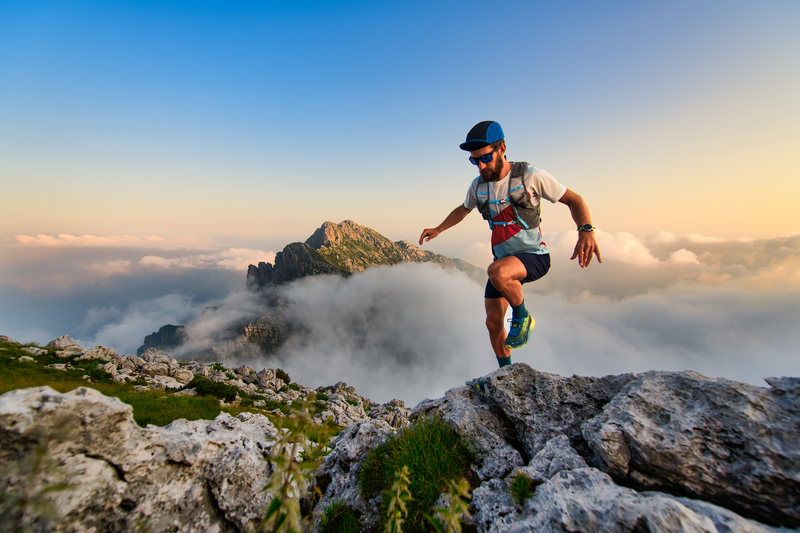
94% of researchers rate our articles as excellent or good
Learn more about the work of our research integrity team to safeguard the quality of each article we publish.
Find out more
REVIEW article
Front. Genet. , 21 October 2021
Sec. Statistical Genetics and Methodology
Volume 12 - 2021 | https://doi.org/10.3389/fgene.2021.760501
This article is part of the Research Topic Prediction and Explanation in Biomedicine using Network-Based Approaches View all 11 articles
Inflammatory bowel disease (IBD) is a chronic immune-mediated condition arising due to complex interactions between multiple genetic and environmental factors. Despite recent advances, the pathogenesis of the condition is not fully understood and patients still experience suboptimal clinical outcomes. Over the past few years, investigators are increasingly capturing multi-omics data from patient cohorts to better characterise the disease. However, reaching clinically translatable endpoints from these complex multi-omics datasets is an arduous task. Network biology, a branch of systems biology that utilises mathematical graph theory to represent, integrate and analyse biological data through networks, will be key to addressing this challenge. In this narrative review, we provide an overview of various types of network biology approaches that have been utilised in IBD including protein-protein interaction networks, metabolic networks, gene regulatory networks and gene co-expression networks. We also include examples of multi-layered networks that have combined various network types to gain deeper insights into IBD pathogenesis. Finally, we discuss the need to incorporate other data sources including metabolomic, histopathological, and high-quality clinical meta-data. Together with more robust network data integration and analysis frameworks, such efforts have the potential to realise the key goal of precision medicine in IBD.
Inflammatory bowel disease (IBD), comprising Ulcerative Colitis (UC) and Crohn’s disease (CD), is a chronic, immune-mediated inflammatory disorder which primarily involves the gastrointestinal tract (Lennard-Jones, 1989; Baumgart and Carding, 2007). It causes significant morbidity and affects almost seven million people worldwide. The prevalence is forecasted to rise steeply in the decades ahead, particularly in newly industrialised countries (GBD 2017 Inflammatory Bowel Disease Collaborators, 2020). IBD arises due to a dysregulated immune response secondary to complex interactions between multiple genetic risk factors, a “dysbiotic” gut microbiota, and environmental factors (Xavier and Podolsky, 2007; Cader and Kaser, 2013). However, the precise mechanistic pathways interlinking these various facets of IBD pathogenesis are still largely unknown (Cader and Kaser, 2013). In addition, despite recent advances in medical management including the use of biologic and small molecule therapies, a significant proportion of patients who wish to avoid surgery fail to achieve sustained clinical remission (Cosnes et al., 2011). This highlights the need for novel, effective therapeutic strategies in IBD.
Unlike rare, and well-defined monogenic disorders (e.g., cystic fibrosis) which occur due to mutations within a single gene, complex diseases such as IBD arise due to interactions between numerous genetic variants and environmental factors. These interactions occur across several layers that transcend the ecologic, genetic, epigenetic, protein and cellular levels, and work collectively to manifest the disease phenotype. Consequently, IBD demonstrates significant heterogeneity across the population i.e., patients may have varying environmental exposures and express different genetic variants which result in the activation of varying pathogenic pathways. Hence, a one-size-fits-all approach to therapy, as is currently practised, may explain the suboptimal clinical outcomes seen in IBD.
As a result, precision medicine has been identified as a key strategy for improving clinical outcomes in IBD (Denson et al., 2019; Verstockt et al., 2021). Precision medicine aims to harness the biological characteristics of individual patients to tailor the right therapy to the right patient at the right time (Whitcomb, 2019). This would require an understanding of the function of individual biological components and also the holistic effects of their multifactorial interactions to stratify patients (Green et al., 2017; Sudhakar et al., 2021). Whilst still in its infancy, an early example of this approach in IBD is the PROFILE study. In this trial, researchers are utilising a transcriptomic signature of peripheral blood CD8+ T lymphocytes as a biomarker to separate CD patients into two subgroups according to predicted disease course to guide therapeutic strategy i.e. “step up” vs “top down” therapy (Noor et al., 2020). This transcriptomic signature was found to be effective for prognostication through an earlier non-interventional study (Biasci et al., 2019). It is anticipated that multi-omics approaches may be even more robust for directing precision therapies in IBD and other complex disorders (Olivera et al., 2019; Borg-Bartolo et al., 2020). In this effort, over the past decade, researchers across the world have begun profiling the transcriptomics, epigenetics, metabolomics, and proteomics data of large patient cohorts. For IBD, a number of biorepositories have become established such as the IBD BioResource in the United Kingdom (Parkes and IBD BioResource Investigators, 2019), the 1000IBD project in the Netherlands (Spekhorst et al., 2017), and the IBD Multiomics Data project in the USA (Imhann et al., 2019). However, this exponential increase in the availability of molecular data harnessed through “omics” technologies has created one of the biggest challenges we face in biology in the 21st century i.e., what is the best way to make meaningful sense of this data to ultimately improve clinical outcomes in individual patients?
Systems biology and artificial intelligence are two complementary fields that are driving novel computational biology approaches to address this challenge. Systems biology is an interdisciplinary field that allows the systematic study of complex interactions in biological systems using a holistic approach (Ahn et al., 2006; Breitling, 2010). Artificial intelligence, on the other hand, is a domain within computer science which leverages computer systems to perform tasks that normally require human intelligence including problem-solving and decision-making (Meskó and Görög, 2020). Machine learning and deep learning, which are subdomains of artificial intelligence, offer a number of potential solutions to tackle this problem. We have previously reviewed these approaches in depth in the context of IBD (Seyed Tabib et al., 2020). In this narrative review, however, we will focus on the utility of network biology, a subfield of systems biology, to facilitate precision medicine in IBD.
Network biology is one of the fundamental tenets of systems biology, which involves using mathematical graph theory to represent, integrate, and analyse biological processes and data through networks (Pavlopoulos et al., 2011). Depending on the type of data, various biological networks can be produced, such as protein-protein interaction networks, gene regulatory networks, and metabolic networks (Vidal et al., 2011). Using network-based methods as an integration and modelling tool, important molecular interactions can be unravelled. When applied to individual patients, personalised network analysis can lead to the identification of new disease subtypes and therapeutic targets, which facilitates novel drug discovery, biomarker discovery, and drug repurposing as has been seen in cancer (Módos et al., 2017). Hence, network biology can be a valuable tool for analysing multi-omics patient data to achieve the key goal of precision medicine in IBD and other complex disorders (Korcsmaros et al., 2017).
Although in its nascent stages, in this narrative review we will highlight a variety of innovative network biology approaches that are bringing the promise of precision medicine closer to a translational reality in IBD (Table 1). First, however, we will briefly discuss some of the fundamental concepts underpinning network biology.
TABLE 1. Characteristics of various network types discussed in this review and their main advantages and disadvantages.
A biological network is the representation of a biological system using graphs. It contains biological entities (e.g., cells, proteins or genes) and their interactions with each other (e.g., protein-protein interactions). In network biology, these are called nodes and edges, respectively (Koutrouli et al., 2020). The topology of a network (i.e., the way in which nodes and edges are arranged within a network) can be evaluated to better understand a biological system (Figure 1). In biological networks, the topology is usually scale-free i.e., the degree distribution of nodes follows a power law, unlike random networks (Barabási and Oltvai, 2004). This means that some nodes in a biological network may have many interactions called “hubs,” whilst other nodes may have fewer connections (Charitou et al., 2016). Furthermore, specific regions of a scale-free network can be more highly interconnected than other parts of the network. These highly connected regions of a network are called modules. Modules often correspond to specific biological functions within the overall system. Specific nodes that connect distinct modules can also be identified. These are termed “bottleneck nodes” as information needs to traverse through them for one module (or biological subtask) to communicate with another (Csermely et al., 2013).
FIGURE 1. Basic network biological nomenclature and concepts. Hubs are nodes with a high number of interactions (edges). Modules are regions of the network where the nodes interact with members of the region more than with non-members. Bottlenecks are nodes which are connecting two.
To further analyse the topology of networks many tools have been developed. One method is to identify network motifs. Network motifs are recurring, significant patterns of interconnections within a network (Milo et al., 2002). Network motifs can provide insights into the type of signalling interactions that occur within different biological networks. For instance, feedforward loops are more common in transcriptional regulatory networks (Hong et al., 2018). Another technique to find the building blocks of a network is to identify graphlets. Graphlets are small, unique (non-isomorphic) subnetworks of a network (Przulj et al., 2004). Using graphlets, the local structure of a network can be better described (Przulj, 2007). Przulj and her colleagues have used graphlets to describe various networks including protein-protein interactions (Przulj et al., 2006) and the world trade network (Sarajlić et al., 2016).
Although it may not be possible to encapsulate all dimensions and features of a complex disease using networks, network analysis can be a valuable approach for better understanding the disease. For instance, disturbance of hubs and bottlenecks in a biological network are likely to have significant consequences on the overall functioning of system. A prime example is the mechanisms driving drug resistance in HER2-amplified breast cancer, in which hub proteins within compensatory circuits and feedback loops were identified (Lee et al., 2012). This led to novel therapeutic strategies for overcoming drug resistance and improving outcomes in these patients (Kirouac et al., 2013). With the successful implementation of network biology in breast cancer and other cancers over the past decade (Yan et al., 2016), researchers are increasingly looking to gain similar translatable insights in complex diseases such as IBD.
Protein-protein interaction (PPI) networks refer to networks consisting of proteins as nodes and the physical interactions between them as edges (Vidal et al., 2011) (Table 1). PPI data can be captured using several different methodologies including experimental approaches such as yeast two-hybrid assays and affinity purification coupled mass spectrometry, as well as computational predictive methods such as text-mining and machine learning approaches (Snider et al., 2015). Several resources containing PPI data are available for use including STRING (Szklarczyk et al., 2019), BioGRID (Chatr-Aryamontri et al., 2015), Bioplex (Huttlin et al., 2021), HAPPI-2 (Chen et al., 2009), HuRI (Luck et al., 2020), and IntAct (Hermjakob et al., 2004) (Table 2). PPI networks that are directed can facilitate better modelling of intra- and inter-cellular signalling. To gain information regarding the direction of PPIs, additional experimental data is often required. Several databases have performed a comprehensive manual curation of such experimental data from the literature to provide information on directed PPIs. These include SignaLink (Fazekas et al., 2013), Reactome (Jassal et al., 2020), and the community-driven WikiPathways (Kutmon et al., 2016) (Table 2). It is important to note that all network resources have drawbacks depending on the methods that were used to compile the data. Manually curated and text mining-based networks overrepresent certain genes which are hot topics of research - for instance, p53 is often a culprit. On the other hand, unbiased approaches like yeast two-hybrid or affinity purification overrepresent proteins that bind easily to other proteins like heat shock proteins. This can inadvertently implicate heat shock proteins as being associated with all diseases (Csermely et al., 2013). Hence, researchers need to be aware of the scope and bias of the network resources they use for their analysis.
By overlaying additional expression data from RNA sequencing or microarrays, PPI networks can be contextualised to specific pathological states or conditions (Figure 2). As a result, proteins are often represented by their transcripts in PPI networks. In this way, PPI networks can be used to detect novel disease-related genes, modules and signalling pathways. However, the application of transcriptomics data to build protein interactions is based on the assumption that a gene transcript accurately represents the amount of protein within the cell. This assumption is only partially true (Kosti et al., 2016).
FIGURE 2. Various methods for generating PPI networks in IBD. (A) Known IBD-associated genes can be mapped to a PPI network and the nearby genes in the network can be associated with IBD as well (guilt by association) (B) Mapping a transcriptome to the PPI network can elucidate disease-specific modules in the network (C) Single-cell RNA-seq data combined with intercellular (ligand-receptor) communication networks can show how various cells are interacting with each other in disease or healthy states. For b) the data from (Olsen et al., 2009) was used. For c) the uniform manifold approximation and projection (UMAP) plot (a nonlinear dimensionality reduction technique for visualising high-dimensional data) was generated using data from Lukassen et al., 2020 (Lukassen et al., 2020).
Network propagation can also be utilised to reveal further disease-associated genes (reviewed by Cowen et al. (2017)). In short, with this approach, a set of known disease-related genes are first mapped to a PPI network and algorithms are used to detect additional proteins (or genes) that are likely to be disease-associated. Such algorithms identify additional proteins (or genes) by finding the interactor partners of the known disease-related genes using a heat propagation algorithm or a random walk approach. These methods assume that proteins (or genes) near a disease-related gene are likely to be associated with the disease as well. This is called guilt by association. Huang et al evaluated various resources that generate PPI networks to see which is the most useful for detecting disease-related genes using network propagation (Huang et al., 2018). They found that the optimal solution came from building a composite network (the parsimonious composite network or PCNet) in which interactions were supported by a minimum of two network resources.
PPI network-based approaches have been frequently used in IBD research over the past decade such as the study by Eguchi et al. (2018). In this study, the authors determined differentially expressed genes (DEGs) from transcriptomic data of IBD patients and extracted a set of known IBD genes from the DisGeNet database (Piñero et al., 2017) to construct an IBD-relevant PPI network (Figure 2B). The authors were able to identify modules within this network by using the DPClusO algorithm (Altaf-Ul-Amin et al., 2012). These IBD gene-enriched modules were used to predict novel IBD-relevant genes and pathways.
In recent years, PPI networks have also been used to generate intercellular communication networks with single-cell RNA sequencing (scRNAseq) data (Figure 2C). The method for overlaying PPI networks with scRNAseq data is dependent on the research question being asked i.e., whether the researcher is interested in studying the overall possible ligand-receptor interactions or the condition-specific changes in the strength of interactions between particular cell populations (see review by Armingol et al. (2021)). In either case, databases containing ligand-receptor interactions are required such as CellPhoneDB (Efremova et al., 2020), the FANTOM5 consortium database (Ramilowski et al., 2015) or a one-stop solution OmniPath, which we co-developed recently (Türei et al., 2021) (Table 2). OmniPath contains both ligand-receptor interactions as well as downstream intracellular signalling connections (Türei et al., 2021).
An example of such an approach using scRNAseq data in IBD is the study by Smillie et al. (2019). They obtained scRNAseq data from healthy, non-inflamed UC, and inflamed UC colonic biopsies to create PPI networks of intercellular communication (Smillie et al., 2019). The authors first identified ligand-receptor interactions within specific cell types in their scRNAseq datasets by using the FANTOM5 consortium database (Ramilowski et al., 2015). They included only ligand and receptor genes that were significantly differentially expressed between the three conditions and that were also highly-expressed cell subset markers. Using the connections between these filtered ligands and receptors they then constructed cell-cell interaction networks. Statistical analysis of this network revealed significant cell-cell interactions in the various states. In this way, the authors were able to reveal the rewiring of intercellular connections between healthy and UC states. In the healthy colonic mucosa, intercellular interactions were largely found to be occurring between cell types typically associated with colonic homeostasis such as T regulatory (Treg) cells, dendritic cell type 1 (DC1) cells, as well as CD8+ intraepithelial lymphocytes (IELs) and CD8+ IL17+ T cells. However, in both uninflamed and inflamed states of the UC colonic mucosa, intercellular interactions were shown to be enriched between M-like cells and inflammatory fibroblasts.
To further discern changes in intercellular communication as well as subsequent downstream intracellular signalling in UC patients, we interrogated the scRNAseq data from Smillie et al using OmniPath (Türei et al., 2021). This enabled us to build an integrated network containing both intercellular PPIs and downstream intracellular PPIs in UC patients and healthy controls. This analysis revealed significant rewiring of intercellular communication between myofibroblasts and T regulatory cells (Tregs) in UC patients in comparison to healthy individuals. These changes in intercellular interactions led to major downstream signalling differences in Tregs in UC patients, in particular the TLR4 and TLR3 pathways. These pathways regulate inflammatory cytokine expression and can decrease the abundance of Treg cells (Türei et al., 2021). These findings support the hypothesis that disruption of myofibroblast-mediated regulation of Tregs may play a key role in UC pathogenesis (Pinchuk et al., 2011).
In metabolic networks, nodes represent metabolites whilst edges refer to enzymes that catalyse metabolic reactions between the substrate and product metabolites (Vidal et al., 2011). The most common way of analysing a metabolic network is using flux-balance analysis, which involves calculating the flow of metabolites through the network in steady state (Orth et al., 2010; Anand et al., 2020). (Figure 3). The aim of the analysis is to find the best potential flux through the various reactions to maximise the output of a given reaction. These reactions are usually represented by cell mass or energy (ATP production). This results in an optimizable linear equation system giving back metabolic fluxes. The constraints of the model can be modified by gene expression or other experimental results. In recent years the metabolic networks of entire organisms have become available. To model the human host, the Recon2 resource provides a comprehensive global reconstruction of human metabolism (Thiele et al., 2013). For the gut microbiome, the semi-automated AGORA approach makes it possible to reconstruct the metabolism of gut microbial communities from metagenomic data (Magnúsdóttir et al., 2017). These genome-scale metabolic networks make it possible to evaluate the metabolism of the human host and gut bacterial species in the context of IBD, and discover important host-microbiome interactions (Jansma and El Aidy, 2021).
FIGURE 3. Flux balance analysis - the basics of metabolic network modelling. For metabolic networks the initial step involves collecting the metabolic reactions that form the network. These reactions are represented by a stoichiometric matrix where each reaction is represented by the nodes and metabolites by the edges. The aim of flux balance analysis is to find the optimal vector (flux) that yields the maximum output for a given metabolite or metabolites (Z) through these reactions. For illustration, the glucose metabolism was used from Köenig et al., 2012 (König et al., 2012).
Out of all the network types reviewed here, metabolic networks have the highest completeness in terms of interactions. This makes them ideal for modelling. However, metabolomic studies are far less numerous in comparison to transcriptomics studies as RNA sequencing technologies are now far more high-throughput. In addition, a disadvantage of the standard flux balance analysis is that it needs to be optimised towards a selected metabolic reaction. When investigating IBD, the usual optimisation functions like cell growth are not relevant, so other appropriate targets need to be selected e.g., bile acid production. An alternative solution to avoid this problem is by using the metabolic network as a template and analysing it topologically (Knecht et al., 2016).
In a recent study, Heinken et al used the COBRA (Heirendt et al., 2019) genome-scale metabolic modelling software to evaluate the metabolic potential of the gut microbiome in IBD patients (Heinken et al., 2021). They found that IBD patients with dysbiosis had reduced metabolic diversity with diminished sulphur production, owing to the reduced diversity in microbial strains. In a separate study, Heinken et al also utilised flux balance analysis and genome-scale metabolic modelling to evaluate the differences in bile acid metabolism between IBD patients and healthy controls (Heinken et al., 2019). Here the optimisation function of the flux balance analysis was bile acid biotransformation. They found that one microbial species alone could not generate the whole spectrum of secondary bile acids present in the gut, but microbial pairs could generate most of these bile acids in silico. The network modelling also revealed that the dysbiotic microbiome of paediatric IBD patients was depleted of secondary bile acids compared to healthy children, as observed in previous studies (Duboc et al., 2013). The analysis also identified strain-specific bottlenecks that limited primary bile acid (PBA) biotransformation to secondary bile acids (SBA). Disruption of these strains may have important consequences on the inflammatory milieu in IBD, as PBAs and SBAs have been found to exert immune modulatory effects on the gut mucosa through their actions on T regulatory cells and Th17 cells (Hang et al., 2019; Sinha et al., 2020; Song et al., 2020).
An alternative approach of utilising metabolic networks to explore host metabolism in IBD was demonstrated by Knecht et al. They constructed metabolic networks by selecting enzymes which were differentially expressed between healthy controls and paediatric IBD patients from gene expression data (Knecht et al., 2016). They found that metabolic network coherence was high and varied significantly between individuals in the IBD patient cohort in comparison to healthy controls. This could have important implications for drug response in IBD patients, as metabolic networks can play a significant role in determining drug metabolism and response to treatment. Further work is needed to identify whether metabolic networks could act as a novel biomarker for determining drug response in IBD.
A gene regulatory network (GRN) depicts the molecules that govern expression levels of genes as messenger RNA (mRNA) and proteins (Vidal et al., 2011) (Table 1). Nodes can represent transcription factor proteins, genes, cis- and trans- DNA regulatory elements, or microRNA (miRNA). Edges represent physical interactions between these molecular entities and are directed i.e. information is provided regarding whether a molecule inhibits or activates another molecule (Schlitt and Brazma, 2007). GRNs can be mapped using yeast one-hybrid (Y1H), chromatin immunoprecipitation (ChIP) approaches, ChIP-sequencing, and DNA affinity purification (Yeh et al., 2019).
GRNs can be modelled with so-called Bayesian-based network inference approaches to predict the hierarchy and the directionality of the interactions in the network. Bayesian networks are founded on the Bayes theorem, which states that the probability of event A given the occurrence of another event B i.e., P (A|B), is equal to the product of the probability of event B given the occurrence of event A i.e., P(B|A) and the probability of event A i.e., P(A), divided by the probability of event B i.e., P(B) (Bayes, 1763) (Figure 4). We can predict the likelihood of event A given the occurrence of event B i.e., P (A|B), if we know how often events A and B occur and how often event B occurs given the prior occurrence of event A. The Bayes theorem can be expanded to be used with transcriptomics data, because the expression of certain genes is dependent on other genes (Friedman et al., 2000). Hence, by applying the Bayes theorem to transcriptomic data it is possible to develop a network to predict which genes are influencing the expression of other genes. As an output, a Bayesian network approach produces a hierarchical graph which reveals the most plausible causal interactions occurring between genes. However, there are two limitations with this approach. Firstly, the Bayesian graph has to be acyclic i.e., it must lack biological feedback loops. Secondly, finding the optimal Bayesian network is a computationally hard optimisation problem as a Bayesian approach results in many equally or similarly good solutions. To tackle the first issue, the research question must be properly defined i.e., research questions involving feedback loops in the biological process cannot be studied using Bayesian network approaches. The second problem can be addressed by reducing the optimisation problem to a limited search space by using predefined biologically meaningful interactions (e.g., interactions from experimentally validated sources).
FIGURE 4. Bayesian network construction for gene regulatory networks. From the high dimension of gene expression data, the correlations between genes can be calculated. These correlations can be modelled as conditional probabilities and, using the Bayes theorem, a casual gene regulatory network can be constructed.
In gene co-expression networks (GCNs), nodes represent genes and edges connect pairs of genes that are considered co-expressed based on a certain measure (Vidal et al., 2011). Unlike GRNs, edges are undirected and simply indicate a correlation in the expression of two genes, from which causality is inferred. GCNs have become a particularly popular method in recent years as they can be constructed directly from data obtained through high-throughput gene expression experiments such as microarrays or RNA-sequencing (van Dam et al., 2018). The gene co-expression can be measured using a variety of techniques (we encourage the reader to read the comprehensive review by Sonawane et al for a summary of these algorithms (Sonawane et al., 2019)). Of these, the most commonly used algorithm is the Weighted Gene Co-expression Analysis (WGCNA) (Langfelder and Horvath, 2008) (Figure 5). In essence, the WGCNA algorithm calculates the correlation between the genes. This correlation is raised on a user-defined power to filter out weak interactions resulting in a scale-free network. The adjacency matrix of this network is used for clustering to find modules which represent co-regulated biological functions. GCNs and GRNs are often used together as they complement each other. The biggest advantage of these networks is that only gene expression data is required and this can be specific for the disease in question. Furthermore, the models can be refined by adding biological constraints such as known regulatory interactions like transcription factor-target gene interactions. However, their largest drawback is the a priori assumption that genes which are regulated and expressed together have similar functions. This notion is not always true (Sevilla et al., 2005). GRNs are also based on the assumption that correlation implies causation.
FIGURE 5. Gene co-expression network analysis. Calculating a similarity between the genes from expression data can be used as an adjacency matrix in a co-expression network. The similarity function depends on the used method but after that the most similar parts of the network can be denoted as modules.
An example of using GCNs and GRNs in IBD is the landmark study by Jostins et al. In this paper, the authors performed a meta-analysis of 15 genome-wide association studies (GWAS) of CD and/or UC, to identify 73 novel and a total of 163 IBD-associated genomic loci (Jostins et al., 2012). The authors undertook network biology analysis of this data to understand how IBD-associated loci may influence pathogenesis. They performed WGCNA of gene expression data obtained from a variety of tissues including stomach, liver, adipose tissue, and blood, and identified 211 co-expression modules. These were then screened against the IBD-associated genomic loci. They identified that IBD-associated loci were particularly enriched in a module consisting of 523 genes from omental adipose tissue obtained from morbidly obese patients (i.e. the “IBD-enriched module”). Jostins et al also used a Bayesian network inference method to create a GRN for IBD. To do this, they combined both genotype and gene expression data to infer a direction in terms of causality for the effect of single nucleotide polymorphisms (SNPs) on the identified gene expression. The overlap between this network and the genes in the IBD-enriched module revealed a sub-network of genes that were highly expressed in bone marrow-derived macrophages. Thus, by using gene regulatory and gene co-expression networks, the authors were able to annotate IBD-associated GWAS loci to a particular immune cell network and infer causality.
Peters et al employed network biology approaches in three independent cohorts of IBD patients, representing distinct stages of the disease (treatment naïve paediatric patients, patients refractory to biologic therapy, and patients with advanced disease undergoing bowel resection), to identify key driver genes that regulated IBD networks (Peters et al., 2017). The authors integrated data about known IBD-associated SNPs, and expression quantitative trait loci (eQTL) and cis-regulatory element (CRE) data from the aforementioned IBD cohorts, to identify candidate causal IBD genes in specific immune cell types. These candidate genes from all immune cell types were then intersected with modules found within GCNs obtained from the three IBD patient cohorts. The authors then identified modules in these networks that were significantly enriched for genes within the macrophage-enriched immune network from Jostins et al. (2012). This enabled them to generate “super-immune” modules by taking the union of these modules from each cohort. By evaluating common genes of these super-modules, the authors identified a core set of IBD susceptibility genes that were conserved across all three cohorts that were also enriched for the macrophage-enriched immune network and macrophage expression. This was termed the core immune activation module (IAM). By overlaying the core IAM onto Bayesian networks constructed from gene expression data from each cohort, the authors were able to identify an IBD-specific conserved immune component (CIC) in each network. Ultimately, using this approach, the authors identified 133 key driver genes which could regulate the IBD CIC networks, five of which had not been previously associated with IBD including DOCK2, DOK3, AIF1, GPSM3, NCKAP1L. The expression of these genes were shown to correlate with disease duration and also were upregulated in inflamed IBD patient intestinal biopsies.
Verstockt et al demonstrated the utility of GCNs to evaluate gene dysregulation at various stages of CD (Verstockt et al., 2019). In this study, transcriptomic and miRNA data were obtained from ileal mucosal biopsies of CD patients at three different stages of their disease i.e., newly diagnosed, recurrent disease following ileal resection, and late-stage disease. The authors conducted a WGCNA on this data which revealed modules that correlated with the three disease stages. The modules positively correlating with the different stages of CD were enriched in genes relating to granulocyte adhesion, diapedesis, and fibrosis. Conversely, genes associated with cholesterol biosynthesis were enriched in the module that negatively correlated with these stages of CD. They also constructed a miRNA-target gene GRN using the Ingenuity Pathway Analysis (IPA) microRNA Target Filter tool. This revealed that dysregulated miRNAs were more abundant in newly diagnosed and late-stage CD in comparison to post-operative recurrent CD. This suggests that surgical resection of the ileum followed by ileo-colonic anastomosis may reset the gene dysregulation occurring in CD.
A recent study by Aschenbrenner et al showed how GCNs could also be used to study cytokine signalling in CD (Aschenbrenner et al., 2021). They utilised transcriptomic data of ileal biopsies from a cohort of treatment naïve paediatric CD patients and non-inflamed controls to investigate the regulation of IL23. IL23 is a pro-inflammatory cytokine that has been implicated in IBD pathogenesis. Genetic studies have previously identified IBD-associated SNPs affecting the IL23R gene (Duerr et al., 2006). Furthermore, increased production of IL23 by macrophages and dendritic cells have been detected in mouse models of colitis and IBD patients (Maloy and Kullberg, 2008). Aschenbrenner et al conducted a WGCNA to see which modules of the transcriptome from inflamed and non-inflamed tissues correlate with IL23 expression. This analysis identified 22 gene co-expression modules. Analysis of these modules revealed that IL23A expression strongly correlated with the modules enriched in functions for “immune cell differentiation” and “lymphocyte differentiation.” These modules were found not to be significantly enriched in CD patients. However, an “inflammatory cytokine” module containing myeloid and stromal marker genes, proinflammatory cytokines (including OSM, IL1B, and IL6) and fibroblast activation protein, was identified that significantly correlated with IL23A expression and were also enriched in CD patients. This work supports the hypothesis that a subgroup of IBD patients may possess a pathogenic myeloid-stromal cell circuit involving OSM as identified in recent landmark studies (West et al., 2017; Smillie et al., 2019).
Over the past decade, there has been an increased appetite for the capture of different types of omics data from a single sample as it is believed this could provide greater insights into disease biology. This multi-omics revolution necessitates the combination of various network modelling approaches. Multi-layered networks can be used to integrate the many facets of multi-omics data including the different time scales of biological processes (Hammoud and Kramer, 2020). In recent years, various databases have been developed such as OmniPath (Türei et al., 2021), SignaLink2 (Fazekas et al., 2013), TranscriptomeBrowser (Lepoivre et al., 2012) or ConsensusPathDB (Kamburov et al., 2011), that can be used to generate multi-layered networks to integrate multi-omics data (Santra et al., 2014).
Combining different types of networks together has unravelled important insights into IBD pathogenesis. However, such multi-layered network approaches have largely been performed on a single type of omics data so far i.e., most commonly, gene expression data. This was seen in the earlier landmark study by Jostins et al where GCNs and GRNs were used together as mentioned earlier (Jostins et al., 2012). More recently, Martin et al generated intercellular ligand-receptor networks (a type of PPI network) and GCNs from scRNAseq data obtained from ileal biopsies of patients with ileal CD (Martin et al., 2019). By applying gene co-expression analysis to the scRNAseq data, they first identified a group of cell types which strongly correlated with ileal inflammation in a subset of ileal CD patients and also lack of response to anti-TNF therapy. They termed this group the GIMATS (IgG plasma cells, inflammatory mononuclear phagocytes, activated T cells and stromal cells) module. Next they evaluated intercellular interactions communicating with the GIMATS module by using the scRNAseq data to identify experimentally validated cytokine-cytokine receptor pairs (Ramilowski et al., 2015). This revealed a distinct intercellular network driving the GIMATS module including T cells, mononuclear phagocytes, fibroblasts and endothelial cells.
Cell signalling networks are another important type of multi-layered network consisting of two components: an upstream component which is a directed PPI network containing various intracellular signaling pathways, and a downstream component which is a GRN of transcription factor-target interactions (Csermely et al., 2013). The OmniPath database is particularly useful for generating cell signalling networks as it allows the user to not only access the intracellular PPI network of a cell but also GRNs and even the extracellular ligand-receptor networks from a myriad of databases (Türei et al., 2021). Although examples of cell signalling networks have been limited in IBD thus far, recently we established a novel bioinformatic pipeline termed “iSNP”, to create a UC-specific cell signalling network from patient-derived SNP data (Brooks et al., 2019). In this approach we focused on SNPs located within non-coding regions of the genome, which represent the vast majority of SNPs associated with UC. These non-coding SNPs were annotated to transcription factor binding sites (TFBS) and miRNA-target sites (miRNA-TS) using available databases reporting transcription factor binding profiles and miRNA sequences. Protein-coding genes located within the vicinity of SNP-affected TFBS and those targeted by the SNP-affected miRNA-TS were identified using regulatory interaction data sources. In this way SNP-affected proteins were revealed. Using OmniPath, the first neighbours of these SNP-affected proteins were also pinpointed. Utilising genotyped patient data from an IBD patient cohort in East Anglia in the United Kingdom, we created individual patient-specific cell signalling networks. By applying unsupervised clustering algorithms to these patient-specific cell signalling networks, we revealed that patients clustered into four main groups and identified distinct pathogenic pathways involved in each cluster. Thus, using a novel network biology workflow involving cell signalling networks, we were able to identify distinct regulatory effects of disease-associated non-coding SNPs in subgroups of UC patients.
Despite the recent strides made in unravelling IBD pathogenesis using the aforementioned network biology approaches, there are several challenges that need to be overcome to achieve the goal of precision medicine in IBD (Fiocchi and Iliopoulos, 2021).
First and foremost, research efforts must focus on acquiring patient-specific data from a variety of relevant data sources that could provide a more holistic picture of the disease biology of individual patients. In the past, network biology models used only one or two dimensions of data such as PPI networks, sets of DEGs, or transcriptomic information to reconstruct biological networks (Seyed Tabib et al., 2020). However, recent breakthroughs made in cancer demonstrate that multi-layered networks which incorporate various omics data are likely to yield more powerful and translatable insights for complex diseases (Du and Elemento, 2015). There is a paucity of such approaches in IBD to date, although the aforementioned studies by Jostins et al. (2012), Martin et al. (2019) and Brooks et al. (2019) demonstrate the potential of such methods. In addition, despite the exponential increase in transcriptomics and metatranscriptomics studies in IBD in the past decade, such datasets are often limited by low patient numbers. Recently, a novel meta-analysis framework for transcriptome and metatranscriptome data in IBD has been introduced, called the IBD Transcriptome and Metatranscriptome Meta-Analysis (TaMMA) platform (Massimino et al., 2021). The TaMMA platform collates and integrates transcriptomics (and metatranscriptomics) data from multiple IBD patient cohorts using a standardised pipeline that corrects batch effects and performs differential analysis of the data. This significantly increases the sample size and statistical power for downstream analysis (Modos et al., 2021). This platform, which is available as a user-friendly, open-source web application, can maximise the utility of existing transcriptomics and metatranscriptomics datasets generated from various research centers across the world. Such meta-analysis frameworks could be a powerful way for analysing other omic layers too in the future.
In IBD, it is particularly important to consider the effects of the gut luminal microenvironment which contains bacterial cells up to 1013 in number and their repertoire of metabolite products on the host. However, this is an extremely complex ecosystem to model. Adding to this complexity is the dynamic nature of the gut microbiota, which can be affected by the age of the individual and environmental exposures such as diet and drugs. The development of novel genome-scale metabolic models as mentioned earlier as well as strain-specific metabolomics have potential to enhance our understanding of the IBD metabolome and the intestinal microflora (Han et al., 2021; Heinken et al., 2021). In addition to the metabolome, another data source for integration in IBD that should be strongly considered is histopathological data. The importance of integrating histopathological data for precision medicine has been clearly demonstrated in colorectal cancer (CRC) (Thomas et al., 2019). Over the past couple of decades it has been revealed that the type, density, and location of immune cells (i.e., the “immune contexture”) within CRC tissues are a better prognostic tool than the traditional Dukes staging for predicting CRC survival and recurrence (Galon et al., 2006; Fridman et al., 2012). Subsequently, transcriptomic data from CRC tissues were integrated with this histological classification, to shed light on the remarkable immunogenomic heterogeneity of CRC (Becht et al., 2016). Similar efforts to amalgamate histopathological and genomic data have thus far been scarce in IBD, but appear to be on the horizon: Friedrich et al have recently revealed distinct pathotypes in IBD that are associated with non-response to several therapies using such an approach (Friedrich et al., 2021). Furthermore, to fully realise the potential of molecular, metabolomic, and histopathological data, it is integral that they are matched with pertinent clinical metadata i.e., information of the patients’ treatment(s), age, comorbidities etc (Ahmed, 2020). This has been lacking in many previous studies (Olivera et al., 2019). However, acquiring good quality clinical metadata is challenging due to the use of paper medical records by many hospitals. Also despite the increasing use of electronic medical records (EMRs), hospitals seldom use the same EMR software resulting in interoperability issues and fragmentation of data (Warren et al., 2019). Nevertheless, artificial intelligence, including natural language processing (NLP), may help transform the extraction of clinical metadata from EMRs in the coming decades. Such big data methods can help to better understand and personalise network biology models and can also be used for validation of findings (Olivera et al., 2019; Seyed Tabib et al., 2020) (Figure 6).
FIGURE 6. Future perspectives of using network biology and network based modeling in IBD research. From the large amount of omics datasets (genomics, transcriptomics, metabolomics, metagenomics), various interaction networks can be used to develop sophisticated network models, ideally in a multi-layered fashion. Adding granularity with patient metadata from large databases can help to validate these models and will result in better understanding of IBD pathogenesis, novel/personalised therapeutic strategies, and clinical decision-driving signatures.
Another strategy that may yield important insights into IBD disease pathogenesis is to evaluate omics data in IBD in the context of other comorbid disorders. Patients with IBD are more likely to develop other disorders with a significant immune component such as rheumatoid arthritis (RA), psoriasis, asthma and colorectal cancer (García et al., 2020). These disorders share underlying genetic risk factors and environmental exposures which can result in similarities in the immune pathways and cytokines driving inflammatory responses in these conditions (Moni and Liò, 2015). This is reflected in the fact that biologic agents targeting TNFα are effective in IBD as well as inflammatory arthritides such as RA, axial spondyloarthritis and psoriatic arthritis (Schett et al., 2021). However, thus far, there has been limited work which has evaluated multi-omics data between comorbid disease networks involving IBD. Nevertheless, bioinformatics tools have recently been generated that could be readily utilised to generate comorbidity networks from published multi-omics datasets for estimating disease comorbidity risks and patient stratification (Moni and Liò, 2015; Xiao et al., 2018).
One of the major challenges that will need to be addressed in all such approaches is how to integrate the vast amounts of multi-omics data generated from disparate sources to reveal clinically meaningful insights in IBD. Integrating genomics, transcriptomics (ideally single-cell transcriptomics), epigenomics, metabolomics, and metagenomic datasets of patients together with robust clinical meta-data and histopathological data over time will be critical for realising the goal of precision medicine in IBD (Figure 6). However, there is often a low degree of agreement between networks generated from different omics datasets, making it difficult to identify salient features that are shared between them. Therefore, more advanced data integration and analysis methods for multi-omics data are necessary.
Recently, a number of novel multi-omic data integration tools have been developed but their use has not yet penetrated the field of IBD. These include early data integration (i.e., combining all datasets into a single dataset first before developing the model) and late data integration (i.e., generating individual models from each dataset first and then finally integrating the models together) methods. Early examples of the former approach which create an aggregative layer within a multiplex network include iCluster (Shen et al., 2009), a joint latent variable model, and a similarity network fusion method by Wang et al. (2014). A weighted network fusion method has also been developed which incorporates the relative weight or importance of each layer when integrating omics layers (Angione et al., 2016). At present, one of the most common methods of omics integration is an early integration method called non-negative matrix factorisation as implemented in the MOFA package (Argelaguet et al., 2018). In short, in this method a large matrix is first constructed where the columns are the patient samples and the rows are the measurements from the various types of omics data. This large matrix is then deconvoluted into two matrices. The first matrix contains the various omics measurements as rows and factors as columns, with cells referring to the contribution of each omics measurement to a factor. Here, factors represent biological information such as signalling pathways or metabolomic circuits. The second matrix is composed of samples as columns and factors as rows, with cells referring to each factor’s value for a sample. Each factor can be traced back to the input measurements whether they are genomics, transcriptomics or metagenomic inputs. This can be used to uncover hidden interactions between various modalities of measurements. Clustering the samples based on the factors helps to reduce the noise that naturally arises when combining disparate data types. This approach was shown to identify major causes of disease heterogeneity in chronic lymphocytic leukaemia (Argelaguet et al., 2018). Late data integration methods have also revealed important insights into disease pathogenesis. An example is the COSMOS tool, in which multiple networks generated from different omics data are integrated using causal reasoning (Dugourd et al., 2021). In this paper, the investigators demonstrated the capability of COSMOS to integrate PPI, GRN and two different metabolic networks from transcriptomics, phosphoproteomics, and metabolomics data in clear cell renal cell carcinoma. Similar non-matrix-based omics methods were used in bacteria such as the MORA approach, which integrates various layers of omics data (transcriptomic, proteomics, metabolomics, genomics) to identify the affected pathways (Bardozzo et al., 2018). This method used mutual synchronisation of binarised omics measurements rather than a matrix deconvolution approach to identify affected pathways.
Recently, Malod-Dognin et al described the application of a novel multi-omics data integration and analysis framework in four different cancer types based on a machine learning technique called non-negative matrix tri-factorisation (NMTF) (Malod-Dognin et al., 2019). For each cancer type, using this approach they were able to integrate three different types of omics tissue-specific molecular interaction networks (i.e., PPI, GCN and gene interaction network) into a single, unified representation of a tissue-specific cell, which they termed “iCell.” The NMTF algorithm is an intermediate data integration method i.e., it integrates the information from the various models (networks) and source data (gene expression) giving back valuable information such as clustering of genes or local rewiring of various genes in many networks. It uses an already filtered network for this purpose. The method deconvolutes the adjacency matrices of networks into three smaller matrices per network. Two of the matrices are the same in the various networks and they are transpose of each other that capture sample-specific features, whilst the third matrix displays network-specific features. This was shown to overcome the problems associated with early data integration and late data integration approaches that have been used previously, leading to more accurate predictions. To further analyse these integrated networks, they then utilised graphlets as a more sensitive method for evaluating network topology (Przulj, 2007; Yaveroğlu et al., 2014). The distribution of graphlets can act as a fingerprint for a network, allowing comparisons to be made between networks (Sarajlić et al., 2016). Overall, this innovative integrative and analytical approach was shown to better detect the functional organisation of cancer cells than from a single omics layer and it identified 63 new cancer-related genes.
Network biology approaches have provided unique insights into the pathogenesis of IBD which could not have been ascertained through simple evaluation of molecular data. With the recent establishment of several large biorepositories for IBD and the advent of next-generation sequencing, we will soon be able to access high-quality omics patient data with sufficient power to tackle some of the key unanswered questions in the field. It is important that this data is complemented with other relevant data sources, especially reliable clinical metadata. Network biology will be critical for integrating the resulting multifaceted datasets to generate clinically translatable end-points. In recent years, multi-omics integrative methods have been developed and then applied successfully in the field of cancer, but have been limited in IBD and other complex diseases. Further research is required to develop more robust integrative and analytical network biology approaches for various types of omics data. Such efforts will allow us to fully harness the potential of multi-omic patient datasets to provide deeper insights into the pathogenesis of IBD and achieve the goal of precision medicine in this complex disease.
Node/vertex: A point in a network. In biological networks it is usually a gene or protein.
Link/edge: The interaction between nodes. In network biology, it can be a physical interaction such as an enzymatic reaction or similarity e.g. correlation between the expression of two genes.
Directed network: The network’s edges are directed meaning from node “v” to node “u” is not the same as from node “u” to node “v”.
Weighted network: The edges of the network have weight. In network biology, weights often represent the number of interactions between cells or the strength of the interaction between proteins which can depend on the concentration or measured amount of the proteins (in case of proteomic analysis) or the amount of the genes encoding the protein (in case of transcriptomics analysis).
Signed interaction: It is a type of weight of the network, which informs whether the interaction is positive or negative. A negative sign means the interaction is inhibitory, whereas a positive sign means it is excitatory.
Degree: The number of neighbouring nodes that a particular node connects to in a network.
Hub: A node with high degree.
Path: The set of edges connecting any two nodes.
Shortest path: The path between two nodes which involves the least number of edges.
Betweenness centrality: The number of shortest paths which go through a given node or edge. It is often normalised by the number of all possible shortest paths between all nodes.
Bottleneck: A node with high betweenness centrality but low degree. These are critical nodes in the network because a high amount of information goes through them.
Module/community: A set of nodes in a network which are interacting with each other more strongly than with other nodes outside the module.
Scale free network: A network which has a degree (k) distribution of P(k) = k−γ. In practice it means that the network has a low number of high degree nodes whilst most of the nodes have a really low degree. Most biological networks closely resemble a scale free distribution.
Adjacency matrix: A matrix which models the network where columns and rows represent nodes and each value is an edge. If the network is undirected, then the adjacency matrix is symmetric, whereas in directed networks the adjacency matrix is asymmetric. If the network is not weighted then the values in the adjacency matrix are 1. However, in a weighted network the values are the weights.
Gene interaction network: A network where the edges represent whether the mutations of the genes together influence a phenotype e.g. synthetic lethality.
Matrix deconvolution: Representing the matrix with multiple smaller order matrices.
Causal reasoning: Finding the best possible path in a network where the signs match with the output of the network.
Graphlet: A local unique (non-isomorphic) structure of a network.
Network motif: An overrepresented local structure of a network (for instance a common graphlet).
JT and DM wrote and reviewed/edited the article before submission. JB-W and TK made substantial contributions to the discussion of content and reviewed/edited the article before submission.
JT is an Academic Clinical Fellow supported by the National Institute of Health Research (NIHR) and has been awarded funding through the Health Education England (HEE) Genomics Education Programme. DM and TK are supported by the Earlham Institute (Norwich, United Kingdom) in partnership with the Quadram Institute (Norwich, United Kingdom) and strategically supported by a United Kingdom Research and Innovation (UKRI) Biotechnological and Biosciences Research Council (BBSRC) Core Strategic Programme Grant for Genomes to Food Security (BB/CSP1720/1) and its constituent work packages, BBS/E/T/000PR9819 and BBS/E/T/000PR9817, as well as a BBSRC ISP grant for Gut Microbes and Health (BB/R012490/1) and its constituent projects, BBS/E/F/000PR10353 and BBS/E/F/000PR10355.
The authors declare that the research was conducted in the absence of any commercial or financial relationships that could be construed as a potential conflict of interest.
All claims expressed in this article are solely those of the authors and do not necessarily represent those of their affiliated organizations, or those of the publisher, the editors and the reviewers. Any product that may be evaluated in this article, or claim that may be made by its manufacturer, is not guaranteed or endorsed by the publisher.
Ahmed, Z. (2020). Practicing Precision Medicine with Intelligently Integrative Clinical and Multi-Omics Data Analysis. Hum. Genomics 14, 35. doi:10.1186/s40246-020-00287-z
Ahn, A. C., Tewari, M., Poon, C.-S., and Phillips, R. S. (2006). The Limits of Reductionism in Medicine: Could Systems Biology Offer an Alternative? Plos Med. 3, e208. doi:10.1371/journal.pmed.0030208
Altaf-Ul-Amin, M., Wada, M., and Kanaya, S. (2012). Partitioning a PPI Network into Overlapping Modules Constrained by High-Density and Periphery Tracking. ISRN Biomathematics 2012, 1–11. doi:10.5402/2012/726429
Anand, S., Mukherjee, K., and Padmanabhan, P. (2020). An Insight to Flux-Balance Analysis for Biochemical Networks. Biotechnol. Genet. Eng. Rev. 36, 32–55. doi:10.1080/02648725.2020.1847440
Angione, C., Conway, M., and Lió, P. (2016). Multiplex Methods Provide Effective Integration of Multi-Omic Data in Genome-Scale Models. BMC Bioinformatics 17, 83. doi:10.1186/s12859-016-0912-1
Argelaguet, R., Velten, B., Arnol, D., Dietrich, S., Zenz, T., Marioni, J. C., et al. (2018). Multi‐Omics Factor Analysis-A Framework for Unsupervised Integration of Multi‐omics Data Sets. Mol. Syst. Biol. 14, e8124. doi:10.15252/msb.20178124
Armingol, E., Officer, A., Harismendy, O., and Lewis, N. E. (2021). Deciphering Cell-Cell Interactions and Communication from Gene Expression. Nat. Rev. Genet. 22, 71–88. doi:10.1038/s41576-020-00292-x
Aschenbrenner, D., Quaranta, M., Banerjee, S., Ilott, N., Jansen, J., Steere, B., et al. (2021). Deconvolution of Monocyte Responses in Inflammatory Bowel Disease Reveals an IL-1 Cytokine Network that Regulates IL-23 in Genetic and Acquired IL-10 Resistance. Gut 70, 1023–1036. doi:10.1136/gutjnl-2020-321731
Barabási, A.-L., and Oltvai, Z. N. (2004). Network Biology: Understanding the Cell's Functional Organization. Nat. Rev. Genet. 5, 101–113. doi:10.1038/nrg1272
Bardozzo, F., Lió, P., and Tagliaferri, R. (2018). A Study on Multi-Omic Oscillations in Escherichia coli Metabolic Networks. BMC Bioinformatics 19, 194. doi:10.1186/s12859-018-2175-5
Baumgart, D. C., and Carding, S. R. (2007). Inflammatory Bowel Disease: Cause and Immunobiology. Lancet 369, 1627–1640. doi:10.1016/S0140-6736(07)60750-8
Bayes, T. (1763). LII. An Essay towards Solving a Problem in the Doctrine of Chances. By the Late Rev. Mr. Bayes, F. R. S. Communicated by Mr. Price, in a Letter to John Canton, A. M. F. R. S. Phil. Trans. R. Soc. 53, 370–418. doi:10.1098/rstl.1763.0053
Becht, E., de Reyniès, A., Giraldo, N. A., Pilati, C., Buttard, B., Lacroix, L., et al. (2016). Immune and Stromal Classification of Colorectal Cancer Is Associated with Molecular Subtypes and Relevant for Precision Immunotherapy. Clin. Cancer Res. 22, 4057–4066. doi:10.1158/1078-0432.CCR-15-2879
Biasci, D., Lee, J. C., Noor, N. M., Pombal, D. R., Hou, M., Lewis, N., et al. (2019). A Blood-Based Prognostic Biomarker in IBD. Gut 68, 1386–1395. doi:10.1136/gutjnl-2019-318343
Borg-Bartolo, S. P., Boyapati, R. K., Satsangi, J., and Kalla, R. (2020). Precision Medicine in Inflammatory Bowel Disease: Concept, Progress and Challenges. F1000Res 9, 54. doi:10.12688/f1000research.20928.1
Brooks, J., Modos, D., Sudhakar, P., Fazekas, D., Zoufir, A., Kapuy, O., et al. (2019). A Systems Genomics Approach to Uncover Patient-specific Pathogenic Pathways and Proteins in a Complex Disease. BioRxiv. doi:10.1101/692269
Cader, M. Z., and Kaser, A. (2013). Recent Advances in Inflammatory Bowel Disease: Mucosal Immune Cells in Intestinal Inflammation. Gut 62, 1653–1664. doi:10.1136/gutjnl-2012-303955
Charitou, T., Bryan, K., and Lynn, D. J. (2016). Using Biological Networks to Integrate, Visualize and Analyze Genomics Data. Genet. Sel. Evol. 48, 27. doi:10.1186/s12711-016-0205-1
Chatr-Aryamontri, A., Breitkreutz, B.-J., Oughtred, R., Boucher, L., Heinicke, S., Chen, D., et al. (2015). The BioGRID Interaction Database: 2015 Update. Nucleic Acids Res. 43, D470–D478. doi:10.1093/nar/gku1204
Chen, J., Mamidipalli, S., and Huan, T. (2009). HAPPI: an Online Database of Comprehensive Human Annotated and Predicted Protein Interactions. BMC Genomics 10, S16. doi:10.1186/1471-2164-10-S1-S16
Chen, J. Y., Pandey, R., and Nguyen, T. M. (2017). HAPPI-2: a Comprehensive and High-Quality Map of Human Annotated and Predicted Protein Interactions. BMC Genomics 18, 182. doi:10.1186/s12864-017-3512-1
Cosnes, J., Gower–Rousseau, C., Seksik, P., and Cortot, A. (2011). Epidemiology and Natural History of Inflammatory Bowel Diseases. Gastroenterology 140, 1785–1794. doi:10.1053/j.gastro.2011.01.055
Cowen, L., Ideker, T., Raphael, B. J., and Sharan, R. (2017). Network Propagation: a Universal Amplifier of Genetic Associations. Nat. Rev. Genet. 18, 551–562. doi:10.1038/nrg.2017.38
Csermely, P., Korcsmáros, T., Kiss, H. J. M., London, G., and Nussinov, R. (2013). Structure and Dynamics of Molecular Networks: A Novel Paradigm of Drug Discovery. Pharmacol. Ther. 138, 333–408. doi:10.1016/j.pharmthera.2013.01.016
Denson, L. A., Curran, M., McGovern, D. P. B., Koltun, W. A., Duerr, R. H., Kim, S. C., et al. (2019). Challenges in IBD Research: Precision Medicine. Inflamm. Bowel Dis. 25, S31–S39. doi:10.1093/ibd/izz078
Du, W., and Elemento, O. (2015). Cancer Systems Biology: Embracing Complexity to Develop Better Anticancer Therapeutic Strategies. Oncogene 34, 3215–3225. doi:10.1038/onc.2014.291
Duboc, H., Rajca, S., Rainteau, D., Benarous, D., Maubert, M.-A., Quervain, E., et al. (2013). Connecting Dysbiosis, Bile-Acid Dysmetabolism and Gut Inflammation in Inflammatory Bowel Diseases. Gut 62, 531–539. doi:10.1136/gutjnl-2012-302578
Duerr, R. H., Taylor, K. D., Brant, S. R., Rioux, J. D., Silverberg, M. S., Daly, M. J., et al. (2006). A Genome-wide Association Study Identifies IL23R as an Inflammatory Bowel Disease Gene. Science 314, 1461–1463. doi:10.1126/science.1135245
Dugourd, A., Kuppe, C., Sciacovelli, M., Gjerga, E., Gabor, A., Emdal, K. B., et al. (2021). Causal Integration of Multi‐omics Data with Prior Knowledge to Generate Mechanistic Hypotheses. Mol. Syst. Biol. 17, e9730. doi:10.15252/msb.20209730
Efremova, M., Vento-Tormo, M., Teichmann, S. A., and Vento-Tormo, R. (2020). CellPhoneDB: Inferring Cell-Cell Communication from Combined Expression of Multi-Subunit Ligand-Receptor Complexes. Nat. Protoc. 15, 1484–1506. doi:10.1038/s41596-020-0292-x
Eguchi, R., Karim, M. B., Hu, P., Sato, T., Ono, N., Kanaya, S., et al. (2018). An Integrative Network-Based Approach to Identify Novel Disease Genes and Pathways: a Case Study in the Context of Inflammatory Bowel Disease. BMC Bioinformatics 19, 264. doi:10.1186/s12859-018-2251-x
Fazekas, D., Koltai, M., Türei, D., Módos, D., Pálfy, M., Dúl, Z., et al. (2013). SignaLink 2 - a Signaling Pathway Resource with Multi-Layered Regulatory Networks. BMC Syst. Biol. 7, 7. doi:10.1186/1752-0509-7-7
Fiocchi, C., and Iliopoulos, D. (2021). IBD Systems Biology Is Here to Stay. Inflamm. Bowel Dis. 27, 760–770. doi:10.1093/ibd/izaa343
Fridman, W. H., Pagès, F., Sautès-Fridman, C., and Galon, J. (2012). The Immune Contexture in Human Tumours: Impact on Clinical Outcome. Nat. Rev. Cancer 12, 298–306. doi:10.1038/nrc3245
Friedman, N., Linial, M., Nachman, I., and Pe'er, D. (2000). Using Bayesian Networks to Analyze Expression Data. J. Comput. Biol. 7, 601–620. doi:10.1089/106652700750050961
Friedrich, M., Pohin, M., Jackson, M. A., Korsunsky, I., Bullers, S., Rue-Albrecht, K., et al. (2021). IL-1-driven Stromal-Neutrophil Interaction in Deep Ulcers Defines a Pathotype of Therapy Non-responsive Inflammatory Bowel Disease. BioRxiv. doi:10.1101/2021.02.05.429804
Galon, J., Costes, A., Sanchez-Cabo, F., Kirilovsky, A., Mlecnik, B., Lagorce-Pagès, C., et al. (2006). Type, Density, and Location of Immune Cells within Human Colorectal Tumors Predict Clinical Outcome. Science 313, 1960–1964. doi:10.1126/science.1129139
García, M. J., Pascual, M., Del Pozo, C., Díaz-González, A., Castro, B., Rasines, L., et al. (2020). Impact of Immune-Mediated Diseases in Inflammatory Bowel Disease and Implications in Therapeutic Approach. Sci. Rep. 10, 10731. doi:10.1038/s41598-020-67710-2
Garcia-Alonso, L., Iorio, F., Matchan, A., Fonseca, N., Jaaks, P., Peat, G., et al. (2018). Transcription Factor Activities Enhance Markers of Drug Sensitivity in Cancer. Cancer Res. 78, 769–780. doi:10.1158/0008-5472.CAN-17-1679
GBD 2017 Inflammatory Bowel Disease Collaborators (2020). The Global, Regional, and National burden of Inflammatory Bowel Disease in 195 Countries and Territories, 1990-2017: a Systematic Analysis for the Global Burden of Disease Study 2017. Lancet Gastroenterol. Hepatol. 5, 17–30. doi:10.1016/S2468-1253(19)30333-4
Green, S., Şerban, M., Scholl, R., Jones, N., Brigandt, I., and Bechtel, W. (2017). Network Analyses in Systems Biology: New Strategies for Dealing with Biological Complexity. Synthese 195, 1751–1777. doi:10.1007/s11229-016-1307-6
Hammoud, Z., and Kramer, F. (2020). Multilayer Networks: Aspects, Implementations, and Application in Biomedicine. Big Data Anal. 5, 2. doi:10.1186/s41044-020-00046-0
Han, H., Shim, H., Shin, D., Shim, J. E., Ko, Y., Shin, J., et al. (2015). TRRUST: a Reference Database of Human Transcriptional Regulatory Interactions. Sci. Rep. 5, 11432. doi:10.1038/srep11432
Han, S., Van Treuren, W., Fischer, C. R., Merrill, B. D., DeFelice, B. C., Sanchez, J. M., et al. (2021). A Metabolomics Pipeline for the Mechanistic Interrogation of the Gut Microbiome. Nature 595, 415–420. doi:10.1038/s41586-021-03707-9
Hang, S., Paik, D., Yao, L., Kim, E., Trinath, J., Lu, J., et al. (2019). Bile Acid Metabolites Control TH17 and Treg Cell Differentiation. Nature 576, 143–148. doi:10.1038/s41586-019-1785-z
Heinken, A., Hertel, J., and Thiele, I. (2021). Metabolic Modelling Reveals Broad Changes in Gut Microbial Metabolism in Inflammatory Bowel Disease Patients with Dysbiosis. NPJ Syst. Biol. Appl. 7, 19. doi:10.1038/s41540-021-00178-6
Heinken, A., Ravcheev, D. A., Baldini, F., Heirendt, L., Fleming, R. M. T., and Thiele, I. (2019). Systematic Assessment of Secondary Bile Acid Metabolism in Gut Microbes Reveals Distinct Metabolic Capabilities in Inflammatory Bowel Disease. Microbiome 7, 75. doi:10.1186/s40168-019-0689-3
Heirendt, L., Arreckx, S., Pfau, T., Mendoza, S. N., Richelle, A., Heinken, A., et al. (2019). Creation and Analysis of Biochemical Constraint-Based Models Using the COBRA Toolbox v.3.0. Nat. Protoc. 14, 639–702. doi:10.1038/s41596-018-0098-2
Hermjakob, H., Montecchi-Palazzi, L., Lewington, C., Mudali, S., Kerrien, S., Orchard, S., et al. (2004). IntAct: an Open Source Molecular Interaction Database. Nucleic Acids Res. 32, 452D–455D. doi:10.1093/nar/gkh052
Hong, J., Brandt, N., Abdul-Rahman, F., Yang, A., Hughes, T., and Gresham, D. (2018). An Incoherent Feedforward Loop Facilitates Adaptive Tuning of Gene Expression. eLife 7, e32323. doi:10.7554/eLife.32323
Huang, J. K., Carlin, D. E., Yu, M. K., Zhang, W., Kreisberg, J. F., Tamayo, P., et al. (2018). Systematic Evaluation of Molecular Networks for Discovery of Disease Genes. Cel Syst. 6, 484–495. doi:10.1016/j.cels.2018.03.001
Huttlin, E. L., Bruckner, R. J., Navarrete-Perea, J., Cannon, J. R., Baltier, K., Gebreab, F., et al. (2021). Dual Proteome-Scale Networks Reveal Cell-specific Remodeling of the Human Interactome. Cell 184, 3022–3040. doi:10.1016/j.cell.2021.04.011
Imhann, F., Van der Velde, K. J., Barbieri, R., Alberts, R., Voskuil, M. D., Vich Vila, A., et al. (2019). The 1000IBD Project: Multi-Omics Data of 1000 Inflammatory Bowel Disease Patients; Data Release 1. BMC Gastroenterol. 19, 5. doi:10.1186/s12876-018-0917-5
Jansma, J., and El Aidy, S. (2021). Understanding the Host-Microbe Interactions Using Metabolic Modeling. Microbiome 9, 16. doi:10.1186/s40168-020-00955-1
Jassal, B., Matthews, L., Viteri, G., Gong, C., Lorente, P., Fabregat, A., et al. (2020). The Reactome Pathway Knowledgebase. Nucleic Acids Res. 48, D498–D503. doi:10.1093/nar/gkz1031
Jostins, L., Ripke, S., Weersma, R. K., Duerr, R. H., McGovern, D. P., Hui, K. Y., et al. (2012). Host-microbe Interactions Have Shaped the Genetic Architecture of Inflammatory Bowel Disease. Nature 491, 119–124. doi:10.1038/nature11582
Kamburov, A., Pentchev, K., Galicka, H., Wierling, C., Lehrach, H., and Herwig, R. (2011). ConsensusPathDB: toward a More Complete Picture of Cell Biology. Nucleic Acids Res. 39, D712–D717. doi:10.1093/nar/gkq1156
Kerrien, S., Aranda, B., Breuza, L., Bridge, A., Broackes-Carter, F., Chen, C., et al. (2012). The IntAct Molecular Interaction Database in 2012. Nucleic Acids Res. 40, D841–D846. doi:10.1093/nar/gkr1088
Kirouac, D. C., Du, J. Y., Lahdenranta, J., Overland, R., Yarar, D., Paragas, V., et al. (2013). Computational Modeling of ERBB2 -Amplified Breast Cancer Identifies Combined ErbB2/3 Blockade as Superior to the Combination of MEK and AKT Inhibitors. Sci. Signal. 6, ra68. doi:10.1126/scisignal.2004008
Knecht, C., Fretter, C., Rosenstiel, P., Krawczak, M., and Hütt, M.-T. (2016). Distinct Metabolic Network States Manifest in the Gene Expression Profiles of Pediatric Inflammatory Bowel Disease Patients and Controls. Sci. Rep. 6, 32584. doi:10.1038/srep32584
König, M., Bulik, S., and Holzhütter, H.-G. (2012). Quantifying the Contribution of the Liver to Glucose Homeostasis: a Detailed Kinetic Model of Human Hepatic Glucose Metabolism. Plos Comput. Biol. 8, e1002577. doi:10.1371/journal.pcbi.1002577
Korcsmaros, T., Schneider, M. V., and Superti-Furga, G. (2017). Next Generation of Network Medicine: Interdisciplinary Signaling Approaches. Integr. Biol. (Camb) 9, 97–108. doi:10.1039/c6ib00215c
Kosti, I., Jain, N., Aran, D., Butte, A. J., and Sirota, M. (2016). Cross-tissue Analysis of Gene and Protein Expression in Normal and Cancer Tissues. Sci. Rep. 6, 24799. doi:10.1038/srep24799
Koutrouli, M., Karatzas, E., Paez-Espino, D., and Pavlopoulos, G. A. (2020). A Guide to Conquer the Biological Network Era Using Graph Theory. Front. Bioeng. Biotechnol. 8, 34. doi:10.3389/fbioe.2020.00034
Kutmon, M., Riutta, A., Nunes, N., Hanspers, K., Willighagen, E. L., Bohler, A., et al. (2016). WikiPathways: Capturing the Full Diversity of Pathway Knowledge. Nucleic Acids Res. 44, D488–D494. doi:10.1093/nar/gkv1024
Langfelder, P., and Horvath, S. (2008). WGCNA: an R Package for Weighted Correlation Network Analysis. BMC Bioinformatics 9, 559. doi:10.1186/1471-2105-9-559
Lee, M. J., Ye, A. S., Gardino, A. K., Heijink, A. M., Sorger, P. K., MacBeath, G., et al. (2012). Sequential Application of Anticancer Drugs Enhances Cell Death by Rewiring Apoptotic Signaling Networks. Cell 149, 780–794. doi:10.1016/j.cell.2012.03.031
Lennard-Jones, J. E. (1989). Classification of Inflammatory Bowel Disease. Scand. J. Gastroenterol. 24, 2–6. doi:10.3109/00365528909091339
Lepoivre, C., Bergon, A., Lopez, F., Perumal, N. B., Nguyen, C., Imbert, J., et al. (2012). TranscriptomeBrowser 3.0: Introducing a New Compendium of Molecular Interactions and a New Visualization Tool for the Study of Gene Regulatory Networks. BMC Bioinformatics 13, 19. doi:10.1186/1471-2105-13-19
Licata, L., Lo Surdo, P., Iannuccelli, M., Palma, A., Micarelli, E., Perfetto, L., et al. (2020). SIGNOR 2.0, the SIGnaling Network Open Resource 2.0: 2019 Update. Nucleic Acids Res. 48, D504–D510. doi:10.1093/nar/gkz949
Luck, K., Kim, D.-K., Lambourne, L., Spirohn, K., Begg, B. E., Bian, W., et al. (2020). A Reference Map of the Human Binary Protein Interactome. Nature 580, 402–408. doi:10.1038/s41586-020-2188-x
Lukassen, S., Chua, R. L., Trefzer, T., Kahn, N. C., Schneider, M. A., Muley, T., et al. (2020). SARS-CoV-2 Receptor ACE2 and TMPRSS2 Are Primarily Expressed in Bronchial Transient Secretory Cells. EMBO J. 39, e105114. doi:10.15252/embj.2010511410.15252/embj.2020105114
Magnúsdóttir, S., Heinken, A., Kutt, L., Ravcheev, D. A., Bauer, E., Noronha, A., et al. (2017). Generation of Genome-Scale Metabolic Reconstructions for 773 Members of the Human Gut Microbiota. Nat. Biotechnol. 35, 81–89. doi:10.1038/nbt.3703
Malod-Dognin, N., Petschnigg, J., Windels, S. F. L., Povh, J., Hemingway, H., Ketteler, R., et al. (2019). Towards a Data-Integrated Cell. Nat. Commun. 10, 805. doi:10.1038/s41467-019-08797-8
Maloy, K. J., and Kullberg, M. C. (2008). IL-23 and Th17 Cytokines in Intestinal Homeostasis. Mucosal Immunol. 1, 339–349. doi:10.1038/mi.2008.28
Martens, M., Ammar, A., Riutta, A., Waagmeester, A., Slenter, D. N., Hanspers, K., et al. (2021). WikiPathways: Connecting Communities. Nucleic Acids Res. 49, D613–D621. doi:10.1093/nar/gkaa1024
Martin, J. C., Chang, C., Boschetti, G., Ungaro, R., Giri, M., Grout, J. A., et al. (2019). Single-Cell Analysis of Crohn's Disease Lesions Identifies a Pathogenic Cellular Module Associated with Resistance to Anti-TNF Therapy. Cell 178, 1493–1508. doi:10.1016/j.cell.2019.08.008
Massimino, L., Lamparelli, L. A., Houshyar, Y., D’Alessio, S., Peyrin-Biroulet, L., Vetrano, S., et al. (2021). The Inflammatory Bowel Disease Transcriptome and Metatranscriptome Meta-Analysis (IBD TaMMA) Framework. Nat. Comput. Sci. 1, 511–515. doi:10.1038/s43588-021-00114-y
Meskó, B., and Görög, M. (2020). A Short Guide for Medical Professionals in the Era of Artificial Intelligence. Npj Digit. Med. 3, 126. doi:10.1038/s41746-020-00333-z
Milo, R., Shen-Orr, S., Itzkovitz, S., Kashtan, N., Chklovskii, D., and Alon, U. (2002). Network Motifs: Simple Building Blocks of Complex Networks. Science 298, 824–827. doi:10.1126/science.298.5594.824
Módos, D., Bulusu, K. C., Fazekas, D., Kubisch, J., Brooks, J., Marczell, I., et al. (2017). Neighbours of Cancer-Related Proteins Have Key Influence on Pathogenesis and Could Increase the Drug Target Space for Anticancer Therapies. NPJ Syst. Biol. Appl. 3, 2. doi:10.1038/s41540-017-0003-6
Modos, D., Thomas, J. P., and Korcsmaros, T. (2021). A Handy Meta-Analysis Tool for IBD Research. Nat. Comput. Sci. 1, 571–572. doi:10.1038/s43588-021-00124-w
Moni, M. A., and Liò, P. (2015). How to Build Personalized Multi-Omics Comorbidity Profiles. Front. Cel Dev. Biol. 3, 28. doi:10.3389/fcell.2015.00028
Noor, N. M., Verstockt, B., Parkes, M., and Lee, J. C. (2020). Personalised Medicine in Crohn's Disease. Lancet Gastroenterol. Hepatol. 5, 80–92. doi:10.1016/S2468-1253(19)30340-1
Olivera, P., Danese, S., Jay, N., Natoli, G., and Peyrin-Biroulet, L. (2019). Big Data in IBD: a Look into the Future. Nat. Rev. Gastroenterol. Hepatol. 16, 312–321. doi:10.1038/s41575-019-0102-5
Olsen, J., Gerds, T. A., Seidelin, J. B., Csillag, C., Bjerrum, J. T., Troelsen, J. T., et al. (2009). Diagnosis of Ulcerative Colitis before Onset of Inflammation by Multivariate Modeling of Genome-wide Gene Expression Data. Inflamm. Bowel Dis. 15, 1032–1038. doi:10.1002/ibd.20879
Orth, J. D., Thiele, I., and Palsson, B. Ø. (2010). What Is Flux Balance Analysis? Nat. Biotechnol. 28, 245–248. doi:10.1038/nbt.1614
Parkes, M.IBD BioResource Investigators (2019). IBD BioResource: an Open-Access Platform of 25 000 Patients to Accelerate Research in Crohn's and Colitis. Gut 68, 1537–1540. doi:10.1136/gutjnl-2019-318835
Pavlopoulos, G. A., Secrier, M., Moschopoulos, C. N., Soldatos, T. G., Kossida, S., Aerts, J., et al. (2011). Using Graph Theory to Analyze Biological Networks. BioData Mining 4, 10. doi:10.1186/1756-0381-4-10
Peters, L. A., Perrigoue, J., Mortha, A., Iuga, A., Song, W.-M., Neiman, E. M., et al. (2017). A Functional Genomics Predictive Network Model Identifies Regulators of Inflammatory Bowel Disease. Nat. Genet. 49, 1437–1449. doi:10.1038/ng.3947
Pinchuk, I. V., Beswick, E. J., Saada, J. I., Boya, G., Schmitt, D., Raju, G. S., et al. (2011). Human Colonic Myofibroblasts Promote Expansion of CD4+ CD25high Foxp3+ Regulatory T Cells. Gastroenterology 140, 2019–2030. doi:10.1053/j.gastro.2011.02.059
Piñero, J., Bravo, À., Queralt-Rosinach, N., Gutiérrez-Sacristán, A., Deu-Pons, J., Centeno, E., et al. (2017). DisGeNET: a Comprehensive Platform Integrating Information on Human Disease-Associated Genes and Variants. Nucleic Acids Res. 45, D833–D839. doi:10.1093/nar/gkw943
Przulj, N. (2007). Biological Network Comparison Using Graphlet Degree Distribution. Bioinformatics 23, e177–e183. doi:10.1093/bioinformatics/btl301
Przulj, N., Corneil, D. G., and Jurisica, I. (2006). Efficient Estimation of Graphlet Frequency Distributions in Protein-Protein Interaction Networks. Bioinformatics 22, 974–980. doi:10.1093/bioinformatics/btl030
Przulj, N., Corneil, D. G., and Jurisica, I. (2004). Modeling Interactome: Scale-free or Geometric? Bioinformatics 20, 3508–3515. doi:10.1093/bioinformatics/bth436
Ramilowski, J. A., Goldberg, T., Harshbarger, J., Kloppmann, E., Lizio, M., Satagopam, V. P., et al. (2015). A Draft Network of Ligand-Receptor-Mediated Multicellular Signalling in Human. Nat. Commun. 6, 7866. doi:10.1038/ncomms8866
Santra, T., Kolch, W., and Kholodenko, B. N. (2014). Navigating the Multilayered Organization of Eukaryotic Signaling: a New Trend in Data Integration. Plos Comput. Biol. 10, e1003385. doi:10.1371/journal.pcbi.1003385
Sarajlić, A., Malod-Dognin, N., Yaveroğlu, Ö. N., and Pržulj, N. (2016). Graphlet-based Characterization of Directed Networks. Sci. Rep. 6, 35098. doi:10.1038/srep35098
Schett, G., McInnes, I. B., and Neurath, M. F. (2021). Reframing Immune-Mediated Inflammatory Diseases through Signature Cytokine Hubs. N. Engl. J. Med. 385, 628–639. doi:10.1056/NEJMra1909094
Schlitt, T., and Brazma, A. (2007). Current Approaches to Gene Regulatory Network Modelling. BMC Bioinformatics 8, S9. doi:10.1186/1471-2105-8-S6-S9
Sevilla, J. L., Segura, V., Podhorski, A., Guruceaga, E., Mato, J. M., Martínez-Cruz, L. A., et al. (2005). Correlation between Gene Expression and GO Semantic Similarity. IEEE/ACM Trans. Comput. Biol. Bioinf. 2, 330–338. doi:10.1109/TCBB.2005.50
Seyed Tabib, N. S., Madgwick, M., Sudhakar, P., Verstockt, B., Korcsmaros, T., and Vermeire, S. (2020). Big Data in IBD: Big Progress for Clinical Practice. Gut 69, 1520–1532. doi:10.1136/gutjnl-2019-320065
Shen, R., Olshen, A. B., and Ladanyi, M. (2009). Integrative Clustering of Multiple Genomic Data Types Using a Joint Latent Variable Model with Application to Breast and Lung Cancer Subtype Analysis. Bioinformatics 25, 2906–2912. doi:10.1093/bioinformatics/btp543
Sinha, S. R., Haileselassie, Y., Nguyen, L. P., Tropini, C., Wang, M., Becker, L. S., et al. (2020). Dysbiosis-Induced Secondary Bile Acid Deficiency Promotes Intestinal Inflammation. Cell Host Microbe 27, 659–670. doi:10.1016/j.chom.2020.01.021
Smillie, C. S., Biton, M., Ordovas-Montanes, J., Sullivan, K. M., Burgin, G., Graham, D. B., et al. (2019). Intra and Inter-cellular Rewiring of the Human Colon during Ulcerative Colitis. Cell 178, 714–730. doi:10.1016/j.cell.2019.06.029
Snider, J., Kotlyar, M., Saraon, P., Yao, Z., Jurisica, I., and Stagljar, I. (2015). Fundamentals of Protein Interaction Network Mapping. Mol. Syst. Biol. 11, 848. doi:10.15252/msb.20156351
Sonawane, A. R., Weiss, S. T., Glass, K., and Sharma, A. (2019). Network Medicine in the Age of Biomedical Big Data. Front. Genet. 10, 294. doi:10.3389/fgene.2019.00294
Song, X., Sun, X., Oh, S. F., Wu, M., Zhang, Y., Zheng, W., et al. (2020). Microbial Bile Acid Metabolites Modulate Gut RORγ+ Regulatory T Cell Homeostasis. Nature 577, 410–415. doi:10.1038/s41586-019-1865-0
Spekhorst, L. M., Imhann, F., Festen, E. A., Bodegraven, A. A. v., Boer, N. K. d., Bouma, G., et al. (2017). Cohort Profile: Design and First Results of the Dutch IBD Biobank: a Prospective, Nationwide Biobank of Patients with Inflammatory Bowel Disease. BMJ Open 7, e016695. doi:10.1136/bmjopen-2017-016695
Stark, C., Breitkreutz, B.-J., Reguly, T., Boucher, L., Breitkreutz, A., and Tyers, M. (2006). BioGRID: a General Repository for Interaction Datasets. Nucleic Acids Res. 34, D535–D539. doi:10.1093/nar/gkj109
Sudhakar, P., Machiels, K., Verstockt, B., Korcsmaros, T., and Vermeire, S. (2021). Computational Biology and Machine Learning Approaches to Understand Mechanistic Microbiome-Host Interactions. Front. Microbiol. 12, 618856. doi:10.3389/fmicb.2021.618856
Szklarczyk, D., Gable, A. L., Lyon, D., Junge, A., Wyder, S., Huerta-Cepas, J., et al. (2019). STRING V11: Protein-Protein Association Networks with Increased Coverage, Supporting Functional Discovery in Genome-wide Experimental Datasets. Nucleic Acids Res. 47, D607–D613. doi:10.1093/nar/gky1131
Thiele, I., Swainston, N., Fleming, R. M. T., Hoppe, A., Sahoo, S., Aurich, M. K., et al. (2013). A Community-Driven Global Reconstruction of Human Metabolism. Nat. Biotechnol. 31, 419–425. doi:10.1038/nbt.2488
Thomas, J. P., Divekar, D., Brooks, J., and Watson, A. J. M. (2019). Gut Microbes Drive T-Cell Infiltration into Colorectal Cancers and Influence Prognosis. Gastroenterology 156, 1926–1928. doi:10.1053/j.gastro.2019.03.035
Türei, D., Valdeolivas, A., Gul, L., Palacio‐Escat, N., Klein, M., Ivanova, O., et al. (2021). Integrated Intra‐ and Intercellular Signaling Knowledge for Multicellular Omics Analysis. Mol. Syst. Biol. 17, e9923. doi:10.15252/msb.20209923
van Dam, S., Võsa, U., van der Graaf, A., Franke, L., and de Magalhães, J. P. (2018). Gene Co-expression Analysis for Functional Classification and Gene-Disease Predictions. Brief Bioinform. 19, bbw139–592. doi:10.1093/bib/bbw139
Verstockt, B., Noor, N. M., Marigorta, U. M., Pavlidis, P., Deepak, P., Ungaro, R. C., et al. (2021). Results of the Seventh Scientific Workshop of ECCO: Precision Medicine in IBD-Disease Outcome and Response to Therapy. J. Crohns Colitis 15, 1431–1442. doi:10.1093/ecco-jcc/jjab050
Verstockt, S., De Hertogh, G., Van der Goten, J., Verstockt, B., Vancamelbeke, M., Machiels, K., et al. (2019). Gene and Mirna Regulatory Networks during Different Stages of Crohn's Disease. J. Crohns Colitis 13, 916–930. doi:10.1093/ecco-jcc/jjz007
Vidal, M., Cusick, M. E., and Barabási, A.-L. (2011). Interactome Networks and Human Disease. Cell 144, 986–998. doi:10.1016/j.cell.2011.02.016
Wang, B., Mezlini, A. M., Demir, F., Fiume, M., Tu, Z., Brudno, M., et al. (2014). Similarity Network Fusion for Aggregating Data Types on a Genomic Scale. Nat. Methods 11, 333–337. doi:10.1038/nmeth.2810
Warren, L. R., Clarke, J., Arora, S., and Darzi, A. (2019). Improving Data Sharing between Acute Hospitals in England: an Overview of Health Record System Distribution and Retrospective Observational Analysis of Inter-hospital Transitions of Care. BMJ Open 9, e031637. doi:10.1136/bmjopen-2019-031637
West, N. R., Hegazy, A. N., Hegazy, A. N., Owens, B. M. J., Bullers, S. J., Linggi, B., et al. (2017). Oncostatin M Drives Intestinal Inflammation and Predicts Response to Tumor Necrosis Factor-Neutralizing Therapy in Patients with Inflammatory Bowel Disease. Nat. Med. 23, 579–589. doi:10.1038/nm.4307
Whitcomb, D. C. (2019). Primer on Precision Medicine for Complex Chronic Disorders. Clin. Transl. Gastroenterol. 10, e00067. doi:10.14309/ctg.0000000000000067
Xavier, R. J., and Podolsky, D. K. (2007). Unravelling the Pathogenesis of Inflammatory Bowel Disease. Nature 448, 427–434. doi:10.1038/nature06005
Xiao, H., Bartoszek, K., and Lio’, P. (2018). Multi-omic Analysis of Signalling Factors in Inflammatory Comorbidities. BMC Bioinformatics 19, 439. doi:10.1186/s12859-018-2413-x
Yan, W., Xue, W., Chen, J., and Hu, G. (2016). Biological Networks for Cancer Candidate Biomarkers Discovery. Cancer Inform. 15s3, CIN.S39458. doi:10.4137/CIN.S39458
Yaveroğlu, Ö. N., Malod-Dognin, N., Davis, D., Levnajic, Z., Janjic, V., Karapandza, R., et al. (2014). Revealing the Hidden Language of Complex Networks. Sci. Rep. 4, 4547. doi:10.1038/srep04547
Keywords: inflammatory bowel disease, network biology, protein-protein interaction network, gene coexpression network, multilayered network, precision medicine, gene regulatory network, metabolic network
Citation: Thomas JP, Modos D, Korcsmaros T and Brooks-Warburton J (2021) Network Biology Approaches to Achieve Precision Medicine in Inflammatory Bowel Disease. Front. Genet. 12:760501. doi: 10.3389/fgene.2021.760501
Received: 18 August 2021; Accepted: 08 October 2021;
Published: 21 October 2021.
Edited by:
Alessio Martino, National Research Council (CNR), ItalyReviewed by:
Francesco Bardozzo, University of Salerno, ItalyCopyright © 2021 Thomas, Modos, Korcsmaros and Brooks-Warburton. This is an open-access article distributed under the terms of the Creative Commons Attribution License (CC BY). The use, distribution or reproduction in other forums is permitted, provided the original author(s) and the copyright owner(s) are credited and that the original publication in this journal is cited, in accordance with accepted academic practice. No use, distribution or reproduction is permitted which does not comply with these terms.
*Correspondence: Tamas Korcsmaros, dGFtYXMua29yY3NtYXJvc0BlYXJsaGFtLmFjLnVr
Disclaimer: All claims expressed in this article are solely those of the authors and do not necessarily represent those of their affiliated organizations, or those of the publisher, the editors and the reviewers. Any product that may be evaluated in this article or claim that may be made by its manufacturer is not guaranteed or endorsed by the publisher.
Research integrity at Frontiers
Learn more about the work of our research integrity team to safeguard the quality of each article we publish.