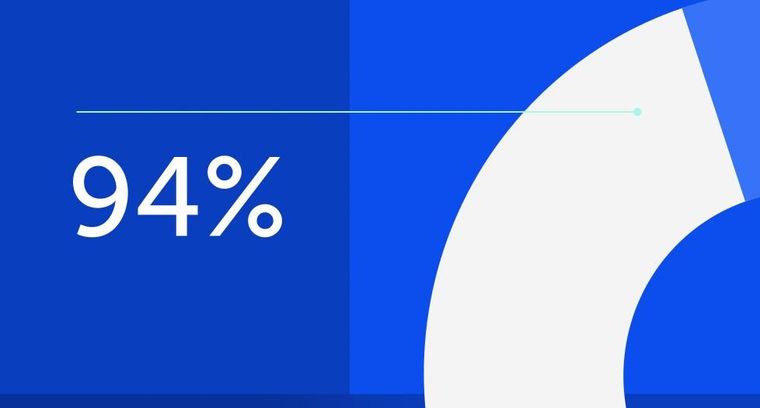
94% of researchers rate our articles as excellent or good
Learn more about the work of our research integrity team to safeguard the quality of each article we publish.
Find out more
ORIGINAL RESEARCH article
Front. Genet., 18 October 2021
Sec. Livestock Genomics
Volume 12 - 2021 | https://doi.org/10.3389/fgene.2021.753839
This article is part of the Research TopicGene Regulation Explored by Systems Biology in Livestock ScienceView all 13 articles
A correction has been applied to this article in:
Corrigendum: Integrated Network Analysis to Identify Key Modules and Potential Hub Genes Involved in Bovine Respiratory Disease: A Systems Biology Approach
Background: Bovine respiratory disease (BRD) is the most common disease in the beef and dairy cattle industry. BRD is a multifactorial disease resulting from the interaction between environmental stressors and infectious agents. However, the molecular mechanisms underlying BRD are not fully understood yet. Therefore, this study aimed to use a systems biology approach to systematically evaluate this disorder to better understand the molecular mechanisms responsible for BRD.
Methods: Previously published RNA-seq data from whole blood of 18 healthy and 25 BRD samples were downloaded from the Gene Expression Omnibus (GEO) and then analyzed. Next, two distinct methods of weighted gene coexpression network analysis (WGCNA), i.e., module–trait relationships (MTRs) and module preservation (MP) analysis were used to identify significant highly correlated modules with clinical traits of BRD and non-preserved modules between healthy and BRD samples, respectively. After identifying respective modules by the two mentioned methods of WGCNA, functional enrichment analysis was performed to extract the modules that are biologically related to BRD. Gene coexpression networks based on the hub genes from the candidate modules were then integrated with protein–protein interaction (PPI) networks to identify hub–hub genes and potential transcription factors (TFs).
Results: Four significant highly correlated modules with clinical traits of BRD as well as 29 non-preserved modules were identified by MTRs and MP methods, respectively. Among them, two significant highly correlated modules (identified by MTRs) and six nonpreserved modules (identified by MP) were biologically associated with immune response, pulmonary inflammation, and pathogenesis of BRD. After aggregation of gene coexpression networks based on the hub genes with PPI networks, a total of 307 hub–hub genes were identified in the eight candidate modules. Interestingly, most of these hub–hub genes were reported to play an important role in the immune response and BRD pathogenesis. Among the eight candidate modules, the turquoise (identified by MTRs) and purple (identified by MP) modules were highly biologically enriched in BRD. Moreover, STAT1, STAT2, STAT3, IRF7, and IRF9 TFs were suggested to play an important role in the immune system during BRD by regulating the coexpressed genes of these modules. Additionally, a gene set containing several hub–hub genes was identified in the eight candidate modules, such as TLR2, TLR4, IL10, SOCS3, GZMB, ANXA1, ANXA5, PTEN, SGK1, IFI6, ISG15, MX1, MX2, OAS2, IFIH1, DDX58, DHX58, RSAD2, IFI44, IFI44L, EIF2AK2, ISG20, IFIT5, IFITM3, OAS1Y, HERC5, and PRF1, which are potentially critical during infection with agents of bovine respiratory disease complex (BRDC).
Conclusion: This study not only helps us to better understand the molecular mechanisms responsible for BRD but also suggested eight candidate modules along with several promising hub–hub genes as diagnosis biomarkers and therapeutic targets for BRD.
Bovine respiratory disease (BRD) is the most common and costly infectious disease in the beef and dairy cattle industry. It causes 70–80% morbidity and 40–50% mortality in feedlot cattle in the United States (Edwards, 2010; Tizioto et al., 2015). BRD is a multifactorial disease, and its onset is usually associated with stress factors (nutritional or environmental risk factors) and the presence of infectious agents (Gagea et al., 2006; Grissett et al., 2015). Stress factors such as weaning, shipping distance, and commingling that negatively affect the immune system, can predispose cattle to a primary infection (Snowder et al., 2006; Timsit et al., 2016b). Infection is commonly caused by bovine respiratory disease complex (BRDC) including the viral and bacterial pathogens, which can affect the upper and lower respiratory system (Ellis, 2001; Caswell, 2014; Kirchhoff et al., 2014). Clinical diagnosis of BRD is made by visual observations and is usually based on clinical signs such as high rectal temperature, depression/lethargy, nasal or ocular discharge, increased respiration rate, reduced feed intake, and reduced average daily gain (Amrine et al., 2013; Behura et al., 2017). However, this method has low detection sensitivity and specificity, and the diagnosis is often made without identifying the cause of the disease (White and Renter, 2009; Timsit et al., 2016a). On the other hand, among the animals that are vaccinated against BRD, approximately 75% of them are protected (Hodgins et al., 2002), and the animals that are diagnosed based on clinical signs are treated with antimicrobials (Wilkinson, 2009). Moreover, excessive use of antimicrobial therapies for BRD facilitates the antibiotic resistance of microbes (Schaefer et al., 2007; Portis et al., 2012). In addition to vaccination and antimicrobials, other intervention methods such as nutritional manipulation and processing procedures have a limited effect on reducing morbidity and mortality rates, and despite extensive studies, BRD is still an issue (Taylor et al., 2010). Although the predisposing factors, viral and bacterial agents that cause BRD are relatively well known, the pathogenic mechanisms of BRD, the molecular immune response of the host to infection, and their association with the disease outcomes are not fully understood yet (Taylor et al., 2010; Johnston et al., 2019). Also, due to insufficient knowledge of the disease mechanisms, it is not possible to develop an effective method to identify animals with BRD (Sun et al., 2020). Therefore, understanding the infection dynamics and identification of new candidate biomarkers involved in BRD can help to better understanding the molecular mechanisms of BRD.
Functional genomic methods such as RNA-sequencing-based transcriptomics can provide a global gene expression profile, and their use in BRD studies can accelerate the understanding of disease mechanisms and the development of diagnosis (Rai et al., 2015). Several transcriptome studies have been performed on BRD in various tissues, such as the lung, bronchial lymph nodes (Behura et al., 2017; Johnston et al., 2019), and whole blood (Jiminez et al., 2021; Scott et al., 2021). For example, Sun et al. (2020) reported differentially expressed genes (DEGs) in the blood tissue as biomarkers to recognize BRD cattle at entry, such as MX1, IFIT3, ISG15, OAS2, and IFI6 involved in the interferon signaling pathway. Moreover, Tizioto et al. (2015) identified 142 DEGs that were located in quantitative trait locus regions associated with BRD risk. The most common application of differential expression analysis is to identify DEGs between different conditions. On the other hand, it is known that the differential expression analysis focuses only on the individual effect of genes. However, genes and gene products do not work individually but interact in complex gene networks (Liu et al., 2020). Therefore, individual evaluation of gene expression may not explain the cause of complex diseases such as BRD.
Systems biology is one of the suitable methods to better understand the mechanism of diseases (Darzi et al., 2021) and other complex traits (Salleh et al., 2018). In systems biology, there are various computational methods based on the network approach, and one of the fundamental aspects of the network approach in systems biology is the construction of gene coexpression networks using high-throughput gene expression data (Kadarmideen and Watson-Haigh, 2012). In this regard, one of the most widely used methods for building gene coexpression networks is weighted gene coexpression network analysis (WGCNA) (Langfelder and Horvath, 2008). The WGCNA method identifies clusters of coexpressed genes (also called a module) based on correlation patterns between expression profiles of genes across samples (Langfelder and Horvath, 2008). Furthermore, the WGCNA identifies highly connected genes (hub genes) by calculating intramodular gene connectivity, which are centrally in their modules and can be involved in important roles during the disease (Bakhtiarizadeh et al., 2018). The WGCNA approach has been used successfully in different disease studies in humans (Wang Y. et al., 2020), cattle (Yan et al., 2020), swine (Wilkinson et al., 2016), mice (Rangaraju et al., 2018), chickens (Liu and Cai, 2017), and sheep (Kadarmideen et al., 2011). One of the most widely used methods of WGCNA is module–trait relationship analysis. In this method, after identifying the modules across samples, module–trait relationships are calculated according to the correlation between module eigengenes and traits of interest, and finally, significant modules are identified (Kommadath et al., 2014; Sabino et al., 2018). Moreover, WGCNA provides another unique network-based method called module preservation analysis. This method focuses on determining network topology changes across different conditions. For example, it can be checked whether the network density and topological pattern of the modules identified in normal samples (as a reference) are preserved in the disease samples (as a test) (Langfelder et al., 2011). In this regard, the differences in the topology of these two networks indicate a significant perturbation of the coexpression patterns by the disease. Thus, the nonpreserved modules between these conditions, as well as their hub genes may exert crucial roles in the pathological processes of the disease (Mukund and Subramaniam, 2015; Riquelme Medina and Lubovac-Pilav, 2016; Bakhtiarizadeh et al., 2020).
In the present study, we used previously published RNA-seq data (Jiminez et al., 2021) and the WGCNA method for constructing weighted gene coexpression networks to better understand the molecular regulatory mechanisms responsible for the immune response to BRD. It should be noted that in the current study we used two distinct WGCNA methods to identify key modules, their hub genes, hub–hub genes, and regulatory factors involved in BRD: 1) module–trait relationships analysis across all samples to identify significant highly correlated modules with clinical traits of BRD, and 2) module preservation analysis between healthy samples (as reference set) and BRD samples (as test set) to identify nonpreserved modules between these conditions. The main hypothesis was that significant highly correlated modules with clinical traits of BRD (identified by the first method) and nonpreserved modules between healthy and BRD samples (identified by the second method) may contain potential functionally related genes and identifies biological regulatory systems involved in pathological processes of BRD, and it is also expected that these two methods confirm each other’s results.
RNA sequencing data from feedlot cattle with and without BRD were obtained from the Gene Expression Omnibus (GEO) database at the National Center for Biotechnology Information (NCBI) under the accession number of GSE162156. Moreover, clinical traits of BRD were obtained from the supplementary material section of the original paper (Jiminez et al., 2021) and then filtered for useful measurements. The data included samples from the whole blood of 25 and 18 mixed-breed beef heifers with and without BRD, respectively. An Illumina HiSeq 4000 platform was used to generate 43 paired-end (2 × 100 bp) libraries that included 18 healthy and 25 BRD samples. More information about the data can be found in the original paper (Jiminez et al., 2021).
Quality control of the raw sequencing data was performed using FastQC1 (version 0.11.9). Raw reads were then trimmed by removing low-quality bases and adaptor sequences using the Trimmomatic software (version 0.39) (Bolger et al., 2014) with the following options: ILLUMINACLIP: Adapter. fa:2:30:10, LEADING:20, TRAILING:20, and MINLEN:60. To confirm quality improvements, the clean reads were checked again using FastQC. Then the Hisat2 (version 2.2.1) (Kim et al., 2015) software was used for aligning the clean reads to the latest bovine reference genome (ARS-UCD1.2) with default parameters. To calculate counts of uniquely mapped reads to annotated genes based on the bovine GTF file (release 104), the python script HTSeq-count (version 0.13.5) (Anders et al., 2014) was applied using intersection-strict mode. All count files were then merged and finally, a raw gene expression matrix was created containing read counts information of all genes for all samples.
Raw gene expression matrix obtained from the previous steps was normalized to log-counts per million (log-cpm) using the “voom” function of the limma package (version 3.46.0) (Smyth, 2005). This normalization method opens access for the gene expression data generated by the RNA-seq analysis, to various computational methods, such as WGCNA (Law et al., 2014). Because the low-expressed or low-variance genes usually represent sampling noise and correlations based on these genes are not significant, genes with <1 CPM (counts per million) in at least five samples were removed. In addition, genes with a standard deviation >0.25 were selected for further analysis. Weighted gene coexpression network analysis was performed based on the functions of the WGCNA R package (version 1.70) (Langfelder and Horvath, 2008).
To identify significant highly correlated modules with clinical traits of BRD, all 43 samples (18 healthy and 25 BRD) were used for module–trait relationships (MTRs) analysis. Because the gene coexpression analysis is very sensitive to outliers, the distance-based adjacency metrics of samples was calculated and samples with a standardized connectivity < −2.5 were removed, considered as an outlier. In addition, samples and genes with >50% missing entries and genes with zero variance were identified and excluded from the WGCNA analysis. In this study, a signed weighted coexpression network was constructed in which correlation values between 0 and 1 are considered and values <0.50 are considered as negative correlation, and values >0.50 are considered as positive correlation (van Dam et al., 2017). Signed networks considers only positively correlated genes, and especially, network construction based on this method leads to more significantly enriched modules (Mason et al., 2009). Furthermore, bi-weight mid-correlation coefficient was used for the coexpression network construction since it is more robust to outliers in comparison to the Pearson correlation (Zheng et al., 2014). Briefly, a correlation matrix of expression values was constructed using pairwise bi-weight mid-correlation coefficients between all pairs of genes across the selected samples. Then, the correlation matrix at β = 10 as a soft threshold (power) was transformed into weighted adjacency matrix. Subsequently, the weighted adjacency matrix was transformed into topological overlap matrix (TOM), which considers each pairs of genes concerning all other genes by comparing their connections with all other genes in the network (interconnectedness). In other words, the genes in a module share strong interconnectedness (Zhang and Horvath, 2005; Li and Horvath, 2006; Yip and Horvath, 2007). Finally, average linkage hierarchical clustering analysis was performed by the topological overlap-based dissimilarity matrix (1-TOM) as input, and modules were identified by dynamic hybrid tree cutting algorithm. Then the modules with the highly correlated eigengenes were merged. The above steps were performed using automatic, one-step network construction and module detection function “blockwiseModules” of the WGCNA R package with the following parameters: power = 10, corType = “bicor,” maxBlockSize = 12,000, networkType = “signed,” TOMType = “signed,” minModuleSize = 30, reassignThreshold = 0, and mergeCutHeight = 0.25. Next, in order to identify the BRD-related modules, the correlation between the clinical traits of BRD and module eigengenes (the first principal component of the expression matrix for a given module) was taken using Pearson correlation coefficient. The cutoff of significant moderately or highly correlated modules with clinical traits of BRD was defined as p-value < 0.05 and 0.30 < |R| < 0.50, and p-value < 0.05 and |R| > 0.50, respectively. Moreover, gene significance (GS), which is a criterion for biological association of a gene with an interest trait was calculated for each gene through the correlation between gene expression profile and clinical traits of BRD.
In this method, based on the assumption that BRD may cause a topological change in the coexpression patterns of the healthy samples, and that nonpreserved modules between the healthy and disease samples may be biologically related to BRD, the healthy samples (n = 18) were selected as a reference set for construction the coexpression network and modules detection. So, after outlier detection, removing them, and set β = 13 as a soft threshold, automatic module detection function “blockwiseModules” of the WGCNA was used for a signed network construction, as well as identification of modules in healthy samples with following parameters: networkType = “signed,” TOMType = “signed,” corType = “bicor,” mergeCutHeight = 0.25, power = 13, maxBlockSize = 12,000, minModuleSize = 30, and reassignThreshold = 0. After identifying the modules, module preservation analysis was performed using the “module Preservation” function of WGCNA R package to investigate whether the network density and connectivity patterns of the modules were preserved between the healthy and BRD samples. For this purpose, two composite preservation statistics were investigated using a permutation test (based on 200 random permutations). The first preservation composite statistic was Zsummary that was calculated from a combination of several preservation statistics, which investigated whether the mean connection strength among all genes in a module (known as network density) identified in the healthy samples remain highly connected in the disease samples and it also evaluates whether the sum of the connection strengths for a gene with other network genes (known as connectivity) in the healthy samples are similar in the disease samples (Langfelder et al., 2011). A higher value of Zsummary indicates strong preservation between conditions (healthy vs. BRD). However, Zsummary increases with increasing module size, Therefore, it is strongly dependent on the module size (Langfelder et al., 2011). The second preservation composite statistic used is medianRank, which is a module size-independent statistic; this rank-based measure relies on observed preservation statistics. Unlike Zsummary, modules with low medianRank values are highly preserved between conditions. In this study, modules with Zsummary > 10 and medianRank < 8, 5 < Zsummary ≤ 10 and medianRank < 8, and Zsummary ≤ 5 or medianRank ≥ 8 were considered as highly-preserved, semipreserved, and nonpreserved, respectively.
To determine which modules are biologically related to BRD, all genes in each module were analyzed for Gene Ontology (GO) and Kyoto Encyclopedia of Genes and Genomes (KEGG) pathways using the Enrichr web tool (Chen et al., 2013). The cutoffs of significant terms were defined as adjusted p-value <0.05 (correction by the Benjamini–Hochberg method). Moreover, to identify potential regulatory factors in the modules, the genes of each module were aligned to bovine transcription factors (TFs) set from the AnimalTFDB3.0 database (Hu et al., 2018).
Highly connected genes (hub genes) in a coexpression module are suitable candidates for explaining behavior and biological function of that module. In other words, highly connected intramodular hub genes have the highest degree of connection in a module and are central to modules in a network, and compared with other genes, they have more biological relevance to the module functions (van Dam et al., 2017). In this regard, module membership (MM) also known as eigengene-based connectivity kME for each gene was calculated by the WGCNA R package through the correlation between the gene expression profile and the module eigengenes. Next, this criterion was used to identify hub genes in the significant highly correlated modules with clinical traits of BRD that were identified by the MTRs method and nonpreserved modules that were identified by the MP method. In fact, the MM assesses how well the genes of a module correlate with the characteristics of that module. Genes with kME ≥ 0.7 were considered as highly connected hub genes in the respective modules. Furthermore, to investigate the connections of proteins encoded by the hub genes, Search Tool for the Retrieval of Interacting Genes (STRING) database (version 11.0) (Szklarczyk et al., 2018) was used and the protein–protein interaction (PPI) network of the hub genes was attained for further analysis.
For detection of the highly connected and central genes in the PPI network based on the hub genes (hub–hub genes), cytoHubba application (version 0.1) was used (Chin et al., 2014). This application is a cytoscape plugin and explores important genes and subnetworks in a given biological network such as the PPI network by several topological analysis methods including local-based and global-based methods. Local-based methods only considers the direct neighborhood of a gene, including degree (Deg), maximum neighborhood component (MNC), density of maximum neighborhood component (DMNC), clustering coefficient (CC), and maximal clique centrality (MCC) methods. Global-based methods focus on the shortest paths, such as closeness (Clo), eccentricity (EcC), radiality (Rad), bottleneck (BN), stress (Str), and betweenness (BC) methods (Chin et al., 2014). These 12 topological analysis methods were used separately to rank 60 important genes in each PPI network that were derived from the hub genes. Next, for rank aggregation of important genes lists, RankAggreg R package (version 0.6.6) was used based on cross-entropy (CE) algorithm and genetic algorithm (GA) (Pihur et al., 2009). Finally, the common genes between these two methods were considered as hub–hub genes. Significant highly correlated modules (identified by MTRs) and nonpreserved modules (identified by MP) that were biologically associated with BRD were visualized using Cytoscape (version 3.7.1) (Cline et al., 2007).
A summary for the RNA-seq data analysis pipeline and the steps for constructing the weighted gene coexpression network is presented in Figure 1. RNA-seq data included 43 samples (18 healthy and 25 BRD), and there was a mean of 31.781 million paired-end (2 × 100 bp) reads per sample. A total of 1.366 billion reads were analyzed and after trimming, a total of 1.352 billion clean reads were obtained (approximately 31.469 million clean reads per sample). On average, 95% of all clean reads were mapped to the bovine reference genome (ranging from 92 to 97%). Moreover, a mean of 82% of all clean reads were uniquely mapped to the bovine reference genome. The details about RNA-seq data, trimming, and mapping summary of all samples is provided in Supplementary Table S1. Finally, after applying various parameters to filter the low-expressed and low-variance genes, a total of 10,099 genes were further used in the WGCNA analysis. A list of filtered genes along with the normalized values of their expression is provided in Supplementary Table S2.
Figure 1. Schematic pipeline for RNA-seq data analysis and weighted gene coexpression network construction in this study.
To prevent the negative effects of outlier samples on gene coexpression network analysis, after identifying the outliers, two samples (GSM4943645 and GSM4943656) with a standardized connectivity score < −2.5 were removed (Figure 2A). The weighted adjacency matrix was constructed at β = 10 whose scale-free topology fitting index (R2) was ≥0.80 (Figure 2B). After network construction, 12 coexpression modules (excluding grey module with 690 uncorrelated genes) were identified through hierarchical clustering and dynamic hybrid tree cutting with an average size of 784 genes. The turquoise and tan modules were the largest and smallest module with 2,592 and 72 genes each, respectively (Supplementary Table S3). In Figure 3A, a clustering dendrogram is presented in which the branches represent the modules that are labeled with a specific color by the WGCNA R package. Clinical traits related to BRD that were used in MTRs included clinical signs measurements of BRD such as rectal temperature (°C), haptoglobin level (g/L), respiratory rate (per min), and average daily gain. The sample dendrogram and trait heatmap of clinical traits related to BRD across all samples are presented in Figure 3B. The results of MTRs indicate that the rectal temperature, haptoglobin level, average daily gain, and respiratory rate have eight, eight, six, and two significant modules, respectively. Among the significant modules are as follows: MEpurple (R = −0.63, p = 1e−05), MEblue (R = −0.71, p = 2e−07), MEbrown (R = −0.55, p = 2e−04), and MEturquoise (R = 0.7, p = 3e−07) modules were significantly highly correlated and MEyellow (R = −0.41, p = 0.008), MEtan (R = −0.32, p = 0.04), MEgreenyellow (R = 0.37, p = 0.02), and MEpink (R = −0.38, p = 0.01) modules were significantly moderately correlated with rectal temperature, respectively (Figure 3C). Also, MEpurple (R = −0.64, p = 8e−06), MEblue (R = −0.75, p = 1e−08), MEbrown (R = −0.55, p = 2e−04), and MEturquoise (R = 0.72, p = 1e−07) modules were significantly highly-correlated and MEyellow (R = −0.42, p = 0.006), MEtan (R = −0.31, p = 0.04), MEblack (R = 0.33, p = 0.04), and MEpink (R = −0.34, p = 0.03) modules were significantly moderately correlated with haptoglobin level, respectively (Figure 3C). Moreover, MEpurple (R = 0.64, p = 8e−06), MEblue (R = 0.53, p = 4e−04), MEbrown (R = 0.53, p = 4e−04), and MEturquoise (R = −0.59, p = 6e−05) modules were significantly highly correlated, and MEred (R = −0.34, p = 0.03) and MEgreen (R = 0.34, p = 0.03) modules were significantly moderately correlated with average daily gain, respectively (Figure 3C). The MEturquoise (R = 0.32, p = 0.04) and MEbrown (R = −0.32, p = 0.04) modules were significantly moderately correlated with respiratory rate, respectively (Figure 3C), but no significant highly correlated modules were found for this trait. Then, the significant highly correlated modules were selected for downstream analysis. Briefly, the turquoise, blue, brown, and purple modules with module sizes of 2,592, 1,691, 1,214, and 141 genes, respectively, were identified as significantly highly correlated modules with rectal temperature, haptoglobin level, and average daily gain (Figure 3C).
Figure 2. Sample clustering to detect outliers and network topology analysis. (A) All samples except GSM4943645 and GSM4943656 were clustered and then selected for module–trait relationships analysis. (B) Scale-free topology fitting index (left) and mean connectivity (right) for different soft-threshold powers (β). For module–trait relationships analysis, coexpression network was constructed at β = 10 whose scale-free topology fitting index (R2) was ≥0.80. (C) All samples except GSM4943661 and GSM4943667 were clustered and then selected for module preservation analysis. (D) Scale-free topology fitting index (left) and mean connectivity (right) for different soft-threshold powers (β). For module preservation analysis, co-expression network was constructed at β = 13 whose scale-free topology fitting index (R2) was >0.80.
Figure 3. Module–trait relationships analysis. (A) Gene hierarchical clustering dendrogram of 12 detected modules based on a dissimilarity (1-TOM) measure across all samples, the y-axis represents the coexpression distance and the x-axis represents the genes. The branches indicate the modules, and each module is marked with a separate color, the gray module encompass genes that are not assigned to any of the modules. (B) Sample dendrogram and trait heatmap of clinical traits related to BRD across all samples. The gradient from white to red indicates the low to high level of the respective traits in the samples. (C) Module–trait relationships between detected modules and clinical traits of BRD. Module–trait relationships are obtained by calculating the correlation between the traits and the module eigengenes. The red and blue colors indicate strong positive correlation and strong negative correlation, respectively. Rows represent module eigengene (ME) and columns indicate clinical traits of BRD. Rect. Temp, rectal temperature (°C); Haptog. Level, haptoglobin level (g/L); Resp. Rate, respiratory rate (per min), ADG, average daily gain. Asterisks corresponds to significant highly correlated values.
In order to understand the biological performance of the significant highly correlated modules with clinical traits of BRD, functional enrichment analysis was performed and a total of 356 biological process and 129 KEGG pathways were significantly enriched in the respective modules. The turquoise module had the highest number of enriched terms and pathways, including 305 biological processes and 116 KEGG pathways. The most significant GO term and KEGG pathway in the turquoise module were “neutrophil-mediated immunity” (GO:0002446, adjusted p-value = 7.45E−55) and “Lysosome” (adjusted p-value = 5.16E−13), respectively. On the other hand, 19 biological processes and 13 KEGG pathways were significantly enriched in the purple module. The most significant GO term and KEGG pathway in the purple module were “cellular defense response” (GO:0006968, adjusted p-value = 2.27E−09) and “natural killer cell-mediated cytotoxicity” (adjusted p-value = 2.09E−06), respectively. Only 32 biological processes were enriched in the blue module, and no biological process or KEGG pathway was significantly enriched in the brown module. The top 20 significant biological process terms for turquoise, blue, and purple modules are presented in Figure 4A. Moreover, the complete information of the functional enrichment analysis for the significant highly correlated modules with clinical traits of BRD is provided in Supplementary Table S4. Based on the functional enrichment analysis, among the significant highly correlated modules with clinical traits of BRD, turquoise and purple modules were associated with BRD mechanisms and host immune response. To identify potential TFs that may control transcription of coexpressed genes in the modules, a total number of 100 and 11 TFs were found in the turquoise and purple modules, respectively (Supplementary Table S5).
Figure 4. Functional enrichment analysis results. (A) The top 20 significant biological processes for significant highly correlated and biologically related modules to bovine respiratory disease (BRD). Color and size and each point represent −log2(FDR) and number of genes for each term, respectively. (B) The top 15 significant biological processes for significant enriched nonpreserved modules. Color and size and each point represent −log2(FDR) and number of genes for each term, respectively.
In this study, coexpressed genes in both turquoise and purple modules (as significant highly correlated as well as biologically related modules with BRD) were further evaluated. The MM versus GS plots of these modules are presented in Figure 5, which shows a strong correlation between GS and MM. In other words, the most significant genes with clinical traits of BRD are often the central genes in the respective modules. More information about GS for clinical traits of BRD can be found in Supplementary Table S6. Highly connected intramodular hub genes often have high levels of MM and may play an important role in BRD. So, in this regard, a total of 1,476 and 114 hub genes were identified in turquoise and purple modules, respectively (Supplementary Table S7). Then, the connection of proteins encoded by these hub genes in each module was examined and the PPI network related to the hub genes in turquoise and purple modules was obtained. Interestingly, PPI networks based on the hub genes in the turquoise and purple modules were of high density based on the STRING database information. The PPI network based on the hub genes in the turquoise module is presented in Figure 6. Hub–hub genes, which were highly connected in the respective coexpression modules and also are central genes in the hub genes-based PPI networks, can be considered as prognostic and therapeutic targets in BRD development. Here, a total of 42 and 23 hub–hub genes were found in turquoise and purple modules, respectively (Table 1).
Figure 5. Scatterplots of module membership (MM) versus gene significance (GS) plots. (A–D) module membership versus gene significance for rectal temperature, haptoglobin level, average daily gain, and respiratory rate in the turquoise module, respectively. (E–H) module membership versus gene significance for rectal temperature, haptoglobin level, average daily gain, and respiratory rate in the purple module, respectively.
Figure 6. Protein–protein interaction (PPI) network based on the hub genes of the turquoise module (identified by module–trait relationships method). Larger nodes and orange octagons represent hub–hub genes and transcription factors, respectively.
Table 1. List of the hub–hub genes in the turquoise and purple modules as significant highly correlated and biologically related modules with bovine respiratory disease (BRD) along with their module memberships (MM) and gene significance (GS) for rectal temperature (identified by module–trait relationships analysis).
For module preservation analysis, healthy samples were used as a reference set to construct the weighted gene coexpression network. Two samples, GSM4943661 and GSM4943667 were identified as outliers based on the distance adjacency metrics of samples and then removed (Figure 2C). For network construction, the soft threshold power was set to 13 which showed high scale-free topology (R2 > 0.80; Figure 2D). Using WGCNA, a total of 36 modules were identified in the healthy samples. The modules had different sizes ranging from 40 in the yellowgreen module to 1,163 in the turquoise module. In addition, the grey module contained 247 genes that were not assigned to any of the other modules (Supplementary Table S8). The identified modules in the healthy samples with different colors as branches of the hierarchical clustering dendrogram and the relationship between them are presented in Figures 7A,B, respectively. Next, for the module preservation analysis, we used the BRD samples as a test set to investigate whether the network density and connectivity pattern of the modules identified in the healthy samples were preserved in the BRD samples. The results of the preservation analysis showed that based on the thresholds set in the Materials and methods section, six modules, including lightgreen (Zsummary = 26, medianRank = 1), magenta (Zsummary = 24, medianRank = 5), pink (Zsummary = 20, medianRank = 7), grey60 (Zsummary = 19, medianRank = 2), midnightblue (Zsummary = 17, medianRank = 7), and darkred (Zsummary = 11, medianRank = 4), were identified as highly preserved modules, and one module, yellowgreen (Zsummary = 5.8, medianRank = 7) was identified as a semipreserved module (Figure 7C). Interestingly, in agreement with our hypothesis, of the 36 modules identified, 29 modules were non-preserved between healthy and BRD samples, indicating that their connectivity pattern and topological structure have been affected and changed by the BRD (Figure 7C). Skyblue (Zsummary = 1.5, medianRank = 36), darkmagenta (Zsummary = 2.1, medianRank = 34), and darkolivegreen (Zsummary = 3.6, medianRank = 24) modules were identified as the most non-preserved modules between healthy and BRD samples, respectively (Supplementary Table S9).
Figure 7. Module preservation analysis. (A) Gene hierarchical clustering dendrogram of 36 detected modules based on a dissimilarity (1-TOM) measure across healthy samples as reference set, the y-axis represents the coexpression distance and the x-axis represents the genes. The branches indicate the modules, and each module is marked with a separate color, the gray module encompass genes that are not assigned to any of the modules. (B) Eigengene adjacency heatmap indicate relationship among all the modules. (C) The medianRank preservation statistics of the modules. The y-axis and the x-axis represent medianRank values and module size, respectively. Each point indicates a module labeled by a respective color. The green dashed line represents the medianRank threshold (medianRank ≥8). (D) The Zsummary preservation statistics of the modules. The y-axis and the x-axis represent Zsummary values and module size, respectively. Each point indicates a module labeled by a respective color. The red dashed line represents the Zsummary threshold (Zsummary ≤5). Modules with Zsummary ≤5 or medianRank ≥8 were considered as nonpreserved between healthy and BRD conditions.
To understand the biological significance and the functional difference between highly preserved, semipreserved, and nonpreserved modules, all the 36 modules were subjected to GO terms and KEGG pathway analysis, and a total of 521 biological processes and 158 KEGG pathways were significantly enriched. Enrichment analysis of four highly preserved modules that included magenta, pink, grey60, and midnightblue revealed a total of 108 and 27 biological processes and KEGG pathways, respectively. Among them, the magenta module with 37 biological processes and 17 KEGG pathways was identified as the most significant enriched module. The other two highly preserved modules, including lightgreen and darkred, showed no biological process or KEGG pathway enrichment. Moreover, one biological process was enriched in the yellowgreen module as the only semipreserved module. The complete information of the functional enrichment analysis for the highly preserved and semipreserved modules is provided in Supplementary Table S10. On the other hand, among the nonpreserved module, 13 modules including blue, brown, cyan, darkmagenta, darkorange, green, greenyellow, lightcyan, paleturquoise, purple, red, salmon, and yellow were enriched in at least one biological process or KEGG pathway (significantly enriched nonpreserved modules). Furthermore, functional enrichment analysis of the significantly enriched nonpreserved modules identified a total of 412 biological processes and 131 KEGG pathways. The top 15 significant biological process terms for significantly enriched nonpreserved modules are presented in Figure 4B. In addition, the complete information of the functional enrichment analysis for the nonpreserved modules is provided in Supplementary Table S11. Based on the enrichment results, among the significantly enriched nonpreserved modules, six nonpreserved modules including blue, greenyellow, purple, red, salmon, and yellow were associated with pathogenic mechanisms of BRD and immune response. Also, based on the bovine transcription factors set from the AnimalTFDB3.0 database, a total number of 37, 18, 25, 55, 26, and 39 TFs were identified in blue, greenyellow, purple, red, salmon, and yellow modules, respectively (Supplementary Table S12).
Six nonpreserved modules were identified as potential candidate modules and biologically related to BRD based on the module preservation and functional enrichment analysis, respectively. Then, MM measures were used to identify intramodular hub genes as important and central genes in these modules. A total number of 326, 251, 216, 145, 131, and 99 hub genes were detected in blue, yellow, red, purple, greenyellow, and salmon modules, respectively. The complete list of the hub genes in the nonpreserved modules can be found in Supplementary Table S13. Hub genes-based PPI networks extracted from the STRING database identified 303 nodes (proteins) and 2,942 edges (interactions) for the blue module, 204 nodes and 1,085 edges for the yellow module, 183 nodes and 982 edges for the red module, 131 nodes, and 1,905 edges for the purple module, 121 nodes and 716 edges for the greenyellow module, and 91 nodes and 345 edges for the salmon module indicating high connection density of proteins encoded by the genes of these modules. Figure 8 represents the PPI network of the purple module as the nonpreserved and potential biologically BRD-related module. Additionally, based on the PPI networks obtained by hub genes, a total number of 48, 51, 48, 49, 27, and 19 hub–hub genes were found in the blue, yellow, red, purple, greenyellow, and salmon modules, which can be potential biomarkers and candidate disease genes in the etiology and the diagnostics of BRD (Table 2). PPI networks of significant highly correlated modules (identified by MTRs) and nonpreserved modules (identified by MP) that were biologically associated with BRD are available in Supplementary Figure S1.
Figure 8. PPI network based on the hub genes of the purple module (identified by module preservation method). Larger nodes and orange octagons represent hub–hub genes and transcription factors, respectively.
Table 2. List of the hub–hub genes of the nonpreserved and biologically BRD-related modules that were identified by module preservation analysis.
BRD is a multifactorial disease that results from the interaction of environmental stressors and infectious agents of BRDC (Gagea et al., 2006). BRDC includes the viral pathogens such as bovine respiratory syncytial virus (BRSV), bovine parainfluenza type 3 virus (BPIV-3), bovine viral diarrhea virus (BVDV), bovine coronavirus (BCV), and bovine herpes virus type 1 (BHV-1) that affect upper respiratory system and also contain the bacterial pathogens Trueperella pyogenes, Mycoplasma bovis, Pasteurella multocida, Histophilus somni, Bibersteinia trehalosi, and Mannheimia haemolytica, which can affect the lower respiratory system (Caswell, 2014; Kirchhoff et al., 2014). Despite numerous studies, BRD is still the most common disease and the leading cause of morbidity and mortality in the cattle industry (Taylor et al., 2010). Understanding the molecular mechanisms involved in the bovine immune response to BRD is necessary given the persistence of the disease in recent years. Combining high-throughput technologies with various computational methods based on the network approach, can provide an exceptional opportunity to better understand the pathological processes of diseases and the molecular mechanisms of the host immune responses (Kadarmideen and Watson-Haigh, 2012). In this study, we combined gene expression matrix obtained by RNA-seq data analysis with two co-expression network-based methods of WGCNA, module–trait relationships, and module preservation analysis, to identify potential gene modules and candidate genes involved in molecular processes induced by BRD.
Module–trait relationship analysis identified four significant highly correlated modules including turquoise, purple, blue, and brown with clinical traits of BRD. Functional enrichment analysis indicated that three of the four (75%) identified modules including blue, purple, and turquoise modules had significant enriched terms and pathways. This shows the power and high accuracy of signed networks in separating the modules from each other and identifying more significantly enriched terms or pathways in the modules. The significant enriched terms in the blue module were mostly related to basic cellular activities including “tRNA transport,” “transcription DNA-templated,” “DNA metabolism,” “macromolecule biosynthetic,” and “cell cycle.” On the other hand, the turquoise and purple modules had many biological processes and KEGG pathways closely related to the BRD mechanisms. So, our focus on the turquoise and purple modules (identified by MTRs method) as significant highly correlated and key biologically related modules to BRD.
Coregulated genes in the turquoise module were closely related to the mechanisms of the innate immune system as the first defense line against BRD and this shows the great importance of this module during BRD development. We also found a number of enriched terms related to the adaptive immune system in this module. After infection with various viral or bacterial pathogens, extensive complex interactions begin between the host and the pathogen (Kumar et al., 2011). Pathogens produce various molecules known as pathogen-associated molecular pattern (PAMPs) to continue their life cycle and pathogenic activity (Janeway and Medzhitov, 2002; Tang et al., 2012). These PAMPs are recognized by pattern recognition receptors (PRRs) in the host, which are proteins expressed by key innate immune system cells such as neutrophils, macrophages, monocytes, dendritic cells, and epithelial cells (Medzhitov, 2007; Yuki and Koutsogiannaki, 2021). Upon recognition of PAMPs by PRRs, a cascade of signaling pathways is induced, leading to an inflammatory response and subsequent rapid response of the innate immune system to eliminate the pathogens (Lee and Kim, 2007; Kawai and Akira, 2010). In this regard, the turquoise module had important KEGG signaling pathways as well as their downstream biological processes associated with PRRs and inflammatory response including “Toll-like receptor signaling pathway,” “MyD88-dependent Toll-like receptor signaling pathway,” “TRIF-dependent Toll-like receptor signaling pathway,” “C-type lectin receptor signaling pathway,” “NOD-like receptor signaling pathway,” “NF-kappa B signaling pathway,” and “MAPK signaling pathway.”
Toll-like receptors (TLRs) are the most important signaling maker PRRs and act as the primary sensors of pathogens (Iwasaki and Medzhitov, 2004; Akira et al., 2006). The Toll-like receptor signaling pathway is activated through the recognition of PAMPs by membrane and cytoplasmic TLRs (Kumar et al., 2009; Blasius and Beutler, 2010; Heidarzadeh et al., 2020). The TLR signaling pathway is divided into two distinct pathways, including the MyD88-dependent and the TRIF-dependent signaling pathway, depending on the type of TLR sensitized and subsequently equipped adapters (Takeuchi and Akira, 2010). All TLRs family (TLR1 to 10), except TLR3, activate the MyD88-dependent Toll-like receptor signaling pathway, which activates the NF-kappa B signaling pathway and MAPK signaling pathway to produce proinflammatory cytokines and chemokines (Takeda and Akira, 2005; Lin et al., 2010; Kawasaki and Kawai, 2014). On the other hand, TLR3 as well as TLR4 activate the TRIF-dependent Toll-like receptor signaling pathway, which leads to induce production of proinflammatory cytokines and type I interferons by activating NF-κB and IRF3/IRF7 transcription factors (Takeda and Akira, 2005; Lin et al., 2010; Kawasaki and Kawai, 2014). Various studies in cattle diseases such as bovine tuberculosis (Nalpas et al., 2015), Johne’s disease (Ferwerda et al., 2007; Wang et al., 2019), endometritis (Turner et al., 2014), and mastitis (Luoreng et al., 2018; Islam et al., 2020) have reported the TLR-signaling pathway being induced during these diseases. A previous study also reported that the Toll-like receptor signaling pathway was activated during different challenges with a group of BRDC, including BoHV-1, BRSV, BVDV, Mannheimia haemolytica, and Pasteurella multocida (Tizioto et al., 2015).
C-type lectin receptor signaling pathway is another pattern recognition receptor related pathway that is activated by CLRs membrane receptors by identifying different carbohydrates such as mannose, glucan, and fucose in viruses, bacteria, and fungi and activates MAP kinases, the transcription factor NF-AT, and NF-κB that eventually induces the production of proinflammatory cytokines (Geijtenbeek and Gringhuis, 2009; Takeuchi and Akira, 2010). Recent studies have examined the importance of C-type lectin receptors in human infectious diseases (Lugo-Villarino et al., 2018; Zhao et al., 2019). NOD-like receptors are cytosolic receptors that can detect a wide range of bacteria, viruses, and other pathogens that enter the cytoplasm (Franchi et al., 2009). These receptors, like Toll-like receptors activate the NF-kappa B and MAPK signaling pathways, regulate the production of inflammatory cytokines such as IL-1β, and can also induce apoptosis (Chen et al., 2009; Banse et al., 2013).
The NF-kappa B signaling pathway is a key pathway that acts as a major mediator in inflammatory responses. Activation of the NF-κB transcription factor induces the transcription of many genes that encode proinflammatory cytokines and chemokines such as IL-1β, IL-6, TNF-α, IL-12p40, and cyclooxygenase-2 (Oeckinghaus and Ghosh, 2009; Liu et al., 2017). In addition, a study investigated the effect of SH protein expressed by the BRSV genome on the lack of NF-κB phosphorylation in the host, which reduces the production of proinflammatory cytokines and thus modulates the immune system (Pollock et al., 2017). The MAPK signaling pathway is another key mediator pathway during inflammation that regulates cytokine production by phosphorylating and activating certain kinases (Salojin and Oravecz, 2007). Several transcriptomics and proteomics studies have reported MAPK signaling pathway activity during BRD (Tizioto et al., 2015; Behura et al., 2017; Liyang et al., 2021).
Furthermore, other important immune-related terms identified in the turquoise module include “JAK-STAT signaling pathway,” “TNF signaling pathway,” “PI3K-Akt signaling pathway,” “regulation of interferon-gamma production,” and “positive regulation of interleukin-8 secretion.” The JAK-STAT signaling pathway is one of the most important intracellular signaling pathways that regulates communication between cytokine transmembrane receptors and the nucleus and is involved in many biological processes in the body, such as immune regulation, cell differentiation/proliferation, apoptosis, and keep homeostasis in inflammatory conditions (O'Shea et al., 2012; O'Shea et al., 2015; Xin et al., 2020). This pathway, mediates cytokine responses through binding of cytokines such as IL-6/12/17/23, as well as type I (alpha and beta), and II (gamma) interferons to their respective receptors at the cell surface and activation of STATs transcription factors to regulate their target genes in the nucleus (Charles Jay and Eric, 2009). Interferons by activating this pathway, can cause antiviral conditions (Horvath, 2004b; a). BoHV-1, as an infectious agent of BRDC, has the ability to suppress the host immune system by expressing the UL41 gene and subsequently increase its viral replication. The UL41 blocks the JAK-STAT signaling pathway by suppressing STAT1 expression. Thus, this virus has the ability to deal with the antiviral condition resulting from the JAK-STAT signaling pathway (Ma et al., 2019). On the other hand, Ma et al. (2020) reported that Bta-miR-2890, by directly targeting BoHV-1 UL41, increases STAT1 and JAK1 expression and, thus, opens the JAK-STAT signaling pathway as well as prevent viral replication. This suggests the importance of JAK-STAT signaling pathway during viral infections.
The PI3K–Akt signaling pathway is a key pathway in all mammalian cells that is involved in several processes, such as cell growth, migration, proliferation, and metabolism as well as the role of this pathway in regulatory T-cell development and memory CD8 T-cell differentiation, has been reported (Kim and Suresh, 2013; Pompura and Dominguez-Villar, 2018). It has also been suggested that some genes in the PI3K–Akt signaling pathway may play an important role in eliciting downstream cascades in lung lesions during BRD (Behura et al., 2017). Tumor necrosis factor (TNF) is a proinflammatory cytokine that is mainly secreted by macrophages and alerts other cells during the inflammatory response. TNF is also known to be a major regulator of the production of proinflammatory cytokines and has been considered as a therapeutic target for the treatment of some inflammatory diseases such as rheumatoid arthritis and inflammatory disease (Liu, 2005; Parameswaran and Patial, 2010). The TNF signaling pathway plays an important role in the control of inflammation, immunity, and cell survival (Rana et al., 2019). It has been shown that one of the strategies for bacterial survival and immunosuppression by Mycoplasma bovis is to inhibit TNF-α and interferon-gamma production (Mulongo et al., 2014).
Interleukin-8 is a chemokine, that is expressed in various cells especially in macrophages. IL8 is responsible for the induction of chemotaxis and causes guided migration of neutrophils to the site of infection (Remick, 2005). An increase in IL8 expression has been observed in challenges with BRSV and Mannheimia haemolytica as well as a significant association between increased expressions of IL8 with lung lesions. Therefore, it has been suggested that IL8 antagonist drugs be used to prevent inflammatory lung lesions (Caswell et al., 1998; Malazdrewich et al., 2001; Singh et al., 2011; Redondo et al., 2014).
In response to signaling proinflammatory cytokines and chemokine such as IL8, neutrophils are the first cells to migrate from the blood to the infection site (Kolaczkowska and Kubes, 2013). These cells play an important role in killing extracellular pathogens through phagocytosis (Nordenfelt and Tapper, 2011; DeLeo and Allen, 2020). Neutrophil-related biological processes and KEGG pathways in the turquoise module included “neutrophil mediated immunity,” “neutrophil degranulation,” “neutrophil activation involved in immune response,” and “neutrophil extracellular trap formation.” However, neutrophils play an important role in the pathogenesis of BRD by destruction and damaging lung tissue during infection (McGill and Sacco, 2020). Moreover, neutrophils release their nuclear DNA and related proteins in the extracellular environment through NETosis, a unique form of cell death that leads to the formation of neutrophil extracellular traps (McGill and Sacco, 2020). NETs trap and kill bacteria, fungi, viruses, and parasites. In addition to their antimicrobial role, NETs can also play a role in the pathogenesis of inflammatory diseases (Papayannopoulos, 2018). Furthermore, evidence suggests that NETs play a role in the host defense in response to Mannheimia haemolytica and Histophilus somni infection (Aulik et al., 2010; Hellenbrand et al., 2013). The findings also indicate that Mycoplasma bovis, through its endonucleases, is able to digest NETs and escape the immune system (Gondaira et al., 2017). However, Cortjens et al. (2016) showed that NETs has the ability to trap respiratory syncytial virus (RSV), but their excessive accumulation leads to airway obstruction, which can contribute to RSV pathogenesis (Cortjens et al., 2016).
We also identified several microbicidal mechanisms, including “Phagocytosis,” “Fc gamma R-mediated phagocytosis,” “Phagosome,” “Lysosome,” and “phagosome maturation” in the turquoise module. In the immune system of multicellular organisms, phagocytosis is a cellular process for the elimination of pathogens and dead cell debris, and is an essential for maintaining tissue homeostasis (Flannagan et al., 2012). Fc gamma R (FcγR) receptors are glycoproteins found on the surface of immune cells such as neutrophils, macrophages, and natural killer cells that stimulate phagocytosis through antigen-binding IgG antibodies (Rosales, 2017). Phagocytosis involves several stages. After ingestion of pathogens or foreign particles by immune cells, the ingested particles become specialized vacuoles and distinct organelles called phagosomes. During the stage called phagosome maturation, the lysosome fuses with the phagosome membrane, and its contents are poured into the phagosome, and an organelle called phagolysosome is formed. This newly formed organelle contains enzymes that can break down the digested particles (Canton, 2014; Levin et al., 2016; Uribe-Querol and Rosales, 2020). Because of the importance of phagocytosis pathway in inducing an innate and adaptive immune response, pathogens use specific strategies to control and suppress phagocytes (Srikumaran et al., 2007). Mannheimia haemolytica and Pasteurella multocida use their toxins and extracellular components to kill phagocytes, thus preventing phagocytosis and subsequently releasing the reactive oxygen metabolite contents of the phagocytes, which exacerbates pulmonary inflammation (CUSACK et al., 2003). Research also shows that Mycoplasma bovis has the ability to survive long-term in necrotic lung lesions and phagocytic cells by overcoming phagocytosis (Kleinschmidt et al., 2013). Moreover, virulent isolates of Histophilus somni have the ability to survive in phagocytic cells by interfering with phagosome–lysosome maturation (Pan et al., 2018). In addition to the bacterial agents of the BRDC, viruses such as BVDV, PIV3, and BRSV have the ability to inhibit phagocytosis by macrophages (Bell et al., 2021).
The turquoise module also identified some of the major pathways associated with programmed cell death, including “Apoptosis” and “Necroptosis.” Apoptosis is the first type of programmed cell death that clears cells infected with pathogens (especially viruses) to prevent them from replication and spread (Amarante-Mendes et al., 2018). For example, a previous study showed that apoptosis of BRSV-infected epithelial bronchial cells is an effective way to clear the virus (Viuff et al., 2002) as well as it has been suggested that apoptosis may play a role in modulating airway inflammation during BRSV infection (Cristina et al., 2001). On the other hand, the BHV-1 uses the products of the LR gene to prevent apoptosis of infected cells and can therefore proliferate sufficiently in infected cells (Geiser and Jones, 2005; Srikumaran et al., 2007). Moreover, BHV-1 inhibits the efficient immune response by infecting CD41+ T-cells and inducing apoptosis in them (Jones and Chowdhury, 2010). Bacterial pathogens also have the ability to survive and manipulate intracellular mechanisms of host cells to escape the immune system. A recent study showed that Mycoplasma bovis has the ability to inhibit the apoptosis of infected bovine alveolar macrophages by increasing the expression of several anti-apoptotic genes (Maina et al., 2019). Necroptosis is a programmed form of inflammatory cell death (necrosis) that actively causes cell death of infected cells or the propagation of danger signals to stimulate the immune system. However, uncontrolled necroptosis may lead to the pathogenesis of inflammatory diseases. For instance, the findings show that RSV, through the activation of necroptosis, lead to neutrophilic cell death (Muraro et al., 2018). Bedient et al. (2020) showed that the RSV causes cell death of alveolar macrophages through various cell death mechanisms such as necroptosis, pyroptosis, and apoptosis, which can contribute to the pathogenesis of the RSV and exacerbate inflammation in the lungs (Bedient et al., 2020).
Interestingly, we identified the terms “regulation of nitric oxide biosynthetic process,” “positive regulation of nitric oxide metabolic process,” and “positive regulation of nitric oxide biosynthetic process” in the turquoise module. Nitric oxide (NO) is a natural molecule with antimicrobial properties that is produced by most mammalian cells (Crepieux et al., 2016). Several studies have shown the antibacterial and antiviral properties of NO during BRD, and it has also been demonstrated that NO therapy, as a non-antibiotic-based treatment can be a safe and effective method to control BRD (Regev-Shoshani et al., 2013; Regev-Shoshani et al., 2014). Another study demonstrated that NO reduced levels of proinflammatory cytokines, such as IL-1β and TNF, thereby limiting inflammation during BRD. On the other hand, NO increases the ability of the host to detect pathogens by increasing the expression of TLR4 gene (Sheridan et al., 2016).
We also identified some pathways in the turquoise module associated with the adaptive immune system such as “B-cell receptor signaling pathway”, “positive regulation of activated T-cell proliferation,” “Th1/Th2 cell differentiation,” and “Th17 cell differentiation.” B cells are vital cells for the humoral response, and research shows that the B-cell receptor signaling pathway is one of the most important pathways in the immune system of animals to respond to infection with viral agents of BRDC (Tizioto et al., 2015). T cells are essential cells for cell-mediated immunity and include several subtypes that play a variety of roles, including killing virus-infected cells, secreting interferon-gamma, and other cytokines (Platt et al., 2006). The importance and application of helper and cytotoxic T cells in viral clearance in the first and second challenges with BVDV have been investigated. The results show that these T cells in the second challenge with BVDV have the ability to quickly clear the virus (Silflow et al., 2005). Furthermore, T cells have been reported to be critical for the response to BRSV infection (Gaddum et al., 2003).
Intramodular hub genes (especially hub–hub genes) are highly correlated with the biological function of the module. In this regard, hub–hub genes identified in the turquoise module, such as FN1, MAPK14, PSEN1 (Neupane et al., 2018), IL-1β, IL-18 (Taylor et al., 2014), MYD88 (Dubbert et al., 2013), SOD2 (Hofstetter and Sacco, 2020), CTSD (Gray et al., 2019), JAK2 (Amat et al., 2019; Chao et al., 2019), CD68 (Lee et al., 2009), and TLR2 (Mariotti et al., 2009; Tizioto et al., 2015) have been reported as important genes in previous BRD studies. SOCS3 hub–hub gene was another key gene identified in the turquoise module. This gene blocks both the production and signal transduction of type I and II interferons by disrupting the JAK/STAT signaling pathway (Akhtar and Benveniste, 2011). Several studies have shown that viral agents of BRDC disrupt interferon-dependent antiviral responses in the host by inducing the expression of SOCS1 and SOCS3 and subsequently provide a suitable condition for their survival and proliferation (Akhtar and Benveniste, 2011; Ye et al., 2015; Zheng et al., 2015; Alkheraif et al., 2017; Salem et al., 2019). These findings indicate the importance of the SOCS3 gene as a therapeutic target for infections caused by BRDC viral agents.
Moreover, other important hub–hub genes in the turquoise module included IL10 and TLR4. Interleukin-10 (IL10) is an anti-inflammatory cytokine that inhibits inflammatory responses initiated by proinflammatory cytokines and subsequently regulates inflammation (Pestka et al., 2004). Therefore, some studies show that increasing the expression of IL10 regulates the inflammatory response during BRD (Molina et al., 2014; Gondaira et al., 2015; Rodríguez et al., 2015). On the other hand, Risalde et al. (2011) showed that BHV-1 secondary infection in BVDV-infected cows suppressed IL10 expression, which leads to exacerbate the inflammatory response and more severe clinical lesions (Risalde et al., 2011). Furthermore, failure in upregulation of IL10 expression level due to weaning and transport is associated with a doubling of BRD mortality (Hodgson et al., 2005). TLR4 is one of the most important cell surface PRRs that induces an inflammatory response in response to lipopolysaccharide (LPS) derived from Gram-negative bacteria by activation of different signaling pathways (Ciesielska et al., 2021). However, overexpression and abnormal activation of TLR4 leads to chronic and acute inflammatory disorders such as endotoxemia and sepsis in human and equine (Werners and Bryant, 2012; Kuzmich et al., 2017). Also, due to the key role that TLR4 plays in activating the signaling pathways that lead to the secretion of proinflammatory cytokine, this gene has been suggested as a very attractive therapeutic target for inflammatory diseases, such as sepsis in human and equine (Werners and Bryant, 2012; Kuzmich et al., 2017). Moreover, a significant correlation has been reported between increased TLR4 expression level and increased mortality during BRD (Hodgson et al., 2012). Therefore, these results indicate a key role for IL10 and TLR4 during BRD that can be further explored as important targets.
Among the detected TFs, STAT3 is one of the most important hub–hub TFs identified in the turquoise module. Signal transducer and activator of transcription 3 (STAT3) is a transcription factor belonging to the STAT protein family that regulates various biological activities such as apoptosis, angiogenesis, differentiation, cell proliferation, inflammation, and the immune response (Furtek et al., 2016). In different studies, STAT3 has been suggested as a key gene involved in various bovine diseases (Jaslow et al., 2018; Villaseñor et al., 2019; Bakhtiarizadeh et al., 2020). Moreover, several transcriptomic studies have identified STAT3 among the top DEGs in response to infection with agents of BRDC, indicating its important role during BRD (Wu et al., 2017; Chao et al., 2019).
Several functional terms identified in the purple module included “Natural killer cell-mediated cytotoxicity,” “T-cell receptor signaling pathway,” “cellular defense response,” “Chemokine signaling pathway,” and “T-cell activation.” Interestingly, in addition to the antiviral activity of T cells that have been discussed above, the T-cell receptor signaling pathway has also been observed in challenges with Mannheimia haemolytica, which indicates the importance and participation of T cells in responding to different types of pathogens (Tizioto et al., 2015). In agreement with the previous studies, PRF1 (Johnston et al., 2021a), LCK (Smirnova et al., 2009), NCR1 (Osman and Griebel, 2017), CCL5 (N’jai et al., 2013), CD8A (Knapek et al., 2020; Lebedev et al., 2021), CCR8 (Lopez et al., 2020), CX3CR1 (Salem et al., 2019), and TBX21 hub–hub TF (Johnston et al., 2019) were identified as highly connected genes in the purple module and have been reported as immune-related genes during BRD. For instance, the PRF1 hub–hub gene is an important gene that encodes the perforin-1, which is present in cytotoxic T-lymphocytes (CTLs) and natural killer cells (NK cells) and is involved in cytolysis and the regulation of the immune system. This gene has been suggested by Johnston et al. (2021a) as one of the biomarkers for diagnosis of subclinical BRD from blood samples.
Module preservation analysis and functional enrichment showed that six modules (blue, greenyellow, purple, red, salmon, and yellow) first changed their connectivity pattern and network density due to BRD and second, were biologically related to the BRD development. As expected, highly preserved and semipreserved modules were more active in basic and general cellular activities such as “Ribosome,” “rRNA processing,” “DNA packaging,” “peptide biosynthetic process,” and “translation.” Therefore, based on highly preserved and semipreserved modules, it is not possible to explain the molecular mechanisms involved in BRD. On the other hand, the six mentioned nonpreserved modules were closely related to the immune system and the pathogenesis of BRD.
Functional terms in the blue module were mostly related to the innate immune system, including “lysosome,” “phagosome,” “Fc gamma R-mediated phagocytosis,” “leukocyte transendothelial migration,” “Toll-like receptor signaling pathway,” “Chemokine signaling pathway,” “neutrophil activation involved in immune response,” “activation of MAPK activity,” and “positive regulation of NF-kappaB import into nucleus.” The leukocyte transendothelial migration is one of the important steps of the innate immune system in triggering the inflammatory immune response and the migration of the first immune response cells such as neutrophils to the sites of infection (Getter et al., 2019; Bakhtiarizadeh et al., 2020) which that chemokines control cellular responses at inflammatory sites through this pathway (Krishnan et al., 2004). In agreement with similar BRD studies (Tizioto et al., 2015; Behura et al., 2017; Johnston et al., 2021c; Lebedev et al., 2021), the leukocyte transendothelial migration was identified as one of the important pathways of the immune system in the blue module. Additionally, some of the hub–hub genes identified in the blue module have also been reported in the previous BRD studies, including TLR4 (Scott et al., 2021), ANXA5 (Mitchell et al., 2008), C3, PSEN1 (Neupane et al., 2018), CTSB, CD59, FTL (Nilson et al., 2020), CAT (Joshi et al., 2018), TLR7, CD86 (Palomares et al., 2014), ATG7 (Lipkin et al., 2016), and IFNAR1 (Amat et al., 2019). For example, stresses from weaning, transport, and commingling reduced the expression level of the ANXA5 (hub–hub gene) and, thus, lead to an increase in apoptotic cells in the lungs or epithelial lining fluid, which can increase the susceptibility of cattle to a primary infection (Mitchell et al., 2008).
Coexpressed genes in the greenyellow module were enriched in KEGG pathways and biological process such as “T-cell receptor signaling pathway,” “focal adhesion,” “leukocyte transendothelial migration,” and “regulation of cell motility.” These pathways have also been observed in previous transcriptomic studies in response to infection with the agents of BRDC (Tizioto et al., 2015; Behura et al., 2017). Among the hub–hub genes identified in the greenyellow module, the PTEN gene plays an important role in the pathogenesis of BRD. Research has shown that MicroRNA-26b induces the NF-kB signaling pathway by directly targeting PTEN, which exacerbates inflammation in the lungs during infection with Gram-negative bacteria (Zhang et al., 2015). Moreover, other hub–hub genes in the greenyellow module including ADAM10 (Neupane et al., 2018), MGAT4A (Johnston et al., 2021b), and GSK3B (Chao et al., 2019) were identified as important genes involved in BRD.
Functional enrichment analysis revealed that the purple module was enriched in the “NOD-like receptor signaling pathway,” “C-type lectin receptor signaling pathway,” “RIG-I-like receptor signaling pathway,” and “necroptosis” KEGG pathways as well as “inflammatory response,” “type I interferon signaling pathway,” “positive regulation of type I interferon production,” “interferon-gamma-mediated signaling pathway,” and “cellular response to interferon-gamma” biological processes. RIG-I-like receptors are important cytoplasmic PRRs that detect intracellular viruses through their genomic RNA (Takeuchi and Akira, 2010). Recognition of PAMPs by RIG-I-like receptors initiates the RIG-I-like receptor signaling pathway, which activates NF-κB and IRF3/IRF7 transcription factors which subsequently lead to the production of proinflammatory cytokines and type I interferons (Kumar et al., 2011). Type I (IFN-α and IFN-β) and II (IFN-γ) interferons are cytokines that are the first line of defense against viral infections that play a key role in the development of antiviral states during the immune response (Platanias, 2005). Type I interferons are polypeptides that are secreted from virus-infected cells and activate antimicrobial states in infected cells and healthy neighboring cells to prevent the proliferation and growth of infectious agents, especially viruses. They also promote antigen presentation, increase the activity of natural killer cells, and activate the adaptive immune system (Ivashkiv and Donlin, 2014). Furthermore, type II interferon, whose major producers include natural killer cells, T cells, macrophages, dendritic cells, and B cells play a key role in regulating many protective functions such as inducing antiviral states, enhancing antimicrobial functions, increasing leukocyte trafficking, effect on cell proliferation, and apoptosis (Kak et al., 2018).
Several recent studies have shown that the type I interferon signaling pathway has been identified as a key pathway in cows with BRD atfeedlot entry, which indicates that animals show antiviral responses at the entry stage (Sun et al., 2020; Johnston et al., 2021a; Scott et al., 2021). Interestingly, some of the important hub–hub genes, which are involved in anti-viral interferon response were identified in the purple module, such as IFI6, ISG15, MX1, OAS2, IFIH1, DDX58, DHX58, RSAD2, IFI44, IFI44L, EIF2AK2, ISG20, MX2, IFIT5, IFITM3, OAS1Y, and HERC5, have been suggested by these studies as potential biomarkers for diagnostic and prediction of subclinical BRD at early stage of infection (Sun et al., 2020; Johnston et al., 2021a; Scott et al., 2021). Additionally, some of the key hub–hub TFs that regulate the expression of coexpressed genes in this module, and play critical roles in interferon antiviral responses during BRD, were included IRF9, STAT1, STAT2 (Sun et al., 2020), and IRF7 (Johnston et al., 2019). Type I interferons activate the IFN-stimulated gene factor 3 (ISGF3) complex during JAK/STAT signaling pathway. This complex consists of three transcription factors STAT1, STAT2, and IFN-regulatory factor 9 (IRF9), which induce the expression of antiviral genes (Ivashkiv and Donlin, 2014). In addition to type I interferons (IFN-α and IFN-β), type II interferons (IFN-γ) also cause the formation of STAT1-STAT1 homodimers, which, following phosphorylation and after being transferred to the nucleus, induce the expression of antiviral genes (Platanias, 2005; Kak et al., 2018). Besides, several studies have demonstrated that cytoplasmic localized infected cell protein 0 (bICP0) encoded by the BHV-1 (a viral agent of BRDC), through interaction with IRF7, disrupts the activity of the IFN-β promoter (Saira et al., 2007; da Silva et al., 2011; Jones, 2019). Moreover, one study demonstrated that BPIV-3 had a negative effect on the JAK/STAT signaling pathway by reducing phosphorylation of STAT1, thereby inhibiting the production of antiviral molecules (Eberle et al., 2016). These explain why the STAT1, STAT2, IRF9, and IRF7 transcription factors are highlighted in this module as very important regulators. Other members of the purple module, including USP18, OAS1X, CMPK2, GBP4 (Nilson et al., 2020), IFI35 (Sun et al., 2020), PARP14 (Oguejiofor et al., 2015), RTP4 (Johnston et al., 2019), and TRIM21 (Quick et al., 2020), have also been observed in different BRD studies that may play an important role in the immune system in response to BRDC agents.
In agreement with the previous studies, functional terms including “VEGF signaling pathway,” “T-cell receptor signaling pathway” (Tizioto et al., 2015), and “C-type lectin receptor signaling pathway” as well as hub–hub genes including LCK (Smirnova et al., 2009), TLR3 (Marin et al., 2016), TIA1 (Johnston et al., 2021b), TNF (El-Deeb et al., 2020), and STAT5A hub–hub TF (Lin et al., 2015) demonstrate the importance of the red module during BRD. Furthermore, the salmon module was mainly enriched in “cellular defense response,” “regulation of immune response,” “leukocyte cell–cell adhesion,” and “natural killer cell-mediated cytotoxicity.” Recent studies have demonstrated that some of the hub–hub genes in the salmon module, such as CCR5 (Salem et al., 2019), CCR8 (Amat et al., 2019; Lopez et al., 2020), CX3CR1 (Scott et al., 2021), ITGAL, IL12RB2 (Neupane et al., 2018), NCR1 (Osman and Griebel, 2017), CCL5 (N’jai et al., 2013), and PRF1 (Johnston et al., 2021a) tend to participate in BRD. Additionally, GZMB gene, which plays a major role in stimulating cytotoxic T-cell responses and limiting virus replication in the host (Jiminez et al., 2021), was found among hub–hub genes in the salmon module. Granzyme B-protein, which is encoded by this gene, is an important serine protease that is expressed in cytotoxic T-lymphocytes (CTL) and natural killer (NK) cells and kills viral infected cells through apoptosis (Xu et al., 2018). GZMB was the most highly upregulated gene in the bronchial lymph node in response to BRSV infection, indicating a close relationship between this gene and the response to viral infection (Johnston et al., 2019). This gene has also been found to be among the DEGs with the highest expression in animals that are resistant to BRD (Scott et al., 2020). The GZMB gene is likely to play an important role in the host defense against BRSV infection and maybe other BRDC viral agents, and changes in this gene are critical for BRD resistance and susceptibility (Johnston et al., 2019), as shown, the presence of polymorphisms in the GZMB gene in mice caused the cytotoxic T cells to lose their ability to kill viral infected cells (Andoniou et al., 2014).
The yellow module showed that its genes were enriched in some important biological processes and KEGG pathways associated with BRD such as “neutrophil activation involved in immune response,” “regulation of inflammatory response,” “positive regulation of T-cell proliferation,” “MAPK signaling pathway,” “NF-kappa B signaling pathway,” “apoptosis,” “TNF signaling pathway,” “JAK-STAT signaling pathway,” and “Cytokine-cytokine receptor interaction.” Furthermore, examination of the relationship between the hub–hub genes of this module and BRD showed that many of these genes, such as IL1B (Behura et al., 2017), IL15 (Leach et al., 2012; Amat et al., 2019), CD68 (Buchenau et al., 2010; Hermeyer et al., 2011), SOCS3 (Ye et al., 2015; Zheng et al., 2015), NFKBIA, RAF1, SGK1 (Chao et al., 2019), ANXA1 (Mitchell et al., 2008), MYD88 (Dubbert et al., 2013), FAS (Xu et al., 2012), CCR1 (Lindholm-Perry et al., 2018), IFNAR2 (Schlender et al., 2000), NFAM1, NUDT3 (Johnston et al., 2021b), and SLC2A3 (N’jai et al., 2013) as well as DDIT3 hub–hub TF (Wang S. et al., 2020) have important effects on the interaction between the host and the pathogen. For example, stressful stimuli directly increase the expression level of the SGK1 (hub–hub gene), and consequently an upregulation in the expression of this gene leads to stimulate BoHV-1 and HSV-1 replication (Kook and Jones, 2016; Zhu et al., 2017). Therefore, the use of SGK inhibitors may be a suitable strategy to reduce BoHV-1 and HSV-1 replication (Kook and Jones, 2016).
These findings demonstrate the relevance of the mentioned modules as well as their genes, especially hub–hub genes and TFs as important candidates in the development of BRD, helping us to better understand the molecular mechanisms responsible for the immune response to BRD. Further researches are needed to more closely examine the biological behavior and functions of these modules and their genes during BRD.
Given that BRD is the main cause of morbidity and mortality in beef and dairy cattle and has a potential impact on economic losses in the livestock industry, a systems biology approach was used to further investigate the molecular mechanisms of BRD as well as to identify diagnosis biomarkers and therapeutic targets for BRD. In this study by using WGCNA distinct methods (MTRs and MP) and functional enrichment analysis, we identified eight candidate modules that are involved in the immune response and BRD pathogenesis. It is noteworthy that both WGCNA methods showed a similar ability to identify candidate modules during BRD, confirming each other results. Integrated coexpressed hub genes of eight candidate modules with PPI networks, allowed us to identify hub–hub genes that act as central genes in both coexpression and PPI networks and may be important candidates during BRD development. In total, we identified 307 hub–hub genes in eight candidate modules, most of which were potentially involved in BRD. These genes along with other members of the eight candidate modules could be important targets in the pathogenesis of BRD for future researches. Therefore, more research is needed to validate the hub–hub genes reported in this study, especially those whose role in the immune system in response to BRD is still unclear.
The original contributions presented in the study are included in the article/Supplementary Material, further inquiries can be directed to the corresponding authors.
AH conceived the ideas. AH designed the study. AH and NS analyzed the data. AH, AB, and FF interpreted the data. AH wrote the main manuscript. GJ, MB, HK, and SI helped in writing the manuscript. AB and RA reviewed and edited the manuscript. HM, AB, and HB reviewed the manuscript. All authors read and approved the final version of manuscript.
The authors declare that the research was conducted in the absence of any commercial or financial relationships that could be construed as a potential conflict of interest.
All claims expressed in this article are solely those of the authors and do not necessarily represent those of their affiliated organizations, or those of the publisher, the editors and the reviewers. Any product that may be evaluated in this article, or claim that may be made by its manufacturer, is not guaranteed or endorsed by the publisher.
We would like to thank the University of Tehran for supporting this research.
The Supplementary Material for this article can be found online at: https://www.frontiersin.org/articles/10.3389/fgene.2021.753839/full#supplementary-material
BCV, bovine coronavirus; BHV-1, bovine herpes virus type 1; BPIV-3, bovine parainfluenza type 3 virus; BRD, bovine respiratory disease; BRSV, bovine respiratory syncytial virus; BVDV, bovine viral diarrhea virus; GEO, Gene Expression Omnibus; GO, Gene Ontology; GS, gene significance; KEGG, Kyoto Encyclopedia of Genes and Genomes; ME, module eigengene; MM, module membership; MTRs, module–trait relationships; MP, module preservation; PPI, protein–protein interaction; RSV, respiratory syncytial virus; RNA-seq, RNA-sequencing; TF, transcription factor; TOM, topological overlap matrix; WGCNA, weighted gene coexpression network analysis.
Akhtar, L. N., and Benveniste, E. N. (2011). Viral Exploitation of Host SOCS Protein Functions. J. Virol. 85 (5), 1912–1921. doi:10.1128/JVI.01857-10
Akira, S., Uematsu, S., and Takeuchi, O. (2006). Pathogen Recognition and Innate Immunity. Cell 124 (4), 783–801. doi:10.1016/j.cell.2006.02.015
Alkheraif, A. A., Topliff, C. L., Reddy, J., Massilamany, C., Donis, R. O., Meyers, G., et al. (2017). Type 2 BVDV Npro Suppresses IFN-1 Pathway Signaling in Bovine Cells and Augments BRSV Replication. Virology 507, 123–134. doi:10.1016/j.virol.2017.04.015
Amarante-Mendes, G. P., Adjemian, S., Branco, L. M., Zanetti, L. C., Weinlich, R., and Bortoluci, K. R. (2018). Pattern Recognition Receptors and the Host Cell Death Molecular Machinery. Front. Immunol. 9, 2379. doi:10.3389/fimmu.2018.02379
Amat, S., Timsit, E., Baines, D., Yanke, J., Alexander, T. W., and Liu, S.-J. (2019). Development of Bacterial Therapeutics against the Bovine Respiratory Pathogen Mannheimia Haemolytica. Appl. Environ. Microbiol. 85 (21), e01359–01319. doi:10.1128/AEM.01359-19
Amrine, D. E., White, B. J., Larson, R., Anderson, D. E., Mosier, D. A., and Cernicchiaro, N. (2013). Precision and Accuracy of Clinical Illness Scores, Compared with Pulmonary Consolidation Scores, in Holstein Calves with Experimentally inducedMycoplasma Bovispneumonia. Am. J. Vet. Res. 74 (2), 310–315. doi:10.2460/ajvr.74.2.310
Anders, S., Pyl, P. T., and Huber, W. (2014). HTSeq--a Python Framework to Work with High-Throughput Sequencing Data. Bioinformatics 31 (2), 166–169. doi:10.1093/bioinformatics/btu638
Andoniou, C. E., Sutton, V. R., Wikstrom, M. E., Fleming, P., Thia, K. Y. T., Matthews, A. Y., et al. (2014). A Natural Genetic Variant of Granzyme B Confers Lethality to a Common Viral Infection. Plos Pathog. 10 (12), e1004526. doi:10.1371/journal.ppat.1004526
Aulik, N. A., Hellenbrand, K. M., Klos, H., and Czuprynski, C. J. (2010). Mannheimia Haemolytica and its Leukotoxin Cause Neutrophil Extracellular Trap Formation by Bovine Neutrophils. Infect. Immun. 78 (11), 4454–4466. doi:10.1128/IAI.00840-10
Bakhtiarizadeh, M. R., Hosseinpour, B., Shahhoseini, M., Korte, A., and Gifani, P. (2018). Weighted Gene Co-expression Network Analysis of Endometriosis and Identification of Functional Modules Associated with its Main Hallmarks. Front. Genet. 9, 453. doi:10.3389/fgene.2018.00453
Bakhtiarizadeh, M. R., Mirzaei, S., Norouzi, M., Sheybani, N., and Vafaei Sadi, M. S. (2020). Identification of Gene Modules and Hub Genes Involved in Mastitis Development Using a Systems Biology Approach. Front. Genet. 11, 722. doi:10.3389/fgene.2020.00722
Banse, H., Wollums, A., and Step, D. L. (2013). A Review of Host Pulmonary Defenses with Reference to Cattle. Bovine Pract. 48 (1), 13–24. doi:10.21423/bovine-vol48no1p13-24
Bedient, L., Pokharel, S. M., Chiok, K. R., Mohanty, I., Beach, S. S., Miura, T. A., et al. (2020). Lytic Cell Death Mechanisms in Human Respiratory Syncytial Virus-Infected Macrophages: Roles of Pyroptosis and Necroptosis. Viruses 12 (9), 932. doi:10.3390/v12090932
Behura, S. K., Tizioto, P. C., Kim, J., Grupioni, N. V., Seabury, C. M., Schnabel, R. D., et al. (2017). Tissue Tropism in Host Transcriptional Response to Members of the Bovine Respiratory Disease Complex. Sci. Rep. 7 (1), 17938. doi:10.1038/s41598-017-18205-0
Bell, R. L., Turkington, H. L., and Cosby, S. L. (2021). The Bacterial and Viral Agents of BRDC: Immune Evasion and Vaccine Developments. Vaccines 9 (4), 337. doi:10.3390/vaccines9040337
Blasius, A. L., and Beutler, B. (2010). Intracellular Toll-like Receptors. Immunity 32 (3), 305–315. doi:10.1016/j.immuni.2010.03.012
Bolger, A. M., Lohse, M., and Usadel, B. (2014). Trimmomatic: a Flexible Trimmer for Illumina Sequence Data. Bioinformatics 30 (15), 2114–2120. doi:10.1093/bioinformatics/btu170
Buchenau, I., Poumarat, F., Grand, D. L., Linkner, H., Rosengarten, R., and Hewicker-Trautwein, M. (2010). Expression of Mycoplasma Bovis Variable Surface Membrane Proteins in the Respiratory Tract of Calves after Experimental Infection with a Clonal Variant of Mycoplasma Bovis Type Strain PG45. Res. Vet. Sci. 89 (2), 223–229. doi:10.1016/j.rvsc.2010.03.014
Canton, J. (2014). Phagosome Maturation in Polarized Macrophages. J. Leukoc. Biol. 96 (5), 729–738. doi:10.1189/jlb.1MR0114-021R
Caswell, J. L. (2014). Failure of Respiratory Defenses in the Pathogenesis of Bacterial Pneumonia of Cattle. Vet. Pathol. 51 (2), 393–409. doi:10.1177/0300985813502821
Caswell, J. L., Middleton, D. M., Sorden, S. D., and Gordon, J. R. (1998). Expression of the Neutrophil Chemoattractant Interleukin-8 in the Lesions of Bovine Pneumonic Pasteurellosis. Vet. Pathol. 35 (2), 124–131. doi:10.1177/030098589803500206
Chao, J., Han, X., Liu, K., Li, Q., Peng, Q., Lu, S., et al. (2019). Calves Infected with Virulent and Attenuated Mycoplasma Bovis Strains Have Upregulated Th17 Inflammatory and Th1 Protective Responses, Respectively. Genes 10 (9), 656. doi:10.3390/genes10090656
Chen, E. Y., Tan, C. M., Kou, Y., Duan, Q., Wang, Z., Meirelles, G. V., et al. (2013). Enrichr: Interactive and Collaborative HTML5 Gene List Enrichment Analysis Tool. BMC Bioinformatics 14 (1), 128. doi:10.1186/1471-2105-14-128
Chen, G., Shaw, M. H., Kim, Y.-G., and Nuñez, G. (2009). NOD-like Receptors: Role in Innate Immunity and Inflammatory Disease. Annu. Rev. Pathol. Mech. Dis. 4 (1), 365–398. doi:10.1146/annurev.pathol.4.110807.092239
Chin, C.-H., Chen, S.-H., Wu, H.-H., Ho, C.-W., Ko, M.-T., and Lin, C.-Y. (2014). cytoHubba: Identifying Hub Objects and Sub-networks from Complex Interactome. BMC Syst. Biol. 8 (4), S11. doi:10.1186/1752-0509-8-S4-S11
Ciesielska, A., Matyjek, M., and Kwiatkowska, K. (2021). TLR4 and CD14 Trafficking and its Influence on LPS-Induced Pro-inflammatory Signaling. Cell. Mol. Life Sci. 78 (4), 1233–1261. doi:10.1007/s00018-020-03656-y
Cline, M. S., Smoot, M., Cerami, E., Kuchinsky, A., Landys, N., Workman, C., et al. (2007). Integration of Biological Networks and Gene Expression Data Using Cytoscape. Nat. Protoc. 2 (10), 2366–2382. doi:10.1038/nprot.2007.324
Cortjens, B., de Boer, O. J., de Jong, R., Antonis, A. F., Sabogal Piñeros, Y. S., Lutter, R., et al. (2016). Neutrophil Extracellular Traps Cause Airway Obstruction during Respiratory Syncytial Virus Disease. J. Pathol. 238 (3), 401–411. doi:10.1002/path.4660
Crepieux, T., Miller, C., Regev-Shoshani, G., Schaefer, A., Dorin, C., Alexander, T., et al. (2016). Randomized, Non-inferiority Trial Comparing a Nitric Oxide Releasing Solution with a Macrolide Antibiotic for Control of Bovine Respiratory Disease in Beef Feedlot Calves at High-Risk of Developing Respiratory Tract Disease. Res. Vet. Sci. 105, 216–221. doi:10.1016/j.rvsc.2016.02.020
Cristina, J., Yunus, A. S., Rockemann, D. D., and Samal, S. K. (2001). Bovine Respiratory Syncytial Virus Can Induce Apoptosis in MDBK Cultured Cells. Vet. Microbiol. 83 (4), 317–320. doi:10.1016/S0378-1135(01)00435-7
Cusack, P., McMENIMAN, N., and Lean, I. (2003). The Medicine and Epidemiology of Bovine Respiratory Disease in Feedlots. Aust. Vet J 81 (8), 480–487. doi:10.1111/j.1751-0813.2003.tb13367.x
da Silva, L. F., Gaudreault, N., and Jones, C. (2011). Cytoplasmic Localized Infected Cell Protein 0 (bICP0) Encoded by Bovine Herpesvirus 1 Inhibits β Interferon Promoter Activity and Reduces IRF3 (Interferon Response Factor 3) Protein Levels. Virus. Res. 160 (1), 143–149. doi:10.1016/j.virusres.2011.06.003
Darzi, M., Gorgin, S., Majidzadeh-A, K., and Esmaeili, R. (2021). Gene Co-expression Network Analysis Reveals Immune Cell Infiltration as a Favorable Prognostic Marker in Non-uterine Leiomyosarcoma. Sci. Rep. 11 (1), 2339. doi:10.1038/s41598-021-81952-8
DeLeo, F. R., and Allen, L.-A. H. (2020). Phagocytosis and Neutrophil Extracellular Traps. Fac. Rev. 9, 25. doi:10.12703/r/9-25
Dubbert, J., Bowers, A., Su, Y., and McClenahan, D. (2013). Effect of TRIF on Permeability and Apoptosis in Bovine Microvascular Endothelial Cells Exposed to Lipopolysaccharide. Vet. J. 198 (2), 419–423. doi:10.1016/j.tvjl.2013.08.025
Eberle, K. C., McGill, J. L., Reinhardt, T. A., Sacco, R. E., and Perlman, S. (2016). Parainfluenza Virus 3 Blocks Antiviral Mediators Downstream of the Interferon Lambda Receptor by Modulating Stat1 Phosphorylation. J. Virol. 90 (6), 2948–2958. doi:10.1128/JVI.02502-15
Edwards, T. A. (2010). Control Methods for Bovine Respiratory Disease for Feedlot Cattle. Vet. Clin. North America: Food Anim. Pract. 26 (2), 273–284. doi:10.1016/j.cvfa.2010.03.005
El-Deeb, W., Elsohaby, I., Fayez, M., Mkrtchyan, H. V., El-Etriby, D., and ElGioushy, M. (2020). Use of Procalcitonin, Neopterin, Haptoglobin, Serum Amyloid A and Proinflammatory Cytokines in Diagnosis and Prognosis of Bovine Respiratory Disease in Feedlot Calves under Field Conditions. Acta Tropica 204, 105336. doi:10.1016/j.actatropica.2020.105336
Ellis, J. A. (2001). The Immunology of the Bovine Respiratory Disease Complex. Vet. Clin. North America: Food Anim. Pract. 17 (3), 535–550. doi:10.1016/S0749-0720(15)30005-0
Ferwerda, G., Kullberg, B. J., de Jong, D. J., Girardin, S. E., Langenberg, D. M. L., van Crevel, R., et al. (2007). Mycobacterium Paratuberculosisis Recognized by Toll-like Receptors and NOD2. J. Leukoc. Biol. 82 (4), 1011–1018. doi:10.1189/jlb.0307147
Flannagan, R. S., Jaumouillé, V., and Grinstein, S. (2012). The Cell Biology of Phagocytosis. Annu. Rev. Pathol. Mech. Dis. 7 (1), 61–98. doi:10.1146/annurev-pathol-011811-132445
Franchi, L., Warner, N., Viani, K., and Nuñez, G. (2009). Function of Nod-like Receptors in Microbial Recognition and Host Defense. Immunological Rev. 227 (1), 106–128. doi:10.1111/j.1600-065X.2008.00734.x
Furtek, S. L., Backos, D. S., Matheson, C. J., and Reigan, P. (2016). Strategies and Approaches of Targeting STAT3 for Cancer Treatment. ACS Chem. Biol. 11 (2), 308–318. doi:10.1021/acschembio.5b00945
Gaddum, R. M., Cook, R. S., Furze, J. M., Ellis, S. A., and Taylor, G. (2003). Recognition of Bovine Respiratory Syncytial Virus Proteins by Bovine CD8+ T Lymphocytes. Immunology 108 (2), 220–229. doi:10.1046/j.1365-2567.2003.01566.x
Gagea, M. I., Bateman, K. G., van Dreumel, T., McEwen, B. J., Carman, S., Archambault, M., et al. (2006). Diseases and Pathogens Associated with Mortality in Ontario Beef Feedlots. J. VET. Diagn. Invest. 18 (1), 18–28. doi:10.1177/104063870601800104
Geijtenbeek, T. B. H., and Gringhuis, S. I. (2009). Signalling through C-type Lectin Receptors: Shaping Immune Responses. Nat. Rev. Immunol. 9 (7), 465–479. doi:10.1038/nri2569
Geiser, V., and Jones, C. (2005). Localization of Sequences within the Latency-Related Gene of Bovine Herpesvirus 1 that Inhibit Mammalian Cell Growth. J. Neurovirol. 11 (6), 563–570. doi:10.1080/13550280500385286
Getter, T., Margalit, R., Kahremany, S., Levy, L., Blum, E., Khazanov, N., et al. (2019). Novel Inhibitors of Leukocyte Transendothelial Migration. Bioorg. Chem. 92, 103250. doi:10.1016/j.bioorg.2019.103250
Gondaira, S., Higuchi, H., Iwano, H., Nakajima, K., Kawai, K., Hashiguchi, S., et al. (2015). Cytokine mRNA Profiling and the Proliferative Response of Bovine Peripheral Blood Mononuclear Cells to Mycoplasma Bovis. Vet. Immunol. Immunopathology 165 (1), 45–53. doi:10.1016/j.vetimm.2015.03.002
Gondaira, S., Higuchi, H., Nishi, K., Iwano, H., and Nagahata, H. (2017). Mycoplasma Bovis Escapes Bovine Neutrophil Extracellular Traps. Vet. Microbiol. 199, 68–73. doi:10.1016/j.vetmic.2016.12.022
Gray, D., Ellis, J. A., Gow, S. P., Lacoste, S. R., Allan, G. M., and Mooney, M. H. (2019). Profiling of Local Disease-Sparing Responses to Bovine Respiratory Syncytial Virus in Intranasally Vaccinated and Challenged Calves. J. Proteomics 204, 103397. doi:10.1016/j.jprot.2019.103397
Grissett, G. P., White, B. J., and Larson, R. L. (2015). Structured Literature Review of Responses of Cattle to Viral and Bacterial Pathogens Causing Bovine Respiratory Disease Complex. J. Vet. Intern. Med. 29 (3), 770–780. doi:10.1111/jvim.12597
Heidarzadeh, M., Roodbari, F., Hassanpour, M., Ahmadi, M., Saberianpour, S., and Rahbarghazi, R. (2020). Toll-like Receptor Bioactivity in Endothelial Progenitor Cells. Cell Tissue Res 379 (2), 223–230. doi:10.1007/s00441-019-03119-2
Hellenbrand, K. M., Forsythe, K. M., Rivera-Rivas, J. J., Czuprynski, C. J., and Aulik, N. A. (2013). Histophilus Somni Causes Extracellular Trap Formation by Bovine Neutrophils and Macrophages. Microb. Pathogenesis 54, 67–75. doi:10.1016/j.micpath.2012.09.007
Hermeyer, K., Jacobsen, B., Spergser, J., Rosengarten, R., and Hewicker-Trautwein, M. (2011). Detection of Mycoplasma Bovis by In-Situ Hybridization and Expression of Inducible Nitric Oxide Synthase, Nitrotyrosine and Manganese Superoxide Dismutase in the Lungs of Experimentally-Infected Calves. J. Comp. Pathol. 145 (2), 240–250. doi:10.1016/j.jcpa.2010.12.005
Hodgins, D. C., Conlon, J. A., and Shewen, P. E. (2002). “Respiratory Viruses and Bacteria in Cattle,” in Polymicrobial Diseases (Washington (DC): ASM Press), 213–229.
Hodgson, P. D., Aich, P., Manuja, A., Hokamp, K., Roche, F. M., Brinkman, F. S. L., et al. (2005). Effect of Stress on Viral-Bacterial Synergy in Bovine Respiratory Disease: Novel Mechanisms to Regulate Inflammation. Comp. Funct. Genomics 6, 244–250. doi:10.1002/cfg.474
Hodgson, P. D., Aich, P., Stookey, J., Popowych, Y., Potter, A., Babiuk, L., et al. (2012). Stress Significantly Increases Mortality Following a Secondary Bacterial Respiratory Infection. Vet. Res. 43 (1), 21. doi:10.1186/1297-9716-43-21
Hofstetter, A. R., and Sacco, R. E. (2020). Oxidative Stress Pathway Gene Transcription after Bovine Respiratory Syncytial Virus Infection In Vitro and Ex Vivo. Vet. Immunol. Immunopathology 219, 109956. doi:10.1016/j.vetimm.2019.109956
Horvath, C. M. (2004b). The Jak-STAT Pathway Stimulated by Interferon. Sci. Signaling 2004 (260), tr8. doi:10.1126/stke.2602004tr8
Horvath, C. M. (2004a). The Jak-STAT Pathway Stimulated by Interferon or Interferon. Sci. Signaling 2004 (260), tr10. doi:10.1126/stke.2602004tr10
Hu, H., Miao, Y.-R., Jia, L.-H., Yu, Q.-Y., Zhang, Q., and Guo, A.-Y. (2018). AnimalTFDB 3.0: a Comprehensive Resource for Annotation and Prediction of Animal Transcription Factors. Nucleic Acids Res. 47 (D1), D33–D38. doi:10.1093/nar/gky822
Islam, M. A., Takagi, M., Fukuyama, K., Komatsu, R., Albarracin, L., Nochi, T., et al. (2020). Transcriptome Analysis of the Inflammatory Responses of Bovine Mammary Epithelial Cells: Exploring Immunomodulatory Target Genes for Bovine Mastitis. Pathogens 9 (3), 200. doi:10.3390/pathogens9030200
Ivashkiv, L. B., and Donlin, L. T. (2014). Regulation of Type I Interferon Responses. Nat. Rev. Immunol. 14 (1), 36–49. doi:10.1038/nri3581
Iwasaki, A., and Medzhitov, R. (2004). Toll-like Receptor Control of the Adaptive Immune Responses. Nat. Immunol. 5 (10), 987–995. doi:10.1038/ni1112
Janeway, C. A., and Medzhitov, R. (2002). Innateimmunerecognition. Annu. Rev. Immunol. 20 (1), 197–216. doi:10.1146/annurev.immunol.20.083001.084359
Jaslow, S. L., Gibbs, K. D., Fricke, W. F., Wang, L., Pittman, K. J., Mammel, M. K., et al. (2018). Salmonella Activation of STAT3 Signaling by SarA Effector Promotes Intracellular Replication and Production of IL-10. Cel Rep. 23 (12), 3525–3536. doi:10.1016/j.celrep.2018.05.072
Jiminez, J., Timsit, E., Orsel, K., van der Meer, F., Guan, L. L., and Plastow, G. (2021). Whole-Blood Transcriptome Analysis of Feedlot Cattle with and without Bovine Respiratory Disease. Front. Genet. 12, 257. doi:10.3389/fgene.2021.627623
Johnston, D., Earley, B., McCabe, M. S., Kim, J., Taylor, J. F., Lemon, K., et al. (2021a). Messenger RNA Biomarkers of Bovine Respiratory Syncytial Virus Infection in the Whole Blood of Dairy Calves. Sci. Rep. 11 (1), 9392. doi:10.1038/s41598-021-88878-1
Johnston, D., Earley, B., McCabe, M. S., Kim, J., Taylor, J. F., Lemon, K., et al. (2021b). Elucidation of the Host Bronchial Lymph Node miRNA Transcriptome Response to Bovine Respiratory Syncytial Virus. Front. Genet. 12, 526. doi:10.3389/fgene.2021.633125
Johnston, D., Earley, B., McCabe, M. S., Lemon, K., Duffy, C., McMenamy, M., et al. (2019). Experimental challenge with Bovine Respiratory Syncytial Virus in Dairy Calves: Bronchial Lymph Node Transcriptome Response. Sci. Rep. 9 (1), 14736. doi:10.1038/s41598-019-51094-z
Johnston, D., Kim, J., Taylor, J. F., Earley, B., McCabe, M. S., Lemon, K., et al. (2021c). ATAC-seq Identifies Regions of Open Chromatin in the Bronchial Lymph Nodes of Dairy Calves Experimentally Challenged with Bovine Respiratory Syncytial Virus. BMC Genomics 22 (1), 14. doi:10.1186/s12864-020-07268-5
Jones, C. (2019). Bovine Herpesvirus 1 Counteracts Immune Responses and Immune-Surveillance to Enhance Pathogenesis and Virus Transmission. Front. Immunol. 10, 1008. doi:10.3389/fimmu.2019.01008
Jones, C., and Chowdhury, S. (2010). Bovine Herpesvirus Type 1 (BHV-1) Is an Important Cofactor in the Bovine Respiratory Disease Complex. Vet. Clin. North America: Food Anim. Pract. 26 (2), 303–321. doi:10.1016/j.cvfa.2010.04.007
Joshi, V., Gupta, V. K., Bhanuprakash, A. G., Mandal, R. S. K., Dimri, U., and Ajith, Y. (2018). Haptoglobin and Serum Amyloid A as Putative Biomarker Candidates of Naturally Occurring Bovine Respiratory Disease in Dairy Calves. Microb. Pathogenesis 116, 33–37. doi:10.1016/j.micpath.2018.01.001
Kadarmideen, H. N., Watson-Haigh, N. S., and Andronicos, N. M. (2011). Systems Biology of Ovine Intestinal Parasite Resistance: Disease Gene Modules and Biomarkers. Mol. Biosyst. 7 (1), 235–246. doi:10.1039/c0mb00190b
Kadarmideen, H. N., and Watson-Haigh, N. S. (2012). Building Gene Co-expression Networks Using Transcriptomics Data for Systems Biology Investigations: Comparison of Methods Using Microarray Data. Bioinformation 8 (18), 855–861. doi:10.6026/97320630008855
Kak, G., Raza, M., and Tiwari, B. K. (2018). Interferon-gamma (IFN-γ): Exploring its Implications in Infectious Diseases. Biomol. Concepts 9 (1), 64–79. doi:10.1515/bmc-2018-0007
Kawai, T., and Akira, S. (2010). The Role of Pattern-Recognition Receptors in Innate Immunity: Update on Toll-like Receptors. Nat. Immunol. 11 (5), 373–384. doi:10.1038/ni.1863
Kawasaki, T., and Kawai, T. (2014). Toll-Like Receptor Signaling Pathways. Front. Immunol. 5, 461. doi:10.3389/fimmu.2014.00461
Kim, D., Langmead, B., and Salzberg, S. L. (2015). HISAT: a Fast Spliced Aligner with Low Memory Requirements. Nat. Methods 12 (4), 357–360. doi:10.1038/nmeth.3317
Kim, E. H., and Suresh, M. (2013). Role of PI3K/Akt Signaling in Memory CD8 T Cell Differentiation. Front. Immun. 4, 20. doi:10.3389/fimmu.2013.00020
Kirchhoff, J., Uhlenbruck, S., Goris, K., Keil, G. M., and Herrler, G. (2014). Three Viruses of the Bovine Respiratory Disease Complex Apply Different Strategies to Initiate Infection. Vet. Res. 45 (1), 20. doi:10.1186/1297-9716-45-20
Kleinschmidt, S., Spergser, J., Rosengarten, R., and Hewicker-Trautwein, M. (2013). Long-term Survival of Mycoplasma Bovis in Necrotic Lesions and in Phagocytic Cells as Demonstrated by Transmission and Immunogold Electron Microscopy in Lung Tissue from Experimentally Infected Calves. Vet. Microbiol. 162 (2), 949–953. doi:10.1016/j.vetmic.2012.11.039
Knapek, K. J., Georges, H. M., Van Campen, H., Bishop, J. V., Bielefeldt-Ohmann, H., Smirnova, N. P., et al. (2020). Fetal Lymphoid Organ Immune Responses to Transient and Persistent Infection with Bovine Viral Diarrhea Virus. Viruses 12 (8), 816. doi:10.3390/v12080816
Kolaczkowska, E., and Kubes, P. (2013). Neutrophil Recruitment and Function in Health and Inflammation. Nat. Rev. Immunol. 13 (3), 159–175. doi:10.1038/nri3399
Kommadath, A., Bao, H., Arantes, A. S., Plastow, G. S., Tuggle, C. K., Bearson, S. M., et al. (2014). Gene Co-expression Network Analysis Identifies Porcine Genes Associated with Variation in Salmonella Shedding. BMC Genomics 15 (1), 452. doi:10.1186/1471-2164-15-452
Kook, I., and Jones, C. (2016). The Serum and Glucocorticoid-Regulated Protein Kinases (SGK) Stimulate Bovine Herpesvirus 1 and Herpes Simplex Virus 1 Productive Infection. Virus. Res. 222, 106–112. doi:10.1016/j.virusres.2016.06.007
Krishnan, S., Halonen, M., and Welliver, R. C. (2004). Innate Immune Responses in Respiratory Syncytial Virus Infections. Viral Immunol. 17 (2), 220–233. doi:10.1089/0882824041310612
Kumar, H., Kawai, T., and Akira, S. (2011). Pathogen Recognition by the Innate Immune System. Int. Rev. Immunol. 30 (1), 16–34. doi:10.3109/08830185.2010.529976
Kumar, H., Kawai, T., and Akira, S. (2009). Toll-like Receptors and Innate Immunity. Biochem. Biophysical Res. Commun. 388 (4), 621–625. doi:10.1016/j.bbrc.2009.08.062
Kuzmich, N., Sivak, K., Chubarev, V., Porozov, Y., Savateeva-Lyubimova, T., and Peri, F. (2017). TLR4 Signaling Pathway Modulators as Potential Therapeutics in Inflammation and Sepsis. Vaccines 5 (4), 34. doi:10.3390/vaccines5040034
Langfelder, P., and Horvath, S. (2008). WGCNA: an R Package for Weighted Correlation Network Analysis. BMC Bioinformatics 9 (1), 559. doi:10.1186/1471-2105-9-559
Langfelder, P., Luo, R., Oldham, M. C., and Horvath, S. (2011). Is My Network Module Preserved and Reproducible. Plos Comput. Biol. 7 (1), e1001057. doi:10.1371/journal.pcbi.1001057
Law, C. W., Chen, Y., Shi, W., and Smyth, G. K. (2014). Voom: Precision Weights Unlock Linear Model Analysis Tools for RNA-Seq Read Counts. Genome Biol. 15 (2), R29. doi:10.1186/gb-2014-15-2-r29
Leach, R. J., O'Neill, R. G., Fitzpatrick, J. L., Williams, J. L., and Glass, E. J. (2012). Quantitative Trait Loci Associated with the Immune Response to a Bovine Respiratory Syncytial Virus Vaccine. PLOS ONE 7 (3), e33526. doi:10.1371/journal.pone.0033526
Lebedev, M., McEligot, H. A., Mutua, V. N., Walsh, P., Carvallo Chaigneau, F. R., and Gershwin, L. J. (2021). Analysis of Lung Transcriptome in Calves Infected with Bovine Respiratory Syncytial Virus and Treated with Antiviral And/or Cyclooxygenase Inhibitor. PLOS ONE 16 (2), e0246695. doi:10.1371/journal.pone.0246695
Lee, M. S., and Kim, Y.-J. (2007). Signaling Pathways Downstream of Pattern-Recognition Receptors and Their Cross Talk. Annu. Rev. Biochem. 76 (1), 447–480. doi:10.1146/annurev.biochem.76.060605.122847
Lee, S.-R., Nanduri, B., Pharr, G. T., Stokes, J. V., and Pinchuk, L. M. (2009). Bovine Viral Diarrhea Virus Infection Affects the Expression of Proteins Related to Professional Antigen Presentation in Bovine Monocytes. Biochim. Biophys. Acta (Bba) - Proteins Proteomics 1794 (1), 14–22. doi:10.1016/j.bbapap.2008.09.005
Levin, R., Grinstein, S., and Canton, J. (2016). The Life Cycle of Phagosomes: Formation, Maturation, and Resolution. Immunol. Rev. 273 (1), 156–179. doi:10.1111/imr.12439
Li, A., and Horvath, S. (2006). Network Neighborhood Analysis with the Multi-Node Topological Overlap Measure. Bioinformatics 23 (2), 222–231. doi:10.1093/bioinformatics/btl581
Li, L., Li, P., Chen, A., Li, H., Liu, Z., Yu, L., et al. (2021). Quantitative Proteomic Analysis Shows Involvement of the P38 MAPK Pathway in Bovine Parainfluenza Virus Type 3 Replication. Res. Square. doi:10.21203/rs.3.rs-253558/v1
Lin, J., Zhao, D., Wang, J., Wang, Y., Li, H., Yin, X., et al. (2015). Transcriptome Changes upon In Vitro challenge with Mycobacterium Bovis in Monocyte-Derived Macrophages from Bovine Tuberculosis-Infected and Healthy Cows. Vet. Immunol. Immunopathology 163 (3), 146–156. doi:10.1016/j.vetimm.2014.12.001
Lin, S.-C., Lo, Y.-C., and Wu, H. (2010). Helical Assembly in the MyD88-IRAK4-IRAK2 Complex in TLR/IL-1R Signalling. Nature 465 (7300), 885–890. doi:10.1038/nature09121
Lindholm-Perry, A. K., Kuehn, L. A., McDaneld, T. G., Miles, J. R., Workman, A. M., Chitko-McKown, C. G., et al. (2018). Complete Blood Count Data and Leukocyte Expression of Cytokine Genes and Cytokine Receptor Genes Associated with Bovine Respiratory Disease in Calves. BMC Res. Notes 11 (1), 786. doi:10.1186/s13104-018-3900-x
Lipkin, E., Strillacci, M. G., Eitam, H., Yishay, M., Schiavini, F., Soller, M., et al. (2016). The Use of Kosher Phenotyping for Mapping QTL Affecting Susceptibility to Bovine Respiratory Disease. PLoS One 11 (4), e0153423. doi:10.1371/journal.pone.0153423
Liu, B.-H., and Cai, J.-P. (2017). Identification of Transcriptional Modules and Key Genes in Chickens Infected with Salmonella enterica Serovar Pullorum Using Integrated Coexpression Analyses. Biomed. Res. Int. 2017, 1–12. doi:10.1155/2017/8347085
Liu, L., Amorín, R., Moriel, P., DiLorenzo, N., Lancaster, P. A., and Peñagaricano, F. (2020). Differential Network Analysis of Bovine Muscle Reveals Changes in Gene Coexpression Patterns in Response to Changes in Maternal Nutrition. BMC Genomics 21 (1), 684. doi:10.1186/s12864-020-07068-x
Liu, T., Zhang, L., Joo, D., and Sun, S.-C. (2017). NF-κB Signaling in Inflammation. Sig Transduct Target. Ther. 2 (1), 17023. doi:10.1038/sigtrans.2017.23
Liu, Z.-g. (2005). Molecular Mechanism of TNF Signaling and beyond. Cell Res 15 (1), 24–27. doi:10.1038/sj.cr.7290259
Lopez, B. I., Santiago, K. G., Lee, D., Ha, S., and Seo, K. (2020). RNA Sequencing (RNA-Seq) Based Transcriptome Analysis in Immune Response of Holstein Cattle to Killed Vaccine against Bovine Viral Diarrhea Virus Type I. Animals 10 (2), 344. doi:10.3390/ani10020344
Lugo-Villarino, G., Troegeler, A., Balboa, L., Lastrucci, C., Duval, C., Mercier, I., et al. (2018). The C-type Lectin Receptor DC-SIGN Has an Anti-inflammatory Role in Human M(IL-4) Macrophages in Response to Mycobacterium tuberculosis. Front. Immunol. 9, 1123. doi:10.3389/fimmu.2018.01123
Luoreng, Z.-M., Wang, X.-P., Mei, C.-G., and Zan, L.-S. (2018). Expression Profiling of Peripheral Blood miRNA Using RNAseq Technology in Dairy Cows with Escherichia Coli-Induced Mastitis. Sci. Rep. 8 (1), 12693. doi:10.1038/s41598-018-30518-2
Ma, W., Wang, H., and He, H. (2019). Bovine Herpesvirus 1 Tegument Protein UL41 Suppresses Antiviral Innate Immune Response via Directly Targeting STAT1. Vet. Microbiol. 239, 108494. doi:10.1016/j.vetmic.2019.108494
Ma, W., Wang, H., and He, H. (2020). Bta-miR-2890 Up-Regulates JAK-STAT Pathway to Inhibit BoHV-1 Replication by Targeting Viral Gene UL41. Vet. Microbiol. 245, 108709. doi:10.1016/j.vetmic.2020.108709
Maina, T., Prysliak, T., and Perez-Casal, J. (2019). Mycoplasma Bovis Delay in Apoptosis of Macrophages Is Accompanied by Increased Expression of Anti-apoptotic Genes, Reduced Cytochrome C Translocation and Inhibition of DNA Fragmentation. Vet. Immunol. Immunopathology 208, 16–24. doi:10.1016/j.vetimm.2018.12.004
Malazdrewich, C., Ames, T. R., Abrahamsen, M. S., and Maheswaran, S. K. (2001). Pulmonary Expression of Tumor Necrosis Factor Alpha, Interleukin-1 Beta, and Interleukin-8 in the Acute Phase of Bovine Pneumonic Pasteurellosis. Vet. Pathol. 38 (3), 297–310. doi:10.1354/vp.38-3-297
Malemud, C., and Pearlman, E. (2009). Targeting JAK/STAT Signaling Pathway in Inflammatory Diseases. Cst 4 (3), 201–221. doi:10.2174/157436209789057467
Marin, M. S., Quintana, S., Leunda, M. R., Odeón, A. C., and Pérez, S. E. (2016). Distribution of Bovine Alpha-Herpesviruses and Expression of Toll-like Receptors in the Respiratory System of Experimentally Infected Calves. Res. Vet. Sci. 105, 53–55. doi:10.1016/j.rvsc.2016.01.011
Mariotti, M., Williams, J., Dunner, S., Valentini, A., and Pariset, L. (2009). Polymorphisms within the Toll-like Receptor (TLR)-2, -4, and -6 Genes in Cattle. Diversity 1 (1), 7–18. doi:10.3390/d1010007
Mason, M. J., Fan, G., Plath, K., Zhou, Q., and Horvath, S. (2009). Signed Weighted Gene Co-expression Network Analysis of Transcriptional Regulation in Murine Embryonic Stem Cells. BMC Genomics 10 (1), 327. doi:10.1186/1471-2164-10-327
McGill, J. L., and Sacco, R. E. (2020). The Immunology of Bovine Respiratory Disease. Vet. Clin. North America: Food Anim. Pract. 36 (2), 333–348. doi:10.1016/j.cvfa.2020.03.002
Medzhitov, R. (2007). Recognition of Microorganisms and Activation of the Immune Response. Nature 449 (7164), 819–826. doi:10.1038/nature06246
Mitchell, G. B., Clark, M. E., Siwicky, M., and Caswell, J. L. (2008). Stress Alters the Cellular and Proteomic Compartments of Bovine Bronchoalveolar Lavage Fluid. Vet. Immunol. Immunopathology 125 (1), 111–125. doi:10.1016/j.vetimm.2008.05.005
Molina, V., Risalde, M. A., Sánchez-Cordón, P. J., Romero-Palomo, F., Pedrera, M., Garfia, B., et al. (2014). Cell-Mediated Immune Response during Experimental Acute Infection with Bovine Viral Diarrhoea Virus: Evaluation of Blood Parameters. Transbound Emerg. Dis. 61 (1), 44–59. doi:10.1111/tbed.12002
Mukund, K., and Subramaniam, S. (2015). Dysregulated Mechanisms Underlying Duchenne Muscular Dystrophy from Co-expression Network Preservation Analysis. BMC Res. Notes 8 (1), 182. doi:10.1186/s13104-015-1141-9
Mulongo, M., Prysliak, T., Scruten, E., Napper, S., Perez-Casal, J., and Blanke, S. R. (2014). In VitroInfection of Bovine Monocytes with Mycoplasma Bovis Delays Apoptosis and Suppresses Production of Gamma Interferon and Tumor Necrosis Factor Alpha but Not Interleukin-10. Infect. Immun. 82 (1), 62–71. doi:10.1128/IAI.00961-13
Muraro, S. P., De Souza, G. F., Gallo, S. W., Da Silva, B. K., De Oliveira, S. D., Vinolo, M. A. R., et al. (2018). Respiratory Syncytial Virus Induces the Classical ROS-dependent NETosis through PAD-4 and Necroptosis Pathways Activation. Sci. Rep. 8 (1), 14166. doi:10.1038/s41598-018-32576-y
Nalpas, N. C., Magee, D. A., Conlon, K. M., Browne, J. A., Healy, C., McLoughlin, K. E., et al. (2015). RNA Sequencing Provides Exquisite Insight into the Manipulation of the Alveolar Macrophage by Tubercle Bacilli. Sci. Rep. 5 (1), 13629. doi:10.1038/srep13629
Neupane, M., Kiser, J. N., Neibergs, H. L., and Neibergs, H. L. (2018). Gene Set Enrichment Analysis of SNP Data in Dairy and Beef Cattle with Bovine Respiratory Disease. Anim. Genet. 49 (6), 527–538. doi:10.1111/age.12718
Nilson, S. M., Workman, A. M., Sjeklocha, D., Brodersen, B., Grotelueschen, D. M., and Petersen, J. L. (2020). Upregulation of the Type I Interferon Pathway in Feedlot Cattle Persistently Infected with Bovine Viral Diarrhea Virus. Virus. Res. 278, 197862. doi:10.1016/j.virusres.2020.197862
N’jai, A. U., Rivera, J., Atapattu, D. N., Owusu-Ofori, K., and Czuprynski, C. J. (2013). Gene Expression Profiling of Bovine Bronchial Epithelial Cells Exposed In Vitro to Bovine Herpesvirus 1 and Mannheimia Haemolytica. Vet. Immunol. Immunopathology 155 (3), 182–189. doi:10.1016/j.vetimm.2013.06.012
Nordenfelt, P., and Tapper, H. (2011). Phagosome Dynamics during Phagocytosis by Neutrophils. J. Leukoc. Biol. 90 (2), 271–284. doi:10.1189/jlb.0810457
O'Shea, J. J., Plenge, R., and Plenge, R. (2012). JAK and STAT Signaling Molecules in Immunoregulation and Immune-Mediated Disease. Immunity 36 (4), 542–550. doi:10.1016/j.immuni.2012.03.014
O'Shea, J. J., Schwartz, D. M., Villarino, A. V., Gadina, M., McInnes, I. B., and Laurence, A. (2015). The JAK-STAT Pathway: Impact on Human Disease and Therapeutic Intervention. Annu. Rev. Med. 66 (1), 311–328. doi:10.1146/annurev-med-051113-024537
Oeckinghaus, A., and Ghosh, S. (2009). The NF- B Family of Transcription Factors and its Regulation. Cold Spring Harbor Perspect. Biol. 1 (4), a000034. doi:10.1101/cshperspect.a000034
Oguejiofor, C. F., Cheng, Z., Abudureyimu, A., Anstaett, O. L., Brownlie, J., Fouladi-Nashta, A. A., et al. (2015). Global Transcriptomic Profiling of Bovine Endometrial Immune Response In Vitro. II. Effect of Bovine Viral Diarrhea Virus on the Endometrial Response to Lipopolysaccharide1. Biol. Reprod. 93, 4. doi:10.1095/biolreprod.115.128876
Osman, R. A., and Griebel, P. J. (2017). CD335 (NKp46)+ T-Cell Recruitment to the Bovine Upper Respiratory Tract during a Primary Bovine Herpesvirus-1 Infection. Front. Immunol. 8, 1393. doi:10.3389/fimmu.2017.01393
Palomares, R. A., Parrish, J., Woolums, A. R., Brock, K. V., and Hurley, D. J. (2014). Expression of Toll-like Receptors and Co-stimulatory Molecules in Lymphoid Tissue during Experimental Infection of Beef Calves with Bovine Viral Diarrhea Virus of Low and High Virulence. Vet. Res. Commun. 38 (4), 329–335. doi:10.1007/s11259-014-9613-2
Pan, Y., Tagawa, Y., Champion, A., Sandal, I., Inzana, T. J., and Palmer, G. H. (2018). Histophilus Somni Survives in Bovine Macrophages by Interfering with Phagosome-Lysosome Fusion but Requires IbpA for Optimal Serum Resistance. Infect. Immun. 86 (12), e00365–00318. doi:10.1128/IAI.00365-18
Papayannopoulos, V. (2018). Neutrophil Extracellular Traps in Immunity and Disease. Nat. Rev. Immunol. 18 (2), 134–147. doi:10.1038/nri.2017.105
Parameswaran, N., and Patial, S. (2010). Tumor Necrosis Factor-α Signaling in Macrophages. Crit. Rev. Eukaryot. Gene Expr. 20 (2), 87–103. doi:10.1615/CritRevEukarGeneExpr.v20.i2.10
Pestka, S., Krause, C. D., Sarkar, D., Walter, M. R., Shi, Y., and Fisher, P. B. (2004). Interleukin-10andRelatedCytokines andReceptors. Annu. Rev. Immunol. 22 (1), 929–979. doi:10.1146/annurev.immunol.22.012703.104622
Pihur, V., Datta, S., and Datta, S. (2009). RankAggreg, an R Package for Weighted Rank Aggregation. BMC Bioinformatics 10 (1), 62. doi:10.1186/1471-2105-10-62
Platanias, L. C. (2005). Mechanisms of Type-I- and Type-II-Interferon-Mediated Signalling. Nat. Rev. Immunol. 5 (5), 375–386. doi:10.1038/nri1604
Platt, R., Burdett, W., and Roth, J. A. (2006). Induction of Antigen-specific T-Cell Subset Activation to Bovine Respiratory Disease Viruses by a Modified-Live Virus Vaccine. Am. J. Vet. Res. 67 (7), 1179–1184. doi:10.2460/ajvr.67.7.1179
Pollock, N., Taylor, G., Jobe, F., and Guzman, E. (2017). Modulation of the Transcription Factor NF-Κb in Antigen-Presenting Cells by Bovine Respiratory Syncytial Virus Small Hydrophobic Protein. J. Gen. Virol. 98 (7), 1587–1599. doi:10.1099/jgv.0.000855
Pompura, S. L., and Dominguez-Villar, M. (2018). The PI3K/AKT Signaling Pathway in Regulatory T-Cell Development, Stability, and Function. J. Leukoc. Biol. 103 (6), 1065–1076. doi:10.1002/JLB.2MIR0817-349R
Portis, E., Lindeman, C., Johansen, L., and Stoltman, G. (2012). A Ten-Year (2000-2009) Study of Antimicrobial Susceptibility of Bacteria that Cause Bovine Respiratory Disease Complex-Mannheimia Haemolytica, Pasteurella Multocida, and Histophilus Somni-In the United States and Canada. J. VET. Diagn. Invest. 24 (5), 932–944. doi:10.1177/1040638712457559
Quick, A. E., Ollivett, T. L., Kirkpatrick, B. W., and Weigel, K. A. (2020). Genomic Analysis of Bovine Respiratory Disease and Lung Consolidation in Preweaned Holstein Calves Using Clinical Scoring and Lung Ultrasound. J. Dairy Sci. 103 (2), 1632–1641. doi:10.3168/jds.2019-16531
Rai, A. N., Epperson, W. B., and Nanduri, B. (2015). Application of Functional Genomics for Bovine Respiratory Disease Diagnostics. Bioinform Biol. Insights 9s2, BBI.S30525. doi:10.4137/bbi.S30525
Rana, H. K., Akhtar, M. R., Islam, M. B., Ahmed, M. B., Liò, P., Quinn, J. M. W., et al. (2019). Genetic Effects of Welding Fumes on the Development of Respiratory System Diseases. Comput. Biol. Med. 108, 142–149. doi:10.1016/j.compbiomed.2019.04.004
Rangaraju, S., Dammer, E. B., Raza, S. A., Rathakrishnan, P., Xiao, H., Gao, T., et al. (2018). Identification and Therapeutic Modulation of a Pro-inflammatory Subset of Disease-Associated-Microglia in Alzheimer's Disease. Mol. Neurodegeneration 13 (1), 24. doi:10.1186/s13024-018-0254-8
Redondo, E., Gázquez, A., Vadillo, S., García, A., Franco, A., and Masot, A. J. (2014). Induction of Interleukin-8 and Interleukin-12 in Neonatal Ovine Lung Following Experimental Inoculation of Bovine Respiratory Syncytial Virus. J. Comp. Pathol. 150 (4), 434–448. doi:10.1016/j.jcpa.2013.08.002
Regev-Shoshani, G., Church, J. S., Cook, N. J., Schaefer, A. L., and Miller, C. (2013). Prophylactic Nitric Oxide Treatment Reduces Incidence of Bovine Respiratory Disease Complex in Beef Cattle Arriving at a Feedlot. Res. Vet. Sci. 95 (2), 606–611. doi:10.1016/j.rvsc.2013.06.016
Regev-Shoshani, G., Vimalanathan, S., Prema, D., Church, J. S., Reudink, M. W., Nation, N., et al. (2014). Safety, Bioavailability and Mechanism of Action of Nitric Oxide to Control Bovine Respiratory Disease Complex in Calves Entering a Feedlot. Res. Vet. Sci. 96 (2), 328–337. doi:10.1016/j.rvsc.2013.12.012
Remick, D. G. (2005). Interleukin-8. Crit. Care Med. 33 (12), S466–S467. doi:10.1097/01.Ccm.0000186783.34908.18
Riquelme Medina, I., and Lubovac-Pilav, Z. (2016). Gene Co-expression Network Analysis for Identifying Modules and Functionally Enriched Pathways in Type 1 Diabetes. PLOS ONE 11 (6), e0156006. doi:10.1371/journal.pone.0156006
Risalde, M. A., Molina, V., Sánchez-Cordón, P. J., Pedrera, M., Panadero, R., Romero-Palomo, F., et al. (2011). Response of Proinflammatory and Anti-inflammatory Cytokines in Calves with Subclinical Bovine Viral Diarrhea Challenged with Bovine Herpesvirus-1. Vet. Immunol. Immunopathology 144 (1), 135–143. doi:10.1016/j.vetimm.2011.07.022
Rodríguez, F., González, J. F., Arbelo, M., Zucca, D., and Fernández, A. (2015). Cytokine Expression in Lungs of Calves Spontaneously Infected with Mycoplasma Bovis. Vet. Res. Commun. 39 (1), 69–72. doi:10.1007/s11259-014-9620-3
Rosales, C. (2017). Fcγ Receptor Heterogeneity in Leukocyte Functional Responses. Front. Immunol. 8, 280. doi:10.3389/fimmu.2017.00280
Sabino, M., Carmelo, V. A. O., Mazzoni, G., Cappelli, K., Capomaccio, S., Ajmone-Marsan, P., et al. (2018). Gene Co-expression Networks in Liver and Muscle Transcriptome Reveal Sex-specific Gene Expression in Lambs Fed with a Mix of Essential Oils. BMC Genomics 19 (1), 236. doi:10.1186/s12864-018-4632-y
Saira, K., Zhou, Y., and Jones, C. (2007). The Infected Cell Protein 0 Encoded by Bovine Herpesvirus 1 (bICP0) Induces Degradation of Interferon Response Factor 3 and, Consequently, Inhibits Beta Interferon Promoter Activity. J. Virol. 81 (7), 3077–3086. doi:10.1128/JVI.02064-06
Salem, E., Hägglund, S., Cassard, H., Corre, T., Näslund, K., Foret, C., et al. (2019). Pathogenesis, Host Innate Immune Response, and Aerosol Transmission of Influenza D Virus in Cattle. J. Virol. 93 (7), e01853–01818. doi:10.1128/JVI.01853-18
Salleh, S. M., Mazzoni, G., Løvendahl, P., and Kadarmideen, H. N. (2018). Gene Co-expression Networks from RNA Sequencing of Dairy Cattle Identifies Genes and Pathways Affecting Feed Efficiency. BMC Bioinformatics 19 (1), 513. doi:10.1186/s12859-018-2553-z
Salojin, K., and Oravecz, T. (2007). Regulation of Innate Immunity by MAPK Dual-Specificity Phosphatases: Knockout Models Reveal New Tricks of Old Genes. J. Leukoc. Biol. 81 (4), 860–869. doi:10.1189/jlb.1006639
Schaefer, A. L., Cook, N. J., Church, J. S., Basarab, J., Perry, B., Miller, C., et al. (2007). The Use of Infrared Thermography as an Early Indicator of Bovine Respiratory Disease Complex in Calves. Res. Vet. Sci. 83 (3), 376–384. doi:10.1016/j.rvsc.2007.01.008
Schlender, J., Bossert, B., Buchholz, U., and Conzelmann, K.-K. (2000). Bovine Respiratory Syncytial Virus Nonstructural Proteins NS1 and NS2 Cooperatively Antagonize Alpha/Beta Interferon-Induced Antiviral Response. J. Virol. 74 (18), 8234–8242. doi:10.1128/JVI.74.18.8234-8242.2000
Scott, M. A., Woolums, A. R., Swiderski, C. E., Perkins, A. D., Nanduri, B., Smith, D. R., et al. (2021). Comprehensive At-Arrival Transcriptomic Analysis of post-weaned Beef Cattle Uncovers Type I Interferon and Antiviral Mechanisms Associated with Bovine Respiratory Disease Mortality. PLOS ONE 16 (4), e0250758. doi:10.1371/journal.pone.0250758
Scott, M. A., Woolums, A. R., Swiderski, C. E., Perkins, A. D., Nanduri, B., Smith, D. R., et al. (2020). Whole Blood Transcriptomic Analysis of Beef Cattle at Arrival Identifies Potential Predictive Molecules and Mechanisms that Indicate Animals that Naturally Resist Bovine Respiratory Disease. PLOS ONE 15 (1), e0227507. doi:10.1371/journal.pone.0227507
Sheridan, M. P., Regev-Shoshani, G., Martins, J., Vimalanathan, S., and Miller, C. (2016). Nitric Oxide Modulates the Immunological Response of Bovine PBMCs in an In Vitro BRDc Infection Model. Res. Vet. Sci. 109, 21–28. doi:10.1016/j.rvsc.2016.09.004
Silflow, R. M., Degel, P. M., and Harmsen, A. G. (2005). Bronchoalveolar Immune Defense in Cattle Exposed to Primary and Secondary challenge with Bovine Viral Diarrhea Virus. Vet. Immunol. Immunopathology 103 (1), 129–139. doi:10.1016/j.vetimm.2004.09.008
Singh, K., Ritchey, J. W., and Confer, A. W. (2011). Mannheimia Haemolytica. Vet. Pathol. 48 (2), 338–348. doi:10.1177/0300985810377182
Smirnova, N. P., Ptitsyn, A. A., Austin, K. J., Bielefeldt-Ohmann, H., Van Campen, H., Han, H., et al. (2009). Persistent Fetal Infection with Bovine Viral Diarrhea Virus Differentially Affects Maternal Blood Cell Signal Transduction Pathways. Physiol. Genomics 36 (3), 129–139. doi:10.1152/physiolgenomics.90276.2008
Smyth, G. K. (2005). “Limma: Linear Models for Microarray Data,” in Bioinformatics and Computational Biology Solutions Using R and Bioconductor. Editors R. Gentleman, V. J. Carey, W. Huber, R. A. Irizarry, and S. Dudoit (New York, NY: Springer New York), 397–420.
Snowder, G. D., Van Vleck, L. D., Cundiff, L. V., and Bennett, G. L. (2006). Bovine Respiratory Disease in Feedlot Cattle: Environmental, Genetic, and Economic Factors. J. Anim. Sci. 84 (8), 1999–2008. doi:10.2527/jas.2006-046
Srikumaran, S., Kelling, C. L., and Ambagala, A. (2007). Immune Evasion by Pathogens of Bovine Respiratory Disease Complex. Anim. Health Res. Rev. 8 (2), 215–229. doi:10.1017/S1466252307001326
Sun, H.-Z., Srithayakumar, V., Jiminez, J., Jin, W., Hosseini, A., Raszek, M., et al. (2020). Longitudinal Blood Transcriptomic Analysis to Identify Molecular Regulatory Patterns of Bovine Respiratory Disease in Beef Cattle. Genomics 112 (6), 3968–3977. doi:10.1016/j.ygeno.2020.07.014
Szklarczyk, D., Gable, A. L., Lyon, D., Junge, A., Wyder, S., Huerta-Cepas, J., et al. (2018). STRING V11: Protein-Protein Association Networks with Increased Coverage, Supporting Functional Discovery in Genome-wide Experimental Datasets. Nucleic Acids Res. 47 (D1), D607–D613. doi:10.1093/nar/gky1131
Takeda, K., and Akira, S. (2005). Toll-like Receptors in Innate Immunity. Int. Immunol. 17 (1), 1–14. doi:10.1093/intimm/dxh186
Takeuchi, O., and Akira, S. (2010). Pattern Recognition Receptors and Inflammation. Cell 140 (6), 805–820. doi:10.1016/j.cell.2010.01.022
Tang, D., Kang, R., Coyne, C. B., Zeh, H. J., and Lotze, M. T. (2012). PAMPs and DAMPs: Signal 0s that spur Autophagy and Immunity. Immunol. Rev. 249 (1), 158–175. doi:10.1111/j.1600-065X.2012.01146.x
Taylor, G., Wyld, S., Valarcher, J.-F., Guzman, E., Thom, M., Widdison, S., et al. (2014). Recombinant Bovine Respiratory Syncytial Virus with Deletion of the SH Gene Induces Increased Apoptosis and Pro-inflammatory Cytokines In Vitro, and Is Attenuated and Induces Protective Immunity in Calves. J. Gen. Virol. 95 (6), 1244–1254. doi:10.1099/vir.0.064931-0
Taylor, J. D., Fulton, R. W., Lehenbauer, T. W., Step, D. L., and Confer, A. W. (2010). The Epidemiology of Bovine Respiratory Disease: what Is the Evidence for Preventive Measures. Can. Vet. J. 51 (12), 1351–1359. doi:10.1128/CMR.16.1.79
Timsit, E., Dendukuri, N., Schiller, I., and Buczinski, S. (2016a). Diagnostic Accuracy of Clinical Illness for Bovine Respiratory Disease (BRD) Diagnosis in Beef Cattle Placed in Feedlots: A Systematic Literature Review and Hierarchical Bayesian Latent-Class Meta-Analysis. Prev. Vet. Med. 135, 67–73. doi:10.1016/j.prevetmed.2016.11.006
Timsit, E., Holman, D. B., Hallewell, J., and Alexander, T. W. (2016b). The Nasopharyngeal Microbiota in Feedlot Cattle and its Role in Respiratory Health. Anim. Front. 6 (2), 44–50. doi:10.2527/af.2016-0022
Tizioto, P. C., Kim, J., Seabury, C. M., Schnabel, R. D., Gershwin, L. J., Van Eenennaam, A. L., et al. (2015). Immunological Response to Single Pathogen Challenge with Agents of the Bovine Respiratory Disease Complex: An RNA-Sequence Analysis of the Bronchial Lymph Node Transcriptome. PLOS ONE 10 (6), e0131459. doi:10.1371/journal.pone.0131459
Turner, M. L., Cronin, J. G., Healey, G. D., and Sheldon, I. M. (2014). Epithelial and Stromal Cells of Bovine Endometrium Have Roles in Innate Immunity and Initiate Inflammatory Responses to Bacterial Lipopeptides In Vitro via Toll-like Receptors TLR2, TLR1, and TLR6. Endocrinology 155 (4), 1453–1465. doi:10.1210/en.2013-1822
Uribe-Querol, E., and Rosales, C. (2020). Phagocytosis: Our Current Understanding of a Universal Biological Process. Front. Immunol. 11, 1066. doi:10.3389/fimmu.2020.01066
van Dam, S., Võsa, U., van der Graaf, A., Franke, L., and de Magalhães, J. P. (2017). Gene Co-expression Analysis for Functional Classification and Gene-Disease Predictions. Brief Bioinform 19 (4), bbw139–592. doi:10.1093/bib/bbw139
Villaseñor, T., Madrid-Paulino, E., Maldonado-Bravo, R., Pérez-Martínez, L., and Pedraza-Alva, G. (2019). Mycobacterium Bovis BCG Promotes IL-10 Expression by Establishing a SYK/PKCα/β Positive Autoregulatory Loop that Sustains STAT3 Activation. Pathog. Dis. 77 (3), ftz032. doi:10.1093/femspd/ftz032
Viuff, B., Tjørnehøj, K., Larsen, L. E., Røntved, C. M., Uttenthal, A., Rønsholt, L., et al. (2002). Replication and Clearance of Respiratory Syncytial Virus. Am. J. Pathol. 161 (6), 2195–2207. doi:10.1016/S0002-9440(10)64496-3
Wang, S., Ma, X., Wang, H., and He, H. (2020a). Induction of the Unfolded Protein Response during Bovine Alphaherpesvirus 1 Infection. Viruses 12 (9), 974. doi:10.3390/v12090974
Wang, Y., Miao, L., Tao, L., Chen, J.-H., Zhu, C.-M., Li, Y., et al. (2020b). Weighted Gene Coexpression Network Analysis Identifies the Key Role Associated with Acute Coronary Syndrome. Aging 12 (19), 19440–19454. doi:10.18632/aging.103859
Wang, Z., Kong, L. C., Jia, B. Y., Chen, J. R., Dong, Y., Jiang, X. Y., et al. (2019). Analysis of the microRNA Expression Profile of Bovine Monocyte-Derived Macrophages Infected with Mycobacterium avium Subsp. Paratuberculosis Reveals that miR-150 Suppresses Cell Apoptosis by Targeting PDCD4. Ijms 20 (11), 2708. doi:10.3390/ijms20112708
Werners, A. H., and Bryant, C. E. (2012). Pattern Recognition Receptors in Equine Endotoxaemia and Sepsis. Equine Vet. J. 44 (4), 490–498. doi:10.1111/j.2042-3306.2012.00574.x
White, B. J., and Renter, D. G. (2009). Bayesian Estimation of the Performance of Using Clinical Observations and Harvest Lung Lesions for Diagnosing Bovine Respiratory Disease in Post-weaned Beef Calves. J. VET. Diagn. Invest. 21 (4), 446–453. doi:10.1177/104063870902100405
Wilkinson, A. (2009). Future of BRD Research: an Animal Health Industry Perspective. Anim. Health Res. Rev. 10 (2), 163–164. doi:10.1017/S1466252309990235
Wilkinson, J. M., Bao, H., Ladinig, A., Hong, L., Stothard, P., Lunney, J. K., et al. (2016). Genome-wide Analysis of the Transcriptional Response to Porcine Reproductive and Respiratory Syndrome Virus Infection at the Maternal/fetal Interface and in the Fetus. BMC Genomics 17 (1), 383. doi:10.1186/s12864-016-2720-4
Wu, C., Qin, X., Li, P., Pan, T., Ren, W., Li, N., et al. (2017). Transcriptomic Analysis on Responses of Murine Lungs to Pasteurella Multocida Infection. Front. Cel. Infect. Microbiol. 7, 251. doi:10.3389/fcimb.2017.00251
Xin, P., Xu, X., Deng, C., Liu, S., Wang, Y., Zhou, X., et al. (2020). The Role of JAK/STAT Signaling Pathway and its Inhibitors in Diseases. Int. Immunopharmacology 80, 106210. doi:10.1016/j.intimp.2020.106210
Xu, M., Liu, Y., Liu, Y., Li, X., Chen, G., Dong, W., et al. (2018). Genetic Polymorphisms of GZMB and Vitiligo: A Genetic Association Study Based on Chinese Han Population. Sci. Rep. 8 (1), 13001. doi:10.1038/s41598-018-31233-8
Xu, X., Zhang, K., Huang, Y., Ding, L., Chen, G., Zhang, H., et al. (2012). Bovine Herpes Virus Type 1 Induces Apoptosis through Fas-dependent and Mitochondria-Controlled Manner in Madin-Darby Bovine Kidney Cells. Virol. J. 9 (1), 202. doi:10.1186/1743-422X-9-202
Yan, Z., Huang, H., Freebern, E., Santos, D. J. A., Dai, D., Si, J., et al. (2020). Integrating RNA-Seq with GWAS Reveals Novel Insights into the Molecular Mechanism Underpinning Ketosis in Cattle. BMC Genomics 21 (1), 489. doi:10.1186/s12864-020-06909-z
Ye, S., Lowther, S., Stambas, J., and Sandri-Goldin, R. M. (2015). Inhibition of Reactive Oxygen Species Production Ameliorates Inflammation Induced by Influenza A Viruses via Upregulation of SOCS1 and SOCS3. J. Virol. 89 (5), 2672–2683. doi:10.1128/JVI.03529-14
Yip, A. M., and Horvath, S. (2007). Gene Network Interconnectedness and the Generalized Topological Overlap Measure. BMC Bioinformatics 8 (1), 22. doi:10.1186/1471-2105-8-22
Yuki, K., and Koutsogiannaki, S. (2021). Pattern Recognition Receptors as Therapeutic Targets for Bacterial, Viral and Fungal Sepsis. Int. Immunopharmacology 98, 107909. doi:10.1016/j.intimp.2021.107909
Zhang, B., and Horvath, S. (2005). A General Framework for Weighted Gene Co-expression Network Analysis. Stat. Appl. Genet. Mol. Biol. 4, 17. doi:10.2202/1544-6115.1128
Zhang, L., Huang, C., Guo, Y., Gou, X., Hinsdale, M., Lloyd, P., et al. (2015). MicroRNA-26b Modulates the NF- B Pathway in Alveolar Macrophages by Regulating PTEN. J. Immunol. 195 (11), 5404–5414. doi:10.4049/jimmunol.1402933
Zhao, X., Chu, H., Wong, B. H.-Y., Chiu, M. C., Wang, D., Li, C., et al. (2019). Activation of C-type Lectin Receptor and (RIG)-I-Like Receptors Contributes to Proinflammatory Response in Middle East Respiratory Syndrome Coronavirus-Infected Macrophages. J. Infect. Dis. 221 (4), 647–659. doi:10.1093/infdis/jiz483
Zheng, C.-H., Yuan, L., Sha, W., and Sun, Z.-L. (2014). Gene Differential Coexpression Analysis Based on Biweight Correlation and Maximum Clique. BMC Bioinformatics 15 (15), S3. doi:10.1186/1471-2105-15-S15-S3
Zheng, J., Yang, P., Tang, Y., Pan, Z., and Zhao, D. (2015). Respiratory Syncytial Virus Nonstructural Proteins Upregulate SOCS1 and SOCS3 in the Different Manner from Endogenous IFN Signaling. J. Immunol. Res. 2015, 1–11. doi:10.1155/2015/738547
Keywords: bovine respiratory disease, RNA-seq, weighted gene co-expression network, protein-protein interaction, hub-hub genes
Citation: Hasankhani A, Bahrami A, Sheybani N, Fatehi F, Abadeh R, Ghaem Maghami Farahani H, Bahreini Behzadi MR, Javanmard G, Isapour S, Khadem H and Barkema HW (2021) Integrated Network Analysis to Identify Key Modules and Potential Hub Genes Involved in Bovine Respiratory Disease: A Systems Biology Approach. Front. Genet. 12:753839. doi: 10.3389/fgene.2021.753839
Received: 05 August 2021; Accepted: 28 September 2021;
Published: 18 October 2021.
Edited by:
Andressa Oliveira De Lima, University of Washington, United StatesReviewed by:
Bárbara Silva-Vignato, University of São Paulo, BrazilCopyright © 2021 Hasankhani, Bahrami, Sheybani, Fatehi, Abadeh, Ghaem Maghami Farahani, Bahreini Behzadi, Javanmard, Isapour, Khadem and Barkema. This is an open-access article distributed under the terms of the Creative Commons Attribution License (CC BY). The use, distribution or reproduction in other forums is permitted, provided the original author(s) and the copyright owner(s) are credited and that the original publication in this journal is cited, in accordance with accepted academic practice. No use, distribution or reproduction is permitted which does not comply with these terms.
*Correspondence: Aliakbar Hasankhani, QS5oYXNhbmtoYW5pNzRAdXQuYWMuaXI=; Abolfazl Bahrami, QS5CYWhyYW1pQHV0LmFjLmly
Disclaimer: All claims expressed in this article are solely those of the authors and do not necessarily represent those of their affiliated organizations, or those of the publisher, the editors and the reviewers. Any product that may be evaluated in this article or claim that may be made by its manufacturer is not guaranteed or endorsed by the publisher.
Research integrity at Frontiers
Learn more about the work of our research integrity team to safeguard the quality of each article we publish.