- 1Department of Biobehavioral Health, College of Health and Human Development, The Pennsylvania State University, University Park, PA, United States
- 2Carey Business School, Johns Hopkins University, Baltimore, MD, United States
- 3Department of Epidemiology, Gillings School of Global Public Health, University of North Carolina at Chapel Hill, Chapel Hill, NC, United States
- 4Department of Psychology, University of Miami, Coral Gables, FL, United States
- 5Department of Epidemiology and Population Health, Albert Einstein College of Medicine, Bronx, NY, United States
- 6Carolina Center for Genome Sciences, School of Medicine, University of North Carolina at Chapel Hill, Chapel Hill, NC, United States
- 7Department of Psychology, San Diego State University, San Diego, CA, United States
- 8Institute for Minority Health Research, Carle Illinois College of Medicine, University of Illinois at Urbana–Champaign, Champaign, IL, United States
- 9Institute for Minority Health Research, University of Illinois at Chicago, Chicago, IL, United States
- 10Department of Epidemiology, Johns Hopkins University, Baltimore, MD, United States
- 11Department of Biostatistics, Gillings School of Global Public Health, University of North Carolina at Chapel Hill, Chapel Hill, NC, United States
- 12Department of Social Medicine, University of North Carolina School of Medicine, Chapel Hill, NC, United States
Introduction: Hispanic/Latinos experience a disproportionate burden of obesity. Acculturation to US obesogenic diet and practices may lead to an exacerbation of innate genetic susceptibility. We examined the role of gene–environment interactions to better characterize the sociocultural environmental determinants and their genome-scale interactions, which may contribute to missing heritability of obesity. We utilized polygenic risk scores (PRSs) for body mass index (BMI) to perform analyses of PRS-by-acculturation and other environmental interactors among self-identified Hispanic/Latino adults from the Hispanic Community Health Study/Study of Latinos (HCHS/SOL).
Methods: PRSs were derived using genome-wide association study (GWAS) weights from a publicly available, large meta-analysis of European ancestry samples. Generalized linear models were run using a set of a priori acculturation-related and environmental factors measured at visit 1 (2008–2011) and visit 2 (2014–2016) in an analytic subsample of 8,109 unrelated individuals with genotypic, phenotypic, and complete case data at both visits. We evaluated continuous measures of BMI and waist-to-hip ratio. All models were weighted for complex sampling design, combined, and sex-stratified.
Results: Overall, we observed a consistent increase of BMI with greater PRS across both visits. We found the best-fitting model adjusted for top five principal components of ancestry, sex, age, study site, Hispanic/Latino background genetic ancestry group, sociocultural factors and PRS interactions with age at immigration, years since first arrival to the United States (p < 0.0104), and healthy diet (p < 0.0036) and explained 16% of the variation in BMI. For every 1-SD increase in PRS, there was a corresponding 1.10 kg/m2 increase in BMI (p < 0.001). When these results were stratified by sex, we observed that this 1-SD effect of PRS on BMI was greater for women than men (1.45 vs. 0.79 kg/m2, p < 0.001).
Discussion: We observe that age at immigration and the adoption of certain dietary patterns may play a significant role in modifying the effect of genetic risk on obesity. Careful consideration of sociocultural and immigration-related factors should be evaluated. The role of nongenetic factors, including the social environment, should not be overlooked when describing the performance of PRS or for promoting population health in understudied populations in genomics.
Introduction
Obesity is a significant public health issue, with an estimated 34.9% of adults and 16.9% children in the United States reported as obese (Ogden et al., 2014). From 1999 to 2018, the prevalence of both obesity and severe obesity continued to increase among adults (Hales et al., 2020). By 2030, the economic consequences of obesity if left unaddressed could represent up to $66 billion per year in the United States (Wang et al., 2011) and a concomitant increase in health sequelae, most notably cardiovascular disease (CVD), diabetes, cancer, and loss of quality-adjusted life years (National Heart, Lung, and Blood Institute 2013). Hispanic/Latino adults bear a disproportionate burden of obesity, as they are 1.2 times more likely to be obese than non-Hispanic Whites (OMH, 2020). The age-adjusted prevalence of obesity is approximately 42.6% obesity Hispanic adults in the United States (as compared with 37.7% among non-Hispanic White adults) (Flegal et al., 2012). Additionally, there are observed gender disparities, wherein women have a higher prevalence of obesity than men, related, in part, to a complex set of sociocultural and other environmental factors or biologic sex-related differences (Kanter and Caballero 2012; Link and Reue 2017).
Extensive evidence supports the role of lifestyle modification for reducing obesity; however, less attention has been paid to understanding the role of gene–environment (G×E) interactions in population trends or when informing precision medicine initiatives. G×E interactions are believed to play an important role in obesity (Flowers et al., 2012; Ahmad et al., 2013), as they have the potential to inform our understanding of how environmental determinants affect an individual’s health. In fact, G×E studies can lead to a more accurate genetic effect estimation than that observed in the original discovery study and may account in part for “missing heritability” of complex traits, like obesity (Flowers et al., 2012). Obesogenic environments, such as those in the United States, may differentially (Ogden et al., 2007) impact individuals who carry more genetic susceptibility variants. Environmental interactions with a polygenic score for obesity have been previously reported for sugar-sweetened beverages (Qi et al., 2012), dietary patterns, specifically fried food (Qi et al., 2014), calorie (Lee et al., 2021) and alcohol intake (Nakamura et al., 2016), and physical activity (Tyrrell et al., 2017). These PRS–environment interactions informed our a priori selection of environmental factors to evaluate. Yet to our knowledge, limited work has been done to date to also investigate how acculturation—a complex process of an individual’s “maintenance of the original cultural and development of relationships with the new (host) culture” (Berry 2004; Thomson and Hoffman-Goetz 2009; Wallace et al., 2010)—may modify the observed effect of established genetic risk for obesity, given its relevance in the social sciences and public health (Khan et al., 1997; Sundquist and Winkleby 2000; Gordon-Larsen et al., 2003; Barcenas et al., 2007; Pérez-Escamilla and Putnik 2007; Duffey et al., 2008; Roshania et al., 2008; Guendelman et al., 2011; Van Wieren et al., 2011; Liu J.-H. et al., 2012; Murillo et al., 2015).
G×E effects on measures of obesity may be assessed using any of the three following approaches (Nagpal et al., 2018): by measuring 1) individual genotypes or single-nucleotide polymorphisms (SNPs), 2) polygenic genetic liability of disease, and 3) whole-genome common variants (SNP heritability). Herein, we build on a previous study in Hispanic Community Health Study/Study of Latinos (HCHS/SOL) that reported interactions between genetic risk score across 103 SNPs and acculturation, wherein the observed genetic effects were the highest among the most acculturated and among women, as compared with the least acculturated and men (under review at Demography).
In this current study, we aimed to further investigate the G×E interaction by using a more encompassing predictor of disease risk—the polygenic risk score (PRS)—and by taking a more comprehensive view of the environment. PRSs allow for a single measure of genetic liability for disease risk or inherited obesity susceptibility (Khera et al., 2019). PRSs are commonly constructed as the weighted sum of scores using effects sizes from genome-wide association studies (GWASs) as their weights and the count of observed risk allele and linkage disequilibrium based on the dataset of interest (Grinde et al., 2019). Increasingly, there is evidence that obesity is highly polygenic (Choquet and Meyre 2011; Reddon et al., 2016); and to date, over 1,000 unique obesity-related genome wide association study loci have been identified (GWAS Catalog, 2020). Yet most G×E interaction analyses to date of obesity have been limited to <100 genetic variants accounting for <3% variation in body mass index (BMI) (Locke et al., 2015; Reddon et al., 2016; Grinde et al., 2019).
Overall, we sought to investigate the association between a robust measure of genetic susceptibility to BMI (PRSBMI) and BMI and evidence of effect modification by acculturation, immigration, lifestyle, and other environmental factors in a diverse community-based sample of Hispanic/Latino adults. We described the utility of the PRSBMI prior to estimating the G×E interactions with respect to acculturation, immigration, lifestyle, and other environmental factors. We then evaluated the stability of these genetic estimates over time by examining the role of PRSBMI, and G×E interactions at baseline and a 6-years follow-up. We hypothesized that acculturation to the United States may be associated with a number of adaptations to a new host culture that could lead to an additional level of modification of innate genetic susceptibility in the Hispanic/Latino community. We hypothesized that greater acculturation to the United States would exacerbate the PRS association with elevated BMI, obesity, or greater adulthood change in BMI, consistent with a diathesis-stress gene–environment interaction model (Boardman et al., 2014). We tested this hypothesis with genetic (PRSBMI) and BMI information as well as measures of acculturation using a sample of 8,109 US Hispanic/Latino adults aged 20–76 years from four HCHS/SOL urban communities (The Bronx, NY; Chicago, IL; Miami, FL; and San Diego, CA).
Methods
Study Population
The HCHS/SOL is cohort of 16,415 individuals aged 18–74 years in 2008–2011 from four US urban communities and diverse cultural and genetic origins who self-identified as being Hispanic/Latino. Individuals in the HCHS/SOL provided information about their background (heritage) as being of Cuban, Dominican, Puerto Rican, Mexican, Central American, South American, or other/multiple backgrounds. Recruitment for the HCHS/SOL was implemented through a two-stage area household probability design (Lavange et al., 2010). Study individuals completed visit 1 (2008–2011) and visit 2 (2014–2017). As previously reported, HCHS/SOL individuals provided informed consent for genetic testing at visit 1 and passed quality control measures (Sofer et al., 2018), 10,240 of whom were unrelated (defined as second-degree or beyond) first and included in PRS estimation, described below. Complete case analysis based on non-missing values for visit 1 and visit 2 was performed on a subset of individuals with genotype and phenotype information (see Supplementary Figure S1 for inclusion/exclusion flowchart). These exclusions decreased the available analytic sample in HCHS/SOL to 8,109 Hispanic/Latino adults, aged 20–76 years at visit 1 and 6,408 for visit 2. The HCHS/SOL was approved by each site’s local institutional review board as well as at the Coordinating Center.
Genotyping, Quality Control, and Examination Methods
Genotyping
The HCHS/SOL study protocol and examination, genotyping, quality control, and imputation methods have been described previously (Conomos et al., 2016; Sofer et al., 2018). Briefly, DNA was extracted from individual blood samples and genotyped on the MEGA Custom 15041502 array (Illumina Omni2.5M + custom content). Quality control measures included constructing principal components (PCs) of genetic ancestry to account for population stratification. Imputation was then conducted to 1000 Genomes Project phase 3 based on 1000 Genomes Project reference populations.
Polygenic Risk Score Estimation
The PRSBMI was estimated to capture genome-wide risk of elevated BMI for subsequent G×E analyses, using HCHS/SOL genome-wide data and publicly available genome-wide effect sizes from a recent European ancestry meta-analysis of ∼700,000 GIANT and United Kingdom Biobank (UKBB) samples (Yengo et al., 2018). PRS was calculated for SNPs with minor allele frequency >0.5% in HCHS/SOL based on effect sizes from the GWAS of Yengo et al. (2018) using the LDpred method and infinitesimal model described previously (Vilhjálmsson et al., 2015). The best-fitting PRS from the LDpred method predicted 7.4% of the variance in inverse normalized BMI in HCHS/SOL after accounting for age, sex, the first 10 PCs, genetic ancestry background Hispanic/Latino group, and the normalized log of the sample weight. This best-fitting PRS was then standardized to a mean of 0 and standard deviation of 1 and carried forward to our G×E analyses described in detail below, although most results are reported as a 1-SD change in PRS from the mean. In sensitivity analyses, we additionally considered a decile change in PRSBMI to be consistent with other published reports (Khera et al., 2019).
Obesity-Related Measurements
BMI was calculated as the ratio of an individual’s weight (kg) to their height (m) squared. Both weight and height were measured at examination by trained staff following a standardized protocol in HCHS/SOL (Sorlie et al., 2010). Similar to previous analyses of genetic variation in HCHS/SOL samples (Fesinmeyer et al., 2013; Gong et al., 2018), we excluded individuals <20 years of age or with BMIs <18.5 or >70.0 kg/m2 from our analytic sample, to focus on individuals who had both completed growing and were unlikely to have monogenic forms of obesity or gross data errors (Fesinmeyer et al., 2013; Gong et al., 2018). The resulting beta coefficients can be interpreted as change in kg/m2 of BMI for a 1-SD change from the mean PRSBMI, or per decile (10%) change from the median in our sensitivity analyses. In addition, we examined alternate specifications for adiposity including a dichotomous measure of obesity (as a BMI ≥ 30 kg/m2) and a continuous waist-to-hip ratio.
Sociocultural Environments: Acculturation- and Immigration-Related Measures
Acculturation is a multidimensional, directional, and dynamic process, which is heavily influenced by both the timing and pattern of sociocultural exposures across one’s life course (Ben-Shlomo and Kuh 2002; Berry 2004; Wallace et al., 2010). Proxy measures of acculturation, such as language preference, nativity, and time in the United States, are commonly collected in large epidemiologic studies (Thomson and Hoffman-Goetz 2009). Although the ability of such proxies to capture the complexity of the construct of acculturation has been criticized (Carter-Pokras and Bethune 2009; Thomson and Hoffman-Goetz 2009), they remain a staple of epidemiologic research due to their accessibility. The baseline HCHS/SOL visit 1 was conducted in either English or Spanish based on the individual’s preference and included questionnaires about an individual’s immigration history (e.g., nativity of one’s self and their family members, and information about first arrival to the United States), self-identified Hispanic/Latino background, and 10-item modification of the Short Acculturation Scale for Hispanics scale (Marin et al., 1987), a uni-dimensional/uni-directional scale that includes Language Use and Ethnic and Social & Ethnic Relations, each ranging from 1 to 5 low-to-high acculturation. Language preference was the spoken language preferred by the participant at visit 1 (Spanish or English).
In addition to the above measures, five categories of age at immigration (US-born, 0–5, 6–12, 13–20, and ≥21 years) were constructed using nativity status, and time since first arrival to measure the duration of exposure to the obesogenic US culture, consistent with other works using a sensitive period of acculturation (Rumbaut 2005) that evaluates the age at immigration. We categorized this new variable as US-born, 0–5, 6–12, 13–20, and ≥21 years and for simplicity refer to it as an “immigration-related” sociocultural factor, even though it is composed of multiple proxies of acculturation (described above). In addition to this sensitive period of acculturation, immigrant generation status may be an important predictor of duration or pattern of sociocultural exposures (Chrisman et al., 2017) and was also included in our modeling. Thus, in order to understand these migration and acculturation patterns, we examined immigrant generation status (first or second) based on a combination of the individual’s country of origin, maternal and paternal parents, and maternal and grandparents as first generation, which includes foreign-born with foreign-born parents including those born in a US territory, compared with second generation, which includes US-born (50 states and DC) or foreign-born with at least one US-born parent.
We hypothesized that the more adapted an individual is to the host culture (e.g., US-dominant culture), the greater the risk for elevated BMI or obesity. In particular, we expected that this adaptation might be strongest for those who were the youngest at first arrival to the United States (Roshania et al., 2008; Rumbaut 2005; Stevens 1999), individuals of second-generation immigrant status (e.g., US-born to at least one immigrant parent) (Liu J.-H. et al., 2012; Chrisman et al., 2017), and those with a strong English-language (Chrisman et al., 2017) preference.
Additional Environmental Factors
We evaluated additional environmental measures of sociodemographic, chronic disease, and lifestyle factors in our G×E modeling based on previous literature (Qi et al., 2012; 2014; Lee et al., 2021; Nakamura et al., 2016; Tyrrell et al., 2017; Qi et al., 2015). These measures included the following: sex (male, female); age and age2 to account for nonlinear changes in BMI with age; genetic ancestry background Hispanic/Latino group (Central American, Cuban, Dominican, Puerto Rican, South American, and Mexican) (Conomos et al., 2016); marital status (married and living with partner, or not); education (less than high school, or completed high school or greater); employment status (retired, not retired or employed, employed ≤ 35 h/week, employed > 35 h/week); diabetes status (yes, no); prevalent CVD (yes, no); sleep duration (h/day); consumption of sweetened beverages (servings/day); physical activity guidelines met (yes/no); alcohol use level (no current use, low-level use, and high-level use); cigarette use (never, current, and former); Center for Epidemiological Studies Depression (CES-D) 10-item Summary Score (Andresen et al., 1994); and ethnic identity score (5 level ordinal variable). We independently evaluated two overall measures of diet derived from the 24-h dietary recalls collected as a part of visit 1 to better understand a diet-acculturation convention that immigrants consumed healthier diets before migration (Martínez 2013). The first score was a dietary measure published in the Journal of the American Medical Association (JAMA) (Daviglus et al., 2012) and calculated by assigning individuals a score of 1–5 (low = 1 to high = 5) according to sex-specific quintiles of usual predicted daily intake of saturated fatty acids, potassium, calcium, and fiber. The highest 40% on the summed score was defined as a healthy diet (Liu K. et al., 2012). A second score, the Alternate Healthy Eating Index 2010 (AHEI-2010), is a measure of diet quality based on foods and nutrients predictive of chronic disease risk (Chiuve et al., 2012). AHEI-2010 score is the sum of the 11 individual components’ scores, each with a range from 0 (worst) to 10 (best). Hence, AHEI-2010 healthy dietary measure takes values from 0 to 110, where higher scores represent healthy eating habits. In sensitivity analysis, we evaluated information specific to dietary acculturation as self-identified dietary ranking as more American or more Hispanic on a scale of 1–5.
Analysis
We first examined the robustness of our PRSBMI in our target population by describing its distribution, calibration, discrimination, and predictive ability, consistent with recent PRS reporting standards (Wand et al., 2021). We examined the adjusted r2 for models with basic covariates with and without the PRSBMI to assess the incremental variation to ensure that the PRSBMI would be a robust predictor for use in additional G×E analyses.
In our inferential modeling, using survey linear regression, we tested the association of BMI and PRSBMI × acculturation using data from visit 1 and 2. Continuous predictors were survey mean-centered and standardized to avoid multicollinearity in interaction models. Our full modeling used augmented backwards elimination that included sample weights (Pfeffermann 1996) to increase the chance of a reliable outcome that recovered our underlying target population (Heeringa et al., 2017; Pfeffermann 1996; Dunkler et al., 2014; Heinze et al., 2018) with passive explanatory variables, not tested for significance or change in criterion, which included the PRSBMI and the top five PCs, study center, and Hispanic/Latino background. We performed a three-stage model building, first examining linear regression main effects by augmented backwards elimination, then G×E interaction modeling separately, and finally a fully adjusted model with both main and interaction effects based on backwards elimination (see Supplementary Figure S2 for modeling diagram and Supplementary Table S1 for variable and modeling descriptions). In stage 1 of model building, we performed augmented backwards elimination starting with a global model that included passive variable (PRS, top five PCs, study center, and Hispanic/Latino background) and active variables, based on our a priori covariates (sociocultural, immigration-related, acculturation, and additional environmental factors) that were conceptually relevant. We assessed that the global model was fitted by the weighted least squares and used a progressive significance level of p < 0.20 or change in criterion tau 0.05, until all variables contributed to the model and were retained. Stage 2 testing included interaction modeling of all the previous variables tested adjusted for passive variables and interacted with PRS. We assessed joint interaction effects for nominal significance (p < 0.05). We tested the null hypothesis that the H0: β G×E = 0 retaining those interactions with two-sided t-test at significance level α = 0.05 and then retained interactions in Stage 3, the fully adjusted model linear regression modeling with interaction variables, based on Bonferroni significance adjustment (0.05/4 = 0.0125) for multiple comparisons. Parameter estimates for our PRSBMI are reported with Taylor series linearization variance estimator for the full model and sex-stratified.
Linear regression modeling was used to estimate the G×E interactions (PRSBMI × E) on BMI for the above-specified sociocultural environmental and additional environmental factors (E) at visit 1. All interaction models took the form of
where G is the genetic risk of obesity as measured by the PRS for BMI, PRSBMI; Ei is environmental (environmental sociocultural factors); Xcov1 is the vector of top five PCs; Xcov2 is the vector of covariates age, age2;
We then repeated our assessment of interaction for visit 2 to better evaluate those interactions most and least sensitive to age-related BMI changes.
The base model includes adjustment for the top five PCs, study center, and genetic Hispanic/Latino ancestry. The full model includes the base model and age, age2, sex, age at immigration, immigrant generation, sleep duration, meets physical activity guidelines, sweetened beverage consumption, JAMA healthy diet, alcohol use level, cigarette use, CES-D 10-item Summary Score, employment status, income, diabetes and CVD prevalence,
In an exploratory analysis, we further examined our results using an alternative specification for dietary measurement, the AHEI-2010 given that it may be a more culturally appropriate measure of diet quality for this population by measuring a more diverse selection of food choices (Chiuve et al., 2012). Finally, we also examined an incremental model selection stratified by genetic ancestry Hispanic/Latino background group to better understand how genetic ancestry might influence PRS performance and model selection.
Results
In our complete case analytic dataset of 8,109 Hispanic/Latino adults, we examined the variable distributions using survey univariate analysis for visit 1 (Table 1) and reported the weighted means and proportions (see Supplementary Table S2 for target population and for visit 2 demographic characteristics). Overall, the weighted sample was 48.9% female, 41.7% considered to be obese, with 65.3% with an annual income of <$30,000, 54.1% being currently employed at least part time, 69.5% with at least a high school education or equivalent, 56.3% currently married or living with a partner, 16.4% living with type 2 diabetes, and 6.6% living with prevalent CVD, such as cardiovascular heart disease or stroke. Men and women had similar weighted average age at visit 1, scores for acculturation subscales for Language Use and Social & Ethnic Relations (2.1–2.2), and distributions of age at immigration. Although nearly 20.4% of male and 17.1% of female individuals were born to US- and foreign-born individuals who have lived in the United States for an average of 17.8 years, the majority arrived during adulthood (53.8% of males and 57.2% of females). Dietary behaviors differed across the sexes, with males consuming more sweetened beverage servings daily (mean 2.2 compared with 1.3 for females) but with similar Healthy Diet scores by sex, with 48.6% males and 47.5% female in the top 40th percentile of the JAMA dietary value.
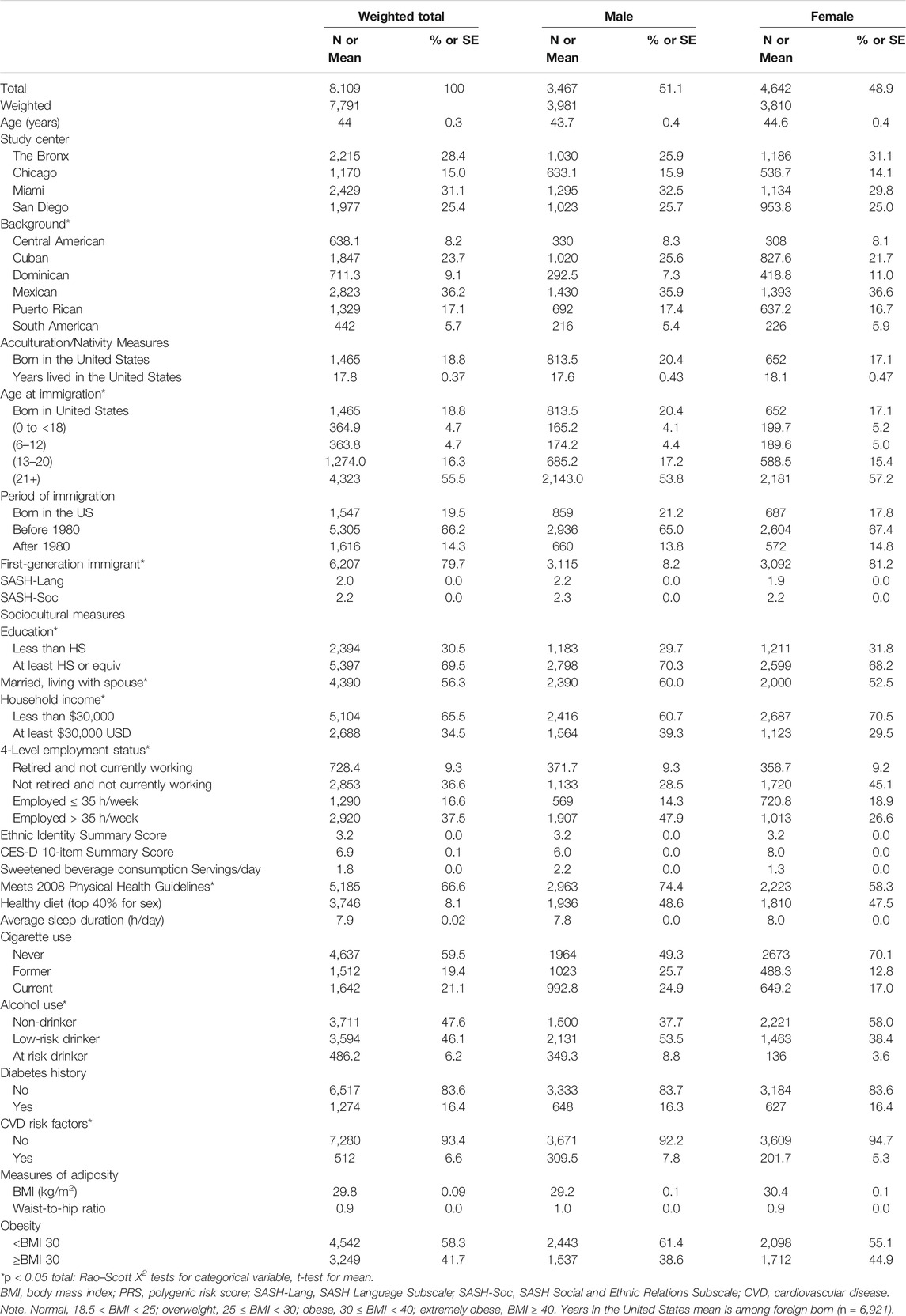
TABLE 1. Demographic characteristics Hispanic Community Health Study/Study of Latinos (HCHS/SOL) analytic subsample from visit 1 (unweighted n = 8,109), overall and sex-stratified.
PRSBMI approximated a normal distribution in the population as shown in Figure 1A, while Supplementary Figure S3 shows that our BMI distribution was right skewed, which is consistent with previous population-based studies of countries like the United States (Ogden et al., 2007). We observed a monotonic gradient of overall increasing genetic risk and obesity as shown by the fitted line (Figure 1B). However, based on the tails of the distribution, we can visually determine that PRSBMI is not strictly deterministic of obesity (Khera et al., 2019). For example, when looking at decile distribution, we find almost 2% of the 90th percentile or more of PRSBMI score show phenotypic normal weight (18.5 > BMI > 25) category (Supplementary Figure S3). Conversely, below the 10th percentile, 2.3% of individuals were considered obese, and 0.1% were extremely obese. This distribution gradient of PRSBMI persisted at the 6-years follow-up, visit 2. Consistent with previous work, women experienced elevated levels of BMI than did men at both visit 1 and visit 2 and herein show a trend in BMI (kg/m2) by PRSBMI, percentile for sex, which remains fairly consistent across visits.
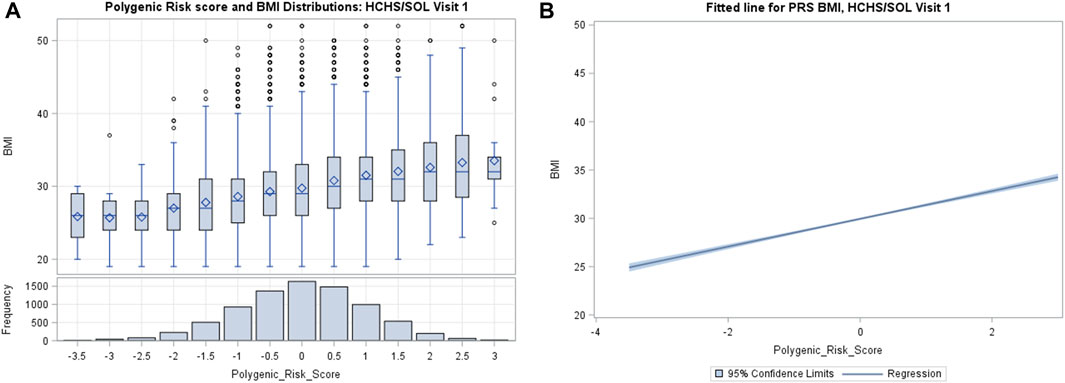
FIGURE 1. (A) Distribution of weighted polygenic risk scores for body mass index (PRSBMI) in participants from Hispanic Community Health Study/Study of Latinos (HCHS/SOL) visit 1 (2008–2011). (B) The fitted line is the regression of BMI and PRSBMI adjusted for top five principal components, age, and sex.
Table 2 summarizes our evaluation of PRS performance by the variance explained (adjusted R2 of BMI and waist-to-hip ratio). We found that the PRSBMI was well calibrated as evidenced by the 10.37% of variance explained in base model for BMI. Even though the full model choice was based on a continuous measure of BMI, the inferential model fit for waist-to-hip ratio performance was robust overall. However, the waist-to-hip measure is not a BMI-adjusted measure and should be interpreted as unadjusted. All outcome models fit, improved with the inclusion of interaction terms (age at immigration; healthy diet). Further evaluation of calibration, discrimination, and fit for outcome measures including dichotomous obesity to evaluate PRS utility can be found in the Supplemental Materials.
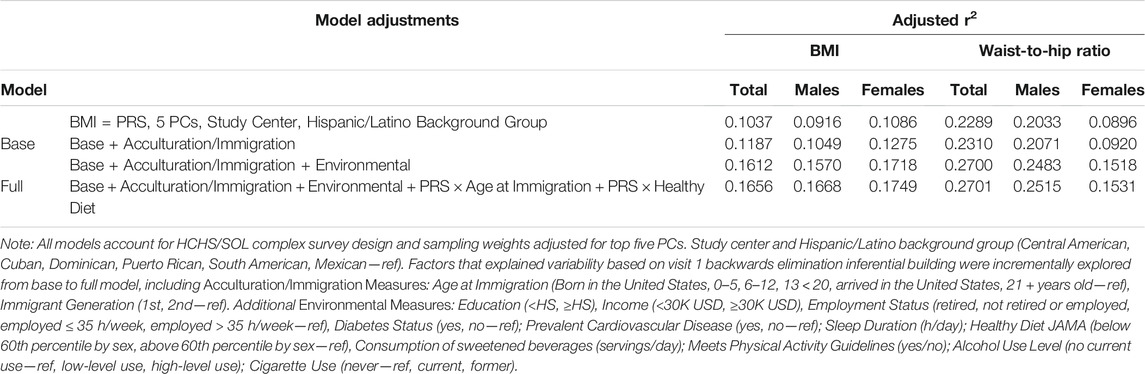
TABLE 2. Model fit for body mass index (BMI), waist-to-hip ratio, polygenic risk scores (PRSs), and their interactions with acculturation- and immigration-related variables in the up to 8,109 HCHS/SOL adults aged 20–76 years at visit 1 (2008–2011).
Next, we examined G×E among our initial model covariates (Supplementary Table S3). We find significant acculturation- and immigration-related interactions based on visit 1 BMI and PRSBMI for age at immigration (US-born p < 0.1896, 0–5: p < 0.0022; 6–12: p < 0.728; 13–20: p < 0.2067) and the SASH Language Use subscale (p < 0.0357). There were other significant G×E interactions as well (e.g., healthy diet p < 0.0095; marital status, p < 0.0076; and sleep duration, p < 0.0219). We used these significant interactions to inform our model choice for a full model that included additional types of covariates and multiple interactions. We then leveraged visit 2 follow-up of the HCHS/SOL cohort to examine the stability of these interactions between the two time periods and observe similar effects reassessed 6 years after (Supplementary Table S3). We do not have dietary data at visit 2 and therefore could not examine the interaction between health diet and PRS-BMI longitudinally.
Our full model, which included significant predictors and interaction terms for visit 1, are presented in Tables 3, 4 (base model and fully adjusted model including PRSBMI interaction terms). Consistent with our hypothesis that acculturation interactions would exacerbate the observed BMI (e.g., have a positive sign) and be significant, we observed significant interactions in both age at immigration and healthy diet (top 40th percentile). The validated subscale measures of SASH Social & Ethnic Relations and Language Use Subscales, as well as marital status, were not significant in our final multivariate model and therefore were excluded from our full model as well as those interaction terms.
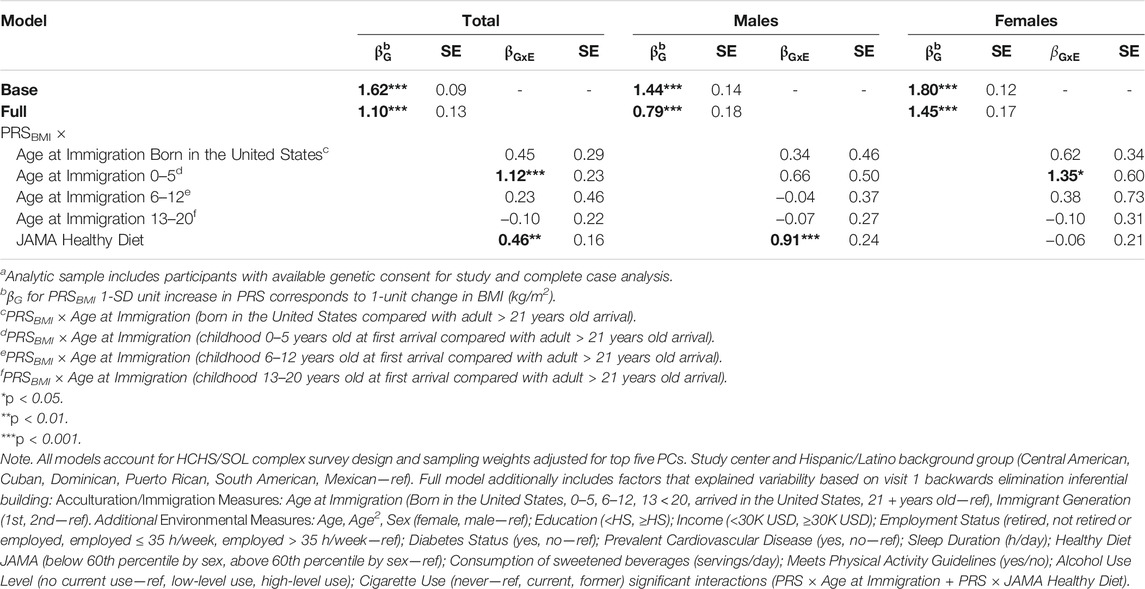
TABLE 3. Polygenic risk score (PRS)—acculturation and environmental interactions for obesity (body mass index (BMI)) among HCHS/SOL participants for visit 1 (2008–2011) n = 8,109a, total and sex-stratified.
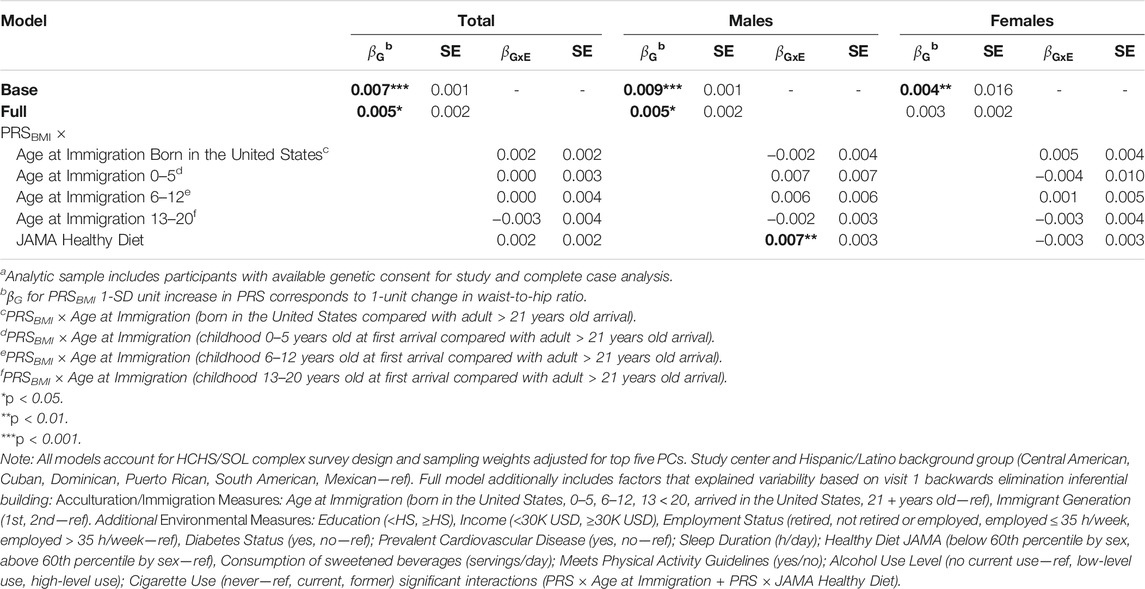
TABLE 4. Polygenic risk score (PRS)—acculturation and environmental interactions for obesity (waist-to-hip Ratio) among HCHS/SOL participants for visit 1 (2008–2011) n = 8,109a, total and sex-stratified.
Based on our full model, a 1-SD increase in PRSBMI corresponds with a 1.10 kg/m2 increase in BMI after adjusting for top five PCs of ancestry, study center, and Hispanic/Latino background group as well as acculturation and other environmental variables that explained a 6% increase in the variation explained in our model (Table 3, BMI). Due to the presence of interaction terms, we can interpret this PRSBMI estimate as conditional on the different values of age at immigration (compared with adult arrival) and JAMA healthy diet (bottom 60th percentile diet for sex compared with top 40th percentile). This elevated risk of BMI by 1-SD increase in PRSBMI increase and with a larger PRSBMI-related increase in BMI for women 1.45 kg/m2 compared with men 0.79 kg/m2 in sex-stratified analysis (full model). Figure 2 illustrates that at low values of PRSBMI, for example, we observe that adult arrival age at immigration group, for both males and females, has a higher BMI. However, at higher values of PRSBMI, adult arrival age at immigration has a lower BMI, effectively crossing over. Most notably, the interaction terms in the stratified models are only significant for men and only for diet, suggesting that these differences may further drive the significance in the full total model. These effect plots show interaction effects (Figure 3) by PRSBMI on BMI wherein the effect of the G×E value on BMI depends on PRSBMI, with the exception of healthy diet in females, which has parallel lines and no significant interaction (p = 0.7938).
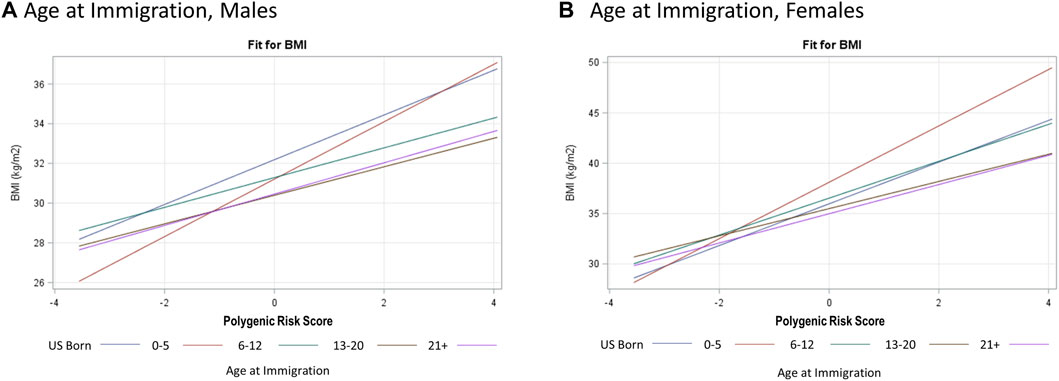
FIGURE 2. Hispanic Community Health Study (HCHS) visit 1 (2008–2011) sex-stratified effect plots showing the gene–environment interaction for PRSBMI × Age at Immigration (A, B) from fully adjusted model. The crossing lines represent interaction and shows that the effect of overall genetic risk on body mass index (BMI) is different for different values of age at immigration.
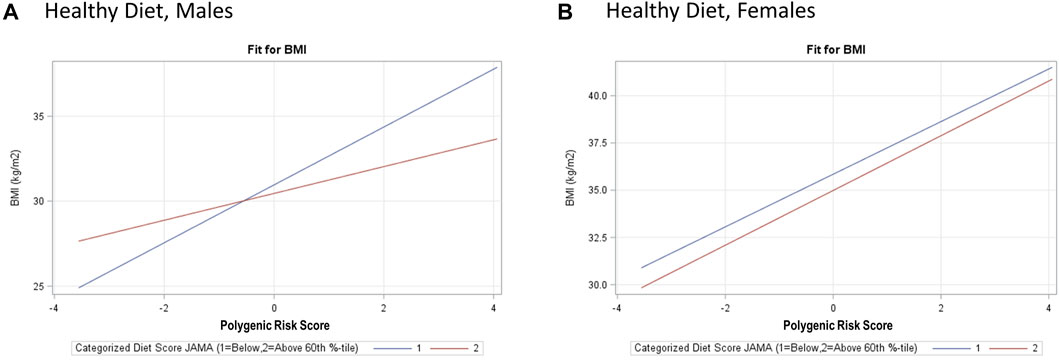
FIGURE 3. Hispanic Community Health Study (HCHS) visit 1 (2008–2011) sex-stratified effect plots showing the gene–environment interaction for and PRSBMI × Healthy Diet (A, B) from the fully adjusted model. The crossing lines represent interaction and show the effect of overall genetic risk on body mass index (BMI) is different for different values of healthy diet for males, with a notable lack of interaction for PRSBMI and healthy diet for females.
Finally, we conducted exploratory analyses examining the fully adjusted interaction model stratified by genetic ancestry background Hispanic/Latino group (Table 5). We compare the difference in each model with and without the PRSBMI main effect to evaluate the incremental difference. In models without the PRSBMI, there was more variation by genetic background group. However, adjusted r2 and effect improves with the inclusion of the PRSBMI both across background groups and in proportion of the variance explained. The PRSBMI estimates were comparable across the background Hispanic/Latino groups, while the PRS interaction terms had more variability by background. In particular, the South American background group had a larger standard error and beta estimate for PRS age at immigration US-born and age 0–5 compared with adult age at immigration, which is discordant with the estimates for other groups.
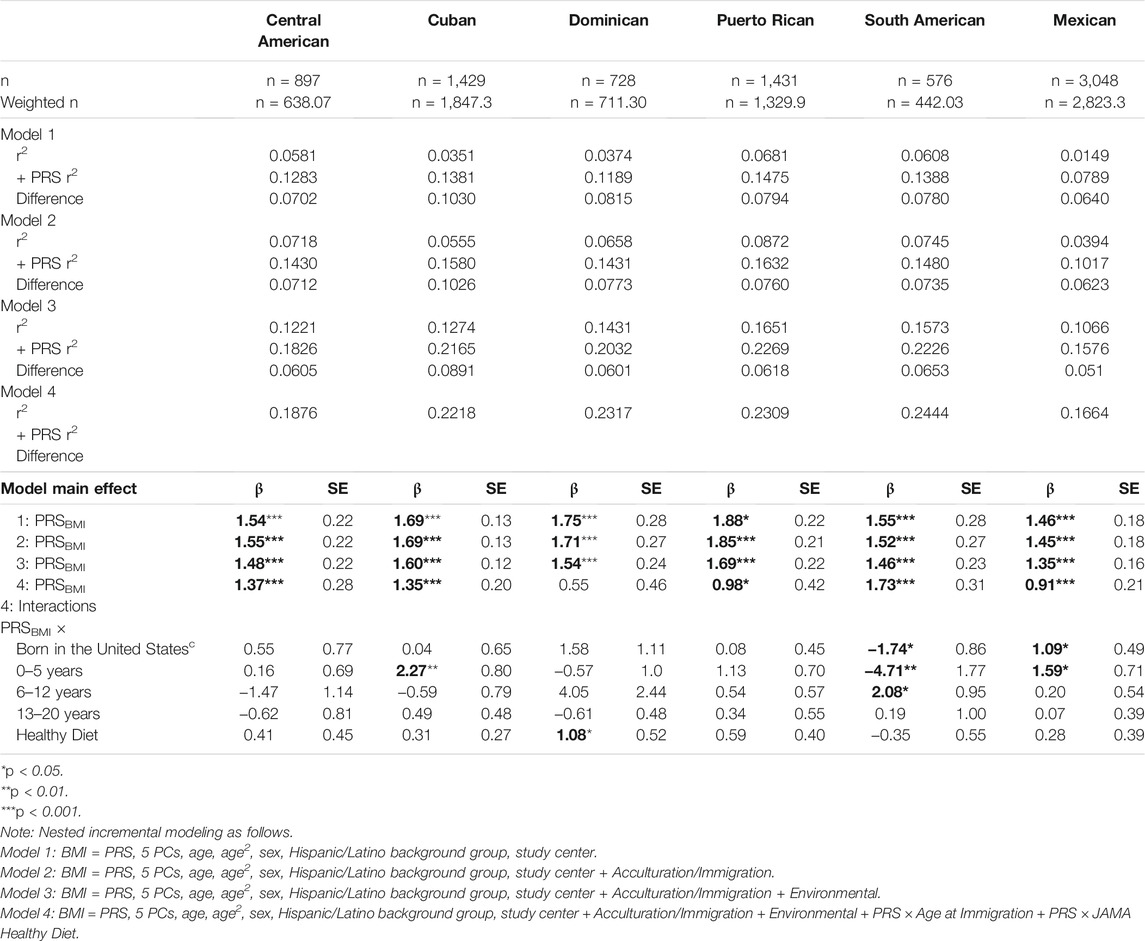
TABLE 5. Exploratory analysis of incremental validity of polygenic risk score (PRS)—acculturation and sociocultural interactions for obesity (BMI) among HCHS/SOL participants for visit 1 (2008–2011) n = 8,109, stratified by background Hispanic/Latino group.
Discussion
Among this ancestrally and socioculturally diverse sample of Hispanic/Latinos, we identify similar G×E interactions reported in other studies (Qi et al., 2014; Nakamura et al., 2016; Reddon et al., 2016; Rask-Andersen et al., 2017) and novel G×E interactions for age at immigration and healthy diet (full model). In other words, we observe that the PRSBMI effect on BMI was different for different values of acculturation and environmental factors alike. Overall, we found that an increase in polygenic risk is strongly associated with an increase in BMI (Khera et al., 2019), and despite the estimated PRSBMI, coefficients were moderately sensitive to model specification across all models and were directionally consistent, and statistically significance remained similar. When we modeled this association using visit 2 BMI data, we observed less of a genetic effect for elevated BMI. These models highlight that PRSBMI was not exclusively deterministic of BMI either cross-sectionally or longitudinally, and the etiology of obesity remains highly multifactorial in nature.
We identified differential sex-stratified effects by examining the same models among men and women separately. Herein, we find that dietary quality and acculturation interaction display sex-specific patterns with age at immigration significant for females only and diet significant for males only. This is consistent with previous PRS literature regarding sex differences in cancer outcomes (Fritsche et al., 2018; Meisner et al., 2020; Roberts et al., 2020) but has not been widely reported in the growing literature on PRSs for obesity (Khera et al., 2019; Torkamani and Topol 2019; Lee et al., 2021). Importantly, our study only captured the binary biological sex categories of male and female, so we are unable to contextualize these differences by broader gender-identity and gender-related processes, which we hypothesize may be underlying some of these observed differences. For example, we do not know to what degree the interaction of dietary score with genetic risk is because males have a different dietary pattern (Wardle et al., 2002) versus biological sex as a genetic modifier of nutrigenomic pathways.
In our study, we examined dietary interaction, which may represent both direct (immigration) and potentially indirect mechanisms of acculturation. We were able to examine some dietary measures that provide additional context, such as consumption of sugar-sweetened beverages, in which males on average consume 2.2 servings per day compared with 1.3 for females. This may help explain our findings that dietary patterns modify genetic risk of elevated BMI for men. In sensitivity analysis, we used an alternate dietary variable, the AHEI, modeled continuously and dichotomized similarly to the original variable for comparison. However, this alternative variable is not significant in the full model. Both dietary measures used National Cancer Institute (NCI) method for predicting usual intake based on 24-h recall, but they measured different dietary components. Recent work highlighting a significant G×E interaction for dietary fiber (Hüls et al., 2021) with polygenic risk for obesity among pan-European children may help explain these differences, as fiber is one of the four components of the Healthy Diet JAMA top 40th percentile variable. Additionally, the AHEI includes two variables already in the model, sugar-sweetened beverages and alcohol use, so the effect may be attenuated for this specific dietary measure compared with the original measure. Nonetheless, it would be important to confirm additional dietary interactions particularly for sex-specific models in future analyses.
In this work, we sought to elucidate the role of acculturation, immigration, sociodemographic lifestyle, and other environmental factors on genetic risk for elevated BMI. In our inferential model building, we ultimately excluded some of the measures that we had conceptually hypothesized would likely explain a portion variability of PRSBMI on BMI or perhaps serve as a better (validated) marker of acculturation or acculturative stress (e.g., the SASH Language Use, Social & Ethnic Relations Validated (Marin et al., 1987) Subscales, or Ethnic Identification score). This may be due in part to the fact that proxy measures of acculturation and immigration were stronger predictors of the same inter-related process and thus retained in our model building. For example, we used age at immigration, as it conceptually informed our model and was significant, and immigration measures represent a combination of broad acculturation trends and information on timing of first exposure to the United States. Yet such proxy measures may be less helpful at explaining life course changes or lifestyle interventions, as they remain fixed exposure, whereas other acculturation measures such as SASH Language Use subscale can be assessed in a more dynamic manner. In subsequent sensitivity analyses, we examined the effect on PRSBMI when we recapture or include alternate measures of acculturation (SASH-Language) that were otherwise not significant in our final modeling. We found directionally consistent results (i.e., smaller PRS coefficient with inclusion of interaction) but less significant interaction terms for these measures. Although conceptually we would expect that as time in the United States increased more variability would be captured by measures of increased acculturation, we did not measure this in our current study.
We reported age at immigration as a significant interaction term included in our full model. This measure aimed to approximate the interplay of the acculturative process as both duration and timing of immigration to the United States. Previous work reported significance differences in obesity outcomes by duration of exposure comparing migration before age 20 to later age at migration in adulthood (Roshania et al., 2008). To test our hypothesis that these differences may be attributable to in utero and early life exposures, we further categorized the less than 20 years, or early, age at migration as 0–5, 6–12, and 13–20 years old and found the age at immigration 0–5 group compared with age at immigration 21 or older had a significant interaction with PRSBMI. Importantly, compared with Roshania et al., where they examined recent immigrants (5 years mean duration of US residence), our target population sample resided in the United States longer (average 20.32 years). While our findings may also represent in utero or early life exposure mechanisms, we cannot conclusively detangle them from duration of exposure. There may also be confounding by the selection into migration (Murphy et al., 2017). Further work is needed to elucidate the sensitivity of this mechanism to critical periods of development, such as early childhood and obesity risk in migrant groups. A challenge in considering interpretation of PRSBMI is the role of lifestyle and other environmental factors. This wide array of interaction also shows that there is potential modification of the genetic risk modeled by PRSBMI by many different factors and highlight the importance of these environmental conditions for optimizing PRSBMI utility. Recent work has used electronic health records to identify risk factors for polygenic risk and prediction of prevalent disease status (Dashti et al., 2019). This type of integration of individual biologic data with the social determinants of health information as part of electronic health records is growing in practice could greatly inform what are the future clinical applications of PRSs (Gottlieb et al., 2013; Cantor and Thorpe 2018; Chen et al., 2020). Any consideration of risk for obesity should also consider environmental components that contribute to that risk. This study may help inform the characterization and advancement towards mature polygenic and environmental risk scores for obesity with clinical applications.
Our study is not without limitations. We could potentially have overfitting in our model (Mak et al., 2018) due to any overlap between the discovery data and the shrinkage applied to the GWAS effect size based on HCHS/SOL data, but it is unlikely given the different data sources. The study design involves complex sampling, and therefore, we utilized complex survey methodology in our analysis. However, the framework for model evaluation and building are less well established compared with non-survey methods and may have resulted in the introduction of additional Type 1 error (Hahs-Vaughn et al., 2011). We did employ a domain analysis that extended the standard errors to include the study target population (n = 16,415) in our estimations. Additionally, the purpose of this analysis was not strictly risk prediction but to illustrate the ability of polygenic risk prediction at informing G×E interaction modeling.
In order to assess the genetic information, we restricted our sample to only those who consented to share genetic data and both visit 1 and reconsented at visit 2. We limited our analysis to complete cases and may therefore have lost some of the representativeness of our data or are missing not at random; however, all standard error estimates presented are calculated, adjusting for the full target population using complex survey methodology. In this diverse sample of Hispanic/Latinos, we found a PRSBMI, derived from a large European ancestry GWAS explained a considerable portion of variability in BMI, with only subtle differences in distributions by Hispanic/Latino ancestry groupings (Table 5). The discovery data (Yengo et al., 2018) and target data (HCHS/SOL) were derived from different populations, indicating that there may be some degree of transportability of PRSBMI for BMI across these two populations. However, we cannot rule out that some of the observed interactions may be due to having an imperfect estimation of genetic effect relation to a weaker instrument based on European-weighted ancestry. While the predominantly European ancestry effect sizes were used as weights in the PRS calculation used herein, other weights were explored as part of our preliminary work (not reported). For example, we leveraged Hispanic/Latino effect sizes provided by the investigators of the Hispanic/Latino Anthropometry Consortium (Young et al., 2018), but these yielded PRS that explained less variance in BMI than when European-ancestry weights were used. We cannot rule out that the PRS measure is more sensitive to sample size compared with ancestry, as the difference is order of magnitude for the European-derived weights (Yengo et al., 2018). This highlights the difficulty of parsing a multifactorial etiology (e.g., genetic, environmental, and G×E) when the best practices for estimating trans-ancestral or sub-population-specific PRS—especially in light of their smaller available sample size—are still being developed.
Our model fit performed well for a model examining a complex disease such as obesity. Notably, we found the combination of predictors of genetic risk and environmental interactions to include two statistically significant interactions with PRSBMI. Although these findings are exploratory, taken together, they warrant future research and endeavors designed to better understand and model risk between the genetic architecture of obesity and environmental factors broadly speaking. Furthermore, future studies should consider ways to model gender and sex differences, as well as adopt a longitudinal approach to better account for age-related BMI changes. Our preliminary observation of G×E interactions across a 6-years time period implies that PRSBMI and environmental factors (either static or time-varying) should be jointly considered when considering the obesity prevention or counseling effects within the realm of precision medicine.
Data Availability Statement
The data presented in the Hispanic Community Health Study/Study of Latinos (HCHS/SOL) are deposited in dbGaP repository, accession number, phs000810.v1.p1. Additionally, the Hispanic Community Health Study/Study of Latinos (HCHS/SOL) data used in this article are publicly available following an approved article proposal. The investigators’ website can be found here: http://www.cscc.unc.edu/hchs/.
Ethics Statement
The studies involving human participants were reviewed and approved by UNC Coordinating Center. The patients/participants provided their written informed consent to participate in this study.
Author Contributions
CM derived the model and the computational framework and analyzed the data. VB, MG, KN, and CD carried out the PRS estimation. HB and CR assisted with the analysis and numerical calculations. KP aided in further developing the theoretical framework. CM and LF-R wrote the manuscript with input from all authors HB,CR, CI, JC, LG, AP, GW, and KP. GW extended the analysis to include ancestry with input from LF-R. All authors discussed results and commented on manuscript. LF-R conceived the study and LF-R and CM were in charge of overall direction and planning.
Funding
The Hispanic Community Health Study/Study of Latinos is a collaborative study supported by contracts from the National Heart, Lung, and Blood Institute (NHLBI) to the University of North Carolina (HHSN268201300001I/N01-HC-65233), University of Miami (HHSN268201300004I/N01-HC-65234), Albert Einstein College of Medicine (HHSN268201300002I/N01-HC-65235), University of Illinois at Chicago (HHSN268201300003I/N01-HC-65236 Northwestern Univ), and San Diego State University (HHSN268201300005I/N01-HC-65237). The following institutes/centers/offices have contributed to the HCHS/SOL through a transfer of funds to the NHLBI: National Institute on Minority Health and Health Disparities, National Institute on Deafness and Other Communication Disorders, National Institute of Dental and Craniofacial Research, National Institute of Diabetes and Digestive and Kidney Diseases, National Institute of Neurological Disorders and Stroke, and NIH Institution-Office of Dietary Supplements. The Genetic Analysis Center at the University of Washington was supported by NHLBI and NIDCR contracts (HHSN268201300005C AM03 and MOD03).
Conflict of Interest
The authors declare that the research was conducted in the absence of any commercial or financial relationships that could be construed as a potential conflict of interest.
Publisher’s Note
All claims expressed in this article are solely those of the authors and do not necessarily represent those of their affiliated organizations, or those of the publisher, the editors and the reviewers. Any product that may be evaluated in this article, or claim that may be made by its manufacturer, is not guaranteed or endorsed by the publisher.
Acknowledgments
The authors thank the staff and participants of HCHS/SOL for their important contributions. Investigators website http://www.cscc.unc.edu/hchs.
Supplementary Material
The Supplementary Material for this article can be found online at: https://www.frontiersin.org/articles/10.3389/fgene.2021.720750/full#supplementary-material
Supplementary Figure 1 | Study Inclusion/Exclusion Flow Chart
Supplementary Figure 2 | Three-Stage Inferential Model Building Diagram.
References
Ahmad, S., Varga, T. V., and Franks, P. W. (2013). Gene × Environment Interactions in Obesity: The State of the Evidence. Hum. Hered. 75 (2–4), 106–115. doi:10.1159/000351070
Andresen, E. M., Malmgren, J. A., Carter, W. B., and Patrick, D. L. (1994). Screening for Depression in Well Older Adults: Evaluation of a Short Form of the CES-D. Am. J. Prev. Med. 10 (2), 77–84. doi:10.1016/s0749-3797(18)30622-6
Barcenas, C. H., Wilkinson, A. V., Strom, S. S., Cao, Y., Saunders, K. C., Mahabir, S., et al. (2007). Birthplace, Years of Residence in the United States, and Obesity Among Mexican-American Adults. Obes. (Silver Spring Md.) 15 (4), 1043–1052. doi:10.1038/oby.2007.537
Ben-Shlomo, Y., and Kuh, D. (2002). A Life Course Approach to Chronic Disease Epidemiology: Conceptual Models, Empirical Challenges and Interdisciplinary Perspectives. Int. J. Epidemiol. 31 (2), 285–293. doi:10.1093/ije/31.2.285
Berry, J. W. (2004). Psychology of Group Relations: Cultural and Social Dimensions. Aviation, Space Environ. Med. 75 (7 Suppl. l), C52–C57.
Boardman, J. D., Domingue, B. W., Blalock, C. L., Haberstick, B. C., and McQueen, M. B. (2014). Is the Gene-Environment Interaction Paradigm Relevant to Genome-wide Studies? the Case of Education and Body Mass Index. Demography 51 (1), 119–139. doi:10.1007/s13524-013-0259-4
Cantor, M. N., and Thorpe, L. (2018). Integrating Data on Social Determinants of Health into Electronic Health Records. Health Aff. (Project Hope) 37 (4), 585–590. doi:10.1377/hlthaff.2017.1252
Carter-Pokras, O., and Bethune, L. (2009). Defining and Measuring Acculturation: A Systematic Review of Public Health Studies with Hispanic Populations in the United States. A Commentary on Thomson and Hoffman-Goetz. Soc. Sci. Med. 69 (7), 992–995. discussion 999-1001. doi:10.1016/j.socscimed.2009.06.042
Chen, M., Tan, X., and Padman, R. (2020). Social Determinants of Health in Electronic Health Records and Their Impact on Analysis and Risk Prediction: A Systematic Review. J. Am. Med. Inform. Assoc. JAMIA 27 (11), 1764–1773. doi:10.1093/jamia/ocaa143
Chiuve, S. E., Fung, T. T., Rimm, E. B., Hu, F. B, McCullough, M. L., Wang, M, et al. (2012). Alternative Dietary Indices Both Strongly Predict Risk of Chronic Disease. J. Nutr. 142 (6), 1009–1018. doi:10.3945/jn.111.157222
Choquet, H., and Meyre, D. (2011). Molecular Basis of Obesity: Current Status and Future Prospects. Curr. Genomics 12 (3), 154–168. doi:10.2174/138920211795677921
Chrisman, M., Chow, W-H., Daniel, C. R., Wu, X., and Zhao, H. (2017). Associations between Language Acculturation, Age of Immigration, and Obesity in the Mexican American Mano A Mano Cohort. Obes. Res. Clin. Pract. 11 (5), 544–557. doi:10.1016/j.orcp.2017.03.005
Conomos, M. P., Laurie, C. A., Stilp, A. M., Gogarten, S. M., McHugh, C. P., Nelson, S. C., et al. (2016). Genetic Diversity and Association Studies in US Hispanic/Latino Populations: Applications in the Hispanic Community Health Study/Study of Latinos. Am. J. Hum. Genet. 98 (1), 165–184. doi:10.1016/j.ajhg.2015.12.001
Dashti, H. S., Redline, S., and Saxena., R. (2019). Polygenic Risk Score Identifies Associations between Sleep Duration and Diseases Determined from an Electronic Medical Record Biobank. Sleep 42 (3), zsy247. doi:10.1093/sleep/zsy247
Daviglus, M. L., Talavera, G. A., Avilés-Santa, M. L., Allison, M., Cai, J., Criqui, M. H., Gellman, M., et al. (2012). Prevalence of Major Cardiovascular Risk Factors and Cardiovascular Diseases Among Hispanic/Latino Individuals of Diverse Backgrounds in the United States. JAMA 308 (17), 1775–1784. doi:10.1001/jama.2012.14517
Duffey, K. J., Gordon-Larsen, P., Ayala, G. X., and Popkin, B. M. (2008). Birthplace Is Associated with More Adverse Dietary Profiles for US-Born Than for Foreign-Born Latino Adults. J. Nutr. 138 (12), 2428–2435. doi:10.3945/jn.108.097105
Dunkler, D., Plischke, M., Leffondré, K., and Heinze, G. (2014). Augmented Backward Elimination: A Pragmatic and Purposeful Way to Develop Statistical Models. Plos One 9 (11), e113677. doi:10.1371/journal.pone.0113677
Fesinmeyer, M. D., North, K. E., Ritchie, M. D., Lim, U., Franceschini, N., Wilkens, L. R., et al. (2013). Genetic Risk Factors for Body Mass Index and Obesity in an Ethnically Diverse Population: Results from the Population Architecture Using Genomics and Epidemiology (PAGE) Study. Obes. (Silver Spring Md) 21 (4), 835–846. doi:10.1002/oby.20268
Flegal, K. M., Carroll, M. D., Kit, B. K., and Ogden, C. L. (2012). Prevalence of Obesity and Trends in the Distribution of Body Mass Index Among US Adults, 1999-2010. JAMA 307 (5), 491. doi:10.1001/jama.2012.39
Flowers, E., Froelicher, E. S., and Aouizerat, B. E. (2012). Gene-Environment Interactions in Cardiovascular Disease. Eur. J. Cardiovasc. Nurs. 11 (4), 472–478. doi:10.1016/j.ejcnurse.2011.06.001
Fritsche, L. G., Gruber, S. B., Wu, Z., Schmidt, E. M., Zawistowski, M., Moser, S. E., et al. (2018). Association of Polygenic Risk Scores for Multiple Cancers in a Phenome-wide Study: Results from the Michigan Genomics Initiative. Am. J. Hum. Genet. 102 (6), 1048–1061. doi:10.1016/j.ajhg.2018.04.001
Gong, J., Nishimura, K. K., Fernandez-Rhodes, L., Haessler, J., Bien, S., Graff, M., et al. (2018). Trans-Ethnic Analysis of Metabochip Data Identifies Two New Loci Associated with BMI. Int. J. Obes. 42 (3), 384–390. doi:10.1038/ijo.2017.304
Gordon-Larsen, P., Ward, D. S., and Popkin, B. M.National Longitudinal Study of Adolescent Health (2003). Acculturation and Overweight-Related Behaviors Among Hispanic Immigrants to the US: The National Longitudinal Study of Adolescent Health. Soc. Sci. Med. 57 (11), 2023–2034. doi:10.1016/s0277-9536(03)00072-8
Gottlieb, L., Sandel, M., and Adler, N. E. (2013). Collecting and Applying Data on Social Determinants of Health in Health Care Settings. JAMA Intern. Med. 173 (11), 1017–1020. doi:10.1001/jamainternmed.2013.560
Grinde, K. E., Qi, Q., Thornton, T. A., Shadyab, A. H., Chan, K. H. K., Reiner, A. P., et al. (2019). Generalizing Polygenic Risk Scores from Europeans to Hispanics/Latinos. Genet. Epidemiol. 43 (1), 50–62. doi:10.1002/gepi.22166
Guendelman, S., Fernandez, A., Thornton, D., and Brindis, C. (2011). Birthplace, Language Use, and Body Size Among Mexican American Women and Men: Findings from the National Health and Nutrition Examination Survey (NHANES) 2001-2006. J. Health Care Poor Underserved 22 (2), 590–605. doi:10.1353/hpu.2011.0045
GWAS Catalog (2020). The NHGRI-EBI Catalog of Human Genome-wide Association Studies. Available at: https://www.ebi.ac.uk/gwas/search?query=obesity (Accessed December 21, 2020).
Hahs-Vaughn, D. L., McWayne, C. M., Bulotsky-Shearer, R. J., Wen, X., and Faria, A-M. (2011). Methodological Considerations in Using Complex Survey Data: An Applied Example with the Head Start Family and Child Experiences Survey. Eval. Rev. 35 (3), 269–303. doi:10.1177/0193841x11412071
Hales, C. M., Carroll, M. D., Fryar, C. D., and Ogden, C. L. (2020). “Prevalence of Obesity and Severe Obesity Among Adults: United States, 2017–2018.” NCHS Data Brief, No 360. Hyattsville, MD: National Center for Health Statistics. Available at:.
Heeringa, S. G., West, B. T., and Berglund, P. A. (2017). Applied Survey Data Analysis. Boca Raton, FL: CRC Press Taylor & Francis Group.
Heinze, G., Wallisch, C., and Dunkler, D. (2018). Variable Selection – A Review and Recommendations for the Practicing Statistician. Biometrical J. 60 (3), 431–449. doi:10.1002/bimj.201700067
Hüls, A., Wright, M. N., Bogl, L. H., Kaprio, J., Lissner, L., Molnár, D., et al. (2021). Polygenic Risk for Obesity and its Interaction with Lifestyle and Sociodemographic Factors in European Children and Adolescents. Int. J. Obes. 45, 1321–1330. doi:10.1038/s41366-021-00795-5
Kanter, R., and Caballero, B. (2012). Global Gender Disparities in Obesity: A Review. Adv. Nutr. (Bethesda, Md 3 (4), 491–498. doi:10.3945/an.112.002063
Khan, L. K., Sobal, J., and Martorell, R. (1997). “Acculturation, Socioeconomic Status, and Obesity in Mexican Americans, Cuban Americans, and Puerto Ricans.” International Journal Of Obesity and Related Metabolic Disorders. J. Int. Assoc. Study Obes. 21 (2), 91–96. doi:10.1038/sj.ijo.0800367
Khera, A. V., Chaffin, M., Wade, K. H., Zahid, S., Joseph, B., Xia, R., et al. (2019). Polygenic Prediction of Weight and Obesity Trajectories from Birth to Adulthood. Cell 177 (3), 587–596.e9. doi:10.1016/j.cell.2019.03.028
Lavange, L. M., Kalsbeek, W. D., Sorlie, P. D., Avilés-Santa, L. M., Kaplan, R. C., Barnhart, J., et al. (2010). Sample Design and Cohort Selection in the Hispanic Community Health Study/Study of Latinos. Ann. Epidemiol. 20 (8), 642–649. doi:10.1016/j.annepidem.2010.05.006
Lee, W-J., Lim, J. E., Jung, H. U., Kang, J-O., Park, T., Won, S., et al. (2021). Analysis of the Interaction between Polygenic Risk Score and Calorie Intake in Obesity in the Korean Population. Lifestyle Genomics 14 (1), 20–29. doi:10.1159/000511333
Link, J. C., and Reue, K. (2017). Genetic Basis for Sex Differences in Obesity and Lipid Metabolism. Annu. Rev. Nutr. 37 (August), 225–245. doi:10.1146/annurev-nutr-071816-064827
Liu, J-H., Chu, Y. H., Frongillo, E. A., and Probst, J. C. (2012). Generation and Acculturation Status Are Associated with Dietary Intake and Body Weight in Mexican American Adolescents. J. Nutr. 142 (2), 298–305. doi:10.3945/jn.111.145516
Liu, K., Daviglus, M. L., Loria, C. M., Colangelo, L. A., Spring, B., Moller, A. C., et al. (2012). Healthy Lifestyle through Young Adulthood and the Presence of Low Cardiovascular Disease Risk Profile in Middle Age: The Coronary Artery Risk Development in (Young) Adults (CARDIA) Study. Circulation 125 (8), 996–1004. doi:10.1161/CIRCULATIONAHA.111.060681
Locke, A. E., Kahali, B., Berndt, S. I., Justice, A. E., Pers, T. H., Day, F. R., et al. (2015). Genetic Studies of Body Mass Index Yield New Insights for Obesity Biology. Nature 518 (7538), 197–206. doi:10.1038/nature14177
Mak, T. S. H., and Porsch, R. M. W. C. (2018). Polygenic Scores for UK Biobank Scale Data. BioRxiv, 252270. October. doi:10.1101/252270
Marin, G., Sabogal, F., and Marin, B. V. R. E. J. (1987). Development of a Short Acculturation Scale for Hispanics. Hispanic J. Behav. Sci. 9 (2), 183–205. doi:10.1177/07399863870092005
Martínez, A. D. (2013). Reconsidering Acculturation in Dietary Change Research Among Latino Immigrants: Challenging the Preconditions of US Migration. Ethn. Health 18 (2), 115–135. doi:10.1080/13557858.2012.698254
Meisner, A., Kundu, P., Zhang, Y. D., Lan, L. V., Kim, S., Ghandwani, D., et al. (2020). Combined Utility of 25 Disease and Risk Factor Polygenic Risk Scores for Stratifying Risk of All-Cause Mortality. Am. J. Hum. Genet. 107 (3), 418–431. doi:10.1016/j.ajhg.2020.07.002
Murillo, R., Albrecht, S. S., Daviglus, M. L., and Kershaw, K. N. (2015). The Role of Physical Activity and Sedentary Behaviors in Explaining the Association between Acculturation and Obesity Among Mexican-American Adults. Am. J. Health Promot. AJHP 30 (1), 50–57. doi:10.4278/ajhp.140128-QUAN-49
Murphy, M., Robertson, W., and Oyebode, O. (2017). Obesity in International Migrant Populations. Curr. Obes. Rep. 6 (3), 314–323. doi:10.1007/s13679-017-0274-7
Nagpal, S., Gibson, G., and Marigorta, U. M. (2018). Pervasive Modulation of Obesity Risk by the Environment and Genomic Background. Genes 9 (8), 411. doi:10.3390/genes9080411
Nakamura, S., Narimatsu, H., Sato, H., Sho, R., Otani, K., Kawasaki, R., et al. (2016). Gene-Environment Interactions in Obesity: Implication for Future Applications in Preventive Medicine. J. Hum. Genet. 61 (4), 317–322. doi:10.1038/jhg.2015.148
National Heart, LungBlood Institute (2013). Managing Overweight and Obesity in Adults: Systematic Evidence Review from the Obesity Expert Panel. Rockville, MD: Agency for Healthcare Research and Quality.
Ogden, C. L., Yanovski, S. Z., Carroll, M. D., and Flegal, K. M. (2007). The Epidemiology of Obesity. Gastroenterology 132 (6), 2087–2102. doi:10.1053/j.gastro.2007.03.052
Ogden, C. L., Carroll, M. D., Kit, B. K., and Flegal, K. M. (2014). Prevalence of Childhood and Adult Obesity in the United States, 2011-2012. JAMA 311 (8), 806–814. doi:10.1001/jama.2014.732
OMH (2020). Obesity and Hispanic Americans - the Office of Minority Health. Available at: (Accessed December 20, 2020).
Pérez-Escamilla, R., and Putnik, P. (2007). The Role of Acculturation in Nutrition, Lifestyle, and Incidence of Type 2 Diabetes Among Latinos. J. Nutr. 137 (4), 860–870. doi:10.1093/jn/137.4.860
Pfeffermann, D. (1996). The Use of Sampling Weights for Survey Data Analysis. Stat. Methods Med. Res. 5 (3), 239–261. doi:10.1177/096228029600500303
Qi, Q., Chu, A. Y., Kang, J. H., Jensen, M. K., Curhan, G. C., Pasquale, L. R., et al. (2012). Sugar-Sweetened Beverages and Genetic Risk of Obesity. New Engl. J. Med. 367 (15), 1387–1396. doi:10.1056/NEJMoa1203039
Qi, Q., Chu, A. Y., Kang, J. H., Huang, J., Rose, L. M., Jensen, M. K., et al. (2014). Fried Food Consumption, Genetic Risk, and Body Mass Index: Gene-Diet Interaction Analysis in Three US Cohort Studies. BMJ (Clinical Res. Ed.) 348 (March), g1610. doi:10.1136/bmj.g1610
Qi, Q., Downer, M. K., Kilpeläinen, T. O., Taal, H. R., Barton, S. J., Ntalla, I., et al. (2015). Dietary Intake, FTO Genetic Variants, and Adiposity: A Combined Analysis of over 16,000 Children and Adolescents. Diabetes 64 (7), 2467–2476. doi:10.2337/db14-1629
Rask-Andersen, M., Karlsson, T., Weronica, E. Ek., and Johansson, Å. (2017). Gene-Environment Interaction Study for BMI Reveals Interactions between Genetic Factors and Physical Activity, Alcohol Consumption and Socioeconomic Status. PLOS Genet. 13 (9), e1006977. doi:10.1371/journal.pgen.1006977
Reddon, H., Guéant, J-L., and Meyre, D. (2016). “The Importance of Gene–Environment Interactions in Human Obesity. Clin. Sci. 130 (18), 1571–1597. doi:10.1042/CS20160221
Roberts, M. R., Sordillo, J. E., Kraft, P., and Asgari, M. M. (2020). Sex-Stratified Polygenic Risk Score Identifies Individuals at Increased Risk of Basal Cell Carcinoma. J. Invest. Dermatol. 140 (5), 971–975. doi:10.1016/j.jid.2019.09.020
Roshania, R., Narayan, K. M., and Oza-Frank, R. (2008). Age at Arrival and Risk of Obesity Among US Immigrants. Obes. (Silver Spring Md 16 (12), 2669–2675. doi:10.1038/oby.2008.425
Rumbaut, R. G. (2005). Sites of Belonging: Acculturation, Discrimination, and Ethnic Identity Among Children of Immigrants. SSRN Scholarly Paper ID 1882684. Rochester, NY: Social Science Research Network. Available at: https://papers.ssrn.com/abstract=1882684.
Sofer, T., Moon, J-Y., Isasi, C. R., Qi, Q., Shah, N. A., Kaplan, R. C., et al. (2018). Relationship of Genetic Determinants of Height with Cardiometabolic and Pulmonary Traits in the Hispanic Community Health Study/Study of Latinos. Int. J. Epidemiol. 47 (6), 2059–2069. doi:10.1093/ije/dyy177
Sorlie, P. D., Avilés-Santa, L. M., Wassertheil-Smoller, S., Kaplan, R. C., Daviglus, M. L., Giachello, A. L., et al. (2010). Design and Implementation of the Hispanic Community Health Study/Study of Latinos. Ann. Epidemiol. 20 (8), 629–641. doi:10.1016/j.annepidem.2010.03.015
Stevens, G. (1999). Age at Immigration and Second Language Proficiency Among Foreign-Born Adults. Lang. Soc. 28 (4), 555–578. doi:10.1017/s0047404599004030
Sundquist, J., and Winkleby, M. (2000). Country of Birth, Acculturation Status and Abdominal Obesity in a National Sample of Mexican-American Women and Men. Int. J. Epidemiol. 29 (3), 470–477. doi:10.1093/intjepid/29.3.470
Thomson, M. D., and Hoffman-Goetz., L. (2009). Defining and Measuring Acculturation: A Systematic Review of Public Health Studies with Hispanic Populations in the United States. Soc. Sci. Med. 69 (7), 983–991. doi:10.1016/j.socscimed.2009.05.011
Torkamani, A., and Topol, E. (2019). Polygenic Risk Scores Expand to Obesity. Cell 177 (3), 518–520. doi:10.1016/j.cell.2019.03.051
Tyrrell, J., Wood, A. R., Ames, R. M., Yaghootkar, H., Beaumont, R. N., Jones, S. E., et al. (2017). Gene-Obesogenic Environment Interactions in the UK Biobank Study. Int. J. Epidemiol. 46 (2), 559–575. doi:10.1093/ije/dyw337
Van Wieren, A. J., Roberts, M. B., Arellano, N., Feller, E. R., and Diaz, J. A. (2011). Acculturation and Cardiovascular Behaviors Among Latinos in California by Country/Region of Origin. J. Immigrant Minor. Health 13 (6), 975–981. doi:10.1007/s10903-011-9483-4
Vilhjálmsson, B. J., Yang, J., and Hilary, K. (2015). Finucane, Alexander Gusev, Sara Lindström, Stephan Ripke, Giulio Genovese, et al.Modeling Linkage Disequilibrium Increases Accuracy of Polygenic Risk Scores. Am. J. Hum. Genet. 97 (4), 576–592. doi:10.1016/j.ajhg.2015.09.001
Wallace, P. M., Pomery, E. A., Latimer, A. E., Martinez, J. L., and Salovey, P. (2010). A Review of Acculturation Measures and Their Utility in Studies Promoting Latino Health. Hispanic J. Behav. Sci. 32 (1), 37–54. doi:10.1177/0739986309352341
Wand, H., Lambert, S. A., Tamburro, C., Iacocca, M. A., O’Sullivan, J. W., Sillari, C., et al. (2021). Improving Reporting Standards for Polygenic Scores in Risk Prediction Studies. Nature 591 (7849), 211–219. doi:10.1038/s41586-021-03243-6
Wang, Y. C., McPherson, K., Marsh, T., Gortmaker, S. L., and Brown., M. (2011). Health and Economic Burden of the Projected Obesity Trends in the USA and the UK. Lancet (London, England) 378 (9793), 815–825. doi:10.1016/S0140-6736(11)60814-3
Wardle, J., Waller, J., and Jarvis, M. J. (2002). Sex Differences in the Association of Socioeconomic Status with Obesity. Am. J. Public Health 92 (8), 1299–1304. doi:10.2105/AJPH.92.8.1299
Yengo, L., Sidorenko, J., Kemper, K. E., Zheng, Z., Wood, A. R., Weedon, M. N., et al. (2018). Meta-Analysis of Genome-wide Association Studies for Height and Body Mass Index in ∼700000 Individuals of European Ancestry. Hum. Mol. Genet. 27 (20), 3641–3649. doi:10.1093/hmg/ddy271
Keywords: polygenic risk score, gene-environment interactions, Hispanic/Latino, acculturation, obesity
Citation: McArdle CE, Bokhari H, Rodell CC, Buchanan V, Preudhomme LK, Isasi CR, Graff M, North K, Gallo LC, Pirzada A, Daviglus ML, Wojcik G, Cai J, Perreira K and Fernandez-Rhodes L (2021) Findings from the Hispanic Community Health Study/Study of Latinos on the Importance of Sociocultural Environmental Interactors: Polygenic Risk Score-by-Immigration and Dietary Interactions. Front. Genet. 12:720750. doi: 10.3389/fgene.2021.720750
Received: 04 June 2021; Accepted: 08 September 2021;
Published: 06 December 2021.
Edited by:
Dana C. Crawford, Case Western Reserve University, United StatesReviewed by:
Paul B. Higgins, Letterkenny Institute of Technology, IrelandMark Z. Kos, The University of Texas Rio Grande Valley, United States
Copyright © 2021 McArdle, Bokhari, Rodell, Buchanan, Preudhomme, Isasi, Graff, North, Gallo, Pirzada, Daviglus, Wojcik, Cai, Perreira and Fernandez-Rhodes. This is an open-access article distributed under the terms of the Creative Commons Attribution License (CC BY). The use, distribution or reproduction in other forums is permitted, provided the original author(s) and the copyright owner(s) are credited and that the original publication in this journal is cited, in accordance with accepted academic practice. No use, distribution or reproduction is permitted which does not comply with these terms.
*Correspondence: Cristin E. McArdle, Y2VtNTk5N0Bwc3UuZWR1