- 1Department of Hematology, The Second Medical Center, Chinese PLA General Hospital, National Clinical Research Center for Geriatric Disease, Beijing, China
- 2Department of Oncology, The Fifth Medical Center of Chinese PLA General Hospital, Beijing, China
- 3Medical School of Chinese PLA, Beijing, China
- 4College of Science, University of Shanghai for Science and Technology, Beijing, China
- 5State Key Laboratory of Toxicology and Medical Countermeasures, Beijing Key Laboratory of Neuropsychopharmacology, Beijing Institute of Pharmacology and Toxicology, Beijing, China
This study aims to determine hub genes related to the incidence and prognosis of EGFR-mutant (MT) lung adenocarcinoma (LUAD) with weighted gene coexpression network analysis (WGCNA). From The Cancer Genome Atlas (TCGA) and Gene Expression Omnibus (GEO) databases, we used 253 EGFR-MT LUAD samples and 38 normal lung tissue samples. At the same time, GSE19188 was additionally included to verify the accuracy of the predicted gene. To discover differentially expressed genes (DEGs), the R package “limma” was used. The R packages “WGCNA” and “survival” were used to perform WGCNA and survival analyses, respectively. The functional analysis was carried out with the R package “clusterProfiler.” In total, 1450 EGFR-MT–specific DEGs were found, and 7 tumor-related modules were marked with WGCNA. We found 6 hub genes in DEGs that overlapped with the tumor-related modules, and the overexpression level of B3GNT3 was significantly associated with the worse OS (overall survival) of the EGFR-MT LUAD patients (p < 0.05). Functional analysis of the hub genes showed the metabolism and protein synthesis–related terms added value. In conclusion, we used WGCNA to identify hub genes in the development of EGFR-MT LUAD. The established prognostic factors could be used as clinical biomarkers. To confirm the mechanism of those genes in EGFR-MT LUAD, further molecular research is required.
Introduction
Lung cancer is the most prominent cancer-related cause of death worldwide. Non–small-cell lung carcinoma (NSCLC) accounts for 75–80 percent of all lung cancers and is often detected at an early stage, resulting in a poor prognosis (Liu et al., 2017). Lung adenocarcinoma is the most prevalent form of NSCLC (LUAD) (Yang et al., 2020a).
Significant advances in the understanding of lung cancer, especially LUAD, have been made in recent years. The epidermal growth factor receptor (EGFR) has been identified as an oncoming engine. Especially in Asian lung adenocarcinoma patients, the frequency of EGFR mutations is higher (Devanagari et al., 2015). Treatments for managing EGFR-mutant (EGFR-MT) LUAD included the following: radiation therapy, surgery, chemotherapy, and EGFR tyrosine kinase inhibitors (TKIs) (Hsu et al., 2018). Based on the diagnosis, suitable variations of the three treatment modalities are chosen. Although the overall survival (OS) of EGFR-MT LUAD patients has been significantly improved due to the emergence of TKIs, there are still some critical patients or TKI-resistant patients with limited survival advantages (Yang et al., 2020b).
In the past few decades, high-throughput technologies such as gene chips and gene sequencing have been widely used to identify driver genes and detect important somatic nucleotide polymorphisms, and gene fusions during tumorigenesis, recurrence, and metastasis (Luo et al., 2018; Nahum et al., 2018; O'Farrell et al., 2019). Understanding these genetic alterations may assist in interpreting the molecular mechanism of EGFR-MT LUAD, but the genetic and cytogenetic complexities intrinsic to EGFR-MT LUAD are difficult to uncover because cancer biology is regulated by several factors, including ferroptosis, hypoxia, and tumor microenvironment (Hanahan and Coussens, 2012; Qiu et al., 2017; Gao et al., 2019). It is important to establish a realistic and accurate diagnostic test that can predict the likelihood of EGFR-MT LUAD metastasis or progression.
Structure network algorithms were widely used to identify important nodes in a network by measuring the leadership role of a node based on all of its links (Zeng et al., 2016; Bu et al., 2020). One of the most remarkable methods is weighted correlation network analysis (WGCNA), a scientific tool for explaining the pattern of gene interaction between different samples (Langfelder and Horvath, 2008). It can be used to locate and scan co-expressed gene modules and essential biomarkers. This method has not been used in EGFR-MT LUAD to our knowledge. The aim of our study was to identify novel gene network co-expression modules associated with EGFR-MT LUAD by WGCNA to determine the key signal pathways and genes involved in EGFR-MT LUAD pathogenesis and prognostics.
Materials and Methods
Figure 1 shows the workflow of the analytical key gene extraction pipeline. In the following subsections, we elaborate on each step. In this study, the data of GSE31210 and TCGA were set as the training set for screening key prognostic genes, and the data of GSE19188 were set as the test set to verify the results.
Data Sources and Data Preprocessing
GSE31210 (Okinawa et al., 2012; Yamauchi et al., 2012) and GSE19188 (Hou et al., 2010) were downloaded from the Gene Expression Omnibus (GEO) database (https://www.ncbi.nlm.nih.gov/geo). The “impute” and “limma” packages were used to supplement the missing data and standardize the two expression profiles. The two data corresponding to clinical information were extracted and integrated for subsequent use.
EGFR-MT LUAD RNA-seq data and corresponding clinical information were downloaded from TCGA (The Cancer Genome Atlas) database (https://portal.gdc.cancer.gov/). 126 EGFR-MT LUAD and 18 normal samples of tissues have been presented. The data were annotated in a human hg38 gene standard track reference transcript. After the count per million (CPM) < 1 gene was filtered using the function space in the “edgeR” package, calculated with gene counts divided according to the gene length, our next analysis was made with 15,213 genes with RPKM values. The detailed information of all the data used in this research is given in Table 1.
Identification of Tumor-Related Modules With WGCNA
The TCGA-LUAD and GSE31210 gene expression data were built using a “WGCNA” package in the form of genetic co-expression modules in R (Zeng et al., 2016). Soft power β = 1 was chosen in both data to create a scale-free network. Next, the adjacency matrix was generated using the following formula: Aid = |Sij|β (aid: adjacency matrix between gene I and gene j, Sij: similarity matrix rendered by Pearson’s association of both gene pairs, β: soft power value) and converted into a topological overlap matrix (TOM) as well as corresponding dissimilarity (1-TOM). A hierarchical clustering dendrogram was formed for the 1-TOM matrix in the subsequent grouping, with a minimum of 50 genes for dendrogram for the same gene expressions, into separate gene co-expression modules. The link between the modules and the details of the clinical characteristics was calculated for tumor-related modules.
Screen DEGs and Hub Genes Shared With Tumor-Related Modules
We used the limma package to screen DEGs of TCGA-EGFR-MT LUAD and GSE31210 (Ritchie et al., 2015). The |log2 (fold change) |>2 and adjust p value < 0.05 were set to screen DEGs. The volcano plot of DEGs was visualized by the R package “ggplot2” (Wickham, 2009). Subsequently, genes overlapping in modules linked to tumors harvested as hub gene candidates for later detection were presented as a diagram of Venn using the package “VennDiagram” (Chen and Boutros, 2011).
Functional Enrichment for Hub Genes
Functional enrichment analysis for hub genes included Gene Ontology (GO) and Kyoto Encyclopedia of Genes and Genomes (KEGG: http://www.kegg.jp/kegg/) pathway enrichment analysis, which were performed for genes by “clusterProfiler” package in R (Yu et al., 2012). An adjusted p-value <0.05 was considered significant.
The Validation of Hub Gene Expression Patterns and Prognostic Values
The expression patterns of the hub gene in various pathological EGFR-MT LA and normal tissue have been tested to validate the reliability of the hub genes. The levels of expression of each hub gene were seen as a box plot graph between EGFR-MT LA and normal tissue. The “survival” package was used to conduct a Kaplan–Meier survival analysis based on the data from TCGA database to explore the correlation between overall survival (OS) and hub genes in patients. For the survival study, only patients who had finished their follow-up period were chosen, and they were split into two classes depending on the median expression value of hub genes. The survival-related hub genes with a log-rank p value < 0.05 were regarded as statistically significant. In order to explore the functions and pathways of hub genes which were statistically significant, gene set enrichment analysis (GSEA) was performed in the high-expression and the low-expression groups; gene sets with |NES|>1, NOM p < 0.05, were considered to be enrichment significant.
Microarray Data and the HPA Database Were Used to Verify Protein Expressions of Survival-Related Hub Genes
To verify the expression level and survival significance of the 6 hub genes, we introduced another microarray data of LUAD (GSE19188) for external data verification. Based on the clinical information of GSE19188, the prognostic significance of the 6 hub genes was verified.
At the same time, we used immunohistochemistry (IHC) from the Human Protein Atlas (HPA) database (https://www.proteinatlas.org/) to further verify the protein expression of survival-related genes (Thul and Lindskog, 2018). Also, the protein expression pattern based on IHC is the most commonly used method for detecting the relative position and abundance of proteins in immunotherapy (Maity et al., 2013).
Results
Identification of Co-Expression Gene Modules With WGCNA
A total of 7 modules in the TCGA–EGFR-MT LUAD (Supplementary Figure S1A) and 9 modules in the GSE31210 (Supplementary Figure S2A) were identified via average linkage clustering (excluding gray modules that were not assigned to any cluster). The results of the module–trait relationships revealed that 3 modules in the TCGA–EGFR-MT LUAD and 4 modules in the GSE6631 were found to have an association with tumor tissues (Supplementary Figures S1B, S2B).
Screen DEGs and Identification of Hub Genes
1,149 DEGs in the TCGA dataset (Figure 2A) and 301 DEGs in the GSE31210 dataset (Figure 2B) were defined as deregulated in tumor tissues using a cutoff criterion (log2 (fold change)≥ 2.0 and adj. p < 0.05). Subsequently, the extracted 6 genes in DEGs that overlapped with the tumor-related modules including beta-1,3-N-acetylglucosaminyltransferase 3 (B3GNT3), adhering 3 (CDH3), cysteine SN (CST1), zinc finger and BTB domain containing 16 (ZBTB16), keratin 15 (KRT15), and cloth beta (KLB) were selected as the hub genes for subsequent analysis (Figure 2C).
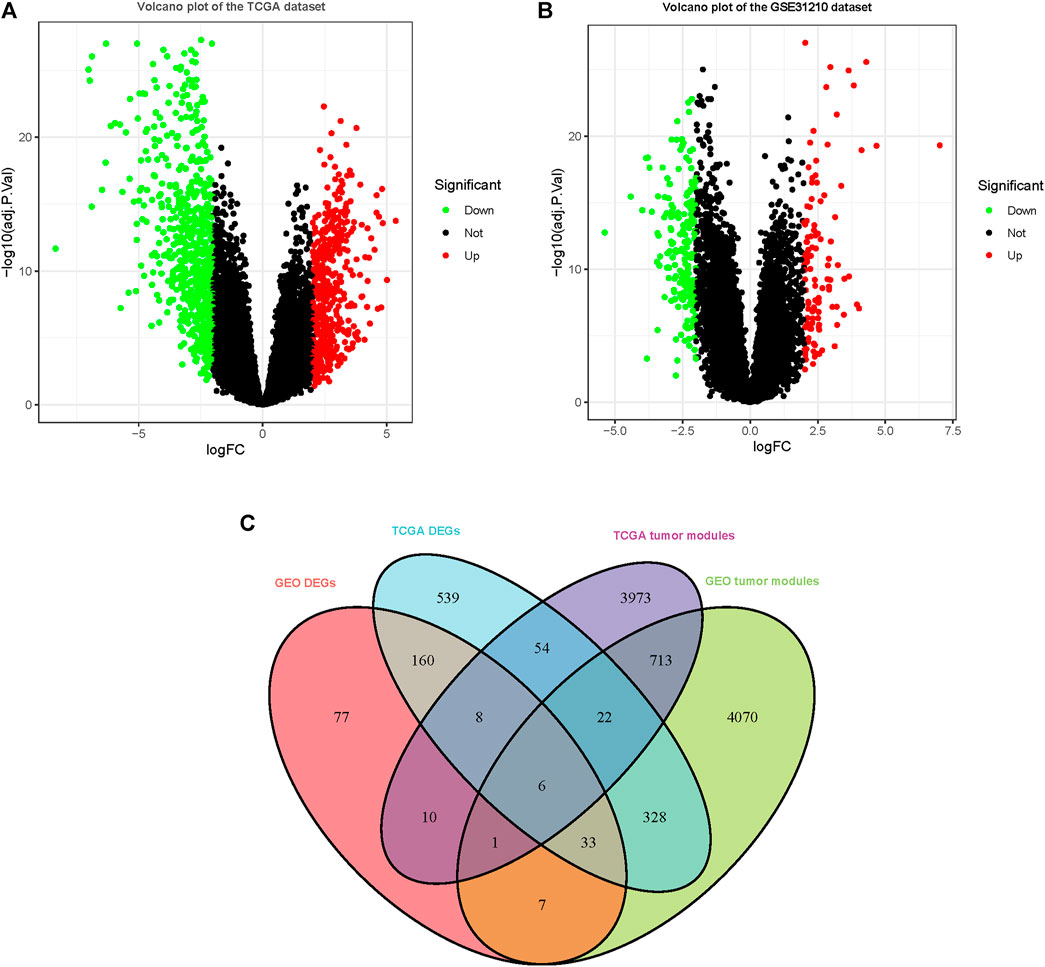
FIGURE 2. DEGs were observed in TCGA and GSE31210 datasets using |logFC|≥2.0 and adj. p < 0.05 as cutoff parameters. (A) Volcano plot of DEGs in TCGA dataset. (B) Volcano plot of DEGs in the GSE31210 dataset. (C) Genes contained in DEGs and tumor-related modules in a Venn diagram. At the intersection of DEGs and modules, there are a total of 6 overlapping genes.
Functional Enrichment Analysis for Hub Genes
After the screening of GO enrichment analysis, the top 5 enriched gene sets are shown in Figure 3A. The biological process (BP) of 6 hub genes is mainly enriched in keratinization and the poly-N-acetyllactosamine biosynthetic process. The cellular component (CC) showed that these genes were mainly involved in the catering complex. Moreover, in the molecular function (MF) analysis, fibroblast growth factor binding and fibroblast growth factor receptor binding were suggested to be related to the 6 genes. As shown in Figure 3B, 6 hub genes were enriched in the KEGG pathway of glycosphingolipid biosynthesis—lacto and neglect series.
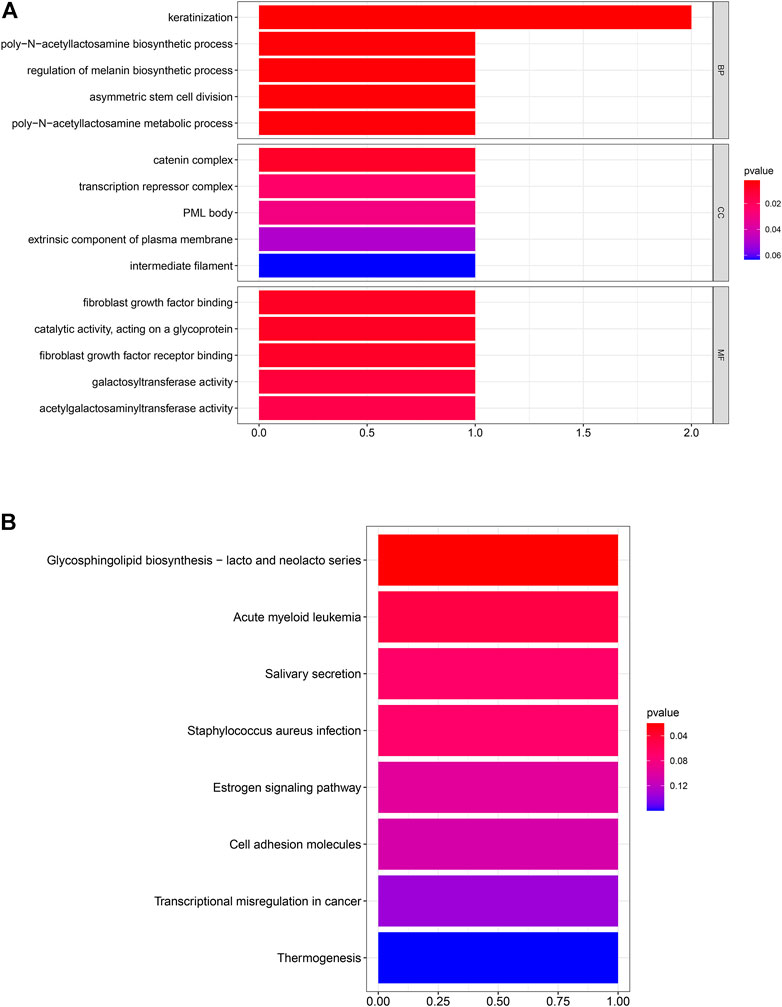
FIGURE 3. Six hub genes were analyzed for enrichment. The size of the spots represents the gene number, and the color represents the adjusted p-values (BH). (A) Result of GO enrichment analysis. (B) Result of KEGG enrichment analysis.
Analysis and Verification of the Hub Gene Expression Level and Survival Significance
In EGFR-MT LUAD tissues (RNA-seq data from TCGA), all of the 6 hub genes were found to be substantially downregulated or unchecked, as shown in Figure 4. Furthermore, Kaplan–Meier survival studies of the 6 hub genes showed that B3GNT3 overexpression was substantially correlated with poorer overall survival of EGFR-MT LUAD patients (p < 0.05) (Figure 5).
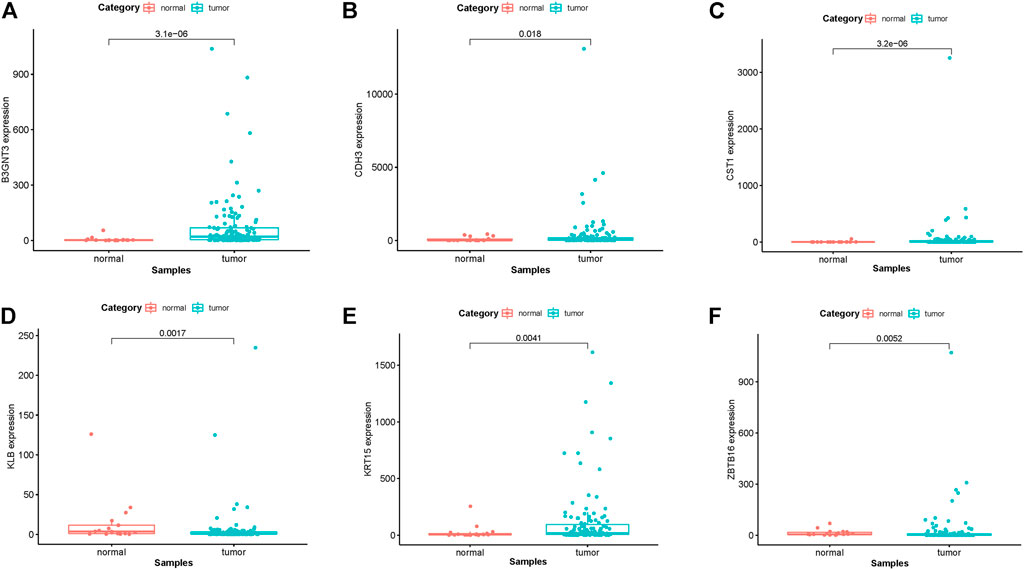
FIGURE 4. TCGA database was used to verify the expression levels of 6 hub genes in EGFR-MT LUAD and normal tissues. (A) Gene expression values of B3GNT3 among samples of TCGA. (B) Gene expression values CDH3 among samples of TCGA. (C) Gene expression values of CST1 among samples of TCGA. (D) Gene expression values of KLB among samples of TCGA. (E) Gene expression values of KRT15 among samples of TCGA. (F) Gene expression values of ZBTB16 among samples of TCGA.
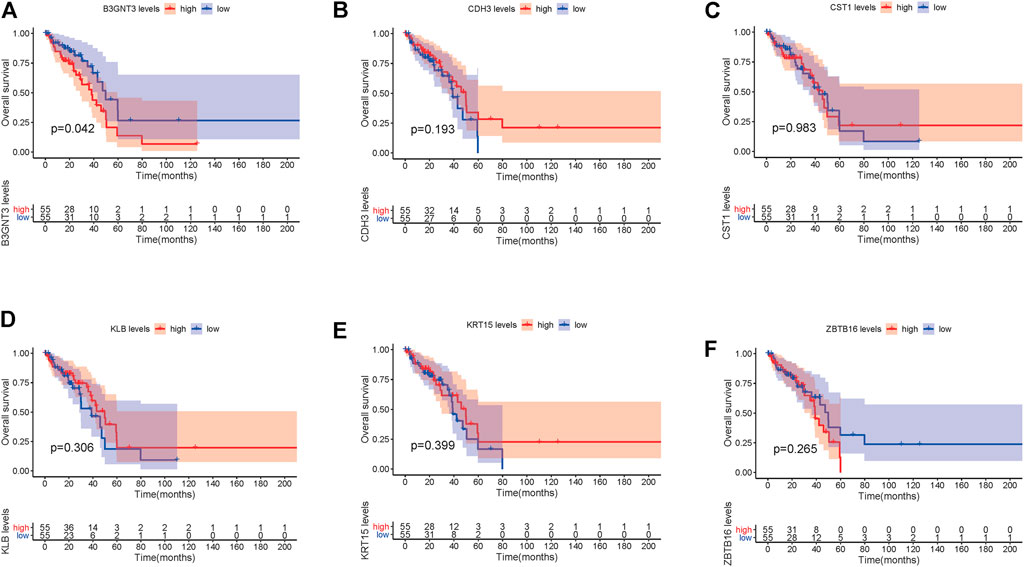
FIGURE 5. TCGA database was used to look at the overall survival (OS) of 6 hub genes in EGFR-MT LUAD patients. (A) Survival analysis for B3GNT3. (B) Survival analysis for CDH3. (C) Survival analysis for CST1. (D) Survival analysis for KLB. (E) Survival analysis for KRT15. (F) Survival analysis for ZBTB16. The patients were stratified into the high-level group (red) and low-level group (blue) according to the median expression of the gene. Log-rank p < 0.05 was considered to be a statistically significant difference.
GSE19188 was used to verify the expression level and survival significance of the 6 hub genes. It was found that compared with normal lung tissues, the 6 hub genes were significantly inhibited or overexpressed, and the results of B3GNT3 were consistent with the results of RNA-seq data analysis from TCGA (Figure 6). The GSEA enrichment term exhibited that high expression of B3GNT3 was mainly associated with ether lipid metabolism, lysosome, steroid biosynthesis, glycan biosynthesis, and so on (Table 2). According to the HPA database, the protein levels of the B3GNT3 gene were substantially higher in tumor tissues than in normal tissues (Figure 7).
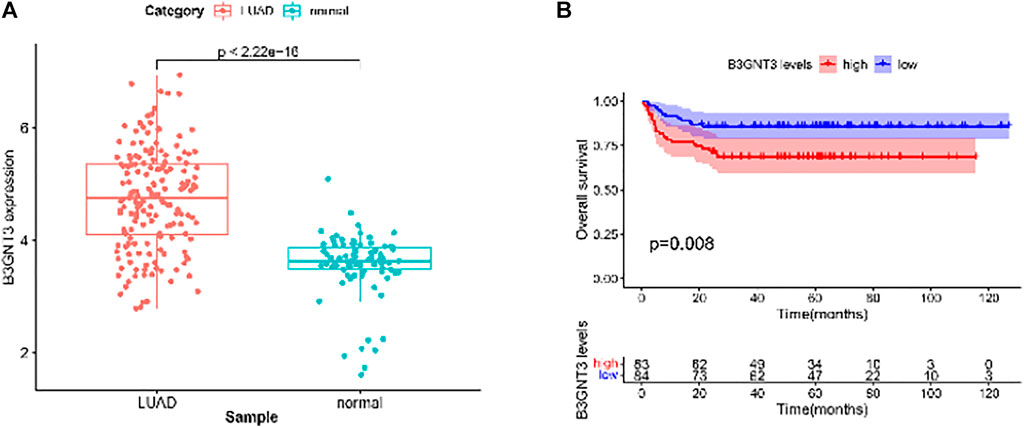
FIGURE 6. GSE19188 was used to verify the expression levels of 6 hub genes. (A) Gene expression values of B3GNT3. The GSE19188 was used to verify the overall survival (OS) of 6 hub genes. (B) Survival analysis for B3GNT3. The patients were stratified into the high-level group (red) and low-level group (blue) according to the median expression of the gene. Log-rank p < 0.05 was considered to be a statistically significant difference.
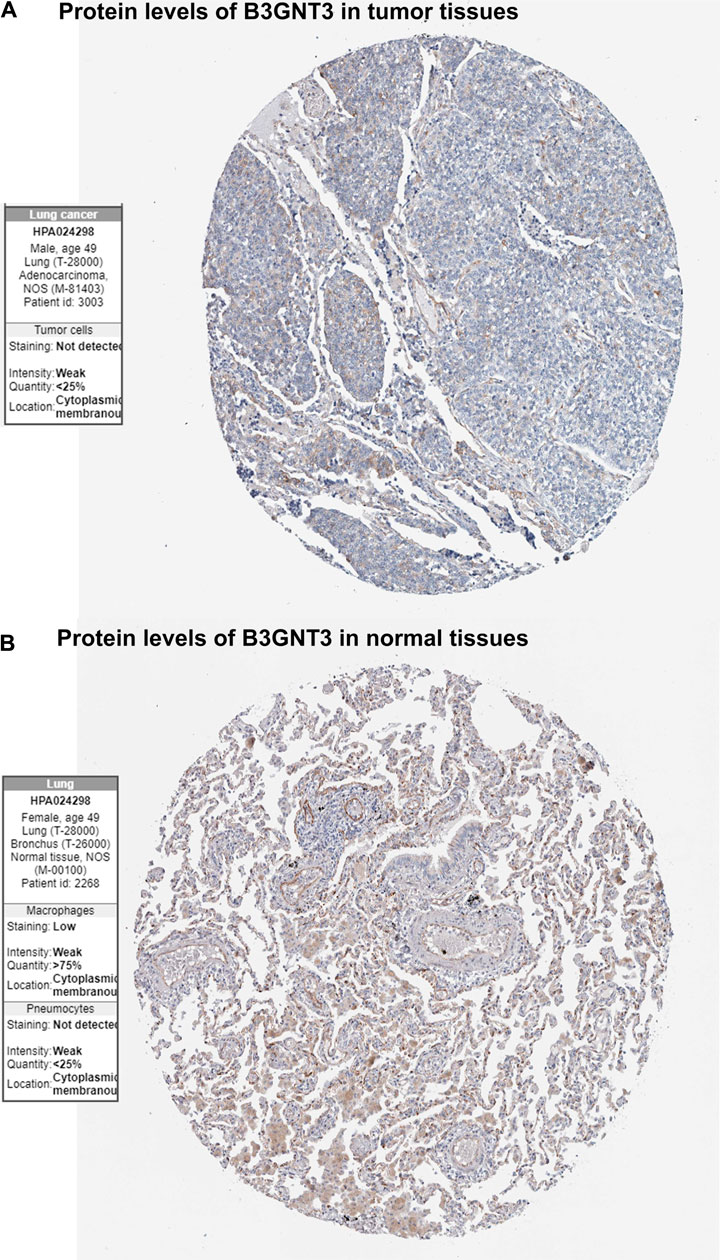
FIGURE 7. Immunohistochemistry of the B3GNT3 gene in LUAD and normal tissues from the Human Protein Atlas (HPA) database. (A) Protein levels of B3GNT3 in LUAD tissues. (B) Protein levels of B3GNT3 in normal lung tissues.
Discussion
The WGCNA is a valuable method for finding highly correlated gene modules. The main module’s intramuscular center could be used for disease detection and prognostication, such as cancer. We use specific DEGs caused by EGFR mutations to perform WGCNA on EGFR-MT LUAD and normal lung samples. We found B3GNT3 correlated with the prognosis of EGFR-MT LUAD patients. Moreover, the functional analysis found these 6 hub genes mainly enriched in keratinization terms and glycosphingolipid biosynthesis—lacto and neglect series pathway.
B3GNT3, also known as acetylglucosaminyltransferase, is a member of the beta-1,3-N-acetylglucosaminyltransferase family (Ho et al., 2013). It plays a dominant role in L-selectin ligand biosynthesis, lymphocyte homing, and lymphocyte trafficking. (Maity et al., 2013). Besides, in early cervical cancer, pancreatic cancer, and neuroblastoma, the level of B3GNT3 mRNA is higher than that of adjacent control tissues (Ho et al., 2013; Zhang et al., 2015; Barkley et al., 2018; Li et al., 2018). B3GNT3 was shown to be upregulated in tumor tissues as opposed to normal tissues in our sample, with a strong link to EGFR-MT LUAD. Higher levels of B3GNT3 have been related to a weak prognosis in patients with NSCLC in previous trials, but it is uncertain which subtype of NSCLC is involved (Gao et al., 2018). That was in line with our survival review results, and our research contributes to the growing body of evidence that B3GNT3 can be used as a diagnostic and prognostic marker for EGFR-MT LUAD.
Although the other 5 hub genes in our study did not suggest significance for the OS of EGFR-MT LUAD patients, studies have confirmed that they are closely related to EGFR-MT LUAD metastasis, recurrence, and drug resistance. Ting et al. found that high CDH3 expression is related to EGFR-TKI resistance (Hsiao et al., 2020a); Cao et al. found that high CST1 expression can be used as a marker for recurrence and metastasis in patients with NSCLC (Cao et al., 2015); Wang et al. found that low expression of ZBTB16 can promote the survival of NSCLC tumor cells and enhance their invasiveness (Wang et al., 2013; Xiao et al., 2015). Our study revealed that these genes are heavily enriched in metabolism-related biological processes such as the poly-N-acetyllactosamine biosynthetic process, glycosphingolipid biosynthesis—lacto and neglect series process. This suggests that they may have an important role in tumor metabolism, to be explored in further studies.
CDH3, a cell adhesion molecule, is associated with the function of cells to bind with other cells and the extracellular matrix (ECM). CDH3 is overexpressed in many malignancies (Kaupmann et al., 1992). In our study, it was also found to be overexpressed in EGFR-MT LUAD. Hsiao et al. (2020b) found that CDH3 overexpression is related to the patients’ EGFR-TKI resistance, and reducing the expression level of CDH3 can increase the sensitivity of EGFR-TKI in patients. Moreover, sCDH3 was positively associated with the tumor stage in non–small-cell lung cancer, although it has not been found to have a significant effect on the prognosis in our study. But these genes’ significance on the metastasis and invasion of EGFR-MT LUAD still needs to be further studied.
CST1 belongs to the type 2 cystatin superfamily, which restricts the proteolytic activities of cysteine proteases. It has been found correlated with multiple tumor metastasis and invasion (Cui et al., 2019). Dai et al. (2017) found that the OS in the low CST1 expression subgroup was significantly superior to the high CST1 expression subgroup. In our study, we found that it is highly expressed in patients with EGFR-MT LUAD, but its effect on the prognosis of patients needs further research to confirm ZBTB16, a member of the Kruppel C2H2-type zinc finger protein family and encodes a zinc finger transcription factor that contains nine Kruppel-type zinc finger domains at the carboxyl terminus. This protein is located in the nucleus, is involved in cell cycle progression, and interacts with a histone deacetylase (Furukawa et al., 2003). Some studies have found that it can be used as a prognostic evaluation marker and potential therapeutic target in reproductive system tumors and Ewing’s sarcoma (Xiao et al., 2016; Xiao et al., 2019), but its role in lung cancer needs further study.
KRT15 is an encoding protein which belongs to the keratin gene family. It has been found to be highly expressed in colon cancer, breast cancer, gastric cancer, and other tumors and has prognostic value (Zhang et al., 2019; Rao et al., 2020; Xu et al., 2020). Ooi et al. (2010) found that this gene is positively expressed in smoking patients with non–small-cell lung cancer and has prognostic value. Its abnormal expression can lead to abnormal airway epithelial damage and repair function, thereby promoting the development of lung cancer.
KLB is a protein-coding gene and mediates binding of fibroblast growth factor (FGF) 21 to the FGF receptor (FGFR). FGF21-KLB-FGFR signaling regulates multiple metabolic systems in the liver (Ji et al., 2019). Andrew et al. (Thompson et al., 2020) found that it is closely related to the increase in the incidence of lung cancer caused by heavy drinking. At the same time, Zhou et al. (2021) found that serum KLB concentration can be used to predict the clinical outcome of NSCLC patients, although in our study, it was found to have an effect on the prognosis of patients. However, more patient omics data are expected to reveal its clinical significance.
As with all research, our work has several limitations. Although we provide a comprehensive bioinformatics analysis to determine the potential diagnostic genes between cancer and normal tissues, it may not be very accurate in evaluating EGFR-MT LUAD patients at every stage. Also, the molecular mechanism of survival-related genes involved in affecting the prognosis of patients with EGFR-MT LUAD needs to be further verified through a series of experiments. In conclusion, our work discovered the important survival-related gene B3GNT3 that can forecast prognosis in EGFR-MT LUAD by combining WGCNA with differential gene expression analysis.
Data Availability Statement
Publicly available datasets were analyzed in this study. These data can be found here: GSE31210 GSE19188 https://portal.gdc.cancer.gov/.
Author Contributions
HZ and DL is responsible for paper writing and data analysis. QL and FL are responsible for paper revision and writing, JZ briefly revised the article and ZW assists in data analysis. JW and XL were responsible for the paper’s overall design, review, and revision.
Funding
This work was supported by National Key Research and Development Plan (2020YFC2002706-2); 2019 the Health Care Special Scientific Research Project (19BJZ28); New business support project of PLA General Hospital (XYW-202107); Scientific Research and Cultivation Program for Health Development of Haidian District, Beijing (HP2021-19-80304).
Conflict of Interest
The authors declare that the research was conducted in the absence of any commercial or financial relationships that could be construed as a potential conflict of interest.
Publisher’s Note
All claims expressed in this article are solely those of the authors and do not necessarily represent those of their affiliated organizations, or those of the publisher, the editors, and the reviewers. Any product that may be evaluated in this article, or claim that may be made by its manufacturer, is not guaranteed or endorsed by the publisher.
Supplementary Material
The Supplementary Material for this article can be found online at: https://www.frontiersin.org/articles/10.3389/fgene.2021.755245/full#supplementary-material
Supplementary Figure S1 | In the TCGA dataset, modules correlated with the clinical trait were identified. (A) Co-expression network module cluster dendrogram. Each module was given its color scheme. (B) Relationships between modules and traits. Each row represents a color module, and each column represents a clinical characteristic (tumor and normal).
Supplementary Figure S2 | Identification of modules in the GSE31210 that are related to the clinical trait. (A) Co-expression network module cluster dendrogram. Different colors were applied to each module. (B) Relationships between modules and traits. Each row represents a color module, and each column represents a clinical characteristic (tumor and normal).
References
Barkley, S., Chugh, S., Karmakar, S., Kaushik, G., Rauth, S., Rachagani, S., et al. (2018). Novel Role of O-Glycosyltransferases GALNT3 and B3GNT3 in the Self-Renewal of Pancreatic Cancer Stems Cells. BMC Cancer 18 (1), 1157. doi:10.1186/s12885-018-5074-2
Bu, D., Xia, Y., Zhang, J., Cao, W., Huo, P., Wang, Z., et al. (2020). FangNet: Mining Herb Hidden Knowledge from TCM Clinical Effective Formulas Using Structure Network Algorithm. Comput. Struct. Biotechnol. J. 19, 62–71. doi:10.1016/j.csbj.2020.11.036
Cao, X., Li, Y., Luo, R.-Z., Zhang, L., Zhang, S.-L., Zeng, J., et al. (2015). Expression of Cystatin SN Significantly Correlates with Recurrence, Metastasis and Survival Duration in Surgically Resected Non-small Cell Lung Cancer Patients. Sci. Rep. 5, 8230. doi:10.1038/srep08230
Chen, H., and Boutros, P. C. (2011). VennDiagram: a Package for the Generation of Highly-Customizable Venn and Euler Diagrams in R. BMC Bioinformatics 12, 35. doi:10.1186/1471-2105-12-35
Cui, Y., Sun, D., Song, R., Zhang, S., Liu, X., Wang, Y., et al. (2019). Upregulation of Cystatin SN Promotes Hepatocellular Carcinoma Progression and Predicts a Poor Prognosis. J. Cel Physiol 234 (12), 22623–22634. doi:10.1002/jcp.28828
Dai, D.-n., Li, Y., Chen, B., Du, Y., Li, S.-b., Lu, S.-x., et al. (2017). Elevated Expression of CST1 Promotes Breast Cancer Progression and Predicts a Poor Prognosis. J. Mol. Med. 95 (8), 873–886. doi:10.1007/s00109-017-1537-1
Devanagari, S., Morgensztern, D., and Govindan, R. (2015). Genomic Alterations in Lung Adenocarcinoma. Lancet Oncol. 16 (7), e342–51.
Furukawa, M., He, Y. J., Borchers, C., and Xiong, Y. (2003). Targeting of Protein Ubiquitination by BTB-Cullin 3-Roc1 Ubiquitin Ligases. Nat. Cel Biol 5 (11), 1001–1007. doi:10.1038/ncb1056
Gao, L., Zhang, H., Zhang, B., Zhu, J., Chen, C., and Liu, W. (2018). B3GNT3 Overexpression Is Associated with Unfavourable Survival in Non-small Cell Lung Cancer. J. Clin. Pathol. 71 (7), 642–647. doi:10.1136/jclinpath-2017-204860
Gao, M., Yi, J., Zhu, J., Minikes, A. M., Monian, P., Thompson, C. B., et al. (2019). Role of Mitochondria in Ferroptosis. Mol. Cel 73 (2), 354–363. doi:10.1016/j.molcel.2018.10.042
Hanahan, D., and Coussens, L. M. (2012). Accessories to the Crime: Functions of Cells Recruited to the Tumor Microenvironment. Cancer Cell 21, 309–322. doi:10.1016/j.ccr.2012.02.022
Ho, W.-L., Che, M.-I., Chou, C.-H., Chang, H.-H., Jeng, Y.-M., Hsu, W.-M., et al. (2013). B3GNT3 Expression Suppresses Cell Migration and Invasion and Predicts Favorable Outcomes in Neuroblastoma. Cancer Sci. 104 (12), 1600–1608. doi:10.1111/cas.12294
Hou, J., Aerts, J., den Hamer, B., van IJcken, W., den Bakker, M., Riegman, P., et al. (2010). Gene Expression-Based Classification of Non-small Cell Lung Carcinomas and Survival Prediction. PLoS One 5 (4), e10312. doi:10.1371/journal.pone.0010312
Hsiao, T. F., Wang, C. L., Wu, Y. C., Feng, H. P., Chiu, Y. C., Lin, H. Y., et al. (2020). Integrative Omics Analysis Reveals Soluble Cadherin-3 as a Survival Predictor and an Early Monitoring Marker of EGFR Tyrosine Kinase Inhibitor Therapy in Lung Cancer. Clin. Cancer Res. 26 (13), 3220–3229. doi:10.1158/1078-0432.CCR-19-3972
Hsiao, T. F., Wang, C. L., Wu, Y. C., Feng, H. P., Chiu, Y. C., Lin, H. Y., et al. (2020). Integrative Omics Analysis Reveals Soluble Cadherin-3 as a Survival Predictor and an Early Monitoring Marker of EGFR Tyrosine Kinase Inhibitor Therapy in Lung Cancer. Clin. Cancer Res. 26 (13), 3220–3229. doi:10.1158/1078-0432.CCR-19-3972
Hsu, W.-H., Yang, J. C.-H., Mok, T. S., and Loong, H. H. (2018). Overview of Current Systemic Management of EGFR-Mutant NSCLC. Ann. Oncol. 29 (Suppl. l_1), i3–i9. doi:10.1093/annonc/mdx702
Ji, F., Liu, Y., Hao, J.-G., Wang, L.-P., Dai, M.-J., Shen, G.-F., et al. (2019). KLB Gene Polymorphism Is Associated with Obesity and Non-alcoholic Fatty Liver Disease in the Han Chinese. Aging 11 (18), 7847–7858. doi:10.18632/aging.102293
Kaupmann, K., Becker-Follmann, J., Scherer, G., Jockusch, H., and Starzinski-Powitz, A. (1992). The Gene for the Cell Adhesion Molecule M-Cadherin Maps to Mouse Chromosome 8 and Human Chromosome 16q24.1-qter and Is Near the E-Cadherin (Uvomorulin) Locus in Both Species. Genomics 14 (2), 488–490. doi:10.1016/s0888-7543(05)80247-2
Langfelder, P., and Horvath, S. (2008). WGCNA: An R Package for Weighted Correlation Network Analysis. BMC Bioinformatics 9, 559. doi:10.1186/1471-2105-9-559
Li, C.-W., Lim, S.-O., Chung, E. M., Kim, Y.-S., Park, A. H., Yao, J., et al. (2018). Eradication of Triple-Negative Breast Cancer Cells by Targeting Glycosylated PD-L1. Cancer Cell 33 (2), 187–201. doi:10.1016/j.ccell.2018.01.009
Liu, X., Wang, P., Zhang, C., and Ma, Z. (2017). Epidermal Growth Factor Receptor (EGFR): A Rising star in the Era of Precision Medicine of Lung Cancer. Oncotarget 8 (30), 50209–50220. doi:10.18632/oncotarget.16854
Luo, W., Tian, P., Wang, Y., Xu, H., Chen, L., Tang, C., et al. (2018). Characteristics of Genomic Alterations of Lung Adenocarcinoma in Young Never‐smokers. Int. J. Cancer 143 (7), 1696–1705. doi:10.1002/ijc.31542
Maity, B., Sheff, D., and Fisher, R. A. (2013). Immunostaining. Methods Cel Biol 113, 81–105. doi:10.1016/b978-0-12-407239-8.00005-7
Nahum, R., Zhai, W., and Zhang, T. (2018). Elucidating Genomic Architecture of Asian EGFR-Mutant Lung Adenocarcinoma through Multi-Region Exon Sequencing. Nat. Commun. 9 (1), 216.
O'Farrell, H., Harbourne, B., Kurlawala, Z., Inoue, Y., Nagelberg, A. L., Martinez, V. D., et al. (2019). Integrative Genomic Analyses Identifies GGA2 as a Cooperative Driver of EGFR-Mediated Lung Tumorigenesis. J. Thorac. Oncol. 14 (4), 656–671. doi:10.1016/j.jtho.2018.12.004
Okinawa, H., Kohno, T., Ishii, Y., Shimada, Y., Shiraishi, K., Iwakawa, R., et al. (2012). Identification of Genes up Regulated in ALK-Positive and EGFR/KRAS/ALK-negative Lung Adenocarcinomas. Cancer Res. 72 (1), 100–111. doi:10.1158/0008-5472.CAN-11-1403
Ooi, A. T., Mah, V., Nickerson, D. W., Gilbert, J. L., Ha, V. L., Hegab, A. E., et al. (2010). Presence of a Putative Tumor-Initiating Progenitor Cell Population Predicts Poor Prognosis in Smokers with Non-small Cell Lung Cancer. Cancer Res. 70 (16), 6639–6648. doi:10.1158/0008-5472.can-10-0455
Qiu, G.-Z., Jin, M.-Z., Dai, J.-X., Sun, W., Feng, J.-H., and Jin, W.-L. (2017). Reprogramming of the Tumor in the Hypoxic Niche: The Emerging Concept and Associated Therapeutic Strategies. Trends Pharmacol. Sci. 38, 669–686. doi:10.1016/j.tips.2017.05.002
Rao, X., Wang, J., Song, H. M., Deng, B., and Li, J. G. (2020). KRT15 Overexpression Predicts Poor Prognosis in Colorectal Cancer. Neoplasma 67 (2), 410–414. doi:10.4149/neo_2019_190531N475
Ritchie, M. E., Phipson, B., Wu, D., Hu, Y., Law, C. W., Shi, W., et al. (2015). Limma powers Differential Expression Analyses for RNA-Sequencing and Microarray Studies. Nucleic Acids Res. 43, e47. doi:10.1093/nar/gkv007
Thompson, A., Cook, J., Choquet, H., Jorgenson, E., Yin, J., Kinnunen, T., et al. (2020). Functional Validity, Role, and Implications of Heavy Alcohol Consumption Genetic Loci. Sci. Adv. 6 (3), eaay5034. doi:10.1126/sciadv.aay5034
Thul, P. J., and Lindskog, C. (2018). The Human Protein Atlas: a Spatial Map of the Human Proteome. Protein Sci. 27, 233–244. doi:10.1002/pro.3307
Wang, X., Wang, L., Guo, S., Bao, Y., Ma, Y., Yan, F., et al. (2013). Hypermethylation Reduces Expression of Tumor‐suppressor PLZF and Regulates Proliferation and Apoptosis in Non‐small‐cell Lung Cancers. FASEB j. 27 (10), 4194–4203. doi:10.1096/fj.13-229070
Xiao, G.-Q., Li, F., Findeis-Hosey, J., Hyrien, O., Unger, P. D., Xiao, L., et al. (2015). Down-regulation of Cytoplasmic PLZF Correlates with High Tumor Grade and Tumor Aggression in Non-small Cell Lung Carcinoma. Hum. Pathol. 46 (11), 1607–1615. doi:10.1016/j.humpath.2015.06.021
Xiao, G.-Q., Li, F., Unger, P. D., Katerji, H., Yang, Q., McMahon, L., et al. (2016). ZBTB16: a Novel Sensitive and Specific Biomarker for Yolk Sac Tumor. Mod. Pathol. 29 (6), 591–598. doi:10.1038/modpathol.2016.46
Xiao, G.-Q., Sherrod, A. E., and Hurth, K. M. (2019). ZBTB16: A New Biomarker for Primitive Neuroectodermal Tumor Element/Ewing Sarcoma. Pathol. - Res. Pract. 215 (10), 152536. doi:10.1016/j.prp.2019.152536
Xu, Y.-H., Deng, J.-L., Wang, L.-P., Zhang, H.-B., Tang, L., Huang, Y., et al. (2020). Identification of Candidate Genes Associated with Breast Cancer Prognosis. DNA Cel Biol. 39 (7), 1205–1227. doi:10.1089/dna.2020.5482
Yamauchi, M., Yamaguchi, R., Nakata, A., Kohno, T., Nagasaki, M., Shimamura, T., et al. (2012). Epidermal Growth Factor Receptor Tyrosine Kinase Defines Critical Prognostic Genes of Stage I Lung Adenocarcinoma. PLoS One 7 (9), e43923. doi:10.1371/journal.pone.0043923
Yang, D., Liu, Y., Bai, C., Wang, X., and Powell, C. A. (2020). Epidemiology of Lung Cancer and Lung Cancer Screening Programs in China and the United States. Cancer Lett. 468, 82–87. doi:10.1016/j.canlet.2019.10.009
Yang, S. R., Schultheis, A. M., Yu, H., Mandelker, D., Ladanyi, M., and Büttner, R. (2020). Precision Medicine in Non-small Cell Lung Cancer: Current Applications and Future Directions. Semin. Cancer Biol. S1044-579X (20), 30164–4. doi:10.1016/j.semcancer.2020.07.009
Yu, G., Wang, L.-G., Han, Y., and He, Q.-Y. (2012). ClusterProfiler: An R Package for Comparing Biological Themes Among Gene Clusters. OMICS: A J. Integr. Biol. 16 (5), 284–287. doi:10.1089/omi.2011.0118
Zeng, X., Zhao, J., Wu, X., Shi, H., Liu, W., Cui, B., et al. (2016). PageRank Analysis Reveals Topologically Expressed Genes Correspond to Psoriasis and Their Functions Are Associated with Apoptosis Resistance. Mol. Med. Rep. 13 (5), 3969–3976. doi:10.3892/mmr.2016.4999
Zhang, C., Liang, Y., Ma, M.-H., Wu, K.-Z., and Dai, D.-Q. (2019). KRT15, INHBA, MATN3, and AGT Are Aberrantly Methylated and Differentially Expressed in Gastric Cancer and Associated with Prognosis. Pathol. - Res. Pract. 215 (5), 893–899. doi:10.1016/j.prp.2019.01.034
Zhang, W., Hou, T., Niu, C., Song, L., and Zhang, Y. (2015). B3GNT3 Expression Is a Novel Marker Correlated with Pelvic Lymph Node Metastasis and Poor Clinical Outcome in Early-Stage Cervical Cancer. PLoS One 10 (12), e0144360. doi:10.1371/journal.pone.0144360
Keywords: EGFR–mutant lung adenocarcinoma, prognosis, WGCNA, TCGA, GEO
Citation: Zhang H, Lu D, Li Q, Lu F, Zhang J, Wang Z, Lu X and Wang J (2021) Identification of Six Prognostic Genes in EGFR–Mutant Lung Adenocarcinoma Using Structure Network Algorithms. Front. Genet. 12:755245. doi: 10.3389/fgene.2021.755245
Received: 08 August 2021; Accepted: 25 October 2021;
Published: 16 November 2021.
Edited by:
Dechao Bu, Institute of Computing Technology (CAS), ChinaReviewed by:
Jing Wang, University of Texas MD Anderson Cancer Center, United StatesQi Liao, Ningbo University, China
Copyright © 2021 Zhang, Lu, Li, Lu, Zhang, Wang, Lu and Wang. This is an open-access article distributed under the terms of the Creative Commons Attribution License (CC BY). The use, distribution or reproduction in other forums is permitted, provided the original author(s) and the copyright owner(s) are credited and that the original publication in this journal is cited, in accordance with accepted academic practice. No use, distribution or reproduction is permitted which does not comply with these terms.
*Correspondence: Xuechun Lu, luxuechun@126.com; Jinliang Wang, wangjinliang301@163.com
†These authors have contributed equally to this work