- 1Department of Gastroenterology, The Second Hospital of Hebei Medical University, Shijiazhuang, China
- 2Department of Neurosurgery, The Second Hospital of Hebei Medical University, Shijiazhuang, China
Background: Ulcerative colitis (UC) is a chronic, complicated, inflammatory disease with an increasing incidence and prevalence worldwide. However, the intrinsic molecular mechanisms underlying the pathogenesis of UC have not yet been fully elucidated.
Methods: All UC datasets published in the GEO database were analyzed and summarized. Subsequently, the robust rank aggregation (RRA) method was used to identify differentially expressed genes (DEGs) between UC patients and controls. Gene functional annotation and PPI network analysis were performed to illustrate the potential functions of the DEGs. Some important functional modules from the protein-protein interaction (PPI) network were identified by molecular complex detection (MCODE), Gene Ontology (GO), and Kyoto Encyclopedia of Genes and Genomes (KEGG), and analyses were performed. The results of CytoHubba, a plug for integrated algorithm for biomolecular interaction networks combined with RRA analysis, were used to identify the hub genes. Finally, a mouse model of UC was established by dextran sulfate sodium salt (DSS) solution to verify the expression of hub genes.
Results: A total of 6 datasets met the inclusion criteria (GSE38713, GSE59071, GSE73661, GSE75214, GSE87466, GSE92415). The RRA integrated analysis revealed 208 significant DEGs (132 upregulated genes and 76 downregulated genes). After constructing the PPI network by MCODE plug, modules with the top three scores were listed. The CytoHubba app and RRA identified six hub genes: LCN2, CXCL1, MMP3, IDO1, MMP1, and S100A8. We found through enrichment analysis that these functional modules and hub genes were mainly related to cytokine secretion, immune response, and cancer progression. With the mouse model, we found that the expression of all six hub genes in the UC group was higher than that in the control group (P < 0.05).
Conclusion: The hub genes analyzed by the RRA method are highly reliable. These findings improve the understanding of the molecular mechanisms in UC pathogenesis.
Introduction
Ulcerative colitis (UC) is a chronic, complicated, inflammatory disease that affects the colonic mucosa and most commonly presents with abdominal pain, diarrhea, and blood in the stools. Pathological characteristics include relapsing and remitting mucosal inflammation, starting in the rectum and sigmoid colon and extending continuously to proximal segments of the colon or even the entire colon, which leads to permanent fibrosis and tissue damage (Ungaro et al., 2017). The incidence and prevalence of UC have been increasing worldwide. It usually has a long, chronic clinical course, and UC patients are at increased risk of colorectal cancer. Thus, UC has become one of the critical threats and challenges to human health (Kaplan and Ng, 2017; Ng et al., 2017).
At the present, the etiology of UC remains unclear. It is believed that multifactorial pathogenesis plays a role in the occurrence and development of UC, and the factors involved include environmental and psychological factors, dysregulated intestinal flora and immune responses, genetic predisposition, and epithelial barrier defects. The colonic epithelium facilitates host-microorganism interactions to control mucosal immunity, coordinate nutrient recycling and form a mucus barrier. Breakdown of the epithelial barrier is underlying in UC pathogenesis (Ungaro et al., 2017; Parikh et al., 2019). Routinely used indices in the clinical diagnosis and dynamic monitoring of UC include C reactive protein (CRP), erythrocyte sedimentation rate (ESR), and fecal calprotectin, but these lack sensitivity and specificity in differentiating between UC and functional gut disorders (Brookes et al., 2018). Therefore, it is of great importance to further understand the pathogenesis and regulation of UC at the molecular level, as well as the identification of key biomarkers for UC.
In recent years, several studies based on microarray technology have been published to identify effective biomarkers in UC (Cheng et al., 2019, 2020; Chen Y. et al., 2020; Shi et al., 2020; Cao et al., 2021). However, differences in measurement platforms, lab protocols, sample sizes, and operators render gene expression levels incomparable. Based on multiple microarray datasets, robust rank aggregation (RRA) is a method that integrates the results of differential expression analysis, expanding the sample size and reducing the influence of different microarray platforms and imbalanced sample sizes (Kolde et al., 2012). To date, RRA has not been applied in microarray studies of UC. Thus, this study involved a comprehensive evaluation of the published datasets. Based on the inclusion criteria, we screened and included six datasets and identified DEGs using RRA. A protein-protein interaction (PPI) network was constructed to analyze the hub genes, the gene modules, and the involved functions and pathways. Several additional biomarkers were identified that may contribute to the diagnosis of UC, thus providing potential therapeutic targets for patients.
Materials and Methods
Search Strategy for the UC Microarray Datasets
A total of 68 datasets were collected from the Gene Expression Omnibus (GEO) Database1 by systematic retrieval using the keywords: (“colitis, ulcerative”[MeSH Terms] OR ulcerative colitis [All Fields]) AND “Homo sapiens”[porgn] AND (“Expression profiling by array”[Filter] AND (“2010/01/01”[PDAT]: “2021/03/05”[PDAT])). The inclusion criteria were as follows: (1) a dataset sample size > 30; (2) the dataset included both cases and normal controls; (3) the sample source was “colon,” and (4) the differentially expressed genes (DEGs) with | logFC| > 1.5 and adjusted P < 0.05 were identified from the dataset (Figure 1).
Identification of DEGs in UC
We first downloaded the gene expression profiles from the GEO database for all the datasets included in the final analysis. The scaled expression values for each gene were averaged when multiple probes target the same gene. Second, PERL software was used to extract the matrix file, and the Limma package in R software was used to perform quantile normalization with the normalizeBetweenArrays function. Additionally, we identified the DEGs for each dataset with the criteria of | logFC| > 1.5 and adjusted P < 0.05 for comparison with the RRA analysis results.
RRA Integrated Analysis
In the RRA analysis, DEGs (both upregulated and downregulated) were sorted for each dataset and ranked according to their logFC using the Limma package of R software. Then, all the DEGs were scored according to the ranked list and aggregately analyzed using the RRA package of R software. The adjusted P-value in this method reflects the probability of the highly ranked genes in the datasets identified as DEGs. The criteria for the identification of DEGs were set as | logFC| > 1.5 and adjusted P < 0.05.
Functional Annotation
Gene Ontology (GO) is a community-based bioinformatics resource for annotating gene sets, which can be divided into three parts: biological process (BP), cellular component (CC), and molecular function (MF) (Dalmer and Clugston, 2019). The Kyoto Encyclopedia of Genes and Genomes (KEGG) is a database covering various biological signaling pathways. GO enrichment and KEGG pathway analysis provide essential perspectives for bioinformatics analysis. Enrichment analysis for DEGs with the criteria of adjusted P < 0.05 was performed using the Cluster Profile package of R software (Yu et al., 2012).
Protein-Protein Interaction (PPI) Network Analysis
For the obtained DEGs, the String database2 was used to construct the PPI network, with the parameter of confidence > 0.4. Visualization of the PPI network was performed by Cytoscape (v3.7.2) (Smoot et al., 2011), and molecular complex detection (MCODE) (a plugin in Cytoscape) (Bader and Hogue, 2003) was used to identify the functional modules. Essential genes were identified by the plugin of CytoHubba (Chin et al., 2014) and sorted by degree scores. The overlap of genes in the PPI network (degree score > 15) and the top 15 DEGs (upregulated or downregulated) in the RRA analysis were determined as hub genes.
Establishment of UC Model
All animal experiments were performed according to Institutional Animal Care and Use Committee (IACUC) guidelines and were approved by the Ethics Committee at Hebei Medical University.
Sixteen BALB/c mice (male, 6–8 weeks) were obtained from Vital River and were reared in a specific pathogen-free (SPF) environment. All mice were randomly divided into standard control and experimental groups. The mice in the regular control group were given normal drinking water. In contrast, the mice in the experimental group were given a 3.5% DSS solution (dextran sulfate sodium salt, MPbio, MW 36,000–50,000 Da, CA, United States) for 7 days continuously to induce acute colitis and to create the UC animal model. After modeling, all mice were given standard drinking water, kept for another 3 days, and then euthanized with CO2. The colon tissue from each mouse was collected. One part was stored in 4% paraformaldehyde (PFA). The other part was homogenized with TRIzol reagent (Invitrogen, Carlsbad, CA, United States), immediately frozen in liquid nitrogen, and stored at −80°C.
Real-Time Quantitative PCR (RT-qPCR)
Total RNA was extracted according to the manufacturer’s instructions. For examination of mRNA expression, the RNA was reverse transcribed into cDNA, followed by an examination of RT-qPCR using SYBR Mix (CWBIO, Beijing, China). β-actin was indicated as internal controls. All samples were examined in triplicate for each specific gene. The primer sequences for PCR are listed in Supplementary Table 1.
Pathological Examination
The distal colons of the mice were fixed with 4% PFA and embedded in paraffin. Tissue sections were stained with hematoxylin-eosin (HE). According to the scoring criteria by Dieleman et al. (1998), the intestinal damage level was evaluated under an optical microscope, as shown in Table 1. Three visual fields were randomly selected from each section, and the scores were averaged to determine the damage level of the colonic tissue.
Results
Characteristics of the Included Microarrays
According to the criteria above, a total of six datasets were included in the final analysis: GSE38713 (Planell et al., 2013), GSE59071 (Vanhove et al., 2015), GSE73661 (Arijs et al., 2018), GSE75214 (Vancamelbeke et al., 2017), GSE87466 (Li et al., 2018), and GSE92415. The flowchart of dataset retrieval, inclusion criteria, and exclusion criteria is shown in Figure 1. From the six datasets, a total of 532 cases of UC (including 46 inactive UC cases) were included in the experimental group, and 89 were included in the standard control group. The characteristics of the included microarray datasets are shown in Table 2.
Identification of DEGs in UC
First, the datasets were standardized to correct batch differences within the datasets (Supplementary Figure 1), showing that the homogeneity of the data met the requirements and could be included in the analysis. Then, the DEGs were identified in each dataset using the Limma package of R software, and the volcano maps are shown in Figure 2.
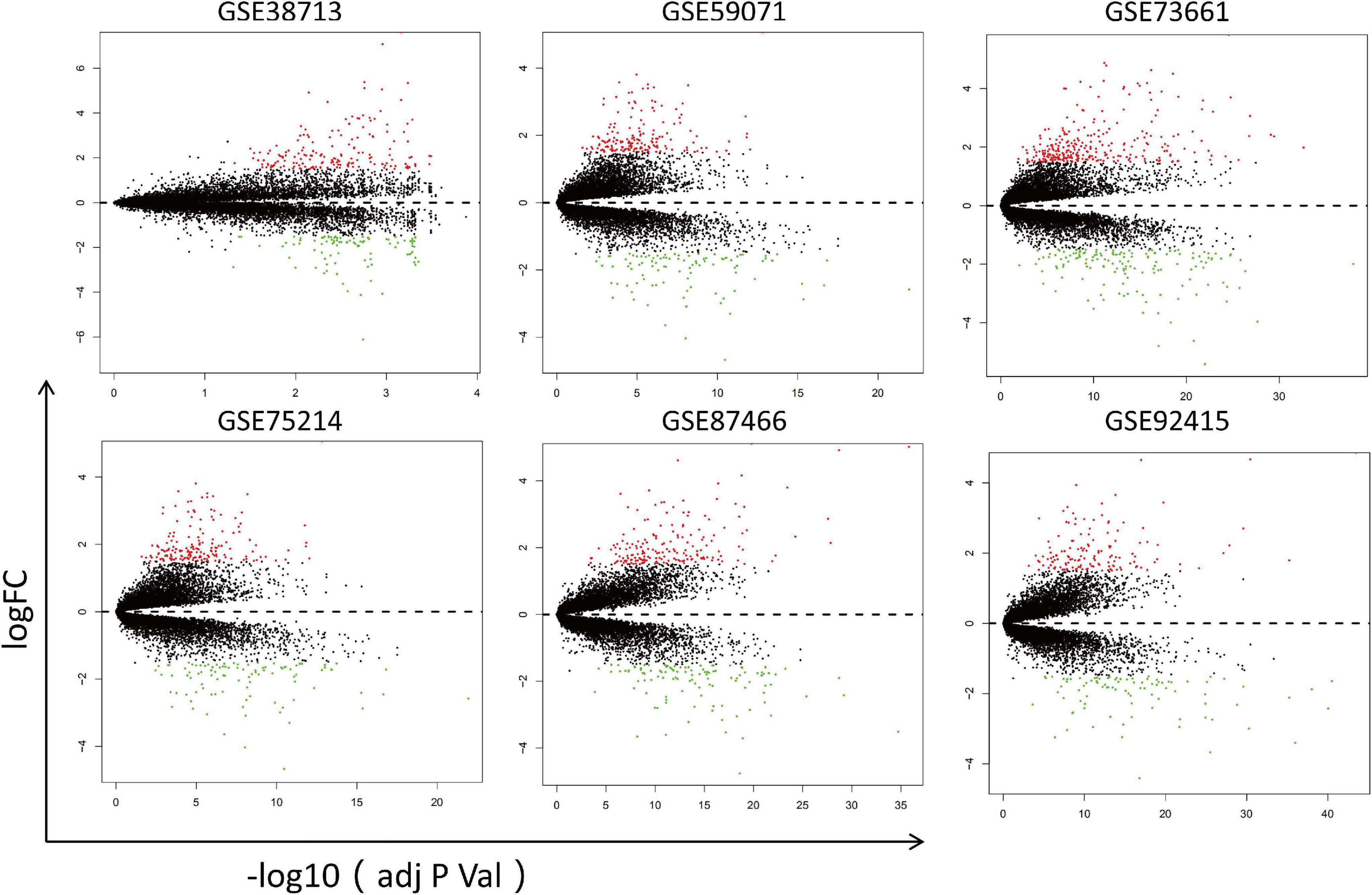
Figure 2. Volcano maps of the six datasets. Red points represent upregulated genes, while green points represent downregulated genes. Black points indicate genes with no significant difference.
RRA Integrated Analysis
A total of 208 DEGs (132 upregulated and 76 downregulated) were identified by RRA analysis, and the heatmap of the top 20 DEGs (upregulated or downregulated) is shown in Figure 3. The top 10 significant genes aberrantly expressed in UC included five upregulated genes [DUOX2 (P = 1.66E-18), SLC6A14 (P = 1.66E-18), MMP3 (P = 6.32E-18), REG1A (P = 1.06E-16), REG1B (P = 1.95E-15)], and five downregulated genes [AQP8 (P = 4.04E-22), HMGCS2 (P = 1.89E-17), PCK1 (P = 1.06E-16), SLC26A2 (P = 2.15E-16), ABCG2 (P = 4.04E-16)]. The overall results from RRA analysis are listed in Supplementary Table 2.
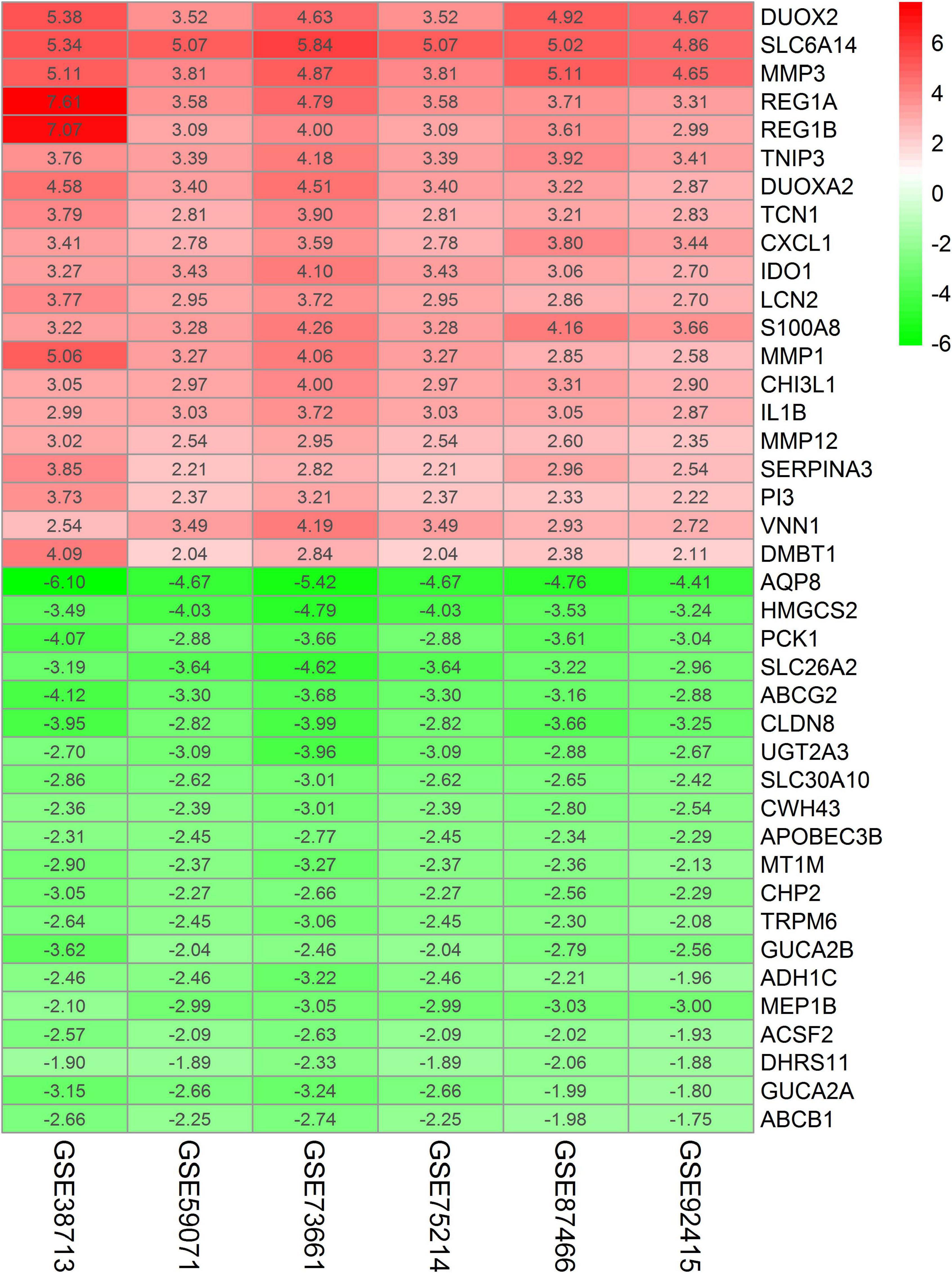
Figure 3. Heatmap of the top 20 DEGs (upregulated or downregulated) identified in the RRA analysis. Red represents a relatively high expression of genes in patients with UC. In contrast, green represents a relatively low expression of genes in patients with UC. The numbers in the heatmap represent logarithmic fold change in each dataset calculated by R software.
Compared with the single dataset analysis, the RRA integrated analysis significantly increased the sample size and reduced the operator influence, thus improving the reliability of the conclusions. In this study, according to the criteria of | logFc| > 1.5 and adjusted P < 0.05, 270, 272, 436, 272, 298, and 231 DEGs were identified in each dataset. After excluding duplicates, 666 DEGs were identified, among which 79 common DEGs were identical in all datasets. The DEGs identified in the RRA analysis (n = 208) accounted for 40.82–71.69% in each dataset, indicating that RRA integrated the results of the datasets, especially when high-throughput sequencing data were collected from different platforms covering different sets of the gene probes (Table 3).
Functional Annotation
We uploaded the 132 upregulated and 76 downregulated DEGs to perform GO (including biological process, molecular function, and cellular component) analysis and KEGG analysis. The results indicated that the upregulated DEGs were particularly enriched in humoral immune response, in leukocyte migration, in response to lipopolysaccharide, in the secretory granule lumen, in the cytoplasmic vesicle lumen, in the vesicle lumen, in receptor-ligand activity, in cytokine activity, and cytokine receptor binding and were the top three enriched terms, depending on the P-value of the respective categories (Figure 4A). The downregulated DEGs were mainly enriched in anion transmembrane transport and inorganic anion transport based on the P-value (Figure 4B).
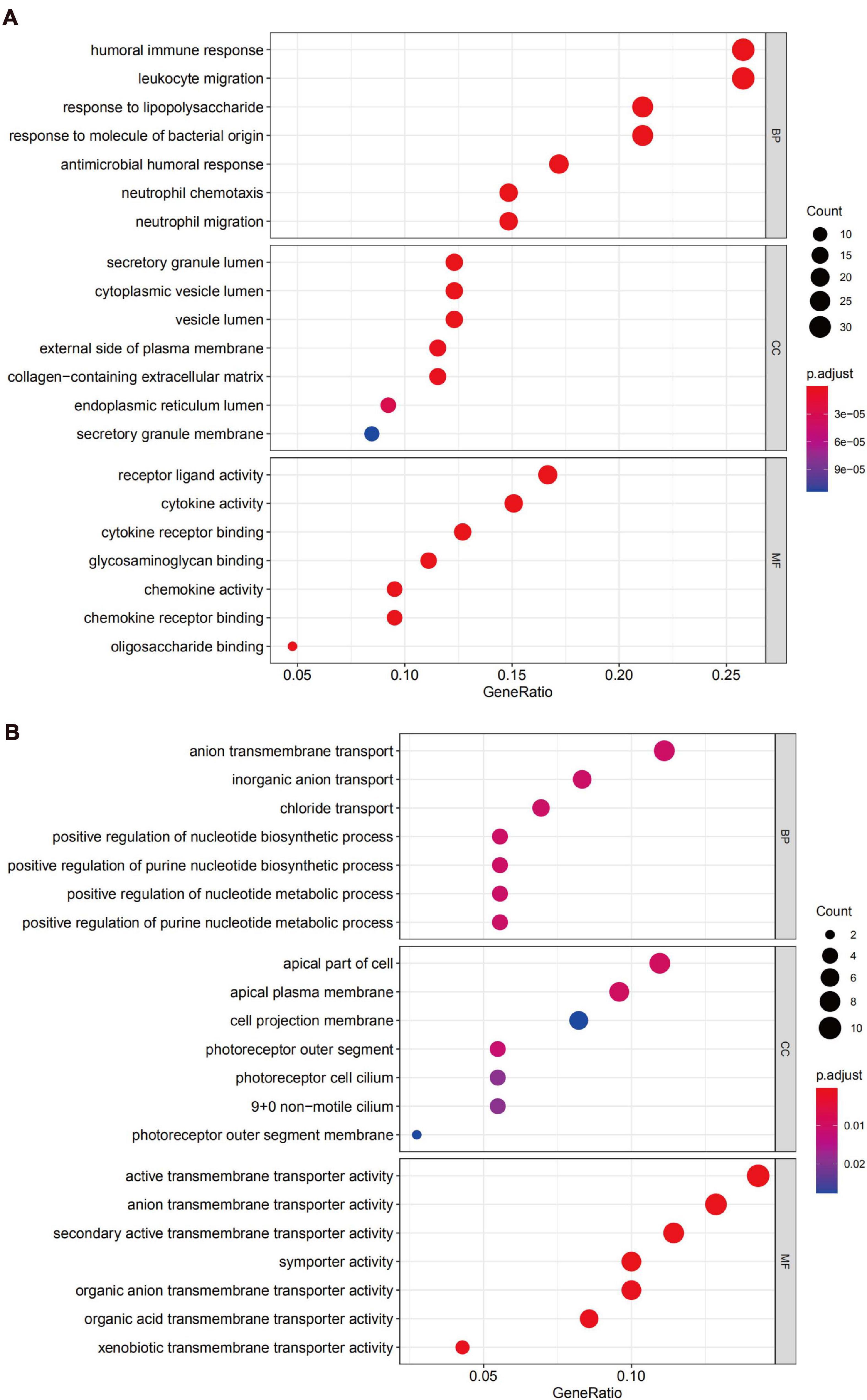
Figure 4. Gene Ontology (GO) analysis of DEGs. (A) Functional enrichment analysis of upregulated genes. (B) Functional enrichment analysis of downregulated genes.
The KEGG pathway enrichment analysis suggested that the upregulated DEGs predominantly participated in inflammation-related pathways, including the cytokine-cytokine receptor interaction and IL-17 signaling pathway, while the downregulated genes were mainly enriched in the bile secretion pathway (Figures 5A,B).
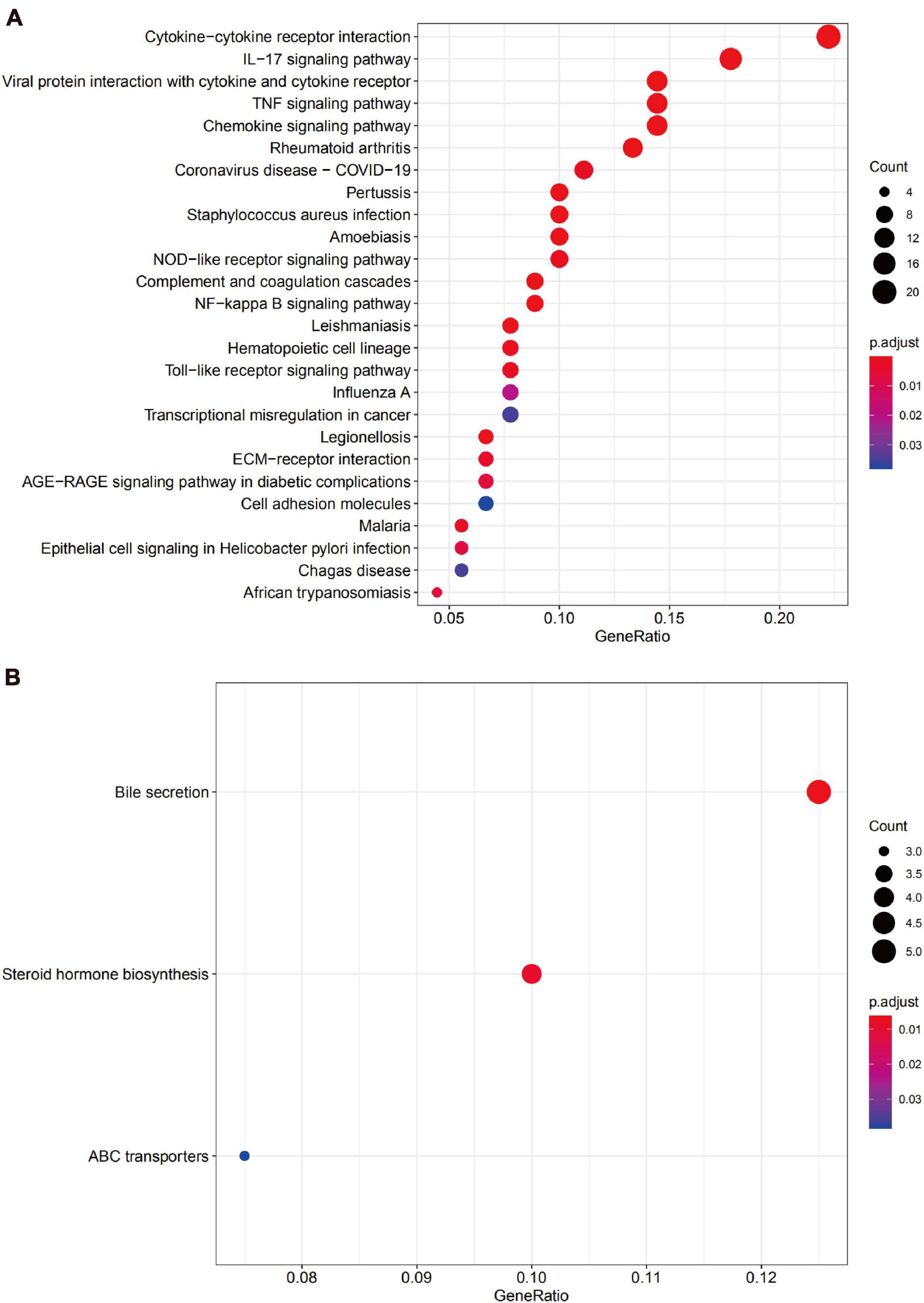
Figure 5. The Kyoto Encyclopedia of Gene and Genome (KEGG) pathway enrichment analysis for DEGs. (A) The functional enrichment analysis of upregulated genes. (B) Functional enrichment analysis of downregulated genes.
PPI Network Analysis and Identification of Hub Genes
A visual network of DEGs identified from the RRA analysis was constructed using the String (Search Tool for the Retrieval of Interacting Genes database) website, and these were comprised of 205 nodes and 880 edges. The network was then imported into Cytoscape for subsequent genetic analysis (Figure 6A).
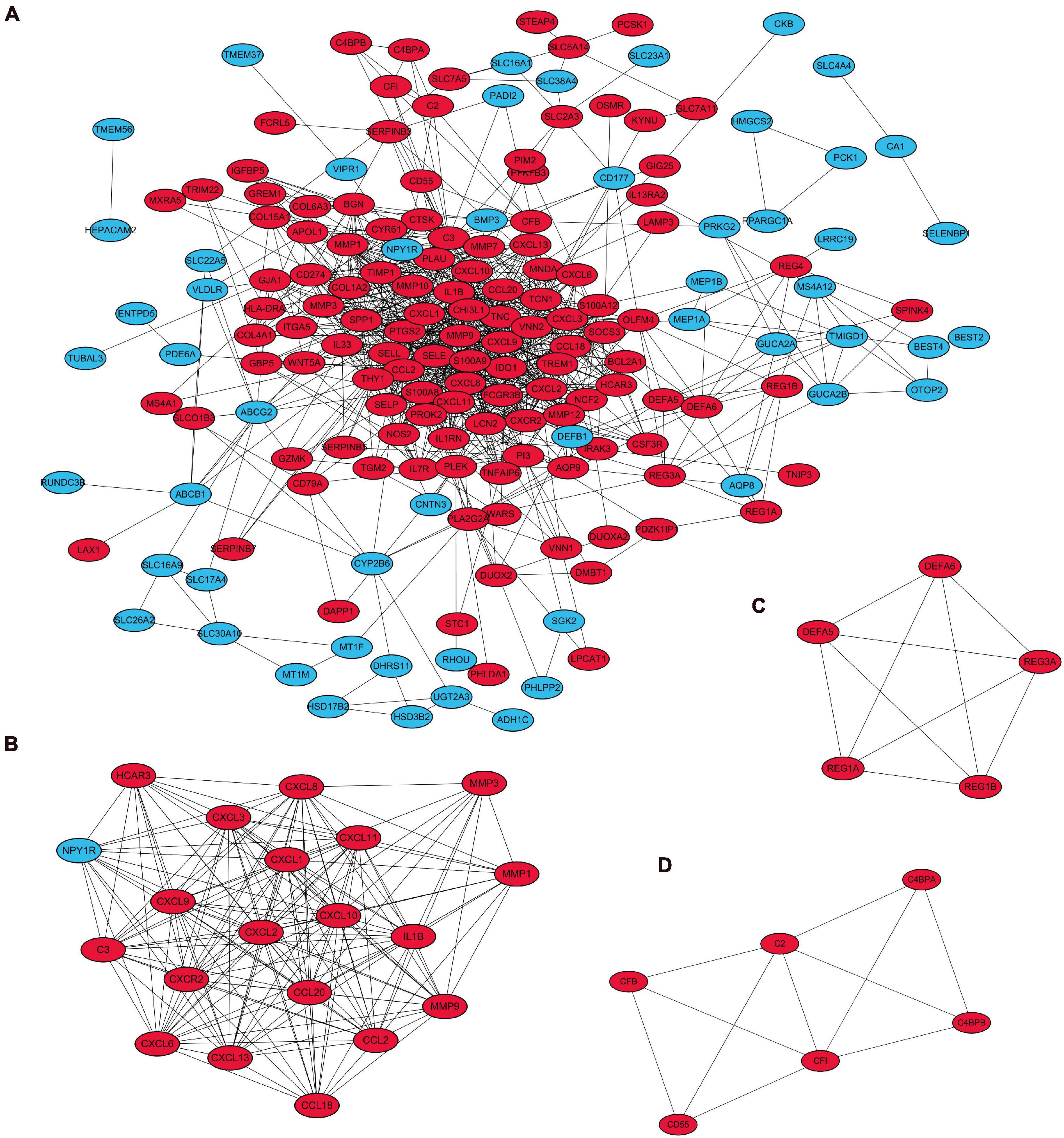
Figure 6. Visualization and module identification of the PPI network. (A) A total of 208 DEGs were mapped using Cytoscape software. Three modules of the PPI networks were identified by the MCODE plug-in. (B) Module 1 comprised CXCL6, CXCL8, CCL18, C3, HCAR3, CXCL, CXCL13, IL1β, NPY1R, MMP3, CCL20, MMP1, CXCR2, CCL2, CXCL1, CXCL10, CXCL9, CXCL3, and CXCL11 with the seed gene MMP9. (C) Module 2 contained DEFA5, DEFA6, REG3A, and REG1B with the seed gene REG1A, and (D) module 3 consisted of C2, C4BPA, CFI, CFB, and CD55 with the seed gene C4BPB. The red points represent upregulated genes, while the blue points represent downregulated genes.
The top three modules with the highest scores were identified by MCODE (Figures 6B–D). Module 1 comprised CXCL6, CXCL8, CCL18, C3, HCAR3, CXCL, CXCL13, IL1β, NPY1R, MMP3, CCL20, MMP1, CXCR2, CCL2, CXCL1, CXCL10, CXCL9, CXCL3, and CXCL11 with the seed gene MMP9; module 2 contained DEFA5, DEFA6, REG3A, and REG1B with the seed gene REG1A; and module 3 consisted of C2, C4BPA, CFI, CFB, and CD55 with the seed gene C4BPB. The score of each module is shown in Supplementary Table 3.
GO enrichment analysis of module 1 showed that the genes were mainly related to inflammatory cell migration and cytokine activity (Figure 7A), and KEGG analysis revealed that these genes were mainly involved in cytokine-cytokine receptor interactions, viral protein interactions with cytokines and cytokine receptors, chemokine signaling pathways, and IL-17 signaling pathways (Figure 7B).
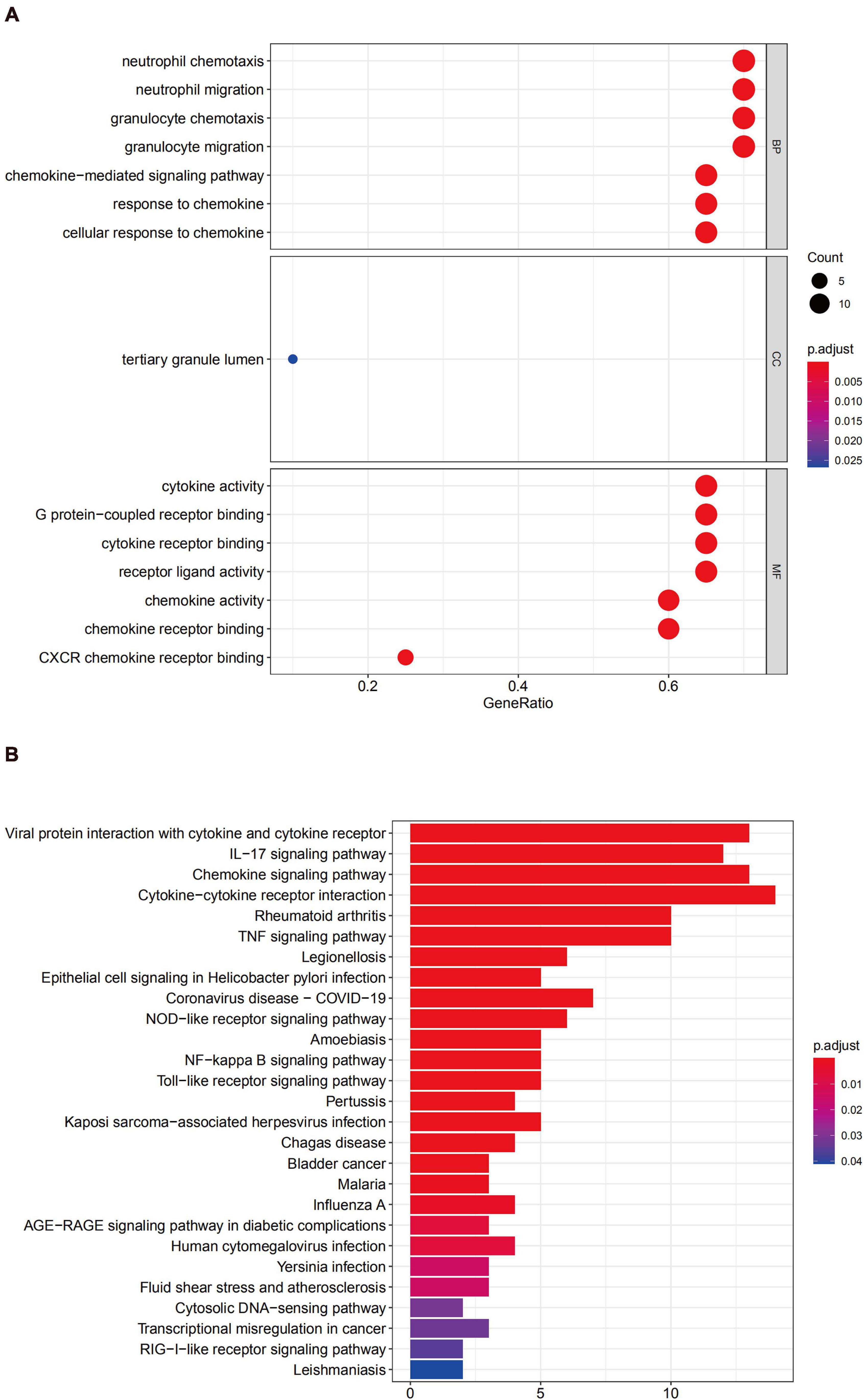
Figure 7. Functional enrichment analysis for the genes in module 1 (A) GO analysis for DEGs. (B) The KEGG analysis for DEGs.
GO enrichment analysis of module 2 revealed that the DEGs were mainly related to the humoral immune response (Figure 8A). The KEGG analysis revealed that these genes were mainly involved with Staphylococcus aureus infection, in the NOD-like receptor signaling pathway, and transcriptional misregulation in cancer (Figure 8B).
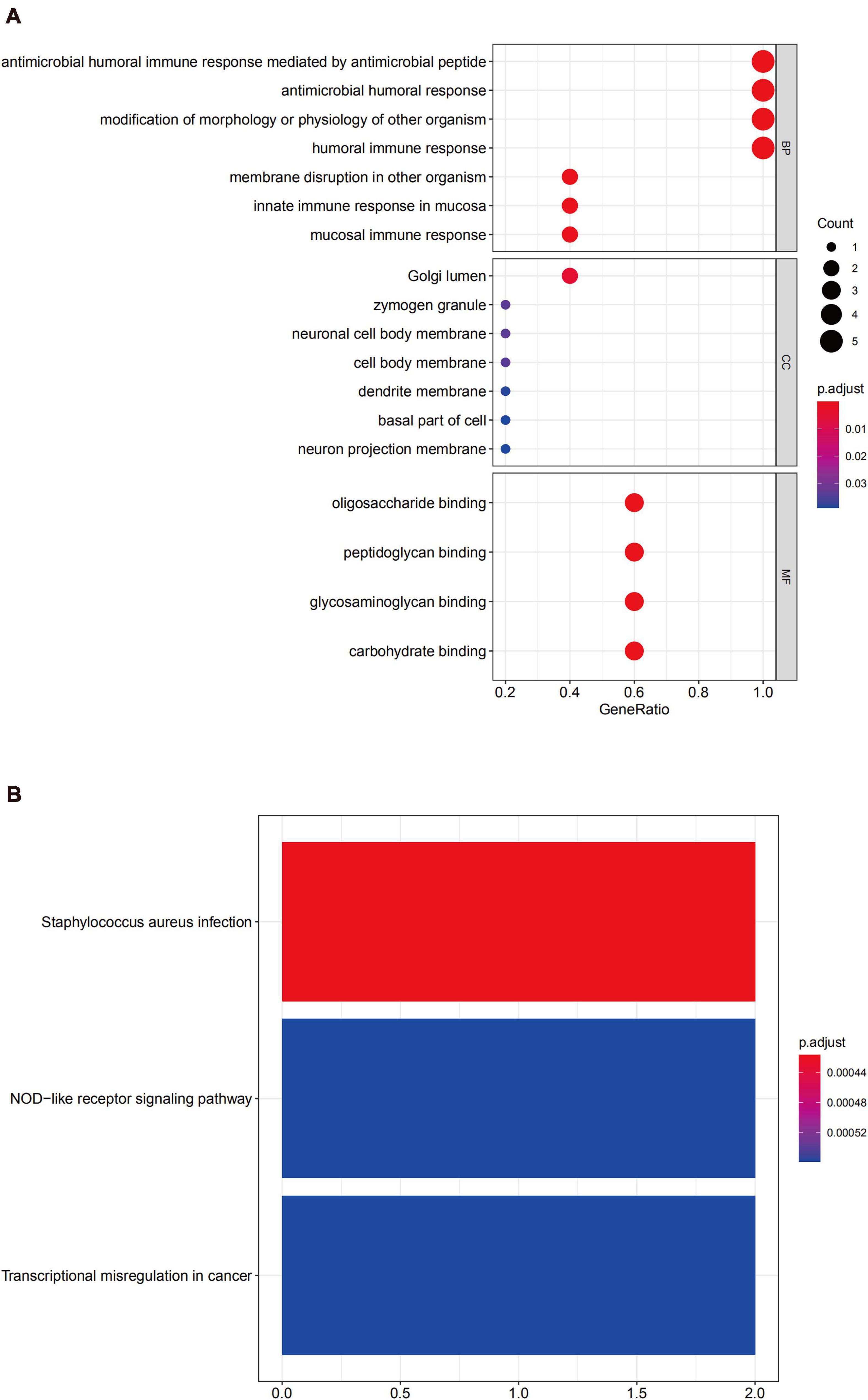
Figure 8. Functional enrichment analysis for the genes in module 2 (A) GO analysis for DEGs. (B) The KEGG analysis for DEGs.
GO enrichment analysis of module 3 suggested that the DEGs were mainly related to the regulation of complement activation, to the regulation of the protein activation cascade, and the regulation of the humoral immune response (Figure 9A), and KEGG analysis revealed that these genes were mainly involved in complement and the coagulation cascade-related pathways (Figure 9B).
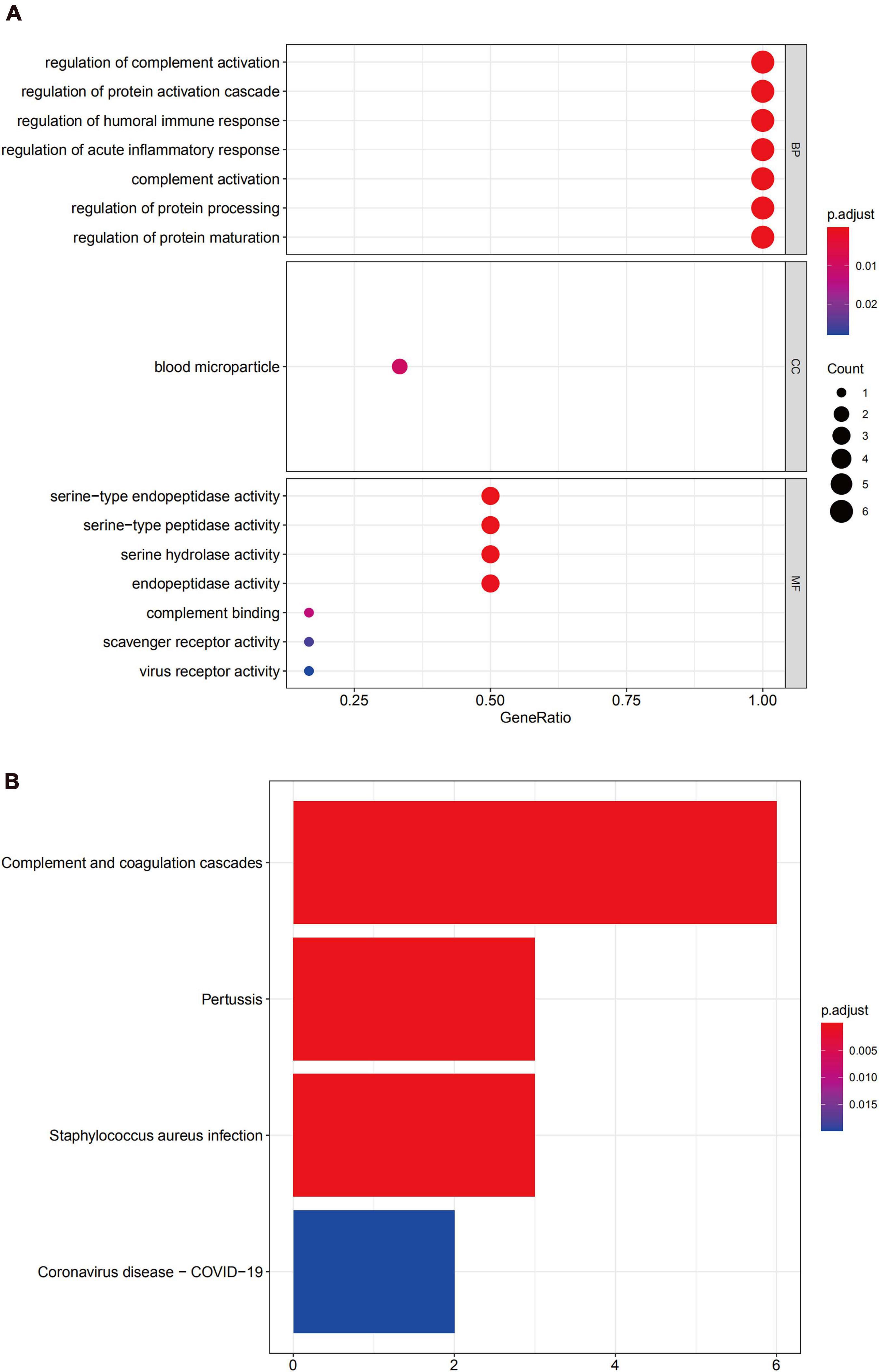
Figure 9. Functional enrichment analysis for the genes in module 3 (A) GO analysis for DEGs. (B) The KEGG analysis for DEGs.
The Determination of Hub Genes
CytoHubba was used to identify the critical genes in the PPI network and sort them by degree scores. Integrating the RRA analysis results, six hub genes were obtained, including LCN2, CXCL1, MMP3, IDO1, MMP1, and S100A8. All results are listed in Supplementary Table 4.
Verification of Hub Genes in a Mouse UC Model
Finally, the expression of the six hub genes was verified in a mouse UC model. All mice were alive at the end of the experiment (n = 8). UC was confirmed by the pathological examination of the colonic tissue. The normal structure of the colonic tissue in UC mice was almost gone, with inflammatory cell infiltration into the submucosa. Hence, the pathological score of the experimental group was significantly higher than that of the normal control group (Figures 10A,B).
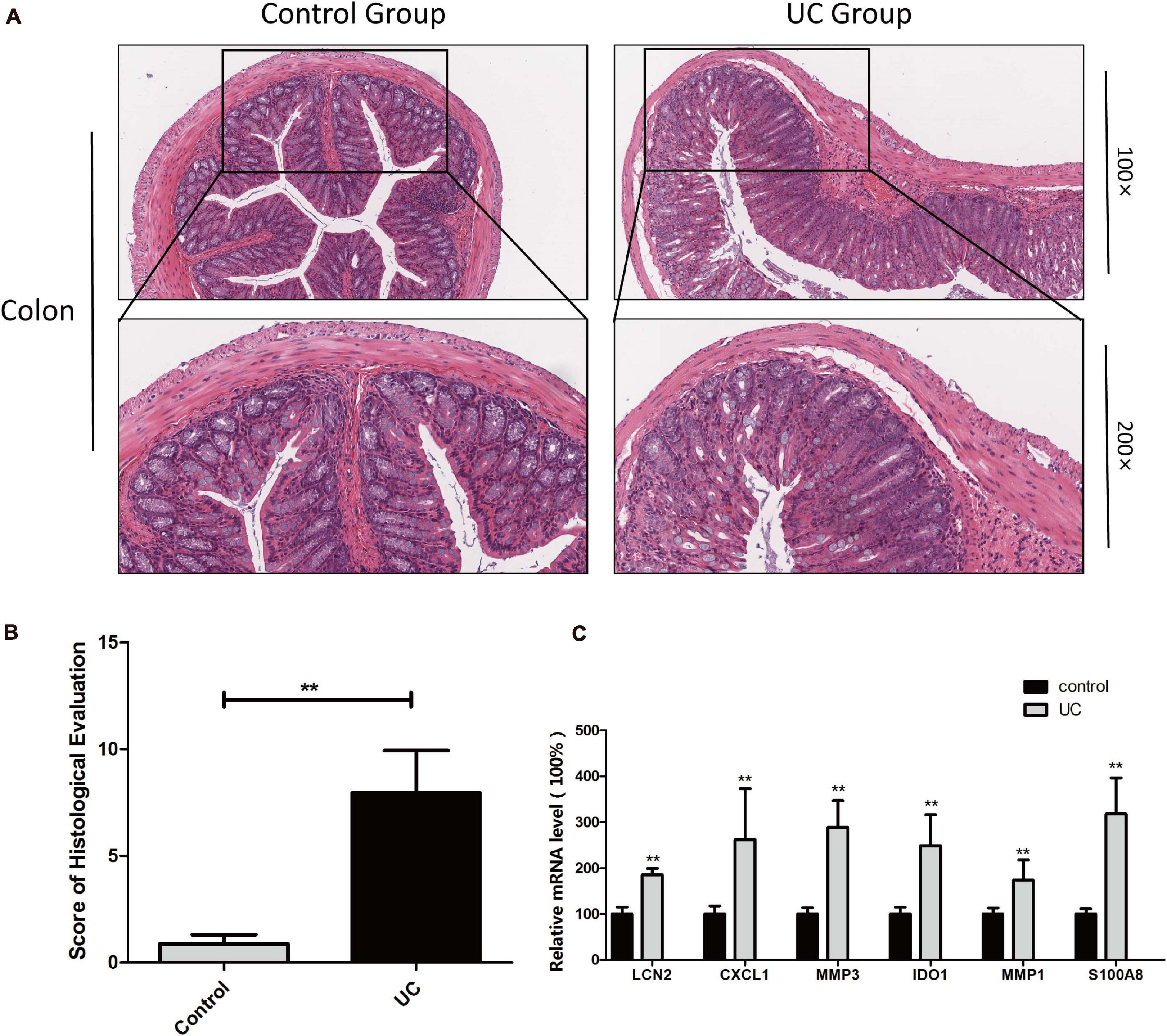
Figure 10. Determination of hub genes in mouse UC model H&E staining of colon tissues from the control and dextran sulfate sodium-induced colitis model mice (A) and histological lesion score of colon tissues (B) were performed. The expression of hub genes was examined by qPCR, and β-actin served as an internal reference (C). **Indicates p < 0.05.
The expression of the six hub genes in colon tissue was quantified by qPCR, showing that the expression was significantly higher in the experimental group than in the normal control group (Figure 10C).
Discussion
UC is an intestinal inflammation disease with multiple causes; it is becoming increasingly common and is characterized by prolonged clinical courses and recurrent attacks. The etiology remains unclear, as the genetic, environmental, and psychological factors involved in the pathogenesis make interpreting its pathological mechanism and diagnosis difficult (Kaplan and Ng, 2017; Ungaro et al., 2017). In this study, several published datasets were combined for bioinformatics analysis.
GEO (see text footnote 1) is an international public repository for high-throughput microarray and next-generation sequence functional genomic datasets submitted by the research community. Currently, it is the world’s largest public database for storing gene expression data, so it was searched to identify relevant UC datasets. In total, six datasets were retrieved and combined for RRA analysis, which identified 208 DEGs. There are several methods for the combination of multiple microarrays to perform bioinformatics analysis. Batch normalization can integrate different datasets. However, differences in measurement platforms, lab protocols, sample sizes, and operators render gene expression levels incomparable. In recent years, several studies based on microarray technology have been published to identify effective biomarkers in UC, most of which are prone to utilizing intersecting genes from different microarrays to perform analyses (Cheng et al., 2019, 2020; Chen Y. et al., 2020; Shi et al., 2020; Cao et al., 2021). As shown in Table 3, these methods can be applied to fewer datasets (≤3 datasets) because more datasets represent overly strict inclusion criteria, leading to fewer DEGs. In addition, the results based on intersecting genes are prone to be influenced by a single abnormal dataset. In contrast, RRA analysis focuses on the ranking of each gene in each dataset. With the assumption that each gene identified in each dataset is randomly arranged, the RRA compares the ranking of a randomly ordered list with the baseline case, and a higher gene rank is associated with a lower P-value. To our knowledge, RRA was first used to integrate datasets in UC and has preliminarily proven its reliability via our investigation in animal models. Notably, there are dozens of ways to perform meta-analyses of different studies, and RRA is only one of them. We reviewed the current studies on ulcerative colitis (Bopanna et al., 2017; Gubatan et al., 2019; Szemes et al., 2019; Chen M. et al., 2020; Li et al., 2020; Sankarasubramanian et al., 2020; Yoon et al., 2020; de Carvalho et al., 2021; Ye et al., 2021). We believe that the advantage of RRA analysis is that more potential biomarkers can be found at one time, which can provide clues for subsequent research. At the same time, the data were collected from microarray analysis to avoid the interference of human factors such as improper blind method. However, there are also some shortcomings, such as the biomarkers may come from data overfitting, and the follow-up validation of these biomarkers still needs follow-up experimental studies, clinical validation, and multi-center clinical trials.
PPI network analysis was performed for all DEGs. MCODE, an algorithm that allows the automated prediction of protein complexes from qualitative protein-protein interaction data, was used to identify multiple functional gene modules (Bader and Hogue, 2003). The top three modules with the highest scores were further analyzed, revealing that module 1 was mainly related to inflammation, with the genes involved in the chemotaxis, aggregation, and cytokine activity of inflammatory cells, and these genes in module 1 were previously reported on in several bioinformatic analyses of UC. The genes in modules 2 and 3 have been less frequently reported on in the literature; however, all genes were upregulated in UC. Module 2 comprised REG1A, DEFA5, DEFA6, REG3A, and REG1B. The results from the GO analysis linked module 2 to various humoral responses, while KEGG analysis showed that it was primarily involved in Staphylococcus aureus infection. Studies have reported that the severity of UC is related to the imbalanced intestinal flora in patients. Intestinal antigens from intestinal bacteria and their metabolites are common, with various antibodies produced in the intestinal immune response (Frehn et al., 2014; Jansen et al., 2016; Soontararak et al., 2019). Additionally, some studies have shown that the REG family proteins play a role in mucosal regeneration in UC (Sekikawa et al., 2010; Tsuchida et al., 2017; Takasawa et al., 2018). Module 3 contained C4BPB and was mainly related to the regulation of complement activation, while KEGG analysis revealed that it mainly participated in complement and coagulation cascades. The complement system is part of the innate sensor and effector systems, such as the Toll-like receptors (TLRs), which recognize and quickly systemically and locally respond to microbial-associated molecular patterns (MAMPs) with a tailored defense reaction. MAMP recognition of microbial-associated molecular patterns by intestinal epithelial cells (IECs) and appropriate immune responses are of significant importance for maintaining intestinal barrier function. Proper activation of the intestinal complement system might play an essential role in resolving chronic intestinal inflammation, while overactivation and/or dysregulation might worsen intestinal inflammation. Hence, how IECs, intestinal bacteria, and epithelial cells express complement and interact in the long-term course of UC remains to be elucidated (Geremia et al., 2014; Sina et al., 2018). Furthermore, the activation of the complement system may promote UC-associated carcinogenesis (Ning et al., 2015).
The DEGs were screened using CytoHubba, a novel Cytoscape plugin for scoring and ranking nodes in a network through different algorithms to evaluate the importance of nodes and gene connectivity in a biological network. It provides eleven topological analysis methods, among which degree is the most commonly used (Chin et al., 2014). The integrated analysis of the top 20 DEGs ranked by degree scores (≥ 15), and RRA identified six potential hub genes, LCN2, CXCL1, MMP3, IDO1, MMP1, and S100A8.
The C-X-C motif chemokine ligand 1 (CXCL1) has been implicated in the malignant behavior of solid and hematological neoplasms in combination with the C-X-C motif chemokine receptor 2 (CXCR2), and these two ligands act indirectly on tumor angiogenesis by regulating the trafficking of leukocytes that produce angiogenic factors and a variety of inflammatory cytokines (Mantovani et al., 2010). Recently, studies have shown that the CXCL1/CXCR2 signaling pathway could regulate the inflammatory response and promote tumor cell proliferation, invasion, and transvascular metastasis, acting as essential molecules in the progression of inflammation (Acharyya et al., 2012). Studies have also shown that the blockage of CXCR2 in neutrophils by a selective inhibitor could significantly alleviate the symptoms of DSS-induced colitis in mice and could suppress the production of proinflammatory cytokines. Hence, CXCR2 is a potential target for UC treatment (Zhu et al., 2020). LCN2 (Østvik et al., 2013; Stallhofer et al., 2015; Buisson et al., 2018; Zollner et al., 2021) and S100A8 (Manolakis et al., 2011; Azramezani Kopi et al., 2019; Okada et al., 2019, 2020) are critical proinflammatory cytokines that have been reported in recent studies as potential molecular markers of UC in serum and stool samples. LCN2 and S100A8 also have antimicrobial effects and may be involved in the regulation of intestinal flora as antimicrobial peptides, which may be indirectly related to UC. Host and microbial tryptophan (Trp) metabolism have emerged as critical regulators in mucosal homeostasis. Indoleamine 2,3 dioxygenase-1 (IDO1) is the first enzyme in Trp metabolism in the kynurenine (Kyn) pathway and is perhaps most relevant in the context of homeostasis. Therefore, IDO1 may be an important molecular marker (Sofia et al., 2018; Alvarado et al., 2019). Although the specific mechanisms of IDO1 remain obscure, numerous studies have shown an increased expression of IDO1 in inflammatory bowel disease, infection, and diverticulosis (Ferdinande et al., 2008; Nikolaus et al., 2017; Vancamelbeke et al., 2017). IDO1 has also been closely related to disease remission, with genetic abnormalities or drug inhibition of IDO1 aggravating the disease (Ciorba et al., 2010; Gupta et al., 2012; Nikolaus et al., 2017).
In recent years, the association between UC and colon cancer has attracted significant attention. CXCL1, LCN2, S100A8, and IDO1 may play essential roles in cancer progression. Studies have shown that IDO1 could promote colitis-associated tumorigenesis in mice. This approach revealed a cell-autonomous mechanism by which IDO1 tryptophan catabolites (kynurenine and quinolinic acid) directly promote cancer cell proliferation (Thaker et al., 2013). Further research is needed regarding the related evolutionary mechanism of UC and colon cancer.
Matrix metalloproteinases (MMPs) belong to a family of zinc-dependent endopeptidases, which are mainly produced and secreted by connective tissue, endothelial cells, mononuclear macrophages, neutrophils, and tumor cells. MMPs participate in the degradation of ECM components (Karamanos et al., 2021), with increased expression in the UC lesion area (Schuppan and Hahn, 2000; Medina et al., 2003; Wang and Yan, 2006; Meijer et al., 2007). Additionally, genetic variations in MMPs may be associated with an increased risk of UC differences in clinical symptoms (Morgan et al., 2011). Studies have shown that the overexpression of MMP-1 and MMP-3 play an important role in the pathogenesis of steroid-dependent uncreative colitis (SDUC). The protein expressions of MMP-1 and MMP-3 significantly increased in the healing regions of colonic tissues in SDUC remission patients but not in non-SDUC remission patients, suggesting that the overexpression of MMP-1 and MMP-3 are not the only factors involved in the pathogenesis of UC but also are a critical feature in the steroid dependency in UC (Wang and Qiu, 2010).
The expression of the six hub genes was confirmed in the DSS-induced UC mouse model. In published studies, some scholars screened diagnostic biomarkers for UC from the DEGs identified from a single dataset or an overlap of two or three datasets via bioinformatics analyses (Cheng et al., 2019, 2020; Chen Y. et al., 2020; Shi et al., 2020; Cao et al., 2021). In contrast, the advantage of this research lies in the larger dataset obtained by combining data from six GEO datasets, which increased the sample size and ensured the stability and relative reliability of the conclusions. On the other hand, the RRA method was used to reduce the influences of the measurement platform, the sample size of datasets, the experimental design, and other factors on the final results.
Conclusion
In conclusion, six datasets were integrated for bioinformatics analyses and identified three functional gene modules and six hub genes, the expression of which was confirmed in a mouse model of UC. These results will help to further explore the mechanisms related to the occurrence and development of UC and to provide potential targets for the detection and treatment of UC patients in the future.
Data Availability Statement
The original contributions presented in the study are included in the article/Supplementary Material, further inquiries can be directed to the corresponding author/s.
Ethics Statement
The animal study was reviewed and approved by The Ethics Committee at the Hebei Medical University.
Author Contributions
Z-AC collected the manuscripts and analyzed the data, analyzed the conclusions, and drafted the manuscript. Y-FS reviewed the data and conclusions. Q-XW and H-HM contributed to writing. C-JY and Z-ZM presented the idea of this manuscript, supported the funding, analyzed the conclusions, and drafted and revised the manuscript. All authors contributed to the article and approved the submitted version.
Funding
This study was funded by the Natural Science Foundation of Hebei Province (Grant No. H2020206337).
Conflict of Interest
The authors declare that the research was conducted in the absence of any commercial or financial relationships that could be construed as a potential conflict of interest.
Supplementary Material
The Supplementary Material for this article can be found online at: https://www.frontiersin.org/articles/10.3389/fgene.2021.697514/full#supplementary-material
Footnotes
References
Acharyya, S., Oskarsson, T., Vanharanta, S., Malladi, S., Kim, J., Morris, P. G., et al. (2012). A CXCL1 paracrine network links cancer chemoresistance and metastasis. Cell 150, 165–178. doi: 10.1016/j.cell.2012.04.042
Alvarado, D. M., Chen, B., Iticovici, M., Thaker, A. I., Dai, N., VanDussen, K. L., et al. (2019). Epithelial indoleamine 2,3-dioxygenase 1 modulates aryl hydrocarbon receptor and notch signaling to increase differentiation of secretory cells and alter mucus-associated microbiota. Gastroenterology 157, 1093.e11–1108.e11. doi: 10.1053/j.gastro.2019.07.013
Arijs, I., De Hertogh, G., Lemmens, B., Van Lommel, L., de Bruyn, M., Vanhove, W., et al. (2018). Effect of vedolizumab (anti-α4β7-integrin) therapy on histological healing and mucosal gene expression in patients with UC. Gut 67, 43–52. doi: 10.1136/gutjnl-2016-312293
Azramezani Kopi, T., Amini Kadijani, A., Parsian, H., Shahrokh, S., Asadzadeh Aghdaei, H., Mirzaei, A., et al. (2019). The value of mRNA expression of S100A8 and S100A9 as blood-based biomarkers of inflammatory bowel disease. Arab. J. Gastroenterol. 20, 135–140. doi: 10.1016/j.ajg.2019.07.002
Bader, G. D., and Hogue, C. W. (2003). An automated method for finding molecular complexes in large protein interaction networks. BMC Bioinform. 4:2. doi: 10.1186/1471-2105-4-2
Bopanna, S., Ananthakrishnan, A. N., Kedia, S., Yajnik, V., and Ahuja, V. (2017). Risk of colorectal cancer in Asian patients with ulcerative colitis: a systematic review and meta-analysis. Lancet Gastroenterol. Hepatol. 2, 269–276. doi: 10.1016/S2468-1253(17)30004-3
Brookes, M. J., Whitehead, S., Gaya, D. R., and Hawthorne, A. B. (2018). Practical guidance on the use of faecal calprotectin. Frontline Gastroenterol. 9:87–91. doi: 10.1136/flgastro-2016-100762
Buisson, A., Vazeille, E., Minet-Quinard, R., Goutte, M., Bouvier, D., Goutorbe, F., et al. (2018). Fecal matrix metalloprotease-9 and lipocalin-2 as biomarkers in detecting endoscopic activity in patients with inflammatory bowel diseases. J. Clin. Gastroenterol. 52, e53–e53. doi: 10.1097/MCG.0000000000000837
Cao, F., Cheng, Y. S., Yu, L., Xu, Y. Y., and Wang, Y. (2021). Bioinformatics analysis of differentially expressed genes and protein-protein interaction networks associated with functional pathways in ulcerative colitis. Med. Sci. Monit. 27:e927917. doi: 10.12659/MSM.927917
Chen, M., Ding, Y., and Tong, Z. (2020). Efficacy and safety of sophora flavescens (Kushen) based traditional Chinese medicine in the treatment of ulcerative colitis: clinical evidence and potential mechanisms. Front. Pharmacol. 11:603476. doi: 10.3389/fphar.2020.603476
Chen, Y., Li, H., Lai, L., Feng, Q., and Shen, J. (2020). Identification of common differentially expressed genes and potential therapeutic targets in ulcerative colitis and rheumatoid arthritis. Front. Genet. 11:572194. doi: 10.3389/fgene.2020.572194
Cheng, C., Hua, J., Tan, J., Qian, W., Zhang, L., and Hou, X. (2019). Identification of differentially expressed genes, associated functional terms pathways, and candidate diagnostic biomarkers in inflammatory bowel diseases by bioinformatics analysis. Exp. Ther. Med. 18, 278–288. doi: 10.3892/etm.2019.7541
Cheng, F., Li, Q., Wang, J., Zeng, F., Wang, K., and Zhang, Y. (2020). Identification of differential intestinal mucosa transcriptomic biomarkers for ulcerative colitis by bioinformatics analysis. Dis. Markers 2020:8876565. doi: 10.1155/2020/8876565
Chin, C. H., Chen, S. H., Wu, H. H., Ho, C. W., Ko, M. T., and Lin, C. Y. (2014). cytoHubba: identifying hub objects and sub-networks from complex interactome. BMC Syst. Biol. 8(Suppl. 4):S11. doi: 10.1186/1752-0509-8-S4-S11
Ciorba, M. A., Bettonville, E. E., McDonald, K. G., Metz, R., Prendergast, G. C., Newberry, R. D., et al. (2010). Induction of IDO-1 by immunostimulatory DNA limits severity of experimental colitis. J. Immunol. 184, 3907–3916. doi: 10.4049/jimmunol.0900291
Dalmer, T., and Clugston, R. D. (2019). Gene ontology enrichment analysis of congenital diaphragmatic hernia-associated genes. Pediatr. Res. 85, 13–19. doi: 10.1038/s41390-018-0192-8
de Carvalho, L., Lima, W. G., Coelho, L., Cardoso, V. N., and Fernandes, S. (2021). Circulating leptin levels as a potential biomarker in inflammatory bowel diseases: a systematic review and meta-analysis. Inflamm. Bowel Dis. 27, 169–181. doi: 10.1093/ibd/izaa037
Dieleman, L. A., Palmen, M. J., Akol, H., Bloemena, E., Peña, A. S., Meuwissen, S. G., et al. (1998). Chronic experimental colitis induced by dextran sulphate sodium (DSS) is characterized by Th1 and Th2 cytokines. Clin. Exp. Immunol. 114, 385–391. doi: 10.1046/j.1365-2249.1998.00728.x
Ferdinande, L., Demetter, P., Perez-Novo, C., Waeytens, A., Taildeman, J., Rottiers, I., et al. (2008). Inflamed intestinal mucosa features a specific epithelial expression pattern of indoleamine 2,3-dioxygenase. Int. J. Immunopathol. Pharmacol. 21, 289–295. doi: 10.1177/039463200802100205
Frehn, L., Jansen, A., Bennek, E., Mandic, A. D., Temizel, I., Tischendorf, S., et al. (2014). Distinct patterns of IgG and IgA against food and microbial antigens in serum and feces of patients with inflammatory bowel diseases. PLoS One 9:e106750. doi: 10.1371/journal.pone.0106750
Geremia, A., Biancheri, P., Allan, P., Corazza, G. R., and Di Sabatino, A. (2014). Innate and adaptive immunity in inflammatory bowel disease. Autoimmun. Rev. 13, 3–10. doi: 10.1016/j.autrev.2013.06.004
Gubatan, J., Chou, N. D., Nielsen, O. H., and Moss, A. C. (2019). Systematic review with meta-analysis: association of vitamin D status with clinical outcomes in adult patients with inflammatory bowel disease. Aliment. Pharmacol. Ther. 50, 1146–1158. doi: 10.1111/apt.15506
Gupta, N. K., Thaker, A. I., Kanuri, N., Riehl, T. E., Rowley, C. W., Stenson, W. F., et al. (2012). Serum analysis of tryptophan catabolism pathway: correlation with Crohn’s disease activity. Inflamm. Bowel Dis. 18, 1214–1220. doi: 10.1002/ibd.21849
Jansen, A., Mandić, A. D., Bennek, E., Frehn, L., Verdier, J., Tebrügge, I., et al. (2016). Anti-food and anti-microbial IgG subclass antibodies in inflammatory bowel disease. Scand. J. Gastroenterol. 51, 1453–1461. doi: 10.1080/00365521.2016.1205130
Kaplan, G. G., and Ng, S. C. (2017). Understanding and preventing the global increase of inflammatory bowel disease. Gastroenterology 152, 313.e2–321.e2. doi: 10.1053/j.gastro.2016.10.020
Karamanos, N. K., Theocharis, A. D., Piperigkou, Z., Manou, D., Passi, A., Skandalis, S. S., et al. (2021). A guide to the composition and functions of the extracellular matrix. FEBS J. [Online ahead of print] doi: 10.1111/febs.15776
Kolde, R., Laur, S., Adler, P., and Vilo, J. (2012). Robust rank aggregation for gene list integration and meta-analysis. Bioinformatics 28, 573–580. doi: 10.1093/bioinformatics/btr709
Li, K., Strauss, R., Ouahed, J., Chan, D., Telesco, S. E., Shouval, D. S., et al. (2018). Molecular comparison of adult and pediatric ulcerative colitis indicates broad similarity of molecular pathways in disease tissue. J. Pediatr. Gastroenterol. Nutr. 67, 45–52. doi: 10.1097/MPG.0000000000001898
Li, X., Lee, E. J., Gawel, D. R., Lilja, S., Schäfer, S., Zhang, H., et al. (2020). Meta-analysis of expression profiling data indicates need for combinatorial biomarkers in pediatric ulcerative colitis. J. Immunol. Res. 2020:8279619. doi: 10.1155/2020/8279619
Manolakis, A. C., Kapsoritakis, A. N., Tiaka, E. K., and Potamianos, S. P. (2011). Calprotectin, calgranulin C, and other members of the s100 protein family in inflammatory bowel disease. Dig. Dis. Sci. 56, 1601–1611. doi: 10.1007/s10620-010-1494-9
Mantovani, A., Savino, B., Locati, M., Zammataro, L., Allavena, P., and Bonecchi, R. (2010). The chemokine system in cancer biology and therapy. Cytokine Growth Factor Rev. 21, 27–39. doi: 10.1016/j.cytogfr.2009.11.007
Medina, C., Videla, S., Radomski, A., Radomski, M. W., Antolín, M., Guarner, F., et al. (2003). Increased activity and expression of matrix metalloproteinase-9 in a rat model of distal colitis. Am. J. Physiol. Gastrointest. Liver Physiol. 284, G116–G122. doi: 10.1152/ajpheart.00036.2002
Meijer, M. J., Mieremet-Ooms, M. A., van der Zon, A. M., van Duijn, W., van Hogezand, R. A., Sier, C. F., et al. (2007). Increased mucosal matrix metalloproteinase-1, -2, -3 and -9 activity in patients with inflammatory bowel disease and the relation with Crohn’s disease phenotype. Dig. Liver Dis. 39, 733–739. doi: 10.1016/j.dld.2007.05.010
Morgan, A. R., Han, D. Y., Lam, W. J., Triggs, C. M., Fraser, A. G., Barclay, M., et al. (2011). Genetic variations in matrix metalloproteinases may be associated with increased risk of ulcerative colitis. Hum. Immunol. 72, 1117–1127. doi: 10.1016/j.humimm.2011.08.011
Ng, S. C., Shi, H. Y., Hamidi, N., Underwood, F. E., Tang, W., Benchimol, E. I., et al. (2017). Worldwide incidence and prevalence of inflammatory bowel disease in the 21st century: a systematic review of population-based studies. Lancet 390, 2769–2778. doi: 10.1016/S0140-6736(17)32448-0
Nikolaus, S., Schulte, B., Al-Massad, N., Thieme, F., Schulte, D. M., Bethge, J., et al. (2017). Increased tryptophan metabolism is associated with activity of inflammatory bowel diseases. Gastroenterology 153, 1504.e2–1516.e2. doi: 10.1053/j.gastro.2017.08.028
Ning, C., Li, Y. Y., Wang, Y., Han, G. C., Wang, R. X., Xiao, H., et al. (2015). Complement activation promotes colitis-associated carcinogenesis through activating intestinal IL-1β/IL-17A axis. Mucosal. Immunol. 8, 1275–1284. doi: 10.1038/mi.2015.18
Okada, K., Itoh, H., and Ikemoto, M. (2020). Circulating S100A8/A9 is potentially a biomarker that could reflect the severity of experimental colitis in rats. Heliyon 6:e03470. doi: 10.1016/j.heliyon.2020.e03470
Okada, K., Okabe, M., Kimura, Y., Itoh, H., and Ikemoto, M. (2019). Serum S100A8/A9 as a potentially sensitive biomarker for inflammatory bowel disease. Lab. Med. 50, 370–380. doi: 10.1093/labmed/lmz003
Østvik, A. E., Granlund, A. V., Torp, S. H., Flatberg, A., Beisvåg, V., Waldum, H. L., et al. (2013). Expression of Toll-like receptor-3 is enhanced in active inflammatory bowel disease and mediates the excessive release of lipocalin 2. Clin. Exp. Immunol. 173, 502–511. doi: 10.1111/cei.12136
Parikh, K., Antanaviciute, A., Fawkner-Corbett, D., Jagielowicz, M., Aulicino, A., Lagerholm, C., et al. (2019). Colonic epithelial cell diversity in health and inflammatory bowel disease. Nature 567, 49–55. doi: 10.1038/s41586-019-0992-y
Planell, N., Lozano, J. J., Mora-Buch, R., Masamunt, M. C., Jimeno, M., Ordás, I., et al. (2013). Transcriptional analysis of the intestinal mucosa of patients with ulcerative colitis in remission reveals lasting epithelial cell alterations. Gut 62, 967–976. doi: 10.1136/gutjnl-2012-303333
Sankarasubramanian, J., Ahmad, R., Avuthu, N., Singh, A. B., and Guda, C. (2020). Gut microbiota and metabolic specificity in ulcerative colitis and crohn’s disease. Front. Med. (Lausanne) 7:606298. doi: 10.3389/fmed.2020.606298
Schuppan, D., and Hahn, E. G. (2000). MMPs in the gut: inflammation hits the matrix. Gut 47, 12–14. doi: 10.1136/gut.47.1.12
Sekikawa, A., Fukui, H., Suzuki, K., Karibe, T., Fujii, S., Ichikawa, K., et al. (2010). Involvement of the IL-22/REG Ialpha axis in ulcerative colitis. Lab. Invest. 90, 496–505. doi: 10.1038/labinvest.2009.147
Shi, L., Han, X., Li, J. X., Liao, Y. T., Kou, F. S., Wang, Z. B., et al. (2020). Identification of differentially expressed genes in ulcerative colitis and verification in a colitis mouse model by bioinformatics analyses. World J. Gastroenterol. 26, 5983–5996. doi: 10.3748/wjg.v26.i39.5983
Sina, C., Kemper, C., and Derer, S. (2018). The intestinal complement system in inflammatory bowel disease: shaping intestinal barrier function. Semin. Immunol. 37, 66–73. doi: 10.1016/j.smim.2018.02.008
Smoot, M. E., Ono, K., Ruscheinski, J., Wang, P. L., and Ideker, T. (2011). Cytoscape 2.8: new features for data integration and network visualization. Bioinformatics 27, 431–432. doi: 10.1093/bioinformatics/btq675
Sofia, M. A., Ciorba, M. A., Meckel, K., Lim, C. K., Guillemin, G. J., Weber, C. R., et al. (2018). Tryptophan metabolism through the kynurenine pathway is associated with endoscopic inflammation in ulcerative colitis. Inflamm. Bowel Dis. 24, 1471–1480. doi: 10.1093/ibd/izy103
Soontararak, S., Chow, L., Johnson, V., Coy, J., Webb, C., Wennogle, S., et al. (2019). Humoral immune responses against gut bacteria in dogs with inflammatory bowel disease. PLoS One 14:e0220522. doi: 10.1371/journal.pone.0220522
Stallhofer, J., Friedrich, M., Konrad-Zerna, A., Wetzke, M., Lohse, P., Glas, J., et al. (2015). Lipocalin-2 is a disease activity marker in inflammatory bowel disease regulated by IL-17A, IL-22, and TNF-α and modulated by IL23R genotype status. Inflamm. Bowel Dis. 21, 2327–2340. doi: 10.1097/MIB.0000000000000515
Szemes, K., Soós, A., Hegyi, P., Farkas, N., Erős, A., Erőss, B., et al. (2019). Comparable long-term outcomes of cyclosporine and infliximab in patients with steroid-refractory acute severe ulcerative colitis: a meta-analysis. Front. Med. (Lausanne) 6:338. doi: 10.3389/fmed.2019.00338
Takasawa, S., Tsuchida, C., Sakuramoto-Tsuchida, S., Takeda, M., Itaya-Hironaka, A., Yamauchi, A., et al. (2018). Expression of human REG family genes in inflammatory bowel disease and their molecular mechanism. Immunol. Res. 66, 800–805. doi: 10.1007/s12026-019-9067-2
Thaker, A. I., Rao, M. S., Bishnupuri, K. S., Kerr, T. A., Foster, L., Marinshaw, J. M., et al. (2013). IDO1 metabolites activate β-catenin signaling to promote cancer cell proliferation and colon tumorigenesis in mice. Gastroenterology 145, 416.e1–4–425.e1–4. doi: 10.1053/j.gastro.2013.05.002
Tsuchida, C., Sakuramoto-Tsuchida, S., Taked, M., Itaya-Hironaka, A., Yamauchi, A., Misu, M., et al. (2017). Expression of REG family genes in human inflammatory bowel diseases and its regulation. Biochem. Biophys. Rep. 12, 198–205. doi: 10.1016/j.bbrep.2017.10.003
Ungaro, R., Mehandru, S., Allen, P. B., Peyrin-Biroulet, L., and Colombel, J. F. (2017). Ulcerative colitis. Lancet 389, 1756–1770. doi: 10.1016/S0140-6736(16)32126-2
Vancamelbeke, M., Vanuytsel, T., Farré, R., Verstockt, S., Ferrante, M., Van Assche, G., et al. (2017). Genetic and transcriptomic bases of intestinal epithelial barrier dysfunction in inflammatory bowel disease. Inflamm. Bowel Dis. 23, 1718–1729. doi: 10.1097/MIB.0000000000001246
Vanhove, W., Peeters, P. M., Staelens, D., Schraenen, A., Van der Goten, J., Cleynen, I., et al. (2015). Strong upregulation of AIM2 and IFI16 inflammasomes in the mucosa of patients with active inflammatory bowel disease. Inflamm. Bowel Dis. 21, 2673–2682. doi: 10.1097/MIB.0000000000000535
Wang, Y. D., and Yan, P. Y. (2006). Expression of matrix metalloproteinase-1 and tissue inhibitor of metalloproteinase-1 in ulcerative colitis. World J. Gastroenterol. 12, 6050–6053. doi: 10.3748/wjg.v12.i37.6050
Wang, Z. Y., and Qiu, B. F. (2010). Increased expression of matrix metalloproteinase-1 and 3 in remission patients of steroid-dependent ulcerative colitis. Gastroenterology Res. 3, 120–124. doi: 10.4021/gr2010.05.208w
Ye, X., Wang, Y., Wang, H., Feng, R., Ye, Z., Han, J., et al. (2021). Can fecal calprotectin accurately identify histological activity of ulcerative colitis? A meta-analysis. Therap. Adv. Gastroenterol. 14:1756284821994741. doi: 10.1177/1756284821994741
Yoon, H., Jangi, S., Dulai, P. S., Boland, B. S., Prokop, L. J., Jairath, V., et al. (2020). Incremental benefit of achieving endoscopic and histologic remission in patients with ulcerative colitis: a systematic review and meta-analysis. Gastroenterology 159, 1262.e7–1275.e7. doi: 10.1053/j.gastro.2020.06.043
Yu, G., Wang, L. G., Han, Y., and He, Q. Y. (2012). clusterProfiler: an R package for comparing biological themes among gene clusters. OMICS 16, 284–287. doi: 10.1089/omi.2011.0118
Zhu, F., He, H., Fan, L., Ma, C., Xu, Z., Xue, Y., et al. (2020). Blockade of CXCR2 suppresses proinflammatory activities of neutrophils in ulcerative colitis. Am. J. Transl. Res. 12, 5237–5251.
Keywords: ulcerative colitis, robust rank aggregation, differentially expressed genes, GEO database, microarray
Citation: Chen Z-A, Sun Y-F, Wang Q-X, Ma H-H, Ma Z-Z and Yang C-J (2021) Integrated Analysis of Multiple Microarray Studies to Identify Novel Gene Signatures in Ulcerative Colitis. Front. Genet. 12:697514. doi: 10.3389/fgene.2021.697514
Received: 19 April 2021; Accepted: 07 June 2021;
Published: 09 July 2021.
Edited by:
Shulan Tian, Mayo Clinic, United StatesReviewed by:
Espiridión Ramos-Martínez, Universidad Nacional Autónoma de México, MexicoPanwen Wang, Mayo Clinic Arizona, United States
Copyright © 2021 Chen, Sun, Wang, Ma, Ma and Yang. This is an open-access article distributed under the terms of the Creative Commons Attribution License (CC BY). The use, distribution or reproduction in other forums is permitted, provided the original author(s) and the copyright owner(s) are credited and that the original publication in this journal is cited, in accordance with accepted academic practice. No use, distribution or reproduction is permitted which does not comply with these terms.
*Correspondence: Zhi-Zhao Ma, bWF6aGl6aGFvMjAwN0AxNjMuY29t; Chuan-Jie Yang, eWFuZ2NodWFuamllMjAwNEBzaW5hLmNvbQ==