- 1The African Computational Genomics (TACG) Research Group, MRC/UVRI and LSHTM, Entebbe, Uganda
- 2H3Africa Bioinformatics Network (H3ABioNet) Node, Centre for Genomics Research and Innovation, NABDA/FMST, Abuja, Nigeria
- 3Department of Medicine, University of Cape Town, Groote Schuur Hospital, Cape Town, South Africa
- 4The Department of Pathology and Molecular Medicine, Population Health Research Institute (PHRI), Michael G. DeGroote School of Medicine, McMaster University, Hamilton, ON, Canada
- 5Molecular Bio-Computation and Drug Design Laboratory, School of Health Sciences, University of KwaZulu-Natal, Westville Campus, Durban, South Africa
- 6Faculty of Health Sciences, Sydney Brenner Institute for Molecular Bioscience, University of the Witwatersrand, Johannesburg, South Africa
- 7MRC/Wits Developmental Pathways for Health Research Unit, Department of Paediatrics, Faculty of Health Sciences, University of the Witwatersrand, Johannesburg, South Africa
- 8Department of Non-Communicable Disease Epidemiology, London School of Hygiene and Tropical Medicine, London, United Kingdom
Background: White blood cell (WBC) traits and their subtypes such as basophil count (Bas), eosinophil count (Eos), lymphocyte count (Lym), monocyte count (Mon), and neutrophil counts (Neu) are known to be associated with diseases such as stroke, peripheral arterial disease, and coronary heart disease.
Methods: We meta-analyze summary statistics from genome-wide association studies in 17,802 participants from the African Partnership for Chronic Disease Research (APCDR) and African ancestry individuals from the Blood Cell Consortium (BCX2) using GWAMA. We further carried out a Bayesian fine mapping to identify causal variants driving the association with WBC subtypes. To access the causal relationship between WBC subtypes and asthma, we conducted a two-sample Mendelian randomization (MR) analysis using summary statistics of the Consortium on Asthma among African Ancestry Populations (CAAPA: ncases = 7,009, ncontrol = 7,645) as our outcome phenotype.
Results: Our metanalysis identified 269 loci at a genome-wide significant value of (p = 5 × 10−9) in a composite of the WBC subtypes while the Bayesian fine-mapping analysis identified genetic variants that are more causal than the sentinel single-nucleotide polymorphism (SNP). We found for the first time five novel genes (LOC126987/MTCO3P14, LINC01525, GAPDHP32/HSD3BP3, FLG-AS1/HMGN3P1, and TRK-CTT13-1/MGST3) not previously reported to be associated with any WBC subtype. Our MR analysis showed that Mon (IVW estimate = 0.38, CI: 0.221, 0.539, p < 0.001), Neu (IVW estimate = 0.189, CI: 0.133, 0.245, p < 0.001), and WBCc (IVW estimate = 0.185, CI: 0.108, 0.262, p < 0.001) are associated with increased risk of asthma. However, there was no evidence of causal relationship between Lym and asthma risk.
Conclusion: This study provides insight into the relationship between some WBC subtypes and asthma and potential route in the treatment of asthma and may further inform a new therapeutic approach.
Introduction
Hematological cells play critical roles in protecting the host organism against immune assault (Li et al., 2013). Dysregulation or aberration within the hematopoietic system has been implicated in several diseases, and this could as well serve as prognostic markers. For example, an aberration in leukocytes could be an indicator of lymphoma, leukemia, heart failure, polycythemia, and hypertension (Buttari et al., 2015). White blood cells (WBCs) play a major role in both innate and adaptive immune systems, serving as the primary defense system against foreign assaults. Due to the role they play in defense and immunity, they are used as biomarkers for detecting inflammation (Gopal et al., 2012). High WBC count has been linked to the pathogenesis of different disease conditions such as cardiovascular disease and cancer (Keller et al., 2014). WBCs are categorized into five subtypes based on their functions and morphology: basophils (Baso), eosinophils (Eos), lymphocytes (Lym), monocytes (Mon), and neutrophils (Neu). WBC is an averagely heritable trait, with h2 estimates between 0.14 and 0.40 across all the WBC types (Chinchilla-Vargas et al., 2020).
Asthma is a major health problem in the world, and scientific advances in the last two decades have improved our understanding and means of managing it effectively (Bateman et al., 2008). Studies have estimated the global prevalence of asthma to be 1%–18% (Masoli et al., 2004; Bateman et al., 2008; To et al., 2012). Despite its burden in Africa, asthma is classified as one of the neglected diseases with an estimated average of 12% (Asher et al., 2006; Adeloye et al., 2013; Hodonsky et al., 2020).
Several genome-wide association studies that have been carried out on WBC have reported more than 600 associated loci (Yang et al., 2007; Ferreira et al., 2009; Lo et al., 2011; Okada et al., 2011; Reiner et al., 2011; Keller et al., 2014; Astle et al., 2016). Despite the genetic diversity inherent within the African population, most WBC GWAS have been carried out in European- or East Asian-ancestry populations.
Mendelian randomization (MR) is a method that uses genetic proxies as instrumental variables and has been employed to investigate causal inference between an exposure and outcome phenotype (Fall et al., 2015).
Therefore, in this study, we performed an ancestry-specific metanalysis of GWAS of WBC and the five subtypes, Baso, Eos, Lym, Mon, and Neu in participants from two cohorts. The main aim of this study is to identify novel loci and signals associated with WBC traits and assess the causal relationships between these traits and asthma using SNPs as genetic instruments. Findings from this study may provide some biological and pathogenic insight into hematological disorders within the African population.
Methods
Study Population
APCDR Cohort
The African Partnership for Chronic Disease Research (APCDR) is an organization set out to advance collaboration of epidemiological and genomic research of non-communicable diseases in sub-Saharan Africa. The APCDR cohort comprises four studies: the Ugandan Genome Resource (UGR), the Durban Diabetes Study (DDS), the Durban Case–Control Study (DCC), and the Africa America Diabetes Mellitus Study (AADM).
DDS is a population-based study carried out among non-pregnant black African individuals resident in eThekwini municipality in Durban South Africa from November 2013 to December 2014 (Hird et al., 2016). The survey, which had 1,165 individuals, combined different socioeconomic metrics with anthropometric measurements for infectious and non-communicable diseases. A detailed description of this study population and study design can be accessed in the paper (Hird et al., 2016).
The General Population Cohort (GPC) is a two-phase sample collection study composed of UGWAS (the first sample collected) and UG2G (second samples collected). GPC is a population-based study comprising approximately 22,000 residents of Kyamulibwa located in the southwestern part of Uganda. The goal of the study was to unravel the epidemiology and genetic drivers of non-communicable and communicable diseases using an Afrocentric population (Gurdasani et al., 2019).
The Diabetes Case–Control study is a study consisting of individuals of Zulu ancestry residing in KwaZulu-Natal, aged 40 and above that have diabetes, recruited in a tertiary health facility in Durban. A total of 1,600 individuals were recruited for this study.
The AADM study is a genetic epidemiological study of individuals with type 2 diabetes and associated diseases in Africans; this study has extensively been described in other studies (Rotimi et al., 2004; Adeyemo et al., 2015).
The Blood Cell Consortium Cohort
BCX2 is a consortium comprising of trans-ethnic data of blood cell traits of 746,667 individuals from five different ancestries. From the BCX2, we retrieved WBC traits from individuals with African ancestry composed of data pulled from the BioMe™ BioBank Program, Cardiovascular Health Study, Genetic Epidemiology Research on Adult Health and Aging, Jackson Heart Study, The Multi-Ethnic Study of Atherosclerosis, and the UK Biobank African ancestry. The same units were used across the WBC traits in all the cohorts after inverse transformation [WBCc (109/L), Mon (109/L), Neu (109/L), Eos (109/L), Baso (109/L), and Lym (109/L)]. To obtain the pool effects of all the studies involved in the BCX2, an inverse variance-weighted fixed-effect meta-analyses was performed with the aid of GWAMA. For the study level association analysis, an additive genetic model of association was used to determine SNP association while a linear mixed-effect model was employed to factor in cryptic relatedness.
Hematological Phenotype
White blood and red blood indices of all participants in the DDS cohort were determined using a SYSMEX XT-2000i machine. For the UGR cohort, an 8.5-ml vacutainer was used to collect the venous blood while a 6-ml EDTA bottle was used to collect whole blood. The collected blood samples were stored at a temperature of 4–8°C. For hematological analysis, a swing bucket centrifuge was used to centrifuge the samples at 1,000–13,000 RCF (g) for 10 min.
Genotyping, Quality Control, and Imputation
Genotyping and quality control techniques have been described for each population previously (Gurdasani et al., 2019). Briefly, the DDS samples were genotyped on Illumina HumanOmni Multi-Ethnic GWAS/Exome Array employing the Infinium Assay. Illumina GenCall algorithm was used for genotype calling. The 5,000 GPC samples were genotyped on the Illumina HumanOmni 2.5M BeadChip array (Table 1). Quality control of the DDS cohort took into consideration the following criteria: exclusion of SNPs with heterozygosity > 4 SD from the mean, called proportion <97%, and sex check fails (F statistic >0.2 for women and <0.8 for men). Likewise, SNP QC ensured that called proportion was <−97%, relatedness (IBD >0.90), and Hardy–Weinberg disequilibrium (p < 10−6). Imputation was done on pre-phased data with IMPUTE2 using a merged reference label of the whole genome sequence data from the African Genome Variation Project. Affymetrix Axiom PANAFR SNP array was used to genotype the AADM data as described previously (Adeyemo et al., 2015). Genotyping, imputation, and quality control of the African ancestry cohort of the BCX2 has been described somewhere else (Chen et al., 2020a).
Meta-Analysis
Prior to metanalysis, all summary statistics data were manually checked for integrity and accuracy (i.e., summary statistics downloaded have the required variables and are appropriately labeled). Some quality control measures were applied to the summary statistics, SNPs in each cohort having MAF >0.05 were selected. The SNP association p values from the summary statistics were meta-analyzed with the aid of GWAMA (Genome-Wide Association Meta-Analysis) (Morris, 2010). We further applied genomic control, and Manhattan plots and quantile–quantile plots were plotted for the meta-analyzed result.
Statistical Fine Mapping
Following our result output from meta-analysis, we used fine-mapping analysis to pick out possible causal SNPs for the locus ±250 kb of all the lead SNPs, using a Bayesian approach (Maller et al., 2012). The Z score was used to calculate the Bayes factor for each SNP denoted as
Where K is the number of studies. The posterior probability of driving the association for each SNP was computed by
Where the summation in the denominator is over all SNPs at the locus.
Ninety-nine percent credible set sizes were derived by sorting all the SNPs according to their posterior probability
Identification of Potential Novel Variants and Locus Definition
Chen et al. (2020a) had previously performed a trans-ethnic and ancestry-specific GWAS of blood cell traits using 746,667 individuals (Chen et al., 2020b). To determine if the variants derived from our study are novel, and perhaps identify novel variants, we checked if any of our loci was reported or fall within ±250 kb window of those identified by Chen et al. A locus is further defined as ±250 kb around the significant SNPs (p < 5 × 10−09).
Two-Sample Mendelian Randomization
We performed a two-sample Mendelian randomization analysis using the R-based MR package Mendelian Randomization (Yavorska and Burgess, 2017). To identify independent genetic instruments, we used significant SNPs (p < 5 × 10−09) in the summary statistics of Lym, Mon, Neu, and WBCc derived from the metanalysis of APCDR and BCX2 summary statistics. We ensured that the instruments selected were not in LD with each other so that their impact on the exposure and outcome are uncorrelated. This was achieved by using r2 < 0.001 and a 250-kb clumping upstream and downstream of the lead SNPs. We used asthma as our outcome phenotype. These data were selected from the Consortium on Asthma among African Ancestry Populations (CAAPA; 7,009 cases and 7,645 controls) (Daya et al., 2019). Causal estimates were calculated based on IVW and sensitivity analysis was carried out using MR-Egger and median-weighted methods. For each trait, we used Q-statistics to account for heterogeneity in the instruments and also excluded SNPs that may show pleiotropy. Proxy SNP for each missing SNP was obtained from LDProxy (Machiela and Chanock, 2015).
Functional Analysis
Functional analysis of SNPs identified by the metanalysis was carried out using FUMA (Watanabe et al., 2017). Independent SNPs in linkage disequilibrium or within the same genomic location with the sentinel SNPs were separated. A p-value cutoff of p < 5 × 10−09 and 1,000 G Phase3 AFR reference panel were used. We carried out other functional analysis such as eQTL tissue expression, pathway enrichment analysis, and biological process to get more insights into the functionality of the loci identified. eQTL mapping was performed by mapping SNPs to genes up to 1 Mb and using the Blood eQTL. We used GeneCards (Stelzer et al., 2016) to determine the functions of the gene. GWAS catalogue and Open Targets were used to identify any previously associated phenotypes of the lead SNPs. Annotation of all the genes identified in this study was done using NCBI’s Genome data viewer. Pathway analysis of the identified loci was performed using Enrichr; Enrichr is an integrative web-based server that facilitates the visualization of the functional characteristics of a gene set. Enrichr is available online at http://amp.pharm.mssm.edu/Enrichr.
Results
Description of Study
The total samples analyzed for each WBC trait are shown in Table 1. WBCc is the trait with the highest number of individuals and Baso has the lowest samples analyzed.
Metanalysis of WBC From APCDR and BCX2
For each of the WBC trait subtypes: Lym: 13 SNPs, Mon: 680 SNPs, Neu: 5,308 SNPs, and WBCc: 4,462 SNPs attained genome-wide significance (p < 5 × 10−09) (Figure 1). No SNPs in the Baso and Eos were significant at p < 5 × 10−09. Using a genomic distance of ±250 kb, we identified 4, 34, 124, and 108 lead SNPs within different loci in Lym, Mon, Neu, and WBCc, respectively, at p < 5 × 10−09 [Supplementary Table S1 (ST1–ST4)]. We examined if the sentinel SNPs were within ±250 kb of SNPs reported in the Chen et al. study. When compared to this, all the SNPs in Lym fall with ±250 kb of those previously reported, while only eight SNPs in Mon, 38 SNPs in Neu, and 13 SNPs in WBCc were unique. However, 4 SNPs out of the 8 SNPs unique in Mon, 3 out of 38 in Neu, and 1 out of 13 in WBCc have been previously identified to be associated with WBC traits when queried on the GWAS catalogue (Buniello et al., 2019) and Open Targets (Koscielny et al., 2017). Two SNPs (rs1103700 and rs6693634 mapped near RPS10P8/CD1A and UHMK1/UQCRBP2) are common in Mon and Neu.
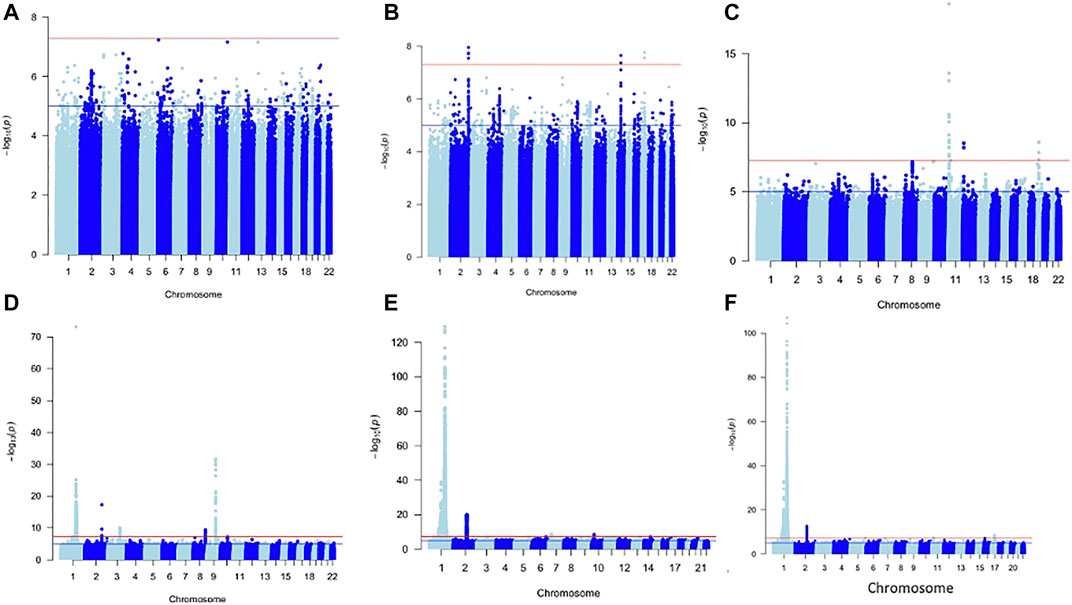
FIGURE 1. Manhattan plot of metanalysis results of Baso (A), Eos (B), Lym (C), Mon (D), Neu (E), and WBC (F).
Fine Mapping
Fine mapping seeks to analyze a trait-associated region to determine variants that are causal to a trait of interest. Fine mapping of loci was carried out within 250 kb upstream and downstream genomic distance of the sentinel variant identified via metanalysis. For each locus fine-mapped, we established the 99% credible set of SNPs that jointly make 99% of the posterior probability of driving the association (Figure 2). The fine-mapping analysis revealed that some of the lead variants such as rs369124352 (MAGI3), rs10918211 (LRRC52), and rs10800292 (LINCO1363/POU2F1) accounted for more than 50% of the posterior probability driving the association with these SNPs as the only variant within the 99% credible set. Several other variants were identified as the causal SNPs other than the sentinel SNP driving the association in WBC trait subtypes. Summary of the 99% credible set of variants driving the WBC trait can be found in Supplementary Table S2. We further went ahead and checked if these causal SNPs and their corresponding genes have been previously reported using the GWAS catalogue and Open Target; only five variants—rs11184898 (LOC126987, MTCO3P14) (Figure 3), rs907662 (LINC01525), rs7553527 (GAPDHP32, HSD3BP3), rs1923504 (FLG-AS1, HMGN3P1), and rs10733045 (TRK-CTT13-1, MGST3)—have not been reported to be associated with any WBC trait.
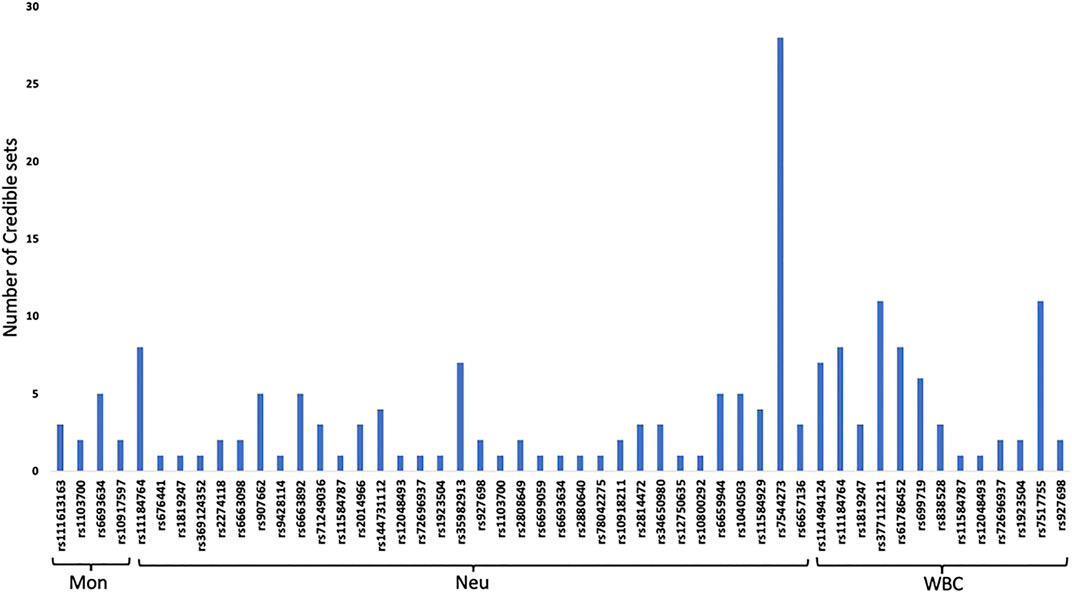
FIGURE 2. 99% credible set of SNPs that jointly make 99% of the posterior probability of driving each WBC traits.
Causal Effects of WBC Traits on Asthma
We set out to evaluate the causal relationship between WBC traits (Lym, Mon, Neu, and WBCc) and asthma. Our MR analysis identified strong positive association of Mon (IVW estimate = 0.38, CI: 0.221, 0.539, p < 0.001), Neu (IVW estimate = 0.189, CI: 0.133, 0.245, p < 0.001), and WBCc (IVW estimate = 0.185, CI: 0.108, 0.262, p < 0.001) with increased risk of asthma. However, there were no evidence of causal relationship between Lym and asthma risk (IVW estimate = −0.079, CI: −0.779, 0.621, p = 0.825) (Figure 4, Supplementary Table S3), though there was an inverse relationship between Lym and asthma risk. The causal estimates of the weighted median and the MR-egger methods of Mon showed a similar effect to the IVW method (Supplementary Table S3).
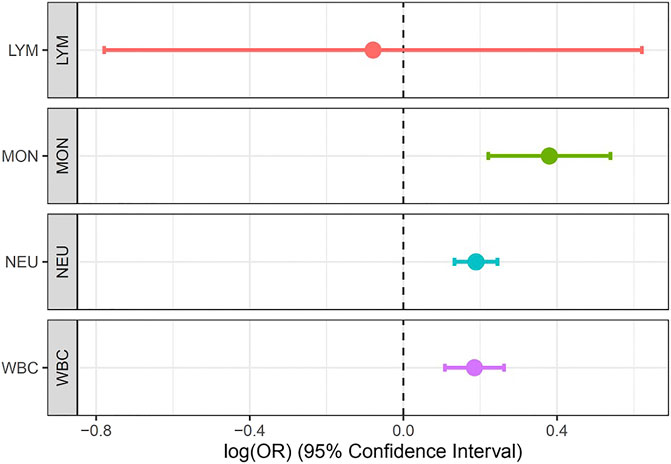
FIGURE 4. Forest plot for the association between Lym, Mon, Neu, and WBC with asthma estimated using MR-IVW method.
Functional Analysis
MAGMA analysis using GTEx v8: 54 tissue types and GTEx v8: 54 general tissue types showed significant expression in whole blood (Figure 5E). The loci were also expressed in other tissues such as stomach and the colon but not at a significant level. Lipid and atherosclerosis, nitrogen metabolism, and FoxO signaling pathway are some of the pathways enriched by these loci [Figure 5A, Supplementary Table S4 (ST1)]. These loci were mapped at a significant level to thrombocytopenia-absent radius syndrome, autoimmune lymphoproliferative syndrome, lactose intolerance, central core myopathy etc. [Figure 5B, Supplementary Table S4 (ST2)]. They are also involved in the regulation of cellular response to transforming growth factor beta stimuli, regulation of transmembrane receptor protein serine/threonine kinase signaling pathway, regulation of cellular biosynthetic process, etc. (Figure 5C), Supplementary Table S4 (ST3)] and also in exogenous lipid antigen binding molecular functions (Figure 5D).
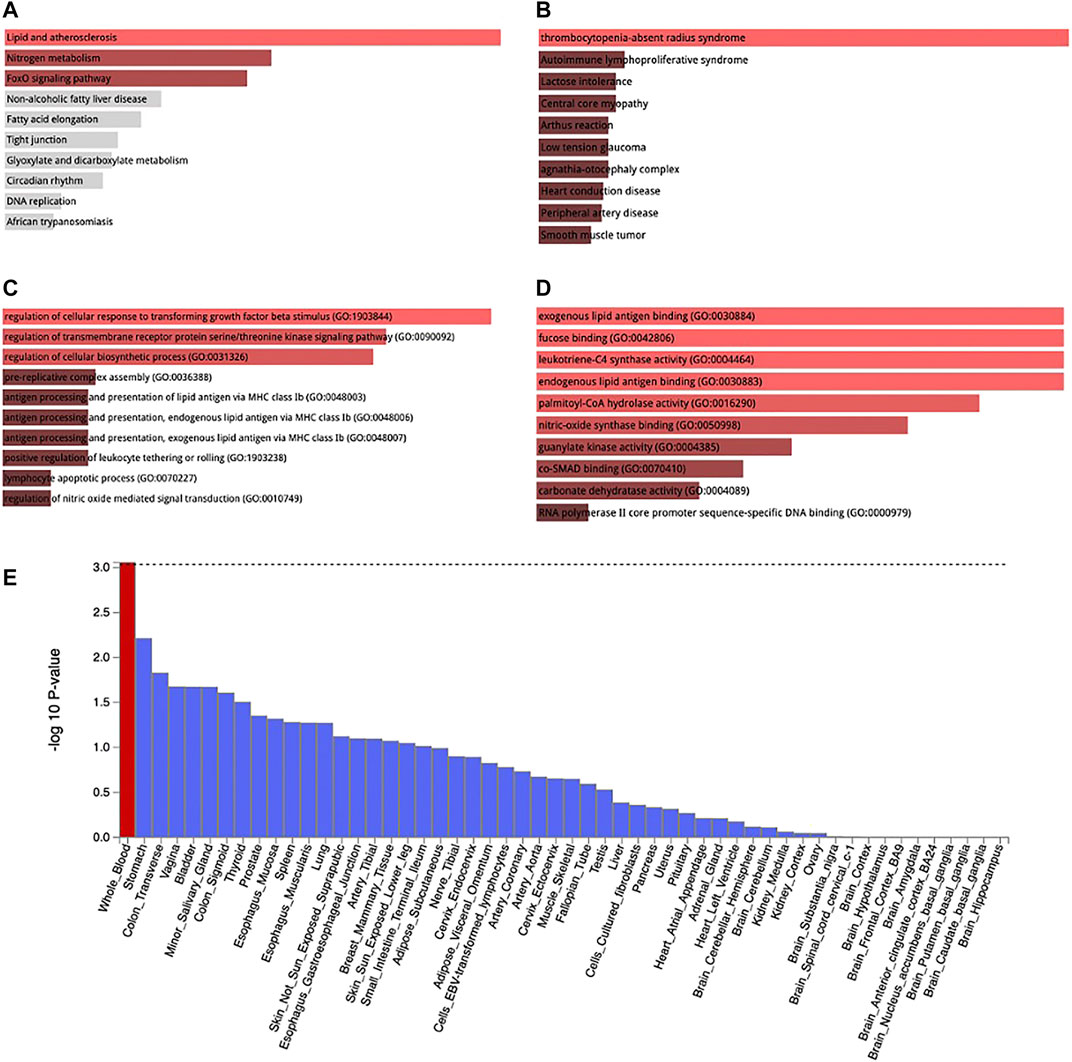
FIGURE 5. KEGG pathway enrichment (A), Jensen disease enrichment (B), Biological process (C), and Molecular function (D) of the genes associated with WBC subtypes identified by metanalysis. Tissue expression of enriched genes (E). Red bar in 3 E connotes tissues with significant enrichment while blue bars indicate tissues with no significant enrichment.
Discussion
To the best of our knowledge, this is the first study to explore the causal relationship of WBC traits and asthma in an African population. Using metanalysis and Bayesian fine mapping, we identified 269 significant SNPs associated with WBC traits. Among these, five genes (LOC126987/MTCO3P14, LINC01525, GAPDHP32/HSD3BP3, FLG-AS1, and TRK-CTT13-1/MGST3) for the first time are reported to be associated with WBC traits. We also found causal relationship between Mon, Neu, and WBCc with asthma, while no causal relationship was seen between Lym and Asthma.
FLG-AS1 (FLG Antisense RNA 1) is an RNA gene that is a member of the long non-coding RNA (lncRNA). FLG-AS1 is associated with asthma (Ferreira et al., 2019; Johansson et al., 2019; Pividori et al., 2019; Zhu et al., 2019; Olafsdottir et al., 2020), melanoma (Rashkin et al., 2020), eczema (Johansson et al., 2019; Kichaev et al., 2019), and acute myeloid leukemia (Lv et al., 2017). Microsomal glutathione S-transferase 3 encoded by MGST3 has been shown to help in cellular defense of host organisms against lipid hydroperoxides, which may arise as a result of oxidative stress (Jakobsson et al., 1997; Stamova et al., 2013). There are evidences of oxidative stress in asthma (Misso and Thompson, 2005; Andrianjafimasy et al., 2017; Sahiner et al., 2018), this may explain the enrichment of this gene in the blood and causal association with asthma.
Interestingly, our result is consistent with the multivariable MR analysis of Astle et al. (2016), which showed a protective effect of monocytes on Asthma. Furthermore, we also found a protective effect of neutrophils against asthma, consistent with Guyatt et al. (2020), which did not find substantial evidence for a harmful effect of neutrophils on asthma.
We found atherosclerosis to be significant in the KEGG pathway enrichment, and atherosclerosis is a major risk factor of coronary heart disease (CHD). Inflammation is an attribute of atherosclerosis; hence, several inflammatory cells such as Mon, Lym., Eos, and Neu have been implicated in CHD (Prentice et al., 1982; Madjid and Fatemi, 2013). Most importantly, several epidemiological studies have revealed that leukocyte count is an independent risk factor for CHD, and a risk factor for future cardiovascular events in individuals who do not have cardiovascular diseases (Weijenberg et al., 1996; Madjid et al., 2004; Chen et al., 2018). WBC count has also been suggested as a risk factor for atherosclerotic vascular diseases (Do LeeDo et al., 2001).
Significant enrichment of thrombocytopenia-absent radius syndrome (TAR), which is a rare congenital disorder (Greenhalgh et al., 2002), suggests the involvement of some WBC trait genes in the pathogenesis of this disease, as this disease is characterized by low levels of platelets in the blood (Greenhalgh et al., 2002).
Compared to other studies, one of the major strengths of this study is the use of African-ancestry data and the increased power from metanalysis. This enables the discovery of loci that have otherwise not been reported by previous studies. In addition, the CAAPA summary statistics is one of the latest cohorts of Asthma in Africa-admixed population.
We also applied different sensitivity analysis in our MR analysis, and we used strong instrumental variables by assessing the instrumental validity.
This study has its limitation, which is characteristic of two-sample MR analysis. Similar to any other non-experimental data that seek to make causal inference, where some experimentally unverifiable assumptions are made, our study is not an exception to this limitation.
Conclusively, we found evidence of causality between some WBC traits and asthma, and though some observational and MR data support these results, we believe more laboratorial experiments are needed to understand the biological mechanism of this causality.
Key Messages
• We carried out a well-powered meta-analysis genome-wide association study of white blood cell (WBC) traits in African populations.
• We identified not previously known genes driving WBC traits in an African population.
• Bayesian fine mapping identified more credible variants with high posterior probability associated with WBC subtypes.
• Mendelian Randomization Analysis found a causal relationship between monocyte count, neutrophil count, and white blood cell count with asthma in African populations.
• Findings from this study could provide more insight into the roles WBC traits play in the pathogenesis of asthma and could as well provide some directions in the treatment of asthma.
Data Availability Statement
The original contributions presented in the study are included in the article/Supplementary Material. Further inquiries can be directed to the corresponding author.
Author Contributions
SF conceptualized the study. OS led the main analyses. CS, TM, TC, and SF contributed to data analyses. OS wrote the first draft of the manuscript. TM, TC, and SF reviewed the first draft. SF and TC supervised the project. All the authors read and provided critical feedback on the paper.
Funding
SF is an international intermediate fellow funded by the Wellcome Trust grant (220740/Z/20/Z) at the MRC/UVRI and LSHTM. TC is an international training fellow supported by the Wellcome Trust grant (214205/Z/18/Z). MN acknowledges the support of Makerere University Non-Communicable Diseases (MakNCD). TM is an RHDGen PhD fellow supported by the Wellcome Trust, the University of Cape Town, and the inaugural Bongani Mayosi UCT-PHRI Scholarship (McMaster University). ON and SF are funded in part by the National Institutes of Health Common Fund to the H3ABioNet Project grant number 5U24HG006941-09.
Conflict of Interest
The authors declare that the research was conducted in the absence of any commercial or financial relationships that could be construed as a potential conflict of interest.
Publisher’s Note
All claims expressed in this article are solely those of the authors and do not necessarily represent those of their affiliated organizations, or those of the publisher, the editors, and the reviewers. Any product that may be evaluated in this article, or claim that may be made by its manufacturer, is not guaranteed or endorsed by the publisher.
Supplementary Material
The Supplementary Material for this article can be found online at: https://www.frontiersin.org/articles/10.3389/fgene.2021.749415/full#supplementary-material
References
Adeloye, D., Chan, K. Y., Rudan, I., and Campbell, H. (2013). An Estimate of Asthma Prevalence in Africa: a Systematic Analysis. Croat. Med. J. 54, 519–531. doi:10.3325/cmj.2013.54.519
Adeyemo, A. A., Tekola-Ayele, F., Doumatey, A. P., Bentley, A. R., Chen, G., Huang, H., et al. (2015). Evaluation of Genome Wide Association Study Associated Type 2 Diabetes Susceptibility Loci in Sub Saharan Africans. Front. Genet. 6, 335–339. doi:10.3389/fgene.2015.00335
Andrianjafimasy, M., Zerimech, F., Akiki, Z., Huyvaert, H., Le Moual, N., Siroux, V., et al. (2017). Oxidative Stress Biomarkers and Asthma Characteristics in Adults of the EGEA Study. Eur. Respir. J. 50, 1701193. doi:10.1183/13993003.01193-2017
Asher, M. I., Montefort, S., Björkstén, B., Lai, C. K., Strachan, D. P., Weiland, S. K., et al. (2006). Worldwide Time Trends in the Prevalence of Symptoms of Asthma, Allergic Rhinoconjunctivitis, and Eczema in Childhood: ISAAC Phases One and Three Repeat Multicountry Cross-Sectional Surveys. The Lancet 368, 733–743. doi:10.1016/s0140-6736(06)69283-0
Astle, W. J., Elding, H., Jiang, T., Allen, D., Ruklisa, D., Mann, A. L., et al. (2016). The Allelic Landscape of Human Blood Cell Trait Variation and Links to Common Complex Disease. Cell 167, 1415–1429. doi:10.1016/j.cell.2016.10.042
Bateman, E. D., Hurd, S. S., Barnes, P. J., Bousquet, J., Drazen, J. M., FitzGerald, M., et al. (2008). Global Strategy for Asthma Management and Prevention: GINA Executive Summary. Eur. Respir. J. 31, 143–178. doi:10.1183/09031936.00138707
Buniello, A., MacArthur, J. A. L., Cerezo, M., Harris, L. W., Hayhurst, J., Malangone, C., et al. (2019). The NHGRI-EBI GWAS Catalog of Published Genome-wide Association Studies, Targeted Arrays and Summary Statistics 2019. Nucleic Acids Res. 47, D1005–D1012. doi:10.1093/nar/gky1120
Buttari, B., Profumo, E., and Riganò, R. (2015). Crosstalk between Red Blood Cells and the Immune System and its Impact on Atherosclerosis. Biomed. Res. Int. 2015, 616834. doi:10.1155/2015/616834
Chen, H., Ding, X., and Li, J. (2018). White Blood Cell Count: An Independent Predictor of Coronary Heart Disease Risk in Middle-Aged and Elderly Population with Hyperuricemia. Medicine (Baltimore), 97. Available from: https://journals.lww.com/md-journal/Fulltext/2018/12210/White_blood_cell_count__an_independent_predictor.90.aspx.
Chen, M.-H., Raffield, L. M., Mousas, A., Sakaue, S., Huffman, J. E., Moscati, A., et al. (2020). Trans-ethnic and Ancestry-specific Blood-Cell Genetics in 746,667 Individuals from 5 Global Populations. Cell 182, 1198–1213. e14. doi:10.1016/j.cell.2020.06.045
Chen, M. H., Raffield, L. M., and Mousas, A. (2020). Trans-ethnic and Ancestry-specific Blood-Cell Genetics in 746,667 Individuals from 5 Global Populations. Cell.
Chinchilla-Vargas, J., Kramer, L. M., Tucker, J. D., Hubbell, D. S., Powell, J. G., Lester, T. D., et al. (2020). Genetic Basis of Blood-Based Traits and Their Relationship with Performance and Environment in Beef Cattle at Weaning. Front. Genet. 11, 717–813. doi:10.3389/fgene.2020.00717
Daya, M., Rafaels, N., Rafaels, N., Brunetti, T. M., Chavan, S., Levin, A. M., et al. (2019). Association Study in African-Admixed Populations across the Americas Recapitulates Asthma Risk Loci in Non-african Populations. Nat. Commun. 10, 880. doi:10.1038/s41467-019-08469-7
Do LeeDo, C., Folsom, A. R., and Nieto, F. J. (2001). White Blood Cell Count and Incidence of Coronary Heart Disease and Ischemic Stroke and Mortality from Cardiovascular Disease in African-American and White Men and Women: Atherosclerosis Risk in Communities Study. Am. J. Epidemiol. 154, 758–764. doi:10.1093/aje/154.8.758
Fall, T., Xie, W., Poon, W., Yaghootkar, H., Mägi, R., Knowles, J. W., et al. (2015). Using Genetic Variants to Assess the Relationship between Circulating Lipids and Type 2 Diabetes. Diabetes 64, 2676–2684. doi:10.2337/db14-1710
Ferreira, M. A. R., Hottenga, J.-J., Warrington, N. M., Medland, S. E., Willemsen, G., Lawrence, R. W., et al. (2009). Sequence Variants in Three Loci Influence Monocyte Counts and Erythrocyte Volume. Am. J. Hum. Genet. 85, 745–749. Available from. doi:10.1016/j.ajhg.2009.10.005
Ferreira, M. A. R., Mathur, R., Vonk, J. M., Szwajda, A., Brumpton, B., Granell, R., et al. (2019). Genetic Architectures of Childhood- and Adult-Onset Asthma Are Partly Distinct. Am. J. Hum. Genet. 104, 665–684. doi:10.1016/j.ajhg.2019.02.022
Gopal, S., Wood, W. A., Lee, S. J., Shea, T. C., Naresh, K. N., Kazembe, P. N., et al. (2012). Meeting the challenge of Hematologic Malignancies in Sub-saharan Africa. Blood 119, 5078–5087. doi:10.1182/blood-2012-02-387092
Greenhalgh, K. L., Howell, R. T., and Bottani, A. (2002). Thrombocytopenia-absent Radius Syndrome: a Clinical Genetic Study. J. Med. Genet. 39, 876–881881. doi:10.1136/jmg.39.12.876
Gurdasani, D., Carstensen, T., Fatumo, S., Chen, G., Franklin, C. S., Prado-Martinez, J., et al. (2019). Uganda Genome Resource Enables Insights into Population History and Genomic Discovery in Africa. Cell 179, 984–1002. doi:10.1016/j.cell.2019.10.004
Guyatt, A. L., John, C., and Williams, A. T. (2020). Mendelian Randomisation Analyses of Eosinophils and Other Blood Cell Types in Relation to Lung Function and Disease. medRxiv. doi:10.1101/2020.07.09.20148726
Hird, T. R., Young, E. H., Pirie, F. J., Riha, J., Esterhuizen, T. M., O'Leary, B., et al. (2016). Study Profile: The Durban Diabetes Study (DDS): A Platform for Chronic Disease Research. Glob. Health Epidemiol. Genom 1, e2. doi:10.1017/gheg.2015.3
Hodonsky, C. J., Baldassari, A. R., Bien, S. A., Raffield, L. M., Highland, H. M., Sitlani, C. M., et al. (2020). Ancestry-specific Associations Identified in Genome-wide Combined-Phenotype Study of Red Blood Cell Traits Emphasize Benefits of Diversity in Genomics. BMC Genomics 21, 228–314. doi:10.1186/s12864-020-6626-9
Jakobsson, P.-J., Mancini, J. A., Riendeau, D., and Ford-Hutchinson, A. W. (1997). Identification and Characterization of a Novel Microsomal Enzyme with Glutathione-dependent Transferase and Peroxidase Activities. J. Biol. Chem. 272, 22934–22939. doi:10.1074/jbc.272.36.22934
Johansson, Å., Rask-Andersen, M., Karlsson, T., and Ek, W. E. (2019). Genome-wide Association Analysis of 350 000 Caucasians from the UK Biobank Identifies Novel Loci for Asthma, hay Fever and Eczema. Hum. .Mol .Genet . 28, 4022–4041. doi:10.1093/hmg/ddz175
Keller, M. F., Reiner, A. P., Okada, Y., van Rooij, F. J. A., Johnson, A. D., Chen, M.-H., et al. (2014). Trans-ethnic Meta-Analysis of white Blood Cell Phenotypes. Hum. Mol. Genet. 23, 6944–6960. doi:10.1093/hmg/ddu401
Kichaev, G., Bhatia, G., Loh, P.-R., Gazal, S., Burch, K., Freund, M. K., et al. (2019). Leveraging Polygenic Functional Enrichment to Improve GWAS Power. Am. J. Hum. Genet. 104, 65–75. doi:10.1016/j.ajhg.2018.11.008
Koscielny, G., An, P., and Carvalho-Silva, D. (2017). Open Targets: a Platform for Therapeutic Target Identification and Validation. Nucleic Acids Res., D985–D994. doi:10.1093/nar/gkw1055
Li, J., Glessner, J. T., Zhang, H., Hou, C., Wei, Z., Bradfield, J. P., et al. (2013). GWAS of Blood Cell Traits Identifies Novel Associated Loci and Epistatic Interactions in Caucasian and African-American Children. Hum. Mol. Genet. 22, 1457–1464. doi:10.1093/hmg/dds534
Lo, K. S., Wilson, J. G., Lange, L. A., Folsom, A. R., Galarneau, G., Ganesh, S. K., et al. (2011). Genetic Association Analysis Highlights New Loci that Modulate Hematological Trait Variation in Caucasians and African Americans. Hum. Genet. 129, 307–317. doi:10.1007/s00439-010-0925-1
Lv, H., Zhang, M., Shang, Z., Li, J., Zhang, S., Lian, D., et al. (2017). Genome-wide Haplotype Association Study Identify the FGFR2 Gene as a Risk Gene for Acute Myeloid Leukemia. Oncotarget 8, 7891–7899. doi:10.18632/oncotarget.13631
Machiela, M. J., and Chanock, S. J. (2015). LDlink: a Web-Based Application for Exploring Population-specific Haplotype Structure and Linking Correlated Alleles of Possible Functional Variants: Fig. 1. Bioinformatics 31, 3555–3557. doi:10.1093/bioinformatics/btv402
Madjid, M., and Fatemi, O. (2013). Components of the Complete Blood Count as Risk Predictors for Coronary Heart Disease: In-Depth Review and Update. Tex. Hear. Inst. J. 40, 17–29. Available from: https://pubmed.ncbi.nlm.nih.gov/23467296.
Madjid, M., Awan, I., Willerson, J. T., and Casscells, S. W. (2004). Leukocyte Count and Coronary Heart Disease. J. Am. Coll. Cardiol. 44, 1945–1956. doi:10.1016/j.jacc.2004.07.056
The Wellcome Trust Case Control Consortium Maller, J. B., McVean, G., McVean, G., Byrnes, J., Vukcevic, D., Palin, K., et al. (2012). Bayesian Refinement of Association Signals for 14 Loci in 3 Common Diseases. Nat. Genet. 44, 1294–1301. doi:10.1038/ng.2435
Masoli, M., Fabian, D., Holt, S., and Beasley, R. (2004). The Global burden of Asthma: Executive Summary of the GINA Dissemination Committee Report. Allergy 59, 469–478. doi:10.1111/j.1398-9995.2004.00526.x
Misso, N. L. A., and Thompson, P. J. (2005). Oxidative Stress and Antioxidant Deficiencies in Asthma: Potential Modification by Diet. Redox Rep. 10, 247–255. doi:10.1179/135100005x70233
Morris, P. A. (2010). GWAMA: Software for Genome-wide Association Meta-Analysis. Bioinformatics 11, 288.
Okada, Y., Hirota, T., Kamatani, Y., Takahashi, A., Ohmiya, H., Kumasaka, N., et al. (2011). Identification of Nine Novel Loci Associated with white Blood Cell Subtypes in a Japanese Population. Plos Genet. 7, e1002067–15. doi:10.1371/journal.pgen.1002067
Olafsdottir, T. A., Theodors, F., Bjarnadottir, K., Bjornsdottir, U. S., Agustsdottir, A. B., Stefansson, O. A., et al. (2020). Eighty-eight Variants Highlight the Role of T Cell Regulation and Airway Remodeling in Asthma Pathogenesis. Nat. Commun. 11, 393. doi:10.1038/s41467-019-14144-8
Pividori, M., Schoettler, N., Nicolae, D. L., Ober, C., and Im, H. K. (2019). Shared and Distinct Genetic Risk Factors for Childhood-Onset and Adult-Onset Asthma: Genome-wide and Transcriptome-wide Studies. Lancet Respir. Med. 7, 509–522. doi:10.1016/s2213-2600(19)30055-4
Prentice, R. L., Szatrowski, T. P., Fujikura, T., Kato, H., Mason, M. W., and Hamilton, H. H. (1982). Leukocyte Counts and Coronary Heart Disease in a Japanese Cohort. Am. J. Epidemiol. 116, 496–509. doi:10.1093/oxfordjournals.aje.a113434
Rashkin, S. R., Graff, R. E., Kachuri, L., Thai, K. K., Alexeeff, S. E., Blatchins, M. A., et al. (2020). Pan-cancer Study Detects Genetic Risk Variants and Shared Genetic Basis in Two Large Cohorts. Nat. Commun. 11, 4423. doi:10.1038/s41467-020-18246-6
Reiner, A. P., Lettre, G., Nalls, M. A., Ganesh, S. K., Mathias, R., Austin, M. A., et al. (2011). Genome-Wide Association Study of white Blood Cell Count in 16,388 African Americans: The continental Origins and Genetic Epidemiology Network (COGENT). Plos Genet. 7, e1002108. doi:10.1371/journal.pgen.1002108
Rotimi, C. N., Chen, G., Adeyemo, A. A., Furbert-Harris, P., Guass, D., Zhou, J., et al. (2004). A Genome-wide Search for Type 2 Diabetes Susceptibility Genes in West Africans: The Africa America Diabetes Mellitus (AADM) Study. Diabetes 53, 838–841. doi:10.2337/diabetes.53.3.838
Sahiner, U. M., Birben, E., Erzurum, S., Sackesen, C., and Kalayci, Ö. (2018). Oxidative Stress in Asthma: Part of the Puzzle. Pediatr. Allergy Immunol. 29, 789–800. doi:10.1111/pai.12965
Stamova, B. S., Tian, Y., Nordahl, C. W., Shen, M. D., Rogers, S., Amaral, D. G., et al. (2013). Evidence for Differential Alternative Splicing in Blood of Young Boys with Autism Spectrum Disorders. Mol. Autism 4, 30. doi:10.1186/2040-2392-4-30
Stelzer, G., Rosen, N., Plaschkes, I., Zimmerman, S., Twik, M., Fishilevich, S., et al. (2016). The GeneCards Suite: From Gene Data Mining to Disease Genome Sequence Analyses. Curr. Protoc. Bioinformatics 54, 1–33. doi:10.30.110.1002/cpbi.5
To, T., Stanojevic, S., Moores, G., Gershon, A. S., Bateman, E. D., Cruz, A. A., et al. (2012). Global Asthma Prevalence in Adults: Findings from the Cross-Sectional World Health Survey. BMC Public Health 12, 204. doi:10.1186/1471-2458-12-204
Watanabe, K., Taskesen, E., Van Bochoven, A., and Posthuma, D. (2017). Functional Mapping and Annotation of Genetic Associations with FUMA. Nat. Commun. 8, 1–10. doi:10.1038/s41467-017-01261-5
Weijenberg, M. P., Feskens, E. J. M., and Kromhout, D. (1996). White Blood Cell Count and the Risk of Coronary Heart Disease and All-Cause Mortality in Elderly Men. Atvb 16, 499–503. doi:10.1161/01.atv.16.4.499
Yang, Q., Kathiresan, S., Lin, J. P., Tofler, G. H., and O'Donnell, C. J. (2007). Genome-wide Association and Linkage Analyses of Hemostatic Factors and Hematological Phenotypes in the framingham Heart Study. BMC Med. Genet. 8 Suppl 1, S12–S11. doi:10.1186/1471-2350-8-S1-S12
Yavorska, O. O., and Burgess, S. (2017). MendelianRandomization: an R Package for Performing Mendelian Randomization Analyses Using Summarized Data. Int. J. Epidemiol. 46, 1734–1739. doi:10.1093/ije/dyx034
Keywords: white blood cell traits, asthma, metanalysis, Mendelian randomization, fine mapping
Citation: Soremekun O, Soremekun C, Machipisa T, Soliman M, Nashiru O, Chikowore T and Fatumo S (2021) Genome-Wide Association and Mendelian Randomization Analysis Reveal the Causal Relationship Between White Blood Cell Subtypes and Asthma in Africans. Front. Genet. 12:749415. doi: 10.3389/fgene.2021.749415
Received: 29 July 2021; Accepted: 01 November 2021;
Published: 02 December 2021.
Edited by:
Tommaso Pippucci, Policlinico Sant’Orsola-Malpighi, ItalyReviewed by:
Diana Dunca, University College London, United KingdomAlbert Henry, University College London, United Kingdom
Copyright © 2021 Soremekun, Soremekun, Machipisa, Soliman, Nashiru, Chikowore and Fatumo. This is an open-access article distributed under the terms of the Creative Commons Attribution License (CC BY). The use, distribution or reproduction in other forums is permitted, provided the original author(s) and the copyright owner(s) are credited and that the original publication in this journal is cited, in accordance with accepted academic practice. No use, distribution or reproduction is permitted which does not comply with these terms.
*Correspondence: Segun Fatumo, segun.fatumo@lshtm.ac.uk