- 1The Second Affiliated Hospital of Harbin Medical University, Harbin, China
- 2The First Hospital of Qiqihar, Qiqihar, China
Diabetic mellitus erectile dysfunction (DMED) is one of the most common complications of diabetes mellitus (DM), which seriously affects the self-esteem and quality of life of diabetics. MicroRNAs (miRNAs) are endogenous non-coding RNAs whose expression levels can affect multiple cellular processes. Many pieces of studies have demonstrated that miRNA plays a role in the occurrence and development of DMED. However, the exact mechanism of this process is unclear. Hence, we apply miRNA sequencing from blood samples of 10 DMED patients and 10 DM controls to study the mechanisms of miRNA interactions in DMED patients. Firstly, we found four characteristic miRNAs as signature by the SVM-RFE method (hsa-let-7E-5p, hsa-miR-30 days-5p, hsa-miR-199b-5p, and hsa-miR-342–3p), called DMEDSig-4. Subsequently, we correlated DMEDSig-4 with clinical factors and further verified the ability of these miRNAs to classify samples. Finally, we functionally verified the relationship between DMEDSig-4 and DMED by pathway enrichment analysis of miRNA and its target genes. In brief, our study found four key miRNAs, which may be the key influencing factors of DMED. Meanwhile, the DMEDSig-4 could help in the development of new therapies for DMED.
Introduction
Erectile dysfunction (ED) refers to the persistent or repeated failure of men to achieve and/or maintain penile erection for satisfactory sexual activity. As a common and the most neglected complication of diabetes (Zhao et al., 2020; Long et al., 2021; Yang et al., 2021), diabetic mellitus erectile dysfunction (DMED) is an important factor affecting psychological well-being, spousal relationship and family life (Malavige and Levy, 2009). The massive research indicated patient of T2MD incidence ED was significantly higher than that of the health. 75%male with diabetes is affected with ED. 66.3% is T2MD among of the data (Kouidrat et al., 2017; Cheng et al., 2018; Zagidullin et al., 2019; Zhu et al., 2021a). DMED is considered as an alternative marker for diabetes and cardiovascular disease, and is the primary feature of diabetes. Meanwhile, DMED has a multifactorial pathological process that can occur simultaneously with cardiovascular disease, neuropathy, and depression. How to effectively intervene in DMED has become an urgent problem in the global medical community.
MicroRNAs (miRNAs) are small non-coding RNAs of 19–25 nucleotides (Cheng et al., 2016; Lu and Rothenberg, 2018; Wu et al., 2019; Mo et al., 2020). They have been used as important regulators of gene expression in recent decades. Changes in their expression levels can affect multiple cellular processes and are used as molecular markers for diagnosis and follow-up (Han et al., 2021). It is widely involved in pathological processes such as cancer (Rupaimoole and Slack, 2017; Liu et al., 2021a; Lei and Shu-Lin, 2021; Sheng et al., 2021; Tang et al., 2021), DM (Vasu et al., 2019), cardiovascular events (Barwari et al., 2016), and ED (Ding et al., 2017). However, there are few related studies in DMED.
Growing evidences have indicated that miRNAs play an important role in the occurrence and development of DM and diabetic complications (Kong et al., 2011; Jiang et al., 2015). Unfortunately, the exact pathogenesis of miRNAs action on DMED remains to be largely unknown. Hence, we adopted the machine learning method (SVM-RFE), identified the characteristic miRNAs of DMED, constructed the signature of DMED and found potentially related pathways. Our work has significance for the identification of the molecular mechanism and the early prediction and diagnosis of DMED.
Materials and Methods
Subjects
Inclusion criteria: T2DM patients admitted to Qiqihar Medical College from December 2020 to June 2021 were selected as the study subjects. Erectile dysfunction was identified by international index of erectile function -5 (IIEF-5). Diagnostic criteria for T2DM: in line with WHO diabetes diagnostic criteria in 1999; Diagnostic and grading criteria for ED: 1) Regular sexual partner and normal sexual life; 2) History of ED for more than 6 months; 3) Erectile dysfunction was assessed according to IIEF-5, and the total score ≤21 was divided into ED; A score of five to seven was severe, 8–11 was moderate, 12–21 was mild, and ≥22 was no ED. Healthy married men with erectile dysfunction aged 30–70 years with a course of 2–10 years were included in the study.
Exclusion criteria: 1) The informed consent is not signed or the medical records are incomplete; 2) Incomplete research data; 3) Type 1 diabetes mellitus (T1DM), adult latent autoimmune diabetes mellitus (LADA), acute complications of diabetes mellitus; 4) Hypogonadism, thyroid disease, adrenal disease, pituitary disease, etc.; 5) Pelvic and urinary genital malformations, inflammation, tumor, trauma, surgical history; 6) Serious blood system, cardiovascular, liver, kidney disease or other disease affecting sexual activity; 7) Spinal cord injury; 8) Smoking, alcohol, drug abuse and masturbation history; 9) A history of drug abuse; (10) Receiving ED treatment or drugs that affect ED; Such as immunosuppressants, glucocorticoids, diuretics, receptor blockers, antioxidants, etc.; 11) History of mental illness, and the ED caused by anxiety, depression and other psychological factors was excluded according to the SAS standard score <50 and SDS standard score <53.
According to the above criteria, a total of 20 male T2DM patients were included and divided into DMED group (10 cases) and DM group (10 cases). The study was conducted in accordance with the Declaration of Helsinki,and with approval from The first hospital of Qiqihar ethics committee for clinical trials (2020-KY-007–01).Written informed consent was obtained from all the participants.
Clinical Data of Patients
General information such as name, age, sex, height, weight, marital history, personal history, infection history, surgery and trauma history were collected. IIEF-5 scores, SAS scale and SDS scale were obtained using a questionnaire. Body mass index (BMI) was calculated by formula: BMI = Body weight/height2 (kg/m2). The remaining indicators were determined by clinical tests.
Collection of Serum Samples
Clinical serum samples were collected by utilizing residual specimens from patients undergoing routine medical care. Each blood sample is 4–6 ml. Samples were then centrifuged and the supernatant was stored at −80°C in the centrifuge tube.
Sample Sequencing
MiRNA sequencing was performed on a total of 20 cases, 10 cases in each group with no statistical difference in age, course of disease and BMI.
The Method of Sample Detecting
Use Agilent 2100 Bioanalyzer to test sample integrity and concentration, and NanoDrop to Inorganic ions orpolycarbonate contamination. This step aimed to provide a reference for library construction and later analysis.
Library Construction
Filter Small RNA: Use the 200ng-1 μg of RNA sample, then separate RNA segment of different size by PAGE gel, select 18–30 nt (14–30 ssRNA Ladder Marker, TAKARA) stripe and recycle; Adaptor ligation: Prepare connection 3′adaptor system (Reaction condition:70°C for 2min; 25°C for 2 h); Secondly add RT-Primer, (Reaction condition: 65°C for 15 min; ramp to 4°C at a rate of 0.3°C/s); Thirdly add 5′adaptor mix system (Reaction condition: 70°C for 2 min; 25°C for 1 h).
RT PCR: Prepare First Strand Master Mix and Super Script II (Invitrogen) reverse transcription (Reaction condition: 42°C for 1 h; 70°C for 15 min); Several rounds of PCR amplification with PCR Primer Cocktail and PCR Master Mix were performed to enrich the cDNA fragments (Reaction condition: 95°C for 3 min; 15–18 cycles of (98°C for 20 s, 56°C for 15 s, 72°C for 15 s); 72°C for 10 min; 4°C hold); Purify PCR products: Then the PCR products were purified with PAGE gel, dissolve the recycled products in EB solution.
Circularization
The double stranded PCR products were heat denatured and circularized by the splint oligo sequence. The single strand circle DNA (ssCir DNA) were formatted as the final library.
Library Quality Control
Library was validating on the Agilent Technologies 2100 bioanalyzer.
Sequencing
The library was amplified with phi29 to make DNA nanoball (DNB) which have more than 300 copies of one molecular. The DNBs were loaded into the patterned nanoarray and single end 50 bases reads were generated in the way of combinatorial Probe-Anchor Synthesis (cPAS).
Feature Selection of Diabetic Mellitus Erectile Dysfunction Based on Support Vector Machine-Recursive Feature Elimination
Support Vector Machine-Recursive Feature Elimination (SVM-RFE) is a sequence backward selection algorithm based on the maximum interval principle of Support Vector Machine (SVM) (Guyon et al., 2002; Tang et al., 2018; Cheng et al., 2019; Yang et al., 2020; Liu et al., 2021b; Joshi et al., 2021).
The counts data based on miRNA sequencing were combined with the improved SVM-REF method proposed by Kai-Bo Duan et al. (Duan et al., 2005) to select miRNAs. Due to the randomness in Kai-Bo Duan’s method, in order to obtain a model with relatively small error, the whole process was repeated for 1,000 times. The model with the smallest error was selected as the final model. If there were multiple models with the same minimum error, the model with a large number of miRNAs was selected. The characteristic miRNAs, called DMEDSig-4, were finally screened.
Differentially Expressed
According to the identified DMEDSig-4, we combined the miRNA read counts data calculated by the sequencing process. In view of the negative binomial distribution of counts data, we used the R package DESeq2 to calculate the differences between the DM groups and DEMD groups (Love et al., 2014).
Identification of miRNA Target Genes
The miRNA-targeted mRNAs of DMEDSig-4 were pooled using the online bioinformatics analysis tool (EncoRI). Firstly, we searched EncoRI database (http://starbase.sysu.edu.cn/) (Li et al., 2014), which included seven databases (microT, miRanda, miRmap, PITA, RNA22, PicTar and TargetScan). Then, we entered characteristic miRNA of DMEDSig-4, set CLIP-Data≥5, Program-Number≥5, and Degradome-Data≥1 to obtain miRNA target genes.
Analysis of Gene Function Enrichment Regulatory Network
The R package FGNet allows functional enrichment analysis (FEA) to be performed on a list of genes or expression sets and the results to be converted into a network (Aibar et al., 2015; Azimi et al., 2021). The network can provide an overview of the biological function of genes/terms, and allows easy seeing of links between genes, the overlap between clusters, etc. We selected the annotation tool topGO for functional annotation of target genes. GO was used to describe gene functions along with three aspects: biological process (BP), cellular component (CC) and molecular function (MF). The p < 0.01 was considered significant.
Results
Statistical Analysis of Clinical Data
Firstly, we collected 20 high-quality samples (10 DMED and 10 DM) from 60 patients according to the inclusion criteria. Then, we collected and collated the clinical information of these 20 samples. Subsequently, statistical analysis was performed for the DMED group and the DM group, including age, diabetes duration, BMI, fasting plasma glucose, glycated hemoglobin, total cholesterol, triglyceride, testosterone, thyroid stimulating hormone, serum creatinine, carbamide, alanine aminotransferase, aspartate aminotransferase. Meanwhile, we also conducted a questionnaire survey on these 20 patients, and obtained IIEF-5 score, self-rating anxiety scale (SAS) score and self-rating depression scale (SDS) score. In this project, a total of 20 samples were tested using DNBSEQ platform. The average ratio of sample to genome was 78.22%. A total of 1,044 small RNAs were detected.
SAS9.4 international standard statistical programming software was used for statistical analysis. Measurement data processing normal distribution and variance homogeneity were measured, The measurement data conforming to normal distribution are expressed by mean ± standard deviation,comparison between two groups with sample t tests,non normal distribution adopt median and IQR to express, makes the non-parametric test. p < 0.05 could be considered statistically significant (Table 1). Finally, according to the results, there were no statistically significant differences with regard to age, diabetes duration and BMI (p > 0.05). In addition, there was no significant difference in other statistical indicators (p > 0.05) except IIEF-5 (p < 0.05). This showed that we chose samples to avoid the influence of other factors as much as possible.
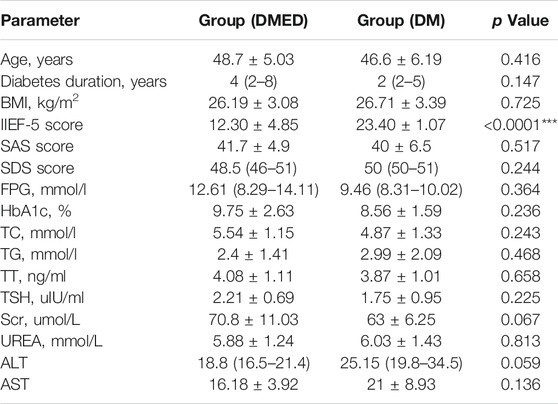
TABLE 1. Comparisons of clinical data. BMI, body mass index; IIEF-5, international index of erectile function 5; SAS, self-rating anxiety scale; SDS, self-rating depression scale; FPG, fasting plasma glucose; HbA1c, glycated hemoglobin; TC, total cholesterol; TG, triglyceride; TT, testosterone; TSH, thyroid stimulating hormone; Scr, serum creatinine; Urea, carbamide; ALT, alanine aminotransferase; AST, aspartate aminotransferase.
Construction of microRNAs Signature Associated With Diabetic Mellitus Erectile Dysfunction
Many pieces of evidence have shown that miRNAs have diagnostic and predictive value for DMED in mice (Li et al., 2017; Cong et al., 2020; Huo et al., 2020). However, few correlational researches of DMED have been conducted on the human body. In order to further explore the mechanism of miRNAs regulating DMED in the human body, we selected 10 DM groups and 10 DMED groups for miRNA sequencing based on the inclusion criteria. The miRNA counts data of 20 samples were obtained. Firstly, miRNA counts data were filtered to delete miRNAs with counts of 0 in most samples. The selected miRNAs should have counts of non-0 in at least 18 samples. Due to the limited sample size of miRNA expression profiles in DM groups and DMED groups, we adopted the machine learning method of SVM-RFE to screen characteristic miRNAs (Sanz et al., 2018).
Firstly, the miRNA counts data contained all miRNAs that will be imported. Secondly, the algorithm used SVM model training samples to calculate the weight of each miRNA. Subsequently, we ranked miRNAs according to their weights and deleted the bottom-ranked miRNAs from the subset (Lin et al., 2017). Meanwhile, the remaining miRNAs were used to train the model again for the next iteration. Finally, the required number of miRNAs were selected. The later the miRNA was removed from the subset, the more significant the miRNAs were. We employed SVM-RFE machine learning method. Firstly, SVM-RFE method can be used for linear/nonlinear classification as well as regression with low generalization error rate. That is to say, he has a good learning ability, and the results of learning have a good extension. Secondly, SVM-RFE method can solve the problem of machine learning in the case of small samples, solve the problem of high dimension, and avoid the problem of neural network structure selection and local minimum point. Finally, SVM-RFE method is the best off-the-shelf classifier and can get a low error rate. Meanwhile, SVM-RFE method can make good classification decisions for data points outside the training set.
Since SVM-REF was more sensitive to feature changes, the ranking of features was different each time. For the robustness of feature selection, we refer to the method of Kai-Bo Duan (Duan et al., 2005). We used ten-fold cross-validation here by adding resampling to each iteration to stabilize the ranking (Zhu et al., 2021b). After 1,000 cycles of the algorithm (Supplementary Table S1), four characteristic miRNAs (hsa-let-7E-5p, hsa-miR-30 days-5p, hsa-miR-199b-5p, and hsa-miR-342–3p) were obtained according to the lowest error rate of 0.25 in ten-fold cross-validation (Figure 1). We found that the error rate decreased significantly from the first to the fourth feature number, and then increased significantly from the fifth. Obviously, the feature number of four had the best differentiation between DMED groups and DM groups. These four miRNAs (hsa-let-7E-5p, hsa-miR-30 days-5p, hsa-miR-199b-5p, and hsa-miR-342–3p) obtained by machine learning methods had the best classification performance in the DMED and DM groups. Therefore, we referred to this predictive signature as DMEDSig-4. In the following results, the performance of DMEDSig-4 was verified and analyzed.
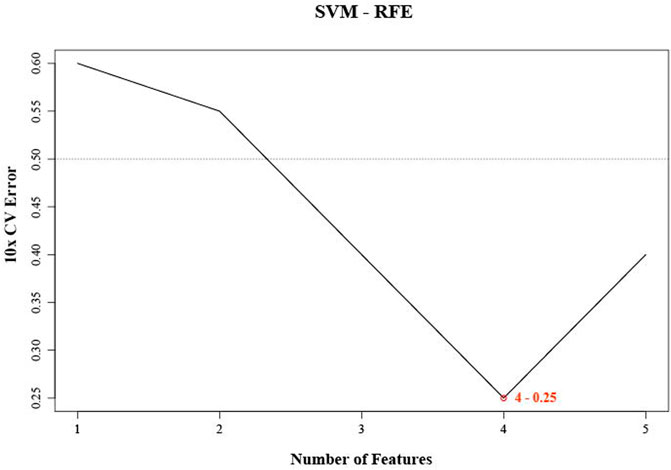
FIGURE 1. SVM-RFE were used for feature selection. The point highlighted indicates the lowest error rate, and the corresponding miRNA at this point are the best signature selected by SVM-RFE.
Classification Capability Verification and Clinical Association Analysis of Diabetic Mellitus Erectile DysfunctionSig-4
In order to investigate the expression patterns of four characteristic miRNAs predicted by DMEDSig-4, we first conducted hierarchical clustering according to counts data of these four miRNAs and plotted the clustering heat map of DMEDSig-4 in 20 samples (Figure 2A). The results of hierarchical clustering analysis showed that the 20 samples were clustered into three clusters by DMEDSig-4. The two clusters on the right were completely composed of samples from the DM or DMED groups. Although the cluster on the left was a mixture of the two types of samples, except for DMED_3, DM and DMED were significantly clustered into the same cluster. This phenomenon indicated that all samples were characterized by the DMEDSig-4 expression pattern. In addition, we also calculated the similarity between samples according to Euclidean distance (Figure 2B). The results showed that the Euclidean distance between some samples was very small, indicating these samples had a high similarity. For example, samples DM_8, DM_5, DM_9, DM_2, DM_6 and DM_7. Samples DMED_10, DMED_7, DMED_8, DMED_1 and DMED_9.
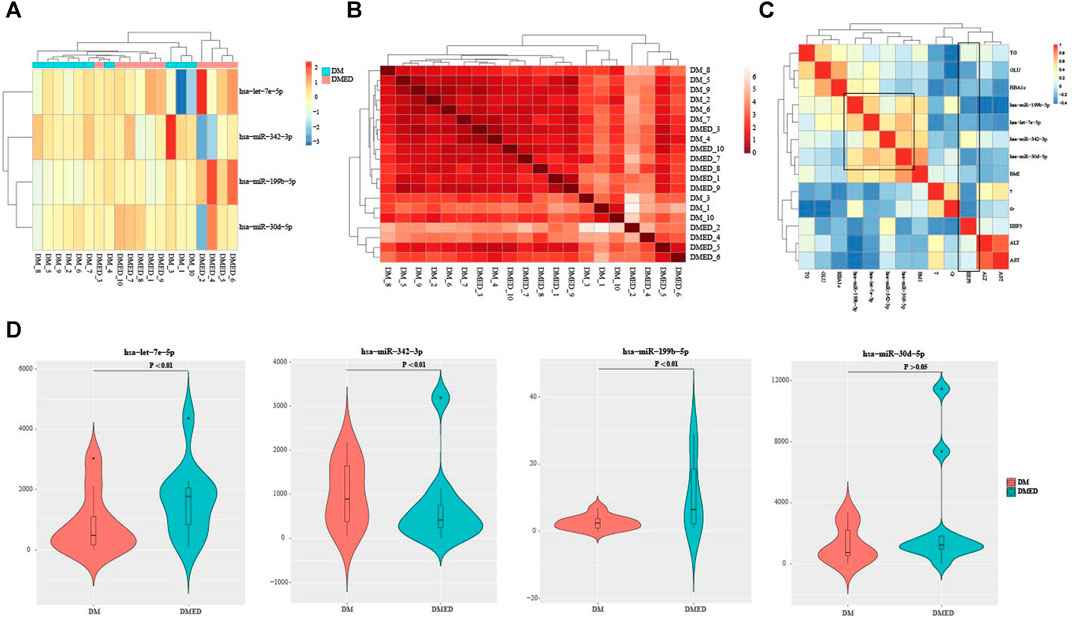
FIGURE 2. The verification of DMEDSig-4 classification ability and the association of clinical information. (A) Clustering heat map of DMEDSig-4 in DM and DMED groups. Red: Up-regulated expression; Blue: Down-regulated expression. (B) Heat map of similarity between DM groups and DMED groups. (C) Correlation analysis of DMEDSig-4 and clinical factors. BMI, body mass index, IIEF5, international index of erectile function 5; HbA1c, glycated hemoglobin; TT, testosterone; GLU, glucose; Cr, creatinine; TG, triglyceride; ALT, Alanine transaminase; AST, aspartic acid transaminase. (D) Violin diagram of differential expression of DMEDSig-4 in DM and DMED samples.
In order to further explore the correlation between DMEDSig-4 and clinical factors, we calculated the Spearman correlation between miRNAs and clinical indicators of all samples (Figure 2C, Supplementary Table S2). It could be observed that the four miRNAs in DMEDSig-4 were positively correlated with each other, indicating that there might be a mechanism of co-operative regulation of DMEDSig-4. In general, the diagnosis of ED depends on the history of disease and the IIEF-5 (Rosen et al., 1997; Hatzichristou et al., 2002). It could be observed that IIEF-5 was negatively correlated with DMEDSig-4. Meanwhile, IIEF-5 was negatively correlated with testosterone, glycated globin, creatinine, fasting blood glucose and other indicators. Previous studies had verified that testosterone was a protective factor in DMED (Diaz-Arjonilla et al., 2009), and the negative correlation between testosterone and indicators confirmed the correctness of IIEF-5 data.
In order to verify the classification ability of the four characteristic miRNAs in DMEDSig-4 for samples, we calculated the significant difference of miRNA expression between the DM groups and the DMED groups. The results showed that the differences of hsa-let-7e-5p, hsa-miR-199b-5p and hsa-miR-342–3p were significant (p < 0.01), while the effect of hsa-miR-30 days-5p was not significant (p > 0.05) (Figure 2D).
These results indicated that the miRNAs we identified have certain characteristics between the DM and DMED groups, regardless of the individual miRNA level or the overall expression pattern, and there were also certain correlations with clinical indicators. These showed that DMEDSig-4 had a certain role in helping to identify DMED.
Identification of Target microRNAs of Diabetic Mellitus Erectile DysfunctionSig-4
Many pieces of evidence demonstrated that miRNAs were capable of regulating various biological and pathological processes via inhibiting target mRNA translation or promoting mRNA degradation (Fabian et al., 2010; Fang et al., 2019; Riaz and Li, 2019; Wang et al., 2020). Meanwhile, the miRNAs could act as signatures of disease, strong indicators of prognosis or potential therapeutic targets (Zhu et al., 2011; Huang, 2018; Norsworthy et al., 2020). In order to further study the effects of the DMEDSig-4 target mRNAs on ED, we used the online bioinformatics database ENCORI (http://starbase.sysu.edu.cn/) to identify the target mRNAs. According to the parameters set, the target genes corresponding to each miRNA were obtained. The results of predicting targeted mRNAs on four miRNAs showed that there were 40 targeted mRNAs for hsa-let-7e-5p, 42 targeted mRNAs for hsa-miR-30 days-5p, nine targeted mRNAs for hsa-miR-199b-5p, and 15 targeted mRNAs hsa-miR-342–3p (Figure 3A).
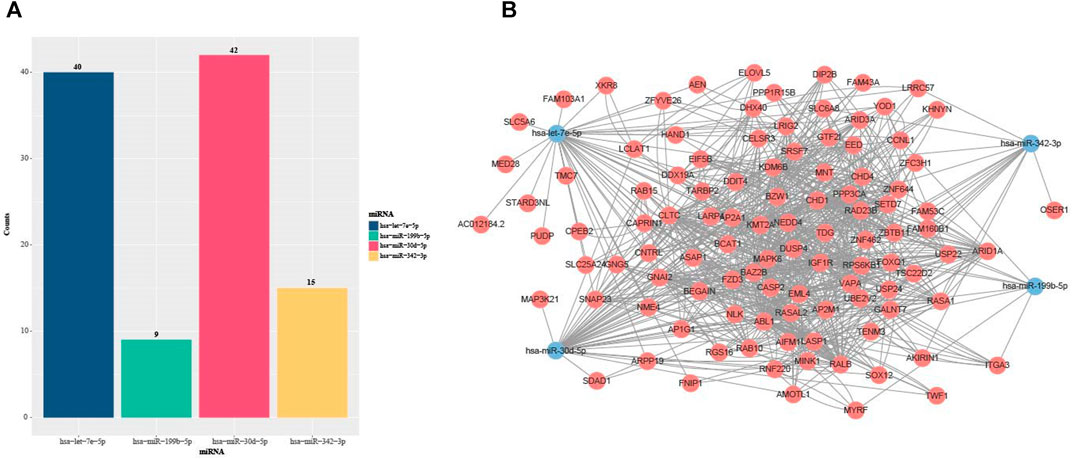
FIGURE 3. Statistics of miRNA target miRNAs in DMEDSig-4. (A) Bar chart of DEMDSig-4 target genes (B) miRNA-mRNA interaction network diagram of DEMDSig-4. Red: target mRNAs; Blue: miRNAs of DMEDSig-4.
In order to observe the relationship between miRNAs and target mRNAs, we constructed the miRNA-mRNA interaction network diagram based on DMEDSig-4 and target mRNAs through STRING database (https://string-db.org/) (Figure 3B). The network consisted of four miRNA nodes, 105 mRNA nodes and 870 edges. Among them, 764 edges were the relationship between target mRNAs, and 106 edges were the relationship between miRNA and target mRNAs. There was only one intersection among miRNA target mRNAs, indicating that these miRNAs did not tend to jointly regulate a target mRNA, and the identified target mRNAs had a close interaction relationship, suggesting that these mRNAs might act together on the same pathway.
Functional Annotation of microRNAs and Its Target microRNAs
In order to investigate the function of DEMDSig-4 targeted mRNAs, we used the topGO (Yang et al., 2021) annotation tool in R package FGNet for functional annotation of DEMDSig-4. We aimed to the functional analysis of each target mRNA searching further to verify the characteristic function of DEMDSig-4. The resulting network represented the links and associations between clusters of mRNAs and enriched terms. We annotated the biological process (BP), cellular component (CC) and molecular function (MF) of the target mRNAs. A total of 255 clusters and descriptions, we provided in the form of supplementary files (Supplementary Table S3). Here, we focused on the biological process of the target mRNAs. Due to the large number of biological processes and the complexity of the network, we manually selected representative biological processes for demonstration, including most of the mRNAs targeted for DMEDSig-4 (Figure 4A). The biological process includes: Regulation of nitrogen metabolic Process non-canonical Wnt signaling Pathway Neuron Projection Development “Wnt Signaling Pathway, Planar Cell Polarity Pathway” Regulation of Neuron projection Development, And “Density Lipoprotein receptor particle metabolic Process”. We found that the subnetwork was divided into two broad functional categories, including metabolic function and neural function, which were closely related to the pathological mechanism of ED (Müller and Mulhall, 2006; Shamloul and Ghanem, 2013).
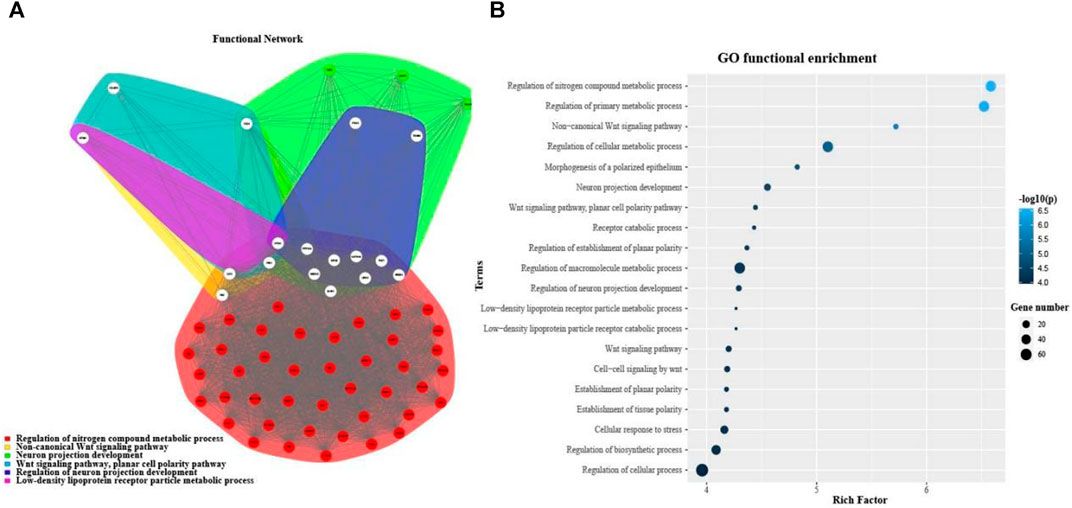
FIGURE 4. Functional annotation of DMEDSig-4. (A) Functional Gene Network (FGN) of clusters defined by topGO for DMEDSig-4 Targeted Genes. Edges: link genes annotated under the same enrichment terms. Node colors: functional clusters containing genes. White nodes: hub genes expressed in multiple clusters. (B) Bubble chart of function enrichment results of DMEDSig-4.
Although FGNet provided a broad overview of the biological effects of human-specific genetic alterations by clustering functional terms within clusters and establishing relationships between such clusters, it lacked the detail that could be obtained by analyzing each functional category individually (Bitar et al., 2019; Zhang et al., 2021). Therefore, we turned to gene ontology (GO) analysis. The top 20 salient biological processes were functionally annotated in terms of p values (Figure 4B). The results demonstrated most of the signaling pathways were associated with DMED at the molecular and cellular levels, which could provide important information for revealing the most significant biological functions of DMED. We found that the biological processes of DMEDSig-4 were mainly divided into cell metabolism, neural signal transmission and planar cell polarity pathway. For example, we queried that the biological process “regulation of nitrogen Compound metabolic process” was associated with endothelial dysfunction (Andersson, 2003; Yuyun et al., 2018; Cyr et al., 2020). Endothelial dysfunction was recognized as a mainstay in the pathophysiology of the disease (Castela and Costa, 2016). As for the “WNT signaling pathway”, studies had demonstrated that the Wnt family contributed to the pathogenesis of diabetes-induced erectile dysfunction (Shin et al., 2014; Ghatak et al., 2017). ED was also involved in the regulation of the metabolic and nervous systems (Burnett, 2005; Ryan and Gajraj, 2012; Mitidieri et al., 2020). For example, GO terms included “regulation of primary metabolic process” “regulation of cellular metabolic process” and “cellular response to stress”. Literature verification showed that the above pathways were related to the pathological mechanism of DMED (Matfin et al., 2005; Zsoldos et al., 2019).
Finally, we annotated the characteristic miRNAs in DMEDSig-4 to prove the mechanism relationship between DMEDSig-4 and DMED. The expression of hsa-miR-342–3p helped to identify patients with cardiovascular disease (Seleem et al., 2019; Ray et al., 2020). Meanwhile, the expression level of this miRNA was significantly increased in diabetic nephropathy (Eissa et al., 2016; Jiang et al., 2020). Importantly, hsa-miR-342–3p was differentially expressed in obese children with and without endothelial dysfunction (Khalyfa et al., 2016), which was one of the important factors causing DMED. Meanwhile, hsa-miR-199b-5p, hsa-miR-30 days-5p and hsa-let-7e-5p were all related to diabetic kidney damage and cardiovascular diseases (Jia et al., 2016; Fedorko et al., 2017; Sun et al., 2018), which were all risk factors for ED.
Discussion
Erectile dysfunction (ED) is a common and often overlooked complication of diabetes that can wreak havoc on men both physically and mentally. Studies have shown that type 2 diabetes mellitus (T2DM) is widely associated with ED and is a risk factor for ED. Interestingly, several studies have demonstrated that miRNAs are involved in the pathogenesis of ED. For example, Rama Natarajan et al. explored the role of miRNAs in the pathology of diabetic complications and also discussed the potential use of miRNAs as novel diagnostic and therapeutic targets for diabetic complications (Natarajan et al., 2012; Wang et al., 2019). Wang et al. found that upregulation of miR-320 was associated with impaired angiogenesis in diabetes (Wang et al., 2009). Yan Wen et al. found that miR-205 may contribute to the pathogenesis of DMED via down-regulation of androgen receptor expressions (Wen et al., 2019). Although a large number of studies have proved the regulatory relationship of miRNAs on ED, there is still a lack of research on the relationship between miRNAs and ED in the context of T2DM. This study aimed to further explore ED signature associated with diabetes by analyzing miRNA expression data in patients with DM. This signature may play a certain role in the diagnosis and treatment of DMED. This study is not only a preliminary attempt on miRNA signature of DMED, but also may serve as the basis for subsequent studies.
In terms of data, we collected a large amount of clinical information and conducted preliminary screening to select patients (disease history/clinical information) as similar as possible and to eliminate the interference of other factors to the greatest extent possible. Finally, we selected 10 DM patients and 10 DMED patients as the final study subjects. However, due to the limited time and cost, our patient cohort is still relatively small, and there is no additional data set verification, so the identified DMEDSig-4 may not have good universality, which will be our further research direction. In this study, we used a machine learning approach (SVM-RFE) to identify potential miRNA features in a sample of DM and DMED patients. First, four optimal feature miRNAs (hsa-miR-342–3p, hsa-miR-199b-5p, hsa-miR-30 days-5p and hsa-let-7e-5p) were identified after 1,000 cycles of the algorithm, called DMEDSig-4. They all had a good classification effect, and there might be a potential mechanism of co-regulation. Subsequently, after associating with clinical factors, we found that DMEDSig-4 was positively correlated with each other and negatively correlated with IIEF-5. Then, we searched for the miRNAs targeted by DMDESig-4 and constructed a miRNA-mRNA interaction network. The results showed that the network consisted of four miRNA nodes, 105 mRNA nodes and 870 edges. Meanwhile, there was only one intersection between the targeted miRNAs of miRNA, indicating that these miRNAs did not tend to jointly regulate a target mRNA. Importantly, the identified target mRNAs had a close interaction relationship, suggesting that these mRNAs might act together on the same pathway. This might play an enlightening role in the subsequent studies of miRNA on DMED. Finally, we searched for the miRNAs targeted by DMDESig-4 and performed functional enrichment. The results showed that the DMDESig-4 pathways were closely related to DM and ED, which might contribute to the pathogenesis of ED. The literature review has shown that DMEDSig-4 was associated with cardiovascular disease, diabetic nephropathy and liver injury, which were all potential risk factors for ED (Hu et al., 2021). Clinical ED patients are often accompanied by cardiovascular disease, kidney and liver damage and other symptoms.
We hope that the characterization of miRNAs will contribute to a comprehensive understanding of their pathways in DMED and improve therapeutic strategies for patients with DMED. We hope that the identification of DMEDSig-4 will contribute to a comprehensive understanding of its pathway mechanism in DMED and improve therapeutic strategies for patients with DMED.
Data Availability Statement
The datasets presented in this study can be found in online repositories. The names of the repository/repositories and accession number(s) can be found below: https://www.ncbi.nlm.nih.gov/geo/query/acc.cgi?acc=GSE182053.
Ethics Statement
The studies involving human participants were reviewed and approved by Qiqihar First Hospital. The patients/participants provided their written informed consent to participate in this study.
Author Contributions
HQ designed the experiment, HX wrote the manuscript and conducted the experiment, HX, BZ, WZ, and PT contributed the data.
Conflict of Interest
The authors declare that the research was conducted in the absence of any commercial or financial relationships that could be construed as a potential conflict of interest.
Publisher’s Note
All claims expressed in this article are solely those of the authors and do not necessarily represent those of their affiliated organizations, or those of the publisher, the editors, and the reviewers. Any product that may be evaluated in this article, or claim that may be made by its manufacturer, is not guaranteed or endorsed by the publisher.
Supplementary Material
The Supplementary Material for this article can be found online at: https://www.frontiersin.org/articles/10.3389/fgene.2021.762136/full#supplementary-material
References
Aibar, S., Fontanillo, C., Droste, C., and De Las Rivas, J. (2015). Functional Gene Networks: R/Bioc Package to Generate and Analyse Gene Networks Derived from Functional Enrichment and Clustering. Bioinformatics 31 (10), 1686–1688. doi:10.1093/bioinformatics/btu864
Andersson, K. E. (2003). Erectile Physiological and Pathophysiological Pathways Involved in Erectile Dysfunction. J. Urol. 170 (2 Pt 2), S6–S13. doi:10.1097/01.ju.0000075362.08363.a4
Azimi, M., Totonchi, M., Rahimi, M., Firouzi, J., Sahranavard, P., Emami Razavi, A., et al. (2021). An Integrated Analysis to Predict micro‐RNAs Targeting Both Stemness and Metastasis in Human Gastric Cancer. J. Gastroenterol. Hepatol. 36 (2), 436–445. doi:10.1111/jgh.15176
Barwari, T., Joshi, A., and Mayr, M. (2016). MicroRNAs in Cardiovascular Disease. J. Am. Coll. Cardiol. 68 (23), 2577–2584. doi:10.1016/j.jacc.2016.09.945
Bitar, M., Kuiper, S., O'Brien, E. A., and Barry, G. (2019). Genes with Human-specific Features Are Primarily Involved with Brain, Immune and Metabolic Evolution. BMC Bioinformatics 20 (Suppl. 9), 406. doi:10.1186/s12859-019-2886-2
Burnett, A. L. (2005). Metabolic Syndrome, Endothelial Dysfunction, and Erectile Dysfunction: Association and Management. Curr. Urol. Rep. 6 (6), 470–475. doi:10.1007/s11934-005-0043-0
Castela, Â., and Costa, C. (2016). Molecular Mechanisms Associated with Diabetic Endothelial-Erectile Dysfunction. Nat. Rev. Urol. 13 (5), 266–274. doi:10.1038/nrurol.2016.23
Cheng, L., Shi, H., Wang, Z., Hu, Y., Yang, H., Zhou, C., et al. (2016). IntNetLncSim: an Integrative Network Analysis Method to Infer Human lncRNA Functional Similarity. Oncotarget 7 (30), 47864–47874. doi:10.18632/oncotarget.10012
Cheng, L., Yang, H., Zhao, H., Pei, X., Shi, H., Sun, J., et al. (2019). MetSigDis: a Manually Curated Resource for the Metabolic Signatures of Diseases. Brief Bioinform. 20 (1), 203–209. doi:10.1093/bib/bbx103
Cheng, L., Zhuang, H., Yang, S., Jiang, H., Wang, S., and Zhang, J. (2018). Exposing the Causal Effect of C-Reactive Protein on the Risk of Type 2 Diabetes Mellitus: A Mendelian Randomization Study. Front. Genet. 9, 657. doi:10.3389/fgene.2018.00657
Cong, R., Wang, Y., Wang, Y., Zhang, Q., Zhou, X., Ji, C., et al. (2020). Comprehensive Analysis of lncRNA Expression Pattern and lncRNA-miRNA-mRNA Network in a Rat Model with Cavernous Nerve Injury Erectile Dysfunction. J. Sex. Med. 17 (9), 1603–1617. doi:10.1016/j.jsxm.2020.05.008
Cyr, A. R., Huckaby, L. V., Shiva, S. S., and Zuckerbraun, B. S. (2020). Nitric Oxide and Endothelial Dysfunction. Crit. Care Clin. 36 (2), 307–321. doi:10.1016/j.ccc.2019.12.009
Diaz-Arjonilla, M., Schwarcz, M., Swerdloff, R. S., and Wang, C. (2009). Obesity, Low Testosterone Levels and Erectile Dysfunction. Int. J. Impot. Res. 21 (2), 89–98. doi:10.1038/ijir.2008.42
Ding, J., Tang, Y., Tang, Z., Zhang, X., and Wang, G. (2017). A Variant in the Precursor of MicroRNA-146a Is Responsible for Development of Erectile Dysfunction in Patients with Chronic Prostatitis via Targeting NOS1. Med. Sci. Monit. 23, 929–937. doi:10.12659/msm.898406
Duan, K.-B., Rajapakse, J. C., Wang, H., and Azuaje, F. (2005). Multiple SVM-RFE for Gene Selection in Cancer Classification with Expression Data. IEEE Trans.on Nanobioscience 4 (3), 228–234. doi:10.1109/tnb.2005.853657
Eissa, S., Matboli, M., and Bekhet, M. M. (2016). Clinical Verification of a Novel Urinary microRNA Panal: 133b, -342 and -30 as Biomarkers for Diabetic Nephropathy Identified by Bioinformatics Analysis. Biomed. Pharmacother. 83, 92–99. doi:10.1016/j.biopha.2016.06.018
Fabian, M. R., Sonenberg, N., and Filipowicz, W. (2010). Regulation of mRNA Translation and Stability by microRNAs. Annu. Rev. Biochem. 79, 351–379. doi:10.1146/annurev-biochem-060308-103103
Fang, S., Pan, J., Zhou, C., Tian, H., He, J., Shen, W., et al. (2019). Circular RNAs Serve as Novel Biomarkers and Therapeutic Targets in Cancers. Cgt 19 (2), 125–133. doi:10.2174/1566523218666181109142756
Fedorko, M., Juracek, J., Stanik, M., Svoboda, M., Poprach, A., Buchler, T., et al. (2017). Detection of Let-7 miRNAs in Urine Supernatant as Potential Diagnostic Approach in Non-metastatic clear-cell Renal Cell Carcinoma. Biochem. Med. (Zagreb) 27 (2), 411–417. doi:10.11613/bm.2017.043
Ghatak, K., Yin, G. N., Choi, M.-J., Limanjaya, A., Minh, N. N., Ock, J., et al. (2017). Dickkopf2 Rescues Erectile Function by Enhancing Penile Neurovascular Regeneration in a Mouse Model of Cavernous Nerve Injury. Sci. Rep. 7 (1), 17819. doi:10.1038/s41598-017-17862-5
Guyon, I., Weston, J., Barnhill, S., and Vapnik, V. (2002). Gene Selection for Cancer Classification Using Support Vector Machines. Machine Learn. 46 (1), 389–422. doi:10.1023/A:1012487302797
Han, W., Lu, D., Wang, C., Cui, M., and Lu, K. (2021). Identification of Key mRNAs, miRNAs, and mRNA-miRNA Network Involved in Papillary Thyroid Carcinoma. Cbio. 16 (1), 146–153. doi:10.2174/1574893615999200608125427
Hatzichristou, D., Hatzimouratidis, K., Bekas, M., Apostolidis, A., Tzortzis, V., and Yannakoyorgos, K. (2002). Diagnostic Steps in the Evaluation of Patients with Erectile Dysfunction. J. Urol. 168 (2), 615–620. doi:10.1097/00005392-200208000-00044
Hu, Y., Qiu, S., and Cheng, L. (2021). Integration of Multiple-Omics Data to Analyze the Population-specific Differences for Coronary Artery Disease. Comput. Math. Methods Med. 2021, 7036592. doi:10.1155/2021/7036592
Huang, Y. (2018). The Novel Regulatory Role of lncRNA-miRNA-mRNA axis in Cardiovascular Diseases. J. Cel. Mol. Med. 22 (12), 5768–5775. doi:10.1111/jcmm.13866
Huo, W., Li, H., Zhang, Y., and Li, H. (2020). Epigenetic Silencing of microRNA‐874‐3p Implicates in Erectile Dysfunction in Diabetic Rats by Activating the Nupr1/Chop‐mediated Pathway. FASEB j. 34 (1), 1695–1709. doi:10.1096/fj.201902086r
Jia, K., Shi, P., Han, X., Chen, T., Tang, H., and Wang, J. (2016). Diagnostic Value of miR-30d-5p and miR-125b-5p in Acute Myocardial Infarction. Mol. Med. Rep. 14 (1), 184–194. doi:10.3892/mmr.2016.5246
Jiang, X., Luo, Y., Zhao, S., Chen, Q., Jiang, C., Dai, Y., et al. (2015). Clinical Significance and Expression of microRNA in Diabetic Patients with Erectile Dysfunction. Exp. Ther. Med. 10 (1), 213–218. doi:10.3892/etm.2015.2443
Jiang, Z. H., Tang, Y. Z., Song, H. N., Yang, M., Li, B., and Ni, C. L. (2020). miRNA-342 S-uppresses R-enal I-nterstitial F-ibrosis in D-iabetic N-ephropathy by T-argeting SOX6. Int. J. Mol. Med. 45 (1), 45–52. doi:10.3892/ijmm.2019.4388
Joshi, P., Vedhanayagam, M., and Ramesh, R. (2021). An Ensembled SVM Based Approach for Predicting Adverse Drug Reactions. Cbio. 16 (3), 422–432. doi:10.2174/1574893615999200707141420
Khalyfa, A., Kheirandish-Gozal, L., Bhattacharjee, R., Khalyfa, A. A., and Gozal, D. (2016). Circulating microRNAs as Potential Biomarkers of Endothelial Dysfunction in Obese Children. Chest 149 (3), 786–800. doi:10.1378/chest.15-0799
Kong, L., Zhu, J., Han, W., Jiang, X., Xu, M., Zhao, Y., et al. (2011). Significance of Serum microRNAs in Pre-diabetes and Newly Diagnosed Type 2 Diabetes: a Clinical Study. Acta Diabetol. 48 (1), 61–69. doi:10.1007/s00592-010-0226-0
Kouidrat, Y., Pizzol, D., Cosco, T., Thompson, T., Carnaghi, M., Bertoldo, A., et al. (2017). High Prevalence of Erectile Dysfunction in Diabetes: a Systematic Review and Meta-Analysis of 145 Studies. Diabet. Med. 34 (9), 1185–1192. doi:10.1111/dme.13403
Lei, T., and Shu-Lin, W. (2021). Exploring miRNA Sponge Networks of Breast Cancer by Combining miRNA-disease-lncRNA and miRNA-target Networks. Curr. Bioinformatics 16 (3), 385–394. doi:10.2174/1574893615999200711171
Li, D.-S., Feng, L., Luo, L.-H., Duan, Z.-F., Li, X.-L., Yin, C.-H., et al. (2017). The Effect of microRNA-328 Antagomir on Erectile Dysfunction in Streptozotocin-Induced Diabetic Rats. Biomed. Pharmacother. 92, 888–895. doi:10.1016/j.biopha.2017.05.071
Li, J. H., Liu, S., Zhou, H., Qu, L. H., and Yang, J. H. (2014). starBase v2.0: Decoding miRNA-ceRNA, miRNA-ncRNA and Protein-RNA Interaction Networks from Large-Scale CLIP-Seq Data. Nucleic Acids Res. 42 (Database issue), D92–D97. doi:10.1093/nar/gkt1248
Lin, X., Li, C., Zhang, Y., Su, B., Fan, M., and Wei, H. (2017). Selecting Feature Subsets Based on SVM-RFE and the Overlapping Ratio with Applications in Bioinformatics. Molecules 23 (1), 52. doi:10.3390/molecules23010052
Liu, D., Huang, Y., Nie, W., Zhang, J., and Deng, L. (2021). SMALF: miRNA-Disease Associations Prediction Based on Stacked Autoencoder and XGBoost. BMC Bioinformatics 22 (1), 219. doi:10.1186/s12859-021-04135-2
Liu, S., Tang, H., Liu, H., and Wang, J. (2021). Multi-label Learning for the Diagnosis of Cancer and Identification of Novel Biomarkers with High-Throughput Omics. Cbio. 16 (2), 261–273. doi:10.2174/1574893615999200623130416
Long, J., Yang, H., Yang, Z., Jia, Q., Liu, L., Kong, L., et al. (2021). Integrated Biomarker Profiling of the Metabolome Associated with Impaired Fasting Glucose and Type 2 Diabetes Mellitus in Large-Scale Chinese Patients. Clin. Transl. Med. 11 (6), e432. doi:10.1002/ctm2.432
Love, M. I., Huber, W., and Anders, S. (2014). Moderated Estimation of Fold Change and Dispersion for RNA-Seq Data with DESeq2. Genome Biol. 15 (12), 550. doi:10.1186/s13059-014-0550-8
Lu, T. X., and Rothenberg, M. E. (2018). MicroRNA. J. Allergy Clin. Immunol. 141 (4), 1202–1207. doi:10.1016/j.jaci.2017.08.034
Malavige, L. S., and Levy, J. C. (2009). Erectile Dysfunction in Diabetes Mellitus. J. Sex. Med. 6 (5), 1232–1247. doi:10.1111/j.1743-6109.2008.01168.x
Matfin, G., Jawa, A., and Fonseca, V. A. (2005). Erectile Dysfunction: Interrelationship with the Metabolic Syndrome. Curr. Diab. Rep. 5 (1), 64–69. doi:10.1007/s11892-005-0070-8
Mitidieri, E., Cirino, G., d’Emmanuele di Villa Bianca, R., and Sorrentino, R. (2020). Pharmacology and Perspectives in Erectile Dysfunction in Man. Pharmacol. Ther. 208, 107493. doi:10.1016/j.pharmthera.2020.107493
Mo, F., Luo, Y., Fan, D., Zeng, H., Zhao, Y., Luo, M., et al. (2020). Integrated Analysis of mRNA-Seq and miRNA-Seq to Identify C-MYC, YAP1 and miR-3960 as Major Players in the Anticancer Effects of Caffeic Acid Phenethyl Ester in Human Small Cell Lung Cancer Cell Line. Cgt 20 (1), 15–24. doi:10.2174/1566523220666200523165159
Müller, A., and Mulhall, J. P. (2006). Cardiovascular Disease, Metabolic Syndrome and Erectile Dysfunction. Curr. Opin. Urol. 16 (6), 435–443. doi:10.1097/01.mou.0000250284.83108.a6
Natarajan, R., Putta, S., and Kato, M. (2012). MicroRNAs and Diabetic Complications. J. Cardiovasc. Trans. Res. 5 (4), 413–422. doi:10.1007/s12265-012-9368-5
Norsworthy, P. J., Thompson, A. G. B., Mok, T. H., Guntoro, F., Dabin, L. C., Nihat, A., et al. (2020). A Blood miRNA Signature Associates with Sporadic Creutzfeldt-Jakob Disease Diagnosis. Nat. Commun. 11 (1), 3960. doi:10.1038/s41467-020-17655-x
Ray, S. L., Coulson, D. J., Yeoh, M. L. Y., Tamara, A., Latief, J. S., Bakhashab, S., et al. (2020). The Role of miR-342 in Vascular Health. Study in Subclinical Cardiovascular Disease in Mononuclear Cells, Plasma, Inflammatory Cytokines and PANX2. Int. J. Mol. Sci. 21 (19), 7217. doi:10.3390/ijms21197217
Riaz, F., and Li, D. (2019). Non-coding RNA Associated Competitive Endogenous RNA Regulatory Network: Novel Therapeutic Approach in Liver Fibrosis. Cgt 19 (5), 305–317. doi:10.2174/1566523219666191107113046
Rosen, R. C., Riley, A., Wagner, G., Osterloh, I. H., Kirkpatrick, J., and Mishra, A. (1997). The International index of Erectile Function (IIEF): a Multidimensional Scale for Assessment of Erectile Dysfunction. Urology 49 (6), 822–830. doi:10.1016/s0090-4295(97)00238-0
Rupaimoole, R., and Slack, F. J. (2017). MicroRNA Therapeutics: towards a new era for the Management of Cancer and Other Diseases. Nat. Rev. Drug Discov. 16 (3), 203–222. doi:10.1038/nrd.2016.246
Ryan, J. G., and Gajraj, J. (2012). Erectile Dysfunction and its Association with Metabolic Syndrome and Endothelial Function Among Patients with Type 2 Diabetes Mellitus. J. Diabetes its Complications 26 (2), 141–147. doi:10.1016/j.jdiacomp.2011.12.001
Sanz, H., Valim, C., Vegas, E., Oller, J. M., and Reverter, F. (2018). SVM-RFE: Selection and Visualization of the Most Relevant Features through Non-linear Kernels. BMC Bioinformatics 19 (1), 432. doi:10.1186/s12859-018-2451-4
Seleem, M., Shabayek, M., and Ewida, H. A. (2019). MicroRNAs 342 and 450 Together with NOX-4 Activity and Their Association with Coronary Artery Disease in Diabetes. Diabetes Metab. Res. Rev. 35 (5), e3130. doi:10.1002/dmrr.3130
Shamloul, R., and Ghanem, H. (2013). Erectile Dysfunction. The Lancet 381 (9861), 153–165. doi:10.1016/s0140-6736(12)60520-0
Sheng, Y., Jiang, Y., Yang, Y., Li, X., Qiu, J., Wu, J., et al. (2021). CNA2Subpathway: Identification of Dysregulated Subpathway Driven by Copy Number Alterations in Cancer. Brief Bioinform. 22 (5), bbaa413. doi:10.1093/bib/bbaa413
Shin, S. H., Kim, W. J., Choi, M. J., Park, J.-M., Jin, H.-R., Yin, G. N., et al. (2014). Aberrant Expression of Wnt Family Contributes to the Pathogenesis of Diabetes-Induced Erectile Dysfunction. Andrology 2 (1), 107–116. doi:10.1111/j.2047-2927.2013.00162.x
Sun, Z., Ma, Y., Chen, F., Wang, S., Chen, B., and Shi, J. (2018). miR-133b and miR-199b Knockdown Attenuate TGF-Β1-Induced Epithelial to Mesenchymal Transition and Renal Fibrosis by Targeting SIRT1 in Diabetic Nephropathy. Eur. J. Pharmacol. 837, 96–104. doi:10.1016/j.ejphar.2018.08.022
Tang, H., Zhao, Y.-W., Zou, P., Zhang, C.-M., Chen, R., Huang, P., et al. (2018). HBPred: a Tool to Identify Growth Hormone-Binding Proteins. Int. J. Biol. Sci. 14 (8), 957–964. doi:10.7150/ijbs.24174
Tang, M., Liu, C., Liu, D., Liu, J., Liu, J., and Deng, L. (2021). PMDFI: Predicting miRNA-Disease Associations Based on High-Order Feature Interaction. Front. Genet. 12, 656107. doi:10.3389/fgene.2021.656107
Vasu, S., Kumano, K., Darden, C. M., Rahman, I., Lawrence, M. C., and Naziruddin, B. (2019). MicroRNA Signatures as Future Biomarkers for Diagnosis of Diabetes States. Cells 8 (12), 1533. doi:10.3390/cells8121533
Wang, J.-y., Yang, Y., Ma, Y., Wang, F., Xue, A., Zhu, J., et al. (2020). Potential Regulatory Role of lncRNA-miRNA-mRNA axis in Osteosarcoma. Biomed. Pharmacother. 121, 109627. doi:10.1016/j.biopha.2019.109627
Wang, L., Xuan, Z., Zhou, S., Kuang, L., and Pei, T. (2019). A Novel Model for Predicting LncRNA-Disease Associations Based on the LncRNA-MiRNA-Disease Interactive Network. Cbio. 14 (3), 269–278. doi:10.2174/1574893613666180703105258
Wang, X., Qian, R., Zhang, W., Chen, S., Jin, H., and Hu, R. (2009). MicroRNA-320 Expression in Myocardial Microvascular Endothelial Cells and its Relationship with Insulin-like Growth Factor-1 in Type 2 Diabetic Rats. Clin. Exp. Pharmacol. Physiol. 36 (2), 181–188. doi:10.1111/j.1440-1681.2008.05057.x
Wen, Y., Liu, G., Zhang, Y., and Li, H. (2019). MicroRNA‐205 Is Associated with Diabetes Mellitus‐induced Erectile Dysfunction via Down‐regulating the Androgen Receptor. J. Cel. Mol. Med. 23 (5), 3257–3270. doi:10.1111/jcmm.14212
Wu, Y., Lu, X., Shen, B., and Zeng, Y. (2019). The Therapeutic Potential and Role of miRNA, lncRNA, and circRNA in Osteoarthritis. Cgt 19 (4), 255–263. doi:10.2174/1566523219666190716092203
Yang, H., Luo, Y., Ren, X., Wu, M., He, X., Peng, B., et al. (2021). Risk Prediction of Diabetes: Big Data Mining with Fusion of Multifarious Physical Examination Indicators. Inf. Fusion 75, 140–149. doi:10.1016/j.inffus.2021.02.015
Yang, X.-F., Zhou, Y.-K., Zhang, L., Gao, Y., and Du, P.-F. (2020). Predicting LncRNA Subcellular Localization Using Unbalanced Pseudo-k Nucleotide Compositions. Cbio. 15 (6), 554–562. doi:10.2174/1574893614666190902151038
Yuyun, M. F., Ng, L. L., and Ng, G. A. (2018). Endothelial Dysfunction, Endothelial Nitric Oxide Bioavailability, Tetrahydrobiopterin, and 5-methyltetrahydrofolate in Cardiovascular Disease. Where Are We with Therapy? Microvasc. Res. 119, 7–12. doi:10.1016/j.mvr.2018.03.012
Zagidullin, B., Aldahdooh, J., Zheng, S., Wang, W., Wang, Y., Saad, J., et al. (2019). DrugComb: an Integrative Cancer Drug Combination Data portal. Nucleic Acids Res. 47 (W1), W43–W51. doi:10.1093/nar/gkz337
Zhang, Z., Zhang, S., Li, X., Zhao, Z., Chen, C., Zhang, J., et al. (2021). Reference Genome and Annotation Updates lead to Contradictory Prognostic Predictions in Gene Expression Signatures: a Case Study of Resected Stage I Lung Adenocarcinoma. Brief Bioinform. 22 (3), bbaa081. doi:10.1093/bib/bbaa081
Zhao, T., Hu, Y., Peng, J., and Cheng, L. (2020). DeepLGP: a Novel Deep Learning Method for Prioritizing lncRNA Target Genes. Bioinformatics 36 (16), 4466–4472. doi:10.1093/bioinformatics/btaa428
Zhu, M., Yi, M., Kim, C. H., Deng, C., Li, Y., Medina, D., et al. (2011). Integrated miRNA and mRNA Expression Profiling of Mouse Mammary Tumor Models Identifies miRNA Signatures Associated with Mammary Tumor Lineage. Genome Biol. 12 (8), R77. doi:10.1186/gb-2011-12-8-r77
Zhu, Q., Fan, Y., and Pan, X. (2021). Fusing Multiple Biological Networks to Effectively Predict miRNA-Disease Associations. Cbio. 16 (3), 371–384. doi:10.2174/1574893615999200715165335
Zhu, Z., Han, X., and Cheng, L. (2021). Identification of Gene Signature Associated with Type 2 Diabetes Mellitus by Integrating Mutation and Expression Data. Curr. Gene Ther. doi:10.2174/1566523221666210707140839
Keywords: micrornas, diabetes mellitus, erectile dysfunction, signature, molecular mechanisms
Citation: Xu H, Zhao B, Zhong W, Teng P and Qiao H (2021) Identification of miRNA Signature Associated With Erectile Dysfunction in Type 2 Diabetes Mellitus by Support Vector Machine-Recursive Feature Elimination. Front. Genet. 12:762136. doi: 10.3389/fgene.2021.762136
Received: 21 August 2021; Accepted: 22 September 2021;
Published: 11 October 2021.
Edited by:
Lei Deng, Central South University, ChinaReviewed by:
Hui Ding, University of Electronic Science and Technology of China, ChinaJuan Wang, Inner Mongolia University, China
Copyright © 2021 Xu, Zhao, Zhong, Teng and Qiao. This is an open-access article distributed under the terms of the Creative Commons Attribution License (CC BY). The use, distribution or reproduction in other forums is permitted, provided the original author(s) and the copyright owner(s) are credited and that the original publication in this journal is cited, in accordance with accepted academic practice. No use, distribution or reproduction is permitted which does not comply with these terms.
*Correspondence: Hong Qiao, qiaohong@hrbmu.edu.cn