- 1Department of Respiratory and Critical Care Medicine, Maoming People’s Hospital, Maoming, China
- 2Department of Otolaryngology, Maoming People’s Hospital, Maoming, China
- 3Department of Pediatrics, Fogang County Hospital of Traditional Chinese Medicine, Qingyuan, China
- 4Southern Medical University, Guangzhou, China
- 5Division of Pulmonary, Critical Care and Sleep Medicine, Marshall University, Huntington, WV, United States
Background: Lung adenocarcinoma is one of the most common malignant tumors of the respiratory system, ranking first in morbidity and mortality among all cancers. This study aims to establish a ferroptosis-related gene-based prognostic model to investigate the potential prognosis of lung adenocarcinoma.
Methods: We obtained gene expression data with matching clinical data of lung adenocarcinoma from the The Cancer Genome Atlas (TCGA) and Gene Expression Omnibus (GEO) databases. The ferroptosis-related genes (FRGs) were downloaded from three subgroups in the ferroptosis database. Using gene expression differential analysis, univariate Cox regression, and LASSO regression analysis, seven FRGs with prognostic significance were identified. The result of multivariate Cox analysis was utilized to calculate regression coefficients and establish a risk-score formula that divided patients with lung adenocarcinoma into high-risk and low-risk groups. The TCGA results were validated using GEO data sets. Then we observed that patients divided in the low-risk group lived longer than the overall survival (OS) of the other. Then we developed a novel nomogram including age, gender, clinical stage, TNM stage, and risk score.
Results: The areas under the curves (AUCs) for 3- and 5-years OS predicted by the model were 0.823 and 0.852, respectively. Calibration plots and decision curve analysis also confirmed the excellent predictive performance of the model. Subsequently, gene function enrichment analysis revealed that the identified FRGs are important in DNA replication, cell cycle regulation, cell adhesion, chromosomal mutation, oxidative phosphorylation, P53 signaling pathway, and proteasome processes.
Conclusions: Our results verified the prognostic significance of FRGs in patients with lung adenocarcinoma, which may regulate tumor progression in a variety of pathways.
Background
Lung cancer is the most commonly diagnosed malignant tumor worldwide, whose morbidity and mortality rate rank first among all cancers, and the severity is increasing year by year, posing a great threat to human health (Sung et al., 2021). According to pathological classification, the disease can be categorized as small cell lung adenocarcinoma and non-small cell lung cancer (NSCLC), among which, the latter accounts for about 2/3 cases (Rodríguez-Martínez et al., 2018). NSCLC can be divided into three types, including lung adenocarcinoma, squamous cell lung cancer, and non-small cell lung cancer, of which lung adenocarcinoma accounts for about 40% of cases (Bender, 2014). Epidemiological data reveal that the 5-years overall survival rate of lung cancer in all stages is as low as 15.9% (Ettinger et al., 2013). Therefore, it is of great importance to find biomarkers closely associated with the prognostic outcomes of lung cancer, especially lung adenocarcinoma, as well as to evaluate the prognosis outcome of patients with squamous cell lung cancer through these markers, which can improve the prognosis and formulate individualized diagnosis and treatment (Santarpia et al., 2020).
Ferroptosis, a relatively novel kind of cell death discovered recently, is involved in the pathophysiological process of many diseases including tumors (Wu et al., 2019), and it is different from apoptosis, necrosis, and autophagy due to a feature: being iron-dependent. It is caused by the accumulation of toxic lipid reactive oxygen species and the consumption of polyunsaturated fatty acids (Li et al., 2020). Polyunsaturated fatty acid is an important substrate in ferroptosis, and the C–H bond in the diallyl group of polyunsaturated fatty acid is easily attacked by oxidation. Compared with normal cells, cancer cells have the phenomenon of iron ion aggregation, and the regulation of ferroptosis from the perspective of iron homeostasis can effectively kill tumor cells (Lei et al., 2021). In recent years, for the treatment of advanced tumors, especially drug-resistant tumors, inducing the death of cancer cells through ferroptosis has become a very promising option (Xu et al., 2021). In addition to various induction molecules, many genes can also be markers of ferroptosis (Chen et al., 2021a). At present, ferroptosis-related genes have shown good predictive performance in not a few tumors, including glioma (Zhuo et al., 2020), liver cancer (Tang et al., 2020), pancreatic cancer (Jiang et al., 2021a), gastroenteric tumor (Angius et al., 2019), urologic neoplasms (Liu et al., 2021), and thyroid cancer (Ge et al., 2021). However, the relationship between FRGs and prognosis and the outcome of patients with lung adenocarcinoma has not been reported in depth.
Therefore, our study aims to explore the role of FRGs to predict outcome, and on the basis of which, establish a prognostic model to assess the prognostic outcome of patients with lung adenocarcinoma. Based on the differentially expressed genes (DEGs) related to ferroptosis, a prognostic model was constructed according to the training set, and the predictive power of the model was verified in the validation set. Finally, we proceeded with a functional enrichment analysis to investigate the biological mechanism of FRGs in lung adenocarcinoma.
In this study, a prognostic model consisting of seven genes associated with ferroptosis was established with excellent predictive power. Enrichment analysis showed that these genes were associated with the development of lung adenocarcinoma.
Methods
Resources and Pre-processing
The gene expression data and related clinical information of lung tumors were extracted from the The Cancer Genome Atlas (TCGA) database (https://genome.nih.gov/). Ferroptosis-related gene sets were extracted from three subgroups in the ferroptosis database (http://www.zhounan.org/ferrdb/). Edge R package from R was used to normalize the entire data set, set |log2FC| > 0.5 and false discovery rate (FDR) < 0.05 as the threshold to construct a volcano map, to further obtain differentially expressed ferroptosis-related genes.
Construction of the Prognostic Model
According to downloaded clinical data of lung adenocarcinoma cases from TCGA, patients with an adequate follow-up time (>30 days) were screened and divided into a training set and an internal validation set at a ratio of 2:1. The sets were divided to construct a prognostic model and verify the model, respectively. In addition, two Gene Expression Omnibus (GEO) (https://www.ncbi.nlm.nih.gov/geo/) data sets are applied for external validation of the model. Based on the training set, we performed univariate Cox regression analysis on FRGs and survival data and set p < 0.05 to identify differential FRGs related to prognosis, and LASSO regression was performed to further screen the genes. After obtaining seven target genes, a multi-factor stepwise Cox regression was performed to analyze their respective coefficients (βi). Finally, a risk-scoring formula consisting of βi and gene expression level (Expi) was constructed as follow:
According to the model, the risk score of invidual could be acquired. In addition, the median of the risk score was set as a critical value, and all patients included were divided into high- and low-risk groups. To reveal the prognostic outcome difference between the groups Kaplan–Meier survival curve was used. Then, the above results were verified by the validation set. To further assess the ability to predict, we conducted a subgroup analysis to compare the OS between the two groups.
Construction of the Nomogram
We incorporated clinical features including risk score, clinical and TNM stage, age, and gender into the final model to establish a novel nomogram to predict the OS of patients individually. In addition, AUC was obtained through a receiver operating characteristic (ROC) curve to assess the accuracy of the nomogram. Subsequently, we used the calibration chart and decision curve analysis to verify the predictive ability of the model (Ge et al., 2021). The validation set was used to verify the results obtained finally.
Gene Set Enrichment Analysis
The reference set (2. cp.kegg.v6.2. symbols.gmt, c5. all. v6.2. symbols.gmt) was downloaded from the Molecular Signatures Database (http://www.gsea-msigdb.org/gsea/msigdb/index.jsp), and the number of random combinations was set as 1,000 according to the default weighted enrichment method (NPM p < 0.05, FDR p < 0.05). The study conducted gene ontology (GO) and Kyoto Encyclopedia of Genes and Genomics (KEGG) analysis on the DEGs in the groups and deduced their functions by analyzing gene sets. Therefore, this way it can be used to clarify the question on whether the gene set shows a statistically significant difference between the two biological states. The study explored whether the DEGs between the two groups are enriched during the disease progression as well.
Statistical Analysis
All statistical analyses were conducted using R version 4.1.0 (package: limma, pheatmap, survival, glmnet, survminer, survivalROC, rms, and timeROC). Univariate and multivariate Cox regression were used to analyze the correlation between clinical features, risk scores, and the OS of patients. The ROC curve, C-index, the calibration curve, and DCA curve were used to assess the predictive power of the model. Two-tailed p < 0.05 was considered statistically significant.
Results
Extraction of Ferroptosis-Related Genes in Lung Adenocarcinoma
The gene expression result with matching clinical data of lung adenocarcinoma (497 tumor tissues and 54 paracancerous tissues) were extracted from the TCGA database, and the ferroptosis-related gene set (259 genes) was obtained from the ferroptosis database. Expression matrices of all ferroptosis-related genes were extracted from the TCGA dataset and differential expression analysis was performed (Supplementary Table S1). Seventy-two differential ferroptosis-related genes in lung adenocarcinoma tissues and adjacent tissues were screened out, of which 49 were upregulated, and 23 were downregulated (Figure 1 and Supplementary Table S2).
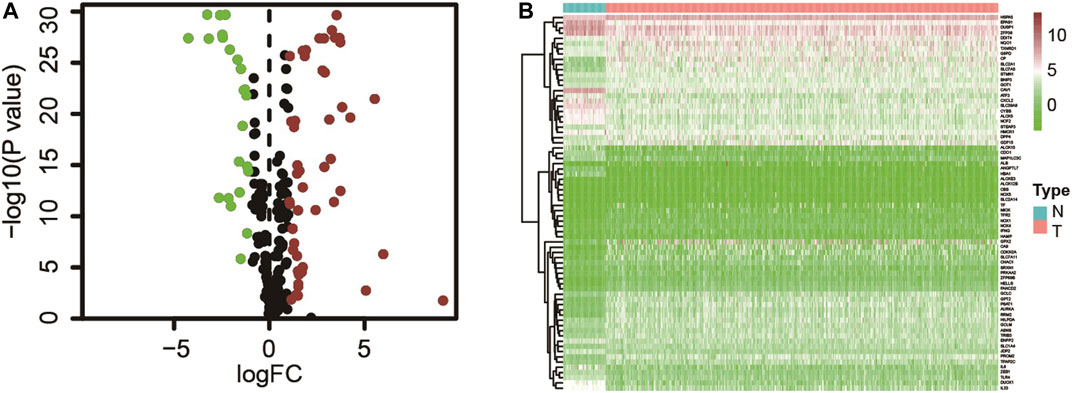
FIGURE 1. (A) Ferroptosis-related genes differentially expressed in tumor and adjacent tissues; (B) Expression of ferroptosis-related genes in tumors and adjacent tissues.
Construction of the Prognostic Model
The patients who met an adequate follow-up time (>30 days) were divided into training and validation sets at a ratio of 2 to 1. In the training set, we performed univariate Cox regression on FRGs and corresponding clinical survival data, and initially screened 11 FRGs related to prognosis (Figure 2A). Seven target genes were further screened by LASSO regression analysis, namely TXNRD1, TRIB3, SLC2A1, CDKN2A, RRM2, SLC7A11, and G6PD (Figures 2B,C). The obtained target genes were adapted to calculate the risk score of the individual through the Cox proportional hazard regression model (Supplementary Table S3). The median of the risk score was set as a cut-off value, on which basis we divided patients into two groups (Figure 3). The Kaplan–Meier curve showed that the OS of the high-risk group was worse than the other group (Figure 3A). The risk curves and scatter plots can reveal the risk score and survival status of each patient. As shown in Figures 3B,C, the mortality and risk coefficient of the high-risk group were significantly higher than that of the low-risk group. Figure 3D displays the expression profile of these seven genes. The ROC curve analysis of the 3- and 5-years OS yielded AUCs of 0.731 and 0.709, respectively (Figure 3E). Similar results were observed using the same process in the internal validation set (Figures 3F–J) and external validation (GSE37745 and GSE68465) (Supplementary Figure S1). Subgroup analysis showed that according to age, gender, TNM stage, or clinical stage, the prognosis of patients in the low-risk group are more favorable (Figure 4).
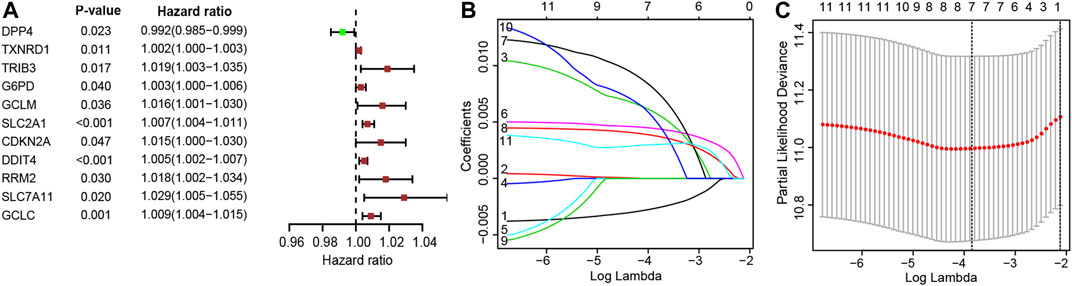
FIGURE 2. (A) 11 Ferroptosis-related genes significantly associated with overall survival (OS) in lung adenocarcinoma; (B) Adjustment parameters in LASSO regression model; (C) LASSO coefficient spectrum of ferroptosis-related genes.
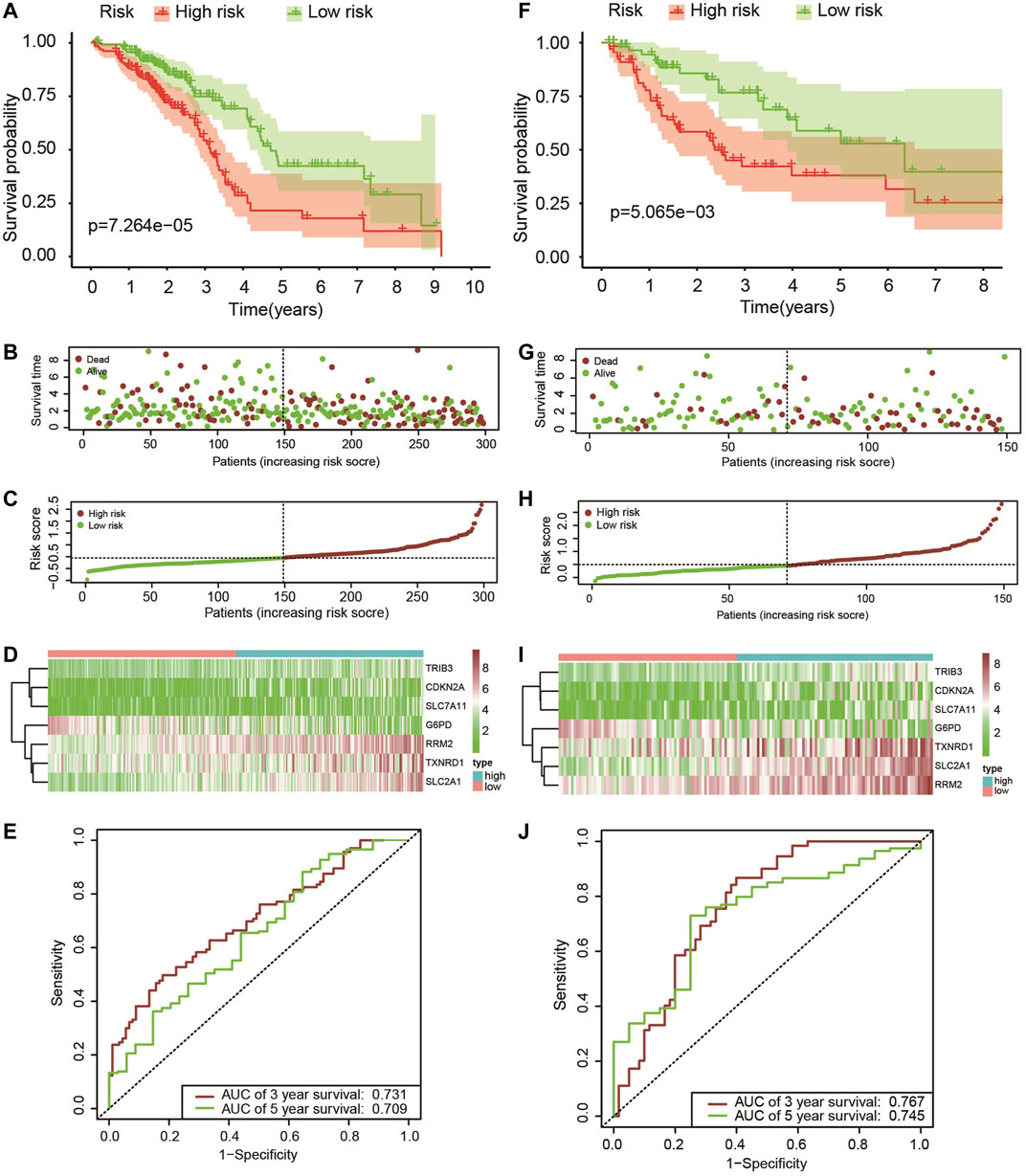
FIGURE 3. (A–F) Kaplan–Meier survival analysis of patients with lung adenocarcinoma in high- and low-risk groups; (B,G) The overall survival rate and status of patients with lung adenocarcinoma; (C,H) The distribution of risk scores; (D,I) The expression level of these seven ferroptosis-related genes in the low-risk group and the high-risk group, the cool color represents low expression, while the warm color represents high expression; (E,J) 3-years and 5-years ROC curve analysis of the prognostic model.
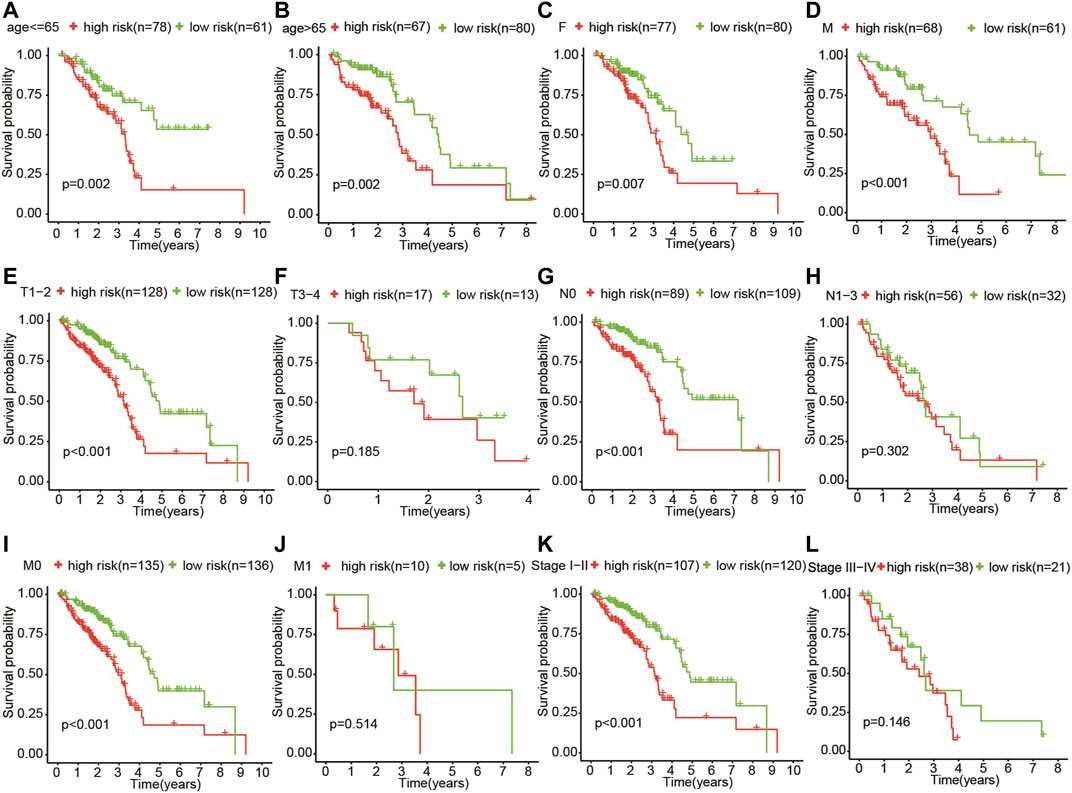
FIGURE 4. Subgroup analysis showed that according to age (A,B), gender (C,D), TNM stage (E–K), and clinical stage (L,M), the OS of patients in the high-risk group was more unfavorable than that in the other group.
Construction of the Nomogram
We then developed a nomogram, including age, gender, clinical stage, TNM stage, and risk score, as shown in Figure 5A. The ROC curve analysis of the 3- and 5-years OS of the prognostic model yielded AUCs of 0.823 and 0.852, respectively (Figure 5B). The established calibration chart and decision curve analysis show that the nomogram has a favorable predictive effect (Figures 5D–G). In the validation set, the 3- and 5-years AUCs obtained by analyzing the ROC curve of the novel prediction model were 0.821 and 0.800, respectively, as shown in Figure 5C.
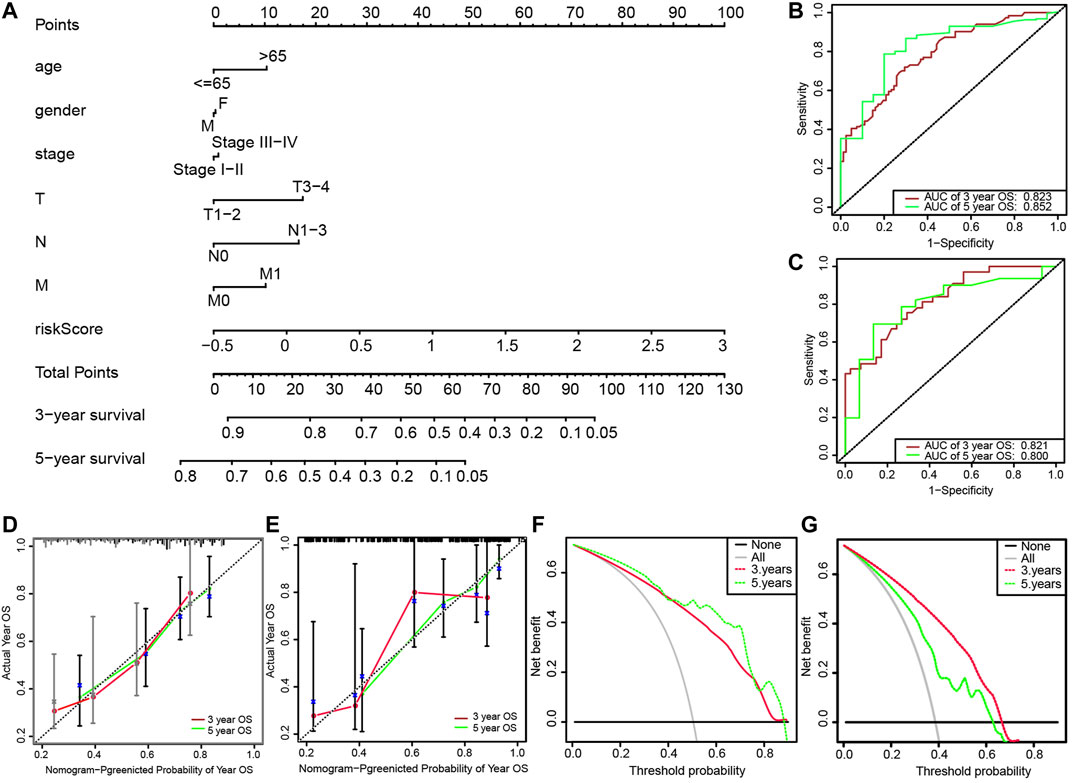
FIGURE 5. (A) Nomogram for predicting 3-years and 5-years survival rates of patients with lung adenocarcinoma; (B) ROC curve analysis for predicting 3-years and 5-years OS through nomogram in the training set; (C) ROC curve analysis for predicting 3-years and 5-years OS through the nomogram in the validation set; (D) Calibration of 3-years survival rate based on the nomogram; (E) Calibration of 5-years survival rate based on the nomogram; (F) Decision curve analysis of 3-years survival rate based on the nomogram; (G) Decision curve analysis of 5-years survival rate based on the nomogram.
Gene Set Enrichment Analysis
The GO and KEGG enrichment analysis were performed on the DEGs of the above-mentioned high-and low-risk groups (Figure 6). The result of the analysis disclosed that the gene set was enriched in DNA replication, cell cycle regulation, cell adhesion, and chromosome mutation. As shown by the KEGG pathway enrichment analysis, screened genes were deeply involved in the cell cycle, oxidative phosphorylation, P53 signaling pathway, proteasomes, and so on. These results may provide a direction for researchers to study the mechanism of ferroptosis-related genes on lung adenocarcinoma in the future.
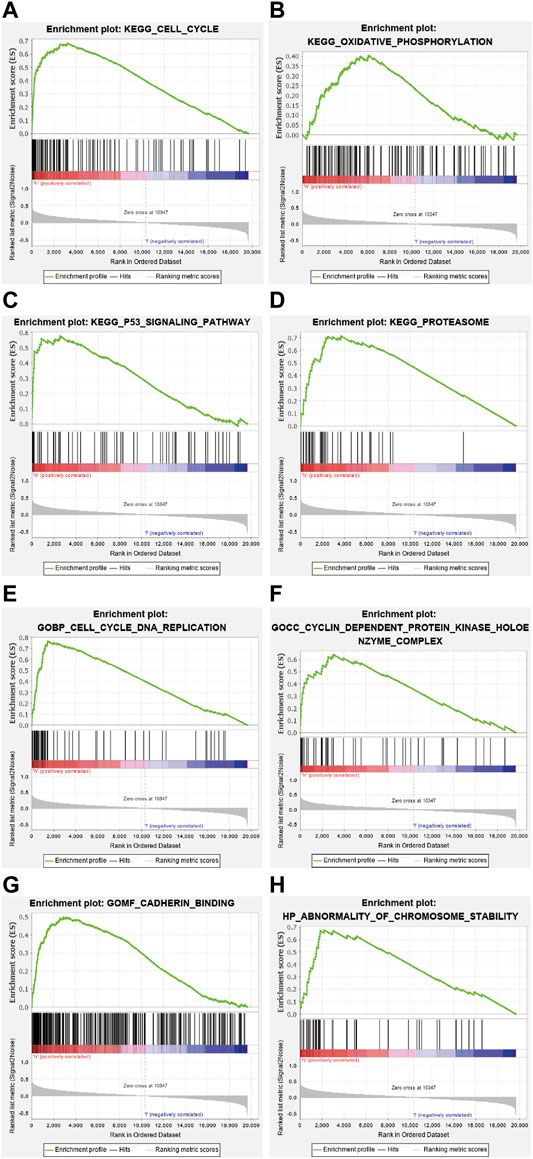
FIGURE 6. Kyoto Encyclopedia of Genes and Genomics (KEGG) enrichment analysis revealed that genes identified were involved in the following processes (A) cell cycle, (B) oxidative phosphorylation, (C) P53 signaling pathway, and (D) proteasome. Gene Ontology (GO) enrichment analysis demonstrated that the gene set was enriched in (E) DNA replication, (F) cell cycle regulation, (G) cell adhesion, and (H) chromosome variation.
Discussion
Lung adenocarcinoma is a common malignant tumor with a poor prognosis (Devarakonda et al., 2015). Predicting the outcome of tumors is of incontestable clinical significance in the diagnosis and treatment of patients with lung adenocarcinoma (Chen et al., 2021b). Previous studies have shown that chest CT, serum tumor markers, and TNM staging can be used as prognostic indicators of lung cancer (Calvayrac et al., 2017). However, there are certain limitations. In case of a risk of radiation exposure, the sensitivity and specificity are relatively low (Hoseok and Cho, 2015; Welch, 2017). Therefore, it is important to find predictors that can accurately predict the prognosis of patients with lung cancer.
According to new studies, ferroptosis has shown non-negligible potential in cancer treatment, especially for tumors that are not sensitive to traditional therapies (Liang et al., 2019; Proneth and Conrad, 2019). P53 is a widely studied gene that can suppress tumors, and inhibit the expression of cystine/glutamate antiporter at the transcriptional level to regulate the process of ferroptosis (Xie et al., 2017). In addition, studies have shown that the increase in iron-dependent reactive oxygen species can cause lipid peroxidation outside the mitochondria triggering ferroptosis, thereby inhibiting tumor development (Liang et al., 2019). In lung cancer, due to the upregulation of cystine/glutamate antiporter and the decrease in iron, ferroptosis is usually inhibited, which leads to the relapse and development of tumors (Lai et al., 2019). Therefore, our study aims to explore the relationshipbetween FRGs and the prognosis of patients with lung adenocarcinoma with the described underlying mechanism.
We obtained gene expression data with clinical data of lung adenocarcinoma from the public database. FRGs were extracted from the ferroptosis database. First, we identified seven target genes through DEGs and regression analysis. Multivariate Cox analysis was adapted to calculate regression coefficients and a prognostic model was developed, thereby dividing patients with lung adenocarcinoma into high- and low-risk groups. We observed that patients in the latter group lived longer OS than the other. Furthermore, we developed a nomogram according to the outcomes of multivariate Cox regression. ROC curve, calibration chart, and decision curve confirmed the prediction power of the nomogram. Compared with previous studies, the AUC value of the prognostic model based on ferroptosis-related genes (AUC = 0.823) was higher than that of the prognostic model based on metabolic genes (AUC = 0.767) (Yu et al., 2020), immune genes (AUC = 0.718) (Song et al., 2020), and autophagy genes (AUC = 0.810) (Wang et al., 2020).
A risk scoring model consisting of seven genes (TXNRD1, TRIB3, SLC2A1, CDKN2A, RRM2, SLC7A11, and G6PD) associated with ferroptosis was constructed. Thioredoxin reductase (TXNRD1) is overexpressed in lung cancer cells to maintain tumor survival, and this overexpression has been shown to be associated with clinical outcomes (Zhu et al., 2019). Studies have shown that TRIB3 is significantly upregulated in LUAD cell lines and tissues. TRIB3 gene knockdown significantly inhibited the growth and invasion of LUAD cells (Xing et al., 2020). The progression of lung adenocarcinoma can be inhibited by inhibiting SLC2A1 expression (Wang et al., 2017). CDKN2A is associated with DNA methylation and is closely related to the prognosis of patients (Tsou et al., 2007). Inhibition of RRM2 can activate STING signaling pathway and inhibit the enhancement of radiosensitivity of lung adenocarcinoma (Jiang et al., 2021b). Inhibition of SLC7A11 leads to poor prognosis in KRAS-mutated lung adenocarcinoma (Hu et al., 2020). Previous studies have shown that G6PD is an independent prognostic factor for lung adenocarcinoma (Nagashio et al., 2019).
Subsequently, we conducted a gene function enrichment analysis to reveal the mechanism of ferroptosis genes on lung adenocarcinoma. The results demonstrated that FRGs we screened were involved in DNA replication, cell cycle regulation, cell adhesion, chromosomal mutation, oxidative phosphorylation, P53 signaling pathway, and proteasome processes. Hence, FRGs can be used as predictors of lung adenocarcinoma prognosis and may play a crucial role in lung adenocarcinoma biology.
In conclusion, the study found seven ferroptosis-related genes by searching the database with prognostic value for patients with lung adenocarcinoma. We constructed a clinical prognostic model of FRGs, which possesses a good effect on predicting the survival rate of patients with lung adenocarcinoma, indicating that FRGs can very well predict the prognosis outcome of patients with lung adenocarcinoma, and play a crucial role in the relapse and development of lung adenocarcinoma.
Data Availability Statement
The original contributions presented in the study are included in the article/Supplementary Material, further inquiries can be directed to the corresponding author.
Author Contributions
ML conceptualized the study. ML and MC provided the sudy design. SvS and StS acquired the data and performed the statistical analyses. YZ and ML performed the data analysis and interpretation. ML and MC prepared the manuscript. SvS edited the manuscript. StS reviewed the manuscript.
Funding
The funding for this study was provided by the High-level Hospital Construction Project of Maoming People's Hospital, the Research Project of Maoming Science and Technology Bureau (Grant No. 2021121), and the Outstanding Young Talents Program of Maoming people's hospital. The funders had no role in the design of the study and collection, analysis, and interpretation of the data, and in writing the manuscript.
Conflict of Interest
The authors declare that the research was conducted in the absence of any commercial or financial relationships that could be construed as a potential conflict of interest.
Publisher’s Note
All claims expressed in this article are solely those of the authors and do not necessarily represent those of their affiliated organizations, or those of the publisher, the editors and the reviewers. Any product that may be evaluated in this article, or claim that may be made by its manufacturer, is not guaranteed or endorsed by the publisher.
Acknowledgments
The authors thank Mrs. Yunru Fan and Dr. Alexandra Lam for providing instructive advice and useful suggestions on the manuscript.
Supplementary Material
The Supplementary Material for this article can be found online at: https://www.frontiersin.org/articles/10.3389/fgene.2021.739520/full#supplementary-material
Abbreviations
AUCs, areas under the curves; DEGs, differentially expressed genes; FDR, false discovery rate; FRGs, ferroptosis-related genes; GEO, Gene Expression Omnibus; GO, Gene ontology; KEGG, Kyoto Encyclopedia of Genes and Genomics; NSCLC, non-small cell lung cancer; OS, overall survival; ROC, receiver operating characteristic; TCGA, The Cancer Genome Atlas Program
References
Angius, A., Uva, P., Pira, G., Muroni, M. R., Sotgiu, G., Saderi, L., et al. (2019). Integrated Analysis of miRNA and mRNA Endorses a Twenty miRNAs Signature for Colorectal Carcinoma. Int. J. Mol. Sci. 20 (16), 4067. doi:10.3390/ijms20164067
Bender, E. (2014). Epidemiology: The Dominant Malignancy. Nature 513 (7517), S2–S3. doi:10.1038/513S2a
Calvayrac, O., Pradines, A., Pons, E., Mazières, J., and Guibert, N. (2017). Molecular Biomarkers for Lung Adenocarcinoma. Eur. Respir. J. 49 (4), 1601734. doi:10.1183/13993003.01734-2016
Chen, X., Comish, P. B., Tang, D., and Kang, R. (2021). Characteristics and Biomarkers of Ferroptosis. Front. Cel Dev. Biol. 9, 637162. doi:10.3389/fcell.2021.637162
Chen, Y., Zitello, E., Guo, R., and Deng, Y. (2021). The Function of LncRNAs and Their Role in the Prediction, Diagnosis, and Prognosis of Lung Cancer. Clin. Transl. Med. 11 (4), e367. doi:10.1002/ctm2.367
Devarakonda, S., Morgensztern, D., and Govindan, R. (2015). Genomic Alterations in Lung Adenocarcinoma. Lancet Oncol. 16 (7), e342–e351. doi:10.1016/S1470-2045(15)00077-7
Ettinger, D. S., Akerley, W., Borghaei, H., Chang, A. C., Cheney, R. T., Chirieac, L. R., et al. (2013). Non-small Cell Lung Cancer, Version 2.2013. J. Natl. Compr. Canc Netw. 11 (6), 645–653. doi:10.6004/jnccn.2013.0084
Ge, M., Niu, J., Hu, P., Tong, A., Dai, Y., Xu, F., et al. (2021). A Ferroptosis-Related Signature Robustly Predicts Clinical Outcomes and Associates with Immune Microenvironment for Thyroid Cancer. Front. Med. 8, 637743. doi:10.3389/fmed.2021.637743
Hoseok, I., and Cho, J. Y. (2015). Lung Cancer Biomarkers. Adv. Clin. Chem. 72, 107–170. doi:10.1016/bs.acc.2015.07.003
Hu, K., Li, K., Lv, J., Feng, J., Chen, J., Wu, H., et al. (2020). Suppression of the SLC7A11/glutathione axis Causes Synthetic Lethality in KRAS-Mutant Lung Adenocarcinoma. J. Clin. Invest. 130 (4), 1752–1766. doi:10.1172/JCI124049
Jiang, P., Yang, F., Zou, C., Bao, T., Wu, M., Yang, D., et al. (2021). The Construction and Analysis of a Ferroptosis-Related Gene Prognostic Signature for Pancreatic Cancer. Aging 13 (7), 10396–10414. doi:10.18632/aging.202801
Jiang, X., Li, Y., Zhang, N., Gao, Y., Han, L., Li, S., et al. (2021). RRM2 Silencing Suppresses Malignant Phenotype and Enhances Radiosensitivity via Activating cGAS/STING Signaling Pathway in Lung Adenocarcinoma. Cell Biosci. 11 (1), 74. doi:10.1186/s13578-021-00586-5
Lai, Y., Zhang, Z., Li, J., Li, W., Huang, Z., Zhang, C., et al. (2019). STYK1/NOK Correlates with Ferroptosis in Non-small Cell Lung Carcinoma. Biochem. Biophys. Res. Commun. 519 (4), 659–666. doi:10.1016/j.bbrc.2019.09.032
Lei, P., Ayton, S., and Bush, A. I. (2021). The Essential Elements of Alzheimer's Disease. J. Biol. Chem. 296, 100105. doi:10.1074/jbc.REV120.008207
Li, J., Cao, F., Yin, H.-l., Huang, Z.-j., Lin, Z.-t., Mao, N., et al. (2020). Ferroptosis: Past, Present and Future. Cell Death Dis. 11 (2), 88. doi:10.1038/s41419-020-2298-2
Liang, C., Zhang, X., Yang, M., and Dong, X. (2019). Recent Progress in Ferroptosis Inducers for Cancer Therapy. Adv. Mater. 31 (51), 1904197. doi:10.1002/adma.201904197
Liu, J., Ma, H., Meng, L., Liu, X., Lv, Z., Zhang, Y., et al. (2021). Construction and External Validation of a Ferroptosis-Related Gene Signature of Predictive Value for the Overall Survival in Bladder Cancer. Front. Mol. Biosci. 8, 675651. doi:10.3389/fmolb.2021.675651
Nagashio, R., Oikawa, S., Yanagita, K., Hagiuda, D., Kuchitsu, Y., Igawa, S., et al. (2019). Prognostic Significance of G6PD Expression and Localization in Lung Adenocarcinoma. Biochim. Biophys. Acta Proteins Proteom. 1867 (1), 38–46. doi:10.1016/j.bbapap.2018.05.005
Proneth, B., and Conrad, M. (2019). Ferroptosis and Necroinflammation, a yet Poorly Explored Link. Cell Death Differ. 26 (1), 14–24. doi:10.1038/s41418-018-0173-9
Rodríguez-Martínez, Á., Torres-Durán, M., Barros-Dios, J. M., and Ruano-Ravina, A. (2018). Residential Radon and Small Cell Lung Cancer. A Systematic Review. Cancer Lett. 426, 57–62. doi:10.1016/j.canlet.2018.04.003
Santarpia, M., Aguilar, A., Chaib, I., Cardona, A. F., Fancelli, S., Laguia, F., et al. (2020). Non-Small-Cell Lung Cancer Signaling Pathways, Metabolism, and PD-1/PD-L1 Antibodies. Cancers 12 (6), 1475. doi:10.3390/cancers12061475
Song, C., Guo, Z., Yu, D., Wang, Y., Wang, Q., Dong, Z., et al. (2020). A Prognostic Nomogram Combining Immune-Related Gene Signature and Clinical Factors Predicts Survival in Patients with Lung Adenocarcinoma. Front. Oncol. 10, 1300. doi:10.3389/fonc.2020.01300
Sung, H., Ferlay, J., Siegel, R. L., Laversanne, M., Soerjomataram, I., Jemal, A., et al. (2021). Global Cancer Statistics 2020: GLOBOCAN Estimates of Incidence and Mortality Worldwide for 36 Cancers in 185 Countries. CA A. Cancer J. Clin. 71, 209–249. doi:10.3322/caac.21660
Tang, B., Zhu, J., Li, J., Fan, K., Gao, Y., Cheng, S., et al. (2020). The Ferroptosis and Iron-Metabolism Signature Robustly Predicts Clinical Diagnosis, Prognosis and Immune Microenvironment for Hepatocellular Carcinoma. Cell Commun. Signal 18 (1), 174. doi:10.1186/s12964-020-00663-1
Tsou, J. A., Galler, J. S., Siegmund, K. D., Laird, P. W., Turla, S., Cozen, W., et al. (2007). Identification of a Panel of Sensitive and Specific DNA Methylation Markers for Lung Adenocarcinoma. Mol. Cancer 6, 70. doi:10.1186/1476-4598-6-70
Wang, X., Yao, S., Xiao, Z., Gong, J., Liu, Z., Han, B., et al. (2020). Development and Validation of a Survival Model for Lung Adenocarcinoma Based on Autophagy-Associated Genes. J. Transl. Med. 18 (1), 149. doi:10.1186/s12967-020-02321-z
Wang, Y., Shi, S., Ding, Y., Wang, Z., Liu, S., Yang, J., et al. (2017). Metabolic Reprogramming Induced by Inhibition of SLC2A1 Suppresses Tumor Progression in Lung Adenocarcinoma. Int. J. Clin. Exp. Pathol. 10 (11), 10759–10769.
Welch, H. G. (2017). Cancer Screening, Overdiagnosis, and Regulatory Capture. JAMA Intern. Med. 177 (7), 915–916. doi:10.1001/jamainternmed.2017.1198
Wu, Y., Song, J., Wang, Y., Wang, X., Culmsee, C., and Zhu, C. (2019). The Potential Role of Ferroptosis in Neonatal Brain Injury. Front. Neurosci. 13, 115. doi:10.3389/fnins.2019.00115
Xie, Y., Zhu, S., Song, X., Sun, X., Fan, Y., Liu, J., et al. (2017). The Tumor Suppressor P53 Limits Ferroptosis by Blocking DPP4 Activity. Cel Rep. 20 (7), 1692–1704. doi:10.1016/j.celrep.2017.07.055
Xing, Y., Luo, P., Hu, R., Wang, D., Zhou, G., and Jiang, J. (2020). TRIB3 Promotes Lung Adenocarcinoma Progression via an Enhanced Warburg Effect. Cancer Manag. Res. 12, 13195–13206. doi:10.2147/CMAR.S287956
Xu, G., Wang, H., Li, X., Huang, R., and Luo, L. (2021). Recent Progress on Targeting Ferroptosis for Cancer Therapy. Biochem. Pharmacol. 190, 114584. doi:10.1016/j.bcp.2021.114584
Yu, X., Zhang, X., and Zhang, Y. (2020). Identification of a 5-Gene Metabolic Signature for Predicting Prognosis Based on an Integrated Analysis of Tumor Microenvironment in Lung Adenocarcinoma. J. Oncol. 2020, 1–12. doi:10.1155/2020/5310793
Zhu, B., Ren, C., Du, K., Zhu, H., Ai, Y., Kang, F., et al. (2019). Olean-28,13b-olide 2 Plays a Role in Cisplatin-Mediated Apoptosis and Reverses Cisplatin Resistance in Human Lung Cancer through Multiple Signaling Pathways. Biochem. Pharmacol. 170, 113642. doi:10.1016/j.bcp.2019.113642
Keywords: lung adenocarcinoma, ferroptosis, gene, prognosis, prognostic
Citation: Liang M, Chen M, Zhong Y, Singh S and Singh S (2021) Construction of a Prognostic Model in Lung Adenocarcinoma Based on Ferroptosis-Related Genes. Front. Genet. 12:739520. doi: 10.3389/fgene.2021.739520
Received: 11 July 2021; Accepted: 30 August 2021;
Published: 22 September 2021.
Edited by:
Jiangning Song, Monash University, AustraliaReviewed by:
Chen Li, Monash University, AustraliaYong-Zi Chen, Tianjin Medical University Cancer Institute and Hospital, China
Copyright © 2021 Liang, Chen, Zhong, Singh and Singh. This is an open-access article distributed under the terms of the Creative Commons Attribution License (CC BY). The use, distribution or reproduction in other forums is permitted, provided the original author(s) and the copyright owner(s) are credited and that the original publication in this journal is cited, in accordance with accepted academic practice. No use, distribution or reproduction is permitted which does not comply with these terms.
*Correspondence: Min Liang, aW10dWFza2FAMTYzLmNvbQ==
†ORCID:Min Liangorcid.org/0000-0002-6313-2022