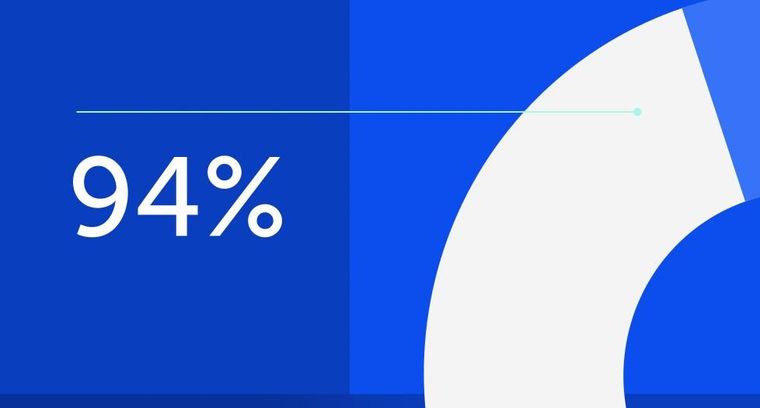
94% of researchers rate our articles as excellent or good
Learn more about the work of our research integrity team to safeguard the quality of each article we publish.
Find out more
ORIGINAL RESEARCH article
Front. Genet., 25 November 2021
Sec. Statistical Genetics and Methodology
Volume 12 - 2021 | https://doi.org/10.3389/fgene.2021.724785
This article is part of the Research TopicData Mining and Statistical Methods for Knowledge Discovery in Diseases Based on Multimodal OmicsView all 15 articles
Ovarian cancer is the second most dangerous gynecologic cancer with a high mortality rate. The classification of gene expression data from high-dimensional and small-sample gene expression data is a challenging task. The discovery of miRNAs, a small non-coding RNA with 18–25 nucleotides in length that regulates gene expression, has revealed the existence of a new array for regulation of genes and has been reported as playing a serious role in cancer. By using LASSO and Elastic Net as embedded algorithms of feature selection techniques, the present study identified 10 miRNAs that were regulated in ovarian serum cancer samples compared to non-cancer samples in public available dataset GSE106817: hsa-miR-5100, hsa-miR-6800-5p, hsa-miR-1233-5p, hsa-miR-4532, hsa-miR-4783-3p, hsa-miR-4787-3p, hsa-miR-1228-5p, hsa-miR-1290, hsa-miR-3184-5p, and hsa-miR-320b. Further, we implemented state-of-the-art machine learning classifiers, such as logistic regression, random forest, artificial neural network, XGBoost, and decision trees to build clinical prediction models. Next, the diagnostic performance of these models with identified miRNAs was evaluated in the internal (GSE106817) and external validation dataset (GSE113486) by ROC analysis. The results showed that first four prediction models consistently yielded an AUC of 100%. Our findings provide significant evidence that the serum miRNA profile represents a promising diagnostic biomarker for ovarian cancer.
Ovarian cancer is a major clinical challenge in gynecologic oncology. Due to the lack of a proper biomarker-based screening method, most patients are asymptomatic until the disease has metastasized and two-thirds of patients are diagnosed with advanced stages (Lheureux et al., 2019). The International Federation of Gynecology and Obstetrics (FIGO) reported that in the majority of those diagnosed in stage three or four ovarian cancer (2014), more than 70% will have a relapse of their disease within the first 5 years (Reid et al., 2017). Currently, there is an acute need to know potential biomarkers that could lead to the growth of modern and more accurate predictors for ovarian cancer diagnosis and prognosis. As noted, one of the most common gynecologic malignancy is epithelial ovarian cancer (EOC), with each year of about 230,000 new cases and almost 140,000 deaths (Greenlee et al., 2001). In 2020, it is estimated that approximately 21,750 new cases and 13,940 deaths occurred in the United States and 29,000 deaths happened in Europe due to ovarian cancer (Iorio et al., 2007). Therefore, the underlying molecular mechanism has not yet been elucidated. The timely prediction of ovarian cancer would benefit women, healthcare systems, and society as a whole. Accurate and reliable prediction models would enable preventative interventions to reduce the morbidity and mortality associated with ovarian cancer (Harter et al., 2008).
MicroRNAs (miRNA) are important genomic datasets in the human genome that play a regulative impress in cellular processes. miRNAs are a type of non-coding RNA with 18–25 nucleotides in length and reported to play a serious role in human cancers. miRNAs are often copied from DNA sequences to primary miRNAs. Subsequent processes lead to the production of precursor miRNAs and mature miRNAs. The most common mode of action of miRNAs is their interaction with the 3′ untranslated region (3′ UTR) of target mRNAs and increased mRNA degradation and translation suppression. miRNAs can also interact with the five UTR, coding sequence, and promoter regions of their target. In some cases, miRNA interaction with target sequences can induce transcription or regulate transcription. Various parameters modulate miRNA-mRNA interaction, including the subcellular state of miRNAs, the amount of miRNAs and target mRNAs, and the affinity of the interactions (Chen et al., 2015). miRNAs play a role in almost all aspects of cancer biology, such as apoptosis, proliferation, metastasis, and angiogenesis (Lee and Dutta, 2009). In addition, miRNAs have been proposed as potential biomarkers for the recognition of various different cancer types (Lin et al., 2015). Some studies also reported that several miRNAs have a potential value as diagnostic biomarkers of ovarian cancer (Banka and Dara, 2012; Yao et al., 2020).
The down-regulation of miRNAs was found to be related to the progression and the prognoses of cancers. Falzone et al. determined that a group of 16 miRNAs were significantly expressed between bladder cancer patients and normal samples; they serve to modulate the expression of both EMT and NGAL/MMP-9 pathways (Falzone et al., 2016). Falzone et al. identified a series of novel microRNAs and their diagnostic and prognostic significance in oral cancer and their study has therefore developed a molecular detector (Falzone et al., 2019). Another study by Asano et al. reported circulating serum miRNA profile classifier for the detection of sarcoma samples using seven miRNAs (Asano et al., 2019). Table 1 summarizes the results of miRNA associations with ovarian cancer in three recent genetic biomarker studies.
TABLE 1. Summary of miRNA genes shown to be statistically significantly associated with ovarian cancer.
To identify a robust circulating miRNA biomarker, we searched the Gene Expression Omnibus (GEO) database with specific keywords, namely, [“ovarian neoplasms” (MeSH Terms) OR ovarian cancer (All Fields)] AND “Homo sapiens” (porgn) AND [“microRNAs” (MeSH Terms) OR miRNA (All Fields)]. Then, two datasets using the same platform (3D-Gene Human miRNA V21_1.0.0) with larger sample sizes GSE106817 and GSE113486 were included (360 ovarian cancer patients and 2,811 non-cancer controls in total) for our analysis. GSE106817 (320 ovarian cancer patients and 2,759 non-cancer controls) was used as the internal discovery cohort, and GSE113486 (40 ovarian cancer patients and 52 non-cancer controls) was used for independent validation. This study was approved by the Ethics Committee of Tabriz University of Medical Sciences (No: IR. TBZMED.REC.1400.006).
Our analytical process is summarized in Figure 1. To discover biomarkers for ovarian cancer, the free available dataset GSE106817 includes 320 ovarian cancer patients and 2,759 non-cancer controls (11% ovarian cancer and 89% non-cancer). For machine learning analysis purpose, we preprocessed, cleaned, and then normalized by min-max normalization the data (Huang J. et al., 2015).
Feature (variable) selection is the main phase for selecting biomarkers in biological data with high dimension and small sample (p > n). Regularization is a kind of various technique of feature selection methods that use different penalty function to reduce the risk of overfitting and also reduce the complexity of the models (Drotár et al., 2015). Least Absolute Shrinkage and Selection Operation (LASSO) and Elastic Net are the most common embedded feature selection method which are an alternative to the subset selection and dimension reduction techniques. Thus, these algorithms can significantly reduce the variance by performing the variable selection. In the first phase, the expression levels of all 2,568 miRNAs from GSE106817 were analyzed to identify miRNAs as the candidate biomarkers by LASSO and Elastic Net (Zou and Hastie, 2005). For this sake, we used the “glmnet” package in R version 4.0.3. The next subsection gives a brief introduction to the LASSO and Elastic-Net.
LASSO has been proposed by Tibshirani (Hastie et al., 2009) for parameter estimation and variable selection simultaneously in regression analysis. LASSO is a special instance of the penalized least squares regression with L1-penalty function. LASSO estimate of β can be defined as
Where
Elastic Net (ENET) is a convex combination of Ridge and LASSO which shrinks some coefficients to be very small, and on the other hand, similar to the LASSO, ENET set some coefficients to be exactly zero. Elastic Net is an extension of the LASSO that is robust to extreme correlations among the predictors (Zou and Hastie, 2005). When the number of variables exceeds the number of instances (p > n), ENET performs better than LASSO. To trim the instability of the LASSO solution paths, when predictors are highly correlated, the Elastic Net was proposed for analyzing high dimensional data (Liang and Jacobucci, 2020). The Elastic Net uses a mixture of the LASSO and ridge regression penalties and can be formulated as:
The entire path of variable selection by LASSO and ENET algorithms is computed by the path coordinate descent algorithms which is available “glmnet” package in R (Friedman et al., 2010).
Over the last decade, machine learning has been used for successful classification, both for identifying specific classes and for diagnosing cancers (Wang et al., 2005). We use this approach to characterize miRNAs with biomarker potential that could be useful for the diagnosis and/or prognosis of ovarian cancer for potential benefit for public health (screening) and for reduction in economic burden (Deb et al., 2018).
Logistic regression (LR) analyzes the relationship among multiple independent variables and a univariate binary outcome variable (Menard, 2010). One of the main advantage of the logistic regression is its simplicity and interpretability by providing the odds ratio for an outcome (Stoltzfus, 2011). The goodness of fit of a logistic regression model is evaluated using the area under the curve (AUC) (Abdulqader, 2017).
Artificial neural networks (ANN) have been broadly used in medical studies (DeGregory et al., 2018). Such algorithms perform well when there are complex and non-linear associations between variables (Hassanipour et al., 2019). Briefly, artificial neural networks use predictors as inputs and connect them to multiple hidden layer combinations by assigning suitable weights to predict the outcome (Lisboa and Taktak, 2006). The hidden layers and weights must be appropriately selected by the analyst (Sherriff et al., 2004).
Decision trees (DT) (Hassanipour et al., 2019) are a type of supervised machine learning that can be used to find attributes and extract patterns from big databases that are important for predictive modeling (Lisboa and Taktak, 2006). Decision trees are the most direct forward algorithm that processes a visual representation of the relationships between the independents and dependent variables (Hassanipour et al., 2019). However, the variation in the decision trees, in some instances, can be improved by using random forests for the outcomes of randomly generated decision trees to produce a more robust model (Vens et al., 2008).
Among several machine learning algorithms, random forest (RF) has a number of interesting characteristics. Firstly, RF does not overfit when the number of features exceeds the number of instances. Secondly, it does feature selection implicitly. Thirdly, it takes into account the interactions between variables (Okun and Priisalu, 2007). RF is an instance of ensemble learning, in which a complex model is made by combining many simple decision tree models to decrease the variance (Qi, 2012).
XGBoost (XGB) abbreviated for extreme Gradient Boosting package. XGB is a decision-tree-based ensemble of machine learning algorithms that uses a scalable implementation of gradient boosting XGB framework tree boosting (Chen et al., 2015). The most significant component in XGB success is its scalability across all scenarios which is due to a number of major systems and algorithmic enhancements (Chen and Guestrin, 2016).
We started by removing the noise variables with LASSO and ENET. We then implemented SMOTE random oversampling techniques to balance cancer and non-cancer cases in the training data (GSE106817) using the “ROSE” package (Lunardon et al., 2014). We find the optimal prediction models in the training data by using 5-fold cross-validation. We performed ovarian cancer classification using ANN, LR, RF, DT, and XGB (James et al., 2013) algorithms to build our models, after finalizing the optimal hyperparameters for each model. The varImp () function in the caret package was used to determine the miRNAs that are the most important. In this, study we select the most important variables (variable importance >80%) from each of the models. We evaluated our model prediction performances based on several measures of accuracy, including sensitivity, specificity, area under the receiver operating characteristic (AUC), positive predictive value, negative predictive values, and Kappa (Collins et al., 2015). The ROC curves were analyzed by “pROC” in the R software.
Further, two online tools are applied to assess the biological plausibility of the selected miRNAs. To compare the microarray expression profiles of ovarian cancer to the non-cancer group, GEO2R is an interactive web tool that allows users to compare two or more groups of samples in a GEO Series. This procedure will enable the users to identify indicators that are differentially expressed across experimental conditions. To do this end, the limma R package implemented in GEO2R online tool, which generated adjusted p-value, B-statistic (or log-odds), Log2-fold change (logfc), and moderated t-statistic. MiRNet is an online tool for precision miRNA and xeno-miRNA analysis and functional interpretation. This tool contains a large amount of high-quality scientific data that connects miRNAs to their targets and other associated compounds (Fan et al., 2016).
GSE106817 included 2,568 miRNAs. Of those, LASSO and ENET identified 76 and 162 miRNAs, respectively. Then, the dataset was divided with a ratio of 70:30 for the training and testing set, respectively. For the training set, there were 2,156 samples and there were 923 samples in the testing set. The training set had 224 ovarian cancerous and 1,932 non-cancerous samples. After balancing the training data, the samples of non-cancerous decreased to 1,121 and cancerous samples increased to 1,035. Model fitting and tuning parameter selection by 5-fold cross-validation were done on the training data. The dataset with reduced features is classified using LR (statistical), DT and RF (tree-based), ANN and XGB (machine learning) classifier. In this study, the features with higher importance (over 80%) implemented in proposed models are shown in Table 2.
TABLE 2. miRNAs identified with threshold over 80% importance in both Lasso and Elastic net in the dataset GSE106817 with miRNA status.
We identified 10 potential miRNAs hsa-miR-5100, hsa-miR-6800-5p, hsa-miR-1233-5p, hsa-miR-4532, hsa-miR-4783-3p, hsa-miR-4787-3p, hsa-miR-1228-5p, hsa-miR-1290, hsa-miR-3184-5p, and hsa-miR-320b from the GSE106817 datasets and were defined as the candidate miRNAs for ovarian cancer diagnosis. It is clear that hsa-miR-1233-5p, hsa-miR-4783-3p, hsa-miR-5100, and hsa-miR-1290 are features identified by both feature selection methods. hsa-miR-320b and hsa-miR-6800-5p have been identified as important features by LASSO, and hsa-miR-4532, hsa-miR-3184-5p, hsa-miR-4787-3p, and hsa-miR-1228-5p have been recognized by ENET.
The results of GEO2R (generated by the limma) are presented in Table function (Table 2). Note that the column of adjusted p-value is generally recommended as the primary statistic in the interpretation of results. The miRNAs with the smallest p-values will be the most reliable, and column B shows that the represented miRNAs are differentially expressed and logfc presented change between normal and cancerous conditions. As shown in Table 2, all upregulated miRNAs have logfc > 2 and all of miRNAs have adjusted p-value <0.0001. Based on the 10 selected miRNAs, the final machine learning models with optimal hyperparameters are presented in Table 3.
TABLE 3. Predictive power of models for ovarian cancer classification and prediction in the external (GSE113486) validation data.
We showed the expression levels of these 10 identified miRNAs in the internal datasets using a boxplot (Figure 2); among them, seven miRNAs (hsa-miR-320b, hsa-miR-5100, hsa-miR-4783-3p, hsa-miR-1290, hsa-miR-4532, hsa-miR-4787-3p, and hsa-miR-1233-5p) identified the most significantly up-regulated in ovarian cancer samples compared to non-cancer samples. The heatmap using the “pheatmap” package shows differences between samples in each group. In Figure 3 (the heatmap of GSE106817), the miRNAs has-mir-3184-5p, has-mir-6800-5p, and has-mir-1228-5p in the left hand side of the figure show a significantly low expression level in the ovarian cancer group (red color). However, hsa-mir-5100, hsa-mir-1290, hsa-mir-320b, hsa-mir-1233-5p, hsa-mir-4532, hsa-mir-4783-3p, and hsa-mir-4783-3p have the high expression levels in the cancerous group (light yellow color). The individual AUCs of these 10 identified miRNAs are listed in Figure 4 which shows that each of 10 miRNAs has high AUC in all proposed models. Next, AUCs of all selected miRNAs are presented in Figure 5 which clearly indicates that all moles, except DT, have above 99% AUC. All miRNA-target gene interactions are represented in Figure 6. The purple circles represent the target genes implicated in cancer-related pathways that are shown by yellow circles.
FIGURE 2. Boxplots of the 10 identified miRNAs in ovarian cancer patients compared with the non-cancer control patients in the dataset GSE106817.
FIGURE 3. Heatmap of hierarchical clustering analysis using the 10 identified miRNAs to distinguish different samples in the dataset GSE106817.
FIGURE 4. Diagnostic performance of the 10 identified serum miRNA signatures in the internal (GSE106817) data.
FIGURE 5. AUC of proposed models of all identified microRNAs in the internal (GSE106817) validation data.
Given the robust performance of 10 miRNAs in the internal datasets, we further examined their performance in independent external validation (GSE113486). External validation dataset (GSE113486) has 40 ovarian cancer patients and 52 non-cancer controls (43% ovarian cancer, 57% non-cancer). We found that all the miRNAs had high performance and could efficiently distinguish the ovarian cancer samples from non-cancer controls.
As shown in Figure 7, hsa-miR-320b, hsa-miR-1233-5p, hsa-miR-3184-5p, and hsa-miR-4783-3p have 100% of AUC in all proposed models. In the external validation dataset (GSE113486), the AUC of each candidate miRNAs was over 95% (minimum AUC: 95.7%, maximum AUC: 100%) for ovarian cancer classification (Figure 7). From Supplementary Figure S2, it is clear that, except DT, other machine learning models have an AUC over 100% in the external validation dataset with 10 selected miRNAs.
FIGURE 7. Validation of the diagnostic performance of the three selected miRNA high signatures in the external (GSE113486) data.
The models that yielded the highest AUC, accuracy, and sensitivity are shown in Table 3. As displayed in Table 3 (and also Supplementary Figure S2), we found four models yielded 100% AUC; however, DT did not have a strong performance because it is weak learner (Drucker and Cortes, 1996).
Finally, to make use of our prediction models, the practitioners can give the values of the 10 selected miRNAs in the online excel sheet (https://ufile.io/t2exrfph) and calculate the probability of the ovarian cancer for the patient (Supplementary Figure S1).
In the early phases, ovarian cancer is mostly asymptomatic or existent with only non-specific symptoms (Desai et al., 2014; Tuncer et al., 2020). Intervention at this phase makes ovarian cancer almost curable, and thus, early detection and diagnosis are critical to decrease the incidence and mortality of ovarian cancer (Zhang et al., 2011). Therefore, in this study, we used effective strategies and identified 10 miRNAs (hsa-miR-5100, hsa-miR-6800-5p, hsa-miR-1233-5p, hsa-miR-4532, hsa-miR-4783-3p, hsa-miR-4787-3p, hsa-miR-1228-5p, hsa-miR-1290, hsa-miR-3184-5p, and hsa-miR-320b) as strong potential biomarkers for ovarian cancer. We found that these miRNAs (all together) had high enough prediction accuracy for identification of ovarian cancer from non-cancer (logistic regression had an AUC 100%, sensitivity 100%, and specificity 100%; decision trees had an AUC 92.60%, sensitivity 92.5%, and specificity 90.38%; random forest had an AUC 100%, sensitivity 95%, and specificity 100%; artificial neural network had an AUC 100%, sensitivity 100%, and specificity 100.0%; and XGBoost had an AUC 100%, sensitivity 97.50%, and specificity 100%). Furthermore, hsa-miR-5100, hsa-miR.4532, hsa-miR.4783.3p, and hsa-miR-320b were more stable in the discovery and validation datasets.
There is evidence in the literature for the biomarkers included in our study. Huang et al. (2011) showed that modulation of miR-5100 could potentially be employed as a therapeutic target for cancer (Huang H. et al., 2015). It has shown that major target gene of miR-5100 is AZIN1. AZIN1 gene encodes antizyme inhibitor 1, the first member of this gene family that is ubiquitously expressed, and is localized in the nucleus and cytoplasm. Overexpression of antizyme inhibitor one gene has been associated with increased proliferation, cellular transformation, and tumorigenesis (Hu et al., 2017). Also, our result is important about the relationship between ovarian cancer and miR-5100 because of target gene function. Tuncer et al. (2020) suggested that hsa-miR-6800-5p is an effective biomarker for ovarian cancer. MiR-1233 is considered an oncomiRNA since it targets p53, inhibiting its function in RCC (Iwamoto et al., 2014). Hu et al., (2017) showed that miR-4532 is involved in the multidrug resistance formation in breast cancer by targeting hypermethylated cancer 1 (HIC-1), a tumor-suppressor gene (Feng et al., 2018). Also, hsa-miR-4783-3p has a major target of INSM1/IA-1 (insulinoma-associated one gene) (http://mirdb.org/) and this gene is a developmentally regulated zinc-finger transcription factor, exclusively expressed in the foetal pancreas and nervous systems, and in tumours of neuroendocrine origin (Juhlin et al., 2020). Li et al., 2016 suggest that miRNA-1228 is deregulated, and the most encompassed biological pathways are apoptosis-related (Li et al., 2016). In another study, miR-1290 is significantly overexpressed in patients with high-grade serous ovarian carcinoma (HGSOC) and they suggested that it is a new potential diagnostic biomarker for HGSOC. Exosomal miR-1290 is a potential biomarker of high-grade serious ovarian carcinoma (Cortez et al., 2018). The study of Tuncer et al. (2020) revealed that miR-320b belonged to the miR-320 family which has low expression levels in ovarian cancer. Prior studies indicated that decreased expression level of the miR-320 family is associated to activate cell proliferation (Tuncer et al., 2020). We have analyzed the major target genes of the upregulated miRNA interactions (Supplementary Figure S3). We found only two gene interactions with string database system, especially TP53 and HIC1 genes associated with a related system in human metabolism (Supplementary Figure S3).
This study has several strengths. Firstly, we applied logistic regression and four of the main machine learning approaches to predict ovarian cancer. Secondly, we identified predictive models to predict the ovarian cancer. Our findings provided strong evidence that the serum miRNA profile represented a promising diagnostic biomarker for ovarian cancer. Thirdly, we used two robust variable selection approaches to identify the important miRNAs. Finally, we evaluated the prediction accuracy of the proposed prediction models in both internal and external data to provide more robust results for practical and clinical applications.
However, there were certain limitations in our study. We had relatively small sample size in ovarian cancer group. Other limitations were the pathological information such as the tumor stage, age, or other factors which were not available in GSE106817 dataset. Nonetheless, the prediction accuracy of our model has high enough (100% AUC) for clinical use. But we still suggest further study to consider age, stage, and other unrecognized factors associated with ovarian cancer that has not included in the current paper. Also, we restricted our analysis to ovarian cancer patients and non-cancer controls, and we did not evaluate the capability of these miRNAs to distinguish ovarian cancer from other cancers.
In this paper, we used the state-of-the-art machine learning algorithms along with so-called penalized statistical approaches to model ovarian cancer with miRNA data. Our algorithms selected 10 important miRNA that can predict the ovarian cancer with an AUC of 100%. Our findings provided significant evidence that the serum miRNA profile represents a promising diagnostic biomarker for ovarian cancer.
The original contributions presented in the study are included in the article/Supplementary Material. Further inquiries can be directed to the corresponding author.
RA, NG, and FH contributed to the conception and design of the study. RA, NG, and FH performed the statistical analysis. FH wrote the first draft of the manuscript. TE wrote the biological discussion section. RA, NG, PS, TE, FH and PS wrote sections of the manuscript. All authors contributed to manuscript revision and read and approved the submitted version.
This study was supported by Tabriz University of Medical Sciences with grant number 66567.
The authors declare that the research was conducted in the absence of any commercial or financial relationships that could be construed as a potential conflict of interest.
All claims expressed in this article are solely those of the authors and do not necessarily represent those of their affiliated organizations, or those of the publisher, the editors, and the reviewers. Any product that may be evaluated in this article, or claim that may be made by its manufacturer, is not guaranteed or endorsed by the publisher.
The authors would like to thank all those who spent their valuable time participating in this research project, and we are also immensely grateful to the reviewers.
The Supplementary Material for this article can be found online at: https://www.frontiersin.org/articles/10.3389/fgene.2021.724785/full#supplementary-material
Abdulqader, Q. M. (2017). Applying the Binary Logistic Regression Analysis on the Medical Data. Sci. J. Univ. Zakho 5 (4), 330–334. doi:10.25271/2017.5.4.388
Asano, N., Matsuzaki, J., Ichikawa, M., Kawauchi, J., Takizawa, S., Aoki, Y., et al. (2019). A Serum microRNA Classifier for the Diagnosis of Sarcomas of Various Histological Subtypes. Nat. Commun. 10 (1), 1299–1310. doi:10.1038/s41467-019-09143-8
Banka, H., and Dara, S. (Editors) (2012). Feature Selection and Classification for Gene Expression Data Using Evolutionary Computation. Vienna, Austria: 23rd International Workshop on Database and Expert Systems Applications, IEEE.
Chen, T., and Guestrin, C. (Editors) (2016). “Xgboost: A Scalable Tree Boosting System,” in Proceedings of the 22nd ACM SIGKDD International Conference on Knowledge Discovery and Data Mining.
Chen, T., He, T., Benesty, M., Khotilovich, V., Tang, Y., and Cho, H. (2015). Xgboost: Extreme Gradient Boosting. R. Package Version 04-2 1 (4), 1–4. doi:10.1038/onc.2014.117
Collins, G. S., Reitsma, J. B., Altman, D. G., and Moons, K. G. M. (2015). Transparent Reporting of a Multivariable Prediction Model for Individual Prognosis or Diagnosis (TRIPOD). Circulation 131 (2), 211–219. doi:10.1161/circulationaha.114.014508
Cortez, A. J., Tudrej, P., Kujawa, K. A., and Lisowska, K. M. (2018). Advances in Ovarian Cancer Therapy. Cancer Chemother. Pharmacol. 81 (1), 17–38. doi:10.1007/s00280-017-3501-8
Deb, B., Uddin, A., and Chakraborty, S. (2018). miRNAs and Ovarian Cancer: An Overview. J. Cel Physiol 233 (5), 3846–3854. doi:10.1002/jcp.26095
DeGregory, K. W., Kuiper, P., DeSilvio, T., Pleuss, J. D., Miller, R., Roginski, J. W., et al. (2018). A Review of Machine Learning in Obesity. Obes. Rev. 19 (5), 668–685. doi:10.1111/obr.12667
Desai, A., Xu, J., Aysola, K., Qin, Y., Okoli, C., Hariprasad, R., et al. (2014). Epithelial Ovarian Cancer: An Overview. World J. Transl. Med. 3 (1), 1. doi:10.5528/wjtm.v3.i1.1
Drotár, P., Gazda, J., and Smékal, Z. (2015). An Experimental Comparison of Feature Selection Methods on Two-Class Biomedical Datasets. Comput. Biol. Med. 66, 1–10. doi:10.1016/j.compbiomed.2015.08.010
Drucker, H., and Cortes, C. (1996).Boosting Decision Trees Adv. Neural Inf. Process. Syst., 479–485.
Falzone, L., Candido, S., Salemi, R., Basile, M. S., Scalisi, A., McCubrey, J. A., et al. (2016). Computational Identification of microRNAs Associated to Both Epithelial to Mesenchymal Transition and NGAL/MMP-9 Pathways in Bladder Cancer. Oncotarget 7 (45), 72758–72766. doi:10.18632/oncotarget.11805
Falzone, L., Lupo, G., Rosa, G. R. M., Crimi, S., Anfuso, C. D., Salemi, R., et al. (2019). Identification of Novel MicroRNAs and Their Diagnostic and Prognostic Significance in Oral Cancer. Cancers 11 (5), 610. doi:10.3390/cancers11050610
Fan, Y., Siklenka, K., Arora, S. K., Ribeiro, P., Kimmins, S., and Xia, J. (2016). miRNet - Dissecting miRNA-Target Interactions and Functional Associations through Network-Based Visual Analysis. Nucleic Acids Res. 44 (W1), W135–W141. doi:10.1093/nar/gkw288
Feng, F., Zhu, X., Wang, C., Chen, L., Cao, W., Liu, Y., et al. (2018). Downregulation of Hypermethylated in Cancer-1 by miR-4532 Promotes Adriamycin Resistance in Breast Cancer Cells. Cancer Cell Int. 18 (1), 127–212. doi:10.1186/s12935-018-0616-x
Friedman, J., Hastie, T., and Tibshirani, R. (2010). Regularization Paths for Generalized Linear Models via Coordinate Descent. J. Stat. Softw. 33 (1), 1–22. doi:10.18637/jss.v033.i01
Greenlee, R. T., Hill-Harmon, M. B., Murray, T., and Thun, M. (2001). Cancer Statistics, 2001. CA Cancer J. Clin. 51 (1), 15–36. doi:10.3322/canjclin.51.1.15
Harter, P., Reuss, A., Pfisterer, J., Pujade-Lauraine, E., Ray, I., and du Bois, A. (2008).The Role of Surgical Outcome as Prognostic Factor in Advanced Epithelial Ovarian Cancer. A Project of the AGO-OVAR and GINECO–Prognostic Factor Surgical Outcome in Advanced Ovarian Cancer. Geburtshilfe Frauenheilkd. 68, 1–4. doi:10.1055/s-0028-1088605
Hassanipour, S., Ghaem, H., Arab-Zozani, M., Seif, M., Fararouei, M., Abdzadeh, E., et al. (2019). Comparison of Artificial Neural Network and Logistic Regression Models for Prediction of Outcomes in Trauma Patients: A Systematic Review and Meta-Analysis. Injury 50 (2), 244–250. doi:10.1016/j.injury.2019.01.007
Hastie, T., Tibshirani, R., and Friedman, J. (2009). The Elements of Statistical Learning: Data Mining, Inference, and Prediction. Berlin, Germany: Springer Science & Business Media.
Hu, X., Chen, J., Shi, X., Feng, F., Lau, K. W., Chen, Y., et al. (2017). RNA Editing of AZIN1 Induces the Malignant Progression of Non-small-cell Lung Cancers. Tumour Biol. 39 (8), 1010428317700001. doi:10.1177/1010428317700001
Huang, , Haili, , Jiang, Y., Wang, Y., Chen, T., Yang, L., et al. (2011). miR-5100 promotes tumor growth in lung cancer by targeting Rab6. Cancer letters 362 (1), 15–24. doi:10.1016/j.canlet.2015.03.004
Huang, H., Jiang, Y., Wang, Y., Chen, T., Yang, L., He, H., et al. (2015). miR-5100 Promotes Tumor Growth in Lung Cancer by Targeting Rab6. Cancer Lett. 362 (1), 15–24. doi:10.1016/j.canlet.2015.03.004
Huang, J., Li, Y.-F., and Xie, M. (2015). An Empirical Analysis of Data Preprocessing for Machine Learning-Based Software Cost Estimation. Inf. Softw. Tech. 67, 108–127. doi:10.1016/j.infsof.2015.07.004
Iorio, M. V., Visone, R., Di Leva, G., Donati, V., Petrocca, F., Casalini, P., et al. (2007). MicroRNA Signatures in Human Ovarian Cancer. Cancer Res. 67 (18), 8699–8707. doi:10.1158/0008-5472.can-07-1936
Iwamoto, H., Kanda, Y., Sejima, T., Osaki, M., Okada, F., and Takenaka, A. (2014). Serum miR-210 as a Potential Biomarker of Early clear Cell Renal Cell Carcinoma. Int. J. Oncol. 44 (1), 53–58. doi:10.3892/ijo.2013.2169
James, G., Witten, D., Hastie, T., and Tibshirani, R. (2013). An Introduction to Statistical Learning. Springer.
Juhlin, C. C., Zedenius, J., and Höög, A. (2020). Clinical Routine Application of the Second-Generation Neuroendocrine Markers ISL1, INSM1, and Secretagogin in Neuroendocrine Neoplasia: Staining Outcomes and Potential Clues for Determining Tumor Origin. Endocr. Pathol. 31 (4), 401–410. doi:10.1007/s12022-020-09645-y
Lee, Y. S., and Dutta, A. (2009). MicroRNAs in Cancer. Annu. Rev. Pathol. Mech. Dis. 4, 199–227. doi:10.1146/annurev.pathol.4.110807.092222
Lheureux, S., Gourley, C., Vergote, I., and Oza, A. M. (2019). Epithelial Ovarian Cancer. Lancet 393 (10177), 1240–1253. doi:10.1016/s0140-6736(18)32552-2
Li, X., Ding, Z., Zhang, C., Zhang, X., Meng, Q., Wu, S., et al. (2016). MicroRNA-1228* Inhibit Apoptosis in A549 Cells Exposed to fine Particulate Matter. Environ. Sci. Pollut. Res. 23 (10), 10103–10113. doi:10.1007/s11356-016-6253-9
Liang, X., and Jacobucci, R. (2020). Regularized Structural Equation Modeling to Detect Measurement Bias: Evaluation of Lasso, Adaptive Lasso, and Elastic Net. Struct. Equ. Modeling 27 (5), 722–734. doi:10.1080/10705511.2019.1693273
Lin, X.-J., Chong, Y., Guo, Z.-W., Xie, C., Yang, X.-J., Zhang, Q., et al. (2015). A Serum microRNA Classifier for Early Detection of Hepatocellular Carcinoma: a Multicentre, Retrospective, Longitudinal Biomarker Identification Study with a Nested Case-Control Study. Lancet Oncol. 16 (7), 804–815. doi:10.1016/s1470-2045(15)00048-0
Lisboa, P. J., and Taktak, A. F. G. (2006). The Use of Artificial Neural Networks in Decision Support in Cancer: a Systematic Review. Neural Netw. 19 (4), 408–415. doi:10.1016/j.neunet.2005.10.007
Lunardon, N., Menardi, G., and Torelli, N. (2014). ROSE: A Package for Binary Imbalanced Learning. R J. 6 (1). doi:10.32614/rj-2014-008
Menard, S. (2010). Logistic Regression: From Introductory to Advanced Concepts and Applications. Thousand Oaks, CA, California: Sage.
Nam, E. J., Yoon, H., Kim, S. W., Kim, H., Kim, Y. T., Kim, J. H., et al. (2008). MicroRNA Expression Profiles in Serous Ovarian Carcinoma. Clin. Cancer Res. 14 (9), 2690–2695. doi:10.1158/1078-0432.ccr-07-1731
Okun, O., and Priisalu, H. (Editors) (2007). “Random forest for Gene Expression Based Cancer Classification: Overlooked Issues,” in Iberian Conference on Pattern Recognition and Image Analysis (Springer).
Qi, Y. (2012). “Random Forest for Bioinformatics,” in Ensemble Machine Learning (Springer), 307–323. doi:10.1007/978-1-4419-9326-7_11
Reid, B. M., Permuth, J. B., and Sellers, T. A. (2017). Epidemiology of Ovarian Cancer: a Review. Cancer Biol. Med. 14 (1), 9–32. doi:10.20892/j.issn.2095-3941.2016.0084
Sherriff, A., Ott, J., and Team, A. S. (2004). Artificial Neural Networks as Statistical Tools in Epidemiological Studies: Analysis of Risk Factors for Early Infant Wheeze. Paediatr. Perinat Epidemiol. 18 (6), 456–463. doi:10.1111/j.1365-3016.2004.00592.x
Stoltzfus, J. C. (2011). Logistic Regression: a Brief Primer. Acad. Emerg. Med. 18 (10), 1099–1104. doi:10.1111/j.1553-2712.2011.01185.x
Tuncer, S. B., Erdogan, O. S., Erciyas, S. K., Saral, M. A., Celik, B., Odemis, D. A., et al. (2020). miRNA Expression Profile Changes in the Peripheral Blood of Monozygotic Discordant Twins for Epithelial Ovarian Carcinoma: Potential New Biomarkers for Early Diagnosis and Prognosis of Ovarian Carcinoma. J. Ovarian Res. 13 (1), 99–15. doi:10.1186/s13048-020-00706-8
Vens, C., Struyf, J., Schietgat, L., Džeroski, S., and Blockeel, H. (2008). Decision Trees for Hierarchical Multi-Label Classification. Mach Learn. 73 (2), 185–214. doi:10.1007/s10994-008-5077-3
Wang, Y., Tetko, I. V., Hall, M. A., Frank, E., Facius, A., Mayer, K. F. X., et al. (2005). Gene Selection from Microarray Data for Cancer Classification-A Machine Learning Approach. Comput. Biol. Chem. 29 (1), 37–46. doi:10.1016/j.compbiolchem.2004.11.001
Yao, Y., Ding, Y., Bai, Y., Zhou, Q., Lee, H., Li, X., et al. (2020). Identification of Serum Circulating MicroRNAs as Novel Diagnostic Biomarkers of Gastric Cancer. Front. Genet. 11, 591515. doi:10.3389/fgene.2020.591515
Zhang, B., Cai, F. F., and Zhong, X. Y. (2011). An Overview of Biomarkers for the Ovarian Cancer Diagnosis. Eur. J. Obstet. Gynecol. Reprod. Biol. 158 (2), 119–123. doi:10.1016/j.ejogrb.2011.04.023
Keywords: Biomarker, Elasticnet, Feature Selection, Gene Expression Omnibus (GEO), Lasso, Machine Learning, Ovarian Cancer
Citation: Hamidi F, Gilani N, Belaghi RA, Sarbakhsh P, Edgünlü T and Santaguida P (2021) Exploration of Potential miRNA Biomarkers and Prediction for Ovarian Cancer Using Artificial Intelligence. Front. Genet. 12:724785. doi: 10.3389/fgene.2021.724785
Received: 14 June 2021; Accepted: 07 October 2021;
Published: 25 November 2021.
Edited by:
Tao Wang, Northwestern Polytechnical University, ChinaReviewed by:
Bor-Sen Chen, National Tsing Hua University, TaiwanCopyright © 2021 Hamidi, Gilani, Belaghi, Sarbakhsh, Edgünlü and Santaguida. This is an open-access article distributed under the terms of the Creative Commons Attribution License (CC BY). The use, distribution or reproduction in other forums is permitted, provided the original author(s) and the copyright owner(s) are credited and that the original publication in this journal is cited, in accordance with accepted academic practice. No use, distribution or reproduction is permitted which does not comply with these terms.
*Correspondence: Reza Arabi Belaghi, ci5hcmFiaUB0YWJyaXp1LmFjLmly
Disclaimer: All claims expressed in this article are solely those of the authors and do not necessarily represent those of their affiliated organizations, or those of the publisher, the editors and the reviewers. Any product that may be evaluated in this article or claim that may be made by its manufacturer is not guaranteed or endorsed by the publisher.
Research integrity at Frontiers
Learn more about the work of our research integrity team to safeguard the quality of each article we publish.