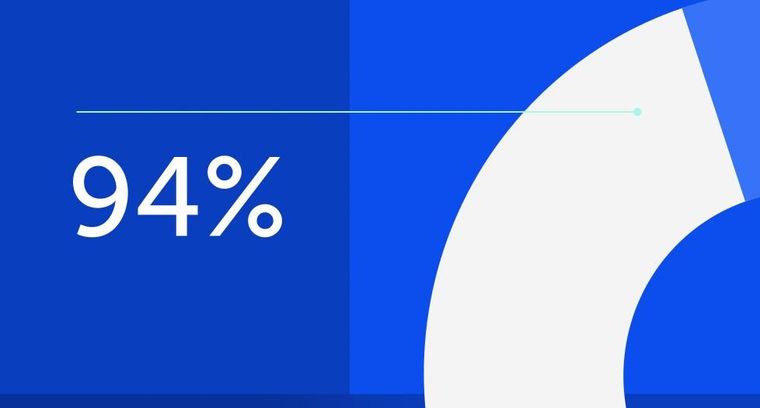
94% of researchers rate our articles as excellent or good
Learn more about the work of our research integrity team to safeguard the quality of each article we publish.
Find out more
REVIEW article
Front. Genet., 21 May 2021
Sec. Genetics of Aging
Volume 12 - 2021 | https://doi.org/10.3389/fgene.2021.686320
This article is part of the Research TopicClinical Evaluation Criteria for Aging and Aging-related MultimorbidityView all 10 articles
Aging affects most living organisms and includes the processes that reduce health and survival. The chronological and the biological age of individuals can differ remarkably, and there is a lack of reliable biomarkers to monitor the consequences of aging. In this review we give an overview of commonly mentioned and frequently used potential aging-related biomarkers. We were interested in biomarkers of aging in general and in biomarkers related to cellular senescence in particular. To answer the question whether a biological feature is relevant as a potential biomarker of aging or senescence in the scientific community we used the PICO strategy known from evidence-based medicine. We introduced two scoring systems, aimed at reflecting biomarker relevance and measurement effort, which can be used to support study designs in both clinical and research settings.
Aging can be described as a time-dependent multifactorial functional decline which affects the majority of living organisms (López-Otín et al., 2013). Very generally, it includes all processes that reduce health and survival of an individual (Fuellen et al., 2019). Notably, the chronological age and the “biological age” of an individual can differ remarkably (Kudryashova et al., 2020).
In this review, we give an overview of commonly mentioned and frequently used potential biomarkers of aging in clinical and research settings. A biomarker is a measurable feature (also called a marker) that predicts a biological state or condition (Siderowf et al., 2001). Biomarkers of aging are suggested to predict future health and survival better than chronological age. Further, they should be reproducible and they should also cause minimal trauma for the proband (Baker and Sprott, 1988; Fuellen et al., 2019). Until now no universally acceptable single-measurement biomarker of aging is known, and due to the complexity of the aging process, it is unlikely that a single universal biomarker of aging can be found. Many researchers believe that sets of biomarkers must be considered to predict aging-related outcomes with confidence (Earls et al., 2019; Kudryashova et al., 2020). Multiple markers may complement each other thereby improving the predictive power. In fact, recent comparisons have shown that composite biomarkers (also dubbed biomarker signatures) are potentially useful as biomarkers of aging. Belsky et al. (2018), Hastings et al. (2019) have both shown composite measures to be superior in predicting age-related outcomes. Belsky et al. (2018) tested the association of 7 different methods (3 epigenetics clocks, 3 composite biomarkers, and telomere length) with outcome measures such as physical functioning, cognitive decline, and subjective signs of aging, including aged facial appearance. The measures had a low agreement with each other. Nonetheless, one of the epigenetic clocks and all composite biomarkers were consistently, albeit modestly, related to the aging-related outcomes. In turn, Hastings et al. (2019) compared four different biomarkers of aging, three composite markers and telomere length, for their association with age-related outcomes such as physical, cognitive and perceptual functioning. Effect sizes tended to be larger for the composite biomarkers, compared to simple markers such as telomere length (Belsky et al., 2018; Hastings et al., 2019). Most bio markers including telomere length lack specificity regarding the mechanisms of aging processes (Hastings et al., 2017) and furthermore, the identification and validation of new biomarkers is important, which track aging-related changes in humans already by young adulthood and may also vary in their rate of change over time (Hastings et al., 2017).
Biomarkers of aging can be based on laboratory measurements (e.g., telomere length, epigenetic clocks) or phenotypic data (e.g., hand grip strength). Routine laboratory biomarkers are commonly measured in accredited clinical laboratories based on standardized methods, e.g., complete blood count, inflammation markers, or surrogate markers for the functional and structural status of organs such as creatinine (kidney function), bilirubin and alkaline phosphatase (liver and bile metabolism), liver transaminase (liver function and integrity), NT-proBNP (heart function), and troponin (heart structural integrity). Other molecular biomarkers are based on high-throughput analyses, which are often of unknown predictive value and are primarily used in a research context only. As molecular biomarkers we consider, in particular, all genome-level (“omics”) biomarkers. Non-molecular phenotypic biomarkers describe physiological functions of the body, specifically physical capability and organ function. Diagnostic biomarkers help to diagnose, confirm, or exclude a disease. In addition, biomarkers can be “prognostic,” for death or for the progression of disease or dysfunction, as well as “predictive,” for monitoring success or failure of some treatment. We do not explicitly distinguish biomarkers by this scheme, though we are mostly interested in prognosis.
We investigated the citation profiles of potential biomarkers of aging to gauge their “relevance.” This “relevance” is intended to be a proxy for their accuracy in predicting future health and survival. Longitudinal human studies investigating the usefulness of biomarkers of aging are lacking. Without such studies, there are no head-to-head comparative data that allow any direct ranking of markers by accuracy. We doubt that such longitudinal studies will become available soon, given the increasing number of new biomarker candidates, for which, in the short-term, validation is only possible in short or non-prospective studies, and given technical problems (lack of standardization and sampling under distinct conditions). Furthermore, each potential biomarker was assigned an effort score (e-score). The effort score should help to estimate the effort required by the measurement of a biomarker.
Many researchers have gone to great lengths to identify potential biomarkers of aging (Engelfriet et al., 2013; López-Otín et al., 2013; Xu and Sun, 2015; Wagner et al., 2016; Khan et al., 2017; Xia et al., 2017; Bai, 2018; Justice et al., 2018; Sahu et al., 2018; Dodig et al., 2019; Kudryashova et al., 2020). Most of the reviews in the field are focusing on specific subgroups of markers (e.g., senescence markers, molecular markers, omics-based markers, epigenetic markers, etc.). Our goal was to summarize the most often mentioned potential biomarkers of aging, and to suggest effort scores. The effort scores are to a certain degree subjective and dependent on circumstances such as experimental setting and location. The citation profiling scores are to some extent subjective, too, since publishing is influenced by scientific as well as other (sociopsychological, political etc.) considerations. The purpose of this review is not to propose a new comprehensive composite marker that measures reliably all aspects of aging. Nevertheless, this review may facilitate the selection of aging-related biomarkers for specific study objectives in terms of relevance and effort.
We considered reviews, and other articles, listed at NCBI-PubMed, which contain listings of potential biomarkers. Based on these listings, we established a set of “potential biomarkers” to begin with. We were interested in biomarkers of aging in general, and in biomarkers related to (cellular) senescence. To answer the question as to whether a “potential biomarker” is indeed an aging or (cellular) senescence biomarker we used the PICO strategy known from evidence based medicine (da Costa Santos et al., 2007). The following research question was formulated:
“Is the “potential biomarker” a biomarker mentioned in the field of aging research?”
In this context, the term “potential biomarker” is a placeholder for the reviewed biomarker. Given this question, a PICO-Scheme was built (Figure 1) and a Pubmed query was done. By the way of query processing by the Pubmed search engine, the pertinent MeSH-terms (Medical Subject Headings, NLM controlled vocabulary thesaurus used for indexing articles for PubMed) are automatically included. Due to the high number of alias names of the term “potential biomarker,” the specific MeSH-terms used by the PubMed search engine are not listed here explicitly.
Figure 1. Simplified graphic of the PICO-based queries employed at NCBI-PubMed. The search strategy was conducted as “((aging) AND (potential biomarker)) AND (biomarker)” for establishing the “c-score” and “((aging) AND (potential biomarker)) AND (biomarker),” filtered for reviews, for establishing the “rc-score.”
The number of publications returned by the search, the count-score, is referred to as the “c-score.” As a last step the results of the PubMed search were filtered for “review;” this results in the “review count score” (rc-score). The rc-score displays how often a potential biomarker was referred to in reviews, based on the above-described NCBI-PubMed queries. For senescence markers, the first query term (#1, “aging”) was replaced by “senescence.” Potential biomarkers often mentioned in reviews are assigned a high rc-score. Potential biomarkers of aging with a high PubMed citation count are not necessarily frequently mentioned in reviews, which results in a low rc-score. The rationale behind this strategy is that reviews may fulfill at least a partial filter function for relevance. The usefulness of the rc-score is expected to be the higher, the more source articles are included. By the use of this strategy we may miss, or disvalue potential biomarkers of aging published in recent studies. Additionally, widely used and long known disease markers may be overrepresented. Further, reviews have biases such as overvaluation of some high-profile studies and undervaluation of studies published in journals with low impact. Moreover, we did not normalize for date of publication. Then again, the results of recent studies are mostly not yet further confirmed. In a final step, the potential biomarkers were sorted according to their properties in the following categories: “routine laboratory,” “research laboratory” (not epigenetic), “research laboratory” (epigenetic), “physical capability and organ function” and “senescence-related” biomarkers.
We then added an effort score for each potential biomarker. We consider the following three values:
• A low e-score (-) describes a potential biomarker which is easy to sample, to handle and to process (usually automated fast and reliable measurements are available from known sources). For example, blood counts from venous blood qualify, since such blood can be sampled easily, the plasma/serum can be stored at room temperature (up to 3 h for many analytes) or in a standard freezer (≥3 month for most analytes) and be processed by equipment that is regularly available in a standard diagnostic/clinical unit or research laboratory. The costs are low to moderate (≤10 €).
• A moderate e-score (–) is assigned if one step (sampling, handling, or processing) is associated with substantial extra effort for routine laboratories. This includes sampling under special conditions, a requirement for prompt sample handling, or the need for elaborate validation.
• A high e-score (—) implies elaborate sampling (e.g., biopsy, lumbar puncture, etc.), handling (e.g., storage in liquid nitrogen) and/or processing (e.g., non-routine nucleotide or protein sequencing). The financial costs are usually high.
In detail, the e-score is based on six main attributes that is, 1: easy sampling; 2: easy sample handling (storability at room temperature (up to 3 h) and/or frozen (≥3 month)); 3: automation availability; 5: routine laboratory availability; 5: costs ≤ 10 € per test; 6: degree to which the method is oblivious to confounding and interfering factors. For each attribute that is true, we incremented the score by 1, for each potential biomarker examined (see Supplementary Data 1). The following scoring system was then used:
• 6 – 5 (T): low e-score (-)
• 4 – 3 (T): moderate e-score (s.o.)
• 2 – 0 (T): high e-score (s.o.)
Just like the rc-score, the e-score is in part subjective.
We define a “routine laboratory” biomarker as a biomarker which is commonly analyzed in accredited laboratories based on standardized methods. It may also help to diagnose, confirm, or exclude a disease. In addition, biomarkers can be prognostic, to determine disease progression, as well as predictive, for monitoring success or failure of some treatment. In this category, cytokines such as interleukins (IL) and tumor necrosis factor alpha (TNFα) and other proteins such as C-reactive protein (CRP) belong to the most often mentioned biomarkers of aging (see Table 1). Interleukins and chemokines are secreted by leukocytes and other cell types and play a major role for the function of the immune system (Brocker et al., 2010; Ben Menachem-Zidon et al., 2011). IL-6, IL-8, IL-15, and IL-1β are often associated with aging-related inflammation, chemotaxis, and production of natural killer cells. Other inflammation-linked biomarkers of aging are high sensitive CRP (hs-CRP) and TNFα (Engelfriet et al., 2013; Wagner et al., 2016; Khan et al., 2017; Niedernhofer et al., 2017; Sebastiani et al., 2017; Bai, 2018; Justice et al., 2018; Levine et al., 2018; Tanaka et al., 2018; Dodig et al., 2019; Kudryashova et al., 2020). Elevated levels of these inflammation-related biomarkers in the blood of older individuals are risk factors for age related conditions and are often subsumed with the term “inflammaging” (marked in Table 1 with [IA]) (Ferrucci and Fabbri, 2018). hs-CRP is an additional less specific marker for age-dependent atherothrombosis, featuring increasing levels in advanced stages of disease. These biomarkers are influenced by inflammation-related diseases such as coronary artery disease and (type 1) diabetes mellitus and immunological diseases. The specificity of established laboratory inflammation markers is low, considering latent as well as temporarily infections as a reason for their elevation. Another frequently mentioned group of biomarkers are related to lipids, which are cardiovascular risk factors in particular, such as total cholesterol, HDL-cholesterol, LDL-cholesterol and triglycerides (Engelfriet et al., 2013; Putin et al., 2016; Wagner et al., 2016; Niedernhofer et al., 2017; Sebastiani et al., 2017; Levine et al., 2018; Tanaka et al., 2018; Dodig et al., 2019; Mamoshina et al., 2019; Kudryashova et al., 2020). Blood lipid measurement levels vary with age. Lipid levels may influence aging and are themselves influenced by aging (Walter, 2009; Johnson and Stolzing, 2019). Studies have shown that effective treatment of dysregulated lipid levels reduces mortality and morbidity. Other routine laboratory biomarkers correlate to the function and integrity of organs, which undergo age-dependent alterations. Creatinine, cystatin C, urea, and albumin are markers of renal and liver function which declines with old age (Lindeman et al., 1985; Weinstein and Anderson, 2010). Still other biomarkers discussed here are related to glucose, and are risk predictors for metabolic age-dependent conditions: glycated hemoglobin (Hba1c) and glucose (fastened or tolerance) are indicators for diabetic risk (Dubowitz et al., 2014). Insulin, despite high fluctuation, is an often-mentioned potential biomarker of aging. In the clinical setting, the C-peptide (the cleaved part of proinsulin) is most frequently measured instead of insulin due to simpler handling, less fluctuation and its equimolar amount compared to insulin. Additionally, C-peptide levels are not influenced by any insulin injections (Chandni et al., 2013). Some more routine laboratory biomarkers of aging are listed in Table 1. Taken alone, most biomarkers do not predict aging-related outcomes with high accuracy. Several studies have shown that some specific combinations of blood-based biomarkers result in more reliable predictions for “biological age” or mortality (Levine, 2013; Liu et al., 2018a). An example is the “Phenotypic Age,” which is based on a linear combination of chronological age and nine multi-system clinical chemistry biomarkers (marked in Table 1 with [PA]) (Liu et al., 2018a,b). With the aid of these nine blood-based biomarkers, an estimation of an individual’s “biological age” is aimed for. Further blood-based biomarkers that are part of the complete blood count are used as input for software predicting “biological age,” e.g., “aging.AI,” in different versions (Zhavoronkov et al., 2019).
A research laboratory biomarker is a laboratory biomarker lacking the routine validation and/or standardization of a clinical laboratory biomarker. These markers often use nucleic acids (DNA and RNA). A frequently discussed biomarker of aging is the telomere length (López-Otín et al., 2013; Wagner et al., 2016; Khan et al., 2017; Niedernhofer et al., 2017; Xia et al., 2017; Bai, 2018; Dodig et al., 2019; Vaiserman and Krasnienkov, 2020). Telomeres are repeats of a hexametric DNA sequence capping the end of chromosomes preventing DNA damage (Aubert and Lansdorp, 2008). Telomeres shorten with each cell division or due to cell stress. This attrition ultimately leads to cellular senescence and can thus function as a biomarker for replicative aging in mitotic cells. Specifically, leukocyte telomere length is used as a potential biomarker for healthy aging (Bekaert et al., 2005; Lulkiewicz et al., 2020), whereby shortened telomeres may represent cellular exhaustion and/or increased cell stress for leukocytes or other cells in the body as a surrogate; however the usefulness of telomere length as a biomarker of aging is discussed critically. Kahl & Allen et al. summed up and described different methods measuring telomere length, covering imaging based methods (TCA, TRF, Q-FISH, Flow-FISH) and PCR-based methods (qPCR) (Kahl et al., 2020). Another very good overview is provided in the review of Lai et al. (2018), comparing known methods and additionally describing methods to measure the shortest telomeres (STELA, TeSLA). Telomere length measurements are characterized by poor standardization and limited comparability. Moreover, telomere length in leucocytes is only partially a surrogate marker for telomere length in other organs. Telomere length is classified as highly relevant according to the rc-score, which exemplifies the limitations of such a score. The telomere length is certainly very interesting from a physiological point of view, but whether it can serve as a relevant (or even accurate) biomarker is questionable. Telomere shortening can only be counteracted in a few cells (germ cells, stem cells, some immune cells) by telomerase, an enzyme that is able to lengthen the telomeres (Shay, 2016). Telomerase is induced in most tumor cells, which renders its induction risky. Nevertheless, telomerase activity plays an important role in longevity. A study showed that centenarians have a particularly active telomerase in T-cells compared to healthy 67 – 80 year old donors (Tedone et al., 2019), which raises the question if telomerase activity could be a biomarker for aging. Another DNA-linked potential biomarker of aging is the degree of DNA damage (Wagner et al., 2016; Khan et al., 2017; Niedernhofer et al., 2017; Dodig et al., 2019). DNA damage accumulates with age, fostering the development of age-related pathologies such as malignancies, cellular senescence and inflammation (Da Silva and Schumacher, 2019). In particular, mitochondrial DNA damage is a factor leading to mitochondrial dysfunction, which is also often related to aging processes (López-Otín et al., 2013; Khan et al., 2017; Niedernhofer et al., 2017; Sahu et al., 2018; Dodig et al., 2019). Mitochondrial DNA has less repair capacity and a higher mutation rate compared to nuclear DNA (Haas, 2019). Mitochondrial, metabolic and respiratory dysfunction can, in addition to exogenous stress, lead to the production of excess reactive oxygen species (ROS) (López-Otín et al., 2013; Wagner et al., 2016; Santos et al., 2018; Dodig et al., 2019; Kudryashova et al., 2020). Potential negative effects of excess ROS are dysregulated protein homeostasis, accumulation of oxidative modified proteins and advanced glycation/lipid peroxidation end products and loss of function of cellular protein maintenance systems. It has been shown that autophagy is another modulator of aging processes. A tissue-specific overexpression of autophagy genes can be sufficient to extend lifespan by preventing the accumulation of dysfunctional cellular components (Simonsen et al., 2008; Hansen et al., 2018; Kumsta et al., 2019). Such hallmarks of aging as discussed here were observed in various organs and tissues (Stadtman and Berlett, 1997; Baraibar and Friguet, 2013; Davies et al., 2017). In response to mitochondrial dysfunction, growth differentiation factor 15 (GDF15) may be generated, protecting tissues against inflammation by suppressing T-cell activation and mediating release of cytokines (Moon et al., 2020). On this basis, GDF15 was suggested as a potential aging biomarker (Justice et al., 2018; Tanaka et al., 2018; Basisty et al., 2020; Sebastiani et al., 2021). TGF-β and GDF11 (from the same protein superfamily) are also regarded as proteins playing a role in aging-associated cellular senescence, frailty, stem cell aging and fibrosis as well as surgical risk in older adults (Schafer et al., 2016; Khan et al., 2017; Niedernhofer et al., 2017; Bai, 2018; Dodig et al., 2019; Tominaga and Suzuki, 2019). Circulating biomarkers based on extracellular vesicles (EVs), including exosomes, microvesicles and apoptotic bodies, are moving into focus for the prediction of age-related diseases (Yáñez-Mó et al., 2015; Kalluri and LeBleu, 2020; Noren Hooten, 2020). Moreover, changes in the community composition of the skin microbiome have been related to age (Kim et al., 2019), and more precise information is obtained when considering the gut microbiome (Maynard and Weinkove, 2018; Guest, 2019; Askarova et al., 2020). In general, there is now ample evidence that microbiome dysbiosis is associated to aging and longevity (Kim and Benayoun, 2020). Other frequently mentioned potential research laboratory biomarkers of aging are listed in Table 2.
Table 2. Frequently mentioned potential “research lab” biomarkers based on non-epigenetic measurements.
The epigenome is a dynamic system playing a major role in aging. Methylation of the DNA (DNAm) and histone modifications ensure appropriate high fidelity gene expression; both change with chronological age and with chronic diseases over time. Even if it is currently not known to what extend these changes cause aging, they can be useful, e.g., for chronological age prediction (Ashapkin et al., 2019). Generally, aging is associated with global hypomethylation and local hypermethylation. For the analysis of DNA methylation, various so-called epigenetic clocks were developed. Famous examples for first generation epigenetic clocks are the Horvath clock, Weidner Clock, and Hannum clock (see Levine, 2019). Basically, these clocks are considering specific sets of CpG sites with respect to their DNA methylation status, as a molecular correlate to predict chronological age (Horvath and Raj, 2018; Bell et al., 2019). Hannum’s clock is based on blood samples and uses 71 CpG sites measured from the Illumina 450k array. Age-related shifts in blood cells are per se informative for changes in chronological age (Hannum et al., 2013; Bell et al., 2019), but they are also considered by some epigenetic clocks. The Horvath clock is specifically designed to be used across multiple tissues, whereby it captures 353 CpG sites on a Illumina 27k array (Horvath, 2013; Bell et al., 2019). Second-generation epigenetic clocks learn to associate clinical data with methylation status, e.g., the DNAm PhenoAge (Levine et al., 2018) or DNAm GrimAge (Lu et al., 2019). More specifically, second-generation epigenetic clocks such as GrimAge and PhenoAge were developed to learn biological endpoints (which in turn are suggested to relate to “biological age”) directly. Recently, it was shown that cytosines, whose methylation levels change with age across mammalian species, are involved in mammalian developmental processes, suggesting that aging is indeed evolutionarily conserved and coupled to developmental processes (Lu et al., 2021). Histone modifications such as H4K16 acetylation, H4K20 methylation, H3K4 methylation, H3K9 methylation and H3K27 methylation were also proposed as epigenetic chronological age predictors (López-Otín et al., 2013; Moskalev, 2019), and these modifications can be influenced by ROS (Wu and Ni, 2015). However, data regarding specific outcomes are scarce. Histone modifications and DNA methylation are closely related to chromatin remodeling and changes in chromatin architecture (Oberdoerffer and Sinclair, 2007; López-Otín et al., 2013). The above mentioned EVs also carry extracellular RNA (exRNA) which changes with age (Dluzen et al., 2017; Noren Hooten, 2020). Other types of RNA such as microRNAs (miRNA), that function in RNA silencing and posttranscriptional regulation of gene expression, and which are often isolated from peripheral blood mononuclear cells (PBMCs), are also reflecting aging and are used to predict age-related diseases (Evans et al., 2010; Noren Hooten et al., 2010; Reid et al., 2011; Kumar et al., 2017). Examples for age-related miRNAs are: miR-34a, miR-9, miR-132, miR-212, miR-21, miR-96, miR-145 (Halper et al., 2015; Budzinska et al., 2016; Owczarz et al., 2017; Hadar et al., 2018). Frequently mentioned epigenetic based biomarkers are covered in Table 3.
Physical and cognitive function are important markers for aging processes (Fuellen et al., 2019), as are anthropometric measurements. For example, brain function and integrity are influenced by aging and aging-associated diseases. Aging encompasses changes at the structural, functional, and molecular levels of most cells, tissues and organ systems. Gradual loss of the maintenance functions of tissues is a characteristic of aging (López-Otín et al., 2013). Non-blood aging markers are not the primary focus of this review. However, due to the ease of implementation, certain analyses can supplement studies in which general metabolic and physiological age aspects need to be measured. These tests may include grip strength or easy to perform locomotor function tests as walking speed, timed up and go test or the standing balance test. As aging is associated with body composition, biomarkers such as BMI or fat and muscle indices should be recorded. Bone mass declines with age in both men and women and may be analyzed (Khosla et al., 1996; Khosla et al., 2006; Riggs et al., 2008; Keaveny et al., 2010). Frequently used other anthropometric markers are muscle mass, waist circumference (Wagner et al., 2016), and (systolic) blood pressure (Pinto, 2007; Crimmins et al., 2008). These measurements are mostly carried out without much effort. Most of these markers are also closely related, and they predict frailty in particular. Frailty is age-dependent and often associated with chronic disorders, resulting in an increasing need for diagnostic, nursing, and therapeutic interventions. Grip strength is a predictor of frailty, all-cause mortality and morbidity (Syddall et al., 2003). Strength itself may provide protection against mortality (Rantanen et al., 2000). With age, a decline in physical and cognitive function is frequently observed, as can be seen in the lifespan data of athletes in comparison to controls (Donato et al., 2003; Baker and Tang, 2010; Harridge and Lazarus, 2017). This is observed for muscle mass but also for physiological changes in organ systems leading to age-related diseases (Boss and Seegmiller, 1981). Additionally, various non-invasive methods were proposed to monitor the cardiovascular system and the vascular wall structure and elasticity, including electrocardiogram (ECG), intima media thickness ultrasonography and ultrasound techniques to evaluate endothelium-dependent vasodilation (EDV). Many if not all aspects of cognitive function change with age, which can be measured in complex tests or in rather simple questionnaires. Frequently mentioned non-blood and biomarkers are covered in Table 4.
Cellular senescence is a cell state characterized by the cessation of cell division, reached through a combination of telomere shortening, oxidative stress and oncogenic stress. It can also be induced by each of these factors alone, and by DNA damage signaling pathways, with ATM and ATR as primary sensors of DNA double and single-strand damage. As a species-specific aging mechanism, telomere attrition limits the number of divisions. The successive shortening of the chromosomal telomeres with each cell cycle (caused by the so-called end replication problem and often referred to as replicative senescence) is observed in large long-lived species and cooperates with other aging mechanisms to activate the senescence program. These signaling pathways are funneled down to activate the p53 protein, the Rb protein, or both. Once the senescence program is activated, a series of changes in morphology, function, and gene expression takes place, associated with autocrine and paracrine effects of secreted cytokines, macromolecular damage, and altered metabolism (Gorgoulis et al., 2019). The limitation of investigating “senescence-related” biomarkers lies in the cumbersome extraction of appropriate patient samples. Furthermore until now, there is no clinically validated senescence-related biomarker available. Indirect biomarkers of cellular senescence can be measured in blood samples, such as markers of proliferation status or components of the SASP (Castleab et al., 1999). However, these measurements are non-specific and only a proxy of the senescence status of the cells providing the sample. Moreover, they cannot reflect the senescence status of the entire organism (Schafer et al., 2020; Yousefzadeh et al., 2020). Additionally, often it is not clear from which tissue(s) the markers originate, since markers found in blood could originate from almost anywhere. The proliferation status of senescent cells can also be gauged by the expression of various cell-cycle-related markers (p16, p21, p53) (Jung et al., 2010; Baker et al., 2011; Burd et al., 2013; Qian and Chen, 2013). On one hand, cellular senescence is a cause or bystander of many age-related diseases contributing to inflammation and/or tumorigenesis (Milanovic et al., 2018; Song et al., 2020). On the other hand, cellular senescence and its generally irreversible loss of proliferative potential is considered necessary for tissue remodeling during development, tissue homeostasis, wound healing as well as for tumor prevention (Ovadya and Krizhanovsky, 2018). Identification and characterization of cellular senescence markers receives more and more attention. However, the difficulty in characterizing cellular senescence by biomarkers and the lack of blood markers or other easily accessible specimen still limit the usefulness of the concept (Khan et al., 2017; Hernandez-Segura et al., 2018).
The senescence-associated secretory phenotype (SASP) is characterized by an enrichment of various inflammatory markers (Ghosh and Capell, 2016; Hernandez-Segura et al., 2018; Basisty et al., 2020) and can be detected in serum or EDTA plasma of probands using ELISA (Tanaka et al., 2018), which is readily available. Some important SASP markers are: interleukins (IL-6, IL-7, IL-8, and IL-15); chemokines (CCL3, CCL4) as well as growth factors (GDF-15 and activin A) (Schafer et al., 2020). Nevertheless, the secretion of these markers is highly heterogeneous and regulated at many levels, making it difficult to consider them as well-standardized biomarkers of cellular senescence (Hernandez-Segura et al., 2017). Assignment of inflammatory markers to the SASP or to another aging-related physical or physiological status (e.g., inflammaging) as well as to other diseases with permanent inflammation status (e.g., infections, tumors) is often difficult (Serrano et al., 1997; Barbé-Tuana et al., 2020). For the investigation of some cellular senescence markers, it is advantageous to have cells in culture, e.g., fibroblasts or PBMCs (Migliaccio and Palis, 2011; Riedhammer et al., 2016; Wang and Dreesen, 2018). For fibroblast isolation, a punch skin biopsy (Zuber, 2002; Vangipuram et al., 2013) may be taken, which may represent a notable trauma for the probands (Vangipuram et al., 2013). Another disadvantage of fibroblast cell culture is the long period of culturing of around 50 days, before investigations can be completed (Vangipuram et al., 2013), the difficult standardization, and other confounders such a population doublings in cell culture conditions. Consequently, this procedure is only used for research purposes. Much more easily done is the isolation and cultivation of PBMCs from blood samples including lymphocytes (T-cells, B-cells, NK-cells) (Migliaccio and Palis, 2011; Riedhammer et al., 2016). Senescent cells, especially fibroblasts in culture, become larger in size, flattened in shape (Figure 2) and can display a disorganized nuclear envelope mediated by reduction of lamin B1 expression (Nishio et al., 2001; Freund et al., 2012). These features of cellular morphology are features of senescent cells in general. Moreover, progerin (a mutated form of lamin A) associated with the premature aging syndrome Hutchinson-Gilford (DeBusk, 1972) can be detected in fibroblasts, also at low levels due to non-premature aging (Scaffidi and Misteli, 2006). A recent study has shown that elevated blood levels of progerin can be detected in people with obesity, suggesting a cause for premature aging of the cardiovascular system. Therefore, progerin might be measured in blood samples and be adapted for diagnostic measurements (Messner et al., 2019). Another marker of cellular senescence, which can be investigated in cultured cells (e.g., based on punch skin biopsy), are senescent-associated histone foci (SAHF). These darker regions within the nucleus of senescent cells can be detected as compacted DNA foci. While DNA staining of healthy and young human cells is relatively uniform, senescent cells show up to 50 punctuated DAPI-stained DNA foci (Narita et al., 2003; Aird and Zhang, 2013). Additionally, SAHF are enriched in markers of heterochromatin (H3K9Me3 and HP1γ) (Figure 2; Sharpless and Sherr, 2015). Furthermore, senescent cells usually have an increased lysosomal content, which can be detected cytochemically by measuring senescence-associated β-galactosidase (SA-βGal) activity at a pH of 6.0 (Debacq-Chainiaux et al., 2009; Hernandez-Segura et al., 2018; Figure 2). Frequently mentioned senescence related biomarkers are covered in Table 5.
Table 5. Frequently mentioned biomarkers (routine or research laboratory) associated with cellular senescence.
Figure 2. Representative microscopy pictures of cellular senescence biomarkers. Note the clear difference in overt morphology due to age of the respective individual at biopsy (cells are larger in size and more flattened; cells were immunostained for vimentin.) SAHF are formed in old fibroblasts and are enriched in heterochromatin markers. (Cells were immunostained for H3K9Me3.) SA-ßGal activity increases with age at pH 6.0. (Cells were treated with X-Gal to make SA-βGal visible in senescent cells.); scale bar: 10 μM for morphology and SAHF column, 50 μM for SA-βGAL column.
In summary, there is currently no biomarker for cellular senescence that can easily be used for diagnostics or prediction. Therefore, in the future, more attention should be paid to the research and establishment of diagnostically applicable biomarkers of cellular senescence. Potentially, measuring senescence markers after cell isolation by flow cytometry can be further developed for use in diagnostics (Adewoye et al., 2020).
Transcriptomic (gene expression) changes also accompany the aging process, and transcriptomic clocks were trained to predict chronological age. Some clocks were trained on samples belonging to a specific tissue, e.g., the clocks by Peters et al. and Huan et al. are based on whole blood or PBMC, the clocks by Fleischer et al. and LaRocca et al. are based on skin fibroblasts, while the one by Mamoshina et al. (2019) is based on muscle tissue, and the clocks by Ren and Kuan (2020); Shokhirev and Johnson (2020) are based on multiple tissues (Peters et al., 2015; Fleischer et al., 2018; Huan et al., 2018; Mamoshina et al., 2018; LaRocca et al., 2020; Ren and Kuan, 2020; Shokhirev and Johnson, 2020). Most of these clocks are taking mRNA as input, while some employ microRNAs (Huan’s clock) or repetitive elements (LaRocca’s clock). Shokhirev and Johnson’s pan-tissue transcriptomic clock is based on a large-scale meta-analysis of transcriptomic data from the Sequence Read Archive. 3060 multi-tissue samples were used as input, and a random forest model was able to learn chronological age with high accuracy (Shokhirev and Johnson, 2020). On a practical level, the e-score of transcriptomic clocks strongly depends on the tissue used for the analysis. Perhaps the best specimen in this context is whole blood or its components. Peripheral blood mononuclear cells (PBMC) are easily accessible as well, and PBMC transcriptomes were measured in the context of nutrigenomic interventions and proved to be sensitive to these (Afman et al., 2014; Herrera-Marcos et al., 2017; Bottero and Potashkin, 2020).
Appropriate preanalytics and exact description of sampling and methodology are critical for reliable results and reproducibility, particularly the use of validated tests and procedures. Details are beyond the scope of this review and are summarized elsewhere (Rai and Vitzthum, 2006). These include blood draw, blood collection, blood processing and storage. We recommend, whenever possible, a morning (before 10 am, and fasting) blood draw to control diurnal fluctuation; Mondays should be avoided due to weekend-specific confounders. For blood collection a 21-gauge needle and slow aspiration is preferred to avoid the activation of coagulation. Needle material and tube material may impact assays, e.g., in the measurement of trace elements. A standardized description of venipuncture and sampling is strongly recommended. There are advantages and disadvantages for serum and plasma respectively (Rai and Vitzthum, 2006). If possible, both serum, heparin plasma, double centrifuged citrate plasma and, if necessary, EDTA blood (e.g., for DNA preparation, telomere length measurements, etc.) should be collected and prepared in fractions, and, if necessary/possible, frozen. Blood counts and other highly standardized routine methods should be performed immediately. In general, tests with high inter-assay variability should be processed batch-wise based on initially frozen samples, provided the samples can be frozen for the respective test. Data on the stability of the frozen samples and on potential confounding by the freeze thawing procedures should be recorded. The position of the probands during blood drawing (standing, lying, sitting) influences almost all analyte values (due to water shifts between vessel and interstitial space). Sampling from probands at a stable position (horizontal or sitting for some minutes) is recommended. Serum/plasma should not be in contact with blood cells for more than 2 h (Kiechle, 2010). Long-term storage should be at –80°C or in liquid nitrogen (Rai et al., 2005).
Aging is a complex process and not fully understood. In this review we propose the “rc-score” and the “e-score” as tools for gauging the suitability of biomarkers of aging, with a focus on clinical settings. Together the two scores reflect the presumed relevance of a potential biomarker of aging, and the effort needed for its measurement. The “e-score” must be seen in relation to the equipment and possibilities of the laboratory performing the measurements. Routine blood biomarkers and easy-to-measure phenotypic markers such as blood pressure often correlate well with age-dependent organ/metabolic dysfunction, including cardiovascular, renal, or diabetic risk. The most cited non-epigenetic biomarkers are telomere length, amount of DNA-damage and mitochondrial dysfunction, reflecting aging-related changes on the genome and cellular level. Telomere length is classified as the most relevant according to rc-score, which exemplary shows the limitations of such a score. Telomere attrition is very interesting from a physiological point of view, but whether it can serve as an accurate biomarker is still questionable due to methodological problems. Novel telomere analyses such as TESLA have yet to be validated. Molecular markers such as cytokines/chemokines and sirtuins show a relatively low rc-score but are of clinical and scientific interest. The strong presence of BMI in reviews might also be attributed to the fact that potentially better markers such as the formal criteria of metabolic syndrome were not considered in many studies because the effort is higher for these. Methylation of CpG sites is among the most interesting candidate biomarkers of aging, but it awaits further validation in longitudinal studies. Functional decline affects all types of tissues and has a negative effect on grip strength and mobility, which can both be used as biomarkers of aging. Cellular senescence is a fundamental part of the aging process. However, it is difficult to analyze to date in a clinical setting due to difficulties in sampling and specificity. A combination of routine laboratory, epigenetic, non-epigenetic and physical capability and organ function biomarkers, and possibly senescence markers, may be the key to a valid composite biomarker of aging. Yet, a standardized (composite) biomarker of aging that specifically measures all important aspects of the aging process has not yet been found.
The general question of which biomarkers should be used in clinical trials to study aging and (cellular) senescence remains difficult and clearly requires further systematic longitudinal studies. Nevertheless, there are numerous potential markers, which differ concerning their difficulty to sample, to handle and to process, including significant differences in costs. Several parameters can be used to select biomarkers of aging for clinical trials, and we point out some specific issues in Table 6.
AHa, CH, and GF wrote the manuscript with input of RS, AHe, and MW. AHa did the analyses underlying the preparation of the tables. All authors approved the final version of the manuscript and made substantial, direct and intellectual contribution to the work, and approved it for publication.
Writing of this review was supported by Karls Erdbeerhof, Rövershagen, Germany, and the “Interdisziplinäre Fakultät” (Department AGIS) of the Rostock University. AHe was supported by the Hermann and Lilly-Schilling Stiftung für medizinische Forschung im Stifterverband. GF was further supported by the BMBF (FKZ 01ZX1903A and 1DS19049) and the European Commission (Aging with elegans, grant agreement 633589).
The authors declare that the research was conducted in the absence of any commercial or financial relationships that could be construed as a potential conflict of interest.
We would like to thank Dr. Andrea Maier for her input and ideas to this topic.
The Supplementary Material for this article can be found online at: https://www.frontiersin.org/articles/10.3389/fgene.2021.686320/full#supplementary-material
Adewoye, A. B., Tampakis, D., Follenzi, A., and Stolzing, A. (2020). Multiparameter flow cytometric detection and quantification of senescent cells in vitro. Biogerontology 21, 773–786. doi: 10.1007/s10522-020-09893-9
Afman, L., Milenkovic, D., and Roche, H. M. (2014). Nutritional aspects of metabolic inflammation in relation to health-insights from transcriptomic biomarkers in PBMC of fatty acids and polyphenols. Mol. Nutr. Food Res. 58, 1708–1720. doi: 10.1002/mnfr.201300559
Aird, K. M., and Zhang, R. (2013). Detection of senescence-associated heterochromatin foci (SAHF). Methods Mol. Biol. 965, 185–196. doi: 10.1007/978-1-62703-239-1_12
Ashapkin, V. V., Kutueva, L. I., and Vanyushin, B. F. (2019). Epigenetic clock: just a convenient marker or an active driver of aging? Adv. Exp. Med. Biol. 1178, 175–206. doi: 10.1007/978-3-030-25650-0_10
Askarova, S., Umbayev, B., Masoud, A.-R., Kaiyrlykyzy, A., Safarova, Y., Tsoy, A., et al. (2020). The links between the gut microbiome, aging, modern lifestyle and Alzheimer’s disease. Front. Cell. Infect. Microbiol. 10:104. doi: 10.3389/fcimb.2020.00104
Aubert, G., and Lansdorp, P. M. (2008). Telomeres and aging. Physiol. Rev. 88, 557–579. doi: 10.1152/physrev.00026.2007
Bai, X. (2018). “Biomarkers of Aging,” in Aging and Aging-Related Diseases: Mechanisms and Interventions, ed. Z. Wang (Singapore: Springer Singapore), 217–234.
Baker, A. B., and Tang, Y. Q. (2010). Aging performance for masters records in athletics, swimming, rowing, cycling, triathlon, and weightlifting. Exp. Aging Res. 36, 453–477. doi: 10.1080/0361073X.2010.507433
Baker, D. J., Wijshake, T., Tchkonia, T., LeBrasseur, N. K., Childs, B. G., van de Sluis, B., et al. (2011). Clearance of p16Ink4a-positive senescent cells delays ageing-associated disorders. Nature 479, 232–236. doi: 10.1038/nature10600
Baker, G. T., and Sprott, R. L. (1988). Biomarkers of aging. Exp. Gerontol. 23, 223–239. doi: 10.1016/0531-5565(88)90025-3
Baraibar, M. A., and Friguet, B. (2013). Oxidative proteome modifications target specific cellular pathways during oxidative stress, cellular senescence and aging. Exp. Gerontol. 48, 620–625. doi: 10.1016/j.exger.2012.10.007
Barbé-Tuana, F., Funchal, G., Schmitz, C. R. R., Maurmann, R. M., and Bauer, M. E. (2020). The interplay between immunosenescence and age-related diseases. Semin. Immunopathol. 42, 545–557. doi: 10.1007/s00281-020-00806-z
Basisty, N., Kale, A., Jeon, O. H., Kuehnemann, C., Payne, T., Rao, C., et al. (2020). A proteomic atlas of senescence-associated secretomes for aging biomarker development. PLoS Biol. 18:e3000599. doi: 10.1371/journal.pbio.3000599
Bekaert, S., De Meyer, T., and van Oostveldt, P. (2005). Telomere attrition as ageing biomarker. Anticancer Res. 25, 3011–3021.
Bell, C. G., Lowe, R., Adams, P. D., Baccarelli, A. A., Beck, S., Bell, J. T., et al. (2019). DNA methylation aging clocks: challenges and recommendations. Genome Biol. 20:249. doi: 10.1186/s13059-019-1824-y
Belsky, D. W., Moffitt, T. E., Cohen, A. A., Corcoran, D. L., Levine, M. E., Prinz, J. A., et al. (2018). Eleven telomere, epigenetic clock, and biomarker-composite quantifications of biological aging: do they measure the same thing? Am. J. Epidemiol. 187, 1220–1230. doi: 10.1093/aje/kwx346
Ben Menachem-Zidon, O., Avital, A., Ben-Menahem, Y., Goshen, I., Kreisel, T., Shmueli, E. M., et al. (2011). Astrocytes support hippocampal-dependent memory and long-term potentiation via interleukin-1 signaling. Brain Behav. Immun. 25, 1008–1016. doi: 10.1016/j.bbi.2010.11.007
Boss, G. R., and Seegmiller, J. E. (1981). Age-related physiological changes and their clinical significance. West. J. Med. 135, 434–440.
Bottero, V., and Potashkin, J. A. (2020). A comparison of gene expression changes in the blood of individuals consuming diets supplemented with olives, nuts or long-chain omega-3 fatty acids. Nutrients 12:3765. doi: 10.3390/nu12123765
Brocker, C., Thompson, D., Matsumoto, A., Nebert, D. W., and Vasiliou, V. (2010). Evolutionary divergence and functions of the human interleukin (IL) gene family. Hum. Genomics 5, 30–55. doi: 10.1186/1479-7364-5-1-30
Budzinska, M., Owczarz, M., Pawlik-Pachucka, E., Roszkowska-Gancarz, M., Slusarczyk, P., and Puzianowska-Kuznicka, M. (2016). miR-96, miR-145 and miR-9 expression increases, and IGF-1R and FOXO1 expression decreases in peripheral blood mononuclear cells of aging humans. BMC Geriatr. 16:200. doi: 10.1186/s12877-016-0379-y
Burd, C. E., Sorrentino, J. A., Clark, K. S., Darr, D. B., Krishnamurthy, J., Deal, A. M., et al. (2013). Monitoring tumorigenesis and senescence in vivo with a p16(INK4a)-luciferase model. Cell 152, 340–351. doi: 10.1016/j.cell.2012.12.010
Castleab, S. C., Uyemuraabc, K., Crawford, W., Wongab, W., Klaustermeyer, W., and Makinodan, T. (1999). Age-related impaired proliferation of peripheral blood mononuclear cells is associated with an increase in both IL-10 and IL-12. Exp. Gerontol. 34, 243–252. doi: 10.1016/s0531-5565(98)00064-3
Chandni, R., Paul, B. J., Udayabhaskaran, V., and Ramamoorthy, K. P. (2013). A study of non-obese diabetes mellitus in adults in a tertiary care hospital in Kerala, India. Int. J. Diabetes Dev. Ctries 33, 83–85. doi: 10.1007/s13410-013-0113-7
Cole, J. H., Leech, R., and Sharp, D. J. (2015). Prediction of brain age suggests accelerated atrophy after traumatic brain injury. Ann. Neurol. 77, 571–581. doi: 10.1002/ana.24367
Crimmins, E., Vasunilashorn, S., Kim, J. K., and Alley, D. (2008). “Chapter 5 Biomarkers related to aging in human populations,” in Advances in Clinical Chemistry, ed. S. M. Gregory (San Diego, CA: Elsevier), 161–216.
da Costa Santos, C. M., de Mattos Pimenta, C. A., and Nobre, M. R. C. (2007). The PICO strategy for the research question construction and evidence search. Rev. Lat. Am. Enfermagem. 15, 508–511. doi: 10.1590/s0104-11692007000300023
Da Silva, P. F. L., and Schumacher, B. (2019). DNA damage responses in ageing. Open Biol. 9:190168. doi: 10.1098/rsob.190168
Davies, J. M. S., Cillard, J., Friguet, B., Cadenas, E., Cadet, J., Cayce, R., et al. (2017). The oxygen paradox, the french paradox, and age-related diseases. Geroscience 39, 499–550. doi: 10.1007/s11357-017-0002-y
Debacq-Chainiaux, F., Erusalimsky, J. D., Campisi, J., and Toussaint, O. (2009). Protocols to detect senescence-associated beta-galactosidase (SA-betagal) activity, a biomarker of senescent cells in culture and in vivo. Nat. Protoc. 4, 1798–1806. doi: 10.1038/nprot.2009.191
DeBusk, F. L. (1972). The Hutchinson-Gilford progeria syndrome. J. Pediatr. 80, 697–724. doi: 10.1016/s0022-3476(72)80229-4
Dluzen, D. F., Noren Hooten, N., and Evans, M. K. (2017). Extracellular RNA in aging. Wiley Interdiscip. Rev. RNA 8:e1385. doi: 10.1002/wrna.1385
Dodig, S., Čepelak, I., and Pavić, I. (2019). Hallmarks of senescence and aging. Biochem. Med. (Zagreb) 29, 30501. doi: 10.11613/BM.2019.030501
Donato, A. J., Tench, K., Glueck, D. H., Seals, D. R., Eskurza, I., and Tanaka, H. (2003). Declines in physiological functional capacity with age: a longitudinal study in peak swimming performance. J. Appl. Physiol. (1985) 94, 764–769. doi: 10.1152/japplphysiol.00438.2002
Dubowitz, N., Xue, W., Long, Q., Ownby, J. G., Olson, D. E., Barb, D., et al. (2014). Aging is associated with increased HbA1c levels, independently of glucose levels and insulin resistance, and also with decreased HbA1c diagnostic specificity. Diabet. Med. 31, 927–935. doi: 10.1111/dme.12459
Earls, J. C., Rappaport, N., Heath, L., Wilmanski, T., Magis, A. T., Schork, N. J., et al. (2019). Multi-Omic biological age estimation and its correlation with wellness and disease phenotypes: a longitudinal study of 3,558 individuals. J. Gerontol. A Biol. Sci. Med. Sci. 74, S52–S60. doi: 10.1093/gerona/glz220
Engelfriet, P. M., Jansen, E. H. J. M., Picavet, H. S. J., and Dollé, M. E. T. (2013). Biochemical markers of aging for longitudinal studies in humans. Epidemiol. Rev. 35, 132–151. doi: 10.1093/epirev/mxs011
Evans, M. K., Lepkowski, J. M., Powe, N. R., LaVeist, T., Kuczmarski, M. F., and Zonderman, A. B. (2010). Healthy aging in neighborhoods of diversity across the life span (HANDLS): overcoming barriers to implementing a longitudinal, epidemiologic, urban study of health, race, and socioeconomic status. Ethn. Dis. 20, 267–275.
Ferrucci, L., and Fabbri, E. (2018). Inflammageing: chronic inflammation in ageing, cardiovascular disease, and frailty. Nat. Rev. Cardiol. 15, 505–522. doi: 10.1038/s41569-018-0064-2
Fleischer, J. G., Schulte, R., Tsai, H. H., Tyagi, S., Ibarra, A., Shokhirev, M. N., et al. (2018). Predicting age from the transcriptome of human dermal fibroblasts. Genome Biol. 19:221. doi: 10.1186/s13059-018-1599-6
Freund, A., Laberge, R.-M., Demaria, M., and Campisi, J. (2012). Lamin B1 loss is a senescence-associated biomarker. Mol. Biol. Cell 23, 2066–2075. doi: 10.1091/mbc.E11-10-0884
Fuellen, G., Jansen, L., Cohen, A. A., Luyten, W., Gogol, M., Simm, A., et al. (2019). Health and aging: unifying concepts, scores, biomarkers and pathways. Aging Dis. 10, 883–900. doi: 10.14336/AD.2018.1030
Ghosh, K., and Capell, B. C. (2016). The senescence-associated secretory phenotype: critical effector in skin cancer and aging. J. Invest. Dermatol. 136, 2133–2139. doi: 10.1016/j.jid.2016.06.621
Gorgoulis, V., Adams, P. D., Alimonti, A., Bennett, D. C., Bischof, O., Bishop, C., et al. (2019). Cellular senescence: defining a path forward. Cell 179, 813–827. doi: 10.1016/j.cell.2019.10.005
Guest, P. C. (2019). Reviews on Biomarker Studies in Aging and Anti-Aging Research. Cham: Springer International Publishing.
Haas, R. H. (2019). Mitochondrial dysfunction in aging and diseases of aging. Biology (Basel) 8:48. doi: 10.3390/biology8020048
Hadar, A., Milanesi, E., Walczak, M., Puzianowska-Kuźnicka, M., Kuźnicki, J., Squassina, A., et al. (2018). SIRT1, miR-132 and miR-212 link human longevity to Alzheimer”s disease. Sci. Rep. 8:8465. doi: 10.1038/s41598-018-26547-6
Halper, B., Hofmann, M., Oesen, S., Franzke, B., Stuparits, P., Vidotto, C., et al. (2015). Influence of age and physical fitness on miRNA-21, TGF-β and its receptors in leukocytes of healthy women. Exerc. Immunol. Rev. 21, 154–163.
Hannum, G., Guinney, J., Zhao, L., Zhang, L., Hughes, G., Sadda, S., et al. (2013). Genome-wide methylation profiles reveal quantitative views of human aging rates. Mol. Cell 49, 359–367. doi: 10.1016/j.molcel.2012.10.016
Hansen, M., Rubinsztein, D. C., and Walker, D. W. (2018). Autophagy as a promoter of longevity: insights from model organisms. Nat. Rev. Mol. Cell. Biol. 19, 579–593. doi: 10.1038/s41580-018-0033-y
Harridge, S. D. R., and Lazarus, N. R. (2017). Physical activity, aging, and physiological function. Physiology (Bethesda) 32, 152–161. doi: 10.1152/physiol.00029.2016
Hastings, W. J., Shalev, I., and Belsky, D. W. (2017). Translating measures of biological aging to test effectiveness of geroprotective interventions: what can we learn from research on telomeres? Front. Genet. 8:164. doi: 10.3389/fgene.2017.00164
Hastings, W. J., Shalev, I., and Belsky, D. W. (2019). Comparability of biological aging measures in the National health and nutrition examination study, 1999-2002. Psychoneuroendocrinology 106, 171–178. doi: 10.1016/j.psyneuen.2019.03.012
Hernandez-Segura, A., de Jong, T. V., Melov, S., Guryev, V., Campisi, J., and Demaria, M. (2017). Unmasking transcriptional heterogeneity in senescent cells. Curr. Biol. 27, 2652–2660.e4. doi: 10.1016/j.cub.2017.07.033
Hernandez-Segura, A., Nehme, J., and Demaria, M. (2018). Hallmarks of cellular senescence. Trends Cell Biol. 28, 436–453. doi: 10.1016/j.tcb.2018.02.001
Herrera-Marcos, L., Lou-Bonafonte, J., Arnal, C., Navarro, M., and Osada, J. (2017). Transcriptomics and the mediterranean diet: a systematic review. Nutrients 9:472. doi: 10.3390/nu9050472
Horvath, S. (2013). DNA methylation age of human tissues and cell types. Genome Biol. 14:R115. doi: 10.1186/gb-2013-14-10-r115
Horvath, S., and Raj, K. (2018). DNA methylation-based biomarkers and the epigenetic clock theory of ageing. Nat. Rev. Genet. 19, 371–384. doi: 10.1038/s41576-018-0004-3
Huan, T., Chen, G., Liu, C., Bhattacharya, A., Rong, J., Chen, B. H., et al. (2018). Age-associated microRNA expression in human peripheral blood is associated with all-cause mortality and age-related traits. Aging Cell 17:e12687. doi: 10.1111/acel.12687
Johnson, A. A., and Stolzing, A. (2019). The role of lipid metabolism in aging, lifespan regulation, and age-related disease. Aging Cell 18:e13048. doi: 10.1111/acel.13048
Jung, Y.-S., Qian, Y., and Chen, X. (2010). Examination of the expanding pathways for the regulation of p21 expression and activity. Cell. Signal. 22, 1003–1012. doi: 10.1016/j.cellsig.2010.01.013
Justice, J. N., Ferrucci, L., Newman, A. B., Aroda, V. R., Bahnson, J. L., Divers, J., et al. (2018). A framework for selection of blood-based biomarkers for geroscience-guided clinical trials: report from the TAME Biomarkers Workgroup. Geroscience 40, 419–436. doi: 10.1007/s11357-018-0042-y
Kahl, V. F. S., Allen, J. A. M., Nelson, C. B., Sobinoff, A. P., Lee, M., Kilo, T., et al. (2020). Telomere length measurement by molecular combing. Front. Cell. Dev. Biol. 8:493. doi: 10.3389/fcell.2020.00493
Kalluri, R., and LeBleu, V. S. (2020). The biology, function, and biomedical applications of exosomes. Science 367:eaau6977. doi: 10.1126/science.aau6977
Keaveny, T. M., Kopperdahl, D. L., Melton, L. J., Hoffmann, P. F., Amin, S., Riggs, B. L., et al. (2010). Age-dependence of femoral strength in white women and men. J. Bone Miner. Res. 25, 994–1001. doi: 10.1359/jbmr.091033
Khan, S. S., Singer, B. D., and Vaughan, D. E. (2017). Molecular and physiological manifestations and measurement of aging in humans. Aging Cell 16, 624–633. doi: 10.1111/acel.12601
Khosla, S., Atkinson, E. J., Riggs, B. L., and Melton, L. J. (1996). Relationship between body composition and bone mass in women. J. Bone Miner. Res. 11, 857–863. doi: 10.1002/jbmr.5650110618
Khosla, S., Riggs, B. L., Atkinson, E. J., Oberg, A. L., McDaniel, L. J., Holets, M., et al. (2006). Effects of sex and age on bone microstructure at the ultradistal radius: a population-based noninvasive in vivo assessment. J. Bone Miner. Res. 21, 124–131. doi: 10.1359/JBMR.050916
Kiechle, F. L. (2010). Procedures for the Handling and Processing of Blood Specimens for Common Laboratory Tests; Approved Guideline. Wayne, PA: Clinical and Laboratory Standards Institute.
Kim, H.-J., Kim, J. J., Myeong, N. R., Kim, T., Kim, D., An, S., et al. (2019). Segregation of age-related skin microbiome characteristics by functionality. Sci. Rep. 9:16748. doi: 10.1038/s41598-019-53266-3
Kim, M., and Benayoun, B. A. (2020). The microbiome: an emerging key player in aging and longevity. Transl. Med. Aging 4, 103–116. doi: 10.1016/j.tma.2020.07.004
Kudryashova, K. S., Burka, K., Kulaga, A. Y., Vorobyeva, N. S., and Kennedy, B. K. (2020). Aging biomarkers: from functional tests to multi-omics approaches. Proteomics 20:e1900408. doi: 10.1002/pmic.201900408
Kumar, S., Vijayan, M., Bhatti, J. S., and Reddy, P. H. (2017). MicroRNAs as peripheral biomarkers in aging and age-related diseases. Prog. Mol. Biol. Transl. Sci. 146, 47–94. doi: 10.1016/bs.pmbts.2016.12.013
Kumsta, C., Chang, J. T., Lee, R., Tan, E. P., Yang, Y., Loureiro, R., et al. (2019). The autophagy receptor p62/SQST-1 promotes proteostasis and longevity in C. elegans by inducing autophagy. Nat. Commun. 10:5648. doi: 10.1038/s41467-019-13540-4
Lai, T.-P., Wright, W. E., and Shay, J. W. (2018). Comparison of telomere length measurement methods. Philos. Trans. R. Soc. Lond. B Biol. Sci. 373:20160451. doi: 10.1098/rstb.2016.0451
LaRocca, T. J., Cavalier, A. N., and Wahl, D. (2020). Repetitive elements as a transcriptomic marker of aging: evidence in multiple datasets and models. Aging Cell 19:e13167. doi: 10.1111/acel.13167
Levine, M. E. (2013). Modeling the rate of senescence: can estimated biological age predict mortality more accurately than chronological age? J. Gerontol. A Biol. Sci. Med. Sci. 68, 667–674. doi: 10.1093/gerona/gls233
Levine, M. E. (2019). “Epigenetic biomarkers of aging,” in Biomarkers of Human Aging, ed. A. Moskalev (Cham: Springer International Publishing), 155–171.
Levine, M. E., Lu, A. T., Quach, A., Chen, B. H., Assimes, T. L., Bandinelli, S., et al. (2018). An epigenetic biomarker of aging for lifespan and healthspan. Aging (Albany NY) 10, 573–591. doi: 10.18632/aging.101414
Lindeman, R. D., Tobin, J., and Shock, N. W. (1985). Longitudinal studies on the rate of decline in renal function with age. J. Am. Geriatr. Soc. 33, 278–285. doi: 10.1111/j.1532-5415.1985.tb07117.x
Liu, Z., Kuo, P.-L., Horvath, S., Crimmins, E., Ferrucci, L., and Levine, M. (2018a). A new aging measure captures morbidity and mortality risk across diverse subpopulations from NHANES IV: a cohort study. PLoS Med. 15:e1002718. doi: 10.1371/journal.pmed.1002718
Liu, Z., Kuo, P.-L., Horvath, S., Crimmins, E., Ferrucci, L., and Levine, M. (2018b). Phenotypic age: a novel signature of mortality and morbidity risk. biorxiv [Preprint]. doi: 10.1101/363291
López-Otín, C., Blasco, M. A., Partridge, L., Serrano, M., and Kroemer, G. (2013). The hallmarks of aging. Cell 153, 1194–1217. doi: 10.1016/j.cell.2013.05.039
Lu, A. T., Fei, Z., Haghani, A., Robeck, T. R., Zoller, J. A., Li, C. Z., et al. (2021). Universal DNA methylation age across mammalian tissues. biorxiv [Preprint]. doi: 10.1101/2021.01.18.426733
Lu, A. T., Quach, A., Wilson, J. G., Reiner, A. P., Aviv, A., Raj, K., et al. (2019). DNA methylation GrimAge strongly predicts lifespan and healthspan. Aging (Albany NY) 11, 303–327. doi: 10.18632/aging.101684
Lulkiewicz, M., Bajsert, J., Kopczynski, P., Barczak, W., and Rubis, B. (2020). Telomere length: how the length makes a difference. Mol. Biol. Rep. 47, 7181–7188. doi: 10.1007/s11033-020-05551-y
Mamoshina, P., Kochetov, K., Cortese, F., Kovalchuk, A., Aliper, A., Putin, E., et al. (2019). Blood biochemistry analysis to detect smoking status and quantify accelerated aging in smokers. Sci. Rep. 9:142. doi: 10.1038/s41598-018-35704-w
Mamoshina, P., Volosnikova, M., Ozerov, I. V., Putin, E., Skibina, E., Cortese, F., et al. (2018). Machine learning on human muscle transcriptomic data for biomarker discovery and tissue-specific drug target identification. Front. Genet. 9:242. doi: 10.3389/fgene.2018.00242
Maynard, C., and Weinkove, D. (2018). The gut microbiota and ageing. Subcell. Biochem. 90, 351–371. doi: 10.1007/978-981-13-2835-0_12
Messner, M., Ghadge, S. K., Schuetz, T., Seiringer, H., Pölzl, G., and Zaruba, M.-M. (2019). High body mass index is associated with elevated blood levels of progerin mRNA. Int. J. Mol. Sci. 20:1976. doi: 10.3390/ijms20081976
Migliaccio, A. R., and Palis, J. (2011). Blood in a dish: in vitro synthesis of red blood cells. Drug Discov. Today Dis. Mech. 8, e3–e8. doi: 10.1016/j.ddmec.2011.10.002
Milanovic, M., Fan, D. N. Y., Belenki, D., Däbritz, J. H. M., Zhao, Z., Yu, Y., et al. (2018). Senescence-associated reprogramming promotes cancer stemness. Nature 553, 96–100. doi: 10.1038/nature25167
Moon, J. S., Goeminne, L. J. E., Kim, J. T., Tian, J. W., Kim, S.-H., Nga, H. T., et al. (2020). Growth differentiation factor 15 protects against the aging-mediated systemic inflammatory response in humans and mice. Aging Cell 19:e13195. doi: 10.1111/acel.13195
Narita, M., Nuñez, S., Heard, E., Narita, M., Lin, A. W., Hearn, S. A., et al. (2003). Rb-Mediated heterochromatin formation and silencing of E2F target genes during cellular senescence. Cell 113, 703–716. doi: 10.1016/S0092-8674(03)00401-X
Niedernhofer, L. J., Kirkland, J. L., and Ladiges, W. (2017). Molecular pathology endpoints useful for aging studies. Ageing Res. Rev. 35, 241–249. doi: 10.1016/j.arr.2016.09.012
Nishio, K., Inoue, A., Qiao, S., Kondo, H., and Mimura, A. (2001). Senescence and cytoskeleton: overproduction of vimentin induces senescent-like morphology in human fibroblasts. Histochem. Cell Biol. 116, 321–327. doi: 10.1007/s004180100325
Noren Hooten, N. (2020). Extracellular vesicles and extracellular RNA in aging and age-related disease. Transl. Med. Aging 4, 96–98. doi: 10.1016/j.tma.2020.07.006
Noren Hooten, N., Abdelmohsen, K., Gorospe, M., Ejiogu, N., Zonderman, A. B., and Evans, M. K. (2010). microRNA expression patterns reveal differential expression of target genes with age. PLoS One 5:e10724. doi: 10.1371/journal.pone.0010724
Oberdoerffer, P., and Sinclair, D. A. (2007). The role of nuclear architecture in genomic instability and ageing. Nat. Rev. Mol. Cell. Biol. 8, 692–702. doi: 10.1038/nrm2238
Ovadya, Y., and Krizhanovsky, V. (2018). Strategies targeting cellular senescence. J. Clin. Invest. 128, 1247–1254. doi: 10.1172/JCI95149
Owczarz, M., Budzinska, M., Domaszewska-Szostek, A., Borkowska, J., Polosak, J., Gewartowska, M., et al. (2017). miR-34a and miR-9 are overexpressed and SIRT genes are downregulated in peripheral blood mononuclear cells of aging humans. Exp. Biol. Med. (Maywood) 242, 1453–1461. doi: 10.1177/1535370217720884
Peters, M. J., Joehanes, R., Pilling, L. C., Schurmann, C., Conneely, K. N., Powell, J., et al. (2015). The transcriptional landscape of age in human peripheral blood. Nat. Commun. 6:8570. doi: 10.1038/ncomms9570
Pinto, E. (2007). Blood pressure and ageing. Postgrad. Med. J. 83, 109–114. doi: 10.1136/pgmj.2006.048371
Putin, E., Mamoshina, P., Aliper, A., Korzinkin, M., Moskalev, A., Kolosov, A., et al. (2016). Deep biomarkers of human aging: application of deep neural networks to biomarker development. Aging (Albany NY) 8, 1021–1033. doi: 10.18632/aging.100968
Qian, Y., and Chen, X. (2013). Senescence regulation by the p53 protein family. Methods Mol. Biol. 965, 37–61. doi: 10.1007/978-1-62703-239-1_3
Rai, A. J., Gelfand, C. A., Haywood, B. C., Warunek, D. J., Yi, J., Schuchard, M. D., et al. (2005). HUPO plasma proteome project specimen collection and handling: towards the standardization of parameters for plasma proteome samples. Proteomics 5, 3262–3277. doi: 10.1002/pmic.200401245
Rai, A. J., and Vitzthum, F. (2006). Effects of preanalytical variables on peptide and protein measurements in human serum and plasma: implications for clinical proteomics. Expert Rev. Proteomics 3, 409–426. doi: 10.1586/14789450.3.4.409
Rantanen, T., Harris, T., Leveille, S. G., Visser, M., Foley, D., Masaki, K., et al. (2000). Muscle strength and body mass index as long-term predictors of mortality in initially healthy men. J. Gerontol. A Biol. Sci. Med. Sci. 55, M168–M173. doi: 10.1093/gerona/55.3.m168
Reid, G., Kirschner, M. B., and van Zandwijk, N. (2011). Circulating microRNAs: association with disease and potential use as biomarkers. Crit. Rev. Oncol. Hematol. 80, 193–208. doi: 10.1016/j.critrevonc.2010.11.004
Ren, X., and Kuan, P. F. (2020). RNAAgeCalc: a multi-tissue transcriptional age calculator. PLoS One 15:e0237006. doi: 10.1371/journal.pone.0237006
Riedhammer, C., Halbritter, D., and Weissert, R. (2016). Peripheral blood mononuclear cells: isolation, freezing, thawing, and culture. Methods Mol. Biol. 1304, 53–61. doi: 10.1007/7651_2014_99
Riggs, B. L., Melton, L. J., Robb, R. A., Camp, J. J., Atkinson, E. J., McDaniel, L., et al. (2008). A population-based assessment of rates of bone loss at multiple skeletal sites: evidence for substantial trabecular bone loss in young adult women and men. J. Bone Miner. Res. 23, 205–214. doi: 10.1359/jbmr.071020
Sahu, A., Mamiya, H., Shinde, S. N., Cheikhi, A., Winter, L. L., Vo, N. V., et al. (2018). Age-related declines in α-Klotho drive progenitor cell mitochondrial dysfunction and impaired muscle regeneration. Nat. Commun. 9:4859. doi: 10.1038/s41467-018-07253-3
Santos, A. L., Sinha, S., and Lindner, A. B. (2018). The good, the bad, and the ugly of ROS: new insights on aging and aging-related diseases from eukaryotic and prokaryotic model organisms. Oxid. Med. Cell. Longev. 2018:1941285. doi: 10.1155/2018/1941285
Scaffidi, P., and Misteli, T. (2006). Lamin A-dependent nuclear defects in human aging. Science 312, 1059–1063. doi: 10.1126/science.1127168
Schafer, M. J., Atkinson, E. J., Vanderboom, P. M., Kotajarvi, B., White, T. A., Moore, M. M., et al. (2016). Quantification of GDF11 and myostatin in human aging and cardiovascular disease. Cell Metab. 23, 1207–1215. doi: 10.1016/j.cmet.2016.05.023
Schafer, M. J., Zhang, X., Kumar, A., Atkinson, E. J., Zhu, Y., Jachim, S., et al. (2020). The senescence-associated secretome as an indicator of age and medical risk. JCI Insight 5:e133668. doi: 10.1172/jci.insight.133668
Schöll, M., Lockhart, S. N., Schonhaut, D. R., O’Neil, J. P., Janabi, M., Ossenkoppele, R., et al. (2016). PET imaging of Tau deposition in the aging human brain. Neuron 89, 971–982. doi: 10.1016/j.neuron.2016.01.028
Sebastiani, P., Federico, A., Morris, M., Gurinovich, A., Tanaka, T., Chandler, K. B., et al. (2021). Protein signatures of centenarians and their offspring suggest centenarians age slower than other humans. Aging Cell 20:e13290. doi: 10.1111/acel.13290
Sebastiani, P., Thyagarajan, B., Sun, F., Schupf, N., Newman, A. B., Montano, M., et al. (2017). Biomarker signatures of aging. Aging Cell 16, 329–338. doi: 10.1111/acel.12557
Serrano, M., Lin, A. W., McCurrach, M. E., Beach, D., and Lowe, S. W. (1997). Oncogenic ras provokes premature cell senescence associated with accumulation of p53 and p16INK4a. Cell 88, 593–602. doi: 10.1016/s0092-8674(00)81902-9
Sharpless, N. E., and Sherr, C. J. (2015). Forging a signature of in vivo senescence. Nat. Rev. Cancer 15, 397–408. doi: 10.1038/nrc3960
Shay, J. W. (2016). Role of telomeres and telomerase in aging and cancer. Cancer Discov. 6, 584–593. doi: 10.1158/2159-8290.CD-16-0062
Shokhirev, M. N., and Johnson, A. A. (2020). Modeling the human aging transcriptome across tissues, health status, and sex. Aging Cell 20:e13280. doi: 10.1111/acel.13280
Siderowf, Aarsland, D., Mollenhauer, B., Goldman, J. G., and Ravina, B. (2001). Biomarkers and surrogate endpoints: preferred definitions and conceptual framework. Clin. Pharmacol. Ther. 69, 89–95. doi: 10.1067/mcp.2001.113989
Simonsen, A., Cumming, R. C., Brech, A., Isakson, P., Schubert, D. R., and Finley, K. D. (2008). Promoting basal levels of autophagy in the nervous system enhances longevity and oxidant resistance in adult Drosophila. Autophagy 4, 176–184. doi: 10.4161/auto.5269
Song, S., Lam, E. W.-F., Tchkonia, T., Kirkland, J. L., and Sun, Y. (2020). Senescent cells: emerging targets for human aging and age-related diseases. Trends Biochem. Sci. 45, 578–592. doi: 10.1016/j.tibs.2020.03.008
Sowell, E. R., Peterson, B. S., Thompson, P. M., Welcome, S. E., Henkenius, A. L., and Toga, A. W. (2003). Mapping cortical change across the human life span. Nat. Neurosci. 6, 309–315. doi: 10.1038/nn1008
Stadtman, E. R., and Berlett, B. S. (1997). Reactive oxygen-mediated protein oxidation in aging and disease. Chem. Res. Toxicol. 10, 485–494. doi: 10.1021/tx960133r
Syddall, H., Cooper, C., Martin, F., Briggs, R., and Aihie Sayer, A. (2003). Is grip strength a useful single marker of frailty? Age Ageing 32, 650–656. doi: 10.1093/ageing/afg111
Tanaka, T., Biancotto, A., Moaddel, R., Moore, A. Z., Gonzalez-Freire, M., Aon, M. A., et al. (2018). Plasma proteomic signature of age in healthy humans. Aging Cell 17:e12799. doi: 10.1111/acel.12799
Tedone, E., Huang, E., O’Hara, R., Batten, K., Ludlow, A. T., Lai, T.-P., et al. (2019). Telomere length and telomerase activity in T cells are biomarkers of high-performing centenarians. Aging Cell 18:e12859. doi: 10.1111/acel.12859
Tominaga, K., and Suzuki, H. I. (2019). TGF-β signaling in cellular senescence and aging-related pathology. Int. J. Mol. Sci. 20:5002. doi: 10.3390/ijms20205002
Vaiserman, A., and Krasnienkov, D. (2020). Telomere length as a marker of biological age: state-of-the-art, open issues, and future perspectives. Front. Genet. 11:630186. doi: 10.3389/fgene.2020.630186
Vangipuram, M., Ting, D., Kim, S., Diaz, R., and Schüle, B. (2013). Skin punch biopsy explant culture for derivation of primary human fibroblasts. J. Vis. Exp. 77, e3779. doi: 10.3791/3779
Wagner, K.-H., Cameron-Smith, D., Wessner, B., and Franzke, B. (2016). Biomarkers of aging: from function to molecular biology. Nutrients 8:338. doi: 10.3390/nu8060338
Walter, M. (2009). Interrelationships among HDL metabolism, aging, and atherosclerosis. Arterioscler. Thromb. Vasc. Biol. 29, 1244–1250. doi: 10.1161/ATVBAHA.108.181438
Wang, A. S., and Dreesen, O. (2018). Biomarkers of cellular senescence and skin aging. Front. Genet 9:247. doi: 10.3389/fgene.2018.00247
Weinstein, J. R., and Anderson, S. (2010). The aging kidney: physiological changes. Adv. Chronic Kidney Dis. 17, 302–307. doi: 10.1053/j.ackd.2010.05.002
Wu, Q., and Ni, X. (2015). ROS-mediated DNA methylation pattern alterations in carcinogenesis. Curr. Drug Targets 16, 13–19. doi: 10.2174/1389450116666150113121054
Xia, X., Chen, W., McDermott, J., and Han, J.-D. J. (2017). Molecular and phenotypic biomarkers of aging. F1000Res 6:860. doi: 10.12688/f1000research.10692.1
Xu, Y., and Sun, Z. (2015). Molecular basis of Klotho: from gene to function in aging. Endocr. Rev. 36, 174–193. doi: 10.1210/er.2013-1079
Yáñez-Mó, M., Siljander, P. R.-M., Andreu, Z., Zavec, A. B., Borràs, F. E., Buzas, E. I., et al. (2015). Biological properties of extracellular vesicles and their physiological functions. J. Extracell. Vesicles 4:27066. doi: 10.3402/jev.v4.27066
Yousefzadeh, M. J., Zhao, J., Bukata, C., Wade, E. A., McGowan, S. J., Angelini, L. A., et al. (2020). Tissue specificity of senescent cell accumulation during physiologic and accelerated aging of mice. Aging Cell 19:e13094. doi: 10.1111/acel.13094
Zhavoronkov, A., Li, R., Ma, C., and Mamoshina, P. (2019). Deep biomarkers of aging and longevity: from research to applications. Aging (Albany NY) 11, 10771–10780. doi: 10.18632/aging.102475
Keywords: aging, biomarker, health, senescence, survival
Citation: Hartmann A, Hartmann C, Secci R, Hermann A, Fuellen G and Walter M (2021) Ranking Biomarkers of Aging by Citation Profiling and Effort Scoring. Front. Genet. 12:686320. doi: 10.3389/fgene.2021.686320
Received: 26 March 2021; Accepted: 23 April 2021;
Published: 21 May 2021.
Edited by:
Ilia Stambler, International Longevity Alliance, FranceReviewed by:
Alexander Vaiserman, Institute of Gerontology (NAN Ukraine), UkraineCopyright © 2021 Hartmann, Hartmann, Secci, Hermann, Fuellen and Walter. This is an open-access article distributed under the terms of the Creative Commons Attribution License (CC BY). The use, distribution or reproduction in other forums is permitted, provided the original author(s) and the copyright owner(s) are credited and that the original publication in this journal is cited, in accordance with accepted academic practice. No use, distribution or reproduction is permitted which does not comply with these terms.
*Correspondence: Michael Walter, bWljaGFlbC53YWx0ZXJAbWVkLnVuaS1yb3N0b2NrLmRl; Georg Fuellen, ZnVlbGxlbkB1bmktcm9zdG9jay5kZQ==
Disclaimer: All claims expressed in this article are solely those of the authors and do not necessarily represent those of their affiliated organizations, or those of the publisher, the editors and the reviewers. Any product that may be evaluated in this article or claim that may be made by its manufacturer is not guaranteed or endorsed by the publisher.
Research integrity at Frontiers
Learn more about the work of our research integrity team to safeguard the quality of each article we publish.