- 1Perelman School of Medicine, University of Pennsylvania, Philadelphia, PA, United States
- 2The Center of Applied Genomics, The Children’s Hospital of Philadelphia, Philadelphia, PA, United States
Despite experimental data linking HIF-1α dysfunction to inflammatory airway conditions, the effect of single nucleotide polymorphisms within the HIF1A gene on these conditions remains poorly understood. In the current study, we complete a phenotype wide association study to assess the link between SNPs with known disease associations and respiratory phenotypes. We report two SNPs of the HIF1A gene, the intronic rs79865957 and the missense rs41508050. In these positions the A and the T allele are significantly associated with allergic rhinitis and acute bronchitis and bronchiolitis, respectively. These findings further support the role of HIF-1α in inflammatory pulmonary conditions and may serve as a basis to refine our understanding of other HIF-1α associated phenotypes.
Introduction
Hypoxia inducible factor (HIF) is central in the mammalian response to hypoxia. (Majmundar et al., 2010) HIF-1 is a nuclear factor that consists of a Hypoxia inducible factor 1α (HIF-1α) and a Hypoxia inducible factor 1β (HIF-1β) subunit. (Wang and Semenza, 1993; Gladek et al., 2017) While HIF-1β is stable regardless of the oxygen concentration, HIF-1α is rapidly degraded under normoxic conditions. (Yu et al., 1998; Gladek et al., 2017) Under hypoxic conditions however, HIF-1α is stabilized, leading to formation of HIF-1. (Ke and Costa, 2006; Slemc and Kunej, 2016) HIF-1 then in turn acts as a transcription factor, affecting over 98 target genes associated with up to 20 biological pathways. (Ke and Costa, 2006; Slemc and Kunej, 2016) Given this central role, it comes as no surprise that variations within the highly conserved HIF1A gene have been associated with a wide array of pathologic conditions. (Majmundar et al., 2010) Apart from playing an important role in normal lung development, HIFs have been shown to play a central role in the development of multiple pulmonary conditions, including pulmonary hypertension, Chronic obstructive pulmonary disease (COPD) and lung cancer angiogenesis. (Shimoda and Semenza, 2011) Despite this, within pulmonology, to date, variations within the HIF1A gene have only been associated with COPD and lung cancer. (Chan et al., 2017; Gladek et al., 2017; Paradowska-Gorycka et al., 2018; Wang et al., 2018; Hoang et al., 2019; Huang et al., 2020) Our current study sets out to examine the association between single nucleotide polymorphisms (SNPs) in the HIF1A gene and respiratory phenotypes. By starting with SNPs of interest, the Phenotype Wide Association Study (PheWAS) design flips the direction of inference commonly used in genome-wide association studies (GWAS). (Bush et al., 2016) To do so, it integrates data captured from patient’s electronic health records (EHRs) with their genetic information. The major benefit of this approach is that it allows us to focus our efforts specifically on SNPs with known disease associations within this master regulator gene, improving the likelihood that found associations are based on molecular mechanisms that are relevant to the disease phenotypes uncovered.
Methods
Single Nucleotide Polymorphisms Selection
SNPs were selected from enriched literature review, including recently completed review by Gladek et al. (Gladek et al., 2017) All studies identified with keywords “HIF1a” and “variant” published after their literature review was completed, were reviewed. SNPs significantly associated with human disease were included in the current study (Table 1).
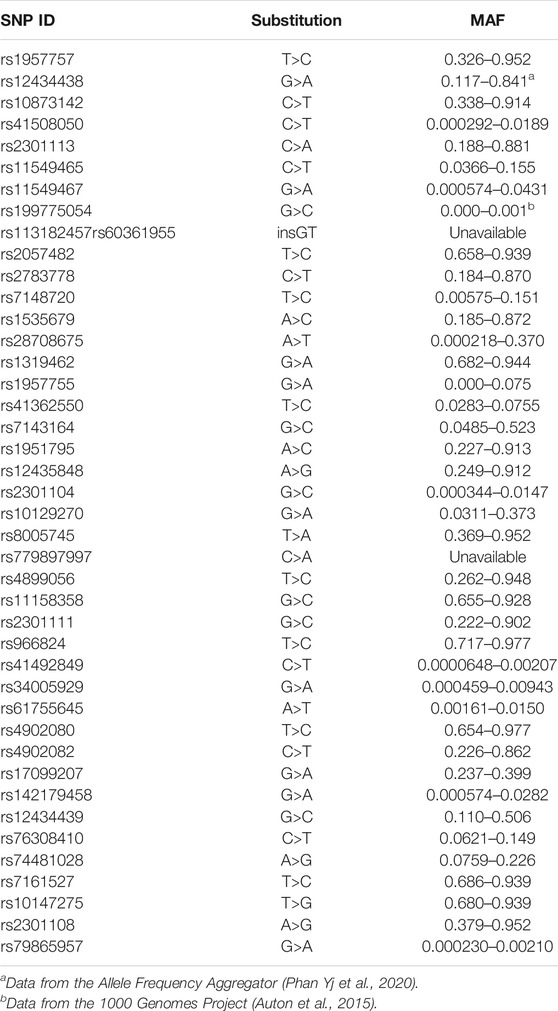
TABLE 1. Minor allele frequency for all SNPs included in the current study, unless otherwise indicated the data was pulled from the genome aggregation database (Karczewski et al., 2020).
Population
Subjects were drawn from The Children’s Hospital of Philadelphia (CHOP) biorepository at the Center for Applied Genomics (CAG). The pediatric samples included in this biorepository are linked to subjects’ EMRs. All subjects have consented to both genomic analysis and EMR mining (Gottesman et al., 2013).
Genotype Imputation
Genotype data were generated by the Center for Applied Genomics on patients recruited from CHOP and were acquired on four major genotyping arrays (HumanHapMap550, 610Q, OMNI2.5M and the GSA array). Where possible, data from similar arrays were merged. Data were filtered for genotype missingness (geno 0.1), individual missingness (0.02), and minor allele frequency (MAF) (0.01) using PLINK v1.9. (Chang et al., 2015) Data were imputed using the TOPMed v2 reference panel on the TOPMed Imputation Server. (Fuchsberger et al., 2015; Das et al., 2016; Taliun et al., 2021) Imputed genotypes were filtered on combinations of Rsq (imputation quality metric) and MAF [(MAF ≥ 0.05 and Rsq > 0.3) OR (MAF < 0.05 and Rsq > 0.5)] using BCFTools v1.10.2, and only SNPs that remained in 85% of samples were retained for use in PheWAS analysis (Danecek et al., 2021).
Ancestry Identification
Subjects in the PheWAS cohort were separated by ancestry based on the results of principal component analysis (PCA). PCA was performed using flashpca on approximately 2.4 million imputed SNPs with MAF >0.01 that had been pruned for linkage disequilibrium using PLINK v1.9 (Abraham and Inouye, 2014; Chang et al., 2015) The first three principle components were plotted, and ancestry designation was performed by comparison to the reference genotypes from the HapMap consortium. (Altshuler et al., 2010) The complete dataset contained 71,600 individuals: 34,410 Caucasians, 31,507 African Americans, 2644 Hispanics, and 3039 East Asians.
PheWAS
A PheWAS was conducting using the published PheWAS R package from Carroll et al. (v0.99.5-5). (Carroll et al., 2014) International Classification of Diseases 9 (ICD-9) codes were obtained from an anonymized extraction of the Children’s Hospital of Philadelphia diagnosis database that contained subjects that had been recruited into the patient collection of the Center for Applied Genomics. Counts of the occurrence of each ICD-9 code for each subject were generated, and the resulting table was converted into the PheWAS phenotype table by a function in the R package. Subjects were included in the case group for each PheWAS phenotype if they possessed two or more occurrences of any of the ICD-9 codes that composed the phenotype in question. Subjects were listed as controls for the PheWAS phenotype if they lacked the case-defining ICD-9 codes, as well as ICD-9 codes corresponding to closely related phenotypes. Conversion from ICD-9 codes to PheWAS phenotypes was performed using the default translation table included in the R package. Phenotypes were analyzed in the PheWAS if they were represented by 20 or more cases in the cohort. The subject’s sex and age were included as covariates in the analysis, as were the 10 flashpca generated principle components and a variable representing the group in which genotyping array had been imputed. Genotypes were extracted from the imputed data as allele dose information to preserve some information regarding genotype probability, and the allele doses were used as the genotype inputs to the PheWAS. The PheWAS analysis was performed individually on each PCA-defined ancestry, and then a meta-analysis was performed combining all four ancestries using the PheWAS-meta function provided in the PheWAS R package. For the association test, a logistic regression model, adjusted for age and sex was used. For defining significance in this study, we set a FDR threshold of 0.05. As a total of 2146 traits were analyzed, the over-conservative significance threshold based on Bonferroni correction was p = 2.3 × 10–5.
In Silico Validation
SNP’s significantly associated with respiratory disease were validated in an independent cohort by querying the publicly available Open Target Genetics database. (Ghoussaini et al., 2021) The Ensembl VEP was then used to assess the likely effect of these variants. (McLaren et al., 2016) To assess chromatin state and regulatory potential associated with the locations of the SNPs, other publicly available databases including Haploreg and Encode were queried.
Results
We found 42 SNPs that have been previously associated with different medical conditions, including various cancers, cardiovascular diseases, metabolic disorders and (auto) immune diseases. This includes the 34 SNPs identified by Gladek et al. (Gladek et al., 2017) In addition, eight more SNPs were identified in studies published after their literature review was completed (Chan et al., 2017; Paradowska-Gorycka et al., 2018; Wang et al., 2018; Hoang et al., 2019; Huang et al., 2020).
Of the 42 SNPs included in our PheWAS, nine were significantly associated with at least one disorder. Table 2 summarizes the data for all the SNP-phenotype associations passing False Discovery Rate (FDR) or Bonferroni test. Most of the detected associations were from cohorts with less than 500 cases. However, the A allele of SNP rs79865957 was found to be significantly associated with allergic rhinitis (Figure 1) in a European cohort of 4,348 cases and 18,794 controls with an allele frequency of 0.08%. The OR was 2.86, Beta 1.05, SE 0.25 and p-value 3.48E−05. The second, rs41508050, the T allele was significantly associated with acute bronchitis and bronchiolitis (Figure 2) in an African American cohort of 2,234 cases and 21,463 controls with an allele frequency of 0.18%. The OR was 0.32, Beta 1.21, SE 3.36 and p-value 0.0001.
Using the Open Target Genetics database rs79865957, the A allele was found to have been previously positively associated with both chronic airway obstruction (OR 1.94, p-value 0.0019, Beta 0.663) and asthma (OR 1.34, p-value 0.033, Beta 0.292). It has also been negatively associated with paternal chronic bronchitis/emphysema (OR 0.75, p-value 0.0069, Beta −0.293). Using Ensemble Variable Effect Predictor (VEP), it was found to be a likely intron variant for HIF1Α. For rs41508050, the T allele was previously negatively correlated with “Bring up phlegm/sputum/mucus on most days” (OR 0.72, p-value 0.0026, Beta −0.328) and is a missense variant for HIF1Α.
The publicly available HaploReg tool was queried for both SNPs. SNP rs79865957 has four SNPs in linkage disequilibrium (r2 ≥ 0.8), two of which (rs76269977 and rs142660658) are intronic in the HIF1A gene. It is located in a regulatory region but not in a constrained sequence. It has histone H3K4me1_Enh enhancer marks in a lung carcinoma line and both H3K4me1_Enh and H3K27ac in a fetal lung fibroblast line. It is also a DNAse hypersensitivity site in a fetal lung fibroblast line. SNP rs41508050 has no other SNPs in linkage disequilibrium, is in a regulatory region and in a constrained sequence both by Genomic Evolutionary Rate Profiling and SiPhycons. It has histone H3K27ac_Enh marks in both lung fibroblast and lung carcinoma lines and is a DNase hypersensitivity site in a lung carcinoma cell line. Looking at Encode it had RFX5 bound in the GM12878 lymphoblastoid cell line. (Table 3)

TABLE 3. Summary of chromatin state and regulatory potential associated with the locations of the SNPs.
Discussion
We present the results of a HIF-1α PheWAS analysis focused on association with respiratory phenotypes. We identified two SNPs that are significantly associated with respiratory disease. Given the allele rarity in our patient population, the Open Target Genetics database was queried in further support. This resource integrates knowledge derived from the UK Biobank with published data from other sources and provides an independent cohort to validate our findings. (Baumann and Cabassa, 2020) The prior associations with allergic airway disease in the form of asthma for rs79865957 and association with bringing up phlegm/sputum/mucus for rs41508050 are consistent with the respective associations with allergic rhinitis and acute bronchitis and bronchiolitis in our cohort, suggesting the association may be driven by the underlying biological “inflammation” process which is the central driver across all these phenotypes involving different organs. To address the likely impact of these variants we used the Ensembl VEP and the publicly available HaploReg tool (Ward and Kellis, 2012; McLaren et al., 2016), both of which underscore the possible significance of both variants. Adding to the evidence supporting a functional impact are the previously published associations between rs79865957 and diabetic kidney disease and between rs41508050 and angina versus myocardial infarction as initial presentation of coronary disease (Hlatky et al., 2007; Huang et al., 2020).
Previously, variations within the HIF1A gene have been associated with COPD, lung cancer and a host of non-pulmonary conditions. (Gladek et al., 2017) Both the SNPs reported here had prior significant disease associations. First, rs79865957 was previously associated with diabetic kidney disease in a Han Chinese population. (Nava-Salazar et al., 2011) While to our knowledge the functional consequences of this SNP have not been eluded, the authors hypothesized that in a high glucose environment HIF1A transcription may be stimulated. Additionally, rs41508050 has a known association with the development of stable angina as opposed to myocardial infarction as initial presentation of coronary artery disease. (Hlatky et al., 2007) In vitro studies have previously linked this variant with a higher transcriptional activity. (Nava-Salazar et al., 2011) However, to our knowledge, the current study is the first to report on the association between SNPs of the HIF1A gene and allergic rhinitis, acute bronchitis and bronchiolitis. The reported association with allergic rhinitis is consistent with previously published experimental data highlighting the role of HIF-1α in allergic airway pathology. In an allergic airway disease model, HIF-1α inhibition decreased Th2 inflammation as measured by reduced IL-4, IL-5 and IL-13. (Kim et al., 2010) Beyond this, in a mouse model downregulation of HIF-1 or blockade of HIF-1α reduced cellular infiltrate in peribronchial lung tissues, thickness of smooth muscle and eosinophil infiltration. (Huerta-Yepez et al., 2008) Likewise, the role of HIF-1α in bronchiolitis is supported by experimental data on the consequences of HIF-1α stabilization by the Respiratory Syncytium Virus. (Kilani et al., 2004)
Traditionally, GWAS identify SNPs significantly associated with human disease. These findings are then used to guide animal studies aiming to prove a causal link between the SNP and the disease. As briefly discussed above, the PheWAS design flips this process. It allowed us to look specifically at a highly conserved gene known to play a central role in the diseases of interest. In doing so, we were able to narrow down the list of SNPs within the HIF1A gene that play a potential role in respiratory pathology. Beyond this, we were able to detect significant effects of rare allelic variants. Conversely, this study design by definition excludes variants on other genes. While this is a limitation of the current study, given the hypoxemia dependent stabilization of HIF-1α and the experimental data supporting a role of HIF-1α in pulmonary conditions as outlined above it seemed reasonable to focus on HIF1A. Future studies may expand on the current work by including other members of the HIF family. Furthermoree, knowing that SNPs within the HIF1A gene are associated with respiratory diseases future studies can now refine our understanding of the associated phenotypes by looking at differences between patients with and without these SNPs.
Data Availability Statement
The data analyzed in this study is subject to the following licenses/restrictions: Some of the data used are available in deidentified format in dbGaP. Requests to access these datasets should be directed to https://www.ncbi.nlm.nih.gov/gap/.
Author Contributions
JK: Conceptualization, Methodology, writing original draft, reviewing, editing. XC: Conceptualization, Methodology, formal analysis, data curation, reviewing, editing, visualization. MM and FM: Methodology, validation, data curation, reviewing. PS: Conceptualization, Methodology, reviewing. HH: Conceptualization, Methodology, reviewing, editing, Supervision.
Funding
This work was supported by Institutional Development Funds and the Endowed Chair in Genomic Research grant from The Children’s Hospital of Philadelphia. Research reported in this publication was supported by the National Center for Advancing Translational Sciences of the National Institutes of Health under award number TL1TR001880. The content is solely the responsibility of the authors and does not necessarily represent the official views of the National Institutes of Health.
Conflict of Interest
The authors declare that the research was conducted in the absence of any commercial or financial relationships that could be construed as a potential conflict of interest.
Publisher’s Note
All claims expressed in this article are solely those of the authors and do not necessarily represent those of their affiliated organizations, or those of the publisher, the editors and the reviewers. Any product that may be evaluated in this article, or claim that may be made by its manufacturer, is not guaranteed or endorsed by the publisher.
Acknowledgments
We thank the patients and their families for their participation in the respective genotyping studies and the Biobank Repository at the Center for Applied Genomics.
References
Abraham, G., and Inouye, M. (2014). Fast Principal Component Analysis of Large-Scale Genome-wide Data. PloS one 9 (4), e93766. doi:10.1371/journal.pone.0093766
Altshuler, D. M., Gibbs, R. A., Peltonen, L., Altshuler, D. M., Gibbs, R. A., Peltonen, L., et al. (2010). Integrating Common and Rare Genetic Variation in Diverse Human Populations. Nature 467 (7311), 52–58. doi:10.1038/nature09298
Auton, A., Auton, A., Brooks, L. D., Durbin, R. M., Garrison, E. P., Kang, H. M., et al. (2015). A Global Reference for Human Genetic Variation. Nature 526 (7571), 68–74. doi:10.1038/nature15393
Baumann, A. A., and Cabassa, L. J. (2020). Reframing Implementation Science to Address Inequities in Healthcare Delivery. BMC Health Serv. Res. 20 (1), 190. doi:10.1186/s12913-020-4975-3
Bush, W. S., Oetjens, M. T., and Crawford, D. C. (2016). Unravelling the Human Genome-Phenome Relationship Using Phenome-wide Association Studies. Nat. Rev. Genet. 17 (3), 129–145. doi:10.1038/nrg.2015.36
Carroll, R. J., Bastarache, L., and Denny, J. C., and (2014). R PheWAS: Data Analysis and Plotting Tools for Phenome-wide Association Studies in the R Environment. Bioinformatics 30 (16), 2375–2376. doi:10.1093/bioinformatics/btu197
Chan, C. H. T., Munusamy, P., Loke, S. Y., Koh, G. L., Wong, E. S. Y., Law, H. Y., et al. (2017). Identification of Novel Breast Cancer Risk Loci. Cancer Res. 77 (19), 5428–5437. doi:10.1158/0008-5472.can-17-0992
Chang, C. C., Chow, C. C., Tellier, L. C., Vattikuti, S., Purcell, S. M., and Lee, J. J. (2015). Second-generation PLINK: Rising to the Challenge of Larger and Richer Datasets. GigaScience 4, 7. doi:10.1186/s13742-015-0047-8
Danecek, P., Bonfield, J. K., Liddle, J., Marshall, J., Ohan, V., Pollard, M. O., et al. (2021). Twelve Years of SAMtools and BCFtools. GigaScience 10 (2), giab008. doi:10.1093/gigascience/giab008
Das, S., Forer, L., Schönherr, S., Sidore, C., Locke, A. E., Kwong, A., et al. (2016). Next-generation Genotype Imputation Service and Methods. Nat. Genet. 48 (10), 1284–1287. doi:10.1038/ng.3656
Fuchsberger, C., Abecasis, G. R., and Hinds, D. A. (2015). Minimac2: Faster Genotype Imputation. Bioinformatics 31 (5), 782–784. doi:10.1093/bioinformatics/btu704
Ghoussaini, M., Mountjoy, E., Carmona, M., Peat, G., Schmidt, E. M., Hercules, A., et al. (2021). Open Targets Genetics: Systematic Identification of Trait-Associated Genes Using Large-Scale Genetics and Functional Genomics. Nucleic Acids Res. 49 (D1), D1311–D1320. doi:10.1093/nar/gkaa840
Gladek, I., Ferdin, J., Horvat, S., Calin, G. A., and Kunej, T. (2017). HIF1Agene Polymorphisms and Human Diseases: Graphical Review of 97 Association Studies. Genes Chromosom. Cancer 56 (6), 439–452. doi:10.1002/gcc.22449
Gottesman, O., Kuivaniemi, H., Kuivaniemi, H., Tromp, G., Faucett, W. A., Li, R., et al. (2013). The Electronic Medical Records and Genomics (eMERGE) Network: Past, Present, and Future. Genet. Med. 15 (10), 761–771. doi:10.1038/gim.2013.72
Hlatky, M. A., Quertermous, T., Boothroyd, D. B., Priest, J. R., Glassford, A. J., Myers, R. M., et al. (2007). Polymorphisms in Hypoxia Inducible Factor 1 and the Initial Clinical Presentation of Coronary Disease. Am. Heart J. 154 (6), 1035–1042. doi:10.1016/j.ahj.2007.07.042
Hoang, T. T., Manso, P. H., Edman, S., Mercer-Rosa, L., Mitchell, L. E., Sewda, A., et al. (2019). Genetic Variants of HIF1α Are Associated with Right Ventricular Fibrotic Load in Repaired Tetralogy of Fallot Patients: A Cardiovascular Magnetic Resonance Study. J. Cardiovasc. Magn. Reson. 21 (1), 51. doi:10.1186/s12968-019-0555-2
Huang, Y., Jin, L., Yu, H., Jiang, G., Tam, C. H. T., Jiang, S., et al. (2020). SNPs in PRKCA-Hif1a-GLUT1 Are Associated with Diabetic Kidney Disease in a Chinese Han Population with Type 2 Diabetes. Eur. J. Clin. Invest.,50 (9), e13264. doi:10.1111/eci.13264
Huerta-Yepez, S., Baay-Guzman, G. J., Garcia-Zepeda, R., Hernandez-Pando, R., Vega, M. I., Gonzalez-Bonilla, C., et al. (2008). 2-Methoxyestradiol (2-ME) Reduces the Airway Inflammation and Remodeling in an Experimental Mouse Model. Clin. Immunol. 129 (2), 313–324. doi:10.1016/j.clim.2008.07.023
Karczewski, K. J., Francioli, L. C., Tiao, G., Cummings, B. B., Alföldi, J., Wang, Q., et al. (2020). The Mutational Constraint Spectrum Quantified From Variation in 141,456 Humans. Nature 581 (7809), 434–443. doi:10.1038/s41586-020-2308-7
Ke, Q., and Costa, M. (2006). Hypoxia-inducible Factor-1 (HIF-1). Mol. Pharmacol. 70 (5), 1469–1480. doi:10.1124/mol.106.027029
Kilani, M. M., Mohammed, K. A., Nasreen, N., Tepper, R. S., and Antony, V. B. (2004). RSV Causes HIF-1α Stabilization via NO Release in Primary Bronchial Epithelial Cells. Inflammation 28 (5), 245–251. doi:10.1007/s10753-004-6047-y
Kim, S. R., Lee, K. S., Park, H. S., Park, S. J., Min, K. H., Moon, H., et al. (2010). HIF-1α Inhibition Ameliorates an Allergic Airway Disease via VEGF Suppression in Bronchial Epithelium. Eur. J. Immunol. 40 (10), 2858–2869. doi:10.1002/eji.200939948
Majmundar, A. J., Wong, W. J., and Simon, M. C. (2010). Hypoxia-inducible Factors and the Response to Hypoxic Stress. Mol. Cel. 40 (2), 294–309. doi:10.1016/j.molcel.2010.09.022
McLaren, W., Gil, L., Hunt, S. E., Riat, H. S., Ritchie, G. R. S., Thormann, A., et al. (2016). The Ensembl Variant Effect Predictor. Genome Biol. 17 (1), 122. doi:10.1186/s13059-016-0974-4
Nava-Salazar, S., Sánchez-Rodríguez, E. N., Mendoza-Rodríguez, C. A., Moran, C., Romero-Arauz, J. F., and Cerbón, M. A. (2011). Polymorphisms in the Hypoxia-Inducible Factor 1 Alpha Gene in Mexican Patients with Preeclampsia: A Case-Control Study. BMC Res. Notes 4 (1), 68. doi:10.1186/1756-0500-4-68
Paradowska-Gorycka, A., Stypinska, B., Pawlik, A., Haladyj, E., Romanowska-Próchnicka, K., and Olesinska, M. (2018). HIF-1A Gene Polymorphisms and its Protein Level in Patients with Rheumatoid Arthritis: a Case-Control Study. Inflamm. Res. 67 (5), 423–433. doi:10.1007/s00011-018-1134-y
Phan Yj, L., Zhang, H., Qiang, W., Shekhtman, E., Shao, D., Revoe, D., et al. (2020). ALFA: Allele Frequency Aggregator. Bethesda, MD: National Center for Biotechnology Information, US National Library of Medicine.
Shimoda, L. A., and Semenza, G. L. (2011). HIF and the Lung. Am. J. Respir. Crit. Care Med. 183 (2), 152–156. doi:10.1164/rccm.201009-1393pp
Slemc, L., and Kunej, T. (2016). Transcription Factor HIF1A: Downstream Targets, Associated Pathways, Polymorphic Hypoxia Response Element (HRE) Sites, and Initiative for Standardization of Reporting in Scientific Literature. Tumor Biol. 37 (11), 14851–14861. doi:10.1007/s13277-016-5331-4
Taliun, D., Harris, D. N., Kessler, M. D., Carlson, J., Szpiech, Z. A., Torres, R., et al. (2021). Sequencing of 53,831 Diverse Genomes from the NHLBI TOPMed Program. Nature 590 (7845), 290–299. doi:10.1038/s41586-021-03205-y
Wang, D., Fan, Y., Malhi, M., Bi, R., Wu, Y., Xu, M., et al. (2018). Missense Variants in HIF1A and LACC1 Contribute to Leprosy Risk in Han Chinese. Am. J. Hum. Genet. 102 (5), 794–805. doi:10.1016/j.ajhg.2018.03.006
Wang, G. L., and Semenza, G. L. (1993). General Involvement of Hypoxia-Inducible Factor 1 in Transcriptional Response to Hypoxia. Proc. Natl. Acad. Sci. 90 (9), 4304–4308. doi:10.1073/pnas.90.9.4304
Ward, L. D., and Kellis, M. (2012). HaploReg: A Resource for Exploring Chromatin States, Conservation, and Regulatory Motif Alterations Within Sets of Genetically Linked Variants. Nucleic Acids Res. 40 (Database issue), D930–D934. doi:10.1093/nar/gkr917
Keywords: phenotype, hypoxia inducible factor, inflammation, airway, HIF1A, rhinitis, bronchiolitis, SNP
Citation: Kelchtermans J, Chang X, March ME, Mentch F, Sleiman PMA and Hakonarson H (2021) HIF-1α Pulmonary Phenotype Wide Association Study Unveils a Link to Inflammatory Airway Conditions. Front. Genet. 12:756645. doi: 10.3389/fgene.2021.756645
Received: 10 August 2021; Accepted: 08 September 2021;
Published: 21 September 2021.
Edited by:
Lei Wang, Changsha University, ChinaReviewed by:
Yulin Dai, University of Texas Health Science Center at Houston, United StatesYongzhang Zhu, Shanghai Jiao Tong University, China
Copyright © 2021 Kelchtermans, Chang, March, Mentch, Sleiman and Hakonarson. This is an open-access article distributed under the terms of the Creative Commons Attribution License (CC BY). The use, distribution or reproduction in other forums is permitted, provided the original author(s) and the copyright owner(s) are credited and that the original publication in this journal is cited, in accordance with accepted academic practice. No use, distribution or reproduction is permitted which does not comply with these terms.
*Correspondence: Jelte Kelchtermans, a2VsY2h0ZXJtakBjaG9wLmVkdQ==
†These authors have contributed equally to this work and share first authorship