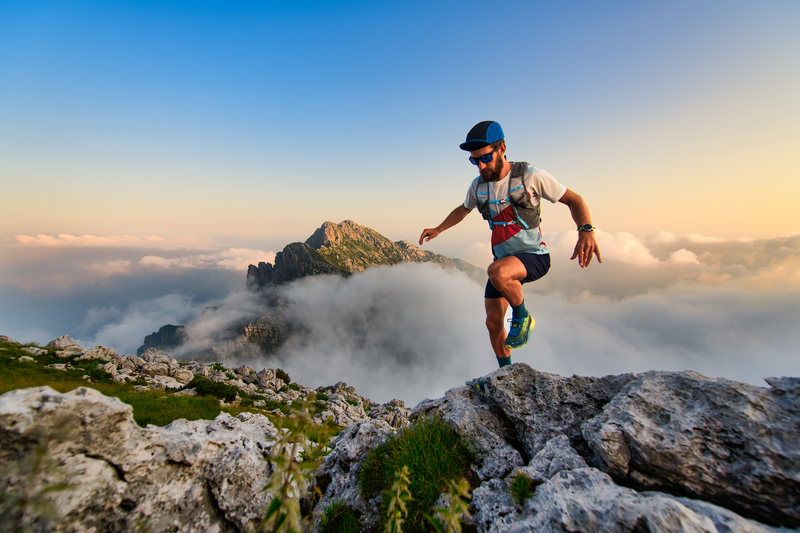
94% of researchers rate our articles as excellent or good
Learn more about the work of our research integrity team to safeguard the quality of each article we publish.
Find out more
ORIGINAL RESEARCH article
Front. Genet. , 23 August 2021
Sec. Computational Genomics
Volume 12 - 2021 | https://doi.org/10.3389/fgene.2021.739470
Background: Gastric cancer is one of the most serious gastrointestinal malignancies with bad prognosis. Ferroptosis is an iron-dependent form of programmed cell death, which may affect the prognosis of gastric cancer patients. Long non-coding RNAs (lncRNAs) can affect the prognosis of cancer through regulating the ferroptosis process, which could be potential overall survival (OS) prediction factors for gastric cancer.
Methods: Ferroptosis-related lncRNA expression profiles and the clinicopathological and OS information were collected from The Cancer Genome Atlas (TCGA) and the FerrDb database. The differentially expressed ferroptosis-related lncRNAs were screened with the DESeq2 method. Through co-expression analysis and functional annotation, we then identified the associations between ferroptosis-related lncRNAs and the OS rates for gastric cancer patients. Using Cox regression analysis with the least absolute shrinkage and selection operator (LASSO) algorithm, we constructed a prognostic model based on 17 ferroptosis-related lncRNAs. We also evaluated the prognostic power of this model using Kaplan–Meier (K-M) survival curve analysis, receiver operating characteristic (ROC) curve analysis, and decision curve analysis (DCA).
Results: A ferroptosis-related “lncRNA–mRNA” co-expression network was constructed. Functional annotation revealed that the FOXO and HIF-1 signaling pathways were dysregulated, which might control the prognosis of gastric cancer patients. Then, a ferroptosis-related gastric cancer prognostic signature model including 17 lncRNAs was constructed. Based on the RiskScore calculated using this model, the patients were divided into a High-Risk group and a low-risk group. The K-M survival curve analysis revealed that the higher the RiskScore, the worse is the obtained prognosis. The ROC curve analysis showed that the area under the ROC curve (AUC) of our model is 0.751, which was better than those of other published models. The multivariate Cox regression analysis results showed that the lncRNA signature is an independent risk factor for the OS rates. Finally, using nomogram and DCA, we also observed a preferable clinical practicality potential for prognosis prediction of gastric cancer patients.
Conclusion: Our prognostic signature model based on 17 ferroptosis-related lncRNAs may improve the overall survival prediction in gastric cancer.
Gastric cancer is one of the most serious gastrointestinal malignant tumors worldwide, which is the third most common cause of cancer-related death. Resulting from unhealthy lifestyle habits, Helicobacter pylori infection, and an increasing social burden, 1,089,103 new cases occurred in 2020, while deaths reached 768,793 cases (Sung et al., 2021). Gastric cancer has contributed to a massive burden for both sexes in China (Feng et al., 2019; Cao et al., 2021). Despite surgical and adjuvant therapy technologies having improved rapidly, the overall survival (OS) rates for gastric cancer patients still remain very poor. Especially for patients suffering advanced gastric cancer, the 5-year survival rate is lower than 20% (Ferlay et al., 2019; Tan, 2019). Nowadays, the TNM classification of malignant tumors is still the globally recognized standard for classifying the extent of spread of gastric cancer (Brierley et al., 2016). However, the responses to treatment and individual differences may affect the prognostic evaluation of patients with the same TNM classification status due to indefinite genetic features. Therefore, novel prognostic markers are urgently needed for gastric cancer.
Ferroptosis is an iron-dependent form of programmed cell death (PCD) (Dixon et al., 2012; Tang et al., 2019) that may regulate cell death by mainly relying on the accumulation of reactive oxygen species (ROS), resulting in the dysregulation of glutathione peroxidase activities (Li et al., 2020). Recent studies have shown that aberrant iron metabolism is an important risk factor that may affect the initiation and progression of cancer, which would control the prognosis of patients (Xie et al., 2016; Roemhild et al., 2021). Long non-coding RNAs (lncRNAs) are endogenic functional RNAs that can regulate the activities of cancer-related metabolic pathways (Ransohoff et al., 2018). lncRNAs have been confirmed to be able to regulate the ferroptosis of cancer cells as epigenetic and metabolic regulators (Mao et al., 2018; Wang et al., 2019; Wu et al., 2020). What is more is that their higher molecular stability makes lncRNAs a better form of biomarker (Statello et al., 2021).
In this study, we collected the transcriptome and clinicopathological and OS information for gastric cancer from The Cancer Genome Atlas (TCGA) database. We also collected 259 ferroptosis-related genes from FerrDb. Using the DESeq2 method, we identified the differentially expressed (DE) lncRNAs and ferroptosis-related genes. Then, we constructed the ferroptosis-related “lncRNA–mRNA” co-expression network. Functional annotation analysis has shown that these lncRNAs would control the metal ion and ROS response, which may affect the OS rates of gastric cancer patients. Based on these ferroptosis-related DE-lncRNAs, we then constructed a model for gastric cancer prognostic evaluation based on 17 ferroptosis-related lncRNAs. The area under the receiver operating characteristic (ROC) curve (AUC) value for this model is 0.751, which is better than those of other published models. The nomogram and the decision curve analysis (DCA) results showed a preferable clinical practicality potential for this model. Therefore, the prognostic evaluation model constructed in our study may improve prognosis prediction for gastric cancer patients.
From TCGA database1 (Hutter and Zenklusen, 2018), we collected the HTSeq-Count data, clinicopathological data, and OS information for stomach adenocarcinoma (STAD). In total, we have downloaded the transcriptome data of 434 tissues (387 STAD tissues and 47 normal tissues from 387 patient samples) and collected the clinicopathological features including age, gender, TNM stage, clinical stage, and grade stage. Then, we also download TCGA gene annotation information version 22 from the GENCODE database2 (Frankish et al., 2019). The 259 ferroptosis-related genes were collected from the FerrDb database3 (Zhou and Bao, 2020). In addition, we downloaded the annotated gene sets for gene set enrichment analysis (GSEA) from MSigDB4 (Subramanian et al., 2005).
Based on R (ver. 4.0.3), we used the “DESeq2” package to screen the DE lncRNAs and genes, with the threshold values | log2(FoldChange)| > 1 and P < 0.05. The read count data were used for the DESeq2 workflow, and the data normalization processes were integrated into the DESeq2 workflow. Subsequently, through Pearson’s correlation analysis, 1,062 ferroptosis-related DE-lncRNAs were identified, with the threshold values | cor| ≥ 0.4 and P < 0.05.
Based on Pearson’s correlation, we visualized the ferroptosis-related lncRNA--mRNA co-expression network using Cytoscape (ver. 3.8.2). Next, protein--protein interactions (PPIs) were identified using the STRING dataset5 (Szklarczyk et al., 2019).
Based on R (ver. 4.0.3), we used the “clusterProfiler” package to perform Gene Ontology (GO) annotation and Kyoto Encyclopedia of Genes and Genomes (KEGG) pathway enrichment analysis, with default parameters. Afterward, we performed GSEA using the gene set “msigdb.v7.4.entrez.gmt” download from MSigDB.
Based on R (ver. 4.0.3), we used the “survival” and “glmnet” packages to perform Cox regression with the least absolute shrinkage and selection operator (LASSO) algorithm and multivariate Cox regression analysis. We used the identified 1,062 DE ferroptosis-related lncRNAs to construct the gastric cancer prognostic model. Then, utilizing the prognostic model, we calculated the RiskScore for each patient samples with the formula: RiskScore = [where CorlncRNAi represents the correlation coefficient of interfering lncRNAi (lncRNAi), ExplncRNAi represents the expression level for lncRNAi, and n represents the number of lncRNA signatures]. Based on the median RiskScore, the patients were divided into a High-Risk group (over the median RiskScore) and a Low-Risk group (no more than the median RiskScore).
Based on R (ver. 4.0.3), we used the “rms” package to construct the nomogram in order to predict the 1-, 3-, and 5-year OS rates of gastric cancer patients. We also constructed the calibration curve and evaluated the consistency between the OS rates predicted by the nomogram and the actually observed OS rates. Using the “rmda” package, we performed the DCA to compare the Net-Benefits with the different predictors.
We used the “survival” and “survminer” packages with R (ver. 4.0.3) to perform the Kaplan–Meier (K-M) survival curve analysis on the OS rates. Then, the “survivalROC” package was also used for ROC analysis. The AUC value was calculated for the evaluation of the prediction accuracy of the ferroptosis-related lncRNA-based prognostic model.
Figure 1 shows the workflow for this study. To identify the ferroptosis-related DE-lncRNAs among gastric cancer tissues, we firstly collected the transcriptome data of 434 tissues (387 STAD tissues and 47 normal tissues from 387 patient samples) from TCGA database. Based on the integrality of the information on OS, a total of 370 patient samples were included for subsequent analysis. Afterward, the patients were divided randomly into a training group (containing 270 patient samples) and a testing group (containing 100 patient samples). From the FerrDb database (Zhou and Bao, 2020), 259 ferroptosis-related genes were collected simultaneously (Supplementary Table 1).
Figure 1. Flowchart for this work. DE, differentially expressed; GO, Gene Oncology; KEGG, Kyoto Encyclopedia of Genes and Genomes; GSEA, gene set enrichment analysis; ROC curve, receiver operating characteristic curve.
Using the DESeq2 method, we have detected 4,466 DE genes (2,125 upregulated and 2,341 downregulated) (Supplementary Table 2) and 3,391 DE-lncRNAs (2,394 upregulated and 997 downregulated) (Supplementary Table 3). Next, we identified 59 ferroptosis-related genes that were aberrantly expressed (21 upregulated and 38 downregulated) (Supplementary Table 4). Through Pearson’s correlation analysis, we identified 1,062 ferroptosis-related DE-lncRNAs among the gastric cancer tissues (Supplementary Table 5).
To analyze the effects of the ferroptosis-related lncRNAs on gastric cancer prognosis, we constructed a ferroptosis-related lncRNA–mRNA co-expression network (Figure 2A, detailed in Supplementary Table 5). Among which, we also screened the PPIs (Figure 2B, detailed in Supplementary Table 6). Subsequently, KEGG and GO annotations were used to predict the potential biological functions for the ferroptosis-related lncRNAs. We identified the KEGG terms “hsa04068 FOXO signaling pathway” and “hsa04066 HIF-1 signaling pathway” as significantly enriched (Figure 2C, detailed in Supplementary Table 7). These results might indicate that, through regulating glucose metabolism, oxidative stress resistance, or cell cycle, ferroptosis-related lncRNAs would regulate the stress resistance abilities of gastric cancer cells, which would affect the OS rates indirectly. The GO annotation results also supported this inference. We identified that the GO biological process terms “GO:0006979 response to oxidative stress,” “GO:0042594 response to starvation,” “GO:0010038 response to metal ion,” and “GO:0062197 cellular response to chemical stress” were most significantly enriched (Figure 2D, detailed in Supplementary Table 8). Furthermore, GSEA was used to identify the ferroptosis-related lncRNA involved pathway enrichment features among gastric cancer tissues (Figure 2E). We identified the “cell cycle” pathway to be activated (Figure 2F). All the above results, together, indicated that the ferroptosis-related lncRNAs would control the prognostic status of gastric cancer patients, which would be potential features of gastric cancer prognosis prediction.
Figure 2. (A) Ferroptosis-related lncRNA–mRNA co-expression network. Blue circles are differentially expressed (DE) ferroptosis-related genes. Yellow diamonds are co-expressed lncRNAs. (B) Protein–protein interaction (PPI) network for the DE ferroptosis-related genes involved in the co-expression network. (C) Kyoto Encyclopedia of Genes and Genomes (KEGG) enrichment analysis for the targets of ferroptosis-related lncRNAs. (D) Gene Ontology (GO) annotation analysis for the targets of ferroptosis-related lncRNAs. (E,F) Gene set enrichment analysis.
Based on the expression levels of the 1,062 DE ferroptosis-related lncRNAs and the OS information, we have built a gastric cancer prognostic model. Using univariate Cox regression analysis with the LASSO algorithm (Supplementary Figure 1) and multivariate Cox regression, we have constructed a prognostic model for gastric cancer patients based on 17 ferroptosis-related lncRNAs (Table 1). Among the 17 lncRNAs, 11 (namely, ENSG00000249835.2, ENSG0000023671 9.2, ENSG00000250241.4, ENSG00000240661.1, ENSG0000026 2061.4, ENSG00000229656.5, ENSG00000175746.6, ENSG00000 248599.1, ENSG00000254333.1, ENSG00000247134.5, and ENSG00000248362.1) were identified as prognostic risk factors, while the other six (namely, ENSG00000234449.2, ENSG0000023 9513.4, ENSG00000265334.1, ENSG00000267201.1, ENSG 00000273293.1, and ENSG00000230107.1) were identified as prognostic protective factors (Figure 3).
Table 1. Cox regression analysis with the LASSO algorithm for the prognostic model based on 17 ferroptosis-related lncRNAs.
Figure 3. Forest plot of the prognostic values of the prognostic model based on 17 ferroptosis-related lncRNAs. **P < 0.01; *P < 0.05.
Using the prognostic model based on 17 ferroptosis-related lncRNAs, we calculated the RiskScore for each patient sample. Then, the patients were divided into a High-Risk group (RiskScore higher than the median RiskScore) and a Low-Risk group (RiskScore no more than the median RiskScore). From the RiskScore distribution dot plot, we found that more dead cases were observed in the High-Risk group (Figures 4A,B); the expressions of the 17 ferroptosis-related lncRNA signatures are exhibited as a heatmap in Figure 4C. Concurrently, the prognostic effectiveness of this model for gastric cancer patients’ OS status was evaluated using K-M survival curve analysis. We identified that the OS rates of the patients in the High-Risk group were significantly lower (P < 0.0001; Figure 4D). We also evaluated the prognostic accuracy of this model using ROC curve analysis. The results showed that the AUC value in the training group is 0.751 (Figure 4E). Additionally, we have verified the prognostic power and accuracy of the 17 ferroptosis-related lncRNA signatures between the test group and the combined group (containing all samples). The K-M survival analysis verified that the OS in the High-Risk group was significantly lower (P < 0.0001; Figure 4D). The AUC value in the test group is 0.747, while that in the combined group is 0.750 (Figure 4E). All these results, together, show that the 17 ferroptosis-related lncRNA signatures would be a valuable gastric cancer prognostic model.
Figure 4. Prognostic risk scores calculated using the ferroptosis-related lncRNA-based prognostic model. (A) Distribuion of patients’ risk scores. (B) Patients’ overall survival (OS) status distribution. (C) Prognostic signature signal heatmaps. (D) Kaplan–Meier (K-M) survival curves for patients separated into the High-Risk and Low-Risk groups. (E) Receiver operating characteristic (ROC) curve analysis for verification of the prognostic value of the prognostic model based on 17 ferroptosis-related lncRNAs.
Next, to analyze whether the prognostic model based on 17 ferroptosis-related lncRNAs is an independent risk factor for gastric cancer prognosis, we performed Cox regression analysis. The results of the univariate COX regression in Figure 5A showed that the model (P < 0.001), N stage (P < 0.001), pathological stage (P < 0.001), age (P = 0.009), and T stage (P = 0.040) were meaningful for prognosis prediction. Concurrently, multivariate Cox regression showed that the model [P < 0.001, hazard ratio (HR) = 1.297, 95% CI = 1.224–1.374] and age (P = 0.001, HR = 1.033, 95% CI = 1.013–1.053) would be independent risk factors for the prognosis of gastric cancer (Figure 5B). In addition, the correlations between the prognostic model based on 17 ferroptosis-related lncRNAs and the clinicopathological features were exhibited as a heatmap (Figure 5C). We also performed the K-M survival analysis among each subset group that was separated according to the clinicopathological features. The results were shown as subset groups separated by age, gender, T stage, N stage, M stage, clinical stage, and grade (shown in Figures 6A–G, respectively). We observed that the OS rates in the High-Risk groups associated with age, gender, T stage, N stage, clinical stage, and grade were significantly lower.
Figure 5. Cox regression analysis for the clinical phenotypes of gastric cancer patients. (A) Forest plot for the overall survival (OS) prognostic values through univariate Cox regression analysis. (B) Forest plot for the OS prognostic values through multivariate Cox regression analysis. (C) Relationship heatmap of the risk scores and clinical phenotypes of patients.
Figure 6. Subset group Kaplan–Meier (K-M) survival curves of patients. (A) Age <65 years (left) and ≥65 years (right). (B) Males (left) and females (right). (C) T1+T2 (left) and T3+T4 (right). (D) N0+N1 (left) and N2+N3 (right). (E) M0 (left) and M1 (right). (F) Stage I+II (left) and stage III+IV (right). (G) G1+G2 (left) and G3+G4 (right).
To evaluate the potential clinical practicality of the prognostic model based on 17 ferroptosis-related lncRNAs, we built a nomogram with the RiskScore and the clinicopathological features to predict the 1-, 3-, and 5-year OS rates of gastric cancer patients. As shown in Figure 7A, we observed that the higher the calculated RiskScore, the worse is the predicted prognosis. Then, we constructed the calibration curve to evaluate the consistency between the OS rates predicted by the nomogram and the actually observed OS rates. The results showed relatively good fits for the 1-, 3-, and 5-year OS prediction (Figures 7B–D, respectively). Finally, the DCA results (Figure 7E) demonstrated that this nomogram (the 17 ferroptosis-related lncRNA signature model) showed better clinical practicality for prognosis prediction of gastric cancer patients.
Figure 7. Nomogram of overall survival (OS) prediction of patients with gastric cancer. (A) Nomogram of both the prognostic model based on 17 ferroptosis-related lncRNAs and the clinical pathological factors. (B–D) Calibration curve analysis of the 1-year (B), 3-year (C), and 5-year (D) survival prediction accuracy of the nomogram. (E) Decision curve analysis (DCA) of the clinical practicality evaluation of the nomogram.
Ferroptosis is an iron-dependent cell death regulatory method that is distinct from apoptosis, necroptosis, and autophagy (Dixon et al., 2012). Ferroptosis is driven by the iron-dependent peroxidation of oxidizable membrane phospholipids (Doll et al., 2017). Recent studies have shown that the drug sorafenib, used to treat advanced hepatocellular carcinoma, exerts its anticancer activity mainly by regulating the ferroptosis process (Capelletti et al., 2020). TP53 could inhibit the ferroptosis process in human colorectal cancer cells by promoting the localization of DPP4 in the non-enzymatically active nucleus, and thus may promote the growth of human colorectal cancer cells (Xie et al., 2017). Apatinib would induce ferroptosis in gastric cancer cells through lipid peroxidation and then exerts its anticancer effect (Zhao et al., 2021). In this study, we have identified the DE ferroptosis-related lncRNAs among gastric cancer tissues. Through GO annotation analysis and KEGG pathway enrichment analysis, we found that the FOXO signaling pathway, the HIF-1 signaling pathway, and the cell cycle pathway were significantly enriched. FOXOs are essential transcription factors that play important roles in tumorigenesis because they have been shown to be dysregulated in many types of human cancers. Some studies have pointed out that the roles of FOXOs in breast cancer, colon cancer, and other cancers are contradictory. FOXO can induce cell death (Coomans, de Brachene and Demoulin, 2016). Besides, FOXO can enhance the proliferation, survival, and invasion abilities of cancer cells, which may promote the development of cancer (Storz et al., 2009; Sisci et al., 2013). Therefore, the FOXO signaling pathway may play an important role in a variety of cellular and physiological activities, such as cell proliferation, regulation of PCD, metabolism, and the disruption of the cell cycle (Ma et al., 2018). There are also some studies showing that the FOXO signaling pathway can be regulated to delay the progression of prostate and liver cancer (Shan et al., 2019; Li et al., 2021). The HIF-1 signaling pathway is involved in tumor growth, proliferation, and migration (Tian et al., 2015; Macklin et al., 2017). The HIF-1α signaling pathway mediates the promotion of CThrC1 in the migration and invasion of gastric cancer cells (Ding et al., 2020). The cell cycle is also important for the occurrence and development of many cancers, and it is an important mechanism of cell growth, development, metabolism, and regeneration (Evan and Vousden, 2001; Icard et al., 2019), which is closely related to the degree of tumor differentiation, invasion area, vascular invasion, and lymphatic metastasis (Machlowska et al., 2018).
Based on these DE ferroptosis-related lncRNAs, we have constructed a prognostic model for gastric cancer. The AUC values for the training and test groups reached 0.751 and 0.747, respectively, in our study. In previous studies, other evaluation models have also been constructed to evaluate the prognosis of gastric cancer patients. In the study of Jiang et al., a prognostic model for gastric cancer was constructed based on ferroptosis-related genes. In their study, the AUC values were 0.654 at 1 year, 0.657 at 3 years, and 0.733 at 5 years (Jiang et al., 2021), which were lower than those of our model. The work of Chen et al. (2021) has built a prognostic signature model based on 10 hypoxia-related lncRNAs; the AUCs of this model were 0.703 and 0.734 in the test group and the combined group, respectively (Chen et al., 2020). Using autophagy-related genes, the work of Chen et al. (2021) has built a prognostic model based on five genes; the AUC for this model was 0.736 (Chen et al., 2021). In addition, some other prognostic models were constructed in recent years; however, the AUC values for these models were lower than 0.7 (Liu et al., 2018; Hu et al., 2019; Yu et al., 2019; Qi et al., 2020). This result indicates that the prognostic model based on 17 ferroptosis-related lncRNAs constructed in our study might improve the prognostic power for gastric cancer. Our study still has some limitations. We constructed a ferroptosis-related lncRNA gastric cancer prognostic model just through statistical analysis. More clinical validation would need to be performed in further studies to improve our prognosis prediction model. Also, in further studies, we will analyze the regulatory functions of the 17 ferroptosis-related lncRNA signatures. All in all, the prognostic power of our model was better than that of other published models. Our study might improve the prognosis prediction for gastric cancer patients.
The datasets presented in this study can be found in online repositories. The names of the repository/repositories and accession number(s) can be found in the article/Supplementary Material.
ZX provided the idea. JP and XZ performed the data statistics. ZX and XZ prepared the manuscript. XF and ZX reviewed and modified the manuscript. All authors have reviewed the final version of the manuscript.
This work was supported by the Science and Technology Research Project of the Education Department of Jilin Province (JJKH20211149KJ) and the Bethune Project of Jilin University (2020B09).
The authors declare that the research was conducted in the absence of any commercial or financial relationships that could be construed as a potential conflict of interest.
All claims expressed in this article are solely those of the authors and do not necessarily represent those of their affiliated organizations, or those of the publisher, the editors and the reviewers. Any product that may be evaluated in this article, or claim that may be made by its manufacturer, is not guaranteed or endorsed by the publisher.
We thank Guoqing Wang for helping us to improve the manuscript.
The Supplementary Material for this article can be found online at: https://www.frontiersin.org/articles/10.3389/fgene.2021.739470/full#supplementary-material
Supplementary Figure 1 | (A) Forest plot for the prognostic values of the ferroptosis related lncRNA signatures through uni-variate COX regression analysis. (B–C) And LASSO regression was performed, calculating the coefficients (B) and minimum criteria (C).
Supplementary Figure 2 | GO annotation analysis for screened 17 ferroptosis related lncRNA signatures.
Supplementary Table 1 | Ferroptosis related download from FerrDb database.
Supplementary Table 2 | Differentially expressed genes among gastric cancer tissues.
Supplementary Table 3 | Differentially expressed lncRNAs among gastric cancer tissues.
Supplementary Table 4 | Differentially expressed ferroptosis related genes.
Supplementary Table 5 | Intermolecular co-expression relationship details for the ferroptosis related lncRNA co-expression network.
Supplementary Table 6 | PPI relationship details.
Supplementary Table 7 | The KEGG enrichment analysis details.
Supplementary Table 8 | GO annotation analysis details.
Brierley, J. D., Gospodarowicz, M. K., and Wittekind, C. (2016). TNM Classification of Malignant Tumours. New York, NY: Wiley.
Cao, W., Chen, H. D., Yu, Y. W., Li, N., and Chen, W. Q. (2021). Changing profiles of cancer burden worldwide and in China: a secondary analysis of the global cancer statistics 2020. Chin. Med. J. 134, 783–791. doi: 10.1097/CM9.0000000000001474
Capelletti, M. M., Manceau, H., Puy, H., and Peoc’h, K. (2020). Ferroptosis in liver diseases: an overview. Int. J. Mol. Sci. 21:4908. doi: 10.3390/ijms21144908
Chen, L., Ma, G., Wang, P., Dong, Y., Liu, Y., Zhao, Z., et al. (2021). Establishment and verification of prognostic model for gastric cancer based on autophagy-related genes. Am. J. Cancer Res. 11, 1335–1346.
Chen, Q., Hu, L., and Chen, K. (2020). Construction of a nomogram based on a hypoxia-related lncRNA signature to improve the prediction of gastric cancer prognosis. Front. Genet. 11:570325. doi: 10.3389/fgene.2020.570325
Coomans, de Brachene, A., and Demoulin, J. B. (2016). FOXO transcription factors in cancer development and therapy. Cell Mol. Life Sci. 73, 1159–1172. doi: 10.1007/s00018-015-2112-y
Ding, X., Huang, R., Zhong, Y., Cui, N., Wang, Y., Weng, J., et al. (2020). CTHRC1 promotes gastric cancer metastasis via HIF-1alpha/CXCR4 signaling pathway. Biomed. Pharmacother. 123, 109742. doi: 10.1016/j.biopha.2019.109742
Dixon, S. J., Lemberg, K. M., Lamprecht, M. R., Skouta, R., Zaitsev, E. M., Gleason, C. E., et al. (2012). Ferroptosis: an iron-dependent form of nonapoptotic cell death. Cell 149, 1060–1072. doi: 10.1016/j.cell.2012.03.042
Doll, S., Proneth, B., Tyurina, Y. Y., Panzilius, E., Kobayashi, S., Ingold, I., et al. (2017). ACSL4 dictates ferroptosis sensitivity by shaping cellular lipid composition. Nat. Chem. Biol. 13, 91–98. doi: 10.1038/nchembio.2239
Evan, G. I., and Vousden, K. H. (2001). Proliferation, cell cycle and apoptosis in cancer. Nature 411, 342–348. doi: 10.1038/35077213
Feng, R. M., Zong, Y. N., Cao, S. M., and Xu, R. H. (2019). Current cancer situation in China: good or bad news from the 2018 Global Cancer Statistics? Cancer Commun. 39:22. doi: 10.1186/s40880-019-0368-6
Ferlay, J., Colombet, M., Soerjomataram, I., Mathers, C., Parkin, D. M., Pineros, M., et al. (2019). Estimating the global cancer incidence and mortality in 2018: GLOBOCAN sources and methods. Int. J. Cancer 144, 1941–1953. doi: 10.1002/ijc.31937
Frankish, A., Diekhans, M., Ferreira, A. M., Johnson, R., Jungreis, I., Loveland, J., et al. (2019). GENCODE reference annotation for the human and mouse genomes. Nucleic Acids Res. 47, D766–D773. doi: 10.1093/nar/gky955
Hu, B. L., Xie, M. Z., Li, K. Z., Li, J. L., Gui, Y. C., and Xu, J. W. (2019). Genome-wide analysis to identify a novel distant metastasis-related gene signature predicting survival in patients with gastric cancer. Biomed. Pharmacother. 117:109159. doi: 10.1016/j.biopha.2019.109159
Hutter, C., and Zenklusen, J. C. (2018). The cancer genome atlas: creating lasting value beyond its data. Cell 173, 283–285. doi: 10.1016/j.cell.2018.03.042
Icard, P., Fournel, L., Wu, Z., Alifano, M., and Lincet, H. (2019). Interconnection between metabolism and cell cycle in cancer. Trends Biochem. Sci. 44, 490–501. doi: 10.1016/j.tibs.2018.12.007
Jiang, X., Yan, Q., Xie, L., Xu, S., Jiang, K., Huang, J., et al. (2021). Construction and validation of a ferroptosis related prognostic model for gastric cancer. J. Oncol. 2021:6635526. doi: 10.1155/2021/6635526
Li, J., Cao, F., Yin, H. L., Huang, Z. J., Lin, Z. T., Mao, N., et al. (2020). Ferroptosis: past, present and future. Cell Death Dis. 11:88. doi: 10.1038/s41419-020-2298-2
Li, Y., Li, P., and Wang, N. (2021). Effect of let-7c on the PI3K/Akt/FoxO signaling pathway in hepatocellular carcinoma. Oncol. Lett. 21:96. doi: 10.3892/ol.2020.12357
Liu, X., Wu, J., Zhang, D., Bing, Z., Tian, J., Ni, M., et al. (2018). Identification of potential key genes associated with the pathogenesis and prognosis of gastric cancer based on integrated bioinformatics analysis. Front. Genet. 9:265. doi: 10.3389/fgene.2018.00265
Ma, J., Matkar, S., He, X., and Hua, X. (2018). FOXO family in regulating cancer and metabolism. Semin. Cancer Biol. 50, 32–41. doi: 10.1016/j.semcancer.2018.01.018
Machlowska, J., Maciejewski, R., and Sitarz, R. (2018). The pattern of signatures in gastric cancer prognosis. Int. J. Mol. Sci. 19:1658. doi: 10.3390/ijms19061658
Macklin, P. S., McAuliffe, J., Pugh, C. W., and Yamamoto, A. (2017). Hypoxia and HIF pathway in cancer and the placenta. Placenta 56, 8–13. doi: 10.1016/j.placenta.2017.03.010
Mao, C., Wang, X., Liu, Y., Wang, M., Yan, B., Jiang, Y., et al. (2018). A G3BP1-interacting lncRNA promotes ferroptosis and apoptosis in cancer via nuclear sequestration of p53. Cancer Res. 78, 3484–3496. doi: 10.1158/0008-5472.CAN-17-3454
Qi, M., Yu, B., Yu, H., and Li, F. (2020). Integrated analysis of a ceRNA network reveals potential prognostic lncRNAs in gastric cancer. Cancer Med. 9, 1798–1817. doi: 10.1002/cam4.2760
Ransohoff, J. D., Wei, Y., and Khavari, P. A. (2018). The functions and unique features of long intergenic non-coding RNA. Nat. Rev. Mol. Cell Biol. 19, 143–157. doi: 10.1038/nrm.2017.104
Roemhild, K., von Maltzahn, F., Weiskirchen, R., Knuchel, R., von Stillfried, S., and Lammers, T. (2021). Iron metabolism: pathophysiology and pharmacology. Trends Pharmacol. Sci. 42, 640–656. doi: 10.1016/j.tips.2021.05.001
Shan, Z., Li, Y., Yu, S., Wu, J., Zhang, C., Ma, Y., et al. (2019). CTCF regulates the FoxO signaling pathway to affect the progression of prostate cancer. J. Cell. Mol. Med. 23, 3130–3139. doi: 10.1111/jcmm.14138
Sisci, D., Maris, P., Cesario, M. G., Anselmo, W., Coroniti, R., Trombino, G. E., et al. (2013). The estrogen receptor alpha is the key regulator of the bifunctional role of FoxO3a transcription factor in breast cancer motility and invasiveness. Cell Cycle 12, 3405–3420. doi: 10.4161/cc.26421
Statello, L., Guo, C. J., Chen, L. L., and Huarte, M. (2021). Gene regulation by long non-coding RNAs and its biological functions. Nat. Rev. Mol. Cell Biol. 22, 96–118. doi: 10.1038/s41580-020-00315-9
Storz, P., Doppler, H., Copland, J. A., Simpson, K. J., and Toker, A. (2009). FOXO3a promotes tumor cell invasion through the induction of matrix metalloproteinases. Mol. Cell Biol. 29, 4906–4917. doi: 10.1128/MCB.00077-09
Subramanian, A., Tamayo, P., Mootha, V. K., Mukherjee, S., Ebert, B. L., Gillette, M. A., et al. (2005). Gene set enrichment analysis: a knowledge-based approach for interpreting genome-wide expression profiles. Proc. Natl. Acad. Sci. U.S.A. 102, 15545–15550. doi: 10.1073/pnas.0506580102
Sung, H., Ferlay, J., Siegel, R. L., Laversanne, M., Soerjomataram, I., Jemal, A., et al. (2021). Global cancer statistics 2020: GLOBOCAN estimates of incidence and mortality worldwide for 36 cancers in 185 countries. CA Cancer J. Clin. 71, 209–249. doi: 10.3322/caac.21660
Szklarczyk, D., Gable, A. L., Lyon, D., Junge, A., Wyder, S., Huerta-Cepas, J., et al. (2019). STRING v11: protein-protein association networks with increased coverage, supporting functional discovery in genome-wide experimental datasets. Nucleic Acids Res. 47, D607–D613. doi: 10.1093/nar/gky1131
Tan, Z. (2019). Recent advances in the surgical treatment of advanced gastric cancer: a review. Med. Sci. Monit. 25, 3537–3541. doi: 10.12659/MSM.916475
Tang, D., Kang, R., Berghe, T. V., Vandenabeele, P., and Kroemer, G. (2019). The molecular machinery of regulated cell death. Cell Res. 29, 347–364. doi: 10.1038/s41422-019-0164-5
Tian, Q., Xue, Y., Zheng, W., Sun, R., Ji, W., Wang, X., et al. (2015). Overexpression of hypoxia-inducible factor 1alpha induces migration and invasion through Notch signaling. Int. J. Oncol. 47, 728–738. doi: 10.3892/ijo.2015.3056
Wang, M., Mao, C., Ouyang, L., Liu, Y., Lai, W., Liu, N., et al. (2019). Long noncoding RNA LINC00336 inhibits ferroptosis in lung cancer by functioning as a competing endogenous RNA. Cell Death Differ. 26, 2329–2343. doi: 10.1038/s41418-019-0304-y
Wu, Y., Zhang, S., Gong, X., Tam, S., Xiao, D., Liu, S., et al. (2020). The epigenetic regulators and metabolic changes in ferroptosis-associated cancer progression. Mol. Cancer 19:39. doi: 10.1186/s12943-020-01157-x
Xie, Y., Hou, W., Song, X., Yu, Y., Huang, J., Sun, X., et al. (2016). Ferroptosis: process and function. Cell Death Differ. 23, 369–379. doi: 10.1038/cdd.2015.158
Xie, Y., Zhu, S., Song, X., Sun, X., Fan, Y., Liu, J., et al. (2017). The tumor suppressor p53 limits ferroptosis by blocking DPP4 activity. Cell Rep. 20, 1692–1704. doi: 10.1016/j.celrep.2017.07.055
Yu, C., Hao, X., Zhang, S., Hu, W., Li, J., Sun, J., et al. (2019). Characterization of the prognostic values of the NDRG family in gastric cancer. Therap. Adv. Gastroenterol. 12:1756284819858507. doi: 10.1177/1756284819858507
Zhao, L., Peng, Y., He, S., Li, R., Wang, Z., Huang, J., et al. (2021). Apatinib induced ferroptosis by lipid peroxidation in gastric cancer. Gastric Cancer 24, 642–654. doi: 10.1007/s10120-021-01159-8
Keywords: ferroptosis, lncRNAs, prognostic model, gastric cancer, bioinformatics and computational biology
Citation: Pan J, Zhang X, Fang X and Xin Z (2021) Construction on of a Ferroptosis-Related lncRNA-Based Model to Improve the Prognostic Evaluation of Gastric Cancer Patients Based on Bioinformatics. Front. Genet. 12:739470. doi: 10.3389/fgene.2021.739470
Received: 11 July 2021; Accepted: 29 July 2021;
Published: 23 August 2021.
Edited by:
Quan Zou, University of Electronic Science and Technology of China, ChinaCopyright © 2021 Pan, Zhang, Fang and Xin. This is an open-access article distributed under the terms of the Creative Commons Attribution License (CC BY). The use, distribution or reproduction in other forums is permitted, provided the original author(s) and the copyright owner(s) are credited and that the original publication in this journal is cited, in accordance with accepted academic practice. No use, distribution or reproduction is permitted which does not comply with these terms.
*Correspondence: Zhuoyuan Xin, eGluenlAamx1LmVkdS5jbg==
†These authors have contributed equally to this work
Disclaimer: All claims expressed in this article are solely those of the authors and do not necessarily represent those of their affiliated organizations, or those of the publisher, the editors and the reviewers. Any product that may be evaluated in this article or claim that may be made by its manufacturer is not guaranteed or endorsed by the publisher.
Research integrity at Frontiers
Learn more about the work of our research integrity team to safeguard the quality of each article we publish.