate gene search, and added also stress-protective agents (e.g., redox homeostasis and antioxidant defense). Across the 23 StHD RMC GWA-QTL regions (Table 4), we identified 10 ABC family transporter proteins, six NIPs, two MATEs, one Si transporter, two membrane proteins, eight enzymes (i.e., vacuolar H + -ATPase subunit C antioxidant protein 1 (ATX1), glycolate oxidase 6 (GLO6), flavonol synthase, oxidoreductase, glutathione S transferase 5 (GST5)), two purple acid phosphatases (PAP3B, PAP20A), and one plant cadmium resistance (PCR1) gene within 1 Mb of 12 of the 23 StHD RMC GWA-QTL. Vacuolar ATPase is a proton pump protein whose role in acidification of the vacuolar compartment activates the uptake and release of ions and metabolites (Sze et al., 1992; Lüttge and Ratajczak, 1997). Furthermore, ATX1 is known to interact with heavy metal P1B-ATPases, suggesting its role in delivering Cu to heavy metal P1B-ATPases for Cu trafficking and distribution in order to maintain Cu homeostasis in rice (Zhang et al., 2018). RLK1 encodes a plasma membrane-localized protein that acts upstream of mitogen-activated protein kinase (MPK) cascades and positively regulates defense-related MPKs and WRKY transcription factors to respond differentially to external stimuli (Meng and Zhang, 2013; Macho and Zipfel, 2014). The ubiquitin-proteasome system (UPS) is one of major protein regulation pathways that enhance the adaptation and survival of plants under various environmental stresses such as toxic metalloid exposure as well as other environmental stresses (drought, salinity, and cold). Recent study showed active E3 ligase activity of OsAIR2 through in vitro ubiquitination assay and further examined that overexpression lines of OsAIR2 in Arabidopsis improved the seed germination and increased the root length under arsenate stress conditions, suggesting its role as a positive regulator of As stress tolerance (Hwang et al., 2017). Germin-like protein (GLP) is a plant glycoprotein associated with the plant cell wall, and its various proposed roles in plant development and defense are known (Dunwell et al., 2008; Caliskan, 2009).
4.4 Further Consideration of Candidate Genes in Genomic Regions Containing Overlapping As and StHD QTL
The region of Chr8 encompassing qAS8 and qStHD8-1 (Chr8 0.45-0.82 MB) contains a series of BTB-domain-containing proteins (MB17&18, MBTB16, 17, 18, 19; BTBN17) that are also of interest. A BTB domain-containing protein was reported to be involved in enhancing iron homeostasis in apple (Zhao et al., 2016). Recent study also showed that rice plants overexpressing a BTB domain protein (OsTAZ24) promoted plant resistance against various heavy metals (Shalmani et al., 2021), demonstrating the role of the BTB-domain-containing protein in heavy metal homeostasis, making these genes candidates for both the grain-As and StHD QTL. We therefore list this cluster of BTB-domain proteins as candidate genes for both qAs8 (Table 4) and qStHD8-1 (Table 3).
There were two RMC GWA-QTL regions that were associated with Si along with As and StHD (qAs1-2/qStHD1-3/qSi1-2, and qAs1-3/qStHD1-4/qSi1-3). Interestingly, these two regions, one on Chr1 at 23.34–24.45 Mb and the other one on Chr1 at 31.72–34.22 Mb, contain eight and 11 F-box domain-containing proteins, respectively. Recent study showed that 12 F-box domain-containing proteins were located in the As QTL regions from a japonica diversity panel of 228 accessions and from 95 advanced breeding lines with japonica genetic backgrounds phenotyped for As concentration in the flag leaf as well as in dehulled grain (Frouin et al., 2019). One of the F-box proteins identified as a candidate gene in our study, OsBOX028, was also identified by Frouin et al. (2019), suggesting that F-box proteins may have a role in affecting As tolerance. F-box proteins are known to define the specific substrates of the SCF complexes that are part of the ubiquitin/26S proteasome pathway for degrading unwanted or misfolded proteins (Zheng et al., 2002; Lechner et al., 2006). Thus, these F-box gene complexes are listed as candidate genes for qStHD1-3 and qStHD1-4 QTL (Table 3), but not for the coincident As and Si QTL (Tables 2, 4).
Another interesting aspect of the QTL overlaps was a region on chr9 containing QTL for StHD and As but not for Si. A QTL for P (qP9) was also located with qStHD9-1/qAs9. When we further examined the genes in this overlapping region, we found that more than 50% of genes in this region were retro/transposon proteins, forty retrotransposon proteins and five transposon proteins out of 99 total genes in this overlapping QTL region of qAs9, qStHF9-1, and qP9 (Chr. 9, 3,356,592–3,937,895). Transposons and retrotransposons play a role in post-transcription regulation through silencing of transposable elements (TEs), which can elicit gene variation and functional changes (Gao et al., 2012). TEs are also a source of small RNAs. A role for small RNAs in regulation of P starvation has been reported (Fang et al., 2009; Hsieh et al., 2009; Chiou and Lin 2011; O’Rourke et al., 2013).
4.5 Potential Impacts of Silica on Arsenic and StHD not Caused by Shared Silica/Arsenic Transport
Eleven of the 33 StHD QTL were co-located with Si QTL in the RMC, only three of which were also co-located with As QTL. This suggests a benefit from Si on StHD that is not directly associated with As and shared Si–As transporters. Numerous recent studies have confirmed Si beneficial effects on a variety of plant species growing under a wide range of environmental conditions. Si not only has a role in ameliorating biotic and abiotic stress but also can alleviate nutrient deficiency and toxicities (Pavlovic et al., 2021). Most of the studies regarding Si nutrient interactions were studies conducted on Si accumulators like rice. A general model for Si interactions has been proposed based on those studies for silicon-mediated response to mineral deficiency and toxicity (Ali et al., 2020). Under nutritional stress, uptake of Si increases through Lsi transporters (Yamaji and Ma, 2011) or aquaporins (Deshmukh et al., 2013). The increase in Si induces genes encoding the uptake and translocation of minerals in roots and facilitates the increase or decrease uptake of the corresponding nutrients such as N (Wu et al., 2017) and P (Owino-Gerroh and Bascho, 2005). In shoots, Si interacts with phytohormones and amino acids, and metabolites lower oxidative stress by further modulating the antioxidant enzymes (Liang et al., 2003; Li et al., 2012; Chalmardi et al., 2014) and simultaneously maintaining or even inducing higher photosynthetic efficiency resulting in plant growth and development (Detmann et al., 2012). On the other hand, for mineral or heavy metal toxicity, Si forms a complex with some heavy metals (Cd) or minerals (Zn, Fe, Cu) in the root cell wall, therefore reducing uptake and translocation to the shoots (Liu et al., 2013; Kopittke et al., 2017; Nozawa et al., 2018; Bosnic et al., 2019). In shoots, Si accumulation further mitigates metal toxicity by sequestration of minerals (Cu, Zn) and heavy metals (As, Cd) into the vacuoles of leaf cells (Keller et al., 2015; Kopittke et al., 2017; Nozawa et al., 2018). Overall, Si has the potential of enabling the plant to react adaptively against nutritional stress and promotes tolerance.
4.6 Conclusion: Implications for Future Research
The initial hypothesis was that both StHD and grain-As would be regulated strongly enough by the concentration of free (unbound, non-sequestered) As in the plant that they would share numerous QTL. We further hypothesized that overlap of the StHD and As QTL with Si, P, or S would provide further insight as to whether the shared StHD and As QTL were being driven by As-uptake or post-uptake metabolism. Because data existed to add Ca and Cu to the study, and because these elements have been shown to increase ROS scavenging, which could in turn reduce StHD severity, they were included as well. Correlations were positive between StHD, As, and Si, as would be expected if our first two hypotheses were true. However, finding a stronger StHD-Cu correlation than seen for StHD-As was unexpected. The BN DAG results cautioned to not overlook possible confounding effects from DHD in our data interpretations. When QTL were identified, and overlaps considered, the number (4 total) and percentage of StHD (0.12) and As (0.27) QTL that were co-located were lower than hypothesized. We also predicted that Si would impact StHD via its influence on As uptake. In contrast, we found that significantly more StHD QTL overlapped with Si QTL (11/33) than with As QTL (4/33). The results indicate that StHD and grain-As are both more complex than initially hypothesized and indicate that Si QTL may be affecting StHD response in ways not connected by As concentration, such as by increasing ROS scavenging, or improving overall plant health and nutrient balance.
Even though the hypothesis based on an StHD-As association was not well supported, the StHD and Si QTL identified among the RMC accessions in this study are the first to be identified using high-density mapping and, thus, are mapped to smaller QTL regions than previously available for these traits. Furthermore, the high proportion of QTL identified among the RMC accessions that were validated from those in literature indicates that the QTL-identification methods used were robust, increasing confidence in both the validated and novel QTL we report. The novel Si QTL, in particular, are worth deeper investigation since many of them were not explainable by currently known Si transporters and because Si did appear to have a stronger impact on StHD than could be explained by As alone. The region of chr8 that has been commonly reported as associated with StHD (qStHD8-2) also warrants further investigation. This qStHD8-2 QTL region was not associated with either As or Si but was found to contain multiple genes of interest including genes for antioxidants and redox factors which could be reducing ROS damage, suggesting that differences in ROS damage should be considered in future research on StHD resistance mechanisms. The region of chr9 containing qStHD9-1, qAs9, and qP9 which was independent from DHD would also be a genomic segment warranting further research. Other genes of interest for future study, because they were found in multiple grain-As and StHD regions, include several multidrug and toxic compound extrusion (MATE) genes, F-box genes, BTB-domain proteins, and NIPs not documented to date to transport As. The candidate genes we identified could be a basis for gene editing studies to determine the genes underlying the StHD, As, and Si QTL identified in the RMC.
Data Availability Statement
The trait data used for the present GWA analyses are in Supplementary Table S1. Genotypic datafiles developed by Huggins et al. (2019) for the Rice Minicore Collection can be downloaded in either VCF or HapMap format at https://www.ars.usda.gov/southeast-area/stuttgart-ar/dale-bumpers-national-rice-research-center/docs/mini-core-collection/.
Author Contributions
All authors listed have made a substantial, direct, and intellectual contribution to the work and approved it for publication.
Funding
This research was supported in part by the USDA-ARS Headquarters Research Associate Program and used facilities and assistance provided by the USDA Agricultural Research Service, Dale Bumpers National Rice Research Center.
Conflict of Interest
The authors declare that the research was conducted in the absence of any commercial or financial relationships that could be construed as a potential conflict of interest.
Publisher’s Note
All claims expressed in this article are solely those of the authors and do not necessarily represent those of their affiliated organizations, or those of the publisher, the editors, and the reviewers. Any product that may be evaluated in this article, or claim that may be made by its manufacturer, is not guaranteed or endorsed by the publisher.
Acknowledgments
We acknowledge Eric Grunden and Alex Humphries for technical assistance with small plot experiments and acknowledge Laduska Sells for field preparation and water and pest management.
Supplementary Material
The Supplementary Material for this article can be found online at: https://www.frontiersin.org/articles/10.3389/fgene.2021.787767/full#supplementary-material
References
Abedi, T., and Mojiri, A. (2020). Arsenic Uptake and Accumulation Mechanisms in Rice Species. Plants 9 (2), 129. doi:10.3390/plants9020129
Agency for Toxic Substances and Disease Registry (2007). Toxicological Profile for Arsenic. U.S. Department of Health and Human Services, Public Health Service. Available at: http://www.atsdr.cdc.gov/toxprofiles/tp2.pdf (Accessed September 1, 2021).
Agrama, H. A., and Yan, W. G. (2009). Association Mapping of Straighthead Disorder Induced by Arsenic inOryza Sativa. Oryza Sativa. Plant Breed. 128, 551–558. doi:10.1111/j.1439-0523.2009.01631.x
Agrama, H. A., Yan, W., Lee, F., Fjellstrom, R., Chen, M.-H., Jia, M., et al. (2009). Genetic Assessment of a Mini-Core Subset Developed from the USDA Rice Genebank. Crop Sci. 49 (4), 1336–1346. doi:10.2135/cropsci2008.06.0551
Ali, N., Réthoré, E., Yvin, J.-C., and Hosseini, S. A. (2020). The Regulatory Role of Silicon in Mitigating Plant Nutritional Stresses. Plants 9, 1779. doi:10.3390/plants9121779
Anjum, N. A., Umar, S., Ahmad, A., Iqbal, M., and Khan, N. A. (2008). Ontogenic Variation in Response ofBrassica campestrisL. To Cadmium Toxicity. J. Plant Interactions 3, 189–198. doi:10.1080/17429140701823164
Bates, D., Maechler, M., and Bolker, B. (2011). Lme4: Linear Mixed Effects Models Using S4 Classes. Available at: http://cran.rproject.org/web/packages/lme4/index.html (Accessed July 29, 2021).
Begum, M., Akter, J., Jahiruddin, M., and Islam, M. R. (2008). Effects of Arsenic and its Interaction with Phosphorus on Yield and Arsenic Accumulation in rice. J. Bangladesh Agril. Univ. 6 (2), 277–284. 2008 ISSN 1810-3030. doi:10.3329/jbau.v6i2.4822
Bienert, G. P., Thorsen, M., Schüssler, M. D., Nilsson, H. R., Wagner, A., Tamás, M. J., et al. (2008). A Subgroup of Plant Aquaporins Facilitate the Bi-directional Diffusion of As(OH)3 and Sb(OH)3across Membranes. BMC Biol. 6, 26. doi:10.1186/1741-7007-6-26
Bosnić, D., Nikolić, D., Timotijević, G., Pavlović, J., Vaculík, M., Samardžić, J., et al. (2019). Silicon Alleviates Copper (Cu) Toxicity in Cucumber by Increased Cu-Binding Capacity. Plant Soil 441, 629–641. doi:10.1007/s11104-019-04151-5
Bradbury, P. J., Zhang, Z., Kroon, D. E., Casstevens, T. M., Ramdoss, Y., and Buckler, E. S. (2007). TASSEL: Software for Association Mapping of Complex Traits in Diverse Samples. Bioinformatics 23 (19), 2633–2635. doi:10.1093/bioinformatics/btm308
Bryant, R., Proctor, A., Hawkridge, M., Jackson, A., Yeater, K., Counce, P., et al. (2011). Genetic Variation and Association Mapping of Silica Concentration in rice Hulls Using a Germplasm Collection. Genetica 139, 1383–1398. doi:10.1007/s10709-012-9637-x
Caliskan, M. (2009). Salt Stress Causes a Shift in the Localization Pattern of Germin Gene Expression. Genet. Mol. Res. 8, 1250–1256. doi:10.4238/vol8-4gmr623
Cao, Y., Sun, D., Ai, H., Mei, H., Liu, X., Sun, S., et al. (2017). Knocking Out OsPT4 Gene Decreases Arsenate Uptake by Rice Plants and Inorganic Arsenic Accumulation in rice Grains. Environ. Sci. Technol. 51 (21), 12131–12138. doi:10.1021/acs.est.7b03028
Chalmardi, Z. K., Abdolzadeh, A., and Sadeghipour, H. R. (2014). Silicon Nutrition Potentiates the Antioxidant Metabolism of Rice Plants under Iron Toxicity. Acta Physiol. Plant 36, 493–502. doi:10.1007/s11738-013-1430-7
Chen, Y., Han, Y.-H., Cao, Y., Zhu, Y.-G., Rathinasabapathi, B., and Ma, L. Q. (2017). Arsenic Transport in Rice and Biological Solutions to Reduce Arsenic Risk from Rice. Front. Plant Sci. 8, 268. doi:10.3389/fpls.2017.00268
Chiou, T.-J., and Lin, S.-I. (2011). Signaling Network in Sensing Phosphate Availability in Plants. Annu. Rev. Plant Biol. 62, 185–206. doi:10.1146/annurev-arplant-042110-103849
Choudhury, B., Chowdhury, S., and Biswas, A. K. (2011). Regulation of Growth and Metabolism in Rice (Oryza sativaL). by Arsenic and its Possible Reversal by Phosphate. J. Plant Interactions 6 (1), 15–24. doi:10.1080/17429140903487552
Cohen, S. P., and Leach, J. E. (2019). Abiotic and Biotic Stresses Induce a Core Transcriptome Response in Rice. Sci. Rep. 9 (1), 6273. doi:10.1038/s41598-019-42731-8
Dai, W.-M., Zhang, K.-Q., Duan, B.-W., Zheng, K.-L., Zhuang, J.-Y., and Cai, R. (2005). Genetic Dissection of Silicon Content in Different Organs of Rice. Crop Sci. 45, 1345–1352. doi:10.2135/cropsci2004.0505
Das, N., Bhattacharya, S., Bhattacharyya, S., and Maiti, M. K. (2018). Expression of rice MATE Family Transporter OsMATE2 Modulates Arsenic Accumulation in Tobacco and Rice. Plant Mol. Biol. 98 (1-2), 101–120. doi:10.1007/s11103-018-0766-1
Deshmukh, R. K., Vivancos, J., Guérin, V., Sonah, H., Labbé, C., Belzile, F., et al. (2013). Identification and Functional Characterization of Silicon Transporters in Soybean Using Comparative Genomics of Major Intrinsic Proteins in Arabidopsis and Rice. Plant Mol. Biol. 83, 303–315. doi:10.1007/s11103-013-0087-3
Detmann, K. C., Araújo, W. L., Martins, S. C. V., Sanglard, L. M. V. P., Reis, J. V., Detmann, E., et al. (2012). Silicon Nutrition Increases Grain Yield, Which, in Turn, Exerts a Feed‐Forward Stimulation of Photosynthetic Rates via Enhanced Mesophyll Conductance and Alters Primary Metabolism in Rice. New Phytol. 196 (3), 752–762. doi:10.1111/j.1469-8137.2012.04299.x
Dixit, S., and Hering, J. G. (2003). Comparison of Arsenic(V) and Arsenic(III) Sorption onto Iron Oxide Minerals: Implications for Arsenic Mobility. Environ. Sci. Technol. 37, 4182–4189. doi:10.1021/es030309t
Duan, G., Shao, G., Tang, Z., Chen, H., Wang, B., Tang, Z., et al. (2017). Genotypic and Environmental Variations in Grain Cadmium and Arsenic Concentrations Among a Panel of High Yielding rice Cultivars. Rice 10, 9. doi:10.1186/s12284-017-0149-2
Dunwell, J. M., Gibbings, J. G., Mahmood, T., and Saqlan Naqvi, S. M. (2008). Germin and Germin-like Proteins: Evolution, Structure, and Function. Crit. Rev. Plant Sci. 27, 342–375. doi:10.1080/07352680802333938
Fang, Z., Shao, C., Meng, Y., Wu, P., and Chen, M. (2009). Phosphate Signaling in Arabidopsis and Oryza Sativa. Plant Sci. 176, 170–180. doi:10.1016/j.plantsci.2008.09.007
FAO/WHO (2013). Codex General Standard for Contaminants and Toxins in Food and Feed. CODEX STAN 193, 1995. Rome, Italy: Food and Agriculture Organization.
Fernández-Baca, C. P., McClung, A. M., Edwards, J. D., Codling, E. E., Reddy, V. R., and Barnaby, J. Y. (2021). Grain Inorganic Arsenic Content in rice Managed through Targeted Introgressions and Irrigation Management. Front. Plant Sci. 11, 612054. doi:10.3389/fpls.2020.612054
Fichman, Y., and Mittler, R. (2021). Integration of Electric, Calcium, Reactive Oxygen Species and Hydraulic Signals during Rapid Systemic Signaling in Plants. Mol. Plant 12, 1203–1210. doi:10.1016/j.molp.2019.06.003
Frouin, J., Labeyrie, A., Boisnard, A., Sacchi, G. A., and Ahmadi, N. (2019). Genomic Prediction Offers the Most Effective Marker Assisted Breeding Approach for Ability to Prevent Arsenic Accumulation in rice Grains. PLoS One 14 (6), e0217516. doi:10.1371/journal.pone.0217516
Gao, C., Xiao, M., Jiang, L., Li, J., Yin, J., Ren, X., et al. (2012). Characterization of Transcriptional Activation and Inserted-Into-Gene Preference of Various Transposable Elements in the Brassica Species. Mol. Biol. Rep. 39, 7513–7523. doi:10.1007/s11033-012-1585-0
Heitkemper, D. T., Kubachka, K. M., Halpin, P. R., Allen, M. N., and Shockey, N. V. (2009). Survey of Total Arsenic and Arsenic Speciation in US-Produced rice as a Reference point for Evaluating Change and Future Trends. Food Additives and Contaminants: B 2 (2), 112–120. doi:10.1080/02652030903148298
Heuschele, D. J., Pinson, S. R. M., and Smith, A. P. (2017). Metabolic Responses to Arsenite in rice Seedlings that Differed in Grain Arsenic Concentration. Crop Sci. 57, 2671–2687. doi:10.2135/cropsci2016.06.0493
Hossain, M. A., Piyatida, P., da Silva, J. A. T., and Fujita, M. (2012). Molecular Mechanism of Heavy Metal Toxicity and Tolerance in Plants: Central Role of Glutathione in Detoxification of Reactive Oxygen Species and Methylglyoxal and in Heavy Metal Chelation. J. Bot. 2012, 1–37. doi:10.1155/2012/872875
Hsieh, L.-C., Lin, S.-I., Shih, A. C.-C., Chen, J.-W., Lin, W.-Y., Tseng, C.-Y., et al. (2009). Uncovering Small RNA-Mediated Responses to Phosphate Deficiency in Arabidopsis by Deep Sequencing. Plant Physiol. 151, 2120–2132. doi:10.1104/pp.109.147280
Huggins, T. D., Chen, M. H., Fjellstrom, R. G., Jackson, A. K., McClung, A. M., and Edwards, J. D. (2019). Association Analysis of Three Diverse Rice ( Oryza Sativa L). Germplasm Collections for Loci Regulating Grain Quality Traits. Plant Genome 12 (1), 170085. doi:10.3835/plantgenome2017.09.0085
Hwang, S.-G., Chapagain, S., Han, A.-R., Park, Y. C., Park, H. M., Kim, Y. H., et al. (2017). Molecular Characterization of Rice Arsenic-Induced RING finger E3 Ligase 2 (OsAIR2 ) and its Heterogeneous Overexpression in Arabidopsis T. Physiol. Plantarum 161 (3), 372–384. doi:10.1111/ppl.12607
Kalita, J., Pradhan, A. K., Shandilya, Z. M., and Tanti, B. (2018). Arsenic Stress Responses and Tolerance in rice: Physiological, Cellular and Molecular Approaches. Rice Sci. 25, 235–249. doi:10.1016/j.rsci.2018.06.007
Kamiya, T., Tanaka, M., Mitani, N., Ma, J. F., Maeshima, M., and Fujiwara, T. (2009). NIP1;1, an Aquaporin Homolog, Determines the Arsenite Sensitivity of Arabidopsis T. J. Biol. Chem. 284, 2114–2120. doi:10.1074/jbc.M806881200
Kawahara, Y., de la Bastide, M., Hamilton, J. P., Kanamori, H., McCombie, W. R., Ouyang, S., et al. (2013). Improvement of the Oryza Sativa Nipponbare Reference Genome Using Next Generation Sequence and Optical Map Data. Rice 6 (1), 4. doi:10.1186/1939-8433-6-4
Keller, C., Rizwan, M., Davidian, J.-C., Pokrovsky, O. S., Bovet, N., Chaurand, P., et al. (2015). Effect of Silicon on Wheat Seedlings (Triticum Turgidum L). Grown in Hydroponics and Exposed to 0 to 30 ΜM Cu. Planta 241, 847–860. doi:10.1007/s00425-014-2220-1
Kim, Y.-H., Khan, A. L., Waqas, M., and Lee, I.-J. (2017). Silicon Regulates Antioxidant Activities of Crop Plants under Abiotic-Induced Oxidative Stress: a Review. Front. Plant Sci. 8, 510. doi:10.3389/fpls.2017.00510
Kopittke, P. M., Gianoncelli, A., Kourousias, G., Green, K., and McKenna, B. A. (2017). Alleviation of Al Toxicity by Si Is Associated with the Formation of Al-Si Complexes in Root Tissues of Sorghum. Front. Plant Sci. 8, 2189. doi:10.3389/fpls.2017.02189
Larrainzar, E., Molenaar, J. A., Wienkoop, S., Gil-Quintana, E., Alibert, B., Limami, A. M., et al. (2014). Drought Stress Provokes the Down-Regulation of Methionine and Ethylene Biosynthesis Pathways inMedicago Truncatularoots and Nodules. Plant Cel. Environ. 37 (9), 2051–2063. doi:10.1111/pce.12285
Lechner, E., Achard, P., Vansiri, A., Potuschak, T., and Genschik, P. (2006). F-Box Proteins Everywhere. Curr. Opin. Plant Biol. 9 (6), 631–638. doi:10.1016/j.pbi.2006.09.003
Li, P., Song, A., Li, Z., Fan, F., and Liang, Y. (2012). Silicon Ameliorates Manganese Toxicity by Regulating Manganese Transport and Antioxidant Reactions in rice (Oryza Sativa L). Plant Soil 354, 407–419. doi:10.1007/s11104-011-1076-4
Li, X., Hu, B., Pan, X., Zhang, N., and Wu, D. (2017). Association Analysis of Arsenic-Induced Straighthead in rice (Oryza Sativa L). Based on the Selected Population with a Modified Model. Biomed. Res. Int. 2017, 7641362. doi:10.1155/2017/7641362
Li, X., Yan, W., Agrama, H., Jackson, A., Jia, M., Jia, L., et al. (2016). Genetic Analysis of Genetic Basis of a Physiological Disorder “Straighthead” in rice (Oryza Sativa L). Genes Genom. 38, 453–457. doi:10.1007/s13258-016-0394-6
Liang, Y., Chen, Q. i. n., Liu, Q., Zhang, W., and Ding, R. (2003). Exogenous Silicon (Si) Increases Antioxidant Enzyme Activity and Reduces Lipid Peroxidation in Roots of Salt-Stressed Barley (Hordeum vulgareL).. J. Plant Physiol. 160, 1157–1164. doi:10.1078/0176-1617-01065
Linscombe, S. D., Jodari, F., Bollich, P. K., Groth, D. E., White, L. M., Chu, Q. R., et al. (2000). Registration of ‘Cocodrie' Rice. Crop Sci. 40, 294. doi:10.2135/cropsci2000.0007rcv
Liu, H., Long, S.-X., Pinson, S. R. M., Tang, Z., Guerinot, M. L., Salt, D. E., et al. (2021). Univariate and Multivariate QTL Analyses Reveal Covariance Among Mineral Elements in the Rice Ionome. Front. Genet. 12, 638555. doi:10.3389/fgene.2021.638555
Liu, J., Ma, J., He, C., Li, X., Zhang, W., Xu, F., et al. (2013). Inhibition of Cadmium Ion Uptake in rice (Oryza Sativa) Cells by a wall-bound Form of Silicon. New Phytol. 200, 691–699. doi:10.1111/nph.12494
Lüttge, U., and Ratajczak, R. (1997). The Physiology, Biochemistry, and Molecular Biology of the Plant Vacuolar ATPase. Adv. Bot. Res. 25, 253–296.
Ma, C., Burd, S., and Lers, A. (2015). miR408is Involved in Abiotic Stress Responses in Arabidopsis. Plant J. 84, 169–187. doi:10.1111/tpj.12999
Ma, J. F., Yamaji, N., Mitani, N., Xu, X.-Y., Su, Y.-H., McGrath, S. P., et al. (2008). Transporters of Arsenite in rice and Their Role in Arsenic Accumulation in rice Grain. Proc. Natl. Acad. Sci. 105, 9931–9935. doi:10.1073/pnas.0802361105
Macho, A. P., and Zipfel, C. (2014). Plant PRRs and the Activation of Innate Immune Signaling. Mol. Cel 54, 263–272. doi:10.1016/j.molcel.2014.03.028
Maguffin, S. C., Abu-Ali, L., Tappero, R. V., Pena, J., Rohila, J. S., McClung, A. M., et al. (2020). Influence of Manganese Abundances on Iron and Arsenic Solubility in rice Paddy Soils. Geochimica et Cosmochimica Acta 276, 50–69. doi:10.1016/j.gca.2020.02.012
Matsumoto, S., Kasuga, J., Taiki, N., Makino, T., and Arao, T. (2015). Reduction of the Risk of Arsenic Accumulation in rice by the Water Management and Material Application in Relation to Phosphate Status. J. Plant Interactions 10, 65–74. doi:10.1080/17429145.2015.1016129
Meharg, A. A., and Hartley‐Whitaker, J. (2002). Arsenic Uptake and Metabolism in Arsenic Resistant and Nonresistant Plant Species. New Phytol. 154, 29–43. doi:10.1046/j.1469-8137.2002.00363.x
Meharg, A. A., Lombi, E., Williams, P. N., Scheckel, K. G., Feldmann, J., Raab, A., et al. (2008). Speciation and Localization of Arsenic in white and Brown rice Grains. Environ. Sci. Technol. 42 (4), 1051–1057. doi:10.1021/es702212p
Meharg, A. A., Williams, P. N., Adomako, E., Lawgali, Y. Y., Deacon, C., Villada, A., et al. (2009). Geographical Variation in Total and Inorganic Arsenic Content of Polished (White) Rice. Environ. Sci. Technol. 43, 1612–1617. doi:10.1021/es802612a
Meng, X., and Zhang, S. (2013). MAPK Cascades in Plant Disease Resistance Signaling. Annu. Rev. Phytopathol. 51, 245–266. doi:10.1146/annurev-phyto-082712-102314
Mosa, K. A., Kumar, K., Chhikara, S., Mcdermott, J., Liu, Z., Musante, C., et al. (2012). Members of rice Plasma Membrane Intrinsic Proteins Subfamily Are Involved in Arsenite Permeability and Tolerance in Plants. Transgenic Res. 21 (6), 1265–1277. doi:10.1007/s11248-012-9600-8
Mosa, K. A., Kumar, K., Chhikara, S., Musante, C., White, J. C., and Dhankher, O. P. (2016). Enhanced boron Tolerance in Plants Mediated by Bidirectional Transport through Plasma Membrane Intrinsic Proteins. Sci. Rep. 6, 21640. doi:10.1038/srep21640
Murugaiyan, V., Ali, J., Mahender, A., Aslam, U. M., Jewel, Z. A., Pang, Y., et al. (2019). Mapping of Genomic Regions Associated with Arsenic Toxicity Stress in a Backcross Breeding Populations of rice (Oryza Sativa L).. Rice 12, 61. doi:10.1186/s12284-019-0321-y
Nath, S., Panda, P., Mishra, S., Dey, M., Choudhury, S., Sahoo, L., et al. (2014). Arsenic Stress in rice: Redox Consequences and Regulation by Iron. Plant Physiol. Biochem. 80, 203–210. doi:10.1016/j.plaphy.2014.04.013
Norton, G. J., Deacon, C. M., Xiong, L., Huang, S., Meharg, A. A., and Price, A. H. (2010). Genetic Mapping of the rice Ionome in Leaves and Grain: Identification of QTLs for 17 Elements Including Arsenic, Cadmium, Iron and Selenium. Plant Soil 329, 139–153. doi:10.1007/s11104-009-0141-8
Norton, G. J., Douglas, A., Lahner, B., Yakubova, E., Guerinot, M. L., Pinson, S. R. M., et al. (2014). Genome Wide Association Mapping of Grain Arsenic, Copper, Molybdenum and Zinc in rice (Oryza Sativa L). Grown at Four International Field Sites. PLoS ONE 9 (2), e89685. doi:10.1371/journal.pone.0089685
Norton, G. J., Duan, G. L., Lei, M., Zhu, Y.-G., Meharg, A. A., and Price, A. H. (2012a). Identification of Quantitative Trait Loci for rice Grain Element Composition on an Arsenic Impacted Soil: Influence of Flowering Time on Genetic Loci. Ann. Appl. Biol. 161, 46–56. doi:10.1111/j.1744-7348.2012.00549.x
Norton, G. J., Pinson, S. R. M., Alexander, J., Mckay, S., Hansen, H., Duan, G. L., et al. (2012b). Variation in Grain Arsenic Assessed in a Diverse Panel of rice (Oryza Sativa) Grown in Multiple Sites. New Phytol. 193, 650–664. doi:10.1111/j.1469-8137.2011.03983.x
Norton, G. J., Travis, A. J., Talukdar, P., Hossain, M., Islam, M. R., Douglas, A., et al. (2019). Genetic Loci Regulating Arsenic Content in rice Grains when Grown Flooded or under Alternative Wetting and Drying Irrigation. Rice 12, 1. doi:10.1186/s12284-019-0307-9
Nozawa, S., Sato, T., and Otake, T. (2018). Effect of Dissolved Silica on Immobilization of boron by Magnesium Oxide. Minerals 8, 76. doi:10.3390/min8020076
O’Rourke, J. A., Yang, S. S., Miller, S. S., Bucciarelli, B., Liu, J., Rydeen, A., et al. (2013). An RNA-Seq Transcriptome Analysis of Orthophosphate-Deficient White Lupin Reveals Novel Insights into Phosphorus Acclimation in Plants. Plant Physiol. 161, 705–724. doi:10.1104/pp.112.209254
Ouyang, S., Zhu, W., Hamilton, J., Lin, H., Campbell, M., Childs, K., et al. (2006). The TIGR Rice Genome Annotation Resource: Improvements and New Features. Nucleic Acids Res. 35 (Suppl. l_1), D883–D887. doi:10.1093/nar/gkl976
Owino-Gerroh, C., and Gascho, G. J. (2005). Effect of Silicon on Low pH Soil Phosphorus Sorption and on Uptake and Growth of Maize. Commun. Soil Sci. Plant Anal. 35, 2369–2378. doi:10.1081/lcss-200030686
Pan, X., Zhang, Q., Yan, W., Jia, M., Jackson, A., Li, X., et al. (2012). Development of Genetic Markers Linked to Straighthead Resistance through fine Mapping in rice (Oryza Sativa L). PLoS ONE 7, e52540. doi:10.1371/journal.pone.0052540
Panda, D., Mishra, S. S., and Behera, P. K. (2021). Drought Tolerance in rice: Focus on Recent Mechanisms and Approaches. Rice Sci. 28, 119–132. doi:10.1016/j.rsci.2021.01.002
Pavlovic, J., Kostic, L., Bosnic, P., Kirkby, E. A., and Nikolic, M. (2021). Interactions of Silicon with Essential and Beneficial Elements in Plants. Front. Plant Sci. 12, 697592. doi:10.3389/fpls.2021.697592
Pearl, J. (1988). Probabilistic Reasoning in Intelligent Systems: Networks of Plausible Inference. San Francisco: Morgan Kaufman.
Piepho, H. P., and Williams, E. R. (2006). A Comparison Of Experimental Designs For Selection In Breeding Trials With Nested Treatment Structure. Theor. Appl. Genet. 113, 1505–1513. doi:10.1007/s00122-006-0398-8
Pinson, S. R. M., Tarpley, L., Yan, W., Yeater, K., Lahner, B., Yakubova, E., et al. (2015). Worldwide Genetic Diversity for mineral Element Concentrations in rice Grain. Crop Sci. 55, 294–311. doi:10.2135/cropsci2013.10.0656
Raab, A., Feldmann, J., and Meharg, A. A. (2004). The Nature of Arsenic-Phytochelatin Complexes in Holcus Lanatus and Pteris Cretica. Plant Physol. 134, 1113–1122. doi:10.1104/pp.103.033506
Raab, A., Schat, H., Meharg, A. A., and Feldmann, J. (2005). Uptake, Translocation and Transformation of Arsenate and Arsenite in sunflower ( Helianthus Annuus ): Formation of Arsenic-Phytochelatin Complexes during Exposure to High Arsenic Concentrations. New Phytol. 168, 551–558. doi:10.1111/j.1469-8137.2005.01519.x
Sakai, H., Lee, S. S., Tanaka, T., Numa, H., Kim, J., Kawahara, Y., et al. (2013). Rice Annotation Project Database (RAP-DB): an Integrative and Interactive Database for rice Genomics. Plant Cel. Physiol. 54 (2), e6. doi:10.1093/pcp/pcs183
Scutari, M. (2010). Learning Bayesian Networks with the Bnlearn R Package. J. Stat. Soft 35, 1–22. doi:10.18637/jss.v035.i03
Seth, S., Debnath, S., and Chakraborty, N. R. (2020). In Silico analysis of Functional Linkage Among Arsenic Induced MATE Genes in rice. Biotechnol. Rep. 26, e00390. doi:10.1016/j.btre.2019.e00390
Seyfferth, A. L., and Fendorf, S. (2012). Silicate mineral Impacts on the Uptake and Storage of Arsenic and Plant Nutrients in rice (Oryza Sativa L).. Environ. Sci. Technol. 46, 13176–13183. doi:10.1021/es3025337
Seyfferth, A. L., Limmer, M. A., and Dykes, G. E. (2018). On the Use of Silicon as an Agronomic Mitigation Strategy to Decrease Arsenic Uptake by Rice. Rice. Adv. Agron. 149, 49–91. doi:10.1016/bs.agron.2018.01.002
Seyfferth, A. L., Morris, A. H., Gill, R., Kearns, K. A., Mann, J. N., Paukett, M., et al. (2016). Soil Incorporation of Silica-Rich rice Husk Decreases Inorganic Arsenic in rice Grain. J. Agric. Food Chem. 64, 3760–3766. doi:10.1021/acs.jafc.6b01201
Seyfferth, A. L., Webb, S. M., Andrews, J. C., and Fendorf, S. (2010). Arsenic Localization, Speciation, and Co-Occurrence with Iron on rice (Oryza Sativa L). Roots Having Variable Fe Coatings. Environ. Sci. Technol. 44 (21), 8108–8113. doi:10.1021/es101139z
Shalmani, A., Ullah, U., Muhammad, I., Zhang, D., Sharif, R., Jia, P., et al. (2021). The TAZ Domain-Containing Proteins Play Important Role in the Heavy Metals Stress Biology in Plants. Environ. Res. 197, 111030. doi:10.1016/j.envres.2021.111030
Sharma, S., Pinson, S. R. M., Gealy, D. R., and Edwards, J. D. (2021). Genomic Prediction and QTL Mapping of Root System Architecture and Above-Ground Agronomic Traits in rice (Oryza Sativa L). with a Multitrait Index and Bayesian Networks. G3 Genes|Genomes|Genetics 11 (10), jkab178. doi:10.1093/g3journal/jkab178
Song, W.-Y., Yamaki, T., Yamaji, N., Ko, D., Jung, K.-H., Fujii-Kashino, M., et al. (2014). A rice ABC Transporter, OsABCC1, Reduces Arsenic Accumulation in the Grain. Proc. Natl. Acad. Sci. 111, 15699–15704. doi:10.1073/pnas.1414968111
Sze, H., Ward, J. M., and Lai, S. (1992). Vacuolar H+-Translocating ATPases from Plants: Structure, Function, and Isoforms. J. Bioenerg. Biomembr. 24, 371–381. doi:10.1007/bf00762530
Talukdar, P., Douglas, A., Price, A. H., and Norton, G. J. (2015). Biallelic and Genome Wide Association Mapping of Germanium Tolerant Loci in Rice (Oryza Sativa L). PLoS ONE 10 (9), e0137577. doi:10.1371/journal.pone.0137577
Tang, Z., Wang, Y., Gao, A., Ji, Y., Yang, B., Wang, P., et al. (2020a). Dimethylarsinic Acid Is the Causal Agent Inducing rice Straighthead Disease. J. Exp. Bot. 71, 5631–5644. doi:10.1093/jxb/eraa253
Tang, Z., and Zhao, F. J. (2020b). The Roles of Membrane Transporters in Arsenic Uptake, Translocation and Detoxification in Plants. Crit. Rev. Env. Sci. Technol. 51, 2449–2484. doi:10.1080/10643389.1795053
Tripathi, P., Tripathi, R. D., Singh, R. P., Dwivedi, S., Goutam, D., Shri, M., et al. (2013). Silicon Mediates Arsenic Tolerance in rice (Oryza Sativa l). through Lowering of Arsenic Uptake and Improved Antioxidant Defence System. Ecol. Eng. 52, 96–103. doi:10.1016/j.ecoleng.2012.12.057
Turner, S. D. (2014). qqman: an R Package for Visualizing GWAS Results Using Q-Q and Manhattan Plots. bioRxiv, 005165. doi:10.1101/005165
US Food and Drug Administration (2016). Arsenic in rice and rice Products Risk Assessment Report. Available at: http://www.fda.gov/Food/FoodScienceResearch/RiskSafetyAssessment/default.htm (Accessed May 8, 2020).
US Food and Drug Administration (2020). Supporting Document for Action Level for Inorganic Arsenic in Rice Cereals for Infants. Available at https://www.fda.gov/media/97121/download (Accessed 5 8, 2020).
Wang, H., Xu, X., Vieira, F. G., Xiao, Y., Li, Z., Wang, J., et al. (2016). The Power of Inbreeding: NGS-Based GWAS of Rice Reveals Convergent Evolution during Rice Domestication. Mol. Plant 9, 975–985. doi:10.1016/j.molp.2016.04.018
WHO/FAO Joint Expert Committee on Food Additives (2010). Evaluation of Certain Contaminants in Food, 72nd Report of the World Health Organization/Food and Agriculture Organization of the United Nations Joint Expert Committee on Food Additives, Arsenic on. Available at: https://apps.who.int/iris/bitstream/handle/10665/44514/WHO_TRS_959_eng.pdf;jsessionid=9B941773BD2EBDEE5F7674F982732C60?sequence=1 (Accessed September 1, 2021).
Williams, P. N., Villada, A., Deacon, C., Raab, A., Figuerola, J., Green, A. J., et al. (2007). Greatly Enhanced Arsenic Shoot Assimilation in rice Leads to Elevated Grain Levels Compared to Wheat and Barley. Environ. Sci. Technol. 41 (19), 6854–6859. doi:10.1021/es070627i
Wu, Q.-S., Wan, X.-Y., Su, N., Cheng, Z.-J., Wang, J.-K., Lei, C.-L., et al. (2006). Genetic Dissection of Silicon Uptake Ability in rice (Oryza Sativa L). Plant Sci. 171, 441–448. doi:10.1016/j.plantsci.2006.05.001
Wu, X., Yu, Y., Baerson, S. R., Song, Y., Liang, G., Ding, C., et al. (2017). Interactions between Nitrogen and Silicon in rice and Their Effects on Resistance toward the Brown Planthopper Nilaparvata Lugens. Front. Plant Sci. 8, 28. doi:10.3389/fpls.2017.00028
Xu, W., Dai, W., Yan, H., Li, S., Shen, H., Chen, Y., et al. (2015). Arabidopsis NIP3;1 Plays an Important Role in Arsenic Uptake and Root-To-Shoot Translocation under Arsenite Stress Conditions. Mol. Plant 8, 722–733. doi:10.1016/j.molp.2015.01.005
Yamaji, N., and Ma, J. F. (2011). Further Characterization of a rice Silicon Efflux Transporter, Lsi2. Soil Sci. Plant Nutr. 57, 259–264. doi:10.1080/00380768.2011.565480
Yamazaki, Y., Sakaniwa, S., Tsuchiya, R., Nonomura, K. I., and Kurata, N. (2010). Oryzabase: An Integrated Information Resource For Rice Science. Breeding Sci. 60 (5), 544–548. doi:10.1270/jsbbs.60.544
Yan, W., Dilday, R. H., Tai, T. H., Gibbons, J. W., McNew, R. W., and Rutger, J. N. (2005). Differential Response of rice Germplasm to Straighthead Induced by Arsenic. Crop Sci. 45, 1223–1228. doi:10.2135/cropsci2004.0348
Yang, M., Lu, K., Zhao, F.-J., Xie, W., Ramakrishna, P., Wang, G., et al. (2018). Genome-wide Association Studies Reveal the Genetic Basis of Ionomic Variation in rice. Plant Cell 30, 2720–2740. doi:10.1105/tpc.18.00375
Ye, Y., Li, P., Xu, T., Zeng, L., Cheng, D., Yang, M., et al. (2017). OsPT4 Contributes to Arsenate Uptake and Transport in rice. Front. Plant Sci. 8, 2197. doi:10.3389/fpls.2017.02197
Zhang, J., Zhu, Y. G., Zeng, D. L., Cheng, W. D., Qian, Q., and Duan, G. L. (2008). Mapping Quantitative Trait Loci Associated with Arsenic Accumulation in rice (Oryza Sativa). New Phytol. 177 (2), 350–356. doi:10.1111/j.1469-8137.2007.02267.x
Zhang, M., Pinson, S. R. M., Tarpley, L., Huang, X.-Y., Lahner, B., Yakubova, E., et al. (2014). Mapping and Validation of Quantitative Trait Loci Associated with Concentrations of 16 Elements in Unmilled rice Grain. Theor. Appl. Genet. 127, 137–165. doi:10.1007/s00122-013-2207-5
Zhang, Y., Chen, K., Zhao, F.-J., Sun, C., Jin, C., Shi, Y., et al. (2018). OsATX1 Interacts with Heavy Metal P1B-Type ATPases and Affects Copper Transport and Distribution. Plant Physiol. 178, 329–344. doi:10.1104/pp.18.00425
Zhang, Z., Ersoz, E., Lai, C.-Q., Todhunter, R. J., Tiwari, H. K., Gore, M. A., et al. (2010). Mixed Linear Model Approach Adapted for Genome-Wide Association Studies. Nat. Genet. 42 (4), 355–360. doi:10.1038/ng.546
Zhao, Q., Poulson, S. R., Obrist, D., Sumaila, S., Dynes, J. J., McBeth, J. M., et al. (2016). Iron-bound Organic Carbon in forest Soils: Quantification and Characterization. Biogeosciences 13, 4777–4788. doi:10.5194/bg-13-4777-2016
Zheng, N., Schulman, B. A., Song, L., Miller, J. J., Jeffrey, P. D., Wang, P., et al. (2002). Structure of the Cul1-Rbx1-Skp1-F boxSkp2 SCF Ubiquitin Ligase Complex. Nature 416 (6882), 703–709. doi:10.1038/416703a
Keywords: rice, arsenic, straighthead disorder, genome-wide association, bayesian network, QTL
Citation: Pinson SRM, Heuschele DJ, Edwards JD, Jackson AK, Sharma S and Barnaby JY (2022) Relationships Among Arsenic-Related Traits, Including Rice Grain Arsenic Concentration and Straighthead Resistance, as Revealed by Genome-Wide Association. Front. Genet. 12:787767. doi: 10.3389/fgene.2021.787767
Received: 01 October 2021; Accepted: 23 December 2021;
Published: 14 March 2022.
Edited by:
Fanrong Zeng, Yangtze University, ChinaReviewed by:
Tian Qing Zheng, Institute of Crop Sciences (CAAS), ChinaDawei Xue, Hangzhou Normal University, China
Copyright © 2022 Pinson, Heuschele, Edwards, Jackson, Sharma and Barnaby. This is an open-access article distributed under the terms of the Creative Commons Attribution License (CC BY). The use, distribution or reproduction in other forums is permitted, provided the original author(s) and the copyright owner(s) are credited and that the original publication in this journal is cited, in accordance with accepted academic practice. No use, distribution or reproduction is permitted which does not comply with these terms.
*Correspondence: Shannon R. M. Pinson, shannon.pinson@usda.gov