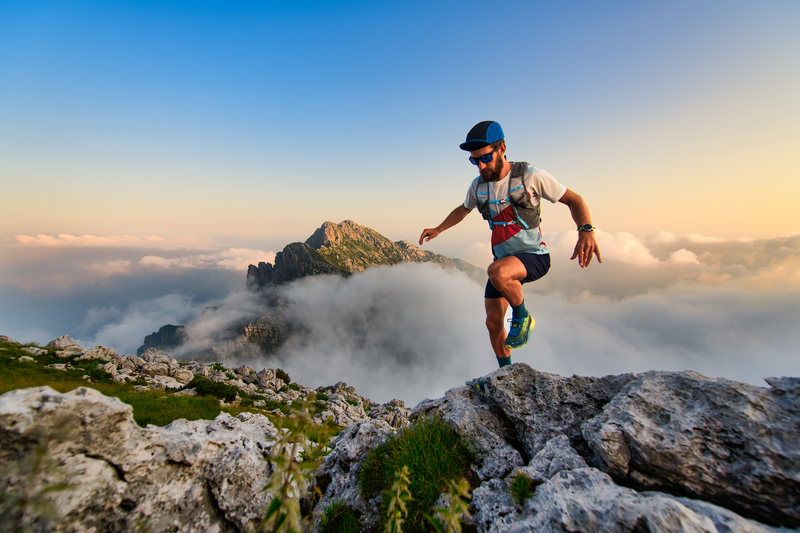
95% of researchers rate our articles as excellent or good
Learn more about the work of our research integrity team to safeguard the quality of each article we publish.
Find out more
ORIGINAL RESEARCH article
Front. Genet. , 19 October 2021
Sec. Behavioral and Psychiatric Genetics
Volume 12 - 2021 | https://doi.org/10.3389/fgene.2021.741429
Background Despite psychiatric traits were associated with intracranial aneurysms (IAs) in observational studies, their causal relationships remain largely undefined. We aimed to assess the causality between psychiatric traits and IAs.
Methods We firstly collected the genome-wide association statistics of IAs (sample size, n = 79,429) and ten psychiatric traits from Europeans, including insomnia (n = 1,331,010), mood instability (n = 363,705), anxiety disorder (n = 83,566), major depressive disorder (MDD) (n = 480,359), subjective wellbeing (n = 388,538), attention deficit/hyperactivity disorder (ADHD) (n = 53,293), autism spectrum disorder (ASD) (n = 46,350), bipolar disorder (BIP) (n = 51,710), schizophrenia (SCZ) (n = 105,318), and neuroticism (n = 168,105). We then conducted a series of bi-directional two-sample Mendelian randomization (MR) analyses, of which the Robust Adjusted Profile Score (RAPS) was the primary method to estimate the causal effects between these psychiatric traits and IAs.
Results We found that insomnia exhibited a significant risk effect on IAs with the odds ratio (OR) being 1.22 (95% CI: 1.11–1.34, p = 4.61 × 10–5) from the RAPS method. There was suggestive evidence for risk effect of mood instability on IAs (RAPS, OR = 4.16, 95% CI: 1.02–17.00, p = 0.047). However, no clear evidence of causal effects on IAs for the rest eight psychiatric traits (anxiety disorder, MDD, subjective wellbeing, ADHD, ASD, BIP, SCZ, and neuroticism) was identified. In the reverse MR analyses, no causal effects of IAs on psychiatric traits were found.
Conclusions Our findings provide strong evidence for a causal risk effect of insomnia on IAs and suggestive evidence for mood instability as a causal risk effect on IAs. These results could inform the prevention and clinical intervention of IAs.
Intracranial aneurysms (IAs) are widespread life-threatening diseases (Vlak et al., 2011). Rupture of IAs results in aneurysmal subarachnoid hemorrhage (aSAH), a form of stroke that accounts for 5% of all strokes. Half of aSAH patients are younger than 55 years and a third of the patients die within the first few days to weeks of bleeding. Most survivors suffer from long-term disability or cognitive impairment (Nieuwkamp et al., 2009).
Among patients with IAs, psychiatric traits usually co-exist. Observational studies revealed IAs and psychiatric traits like insomnia (Colledge et al., 2017; Zhang et al., 2020), mood instability (McGowan et al., 2021), anxiety disorder (Lambiase et al., 2014; Lemos et al., 2020; Zhai et al., 2020), major depressive disorder (MDD) (Henderson et al., 2013; Tang et al., 2020; Zhai et al., 2020), subjective wellbeing (Tabernero et al., 2019), attention deficit/hyperactivity disorder (ADHD) (Jeong et al., 2015), autism spectrum disorder (ASD), bipolar disorder (BIP) (Prieto et al., 2014), schizophrenia (SCZ) (Liang et al., 2016), and neuroticism (Marijnissen et al., 2014) were bidirectionally correlated. For example, Marijnissen et al. found depression was a risk factor for stroke, including IAs, via a 9-year cohort study (Marijnissen et al., 2014). Inversely, among the 200 unruptured IAs treated by endovascular intervention, 31 (15.5%) had depression and 34 (17.0%) had anxiety after discharge (Zhai et al., 2020). Another example, a meta-analysis revealed insomnia might increase the risk of future IAs formation (He et al., 2017), while the reverse relationship between insomnia and IAs was also reported (Colledge et al., 2017). However, there was insufficient evidence on whether these psychiatric traits causally lead to IAs or vice versa, owing to possible residual confounding and reverse causation bias in observational researches (van’t Hof et al., 2017).
Causal inference between diseases and complex traits could be conducted under the development of Mendelian randomization (MR) and genome-wide association studies (GWAS). MR is a causal inference method for estimating the modifiable exposure (for example, insomnia) to an outcome (for example, IAs) via taking genetic variants as instrumental variables (IVs) for the exposure (Davey Smith and Ebrahim, 2003). MR method decreases residual confounding since the IVs are initially realigned in a random manner without connection to environmental factors, behaviors, and self-selected lifestyle factors. Besides, the MR method conquers reverse causality since IVs are determined irrespective of disease progression or development. Here, we conducted bi-directional two-sample MR analyses using GWAS summary data of IAs and the ten above-mentioned psychiatric traits to infer their causality. Clarifying such causal relationships from the genetic perspective may have important implications for primary prevention strategies in IA patients.
Summary data from GWAS for IAs and eight psychiatric traits was gathered from released researches with the biggest European population sample (Table 1). For restriction, only summary data of SNPs that were significantly related to mood instability and anxiety disorders (the other two psychiatric traits) was obtained. GWAS of IAs were generated from the stage 1 association study of European ancestry, consisting of 7,495 cases and 71,934 controls passing quality control thresholds. The definitions of the ten psychiatric traits were laid out in Supplementary Table S1.
Figure 1 depicted our research workflow. The following documented valid IVs were selected based on the suppositions of MR. First, SNPs that under the threshold (p < 5 × 10–8) and associated with the exposure were selected as candidate IVs. Second, We used linkage disequilibrium (LD) (Chang et al., 2015) to further exclude dependent SNPs. Third, the significant SNPs in LD (r2 > 0.05) were filtered out based on bi-directional MR requirements (no LD or overlap in the IVs between the exposure and outcome) (Davey Smith and Hemani, 2014). Fourth, potentially pleiotropic SNPs were removed by excluding those with suggestive association with IAs (p < 10–5) (Bakker et al., 2020). The rest SNPs were defined as valid IVs for MR analyses. Supplementary Tables S2–S19 showed the valid IVs in this study. The F statistics (Lawlor et al., 2008) and the 95% confidence interval (CI) (Burgess et al., 2016) were computed to quantify whether the IVs were strongly related to the exposure. We set the p < 0.005 (0.05/10) for multiple comparisons to test each result to avoid false positive results.
FIGURE 1. The analysis flowchart of this study. 1 the possible pleiotropic SNPs which are associated with significant SNPs of outcome. 2 the possible pleiotropic SNPs which are associated with outcome (below the genome wide suggestive significant level of 10−5) Harmonize effect*: 1 Ensure that the SNPs’ effect on the exposure and outcome correlate to the same allele 2 Remove SNPs with inconsistent alleles between the exposure and outcome.
Here, we used four different methods, specifically the Robust Adjusted Profile Score (RAPS) method, the Inverse Variance Weighted (IVW) method, the Bayesian Weighted Mendelian Randomization (BWMR), and the MR (MR.Corr) method. Compared with the other three methods, the RAPS method is more efficient in dealing with residual horizontal pleiotropy and outliers, thereby is chosen as the primary method (Zhao et al., 2018; Qi and Chatterjee, 2021). RAPS is designed to conduct two-sample MR analysis with summary statistics by Zhao etc (Zhao et al., 2018). We applied the RAPS method in sensitivity analyses to confirm MR presumptions and control for overdispersion as an indicator for systematic pleiotropy. The IVW method is based on the following assumptions: the overall bias is zero, or all SNPs are valid IVs (Bowden et al., 2016). Potential heterogeneity, measured by Cochran’s Q statistic, was evaluated via multiplicative random effects IVW (Hemani et al., 2018). BWMR method could efficiently investigate the causal effect between the exposure and outcome via the standard error and p-value based on GWAS summary statistics (Zhao et al., 2020). MR.Corr is a method employing correlated instrumental variants explaining correlated horizontal pleiotropy during two-sample MR (Cheng et al., 2020). All statistics analyses were executed with two-sample MR and MR-PRESSO packages in R version 3.5.3.
The valid SNPs with IAs over that with psychiatric traits were displayed in scatter plots of Figures 2A,C; Supplementary Figure S1. After removing outlier SNPs via the MR-PRESSO outlier test, all four MR approaches approved well in fitting the linear relation between the SNPs effect on IAs and the exposures. The estimated causal effects of the ten exposures on IAs were listed in Supplementary Table S2. The result showed insomnia exhibited a significant risk effect on IAs with the odds ratio (OR) being 1.22 (95% CI: 1.11–1.34, p = 4.61 × 10–5) from RAPS, and similar risk estimates were obtained using the other three methods (IVW, OR = 1.22, 95% CI :1.11–1.34, p = 5.14 × 10–5, BWMR, OR = 1.23, 95% CI :1.11–1.36, p = 4.87 × 10–5, MR.Corr, OR = 1.23, 95% CI :1.11–1.35, p = 6.22 × 10–5, Figure 2A and Table 2). The result of RAPS method suggested mood instability had a risk effect on IAs (OR = 4.16, 95% CI: 1.02,17.00, p = 0.047, 0.005 < p < 0.05) (Figure 2C). Because the four colored solid lines merged together in Figures 2A,C, the results of the four MR methods were shown separately in Supplementary Figures S2, S3. Similar ORs from IVW, BWMR, MR.Corr methods were 3.83 (95% CI: 0.82–17.88), 3.84 (95% CI: 0.77–19.12), 3.22 (95% CI: 0.84–12.35), respectively, though they were not statistically significant (Table 2). Furthermore, funnel plots of the causal effect point estimate about insomnia and mood instability on IAs displaying a symmetric shape (Figures 2B,D). However, no genetic evidence of causal effects on IAs for the rest eight psychiatric traits (anxiety disorder, MDD, subjective wellbeing, ADHD, ASD, BIP, SCZ, and neuroticism) was identified (Supplementary Figure S1). Null of our IVs were subject to weak instrument bias, as each F statistics was not less than 32 (Table 2). Importantly, Cochran’s Q-test revealed no pleiotropic effect or horizontal heterogeneity.
FIGURE 2. Scatter plots of SNPs with IAs versus. SNPs with insomnia (A) and mood instability (C) for all the valid IVs. Each dot represents one SNP, with corresponding standard error bars of its relation to insomnia, mood instability (y-axis) and IAs (x-axis); the colored solid lines represent estimated causal effect values of four MR methods. Funnel plots of the causal effect point estimate about insomnia (B) and mood instability (D) on IAs displaying a symmetric shape; The vertical colored lines represent the estimated causal effect acquired utilizing all IVs.
Because we could only get access to significantly associated SNPs of the summary data for anxiety disorders and mood instability, MR analyses of IAs on these two traits could not be conducted. The genetic effect sizes for the rest eight psychiatric traits vs. that on IAs for the valid IVs were displayed in Supplementary Figure S4. Although each F statistic was greater than 51, indicating strong instrumental effects, no causal effects of IAs on the eight psychiatric traits were found (detailed OR, 95% CI, and p value were shown in Table 3). Additionally, Cochran’s Q-test indicated null horizontal heterogeneity or pleiotropic effect (Supplementary Figure S4).
In this study, we firstly investigated the causal relationships between the ten psychiatric traits and IAs via two-sample bi-directional MR analyses. Our results demonstrated that insomnia had a risk effect on IAs and mood instability displayed a suggestive risk effect on IAs, whereas the other eight psychiatric traits had no significant effect on IAs. In the reverse MR analyses, no evidence implicated IAs as the cause of insomnia, MDD, subjective wellbeing, ADHD, ASD, BIP, SCZ, and neuroticism. Our instrumental variables were strong enough to avoid weak instrument bias according to the F statistics. The Cochran’s Q-test and scatter plots revealed null heterogeneity or pleiotropic effect.
Emerging evidence from prospective researches indicated that insomnia was correlated with increased risk of cerebral vascular disease (Wu et al., 2014; Zheng et al., 2019), including IAs. A recent meta-analysis including 23 cohorts demonstrated that insomnia significantly increased cardio-cerebral vascular events (He et al., 2017). Bakker et al. used 376 UKB phenotypes as exposure, including insomnia, to assess the risk factor of IAs in Europeans (Bakker et al., 2020). There were 20 valid SNPs used for generalized SMR (GSMR) analysis of insomnia on IAs, with the p = 0.0514. Here, we utilized summary data of insomnia not only from UKB but also 23andMe, expanding the valid SNPs to 159. Moreover, our four MR approaches fitted well in the linear relation between the SNPs effect on IAs and insomnia. Although inconsistent with Bakker’s result, our result indicated that insomnia had a causal risk effect on IAs. The causal relationship between insomnia and IAs reinforces the notion that prevention and early diagnosis of insomnia may help prevent IAs. However, the precise mechanism linking insomnia to IAs is unclear. Some of the proposed pathophysiological mechanisms may shed light on how insomnia might predispose an individual to IAs. Insomnia symptoms may alter cerebrovascular health through elevated circulating catecholamine (Irwin et al., 1999), sympathetic nervous activity (Dettoni et al., 2012), inflammation (Grandner M. A. et al., 2013; Grandner M. et al., 2013; Ferrie et al., 2013), endocrine or metabolic dysregulation (Okun, 2011; Westerlund et al., 2013). Clinical researches revealed that insufficient sleep might affect the levels of circulating catecholamine that influence response to emotional or physical stress (Irwin et al., 1999). Further study is needed to unravel the complex connection between insomnia and the development of IAs.
It has been reported that stroke was positively associated with anxiety (Lambiase et al., 2014), depression (Surtees et al., 2008; Henderson et al., 2013; Barlinn et al., 2015), and BIP (Prieto et al., 2014). A prospective cohort of 6,019 participants revealed higher levels of anxiety symptoms correlated with increased risk of occasional stroke (Lambiase et al., 2015). Are these psychiatric traits the risk factors of IAs (one of the main event of stroke)? However, Our results demonstrated anxiety disorders, MDD, BIP were not causally related to the development of IAs. The causal risk effect of mood instability on IAs was nominal significant according to the RAPS method (p = 0.047, 0.005 < p < 0.05). Although the p-values from IVW, BWMR, and MR.Corr methods were >0.05, similar effect sizes were obtained. Therefore, mood instability may be a potential risk factor for IAs. Further studies with larger sample sizes and additional mood instability genetic instruments are required to disentangle causality.
Several randomized controlled trials (RCT) reported no significant association was observed between subjective wellbeing and cardiovascular events (Lyall et al., 2018; O'Connor et al., 2020). Consistent with these observational studies, we found that subjective wellbeing had a null causal effect on IAs.
Whether there was reverse causality between psychiatric traits (depression etc.) and stroke had been debated previously (Begovac et al., 2008; Brunner et al., 2014; Lemos et al., 2020). Here, we did not detect significant genetic evidence regarding the causality of IAs to the eight psychiatric traits (insomnia, MDD, subjective wellbeing, ADHD, ASD, BIP, SCZ, and neuroticism).
More caution is needed about applying the effect size obtained by the MR method since the OR implies the mean impact of lifetime exposure. Insomnia may differ sharply across time periods. Therefore, the risk effect of insomnia on IAs is time-dependent.
Strengths of our study included the two-sample bi-directional MR analyses and the use of summary-level data from thus far the biggest sample numbers. Hence, the possible effect of reverse causality and conventional confounders could be reduced. In addition, we conducted comprehensive analyses including four MR approaches and the heterogeneity tests to avoid potential pleiotropic effects. However, it should be noted that our results were based on European populations. Thus, we should be cautious about applying this conclusion to non-Europeans since a distinct environment may significantly impact psychiatric traits and IAs.
Several limitations of the present study should also be recognized. Firstly, there was sample overlapping between the exposure and outcome because of the summary level data. Secondly, we did not conduct MR analyses of IAs on anxiety disorders and mood instability because we could only get access to significantly associated SNPs of the summary data for anxiety disorders and mood instability. Thirdly, the follow-up analysis to interpret the biological significance of the current result was lacking.
In conclusion, we performed two-sample bi-directional MR analyses between psychiatric traits and IAs based on the large-scale GWAS summary statistics. Our findings provided strong evidence for a causal risk effect of insomnia on IAs and suggestive evidence for mood instability as a causal risk effect on IAs. There was no genetic support for a causal effect of the other eight psychiatric traits (anxiety disorder, MDD, subjective wellbeing, ADHD, ASD, BIP, SCZ, and neuroticism) on IAs. In the reverse MR analyses, no genetic evidence implicated IAs as the cause of insomnia, MDD, subjective wellbeing, ADHD, ASD, BIP, SCZ, and neuroticism.
The original contributions presented in the study are included in the article/Supplementary Material, further inquiries can be directed to the corresponding author.
Ethical review and approval was not required for the study on human participants in accordance with the local legislation and institutional requirements. Written informed consent for participation was not required for this study in accordance with the national legislation and the institutional requirements.
Concept and design: PP, ZC, XZ, ZG, FW. Acquisition, analysis, or interpretation of data: PP, ZC, FW. Drafting of the article: PP, XZ, FW. Critical revision of the article for important intellectual content: PP, ZC, XZ, ZG, FD, YX, YH, DG, FW. Statistical analysis: PP, ZG, FD, YX. Obtained funding: FW. Administrative, technical, or material support: YH, DG, FW. Supervision: FW.
This study was supported by the grant of the Natural Science Foundation of China (NSFC: 82072795).
The authors declare that the research was conducted in the absence of any commercial or financial relationships that could be construed as a potential conflict of interest.
All claims expressed in this article are solely those of the authors and do not necessarily represent those of their affiliated organizations, or those of the publisher, the editors and the reviewers. Any product that may be evaluated in this article, or claim that may be made by its manufacturer, is not guaranteed or endorsed by the publisher.
The Supplementary Material for this article can be found online at: https://www.frontiersin.org/articles/10.3389/fgene.2021.741429/full#supplementary-material
Bakker, M. K., van der Spek, R. A. A., van Rheenen, W., Morel, S., Bourcier, R., Hostettler, I. C., et al. (2020). Genome-wide Association Study of Intracranial Aneurysms Identifies 17 Risk Loci and Genetic Overlap with Clinical Risk Factors. Nat. Genet. 52 (12), 1303–1313. doi:10.1038/s41588-020-00725-7
Barlinn, K., Kepplinger, J., Puetz, V., Illigens, B., Bodechtel, U., and Siepmann, T. (2015). Exploring the Risk-Factor Association between Depression and Incident Stroke: a Systematic Review and Meta-Analysis. NDT 11, 1–14. doi:10.2147/NDT.S63904
Begovac, B., Begovac, I., and Paladino, J. (2008). Delusional Disorder of the Paranoid Type and Unruptured Intracerebral Aneurysm-Iis There an Association? Acta Med. Croatica 62 (1), 61–64.
Bowden, J., Del Greco M., F., Minelli, C., Davey Smith, G., Sheehan, N. A., and Thompson, J. R. (2016). Assessing the Suitability of Summary Data for Two-Sample Mendelian Randomization Analyses Using MR-Egger Regression: the Role of the I2 Statistic. Int. J. Epidemiol. 45 (6), dyw220–1974. doi:10.1093/ije/dyw220
Brunner, E. J., Shipley, M. J., Britton, A. R., Stansfeld, S. A., Heuschmann, P. U., Rudd, A. G., et al. (2014). Depressive Disorder, Coronary Heart Disease, and Stroke: Dose-Response and Reverse Causation Effects in the Whitehall II Cohort Study. Eur. J. Prev. Cardiolog 21 (3), 340–346. doi:10.1177/2047487314520785
Burgess, S., Davies, N. M., and Thompson, S. G. (2016). Bias Due to Participant Overlap in Two‐sample Mendelian Randomization. Genet. Epidemiol. 40 (7), 597–608. doi:10.1002/gepi.21998
Chang, C. C., Chow, C. C., Tellier, L. C., Vattikuti, S., Purcell, S. M., and Lee, J. J. (2015). Second-generation PLINK: Rising to the challenge of Larger and Richer Datasets. GigaSci 4, 7. doi:10.1186/s13742-015-0047-8
Cheng, Q., Sun, B., Xia, Y., and Liu, J. (2020). Accounting for Correlated Horizontal Pleiotropy in Two-Sample Mendelian Randomization Using Correlated Instrumental Variants. Ithaca, NY: arXiv. arXiv: Methodology.
Colledge, F., Brand, S., Pühse, U., Holsboer-Trachsler, E., Zimmerer, S., Schleith, R., et al. (2017). A Twelve-Week Moderate Exercise Programme Improved Symptoms of Depression, Insomnia, and Verbal Learning in Post-Aneurysmal Subarachnoid Haemorrhage Patients: A Comparison with Meningioma Patients and Healthy Controls. Neuropsychobiology 76 (2), 59–71. doi:10.1159/000486903
Davey Smith, G., and Ebrahim, S. (2003). Mendelian Randomization': Can Genetic Epidemiology Contribute to Understanding Environmental Determinants of Disease? Int. J. Epidemiol. 32 (1), 1–22. doi:10.1093/ije/dyg070
Davey Smith, G., and Hemani, G. (2014). Mendelian Randomization: Genetic Anchors for Causal Inference in Epidemiological Studies. Hum. Mol. Genet. 23 (R1), R89–R98. doi:10.1093/hmg/ddu328
Dettoni, J. L., Consolim-Colombo, F. M., Drager, L. F., Rubira, M. C., Cavasin de Souza, S. B. P., Irigoyen, M. C., et al. (2012). Cardiovascular Effects of Partial Sleep Deprivation in Healthy Volunteers. J. Appl. Physiol. 113 (2), 232–236. doi:10.1152/japplphysiol.01604.2011
Ferrie, J. E., Kivimaki, M., Akbaraly, T. N., Singh-Manoux, A., Miller, M. A., Gimeno, D., et al. (2013). Associations between Change in Sleep Duration and Inflammation: Findings on C-Reactive Protein and Interleukin 6 in the Whitehall II Study. Am. J. Epidemiol. 178 (6), 956–961. doi:10.1093/aje/kwt072
Grandner, M. A., Buxton, O. M., Jackson, N., Sands-Lincoln, M., Pandey, A., and Jean-Louis, G. (2013a). Extreme Sleep Durations and Increased C-Reactive Protein: Effects of Sex and Ethnoracial Group. Sleep 36 (5), 769–779. doi:10.5665/sleep.2646
Grandner, M., Sands-Lincoln, M. R., Pak, V. M., and Garland, S. N. (2013b). Sleep Duration, Cardiovascular Disease, and Proinflammatory Biomarkers. NSS 5, 93–107. doi:10.2147/nss.s31063
He, Q., Zhang, P., Li, G., Dai, H., and Shi, J. (2017). The Association between Insomnia Symptoms and Risk of Cardio-Cerebral Vascular Events: A Meta-Analysis of Prospective Cohort Studies. Eur. J. Prev. Cardiolog 24 (10), 1071–1082. doi:10.1177/2047487317702043
Hemani, G., Bowden, J., and Davey Smith, G. (2018). Evaluating the Potential Role of Pleiotropy in Mendelian Randomization Studies. Hum. Mol. Genet. 27 (R2), R195–r208. doi:10.1093/hmg/ddy163
Henderson, K. M., Clark, C. J., Lewis, T. T., Aggarwal, N. T., Beck, T., Guo, H., et al. (2013). Psychosocial Distress and Stroke Risk in Older Adults. Stroke 44 (2), 367–372. doi:10.1161/strokeaha.112.679159
Irwin, M., Thompson, J., Miller, C., Gillin, J. C., and Ziegler, M. (1999). Effects of Sleep and Sleep Deprivation on Catecholamine and Interleukin-2 Levels in Humans: Clinical Implications1. J. Clin. Endocrinol. Metab. 84 (6), 1979–1985. doi:10.1210/jcem.84.6.5788
Jeong, S. H., Choi, K.-S., Lee, K. Y., Kim, E.-J., Kim, Y.-S., and Joo, E.-J. (2015). Association between the Dopamine Transporter Gene (DAT1) and Attention Deficit Hyperactivity Disorder-Related Traits in Healthy Adults. Psychiatr. Genet. 25 (3), 119–126. doi:10.1097/YPG.0000000000000086
Lambiase, M. J., Kubzansky, L. D., and Thurston, R. C. (2015). Positive Psychological Health and Stroke Risk: The Benefits of Emotional Vitality. Health Psychol. 34 (10), 1043–1046. doi:10.1037/hea0000228
Lambiase, M. J., Kubzansky, L. D., and Thurston, R. C. (2014). Prospective Study of Anxiety and Incident Stroke. Stroke 45 (2), 438–443. doi:10.1161/STROKEAHA.113.003741
Lawlor, D. A., Harbord, R. M., Sterne, J. A. C., Timpson, N., and Davey Smith, G. (2008). Mendelian Randomization: Using Genes as Instruments for Making Causal Inferences in Epidemiology. Statist. Med. 27 (8), 1133–1163. doi:10.1002/sim.3034
Lemos, M., Román-Calderón, J. P., Calle, G., Gómez-Hoyos, J. F., and Jimenez, C. M. (2020). Personality and Anxiety Are Related to Health-Related Quality of Life in Unruptured Intracranial Aneurysm Patients Selected for Non-intervention: A Cross Sectional Study. PLoS One 15 (3), e0229795. doi:10.1371/journal.pone.0229795
Liang, Y., Huang, J., Tian, J., Cao, Y., Zhang, G., Wang, C., et al. (2016). The Prevalence and Risk Factors of Stroke in Patients with Chronic Schizophrenia. Ndt 12, 1131–1134. doi:10.2147/NDT.S106663
Lyall, L. M., Wyse, C. A., Graham, N., Ferguson, A., Lyall, D. M., Cullen, B., et al. (2018). Association of Disrupted Circadian Rhythmicity with Mood Disorders, Subjective Wellbeing, and Cognitive Function: a Cross-Sectional Study of 91 105 Participants from the UK Biobank. Lancet Psychiatry 5 (6), 507–514. doi:10.1016/s2215-0366(18)30139-1
Marijnissen, R. M., Wouts, L., Schoevers, R. A., Bremmer, M. A., Beekman, A. T. F., Comijs, H. C., et al. (2014). Depression in Context of Low Neuroticism Is a Risk Factor for Stroke: a 9-year Cohort Study. Neurology 83 (19), 1692–1698. doi:10.1212/WNL.0000000000000955
McGowan, N. M., Nichols, M., Bilderbeck, A. C., Goodwin, G. M., and Saunders, K. E. A. (2021). Blood Pressure in Bipolar Disorder: Evidence of Elevated Pulse Pressure and Associations between Mean Pressure and Mood Instability. Int. J. Bipolar Disord. 9 (1), 5. doi:10.1186/s40345-020-00209-x
Nieuwkamp, D. J., Setz, L. E., Algra, A., Linn, F. H., de Rooij, N. K., and Rinkel, G. J. (2009). Changes in Case Fatality of Aneurysmal Subarachnoid Haemorrhage over Time, According to Age, Sex, and Region: a Meta-Analysis. Lancet Neurol. 8 (7), 635–642. doi:10.1016/S1474-4422(09)70126-7
O’Connor, E. A., Evans, C. V., Rushkin, M. C., Redmond, N., and Lin, J. S. (2020). Behavioral Counseling to Promote a Healthy Diet and Physical Activity for Cardiovascular Disease Prevention in Adults with Cardiovascular Risk Factors. Jama 324 (20), 2076–2094. doi:10.1001/jama.2020.17108
Okun, M. L. (2011). Biological Consequences of Disturbed Sleep: Important Mediators of Health?1. Jpn. Psychol. Res. 53 (2), 163–176. doi:10.1111/j.1468-5884.2011.00463.x
Prieto, M. L., Cuéllar-Barboza, A. B., Bobo, W. V., Roger, V. L., Bellivier, F., Leboyer, M., et al. (2014). Risk of Myocardial Infarction and Stroke in Bipolar Disorder: a Systematic Review and Exploratory Meta-Analysis. Acta Psychiatr. Scand. 130 (5), 342–353. doi:10.1111/acps.12293
Qi, G., and Chatterjee, N. (2021). A Comprehensive Evaluation of Methods for Mendelian Randomization Using Realistic Simulations and an Analysis of 38 Biomarkers for Risk of Type 2 Diabetes. Int. J. Epidemiol. 50 (4), 1335–1349. doi:10.1093/ije/dyaa262
Surtees, P. G., Wainwright, N. W. J., Luben, R. N., Wareham, N. J., Bingham, S. A., and Khaw, K.-T. (2008). Psychological Distress, Major Depressive Disorder, and Risk of Stroke. Neurology 70 (10), 788–794. doi:10.1212/01.wnl.0000304109.18563.81
Tabernero, C., Gutiérrez-Domingo, T., Vecchione, M., Cuadrado, E., Castillo-Mayén, R., Rubio, S., et al. (2019). A Longitudinal Study on Perceived Health in Cardiovascular Patients: The Role of Conscientiousness, Subjective Wellbeing and Cardiac Self-Efficacy. PLoS One 14 (10), e0223862. doi:10.1371/journal.pone.0223862
Tang, W. K., Wang, L., Kwok Chu Wong, G., Ungvari, G. S., Yasuno, F., Tsoi, K. K. F., et al. (2020). Depression after Subarachnoid Hemorrhage: A Systematic Review. J. Stroke 22 (1), 11–28. doi:10.5853/jos.2019.02103
van 't Hof, F. N., Vaucher, J., Holmes, M. V., de Wilde, A., Baas, A. F., Blankensteijn, J. D., et al. (2017). Genetic Variants Associated with Type 2 Diabetes and Adiposity and Risk of Intracranial and Abdominal Aortic Aneurysms. Eur. J. Hum. Genet. 25 (6), 758–762. doi:10.1038/ejhg.2017.48
Vlak, M. H., Algra, A., Brandenburg, R., and Rinkel, G. J. (2011). Prevalence of Unruptured Intracranial Aneurysms, with Emphasis on Sex, Age, Comorbidity, Country, and Time Period: a Systematic Review and Meta-Analysis. Lancet Neurol. 10 (7), 626–636. doi:10.1016/S1474-4422(11)70109-0
Westerlund, A., Bellocco, R., Sundström, J., Adami, H.-O., Åkerstedt, T., and Trolle Lagerros, Y. (2013). Sleep Characteristics and Cardiovascular Events in a Large Swedish Cohort. Eur. J. Epidemiol. 28 (6), 463–473. doi:10.1007/s10654-013-9802-2
Wu, M.-P., Lin, H.-J., Weng, S.-F., Ho, C.-H., Wang, J.-J., and Hsu, Y.-W. (2014). Insomnia Subtypes and the Subsequent Risks of Stroke. Stroke 45 (5), 1349–1354. doi:10.1161/STROKEAHA.113.003675
Zhai, X.-D., Yu, J.-X., Ma, Y.-J., Xiang, S.-S., Li, G.-L., He, C., et al. (2020). Prevalence of and Risk Factors for Anxiety and Depression in Chinese Patients with Unruptured Intracranial Aneurysms Treated by Endovascular Intervention. BMC Psychiatry 20 (1), 430. doi:10.1186/s12888-020-02834-3
Zhang, S., Shen, Y., Zhou, C., Zhu, W., Zhang, F., Hu, J., et al. (2020). Association between Sleep Duration and Rupture of Intracranial Aneurysms: A Single-center Retrospective Study. J. Clin. Neurosci. 81, 252–258. doi:10.1016/j.jocn.2020.09.060
Zhao, J., Ming, J., Hu, X., Chen, G., Liu, J., and Yang, C. (2020). Bayesian Weighted Mendelian Randomization for Causal Inference Based on Summary Statistics. Bioinformatics 36 (5), 1501–1508. doi:10.1093/bioinformatics/btz749
Zhao, Q., Wang, J., Hemani, G., Bowden, J., and Small, D. S. (2018). Statistical Inference in Two-Sample Summary-Data Mendelian Randomization Using Robust Adjusted Profile Score. Ithaca, NY: arXiv. arXiv: Applications.
Keywords: psychiatric traits, intracranial aneurysms, mendelian randomization, causality, GWAS summary statistics
Citation: Peng P, Chen Z, Zhang X, Guo Z, Dong F, Xu Y, He Y, Guo D and Wan F (2021) Investigating Causal Relationships Between Psychiatric Traits and Intracranial Aneurysms: A Bi-directional Two-Sample Mendelian Randomization Study. Front. Genet. 12:741429. doi: 10.3389/fgene.2021.741429
Received: 20 July 2021; Accepted: 07 October 2021;
Published: 19 October 2021.
Edited by:
Jian-Huan Chen, Jiangnan University, ChinaCopyright © 2021 Peng, Chen, Zhang, Guo, Dong, Xu, He, Guo and Wan. This is an open-access article distributed under the terms of the Creative Commons Attribution License (CC BY). The use, distribution or reproduction in other forums is permitted, provided the original author(s) and the copyright owner(s) are credited and that the original publication in this journal is cited, in accordance with accepted academic practice. No use, distribution or reproduction is permitted which does not comply with these terms.
*Correspondence: Feng Wan, d2FucnVpeWFuQGhvdG1haWwuY29t
Disclaimer: All claims expressed in this article are solely those of the authors and do not necessarily represent those of their affiliated organizations, or those of the publisher, the editors and the reviewers. Any product that may be evaluated in this article or claim that may be made by its manufacturer is not guaranteed or endorsed by the publisher.
Research integrity at Frontiers
Learn more about the work of our research integrity team to safeguard the quality of each article we publish.