- 1Department of Epidemiology, Center for Global Health, School of Public Health, Nanjing Medical University, Nanjing, China
- 2Thomas E. Starzl Transplantation Institute, University of Pittsburgh Medical Center, University of Pittsburgh, Pittsburgh, PA, United States
- 3Department of Biostatistics, Center for Global Health, School of Public Health, Nanjing Medical University, Nanjing, China
Psychiatric disorder, including bipolar disorder (BD), major depression (MDD), and schizophrenia (SCZ), affects millions of persons around the world. Understanding the disease causal mechanism underlying the three diseases and identifying the modifiable risk factors for them hold the key for the development of effective preventative and treatment strategies. We used a two-sample Mendelian randomization method to assess the causal effect of insomnia on the risk of BD, MDD, and SCZ in a European population. We collected one dataset of insomnia, three of BD, one of MDD, and three of SCZ and performed a meta-analysis for each trait, further verifying the analysis through extensive complementarity and sensitivity analysis. Among the three psychiatric disorders, we found that only insomnia is causally associated with MDD and that higher insomnia increases the risk of MDD. Specifically, the odds ratio of MDD increase of insomnia is estimated to be 1.408 [95% confidence interval (CI): 1.210–1.640, p = 1.03E-05] in the European population. The identified causal relationship between insomnia and MDD is robust with respect to the choice of statistical methods and is validated through extensive sensitivity analyses that guard against various model assumption violations. Our results provide new evidence to support the causal effect of insomnia on MDD and pave ways for reducing the psychiatric disorder burden.
Introduction
Insomnia disorder is predominantly characterized by dissatisfaction with sleep duration or quality and difficulties in initiating or maintaining sleep (Morin et al., 2015; Winkelman, 2015). Most cross-sectional and longitudinal studies have also shown that insomnia increases the risks of acute myocardial infarction and coronary heart disease, heart failure, hypertension, diabetes, and death, particularly when insomnia is accompanied by a short total sleep duration (<6 h per night) (Chen et al., 2013; Morin et al., 2015; Parthasarathy et al., 2015; Grandner et al., 2016; Javaheri and Redline, 2017; Bertisch et al., 2018; Dong and Yang, 2019). Emerging evidence show that insomnia associates to both incident and some recurrent psychiatric disorders, including major depression disorder (MDD), anxiety disorder, substance use problems, and suicidality. In addition, a wide range of sociodemographic correlates of insomnia have been identified and include advanced age, female sex, low socioeconomic status, unemployment, and psychological distress. Although insomnia results from environmental factors, it is, in part, attributable to genetic factors (Winkelman, 2015).
The generation and development of psychiatric disorders are influenced by genetic and environmental factors (Sklar et al., 2011; Nagel et al., 2018; Ruderfer et al., 2018; Peng et al., 2020; Peng et al., 2021a; Peng et al., 2021b). For genetic factors, based on genome-wide association analysis (GWAS), Purcell et al. implicate the major histocompatibility complex, constructed a polygenic risk score (PRS) of schizophrenia (SCZ) and verified that the PRS also predicted bipolar disorder (BD) (Purcell et al., 2009). For environmental factors, using a case–control study, Palagini et al. found that insomnia played a mediating role between early life stress and the clinical manifestations of BD, and assessing the evolution of insomnia symptoms can provide a basis for the characteristics and treatment strategies of BD (Palagini et al., 2021). In addition, the result of longitudinal epidemiological studies shows that sleep disturbances and insomnia increase the risk of MDD after 1–3 years (Riemann and Voderholzer, 2003; Franzen and Buysse, 2008). Studies have also shown that up to 80% of patients with SCZ report symptoms of insomnia (Stummer et al., 2018). Sleep disorders have been shown to increase the risk of cognitive impairment and recurrence in patients with schizophrenia (Stummer et al., 2018). However, all these findings are summarized from either observational studies or pilot randomized controlled trials and prone to selection bias, especially unobserved confounding factors—that is, correlation cannot be simply equal to causal association. It is essential and urgent to further investigate the causal association between insomnia and psychiatric disorders, including BD, MDD, and SCZ.
Based on Mendel’s law of inheritance—that is, parental alleles are randomly assigned to offspring—Mendelian randomization (MR), an advanced statistical method, treats single-nucleotide polymorphism (SNP) as an instrumental variable (IV) to adjust the effect of confounders and identifies the causal relationship between two traits (Davey Smith and Hemani, 2014; Paternoster et al., 2017). Then, when we regarded SNPs both with association to insomnia and without association to psychiatric disorders as IVs, MR can establish the causal relationship between insomnia and psychiatric disorders. Because genetic variants are fixed at conception and cannot be modified subsequently, MR can overcome a possible reverse causation. MR assumes that if insomnia causes psychiatric disorders, SNP related to insomnia causes psychiatric disorders through the insomnia pathway. Emerging large-scale GWAS of insomnia and psychiatric disorders gives us opportunities to use MR to study the causal relationship between them (Sleiman and Grant, 2010).
In the present study, our main aim is to investigate the causal relationship between insomnia and three psychiatric disorders (BD, MDD, and SCZ) in a European ancestry. To achieve the aim, we used the summary statistics of eight datasets (including 386,533 samples of insomnia and 719,027 samples of three psychiatric disorders) to perform a series of two-sample MR to comprehensively elucidate the potential causal association between insomnia and BD, MDD, and SCZ. In addition, to ensure the validity of the results of MR, we performed three sensitivity analyses, including heterogeneity test, pleiotropy test, and leave-one-out (LOO) test, and reverse-direction MR analyses (Zeng et al., 2019; Zeng and Zhou, 2019; Gormley et al., 2021).
Materials and Methods
GWAS Meta-Analysis
We collected eight datasets of insomnia and three psychiatric disorders from the GWAS-ATLAS (https://atlas.ctglab.nl/) (Tian et al., 2020), including one insomnia dataset (Jansen et al., 2019), three BD datasets (Smith et al., 2009; Hou et al., 2016; Ruderfer et al., 2018), one MDD dataset (Howard et al., 2019), and three SCZ datasets (Manolio et al., 2007; Ripke et al., 2013; Pardiñas et al., 2018). The insomnia summary statistics was estimated from the UK Biobank datasets with 386,533 individuals (Prev. = 0.283). The MDD summary statistics was estimated from the UK Biobank datasets with 500,199 individuals (Prev. = 0.341). The three BD summary statistics had 34,950 individuals (Prev. = 0.219), 2,035 individuals (Prev. = 0.492), and 41,653 individuals (Prev. = 0.483), repectively. The three SCZ summary statistics had 32,143 individuals (Prev. = 0.430), 2,729 individuals (Prev. = 0.495), and 105,318 individuals (Prev. = 0.386), repectively. The three studies for BD and MDD were without any overlap individuals. All summary statistics were estimated in the European ancestry. Then, we filtered out SNPs 1) with INFO <0.6, 2) with MAF <0.01, 3) with palindromic allele, and 4) whose OR was larger or smaller than mean ±3 SD. Finally, we obtained 7,213,582, 9,018,454, 7,743,682, and 8,679,614 SNPs for the four traits. Details of the meta-dataset and the three datasets for BD and SCZ are shown in Table 1 and Supplementary Table S1.
Furthermore, to obtain an accurate and robust estimation for each variant, we performed GWAS meta-analysis for each trait using METAL (v2011-03-25) (Willer et al., 2010). To control the population stratification, we set the option GENOMICCONTROL to on. In addition, we used Linkage Disequilibrium SCore regression (LDSC) (v1.0.1) to estimate both the observed and liability observed heritability (h2) for each trait. We set the population prevalence (--pop-prev) for the four traits to 0.300, 0.020, 0.086, and 0.010 to estimate liability heritability, respectively (Ayuso-Mateos et al., 2001; Roth, 2007; Di Luca et al., 2011). We also estimated the genetic correlation (Rg) between them in the GWAS analysis results (Tylee et al., 2018).
IV Selection
Based on the meta-datasets, we followed the strict selection procedure for selecting IVs in other previous MR studies (Zeng et al., 2019; Dong et al., 2021) (Figure 1). First, we retained 463 variants for insomnia with a P-value smaller than 5.00E-8. Second, we excluded 450 highly correlated variants with r2 greater than 0.001 in the range of 10 Mb. In addition, following Zeng et al. (2019), we used F statistic to test for weak IVs, and no variant was excluded with a minimum F statistic of 39.37. Finally, we retained a total of 13 independent candidate IVs for studying the causal relationship between insomnia and BD, MDD, and SCZ. The details are shown in Supplementary Table S2.
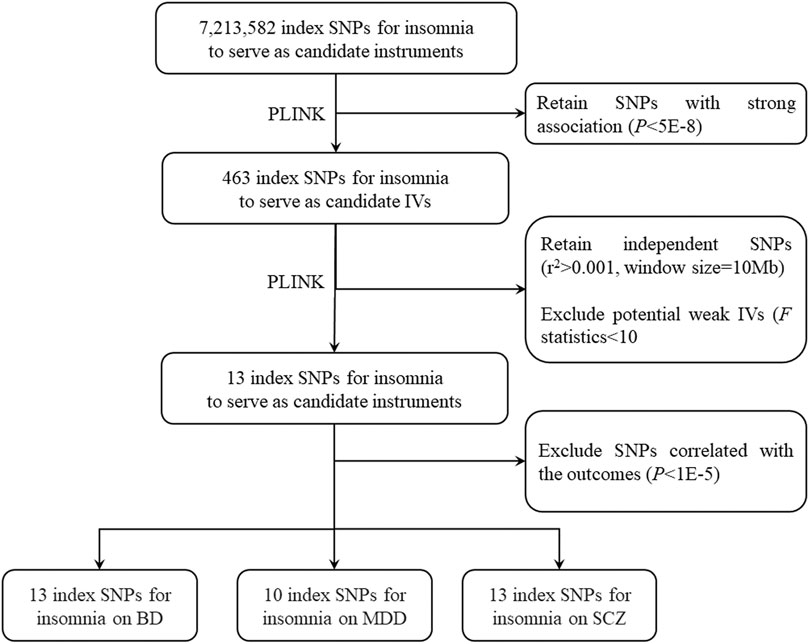
FIGURE 1. Flow chart for instrumental variable (IV) selection. The flow chart shows the selection process of insomnia IVs to estimate the causal effects on bipolar disorder (BD), major depression (MDD), and schizophrenia (SCZ). First, we use p < 5.00E-8 to select index single-nucleotide polymorphisms (SNPs) to ensure that they strongly associate with insomnia. Second, we use r2 > 0.001 in the range of 10,000 Mb to select independent index SNPs. We treat the EUR of 1000 Genome Project as the reference panel. The first two steps are completed by PLINK. Finally, we obtain 13 IVs on BD, 10 IVs on MDD, and 13 IVs on SCZ.
We performed three two-sample MR analyses, including inverse variance weighted (IVW), MR-Egger, and weighted median (WM) method, to estimate the potential causal effect of insomnia to BD, MDD, and SCZ (Bowden et al., 2015; Bowden et al., 2016a). Without consideration for the intercept term, IVW regarded the reciprocal of the outcome variance as the weight. Because of no pleiotropy assumption, IVW was biased when pleiotropy exists (Bowden et al., 2015). Differently to IVW, MR-Egger used an intercept term to measure the horizontal pleiotropy between IVs (Bowden et al., 2016b). The weighted median method assumed that variables that account for at least 50% of the total IVs were valid, so the causal effects could be estimated consistently (Bowden et al., 2016a). We also used MR-Egger intercept to test pleiotropy (Hemani et al., 2018; Verbanck et al., 2018; Ong and MacGregor, 2019). All the analyses are performed by R software (v4.1.1). We specially used TwoSampleMR R package (v0.5.6) to perform a MR analysis.
Sensitivity Analysis
Following Noyce et al. (2017), Zeng et al. (2019), and Zeng and Zhou (2019), we performed a sensitivity analysis to evaluate the potential violations of the model assumptions in the MR analysis: 1) heterogeneity test, 2) pleiotropic test, and 3) LOO sensitivity test. First, heterogeneity analysis estimates heterogeneity between IVs. If heterogeneity existed, it is hardly to direct combinations of IVs. We used the P-value of Q statistics (PQ) < 0.05 as the significant level. Second, we used MR-PRESSO to test pleiotropy, resulting in serious deviations in MR (Hemani et al., 2018; Ong and MacGregor, 2019). Finally, by gradually excluding each variant, LOO estimated the causal effect of the remaining variants and tested whether the difference between each causal effect is significant. Ideally, defining no significant difference meant a robust result (Noyce et al., 2017). The statistically significant level was set to 0.05.
Reverse-Direction MR Analyses
We also performed reverse-direction MR to assess the potential reverse causal effects of BD, MDD, and SCZ on insomnia. Following Savage et al. (2018) and Dong et al. (2021), we used the same settings as the abovementioned MR analysis (p = 5.00E-8, r2 = 0.001, and window size = 10 Mb). We obtained 36 IVs for BD, 44 IVs for MDD, and 50 IVs for SCZ. We used these IVs of three psychiatric disorders to perform reverse causal inferences on insomnia to assess the potential reverse causal effects. The reverse-direction MR analysis process is the same as previously described.
Results
Summary of GWAS Meta-Data and Genetic Correlation
We used the meta-analysis datasets to estimate the genetic correlation. The genetic inflation factor (λgc) of insomnia is 1.310 (LDSC intercept: 1.015), the λgc of BD is 1.421 (LDSC intercept: 1.080), the λgc of MDD is 1.453 (LDSC intercept: 1.000), and the λgc of SCZ is 1.637 (LDSC intercept: 1.044). The LDSC of the four traits are not larger than 1, which indicates that the meta-datasets are without population stratification. Using GWAS summary statistics to estimate SNP-based observed and liability heritability, these are 0.046 and 0.082 for insomnia, 0.405 and 0.286 for BD, 0.060 and 0.067 for MDD, and 0.295 and 0.170 for SCZ, respectively (Table 1). We use Manhattan plot and qqplot to show the GWAS results for the four traits (Supplementary Figure S1).
In addition, we assessed the genetic correlation between BD, MDD, SCZ, and insomnia using cross-trait LDSC. Insomnia was significantly genetically correlated to MDD (Rg = 0.469, p = 2.01E-70), while it was not significantly genetically correlated to BD (Rg = 0.022, p = 0.462) and SCZ (Rg = 0.027, p = 0.276). As expected, we defined three significant genetic correlations between the three psychiatric disorders: genetic correlation between BD and MDD (Rg = 0.287, p = 5.72E-26), between BD and SCZ (Rg = 0.662, p = 5.6E-283), and between MDD and SCZ (Rg = 0.327, p = 4.91E-42) (Figure 2).
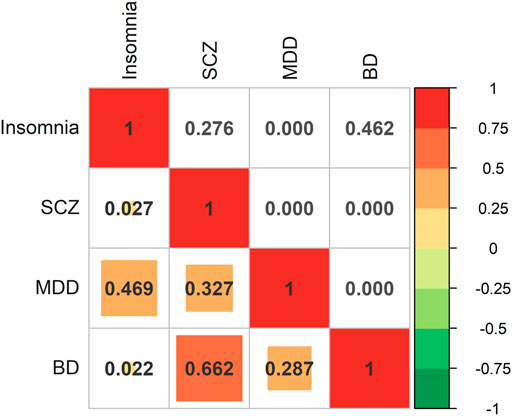
FIGURE 2. The genetic correlation of four traits using Linkage Disequilibrium SCore regression. The heat map shows the Rg (lower triangle) and its P-value (upper triangle). The red color shows a more positive correlation, while the blue color shows a more negative correlation. We use abbreviations to indicate various psychiatric disorders: BD, bipolar disorder; MDD, major depression; SCZ, schizophrenia.
MR Analysis
We use the 13 potential IVs of insomnia with the three psychiatric disorders one by one. Specifically, three psychiatric disorders had 13, 10, and 13 IVs, respectively (Supplementary Table S2). Based on different assumptions, we estimate the potential causal effect by all four models, including IVW (fixed- and random-effects model), MR-Egger, and WM. We use the forest plot to show the potential causal effect of the four methods, scatter plot to show the IV effect of insomnia and three psychiatric disorders, and funnel plot to show the relationship between effect of MR model and effect of each SNP (Figure 3, Supplementary Figures S2, S3, Supplementary Tables S3–S5).
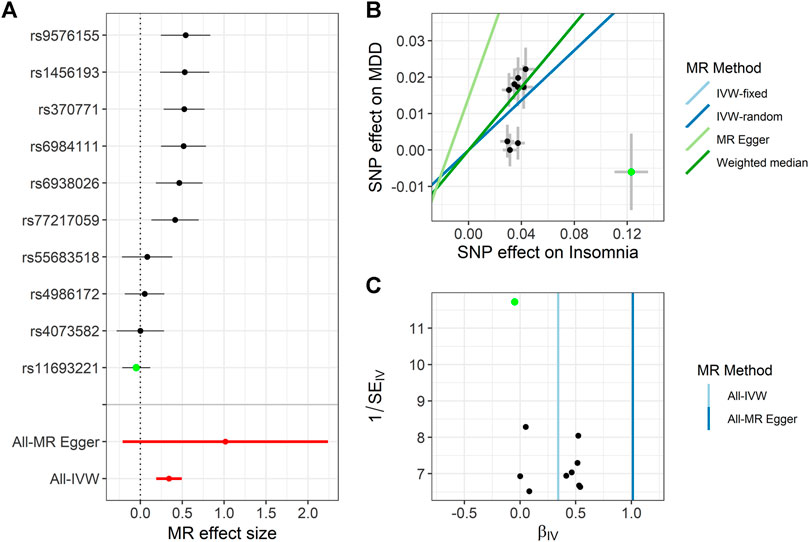
FIGURE 3. Summary of the Mendelian randomization (MR) analysis for insomnia on major depression (MDD). (A) MR effect size of each instrumental variable (IV), MR-Egger, and inverse variance weighted (IVW). (B) Scatter plot of causal effects of insomnia on MDD. We use vertical and horizontal black lines to show 95% CI of the estimated effect of IVs on MDD (x-axis) and that on insomnia (y-axis), respectively. We use the blue line to show the IVW random-effects model. The potential SNP outlier (rs11693221) is highlighted in green. (C) Funnel plot of the causal effect of insomnia on MDD. Each point represents the estimated causal effect of each IV. The vertical dark blue line represents the causal effect estimate obtained using the MR-Egger method; the light blue line represents the causal effect estimate obtained using the IVW method. The potential outlier (rs11693221) is highlighted in green.
For MDD, the estimated OR from fixed-effects IVW method is 1.288 (95% CI: 1.189–1.395), with p = 5.630E-11. As expected, the result of the random-effects IVW method (OR = 1.288, 95% CI: 1.091–1.520, p = 0.003) is similar to that of the random-effects IVW. However, the result of WM (OR = 1.076, 95% CI: 0.915–1.216, p = 0.374) and MR-Egger (OR = 0.916, 95% CI: 0.599–1.401, p = 0.696) is not similar to that of IVW (Figure 4, Supplementary Table S4). The abovementioned results indicate that the risk of MDD increases with the increasing level of insomnia. We should use the result of the sensitivity analysis to determine which one is the main result.
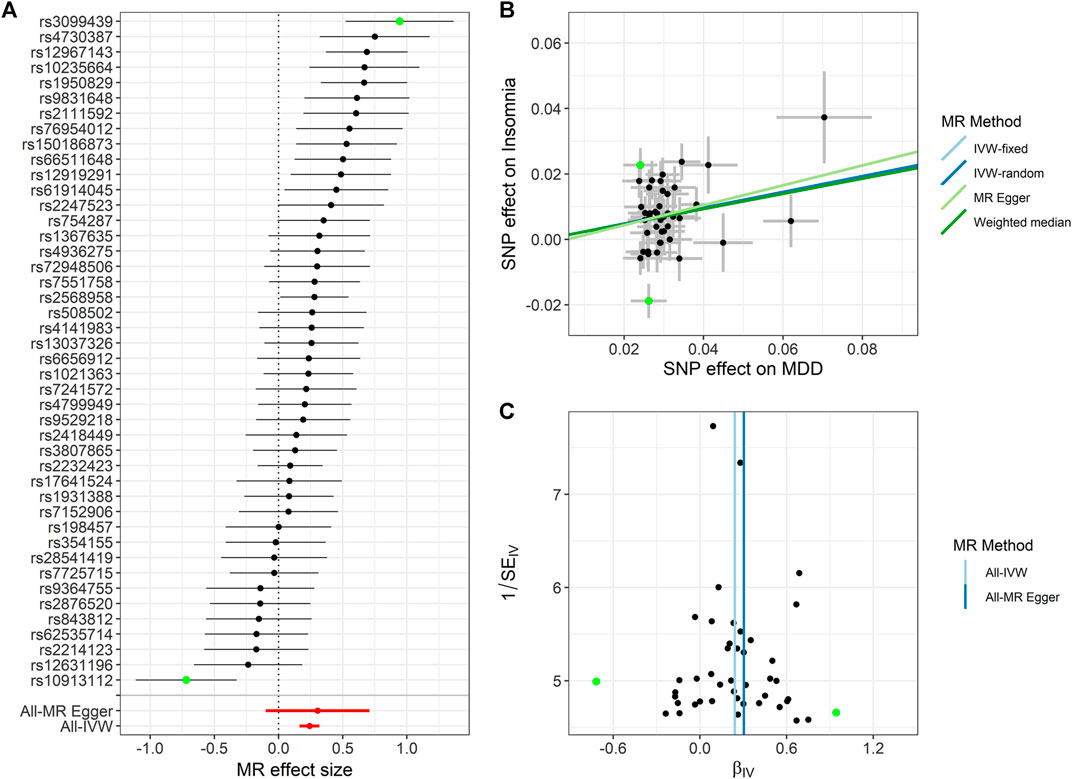
FIGURE 4. Summary of the reverse-directional Mendelian randomization (MR) analysis for insomnia on major depression (MDD). (A) Reverse-directional MR effect size of each instrumental variable (IV), MR-Egger, and inverse variance weighted (IVW). (B) Scatter plot of the causal effects of MDD on insomnia. We use vertical and horizontal black lines to show 95% CI of the estimated effect of IVs on MDD (x-axis) and that on insomnia (y-axis), respectively. We use the blue line to show the IVW random-effects model. The potential SNP outliers (rs3099439 and rs10913112) are highlighted in green. (C) Funnel plot of the causal effect of MDD on insomnia. Each point represents the estimated causal effect of each IV. The vertical dark blue line represents the causal effect estimate obtained using the MR-Egger method; the light blue line represents the causal effect estimate obtained using the IVW method. The potential outliers (rs3099439 and rs10913112) are highlighted in green.
For BD, in terms of the fixed-effects IVW method, the estimated OR of insomnia is 1.216 (95% CI: 0.974–1.518, p = 0.084). As expected, the result of the random-effects IVW method is similar to that of the fixed-effects method, with OR = 1.216 (95% CI: 0.801–1.845) and p = 0.358. The results of WM (OR = 1.351, 95% CI: 0.948–1.917, p = 0.096) and MR-Egger (OR = 1.909, 95% CI: 0.506–7.197, p = 0.360) are similar (Supplementary Figure S2, Supplementary Table S3). Unfortunately, the results of all MR methods are not significant, suggesting that there might be no potential causal association for insomnia on BD. The specific results have to be verified after a sensitivity analysis.
Finally, for SCZ, the estimated OR of insomnia by the fixed-effects IVW method is 0.787 (95% CI: 0.630–0.983, p = 0.035), while the OR from the random-effect model is 0.787 (95% CI: 0.479–1.292, p = 0.344). In addition, the results of the weighted median method (OR = 0.604, 95% CI: 0.413–0.883, p = 0.009) and MR-Egger (OR = 0.566, 95% CI: 0.109–2.950, p = 0.513) are different (Supplementary Figure S3, Supplementary Table S5). Similarly, which specific result is representative also needs to be determined after the sensitivity analysis.
Sensitivity Analyses
Using three kinds of MR methods, we identify the potential causal relationship of insomnia on MDD (IVW method) and SCZ (only WM method). We performed a series of sensitivity analyses to assess whether the results obtained are robust, whether there is potential bias (such as pleiotropy and data heterogeneity), and whether there is a certain IV that seriously affects the outcome variable.
First, we conducted a heterogeneity analysis. Based on IVW, the PQ values of BD, MDD, and SCZ are 2.83E-5, 1.39E-5, and 2.4E-8, respectively. Following Zeng et al. (2019), we selected the result from the random-effects model or deleted SNPs with P-value < 1.00E-5. Because of the similarity between the fix- and random-effects IVW methods, we deleted SNPs with P-value < 1.00E-5 to reduce heterogeneity. For BD, excluding rs6938026, the heterogeneity (PQ = 0.010) is reduced. For MDD, the heterogeneity (PQ = 0.004) is reduced after excluding rs11693221. The heterogeneity of SCZ (PQ = 0.078) is also reduced after excluding rs6938026 and rs370771.
In addition, we performed a series of pleiotropic tests to further ensure the validation of MR analysis. For MDD, MR-Egger showed that the intercept is not statistically significant (p = 0.131), which indicated that there was no horizontal pleiotropy that existed among IVs. The MR-PRESSO outlier test suggested that rs11693221 (RSSobs = 2.32E-3, p < 0.01) was a potential outlier. We used the MR-PRESSO distortion test and LOO test to test whether the causal effect changed with or without the outlier, but their results were different (PMR-PRESSO = 0.282 and PLOO = 1.03E-05). Then, even excluding the outlier, the heterogeneity test showed statistically significant heterogeneity (PQ = 0.004). Therefore, we used the result from random-effects IVW method with the outlier excluded to represent the casual effect of insomnia on MDD (OR = 1.408, 95%CI: 1.209–1.640, p = 1.03E-05) (Figure 3 and Supplementary Table S4).
For BD, MR-Egger showed that the intercept is not statistically significant (p = 0.496). The MR-PRESSO outlier test suggested that rs6938026 (RSSobs = 2.89E-3, p < 0.01) and rs77960 (RSSobs = 2.28E-3, p = 0.013) were the potential outliers. However, the MR-PRESSO distortion test and LOO test indicated that no statistical significance could be identified when excluding the two variants (PMR-PRESSO = 0.979, PLOO1 = 0.147, and PLOO1 = 0.622). Though heterogeneity was reduced without the two outliers, we fail to define a statistically significant causal effect for insomnia on BD (Supplementary Figure S2 and Supplementary Table S3).
For SCZ, MR-Egger showed that the intercept is not statistically significant (p = 0.689). The MR-PRESSO outlier test indicated that four SNPs, including rs1456193, rs370771, rs4986172, and rs6938026, were identified as potential outliers. However, the MR-PRESSO distortion test and LOO test indicated that no statistical significance could be identified when excluding the two variants (PMR-PRESSO = 0.738, PLOO1 = 0.172, PLOO2 = 0.588, PLOO3 = 0.096, and PLOO4 = 0.613). Though there was no significant heterogeneity between models with and without outliers, we used the result from the random-effects IVW method with the outliers excluded to represent the casual effect of insomnia on SCZ for caution (OR = 0.752, 95%CI: 0.524–1.079, p = 0.122) (Supplementary Figure S3 and Supplementary Table S5).
Reverse-Direction MR Analysis
Following a previous MR analysis (Hartwig et al., 2017; Dong et al., 2021), in order to identify the potential confounding factors that mislead the direction of causal effects, we performed reverse-direction MR (Figure 4, Supplementary Figures S4, S5). We found that MDD and SCZ have a significantly potential causal association to insomnia, while a potential causal effect for BD on insomnia is not significant. Specifically, using IVW, the estimated OR for MDD and SCZ on insomnia is 1.273 (p = 1.097E-9) and 1.028 (p = 0.004), respectively (Figure 4 and Supplementary Figure S4). The results indicate that the risk of BD and SCZ could increase the risk of insomnia.
Discussion
Using the summary statistics of four traits and reference LD panel from public sources, we performed a two-sample MR analysis to show the causal effects of insomnia on three psychiatric disorders. We found that the causal OR of insomnia on MDD is 1.288, that the reverse direction causal OR of MDD on insomnia is 1.230, and that no statistical significance is defined for insomnia on BD and SCZ. These results were based on several MR methods to guard against potential model misspecifications and is consistent in the estimates of causal effects, suggesting that the findings are convincing.
As we have known, many observational studies aim to explore the associations between insomnia and BD, MDD, and SCZ. A case–control study found that insomnia significantly affected patients with BP with depressive symptoms (OR = 4.17, p = 0.043), and sleep disturbances also predicted manic symptoms (OR = 8.69, p = 0.001) (Palagini et al., 2020). Integrating 21 observational studies for insomnia on DP, Baglioni et al. show that the overall OR of insomnia is 2.60 (Baglioni et al., 2011). A cross-sectional study found that the effect size of insomnia-caused symptoms of depression or anxiety is 3.01 (Batalla-Martín et al., 2020). The abovementioned studies have shown that insomnia is a risk factor to psychiatric disorders. Differently to previous studies, the effect size from MR is directional.
The causal relationship between insomnia and BD, MDD, and SCZ identified in the European population was estimated using the IVs of insomnia in three different outcomes. However, we also recognize that there is still a large amount of unexplainable diversity in the etiology of BD, MDD, and SCZ in European populations. Further research is needed to understand the genetic and environmental factors behind the differences between BD, MDD, and SCZ. Although many studies have confirmed the potential impact of insomnia symptoms on some psychiatric disorders, as mentioned earlier, there is no clear answer yet, and it is not clear whether insomnia has a significant causal effect on these diseases.
Like other MR analyses, our results are not without any drawbacks. First, MR cannot completely exclude all confounding factors because the relationship between exposure and outcome obtained through the observational data used in MR analysis is not a pure relationship between exposure and outcome (Sekula et al., 2016; Ference et al., 2019). In our research, it may be because the sample size of BD and SCZ is relatively small compared with insomnia, and the effect of exposure on the results is relatively weak. The statistical power of MR analysis for certain exposures is limited, resulting in negative results (Pierce and Burgess, 2013). Second, we defined the bidirectional causal association between insomnia and MDD. This plays an important supplement to support the causal association, such that it is hard to detangle the relationship between them using either a cross-sectional study or a MR analysis (Manber and Chambers, 2009; Fang et al., 2019). Nevertheless, our study also provides help for new developments in psychiatric disorder research and new treatment strategies in the future (Hertenstein et al., 2019).
Conclusion
The result of the MR and additional analyses shows that insomnia has a positive causal effect on MDD in the European population and provides new evidence of the causal relationship with insomnia on BD and SCZ in European populations.
Data Availability Statement
The original contributions presented in the study are included in the article/Supplementary Material, further inquiries can be directed to the corresponding author.
Author Contributions
SY designed the study. XZ, XY, and YW performed the datasets quality control. YZ and XY performed the data analysis. PH, XZ, and XY interpreted the analysis results. PH and YZ wrote the draft manuscript. RY and SY revised the article. All the authors accepted the final manuscript.
Funding
This work was supported by the National Natural Science Foundation of China (No. 81703321) and the Priority Academic Program Development of Jiangsu Higher Education Institutions (PAPD).
Conflict of Interest
The authors declare that the research was conducted in the absence of any commercial or financial relationships that could be construed as a potential conflict of interest.
The reviewer PZ declared a past co-authorship with one of the authors SY to the handling editor.
Publisher’s Note
All claims expressed in this article are solely those of the authors and do not necessarily represent those of their affiliated organizations, or those of the publisher, the editors and the reviewers. Any product that may be evaluated in this article, or claim that may be made by its manufacturer, is not guaranteed or endorsed by the publisher.
Acknowledgments
We acknowledge the participants and investigators of GWAS-ALTAS for making the summary data publicly available for us.
Supplementary Material
The Supplementary Material for this article can be found online at: https://www.frontiersin.org/articles/10.3389/fgene.2021.763259/full#supplementary-material
References
Ayuso-Mateos, J. L., Vázquez-Barquero, J. L., Dowrick, C., Lehtinen, V., Dalgard, O. S., Casey, P., et al. (2001). Depressive Disorders in Europe: Prevalence Figures from the ODIN Study. Br. J. Psychiatry 179, 308–316. doi:10.1192/bjp.179.4.308
Baglioni, C., Battagliese, G., Feige, B., Spiegelhalder, K., Nissen, C., Voderholzer, U., et al. (2011). Insomnia as a Predictor of Depression: a Meta-Analytic Evaluation of Longitudinal Epidemiological Studies. J. Affective Disord. 135, 10–19. doi:10.1016/j.jad.2011.01.011
Batalla-Martín, D., Belzunegui-Eraso, A., Miralles Garijo, E., Martínez Martín, E., Romaní Garcia, R., Heras, J. S. M., et al. (2020). Insomnia in Schizophrenia Patients: Prevalence and Quality of Life. Ijerph 17, 1350. doi:10.3390/ijerph17041350
Bertisch, S. M., Pollock, B. D., Mittleman, M. A., Buysse, D. J., Bazzano, L. A., Gottlieb, D. J., et al. (2018). Insomnia with Objective Short Sleep Duration and Risk of Incident Cardiovascular Disease and All-Cause Mortality: Sleep Heart Health Study. Sleep 41. doi:10.1093/sleep/zsy047
Bowden, J., Davey Smith, G., and Burgess, S. (2015). Mendelian Randomization with Invalid Instruments: Effect Estimation and Bias Detection through Egger Regression. Int. J. Epidemiol. 44, 512–525. doi:10.1093/ije/dyv080
Bowden, J., Davey Smith, G., Haycock, P. C., and Burgess, S. (2016a). Consistent Estimation in Mendelian Randomization with Some Invalid Instruments Using a Weighted Median Estimator. Genet. Epidemiol. 40, 304–314. doi:10.1002/gepi.21965
Bowden, J., Del Greco M., F., Minelli, C., Davey Smith, G., Sheehan, N. A., and Thompson, J. R. (2016b). Assessing the Suitability of Summary Data for Two-Sample Mendelian Randomization Analyses Using MR-Egger Regression: the Role of the I2 Statistic. Int. J. Epidemiol. 45, dyw220–1974. doi:10.1093/ije/dyw220
Chen, H.-C., Su, T.-P., and Chou, P. (2013). A Nine-Year Follow-Up Study of Sleep Patterns and Mortality in Community-Dwelling Older Adults in Taiwan. Sleep 36, 1187–1198. doi:10.5665/sleep.2884
Davey Smith, G., and Hemani, G. (2014). Mendelian Randomization: Genetic Anchors for Causal Inference in Epidemiological Studies. Hum. Mol. Genet. 23, R89–R98. doi:10.1093/hmg/ddu328
Di Luca, M., Baker, M., Corradetti, R., Kettenmann, H., Mendlewicz, J., Olesen, J., et al. (2011). Consensus Document on European Brain Research. Eur. J. Neurosci. 33, 768–818. doi:10.1111/j.1460-9568.2010.07596.x
Dong, S.-S., Zhang, K., Guo, Y., Ding, J.-M., Rong, Y., Feng, J.-C., et al. (2021). Phenome-wide Investigation of the Causal Associations between Childhood BMI and Adult Trait Outcomes: a Two-Sample Mendelian Randomization Study. Genome Med. 13, 48. doi:10.1186/s13073-021-00865-3
Dong, Y., and Yang, F. M. (2019). Insomnia Symptoms Predict Both Future Hypertension and Depression. Prev. Med. 123, 41–47. doi:10.1016/j.ypmed.2019.02.001
Fang, H., Tu, S., Sheng, J., and Shao, A. (2019). Depression in Sleep Disturbance: A Review on a Bidirectional Relationship, Mechanisms and Treatment. J. Cel Mol Med 23, 2324–2332. doi:10.1111/jcmm.14170
Ference, B. A., Ray, K. K., Catapano, A. L., Ference, T. B., Burgess, S., Neff, D. R., et al. (2019). Mendelian Randomization Study of ACLY and Cardiovascular Disease. N. Engl. J. Med. 380, 1033–1042. doi:10.1056/NEJMoa1806747
Franzen, P. L., and Buysse, D. J. (2008). Sleep Disturbances and Depression: Risk Relationships for Subsequent Depression and Therapeutic Implications. Dialogues Clin. Neurosci. 10, 473–481. doi:10.31887/DCNS.2008.10.4/plfranzen
Gormley, M., Yarmolinsky, J., Dudding, T., Burrows, K., Martin, R. M., Thomas, S., et al. (2021). Using Genetic Variants to Evaluate the Causal Effect of Cholesterol Lowering on Head and Neck Cancer Risk: A Mendelian Randomization Study. Plos Genet. 17, e1009525. doi:10.1371/journal.pgen.1009525
Grandner, M. A., Seixas, A., Shetty, S., and Shenoy, S. (2016). Sleep Duration and Diabetes Risk: Population Trends and Potential Mechanisms. Curr. Diab Rep. 16, 106. doi:10.1007/s11892-016-0805-8
Hartwig, F. P., Borges, M. C., Horta, B. L., Bowden, J., and Davey Smith, G. (2017). Inflammatory Biomarkers and Risk of Schizophrenia. JAMA Psychiatry 74, 1226–1233. doi:10.1001/jamapsychiatry.2017.3191
Hemani, G., Bowden, J., and Davey Smith, G. (2018). Evaluating the Potential Role of Pleiotropy in Mendelian Randomization Studies. Hum. Mol. Genet. 27, R195–r208. doi:10.1093/hmg/ddy163
Hertenstein, E., Feige, B., Gmeiner, T., Kienzler, C., Spiegelhalder, K., Johann, A., et al. (2019). Insomnia as a Predictor of Mental Disorders: A Systematic Review and Meta-Analysis. Sleep Med. Rev. 43, 96–105. doi:10.1016/j.smrv.2018.10.006
Hou, L., Bergen, S. E., Akula, N., Song, J., Hultman, C. M., Landén, M., et al. (2016). Genome-wide Association Study of 40,000 Individuals Identifies Two Novel Loci Associated with Bipolar Disorder. Hum. Mol. Genet. 25, 3383–3394. doi:10.1093/hmg/ddw181
Howard, D. M., Adams, M. J., Adams, M. J., Clarke, T.-K., Hafferty, J. D., Gibson, J., et al. (2019). Genome-wide Meta-Analysis of Depression Identifies 102 Independent Variants and Highlights the Importance of the Prefrontal Brain Regions. Nat. Neurosci. 22, 343–352. doi:10.1038/s41593-018-0326-7
Jansen, P. R., Watanabe, K., Watanabe, K., Stringer, S., Skene, N., Bryois, J., et al. (2019). Genome-wide Analysis of Insomnia in 1,331,010 Individuals Identifies New Risk Loci and Functional Pathways. Nat. Genet. 51, 394–403. doi:10.1038/s41588-018-0333-3
Javaheri, S., and Redline, S. (2017). Insomnia and Risk of Cardiovascular Disease. CHEST 152, 435–444. doi:10.1016/j.chest.2017.01.026
Manber, R., and Chambers, A. S. (2009). Insomnia and Depression: A Multifaceted Interplay. Curr. Psychiatry Rep. 11, 437–442. doi:10.1007/s11920-009-0066-1
Manolio, T. A., Rodriguez, L. L., Brooks, L., Abecasis, G., Ballinger, D., Daly, M., et al. (2007). New Models of Collaboration in Genome-wide Association Studies: the Genetic Association Information Network. Nat. Genet. 39, 1045–1051. doi:10.1038/ng2127
Morin, C. M., Drake, C. L., Harvey, A. G., Krystal, A. D., Manber, R., Riemann, D., et al. (2015). Insomnia Disorder. Nat. Rev. Dis. Primers 1, 15026. doi:10.1038/nrdp.2015.26
Nagel, M., Jansen, P. R., Jansen, P. R., Stringer, S., Watanabe, K., de Leeuw, C. A., et al. (2018). Meta-analysis of Genome-wide Association Studies for Neuroticism in 449,484 Individuals Identifies Novel Genetic Loci and Pathways. Nat. Genet. 50, 920–927. doi:10.1038/s41588-018-0151-7
Noyce, A. J., Kia, D. A., Hemani, G., Nicolas, A., Price, T. R., De Pablo-Fernandez, E., et al. (2017). Estimating the Causal Influence of Body Mass index on Risk of Parkinson Disease: A Mendelian Randomisation Study. Plos Med. 14, e1002314. doi:10.1371/journal.pmed.1002314
Ong, J. S., and Macgregor, S. (2019). Implementing MR‐PRESSO and GCTA‐GSMR for Pleiotropy Assessment in Mendelian Randomization Studies from a Practitioner's Perspective. Genet. Epidemiol. 43, 609–616. doi:10.1002/gepi.22207
Palagini, L., Miniati, M., Caruso, D., Massa, L., Novi, M., Pardini, F., et al. (2020). Association between Affective Temperaments and Mood Features in Bipolar Disorder II: The Role of Insomnia and Chronobiological Rhythms Desynchronization. J. Affective Disord. 266, 263–272. doi:10.1016/j.jad.2020.01.134
Palagini, L., Miniati, M., Marazziti, D., Sharma, V., and Riemann, D. (2021). Association Among Early Life Stress, Mood Features, Hopelessness and Suicidal Risk in Bipolar Disorder: The Potential Contribution of Insomnia Symptoms. J. Psychiatr. Res. 135, 52–59. doi:10.1016/j.jpsychires.2020.12.069
Pardiñas, A. F., Holmans, P., Holmans, P., Pocklington, A. J., Escott-Price, V., Ripke, S., et al. (2018). Common Schizophrenia Alleles Are Enriched in Mutation-Intolerant Genes and in Regions under strong Background Selection. Nat. Genet. 50, 381–389. doi:10.1038/s41588-018-0059-2
Parthasarathy, S., Vasquez, M. M., Halonen, M., Bootzin, R., Quan, S. F., Martinez, F. D., et al. (2015). Persistent Insomnia Is Associated with Mortality Risk. Am. J. Med. 128, 268–275.e262. doi:10.1016/j.amjmed.2014.10.015
Paternoster, L., Tilling, K., and Davey Smith, G. (2017). Genetic Epidemiology and Mendelian Randomization for Informing Disease Therapeutics: Conceptual and Methodological Challenges. Plos Genet. 13, e1006944. doi:10.1371/journal.pgen.1006944
Peng, J., Guan, J., Hui, W., and Shang, X. (2021a). A Novel Subnetwork Representation Learning Method for Uncovering Disease-Disease Relationships. Methods 192, 77–84. doi:10.1016/j.ymeth.2020.09.002
Peng, J., Wang, Y., Guan, J., Li, J., Han, R., Hao, J., et al. (2021b). An End-To-End Heterogeneous Graph Representation Learning-Based Framework for Drug-Target Interaction Prediction. Brief. Bioinform. 22. doi:10.1093/bib/bbaa430
Peng, J., Xue, H., Wei, Z., Tuncali, I., Hao, J., and Shang, X. (2020). Integrating Multi-Network Topology for Gene Function Prediction Using Deep Neural Networks. Brief. Bioinform. 22, 2096–2105. doi:10.1093/bib/bbaa036
Pierce, B. L., and Burgess, S. (2013). Efficient Design for Mendelian Randomization Studies: Subsample and 2-sample Instrumental Variable Estimators. Am. J. Epidemiol. 178, 1177–1184. doi:10.1093/aje/kwt084
Purcell, S. M., Wray, N. R., Stone, J. L., Visscher, P. M., O'donovan, M. C., Sullivan, P. F., et al. (2009). Common Polygenic Variation Contributes to Risk of Schizophrenia and Bipolar Disorder. Nature 460, 748–752. doi:10.1038/nature08185
Riemann, D., and Voderholzer, U. (2003). Primary Insomnia: a Risk Factor to Develop Depression?. J. Affective Disord. 76, 255–259. doi:10.1016/s0165-0327(02)00072-1
Ripke, S., O'dushlaine, C., O'Dushlaine, C., Chambert, K., Moran, J. L., Kähler, A. K., et al. (2013). Genome-wide Association Analysis Identifies 13 New Risk Loci for Schizophrenia. Nat. Genet. 45, 1150–1159. doi:10.1038/ng.2742
Roth, T. (2007). Insomnia: Definition, Prevalence, Etiology, and Consequences. J. Clin. Sleep Med. 3, S7–S10. doi:10.5664/jcsm.26929
Ruderfer, D. M., Ripke, S., Mcquillin, A., Boocock, J., Stahl, E. A., Pavlides, J. M. W., et al. (2018). Genomic Dissection of Bipolar Disorder and Schizophrenia, Including 28 Subphenotypes. Cell 173, 1705–e16.e1716. doi:10.1016/j.cell.2018.05.046
Savage, J. E., Jansen, P. R., Stringer, S., Watanabe, K., Bryois, J., De Leeuw, C. A., et al. (2018). Genome-wide Association Meta-Analysis in 269,867 Individuals Identifies New Genetic and Functional Links to Intelligence. Nat. Genet. 50, 912–919. doi:10.1038/s41588-018-0152-6
Sekula, P., Del Greco M, F., Pattaro, C., and Köttgen, A. (2016). Mendelian Randomization as an Approach to Assess Causality Using Observational Data. Jasn 27, 3253–3265. doi:10.1681/asn.2016010098
Sklar, P., Ripke, S., Scott, L. J., Andreassen, O. A., Cichon, S., Craddock, N., et al. (2011). Large-scale Genome-wide Association Analysis of Bipolar Disorder Identifies a New Susceptibility Locus Near ODZ4. Nat. Genet. 43, 977–983. doi:10.1038/ng.943
Sleiman, P. M., and Grant, S. F. (2010). Mendelian Randomization in the Era of Genomewide Association Studies. Clin. Chem. 56, 723–728. doi:10.1373/clinchem.2009.141564
Smith, E. N., Bloss, C. S., Badner, J. A., Barrett, T., Belmonte, P. L., Berrettini, W., et al. (2009). Genome-wide Association Study of Bipolar Disorder in European American and African American Individuals. Mol. Psychiatry 14, 755–763. doi:10.1038/mp.2009.43
Stummer, L., Markovic, M., and Maroney, M. (2018). Pharmacologic Treatment Options for Insomnia in Patients with Schizophrenia. Medicines 5, 88. doi:10.3390/medicines5030088
Tian, D., Wang, P., Tang, B., Teng, X., Li, C., Liu, X., et al. (2020). GWAS Atlas: a Curated Resource of Genome-wide Variant-Trait Associations in Plants and Animals. Nucleic Acids Res. 48, D927–d932. doi:10.1093/nar/gkz828
Tylee, D. S., Sun, J., Hess, J. L., Tahir, M. A., Sharma, E., Malik, R., et al. (2018). Genetic Correlations Among Psychiatric and Immune-Related Phenotypes Based on Genome-wide Association Data. Am. J. Med. Genet. 177, 641–657. doi:10.1002/ajmg.b.32652
Verbanck, M., Chen, C.-Y., Neale, B., and Do, R. (2018). Detection of Widespread Horizontal Pleiotropy in Causal Relationships Inferred from Mendelian Randomization between Complex Traits and Diseases. Nat. Genet. 50, 693–698. doi:10.1038/s41588-018-0099-7
Willer, C. J., Li, Y., and Abecasis, G. R. (2010). METAL: Fast and Efficient Meta-Analysis of Genomewide Association Scans. Bioinformatics 26, 2190–2191. doi:10.1093/bioinformatics/btq340
Winkelman, J. W. (2015). Insomnia Disorder. N. Engl. J. Med. 373, 1437–1444. doi:10.1056/NEJMcp1412740
Zeng, P., Wang, T., Zheng, J., and Zhou, X. (2019). Causal Association of Type 2 Diabetes with Amyotrophic Lateral Sclerosis: New Evidence from Mendelian Randomization Using GWAS Summary Statistics. BMC Med. 17, 225. doi:10.1186/s12916-019-1448-9
Keywords: insomnia, bipolar disorder, depression, schizophrenia, two-sample mendelian randomization, genome-wide association study
Citation: Huang P, Zou Y, Zhang X, Ye X, Wang Y, Yu R and Yang S (2021) The Causal Effects of Insomnia on Bipolar Disorder, Depression, and Schizophrenia: A Two-Sample Mendelian Randomization Study. Front. Genet. 12:763259. doi: 10.3389/fgene.2021.763259
Received: 23 August 2021; Accepted: 13 September 2021;
Published: 11 October 2021.
Edited by:
Tao Wang, Northwestern Polytechnical University, ChinaReviewed by:
Ping Zeng, Xuzhou Medical University, ChinaWenlong Ren, Nantong University, China
Yue Fan, Xi’an Jiaotong University, China
Copyright © 2021 Huang, Zou, Zhang, Ye, Wang, Yu and Yang. This is an open-access article distributed under the terms of the Creative Commons Attribution License (CC BY). The use, distribution or reproduction in other forums is permitted, provided the original author(s) and the copyright owner(s) are credited and that the original publication in this journal is cited, in accordance with accepted academic practice. No use, distribution or reproduction is permitted which does not comply with these terms.
*Correspondence: Sheng Yang, yangsheng@njmu.edu.cn
†These authors have contributed equally to this work and share first authorship