- 1Institute of Genome Biology, Leibniz Institute for Farm Animal Biology (FBN), Dummerstorf, Germany
- 2Departamento de Producción Animal, Facultad de Veterinaria, Universidad de León, León, Spain
- 3Departamento de Sanidad Animal, Facultad de Veterinaria, Universidad de León, León, Spain
- 4Instituto de Ganadería de Montaña, CSIC-Universidad de León, León, Spain
- 5Faculty of Agricultural and Environmental Sciences, University of Rostock, Rostock, Germany
Several recent studies have demonstrated the role of long non-coding RNAs (lncRNAs) in regulating the defense mechanism against parasite infections, but no studies are available that investigated their relevance for immune response to nematode infection in sheep. Thus, the aim of the current study was to (i) detect putative lncRNAs that are expressed in the abomasal lymph node of adult sheep after an experimental infection with the gastrointestinal nematode (GIN) Teladorsagia circumcincta and (ii) to elucidate their potential functional role associated with the differential host immune response. We hypothesized that putative lncRNAs differentially expressed (DE) between samples from animals that differ in resistance to infection may play a significant regulatory role in response to nematode infection in adult sheep. To obtain further support for our hypothesis, we performed co-expression and functional gene enrichment analyses with the differentially expressed lncRNAs (DE lncRNAs). In a conservative approach, we included for this predictive analysis only those lncRNAs that are confirmed and supported by documentation of expression in gastrointestinal tissues in the current sheep gene atlas. We identified 9,105 putative lncRNA transcripts corresponding to 7,124 gene loci. Of these, 457 were differentially expressed lncRNA loci (DELs) with 683 lncRNA transcripts. Based on a gene co-expression analysis via weighted gene co-expression network analysis, 12 gene network modules (GNMs) were found significantly correlated with at least one of 10 selected target DE lncRNAs. Based on the principle of “guilt-by-association,” the DE genes from each of the three most significantly correlated GNMs were subjected to a gene enrichment analysis. The significant pathways associated with DE lncRNAs included ERK5 Signaling, SAPK/JNK Signaling, RhoGDI Signaling, EIF2 Signaling, Regulation of eIF4 and p70S6K Signaling and Oxidative Phosphorylation pathways. They belong to signaling pathway categories like Cellular Growth, Proliferation and Development, Cellular Stress and Injury, Intracellular and Second Messenger Signaling and Apoptosis. Overall, this lncRNA study conducted in adult sheep after GIN infection provided first insights into the potential functional role of lncRNAs in the differential host response to nematode infection.
Introduction
The central dogma of molecular biology states that DNA is transcribed into mRNA and mRNA is translated into protein products (Crick, 1970). There is an increasing interest, however, also in the non-protein coding RNAs (ncRNAs), which are estimated to comprise up to 80% of the entire transcriptome in mammals (The ENCODE Project Consortium, 2012; Hon et al., 2017; Srijyothi et al., 2018). The ncRNAs are classified into several sub-classes, such as small non-coding RNAs, including microRNAs and small interfering RNAs, processed small RNAs (Wilusz et al., 2009), transcription start site–associated RNAs (Seila et al., 2008), promoter associated RNAs (PARs) (Taft et al., 2009), enhancer RNAs (eRNAs) (Kim et al., 2015) and long non-coding RNAs (lncRNAs) (Wilusz et al., 2009). LncRNAs are defined as non-coding RNA transcripts with a length greater than 200 nucleotides. Although lncRNAs are biochemically resembling mRNAs, they generally do not encode protein products (Ponting et al., 2009). LncRNAs are divided into several subgroups according to the positional genomic relationship between lncRNAs and their neighboring protein-coding genes including overlapping, antisense, intronic and intergenic lncRNAs. Advances in computational biology and evolution of sensitive RNA sequencing and epigenomic technologies have facilitated the discovery of numerous lncRNAs and encouraged the study of their functional roles.
Based on their potential function, lncRNAs can be broadly classified into three main categories: (1) lncRNA transcripts, which are non-functional; (2) lncRNAs as regulators of transcription, which act as cis- and trans-active modulators of protein-coding gene expression (Kopp and Mendell, 2018), and (3) lncRNA transcripts involved in post-transcriptional regulation, which includes alternative mRNA splicing regulation, translational control and competing with regulatory endogenous RNAs (Riquelme et al., 2016; Dykes and Emanueli, 2017). It has been found that lncRNAs exhibit splice junctions and introns (Hiller et al., 2009) and cell- and tissue- specific expression patterns. The misexpression of lncRNAs has been shown to contribute to neurological disorders, cancer (Qureshi et al., 2010), susceptibility to infection, metabolic disorders such as diabetes and obesity (Moran et al., 2012; Zhao and Lin, 2015), and other diseases (Wapinski and Chang, 2011). In addition to their identification and cataloging, the functional annotation of the discovered lncRNAs is also challenging, as they are sparsely and cell type-specifically expressed, which might limit their function to few biological states (Derrien et al., 2012). Furthermore, at sequence level lncRNAs are poorly conserved across species (Ulitsky and Bartel, 2013; Hezroni et al., 2015).
The functional annotation of lncRNAs aims to identify or predict the possible biological process with which an lncRNA transcript can be interrelated, its putative mechanism of action, potential interacting partners and putative functional elements within the RNA locus (Cao et al., 2018). The annotation catalog of lncRNAs is far from complete even in humans and mice, and much work remains to be done in the field of lncRNAs annotation in the genomes of livestock species (Weikard et al., 2017). Currently, several lncRNA databases are available (Maracaja-Coutinho et al., 2019), which provide annotations of lncRNAs, mostly referring to the human genome. Among them, the NON-CODE database (Zhao et al., 2016) currently offers a comprehensive collection of lncRNA transcripts that have been experimentally confirmed in humans and 15 animal species (including the farm animals cow, pig, and chicken, but not sheep). With regard to the sheep genome, although there are many predicted protein coding genes known, only 30% of them are carrying an Ensembl identifier in the OAR v3.1 reference genome [Ensembl annotation based on adult Texel sheep (Clark et al., 2017)]. Most of the detected and characterized lncRNAs of the sheep genome have been annotated through the sheep atlas project based on the Texel breed (Clark et al., 2017).
Recently, another sheep reference genome Oar rambouillet v1.0 (GCA_002742125) has become available, providing a highly contiguous sheep genome with an annotation and mapping of transcription start sites (Salavati et al., 2020). However, this new reference genome contains a similar number of coding and non-coding genes compared to the OAR v3.1 reference genome. Currently, the Ensembl database contains 1,858 and 2,236 putative lncRNAs in the OAR v3.1 and the OAR rambouillet v1.0 genome annotation, respectively1 (Oar rambouillet v1.02).
LncRNAs are known to act as key regulators of the immune response by a variety of mechanisms (Chen et al., 2017; Menard et al., 2019). Some recent lncRNA studies conducted in humans (Rochet et al., 2019) and mice (Menard et al., 2018) have demonstrated the role of lncRNAs in regulating the defense mechanisms against parasite infections. However, up to now no sheep-specific studies are available that investigated the importance of lncRNAs for the immune response to nematode or parasite infection.
Gastrointestinal parasite infections in sheep are a major challenge to sheep husbandry due to great economic losses, impairment of animal welfare and difficulties in appropriate treatment and prophylaxis under the dominating extensive pasture system in sheep production (Roeber et al., 2013; Ruano et al., 2019). The aim of the current study was therefore to detect lncRNAs in the transcriptome of abomasal lymph node (ALN) tissue samples extracted from adult Spanish Churra sheep after an experimental infection with the gastrointestinal nematode (GIN) Teladorsagia circumcincta and to elucidate their potential functional role associated with the host immune response against GIN infection in adult sheep. The current study is based on the OAR v3.1 genome annotation, as it was the most widely used annotation and was also used as reference annotation in our previous RNA-sequencing (RNA-seq) studies (Chitneedi et al., 2018, 2020), which were conducted with the same samples as the current study.
We hypothesized that putative lncRNAs differentially expressed between samples from animals that differ in infection resistance may play a significant regulatory role in response to nematode infection in adult sheep. To validate our hypothesis, we performed co-expression and functional gene enrichment analyses with selected lncRNAs that (i) were differentially expressed in our study in response to GIN infection, (ii) are included in the current sheep gene atlas, and (iii) showed expression in gastrointestinal and lymph node tissue (Clark et al., 2017; Bush et al., 2018). Thus, this work will contribute to the aim of the global network for Functional Annotation of Animal Genomes (FAANG) to provide high quality functional annotations of farm animal genomes (Andersson et al., 2015).
Materials and Methods
Experimental Design and RNA Sequencing
The experiment and the criteria for animal selection have been described earlier in detail in Chitneedi et al. (2018). The whole experiment was carried out according to the current National Spanish legislation on the protection of animals used in experimentation (Royal Decree 53/2013) and the approval of the competent body of the regional government, Junta de Castilla y León (ULE_024_2015). Initially, a commercial flock of the Churra dairy sheep breed including 119 dairy ewes, raised under a commercial, semi-intensive management system representing natural GIN infection conditions was sampled to measure the fecal egg count (FEC). Based on the distribution of FEC values in the selected flock [phenotypic values: average = 25.59 eggs per gram (epg); SD = 60.31 epg], we preselected a group of 10 animals showing extremely low FEC values (range 0–15 epg) and a group of eight animals showing very high FEC values (range 90–225 epg). These animals were transferred to the experimental farm of the Mountain Livestock Institute (IGM, León, Spain), where they were exposed to a first standardized experimental infection (EI1) with T. circumcincta third stage larvae (L3) after an antihelmintic treatment (oral dose of ivermectin). T. circumcincta is one of the most common GIN parasites that infects different sheep breeds, in particular with a high incidence in Churra sheep (Diez-Banos et al., 1992; Castilla-Gomez De Aguero et al., 2020). Based on the accumulative FEC at days 14–31 after the first experimental infection (EI1), six ewes were classified as ‘susceptible’ (SUS, range: 2,310–9,666 epg; average: 5,594 ± 2,661 epg) and six ewes as ‘resistant’ (RES, range: 0–915 epg; average: 308 ± 338 epg). After a second experimental infection (EI2), these 12 animals were sacrificed at day 7 after infection, and abomasal lymph node (ALN) tissue samples were collected. Total RNA extracted from these samples was used for library preparation using the KAPA Stranded mRNA-Sequencing Kit that starts with an oligo dT selection step (Roche). RNA sequencing (RNA-seq) using an Illumina Hi-Seq 2000 platform generated ‘paired-end’ reads of 75 bp, with a sequencing depth of 30 M fragments per sample. The FASTQ format data from the 12 ALN samples were subjected to the subsequent bioinformatics workflow summarized in Supplementary Figure 1.
Alignment and Transcript Assembly
The script including the specific parameters used for different tools included in the bioinformatic workflow is provided in Supplementary File 1. Initially, potential adapter sequences were removed from RNA reads with cutadapt_v1.18 (Martin, 2011) followed by trimming of poor-quality sequences from reads using quality trim v1.6.0 (Robinson, 2015) to improve subsequent read alignment. After trimming, the reads were aligned against the Ovis aries reference genome Oar_v3.1 (GCA_000298735.1) with Ensembl annotation release 953 using the alignment tool HISAT2 v2.1.0 (Pertea et al., 2015). The aligned unsorted reads were sorted using samtools v1.9 (Li, 2011). The aligned and sorted read data were assembled into transcripts using StringTie v1.3.5 (Pertea et al., 2015) and the O. aries reference genome Oar_v3.1 (GCA_000298735.1) with Ensembl annotation release 95 (see Text Footnote 3) for a reference-guided transcriptome assembly approach (Pertea et al., 2015). This concept of reference-guided transcriptome assembly, which depends on a genome assembly of the target organism, takes benefit from existing information about transcribed genome elements, but enables detection of previously unknown transcripts. This is of particular importance for livestock lncRNA investigation, because the catalog of lncRNAs is by far not complete up to now. StringTie starts by building clusters from reads aligned to the genome and then creates a splice graph to identify transcripts (Pertea et al., 2015). In addition to tagging the unannotated genes, StringTie also tags those genes provided in the reference genome annotation. After assembling the reads from all 12 samples individually, the full set of transcript assemblies was passed to StringTie’s merge function, which merges the gene structures found in any one of the samples. As in some samples only partially covered transcripts were assembled in the initial StringTie run, the merging step created a set of transcripts that is consistent across all samples, so that the transcripts can be compared in the subsequent steps (Pertea et al., 2015). Additionally, the merged annotation file was compared with the reference annotation Ensembl release 95 (see Text Footnote 3) of the OAR_v3.1 genome using gffcompare v-0.10.6 (Pertea and Pertea, 2020) to identify novel transcripts and to determine how many assembled transcripts were fully or partially consistent with annotated genes.
LncRNA Detection
We used the lncRNA prediction tool FEELnc (Wucher et al., 2017) to indicate lncRNAs in the newly established merged annotation file of the ALN transcriptome in our sheep samples. The selection of this software was based on a previous study of our research group comparing different bioinformatic tools for lncRNA prediction (Weikard et al., 2018). The FEELnc analysis includes three steps: (i) filtering of candidate lncRNA transcripts; (ii) exploring coding potential and nucleotide composition of candidate lncRNA transcripts; and (iii) classification of the finally predicted lncRNA transcripts. In the first step, protein-coding genes, monoexonic transcripts (except for those in antisense direction to a coding gene) and transcripts with a size less than 200 nt were excluded, and a file was generated comprising potential candidate lncRNA transcripts. In the second step, the coding potential score was determined for each of the candidate lncRNA transcripts by considering absence of an open reading frame and k-mer nucleotide composition using the shuffle mode option of FEELnc. Finally, those putative lncRNAs with evidence for non-coding characteristics were classified with regard to their localization and their direction of transcription compared to adjacent RNA transcripts (protein-coding and non-coding loci). This classification of putative lncRNAs with respect to associated mRNAs or protein-coding genes (or other ncRNAs) might provide initial indication on potential lncRNA functions. In addition, to evaluate, if the putative lncRNA transcripts detected in the ALN sheep transcriptome were novel compared to the OAR_v3.1-r 95 annotation, the predicted lncRNAs were classified using the gffcompare -r option, and class code “u” assignment was taken as indication of a novel lncRNA.
Read Count and Differential Gene Expression Analysis
The ‘featureCounts’ option of the package subread v 1.6.3 (Liao et al., 2013) was used with the newly generated merged annotation file to calculate read count matrices for all samples and all annotated loci. Prior to differential gene expression (DGE) analysis of the samples, we plotted the accumulated phenotypic FEC data of the animals and also performed an exploratory Principal Component Analysis (PCA) of the sample transcriptomes for the initial six resistant and six susceptible animals (Supplementary Figure 2). The phenotypic information available for sample ALN_14 (Supplementary Figure 2A) showed that the accumulated FEC value of the respective animal after EI1 was the lowest among the susceptible samples. Considering the first two principle components (PC1 and PC2) of the transcriptome expression data, after excluding the ALN_14 this sample originally allocated into the susceptible group could not be clearly assigned to this group (Supplementary Figure 2B). Thus, in order to perform the subsequent analysis on two groups with clearly distinct phenotypes (Figures 1A,B), sample ALN_14 was considered as an outlier and was excluded from the subsequent DGE analysis. This DGE analysis was performed with the DESeq2 R package (Love et al., 2014) to identify differentially expressed (DE) loci (coding and non-coding) between the two animal groups differing in susceptibility to GIN infection. Loci were considered significantly DE at a FDR < 0.05 after Benjamini–Hochberg correction for multiple testing.
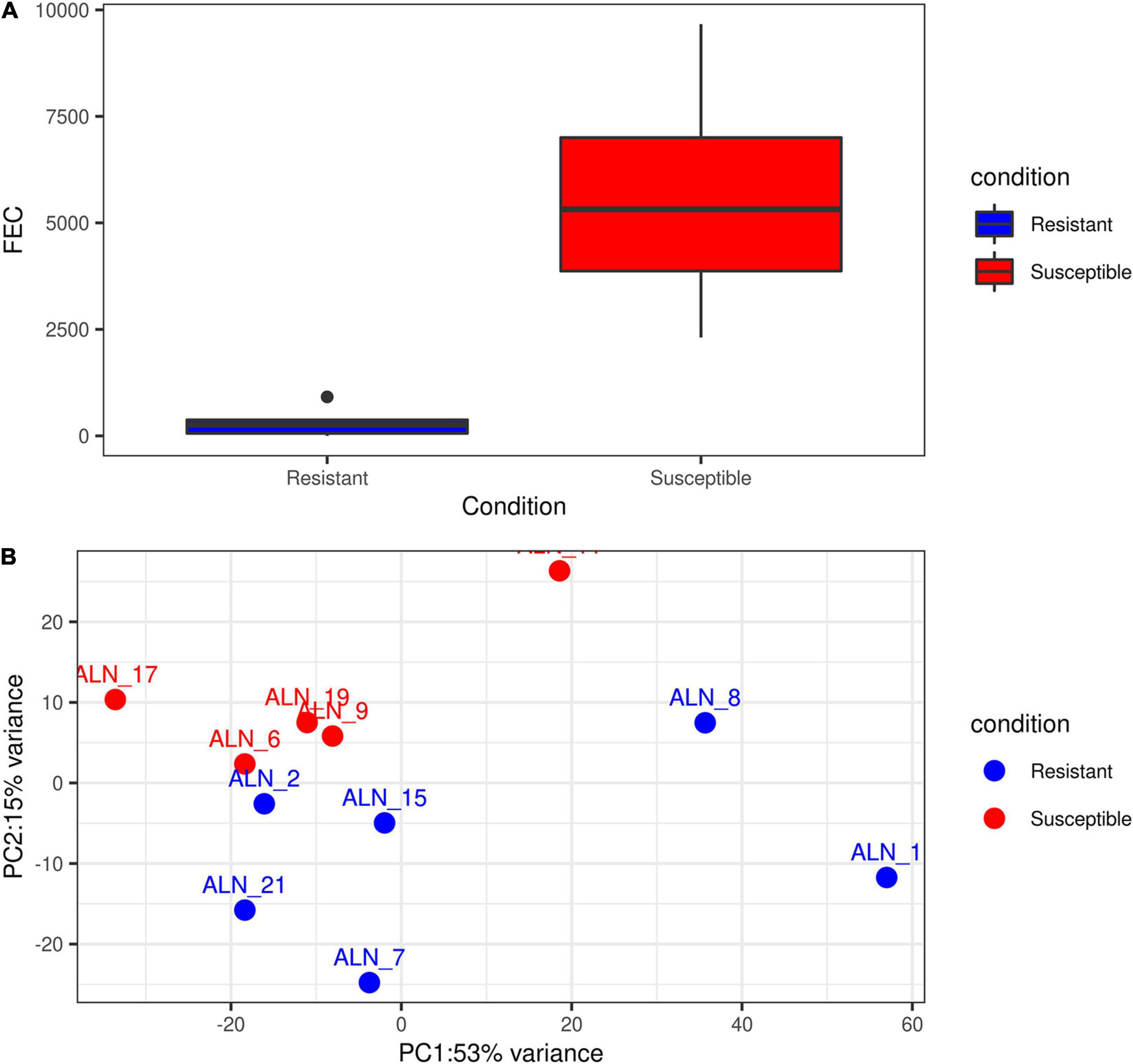
Figure 1. Exploratory plots of GIN infected resistant and susceptible sheep. (A) Distribution of accumulated fecal egg count (FEC) in resistant and susceptible sheep after a second experimental infection with GIN Teladorsagia circumcincta. Blue box represents the group mean of the resistant group and red box represents the group mean of the susceptible group. (B) PCA performed with the read count matrix of the 11 ALN RNA-seq datasets finally included in the differential gene expression analysis specifying the sample condition [resistant (6) and susceptible (5)].
Gene Co-expression Analysis
For a first indication on the potential functional role of lncRNA highlighted as DE in the differential expression analysis, we performed co-expression analyses. To this end, we applied the R package for Weighted gene co-expression network analysis (WGCNA, v1.64) (Langfelder and Horvath, 2008). This method was implemented using the parameters mentioned in Weikard et al. (2018). Briefly, WGCNA was performed initially with all the protein-coding and non-coding genes expressed in the 11 sheep ALN samples serving as expression data input except for those genes serving as target variables. We selected 10 lncRNAs as target variables for the WGCNA according to the following criteria: (i) they displayed significantly differential expression between the RES and SUS sheep in response to GIN infection, (ii) they had been reported previously as expressed in gastrointestinal and lymph node tissue in the sheep gene atlas study (Clark et al., 2017) and had been predicted as lncRNAs in the sheep lncRNA study linked to the sheep gene atlas study (Bush et al., 2018), (iii) finally, they displayed a clear exon-intron structure as verified by visual inspection of reads aligned to the selected DE lncRNA transcripts using the Interactive Genomics Viewer (IGV) (Thorvaldsdottir et al., 2013).
The co-expressed genes within the WGCNA were identified as gene network modules (GNMs) marked with different colors. We assumed that highly interconnected genes within a GNM are co-regulated and might be involved in similar biological pathways. Those GNMs that were highly significantly correlated with at least one DE lncRNA transcript (r > | 0.75| and p < 0.01) were selected for pathway enrichment analysis of the included DE genes to obtain indication on a potential functional role of the correlated DE lncRNA.
Enrichment and Pathway Analysis
To predict the potential biological function of the DE lncRNAs and associated biological pathways, we performed the gene set enrichment pathway analysis using the Qiagen Ingenuity analysis package (IPA4) with the DE genes from those GNM that were correlated with a specific lncRNA. Only annotated protein-coding genes in significantly correlated GNMs were included in the IPA analysis, whereas functionally unannotated and non-protein-coding genes were excluded.
Results
Alignment and Transcriptome Assembly
After trimming of adapters, primers and poor-quality sequences, the data from the 12 samples initially included in the transcriptomic study comprised 30 to 50 M fragments per sample, which were subjected to further analysis. An average of 89.8% of the reads were aligned against the OAR_v3.1 reference genome. Finally, after performing the reference-guided transcriptome assembly, the final new annotation file after merging information across all 12 samples comprised 77,039 transcripts. These transcripts corresponded to a total of 44,203 transcribed gene loci, which is an average of 1.74 isoform transcripts per gene locus.
LncRNA Detection and Classification
After filtering out protein-coding transcripts, based on their coding potential score and nucleotide composition, the FEELnc program identified 9,105 lncRNA transcripts corresponding to 7,124 putative lncRNA loci. This corresponds to 1.28 lncRNA transcripts per lncRNA locus, which is lower than the average number of transcript isoforms across all gene loci. We observed an equal strand distribution of these lncRNA transcripts with 50.2% (3,580 lncRNA loci) identified on the plus strand and 49.7% (3,544 lncRNA loci) on the minus strand. The average number of exons per lncRNA locus was 3.3 (median 2) with a mean exon length of 432.2 nt (median149 nt). The average number of exons per non-lncRNA locus was 14.3 (median 2) with a mean exon length of 289.8 nt (median 130 nt).
To classify the identified lncRNA transcripts, we used the sliding window size of 10,000 to 100,000 nt to check for the possible overlap with the nearest transcript from the reference annotation. In total, we identified a total of 19,162 potential predicted spatial lncRNA interactions between 8,676 lncRNA transcripts (originating from 6,854 lncRNA loci) and other loci in the merged annotation. Out of these, 11,250 were intragenic interactions, called as ‘genic’ by the FEELnc classifier, and 7,912 were intergenic. No potential spatial interaction was predicted for the remaining 429 lncRNA transcripts (270 lncRNA loci). Some of the lncRNA transcripts interacted with both genic and intergenic regions of different partner transcripts. In total, 3,004 lncRNA transcripts were in sense direction with potentially interacting genic regions and 5,216 lncRNA transcripts in antisense direction. In case of intergenic regions, 3,143 lncRNA transcripts were predicted in sense direction and 2,758 in antisense direction. The mean expression of the 7,124 lncRNA loci was 60.6 FPKM, with a median of 9.13 FPKM and in case of the rest 37,079 non-lncRNA gene loci, the mean expression was 12.9 FPKM (median 0.88). Of the total of 9,105 lncRNA transcripts found in our analysis (7,124 lncRNA loci), 2,092 lncRNA transcripts (1,393 lncRNA loci) were categorized as novel by gffcompare. The distribution of novel and known lncRNA transcripts across the ovine chromosomes is shown in Figure 2.
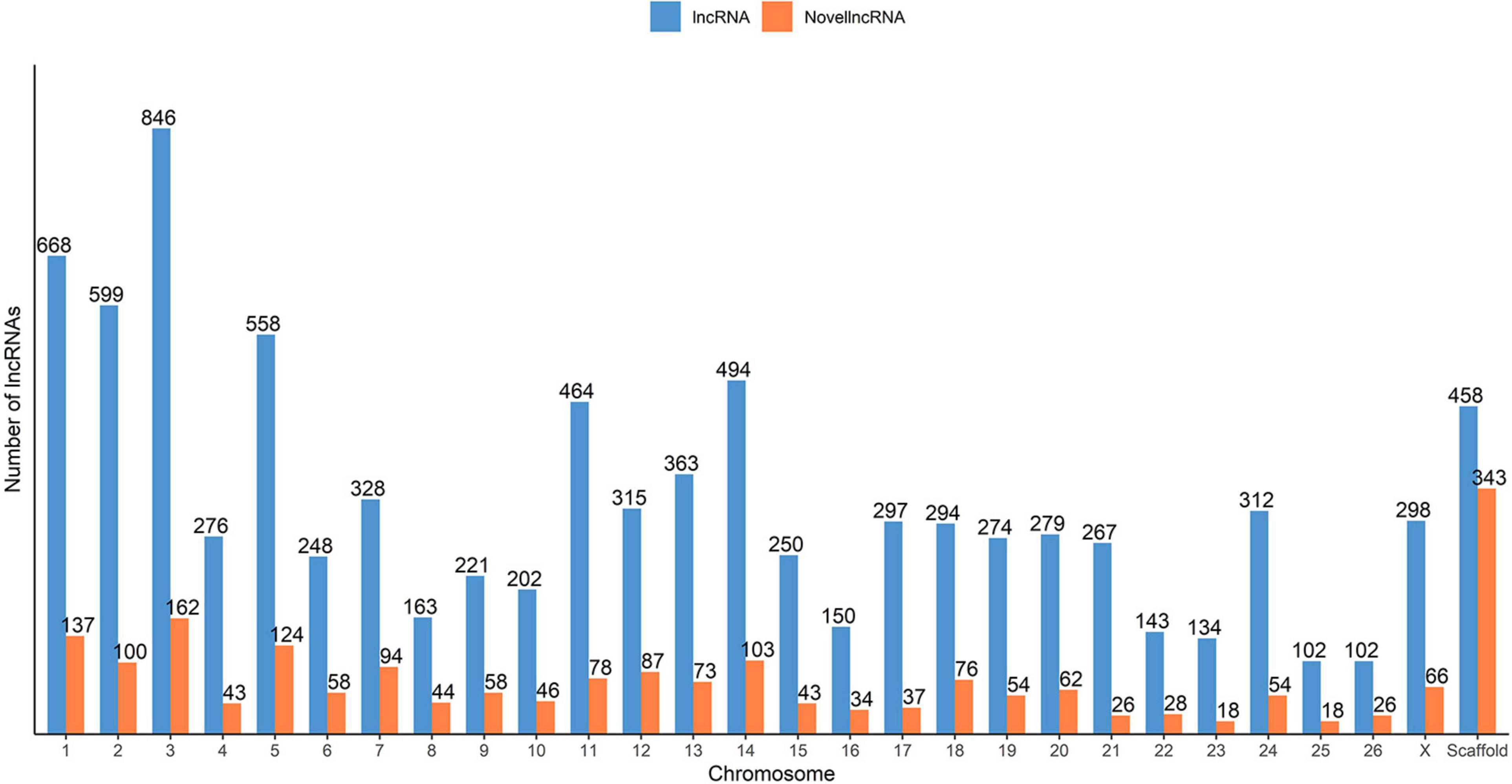
Figure 2. Distribution of the predicted putative lncRNAs across the sheep genome from the merged ALN RNA-seq datasets of the 12 ALN sheep transcriptome samples under study.
Differential Gene Expression Analysis
After excluding the outlier sample (ALN_14) detected through the PCA and evaluation of phenotypic FEC values (Figures 1A,B), a total of 3,148 differentially expressed loci (DELs, protein-coding and non-coding) were detected at a significance threshold of q < 0.05 between the ALN transcriptomes of the RES and SUS sheep groups in response to GIN infection. Of these, 1,635 DELs were higher and 1,513 DELs were lower expressed after GIN infection in RES sheep compared to the SUS group. The list of DELs is shown in Supplementary Table 1. When investigating the 3,148 DELs, we found 683 lncRNA transcripts in 457 DELs. Of these, 263 lncRNA transcripts associated with 153 DELs were considered novel. The list of DE lncRNAs including the novel DE lncRNAs is provided in Supplementary Table 1.
Gene Co-expression and Enrichment Analysis
The list of the 10 DE lncRNA loci selected for this analysis is shown in Table 1 and Figure 3 depicts results of the DE analysis for those 10 target DE lncRNA loci. The cluster dendrogram plot of the ALN transcriptome data from the RES and SUS sheep and the corresponding heatmap (Figure 4) show that the expression level of the 10 selected lncRNAs displayed a different pattern between the groups of differential resistance to GIN infection (Figure 4). Based on the module co-expression analysis with the 10 target lncRNAs, we found 88 GNMs (Supplementary Figure 3). Of them, 12 GNMs were significantly correlated (p < 0.01) with at least one of the 10 target lncRNAs included in the analysis (see Figure 4). Furthermore, we found that most lncRNAs were highly co-expressed with several GNMs at r > | 0.75| and p < 0.01.
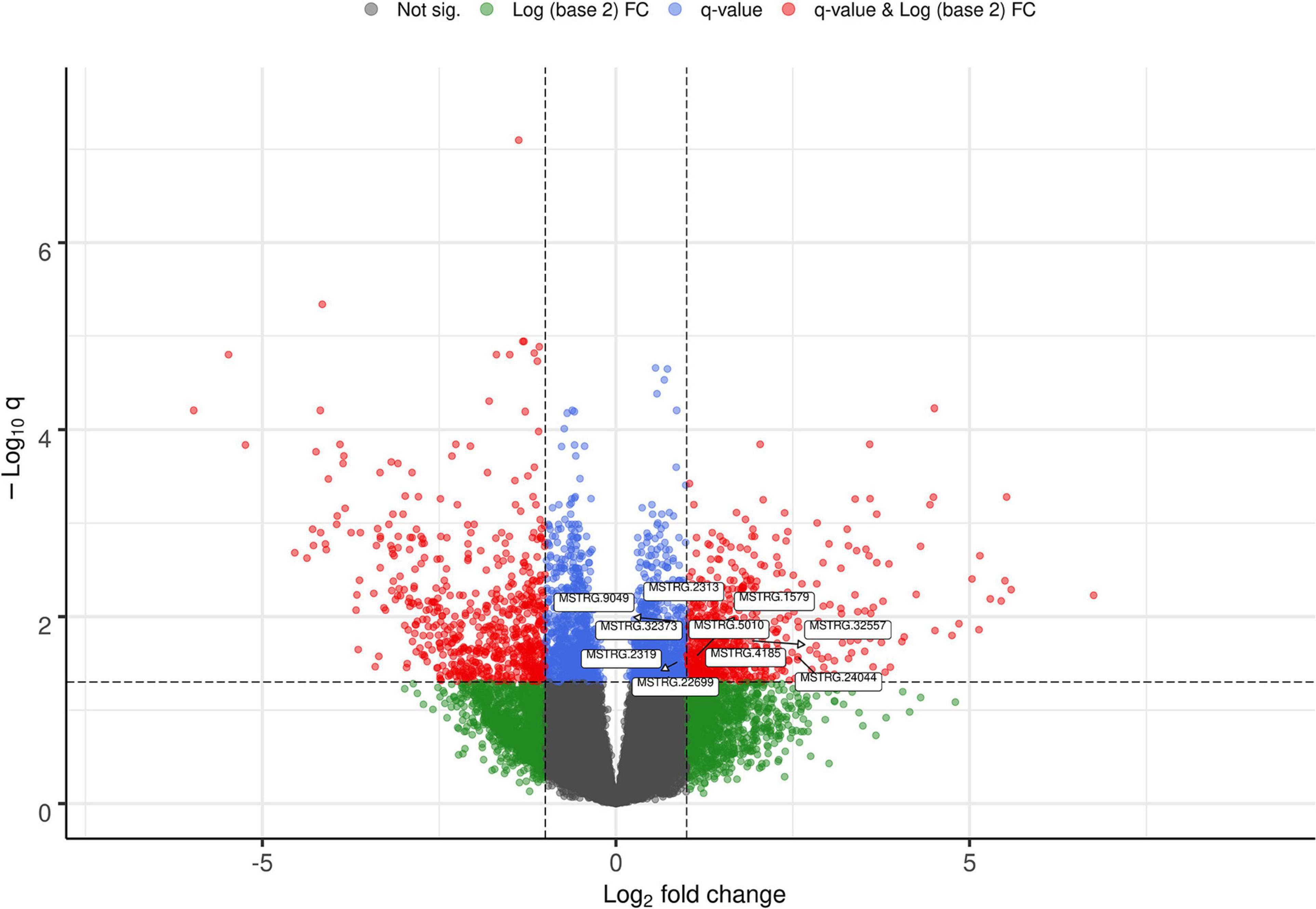
Figure 3. Volcano plot showing the differentially expressed (DE) genes with the selected target lncRNA loci for co-expression analysis.
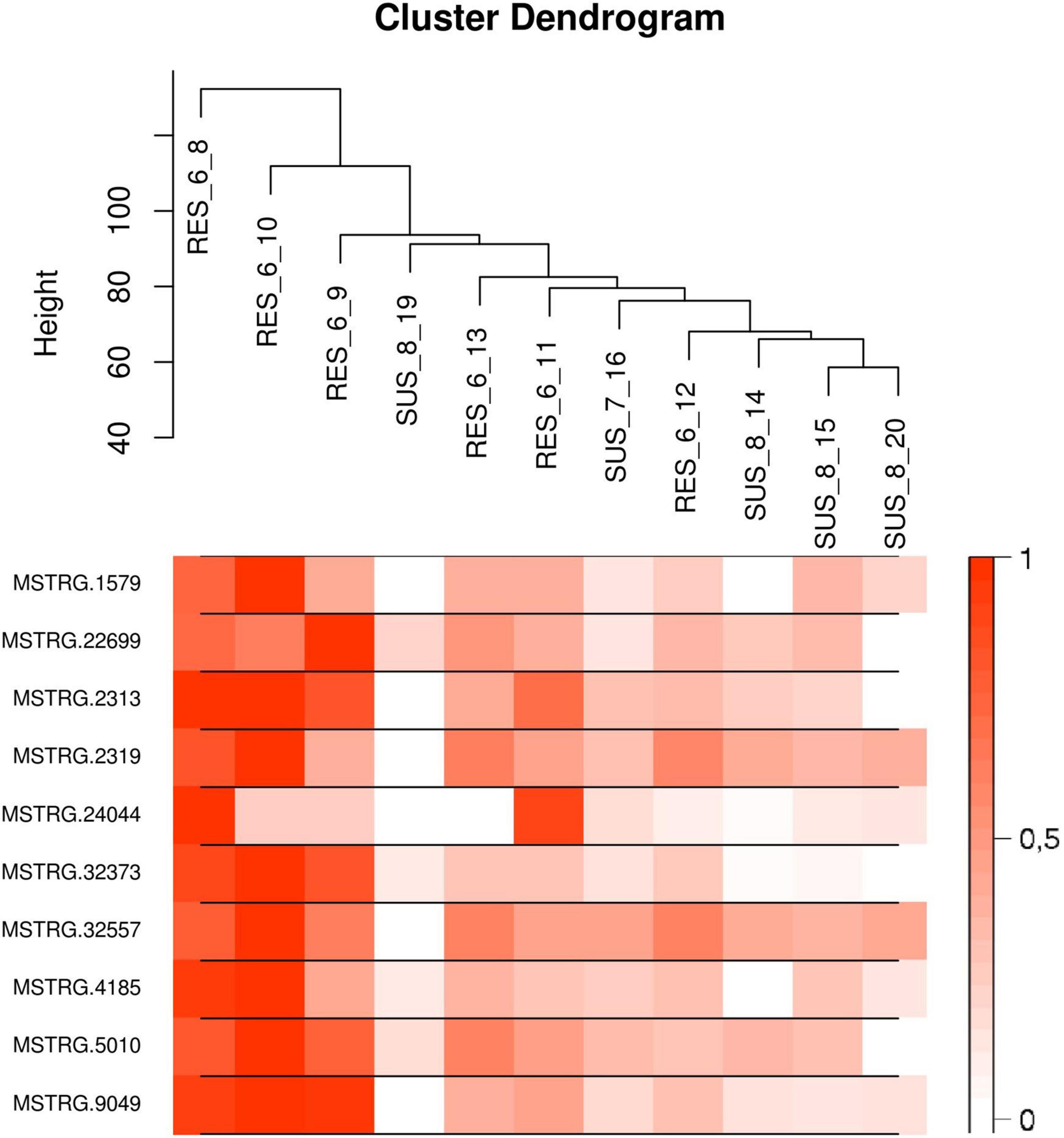
Figure 4. (Top) Cluster dendrogram plot of the ALN RNA-seq data to differentiate the resistant and susceptible sheep. (Bottom) Corresponding heatmap with the expression levels from the 10 selected lncRNAs showing a notable expression differentiation in resistant compared to susceptible samples.
For canonical biological pathway analysis, out of the 12 GNMs significantly correlated with at least one target lncRNA, we selected the DE genes from the three most highly significantly correlated GNMs: green, turquoise and yellow. Although other modules were also significantly co-expressed with the 10 selected lncRNAs, the genes from the other significantly correlated GNMs did not satisfy the criteria that were used to select the genes for enrichment pathway analysis. The blue and darkseagreen4 GNMs did not comprise DE genes and the other modules contained only a single or no protein-coding DE gene(s), which precluded biological pathway analysis. As shown in Table 2, Ingenuity pathway analysis revealed that the canonical pathways ERK5 (Extracellular signal-related kinase) Signaling, SAPK/JNK (stress-activated protein kinases) Signaling, RhoGDI Signaling, EIF2 (eukaryotic translation initiation factors) Signaling, Regulation of eIF4 (Eukaryotic translation initiation factor 4) and p70S6K Signaling and Oxidative Phosphorylation were significantly enriched by genes from GNMs green, turquoise and yellow. Table 2 also contains the list of DE genes within the enriched pathways for the three GNMs significantly correlated to at least one target lncRNA.
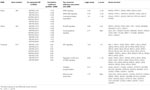
Table 2. Significant gene modules and canonical pathways associated with differentially expressed (DE) lncRNAs.
The co-expression and IPA analyses revealed that the ERK5 Signaling, SAPK/JNK Signaling and RhoGDI Signaling canonical pathways were enriched by genes included in the GNMs ‘green’ and ‘yellow’ (Table 2), which were both negatively correlated with expression levels of several DE lncRNAs (Table 2 and Figure 5). In addition, genes from GNM ‘turquoise’ that showed a positive correlation with DE lncRNA expression levels (Table 2 and Figure 5) were enriched in the canonical pathways EIF2 Signaling and Regulation of eIF4 and p70S6K Signaling (Table 2). It is striking that the same lncRNAs (MSTRG.1579, MSTRG.2313, MSTRG.2319, MSTRG.32373, MSTRG.32557, MSTRG.5010, MSTRG.9049, and MSTRG.4185) correlated with the GNMs ‘green,’ ‘yellow,’ and ‘turquoise’ indicating that they might be involved in the regulation of specific processes within the respective interconnected biological pathways, which display variation regarding resistance against GIN infection. An intersection between these pathways might be the mitogen-activated protein kinase (MAPK) pathway, which comprises the ERK, JNK and p38 mediated kinase cascades. It represents a conserved host-defense repertoire, and dysfunctions are known to result in hypersensitivity toward infection and stress (Johnson and Lapadat, 2002).
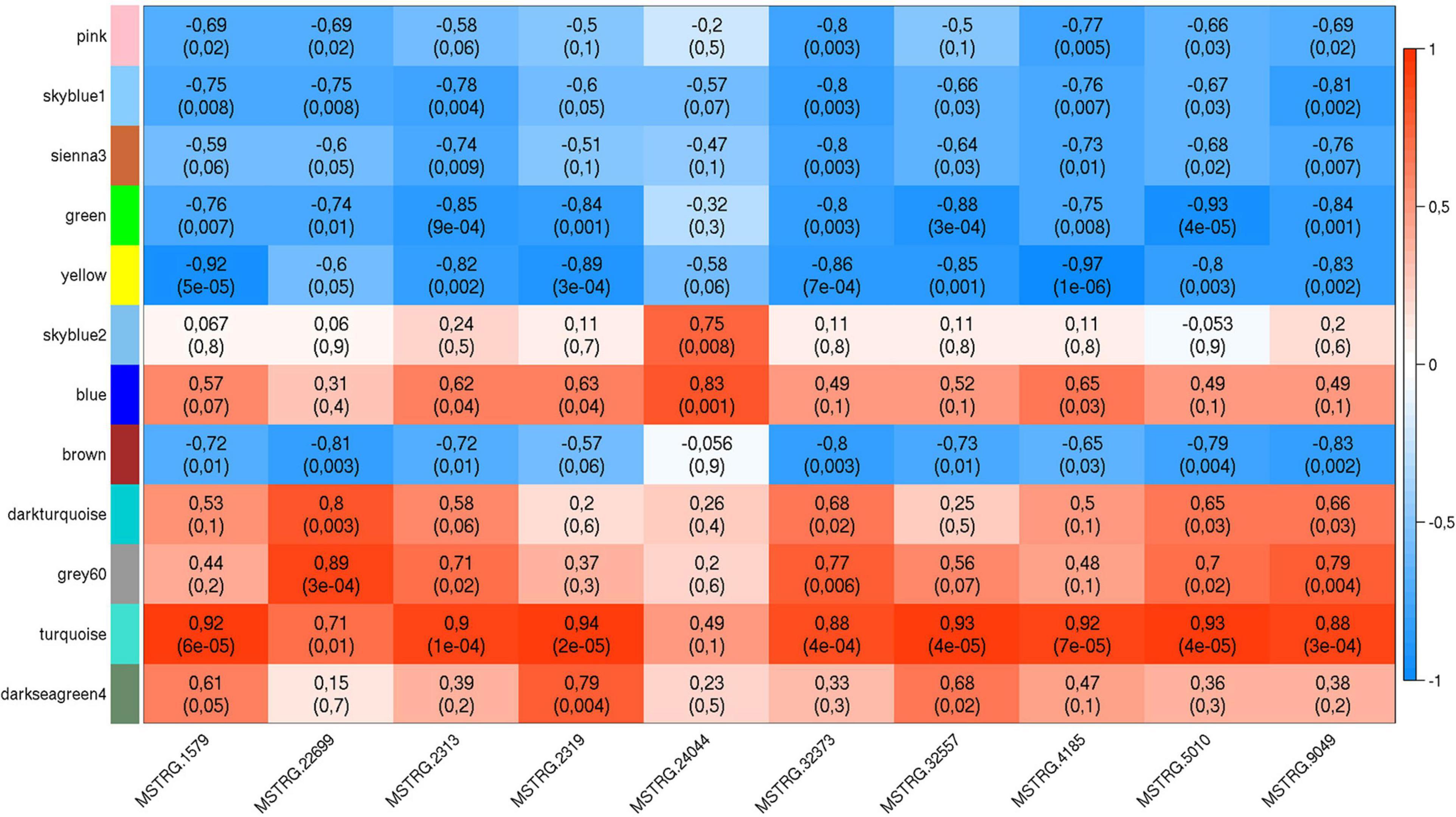
Figure 5. Weighted gene co-expression plot with co-expression blocks corresponding to the significantly co-expressed (p < 0.01) gene network modules (GNMs) (on the left) and the 10 selected DE lncRNAs (on the bottom). The left axis consists of color codes of each GNM, the right axis is the correlation coefficient (r) reference scale with integer value and color intensity (red to blue) adopted for each lncRNA and GNM block. Each block consists of correlation coefficient (on the top) and p-value (in brackets) corresponding to each GNM-lncRNA pair.
Discussion
LncRNAs are known to play multiple biological functions and their expression also varies with the developmental stage of cells and tissues and under different disease states and environmental challenges (Ma et al., 2012). Thus, by inferring the functional role of lncRNAs we can attempt to better understand the possible mechanisms of complex biological processes related to various metabolic disorders, disease conditions, divergent phenotypes and response to environmental challenges. However, compared to regular protein-coding genes, the sequences and secondary structures of lncRNA transcripts are usually not conserved (Mercer et al., 2009; Pang et al., 2009). This makes it difficult to investigate the function of lncRNAs directly based on their physical nucleotide structure and features. The availability of different bioinformatics tools such as CNCI (Sun et al., 2013), PLEK (Li et al., 2014), PLAR (Hezroni et al., 2015), and FEELnc (Wucher et al., 2017) has enabled to predict lncRNAs from unknown transcripts, but the detection of their biological functions is still challenging. It has been reported that lncRNAs exert their functions by regulating or interacting with other molecules like RNA, DNA and proteins (Ma et al., 2012; Schmitz et al., 2016). Thus, one possible approach to predict the function of lncRNAs is to explore the relationship between lncRNAs and other molecular interacting partners. One such approach is to identify the protein-coding genes that are co-expressed with the interrogated lncRNA. According to the principle of “guilt by association,” the putative lncRNA function can be assigned via correlation to a group of co-expressed genes (in a module) with the biological pathway enriched by the group of co-expressed genes (Wolfe et al., 2005). This approach has been applied for a variety of lncRNA studies in other species (Petri et al., 2015; Li et al., 2016; Zhang et al., 2017; Weikard et al., 2018; Zheng et al., 2018; Ling et al., 2019; Nolte et al., 2019; Thiel et al., 2019; Wang et al., 2020).
In this context, the current study was performed to predict ovine lncRNA transcripts in the ALN transcriptome and to investigate the functional role of DE lncRNAs in respective samples obtained from adult sheep after an experimental GIN infection. This was carried out in several steps: In the first step, we adopted a pipeline using bioinformatics tools for the detection and classification of lncRNAs expressed in the transcriptome of the ovine ALN. In the second step, differential gene expression and subsequent gene co-expression analyses were performed to establish co-expression networks of transcripts in gene expression modules, GNMs, followed by a correlation analysis between the expression level of 10 selected DE lncRNA loci and GNMs. In the final step, the potential functional role of these 10 target lncRNAs was predicted indirectly by performing gene enrichment and canonical biological pathway analyses with the DE genes of the significantly correlated GNMs.
Co-expression of LncRNAs With Genes From ERK5 and SAPK/JNK Signaling Pathways
The canonical pathway ERK5 (Extracellular signal-related kinase) Signaling (z-score = −2.5) (Supplementary Figure 4) was on top of the enriched pathways in the GNM ‘green.’ The involved signal transduction molecules are known to elicit several biological responses and to regulate cell functions such as tissue morphogenesis, cell proliferation, differentiation, migration and survival, apoptosis, cytoskeletal rearrangements, immune response and adaptation or stress response as reviewed by different authors (Dong et al., 2002; Drew et al., 2012; Arthur and Ley, 2013; Stecca and Rovida, 2019). The genes from the GNM ‘green,’ belonging to the ERK5 Signaling pathway, were ELK4 (encoding a transcription factor involved in both transcriptional activation and repression) and GNA13 (associated with PKA Signaling and Rho-related Signaling). They had a higher expression level in the ALN transcriptome of RES compared to SUS sheep in response to GIN challenge and were also found to be significantly higher expressed in susceptible goat (Bhuiyan et al., 2017) and in susceptible selection lines of Perendale sheep (Diez-Tascon et al., 2005), respectively, in response to GIN infection (Bhuiyan et al., 2017).
Pathway enrichment analysis of the GNM ‘green’ showed co-expression of lncRNAs with genes included in the SAPK/JNK Signaling pathway (z-score = −2.5). The stress-activated protein kinase/c-Jun N-terminal kinases (SAPK/JNK) are known to be affected by many types of cellular stresses and extracellular signals, such as UV irradiation, inflammatory cytokines and growth factors. They participate in numerous different intracellular signaling pathways that control cellular processes, including cell proliferation, differentiation, transformation and migration, cytoskeletal integrity and DNA repair (Nishina et al., 2004). Finally, the modulation of the SAPK/JNK pathway by stress stimuli results in transcriptional regulation of stress-related genes. In our study, the genes MAP3K13 and MAP3K7 of the MAPK family were included in the SAPK/JNK Signaling pathway (Table 2), and both were lower expressed in the ALN transcriptome of RES compared to SUS sheep. MAP3K7 is a key signaling component of nuclear factor-κB (NF-κB) and MAPK signaling pathways and acts as an essential regulator of innate immune signaling and apoptosis and of the proinflammatory signaling pathway. It also plays a central role in adaptive immunity in response to physical and chemical stresses [reviewed by Dai et al. (2012)]. MAP3K13 is able to activate JNK (Ikeda et al., 2001), is implicated in NF-κB activation (Ikeda et al., 2001; Masaki et al., 2003) and a role in a variety of developmental, stress-sensing, and disease contexts is assumed (Jin and Zheng, 2019).
Co-expression of LncRNAs With Genes Acting in RhoGDI and Axonal Guidance Signaling Pathways
Stress signals are known to be delivered to the SAPK/JNK signaling cascade by small GTPases of the Rho family. Our analysis revealed that lncRNAs mentioned above were also co-expressed with genes involved in pathways, which are linked to GTPases of the Rho family: RhoGDI (Rho-specific guanine nucleotide dissociation inhibitor) Signaling (z-score = 2.33) and 4 Actin-based Motility by Rho (z-score = −1.0) (Supplementary Table 2 and Figure 6). These pathways all were enriched in the GNM ‘yellow.’ Rho GDP dissociation inhibitors (RhoGDIs) play important roles in various cellular processes, including cell migration, adhesion and proliferation, differentiation, cytoskeletal reorganization and membrane trafficking by regulating the functions of the Rho GTPase family members. Dissociation of Rho GTPases from RhoGDIs is necessary for their spatiotemporal activation (Dransart et al., 2005; Garcia-Mata et al., 2011; Cho et al., 2019). Several DE genes in the RhoGDI Signaling pathway, such as ARHGEF10, DLC1 and ARHGDIA, were co-expressed with lncRNAs (Supplementary Table 2). The respective protein, ARHGEF10 is a guanine nucleotide exchange factor regulating activation of Rho GTPases, DLC1 belongs to the GTPase-activating proteins involved in the inactivation of Rho GTPases, whereas ARHGDIA is a RhoGDI, responsible for maintaining a stable pool of inactive Rho-GTPases (Stradal and Schelhaas, 2018). Co-expression of lncRNAs with the ARHGDIA gene that is higher expressed in the ALN transcriptome of RES compared to SUS sheep in our study might indicate a regulatory role of those lncRNAs for ARHGDIA expression. These lncRNAs were also co-expressed with ROCK kinase genes (ROCK1 and ROCK2), which are included in all enriched Rho-associated pathways mentioned above but also in several Ephrin Signaling -related and Axonal Guidance Signaling pathways (Supplementary Table 2). In our study, both ROCK kinase isoform transcripts were lower expressed in the ALN transcriptome of RES compared to SUS sheep. Multiple functions of ROCK kinases have been detected in biological processes including cell contraction, migration, apoptosis, survival, and proliferation (Julian and Olson, 2014). The proteins are key regulators of actin organization, which link them to the actin-depolymerizing factor CFL1 that showed higher expression in the ALN transcriptome of RES versus SUS sheep in our study. CFL1 acts in Rho-induced reorganization of the actin cytoskeleton (actin depolymerisation/filament stabilization) and is known as key player in controlling the temporal and spatial pattern of actin dynamics, which is crucial for mediating host–pathogen interactions (Zheng et al., 2016). All intracellular and even some extracellular pathogens affect the host cell cytoskeleton to promote their own survival, replication, and dissemination (Stradal and Schelhaas, 2018). In summary, the RhoGDI canonical pathway also in conjunction with other Rho- related and Ephrin- related signaling cascades as well as with the Axonal Guidance Signaling pathway might be involved in controlling the differential resistance to nematode infection in sheep and possibly, all of these processes may be modulated by co-expressed lncRNAs.
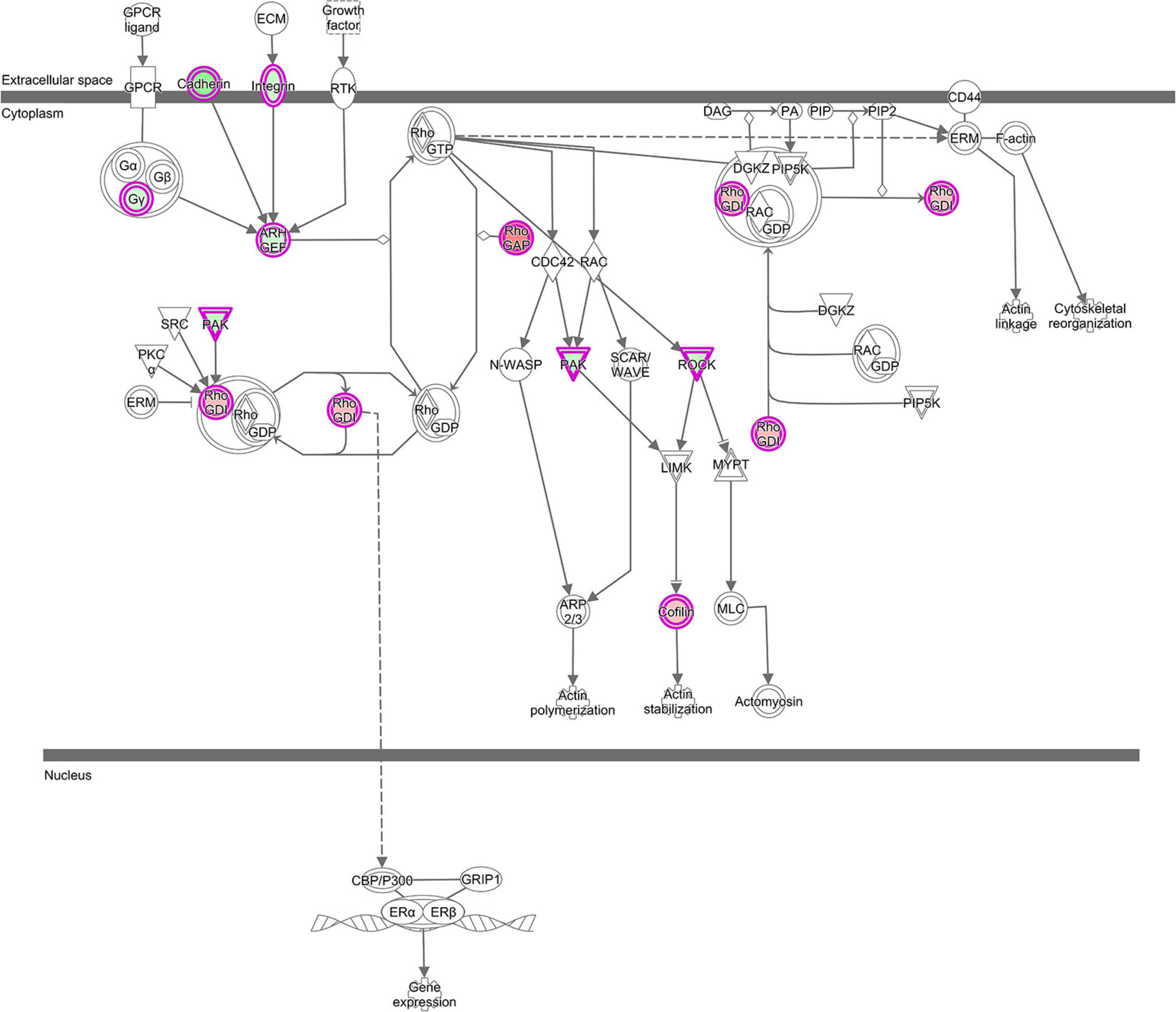
Figure 6. “RhoGDI Signaling canonical pathway” from IPA, was the top enriched pathway with genes from GNM ‘yellow’ with z-score = 2.33. This figure is adapted from Ingenuity Pathway Analysis®.
Co-expression of LncRNAs With Genes Involved in Protein Biosynthesis, Apoptosis and Mitochondrial Function Pathways
Furthermore, the co-expression analysis revealed that these lncRNAs were also connected with genes present in the GNM ‘turquoise’ suggesting a functional role of the canonical pathways EIF2 Signaling (z-score = 1.21) in conjunction with Regulation of eIF4 and p70S6K Signaling and mTOR Signaling (z-score = −1.34 for both) in sheep characterized by differential resistance to nematode challenge. The respective signaling pathways are engaged in the control of protein biosynthesis by regulating the translational machinery at different cascade steps (e.g., Supplementary Figures 5, 6). As translation is a tightly regulated process in response to various stimuli, including extracellular and intracellular signals and environmental stress conditions, translational control plays a major role in host stress responses, including pathogenic infection and defense by enabling rapid responses to the challenge (Mohr and Sonenberg, 2012). In our study, the participation of these biological pathways in the modulation of differential resistance to GIN challenge is supported by the upregulated expression of numerous ribosomal protein genes, and translation initiation factors, which are known to act in the ribosome biogenesis and the mRNA translational machinery. These genes from the GNM ‘turquoise’ showed a higher expression in the ALN transcriptome of sheep with a superior resistance against GIN. A crucial signaling mediator is the PDPK1 gene (3-phosphoinositide dependent protein kinase 1) that is involved in all three canonical pathways EIF2 Signaling, Regulation of eIF4 and p70S6K Signaling and mTOR Signaling. The encoding protein PDK1 is a master kinase, able to control numerous physiological and pathological processes (Di Blasio et al., 2017). In our study, PDPK1 had a lower expression level in the ALN transcriptome of SUS sheep compared to the RES group. But in goats, PDPK1 was significantly higher expressed in susceptible animals after GIN infection (Bhuiyan et al., 2017).
GNM ‘turquoise’ also contained the antiapoptotic BCL2 gene in the gene set associated with EIF2 signaling. The encoded BCL2 protein is known to be tightly connected with cellular survival. It suppresses apoptosis in many cell systems and regulates cell death by controlling the mitochondrial membrane permeability (Youle and Strasser, 2008; Siddiqui et al., 2015). Apoptosis, a mechanism by which cells infected with pathogens can be eliminated without triggering an unwanted inflammatory response, seemed to be upregulated in the RES group in response to GIN challenge in our study, which is supported by the higher BCL2 expression in the RES compared to the SUS sheep. In the study of Ingham et al. (2008), the BCL2 gene was quantified in the mucosa of the GIN tract of lambs of resistant and susceptible lines subjected to of Haemonchus contortus and Trichostrongylus colubriformis challenge, and it was identified as one of the candidate genes for maintaining the epithelial integrity of the gut in response to GIN. Furthermore, the BCL2 gene was higher expressed in the duodenum of in Naïve Perendale sheep genetically susceptible to GIN compared to resistant animals (Keane et al., 2006), which is in line with our observations.
A regulatory link of these pathways is suggested due to the simultaneous co-expression with several lncRNAs that were also upregulated in the ALN transcriptome of those sheep characterized by a better resistance to nematode challenge. The functional interplay between protein biosynthesis cascades and PI3K/AKT- and MAPK- related pathways is clearly illustrated by the intertwining of the GNM ‘turquoise’ with the GNMs ‘green’ and ‘yellow’ and the associated lncRNAs. A closer look at the genomic location of the correlated lncRNAs revealed that some of them (MSTRG.9049, MSTRG.32373, MSTRG.2313, and MSTRG.5010) are located in genomic regions near ribosomal protein genes, which would indicate a regulatory potential for them to control the expression of the respective neighbored genes via cis -regulation. Considering that host cells respond to stress and imbalances by modifying gene expression at epigenetic, transcriptional and translational levels for recovering from the pathogen attacks, Knap et al. (2017) reviewed the state of knowledge on whether host cells would deploy lncRNAs to rapidly control host translation, the most energy-consuming process in cells, to counteract infection. The authors noted that the cause-effect relationship between the expression of lncRNAs and the activation of signaling pathways that control translation is currently unclear, but it is tempting to speculate that host cells could use this class of ncRNAs to fine-tune translation and cope with the imbalances triggered by pathogens.
Interestingly, numerous genes contained in the GNM ‘turquoise’ indicated an association of the correlated lncRNAs with the intertwined canonical pathways Oxidative Phosphorylation (z – score = 2.45) and Mitochondrial Dysfunction. These genes encode for various protein components of all complexes forming the mitochondrial electron transport chain, which drives oxidative phosphorylation and appeared to be upregulated in the ALN transcriptome of the sheep group with a better resistance to GIN challenge (Supplementary Figure 7). Mitochondria are the key organelles of energy production and coordinate essential metabolic processes in the cells. In addition to their central role in metabolism, mitochondria also regulate cellular processes such as cell cycle, innate immunity and apoptosis [summarized by Mills et al. (2017) and Mohanty et al. (2019)]. Recent findings highlight the emerging role of mitochondria as important intracellular signaling platform that regulates innate immune and inflammatory responses (Jin et al., 2017). LncRNAs have been reported to be involved in regulating mitochondrial processes such as mitochondrial respiration, reactive oxygen production and apoptosis (De Paepe et al., 2018) and are hypothesized to coordinate functions between mitochondria and the nucleus (Dong et al., 2017). To maintain homeostasis of the cells, an intense cross-talk between mitochondria and the nucleus, mediated by lncRNAs is conceivable. Thus, lncRNAs that were highly correlated to genes of the GNM ‘turquoise’ might be associated with the regulation of mitochondrial bioenergetics and biosynthesis. Taken together, of the 10 DE lncRNAs selected for co-expression analysis, eight were found to be potentially involved in the regulation of these biological pathways with relevance to GIN infection, namely MSTRG.1579, MSTRG.2313, MSTRG.2319, MSTRG.32373, MSTRG.32557, MSTRG.5010, MSTRG.9049, and MSTRG.4185 (Table 2). Thus, these lncRNAs may contribute to the divergent resistance to gastrointestinal parasites.
Potential Functional Genomic Interaction Partners of Target LncRNAs Potentially Involved in Regulating Divergent Resistance to Gastrointestinal Parasites in Sheep
LncRNAs might activate or repress the transcription of nearby genes (cis-regulation) present on the same (Engreitz et al., 2016) or opposite strand (Villegas and Zaphiropoulos, 2015; Tan-Wong et al., 2019).
As already mentioned, MSTRG.9049, MSTRG.32373, MSTRG.2313, and MSTRG.5010 are located in genomic regions antisense to DE ribosomal protein genes, RPS19, RPS20, and RPL35A, RPL23 indicating a potential functional regulatory link to translation-associated processes.
MSTRG.1579 is overlapping with a known lincRNA (ENSOARG00000025559), and the TAGLN2 gene region. The TAGLN2 is an actin binding protein and regulates the T cell activation in mammals by stabilizing the actin cytoskeleton (Na and Jun, 2015).
MSTRG.2319 represents a novel, not yet annotated intergenic lncRNA localized between the genes MUC20 and TNK2, which however, were not DE between the different GIN-resistance groups. MUC20 encodes a member of the mucin glycoprotein family implicated in protection of all mucosal surfaces. Membrane bound mucins have been suggested to play a functional role in cell signaling linked to health and disease (Corfield et al., 2001; Linden et al., 2008). A MUC20 -lncRNA has been reported to bind ROCK1 and to be functionally involved in tumor suppression (Dai et al., 2020) indicating trans-regulation. TNK2 encodes a tyrosine kinase that binds to the Rho family member Cdc42Hs and inhibits both the intrinsic and GTPase-activating protein-stimulated GTPase activity (Manser et al., 1993). Thus, MSTRG.2319 could be primarily involved in the pathway Signaling by Rho Family GTPases.
The lncRNA MSTRG.32557 is located near the FABP4 gene region, which encodes a member of the fatty acid binding protein family that plays a role in lipid metabolism by binding and intracellular transport of long-chain fatty acids. FABP4 showed a higher expression level in the ALN transcriptome of RES sheep and was found to be DE between RES and SUS sheep groups in our previous transcriptome study performed with the same tissue and conditions (Chitneedi et al., 2018). Other studies also imply roles of FABP family proteins in cell signaling, inhibition of cell growth and cellular differentiation. Furthermore, FABP also modulates tumor cell growth, metabolism, migration, differentiation and development involving the PI3K/AKT signaling and PPAR-associated pathways (Amiri et al., 2018). Specifically, FABP4 has been found to function as an adipokine that is involved in regulating macrophage and adipocyte interactions during inflammation (Thumser et al., 2014). A GWAS study showed that FABP4 contributes to resistance to fleece rot in Australian merino sheep (Smith et al., 2010). This gene was also found to be downregulated in response to GIN Cooperia oncophora infection in cattle (Li and Schroeder, 2012).
The lncRNA MSTRG.4185 is located close to the chemokine (C-C motif) ligand 14 (CCL14) gene region. The cytokine encoded by this gene induces changes in intracellular calcium concentration and enzyme release in monocytes and showed strong correlation with tumor immune cells infiltration (Gu et al., 2020). The expression of CCL14 along with other chemokine ligands was reported to be significantly higher in a resistance sheep flock compared to a susceptible flock after Haemonchus contortus infection (Zhang et al., 2019). As the CCL14 gene was not DE between RES and SUS sheep groups in our study, but MSTRG.4185 was highly correlated with GNMs (most negatively to the ‘yellow’ GNM and also positively correlated to the ‘turquoise’ GNM, Figure 4), a putative trans-regulation of genes included in correlated biological pathways could be assumed.
The lncRNAs, which are co-expressed with the GNMs ‘green,’ ‘yellow,’ and ‘turquoise,’ could possibly be involved in the regulation of the expression of genes included in the respective network modules. These modules, in turn are involved in pathways associated with different physiological and environmental conditions as well as with divergent phenotypic characteristics, such as the modulation of a differential resistance or susceptibility of adult sheep to parasite infection. Results obtained from this study only provide first hints on the genes and pathways that are primarily targeted by individual lncRNAs under interrogation. As this is an initial study, further research in sheep in response to GIN infection will be required to establish the functional role of the detected lncRNA transcripts.
Overall, this preliminary lncRNA study, conducted in adult sheep after GIN infection gave first insights into the potential functional role of selected lncRNAs by investigating their putative functions via co-expressed genes based on the principle of “guilt-by-association.” Future multi-omics studies including DNA, RNA and metabolites will help to gain a better understanding of the general and specific roles of the selected lncRNAs and other lncRNAs significantly involved in the regulation of key physiological pathways associated with resistance against GIN infection in sheep.
Data Availability Statement
The 12 abomasal lymph node raw RNA-Seq datasets analyzed in this study have been submitted to the ENA Read Archive at EMBL-EBI following FAANG DCC guidelines. They are available at https://www.ebi.ac.uk/ena/browser/view/PRJEB33 476 (Bioproject PRJEB33476). Data on the meta data of the samples are submitted as https://www.ebi.ac.uk/biosamples/samples/SAMEG4750853.
Ethics Statement
The animal study was reviewed and approved by the subcommittee for experimentation and animal welfare (OBEA) of the University of León and the competent body of the Junta de Castilla y León regional government (Spain) (Ref. ULE_024_2015).
Author Contributions
BG-G and JA conceived and designed the study, and performed the collection and processing of tissue samples. MM-V designed and performed the experimental infections. BG-G, JA, and MM-V performed sampling in the commercial flock and selected the animals to be experimentally infected. CK and RW optimized the bioinformatics workflow. PC performed the bioinformatics analyses under the supervision of CK. PC and RW wrote the manuscript. BG-G, CK, and JA critically reviewed the manuscript. All authors read and approved the final manuscript.
Funding
Financial support for the experimental work of this project was received from the LE248U14 project of the Junta de Castilla and León regional government, whereas the storage and processing of the generated sequencing datasets have been funded by the RTI2018-093535-B-I00 project from the Spanish Ministry of Science and Innovation (MICINN). PC was funded by a Short-Term Scientific Missions of the Functional Annotation of Animal Genomes-European network (FAANG-Europe) COST Action CA15112 to do a short research stay at Leibniz Institute for Farm Animal Biology (FBN). MM-V was also funded by the “Ramón y Cajal” Programme (RYC-2015-18368) from MICINN.
Conflict of Interest
The authors declare that the research was conducted in the absence of any commercial or financial relationships that could be construed as a potential conflict of interest.
Acknowledgments
The authors acknowledge the FAANG-Europe Cost Action (CA15112) for funding the Short Term Scientific Mission that settled the basis for this collaborative study. Authors thank to the National Breeders Association of Churra sheep for collaboration in the sampling of commercial flocks at the beginning of this project.
Supplementary Material
The Supplementary Material for this article can be found online at: https://www.frontiersin.org/articles/10.3389/fgene.2021.685341/full#supplementary-material
Supplementary Figure 1 | Bioinformatics workflow showing the different steps performed to detect long non-coding RNAs (lncRNAs) in the abomasal lymph node transcriptome from resistant or susceptible sheep to GIN infection and to predict their potential functional role by gene co-expression and pathway enrichment analysis.
Supplementary Figure 2 | Distribution of accumulated fecal egg count (FEC) in resistant and susceptible sheep after a second experimental infection with GIN T. circumcincta. Blue bars represent the FEC values in each resistant sample and yellow bars represent the FEC values in each susceptible sample. (B) PCA performed with the read count matrix of ALN RNA-seq datasets using R function “varianceStabilizingTransformation” by specifying the sample condition (resistant and susceptible) with the initially considered 6 resistant and 6 susceptible samples.
Supplementary Figure 3 | Weighted gene co-expression network matrix plot. Each block corresponds to the co-expression result of each gene network module (GNM, left) and a lncRNA (bottom). The left axis consists of color codes for each GNM and the right axis is the correlation coefficient (r) reference scale with integer values and color intensity (red to blue) adopted for each lncRNA and GNM block. Each block consists of the correlation coefficient (top) and p-value (bottom) corresponding to each GNM-lncRNA pair.
Supplementary Figure 4 | “EIF2 Signaling” from IPA, was the top enriched pathway with genes from the GNM ‘turquoise’ with z-score = 1.21. This figure is adapted from Ingenuity Pathway Analysis®.
Supplementary Figure 5 | “ERK5 Signaling” from IPA, was the top enriched pathway with genes from the GNM ‘green’ with z-score = −2.5. This figure is adapted from Ingenuity Pathway Analysis®.
Supplementary Figure 6 | “Regulation of eIF4 and p70S6K Signaling” from IPA, was the second top enriched pathway with genes from the GNM ‘turquoise’ with z-score = −1.34. This figure is adapted from Ingenuity Pathway Analysis®.
Supplementary Figure 7 | “Oxidative Phosphorylation” from IPA, was one of the top enriched pathways with genes from the GNM ‘turquoise’ with z-score = 4.00. This figure is adapted from Ingenuity Pathway Analysis®.
Supplementary Table 1 | Differentially expressed gene loci sorted based on p-value and the list of associated long non-coding RNAs (lncRNAs) along with the information of novel lncRNAs.
Supplementary Table 2 | List of top 20 pathways based on −log (p-value) enriched by the genes from the three GNMs green, yellow and turquoise according to the Ingenuity Pathway Analysis®.
Supplementary File 1 | Bash script with all the parameters adapted for different tools used in the bioinformatics workflow of lncRNA detection.
Footnotes
- ^ http://www.ensembl.org/Ovis_aries_rambouillet/Info/Strains?db=core
- ^ http://www.ensembl.org/Ovis_aries_rambouillet/Info/Index
- ^ http://ftp.ensembl.org/pub/release-95/gtf/ovis_aries/
- ^ https://digitalinsights.qiagen.com/products-overview/discovery-insights-portfolio/analysis-and-visualization/qiagen-ipa/
References
Amiri, M., Yousefnia, S., Seyed Forootan, F., Peymani, M., Ghaedi, K., and Nasr Esfahani, M. H. (2018). Diverse roles of fatty acid binding proteins (FABPs) in development and pathogenesis of cancers. Gene 676, 171–183. doi: 10.1016/j.gene.2018.07.035
Andersson, L., Archibald, A. L., Bottema, C. D., Brauning, R., Burgess, S. C., Burt, D. W., et al. (2015). Coordinated international action to accelerate genome-to-phenome with FAANG, the functional annotation of animal genomes project. Genome Biol. 16:57. doi: 10.1186/s13059-015-0622-4
Arthur, J. S., and Ley, S. C. (2013). Mitogen-activated protein kinases in innate immunity. Nat. Rev. Immunol. 13, 679–692. doi: 10.1038/nri3495
Bhuiyan, A. A., Li, J., Wu, Z., Ni, P., Adetula, A. A., Wang, H., et al. (2017). Exploring the genetic resistance to gastrointestinal nematodes infection in goat using RNA-sequencing. Int. J. Mol. Sci. 18:e040751. doi: 10.3390/ijms18040751
Bush, S. J., Muriuki, C., Mcculloch, M. E. B., Farquhar, I. L., Clark, E. L., and Hume, D. A. (2018). Cross-species inference of long non-coding RNAs greatly expands the ruminant transcriptome. Genet. Sel. Evol. 50:20. doi: 10.1186/s12711-018-0391-0
Cao, H., Wahlestedt, C., and Kapranov, P. (2018). Strategies to annotate and characterize long noncoding RNAs: advantages and pitfalls. Trends Genet. 34, 704–721. doi: 10.1016/j.tig.2018.06.002
Castilla-Gomez De Aguero, V., Gonzalez, J. F., Hernandez, J. N., Valderas-Garcia, E., Rojo Vazquez, F. A., Arranz, J. J., et al. (2020). Differences within Churra breed sheep in the early immune response to the infection by Teladorsagia circumcincta. Parasitol. Res. 120, 1115–1120. doi: 10.1007/s00436-020-06953-4
Chen, Y. G., Satpathy, A. T., and Chang, H. Y. (2017). Gene regulation in the immune system by long noncoding RNAs. Nat. Immunol. 18, 962–972. doi: 10.1038/ni.3771
Chitneedi, P. K., Arranz, J. J., Suarez-Vega, A., Martinez-Valladares, M., and Gutierrez-Gil, B. (2020). Identification of potential functional variants underlying ovine resistance to gastrointestinal nematode infection by using RNA-Seq. Anim. Genet. 51, 266–277. doi: 10.1111/age.12894
Chitneedi, P. K., Suarez-Vega, A., Martinez-Valladares, M., Arranz, J. J., and Gutierrez-Gil, B. (2018). Exploring the mechanisms of resistance to Teladorsagia circumcincta infection in sheep through transcriptome analysis of abomasal mucosa and abomasal lymph nodes. Vet. Res. 49:39. doi: 10.1186/s13567-018-0534-x
Cho, H. J., Kim, J. T., Baek, K. E., Kim, B. Y., and Lee, H. G. (2019). Regulation of Rho GTPases by RhoGDIs in human cancers. Cells 8:1037. doi: 10.3390/cells8091037
Clark, E. L., Bush, S. J., Mcculloch, M. E. B., Farquhar, I. L., Young, R., Lefevre, L., et al. (2017). A high resolution atlas of gene expression in the domestic sheep (Ovis aries). PLoS Genet. 13:e1006997. doi: 10.1371/journal.pgen.1006997
The ENCODE Project Consortium (2012). An integrated encyclopedia of DNA elements in the human genome. Nature 489, 57–74. doi: 10.1038/nature11247
Corfield, A. P., Carroll, D., Myerscough, N., and Probert, C. S. (2001). Mucins in the gastrointestinal tract in health and disease. Front. Biosci. 6, D1321–D1357. doi: 10.2741/corfield
Dai, L., Aye Thu, C., Liu, X. Y., Xi, J., and Cheung, P. C. (2012). TAK1, more than just innate immunity. IUBMB Life 64, 825–834. doi: 10.1002/iub.1078
Dai, R., Zhou, Y., Chen, Z., Zou, Z., Pan, Z., Liu, P., et al. (2020). Lnc-MUC20-9 binds to ROCK1 and functions as a tumor suppressor in bladder cancer. J. Cell Biochem. 121, 4214–4225. doi: 10.1002/jcb.29626
De Paepe, B., Lefever, S., and Mestdagh, P. (2018). How long Noncoding RNAs enforce their will on mitochondrial activity: regulation of mitochondrial respiration, reactive oxygen species production, apoptosis, and metabolic reprogramming in cancer. Curr. Genet. 64, 163–172. doi: 10.1007/s00294-017-0744-1
Derrien, T., Johnson, R., Bussotti, G., Tanzer, A., Djebali, S., Tilgner, H., et al. (2012). The GENCODE v7 catalog of human long noncoding RNAs: analysis of their gene structure, evolution, and expression. Genome Res. 22, 1775–1789. doi: 10.1101/gr.132159.111
Di Blasio, L., Gagliardi, P. A., Puliafito, A., and Primo, L. (2017). Serine/Threonine Kinase 3-phosphoinositide-dependent protein Kinase-1 (PDK1) as a key regulator of cell migration and cancer dissemination. Cancers 9:25. doi: 10.3390/cancers9030025
Diez-Banos, N., Cabaret, J., and Diez-Banos, P. (1992). Interspecific interactions in naturally acquired nematode communities from sheep abomasum in relation to age of host and season in four areas of Leon (Spain). Int. J. Parasitol. 22, 327–334. doi: 10.1016/s0020-7519(05)80010-4
Diez-Tascon, C., Keane, O. M., Wilson, T., Zadissa, A., Hyndman, D. L., Baird, D. B., et al. (2005). Microarray analysis of selection lines from outbred populations to identify genes involved with nematode parasite resistance in sheep. Physiol. Genom. 21, 59–69. doi: 10.1152/physiolgenomics.00257.2004
Dong, C., Davis, R. J., and Flavell, R. A. (2002). MAP kinases in the immune response. Annu. Rev. Immunol. 20, 55–72. doi: 10.1146/annurev.immunol.20.091301.131133
Dong, Y., Yoshitomi, T., Hu, J. F., and Cui, J. (2017). Long noncoding RNAs coordinate functions between mitochondria and the nucleus. Epigenet. Chromat. 10:41. doi: 10.1186/s13072-017-0149-x
Dransart, E., Olofsson, B., and Cherfils, J. (2005). RhoGDIs revisited: novel roles in Rho regulation. Traffic 6, 957–966. doi: 10.1111/j.1600-0854.2005.00335.x
Drew, B. A., Burow, M. E., and Beckman, B. S. (2012). MEK5/ERK5 pathway: the first fifteen years. Biochim. Biophys. Acta 1825, 37–48. doi: 10.1016/j.bbcan.2011.10.002
Dykes, I. M., and Emanueli, C. (2017). Transcriptional and post-transcriptional gene regulation by long non-coding RNA. Genom. Proteom. Bioinform. 15, 177–186. doi: 10.1016/j.gpb.2016.12.005
Engreitz, J. M., Haines, J. E., Perez, E. M., Munson, G., Chen, J., Kane, M., et al. (2016). Local regulation of gene expression by lncRNA promoters, transcription and splicing. Nature 539, 452–455. doi: 10.1038/nature20149
Garcia-Mata, R., Boulter, E., and Burridge, K. (2011). The ‘invisible hand’: regulation of RHO GTPases by RHOGDIs. Nat. Rev. Mol. Cell Biol. 12, 493–504. doi: 10.1038/nrm3153
Gu, Y., Li, X., Bi, Y., Zheng, Y., Wang, J., Li, X., et al. (2020). CCL14 is a prognostic biomarker and correlates with immune infiltrates in Hepatocellular carcinoma. Aging 12, 784–807. doi: 10.18632/aging.102656
Hezroni, H., Koppstein, D., Schwartz, M. G., Avrutin, A., Bartel, D. P., and Ulitsky, I. (2015). Principles of long noncoding RNA evolution derived from direct comparison of transcriptomes in 17 species. Cell Rep. 11, 1110–1122. doi: 10.1016/j.celrep.2015.04.023
Hiller, M., Findeiss, S., Lein, S., Marz, M., Nickel, C., Rose, D., et al. (2009). Conserved introns reveal novel transcripts in Drosophila melanogaster. Genome Res. 19, 1289–1300. doi: 10.1101/gr.090050.108
Hon, C. C., Ramilowski, J. A., Harshbarger, J., Bertin, N., Rackham, O. J., Gough, J., et al. (2017). An atlas of human long non-coding RNAs with accurate 5’ ends. Nature 543, 199–204. doi: 10.1038/nature21374
Ikeda, A., Masaki, M., Kozutsumi, Y., Oka, S., and Kawasaki, T. (2001). Identification and characterization of functional domains in a mixed lineage kinase LZK. FEBS Lett. 488, 190–195. doi: 10.1016/s0014-5793(00)02432-7
Ingham, A., Reverter, A., Windon, R., Hunt, P., and Menzies, M. (2008). Gastrointestinal nematode challenge induces some conserved gene expression changes in the gut mucosa of genetically resistant sheep. Int. J. Parasitol 38, 431–442. doi: 10.1016/j.ijpara.2007.07.012
Jin, H. S., Suh, H. W., Kim, S. J., and Jo, E. K. (2017). Mitochondrial control of innate immunity and inflammation. Immune Netw. 17, 77–88. doi: 10.4110/in.2017.17.2.77
Jin, Y., and Zheng, B. (2019). Multitasking: dual leucine zipper-bearing kinases in neuronal development and stress management. Annu. Rev. Cell Dev. Biol. 35, 501–521. doi: 10.1146/annurev-cellbio-100617-062644
Johnson, G. L., and Lapadat, R. (2002). Mitogen-activated protein kinase pathways mediated by ERK, JNK, and p38 protein Kinases. Science 298, 1911–1912. doi: 10.1126/science.1072682
Julian, L., and Olson, M. F. (2014). Rho-associated coiled-coil containing kinases (ROCK): structure, regulation, and functions. Small GTPases 5:e29846. doi: 10.4161/sgtp.29846
Keane, O. M., Zadissa, A., Wilson, T., Hyndman, D. L., Greer, G. J., Baird, D. B., et al. (2006). Gene expression profiling of naive sheep genetically resistant and susceptible to gastrointestinal nematodes. BMC Genom. 7:42. doi: 10.1186/1471-2164-7-42
Kim, T. K., Hemberg, M., and Gray, J. M. (2015). Enhancer RNAs: a class of long noncoding RNAs synthesized at enhancers. Cold Spring Harb. Perspect. Biol. 7:a018622. doi: 10.1101/cshperspect.a018622
Knap, P., Tebaldi, T., Di Leva, F., Biagioli, M., Dalla Serra, M., and Viero, G. (2017). The unexpected tuners: are LncRNAs regulating host translation during infections? Toxins 9:357. doi: 10.3390/toxins9110357
Kopp, F., and Mendell, J. T. (2018). Functional classification and experimental dissection of long noncoding RNAs. Cell 172, 393–407. doi: 10.1016/j.cell.2018.01.011
Langfelder, P., and Horvath, S. (2008). WGCNA: an R package for weighted correlation network analysis. BMC Bioinform. 9:559. doi: 10.1186/1471-2105-9-559
Li, A., Zhang, J., and Zhou, Z. (2014). PLEK: a tool for predicting long non-coding RNAs and messenger RNAs based on an improved k-mer scheme. BMC Bioinform. 15:311. doi: 10.1186/1471-2105-15-311
Li, H. (2011). A statistical framework for SNP calling, mutation discovery, association mapping and population genetical parameter estimation from sequencing data. Bioinformatics 27, 2987–2993. doi: 10.1093/bioinformatics/btr509
Li, J., Gao, Z., Wang, X., Liu, H., Zhang, Y., and Liu, Z. (2016). Identification and functional analysis of long intergenic noncoding RNA genes in porcine pre-implantation embryonic development. Sci. Rep. 6:38333. doi: 10.1038/srep38333
Li, R. W., and Schroeder, S. G. (2012). Cytoskeleton remodeling and alterations in smooth muscle contractility in the bovine jejunum during nematode infection. Funct. Integr. Genom. 12, 35–44. doi: 10.1007/s10142-011-0259-7
Liao, Y., Smyth, G. K., and Shi, W. (2013). The Subread aligner: fast, accurate and scalable read mapping by seed-and-vote. Nucleic Acids Res. 41:e108. doi: 10.1093/nar/gkt214
Linden, S. K., Sutton, P., Karlsson, N. G., Korolik, V., and Mcguckin, M. A. (2008). Mucins in the mucosal barrier to infection. Mucosal Immunol. 1, 183–197. doi: 10.1038/mi.2008.5
Ling, Y., Zheng, Q., Sui, M., Zhu, L., Xu, L., Zhang, Y., et al. (2019). Comprehensive analysis of LncRNA reveals the temporal-specific module of goat skeletal muscle development. Int. J. Mol. Sci. 20:3950. doi: 10.3390/ijms20163950
Love, M. I., Huber, W., and Anders, S. (2014). Moderated estimation of fold change and dispersion for RNA-seq data with DESeq2. Genome Biol. 15:550. doi: 10.1186/s13059-014-0550-8
Ma, H., Hao, Y., Dong, X., Gong, Q., Chen, J., Zhang, J., et al. (2012). Molecular mechanisms and function prediction of long noncoding RNA. Sci. World J. 2012:541786. doi: 10.1100/2012/541786
Manser, E., Leung, T., Salihuddin, H., Tan, L., and Lim, L. (1993). A non-receptor tyrosine kinase that inhibits the GTPase activity of p21cdc42. Nature 363, 364–367. doi: 10.1038/363364a0
Maracaja-Coutinho, V., Paschoal, A. R., Caris-Maldonado, J. C., Borges, P. V., Ferreira, A. J., and Durham, A. M. (2019). Noncoding RNAs databases: current status and trends. Methods Mol. Biol. 1912, 251–285. doi: 10.1007/978-1-4939-8982-9_10
Martin, M. (2011). Cutadapt removes adapter sequences from high-throughput sequencing reads. EMBnet J. 17, 10–12. doi: 10.14806/ej.17.1.200
Masaki, M., Ikeda, A., Shiraki, E., Oka, S., and Kawasaki, T. (2003). Mixed lineage kinase LZK and antioxidant protein-1 activate NF-kappaB synergistically. Eur. J. Biochem. 270, 76–83. doi: 10.1046/j.1432-1033.2003.03363.x
Menard, K. L., Haskins, B. E., Colombo, A. P., and Denkers, E. Y. (2018). Toxoplasma gondii manipulates expression of host long Noncoding RNA during intracellular infection. Sci. Rep. 8:15017. doi: 10.1038/s41598-018-33274-5
Menard, K. L., Haskins, B. E., and Denkers, E. Y. (2019). Impact of Toxoplasma gondii infection on host non-coding RNA responses. Front. Cell Infect. Microbiol. 9:132. doi: 10.3389/fcimb.2019.00132
Mercer, T. R., Dinger, M. E., and Mattick, J. S. (2009). Long non-coding RNAs: insights into functions. Nat. Rev. Genet. 10, 155–159. doi: 10.1038/nrg2521
Mills, E. L., Kelly, B., and O’neill, L. A. J. (2017). Mitochondria are the powerhouses of immunity. Nat. Immunol. 18, 488–498. doi: 10.1038/ni.3704
Mohanty, A., Tiwari-Pandey, R., and Pandey, N. R. (2019). Mitochondria: the indispensable players in innate immunity and guardians of the inflammatory response. J. Cell Commun. Signal. 13, 303–318. doi: 10.1007/s12079-019-00507-9
Mohr, I., and Sonenberg, N. (2012). Host translation at the nexus of infection and immunity. Cell Host Microb. 12, 470–483. doi: 10.1016/j.chom.2012.09.006
Moran, I., Akerman, I., Van De Bunt, M., Xie, R., Benazra, M., Nammo, T., et al. (2012). Human beta cell transcriptome analysis uncovers lncRNAs that are tissue-specific, dynamically regulated, and abnormally expressed in type 2 diabetes. Cell Metab. 16, 435–448. doi: 10.1016/j.cmet.2012.08.010
Na, B. R., and Jun, C. D. (2015). TAGLN2-mediated actin stabilization at the immunological synapse: implication for Cytotoxic T cell control of target cells. BMB Rep. 48, 369–370. doi: 10.5483/bmbrep.2015.48.7.132
Nishina, H., Wada, T., and Katada, T. (2004). Physiological roles of SAPK/JNK signaling pathway. J. Biochem. 136, 123–126. doi: 10.1093/jb/mvh117
Nolte, W., Weikard, R., Brunner, R. M., Albrecht, E., Hammon, H. M., Reverter, A., et al. (2019). Biological network approach for the identification of regulatory long Non-Coding RNAs associated with metabolic efficiency in cattle. Front. Genet. 10:1130. doi: 10.3389/fgene.2019.01130
Pang, K. C., Dinger, M. E., Mercer, T. R., Malquori, L., Grimmond, S. M., Chen, W., et al. (2009). Genome-wide identification of long noncoding RNAs in CD8+ T cells. J. Immunol. 182, 7738–7748. doi: 10.4049/jimmunol.0900603
Pertea, G., and Pertea, M. (2020). GFF Utilities: GffRead and GffCompare. F1000Research 9:304. doi: 10.12688/f1000research.23297.2
Pertea, M., Pertea, G. M., Antonescu, C. M., Chang, T. C., Mendell, J. T., and Salzberg, S. L. (2015). StringTie enables improved reconstruction of a transcriptome from RNA-seq reads. Nat. Biotechnol. 33, 290–295. doi: 10.1038/nbt.3122
Petri, A., Dybkaer, K., Bogsted, M., Thrue, C. A., Hagedorn, P. H., Schmitz, A., et al. (2015). Long Noncoding RNA expression during human B-Cell development. PLoS One 10:e0138236. doi: 10.1371/journal.pone.0138236
Ponting, C. P., Oliver, P. L., and Reik, W. (2009). Evolution and functions of long noncoding RNAs. Cell 136, 629–641. doi: 10.1016/j.cell.2009.02.006
Qureshi, I. A., Mattick, J. S., and Mehler, M. F. (2010). Long non-coding RNAs in nervous system function and disease. Brain Res. 1338, 20–35. doi: 10.1016/j.brainres.2010.03.110
Riquelme, I., Ili, C., Roa, J. C., and Brebi, P. (2016). Long non-coding RNAs in gastric cancer: mechanisms and potential applications. Oncotarget 5:48.
Robinson, A. (2015). qualitytrim [Online]. Available online at: https://bitbucket.org/arobinson/qualitytrim (accessed May 28, 2021).
Rochet, E., Appukuttan, B., Ma, Y., Ashander, L. M., and Smith, J. R. (2019). Expression of Long Non-coding RNAs by human retinal muller glial cells infected with clonal and exotic virulent Toxoplasma gondii. Noncoding RNA 5:48. doi: 10.3390/ncrna5040048
Roeber, F., Jex, A. R., and Gasser, R. B. (2013). Impact of gastrointestinal parasitic nematodes of sheep, and the role of advanced molecular tools for exploring epidemiology and drug resistance - an Australian perspective. Parasit Vect. 6:153. doi: 10.1186/1756-3305-6-153
Ruano, Z. M., Cortinhas, A., Carolino, N., Gomes, J., Costa, M., and Mateus, T. L. (2019). Gastrointestinal parasites as a possible threat to an endangered autochthonous portuguese sheep breed. J. Helminthol. 94:e103. doi: 10.1017/S0022149X19000968
Salavati, M., Caulton, A., Clark, R., Gazova, I., Smith, T. P. L., Worley, K. C., et al. (2020). Global analysis of transcription start sites in the new ovine reference genome (oar rambouillet v1.0). Front. Genet. 11:580580. doi: 10.3389/fgene.2020.580580
Schmitz, S. U., Grote, P., and Herrmann, B. G. (2016). Mechanisms of long noncoding RNA function in development and disease. Cell Mol. Life Sci. 73, 2491–2509. doi: 10.1007/s00018-016-2174-5
Seila, A. C., Calabrese, J. M., Levine, S. S., Yeo, G. W., Rahl, P. B., Flynn, R. A., et al. (2008). Divergent transcription from active promoters. Science 322, 1849–1851. doi: 10.1126/science.1162253
Siddiqui, W. A., Ahad, A., and Ahsan, H. (2015). The mystery of BCL2 family: Bcl-2 proteins and apoptosis: an update. Arch. Toxicol. 89, 289–317. doi: 10.1007/s00204-014-1448-7
Smith, W. J., Li, Y., Ingham, A., Collis, E., Mcwilliam, S. M., Dixon, T. J., et al. (2010). A genomics-informed, SNP association study reveals FBLN1 and FABP4 as contributing to resistance to fleece rot in Australian Merino sheep. BMC Vet. Res. 6:27. doi: 10.1186/1746-6148-6-27
Srijyothi, L., Ponne, S., Prathama, T., Cheemala, A., and Baluchamy, S. (2018). Roles of Non-Coding RNAs in Transcriptional Regulation. London, UK: IntechOpen. 50.
Stecca, B., and Rovida, E. (2019). Impact of ERK5 on the hallmarks of cancer. Int. J. Mol. Sci. 20:1426. doi: 10.3390/ijms20061426
Stradal, T. E. B., and Schelhaas, M. (2018). Actin dynamics in host-pathogen interaction. FEBS Lett. 592, 3658–3669. doi: 10.1002/1873-3468.13173
Sun, L., Luo, H., Bu, D., Zhao, G., Yu, K., Zhang, C., et al. (2013). Utilizing sequence intrinsic composition to classify protein-coding and long non-coding transcripts. Nucleic Acids Res. 41:e166. doi: 10.1093/nar/gkt646
Taft, R. J., Kaplan, C. D., Simons, C., and Mattick, J. S. (2009). Evolution, biogenesis and function of promoter-associated RNAs. Cell Cycle 8, 2332–2338. doi: 10.4161/cc.8.15.9154
Tan-Wong, S. M., Dhir, S., and Proudfoot, N. J. (2019). R-Loops promote antisense transcription across the mammalian genome. Mol. Cell 76, 600–616.e606. doi: 10.1016/j.molcel.2019.10.002
Thiel, D., Conrad, N. D., Ntini, E., Peschutter, R. X., Siebert, H., and Marsico, A. (2019). Identifying lncRNA-mediated regulatory modules via ChIA-PET network analysis. BMC Bioinform. 20:292. doi: 10.1186/s12859-019-2900-8
Thorvaldsdottir, H., Robinson, J. T., and Mesirov, J. P. (2013). Integrative Genomics Viewer (IGV): high-performance genomics data visualization and exploration. Brief Bioinform. 14, 178–192. doi: 10.1093/bib/bbs017
Thumser, A. E., Moore, J. B., and Plant, N. J. (2014). Fatty acid binding proteins: tissue-specific functions in health and disease. Curr. Opin. Clin. Nutr. Metab. Care 17, 124–129. doi: 10.1097/MCO.0000000000000031
Ulitsky, I., and Bartel, D. P. (2013). lincRNAs: genomics, evolution, and mechanisms. Cell 154, 26–46. doi: 10.1016/j.cell.2013.06.020
Villegas, V. E., and Zaphiropoulos, P. G. (2015). Neighboring gene regulation by antisense long non-coding RNAs. Int. J. Mol. Sci. 16, 3251–3266. doi: 10.3390/ijms16023251
Wang, J., Chai, Z., Deng, L., Wang, J., Wang, H., Tang, Y., et al. (2020). Detection and integrated analysis of lncRNA and mRNA relevant to plateau adaptation of Yak. Reprod. Domest. Anim. 55, 1461–1469. doi: 10.1111/rda.13767
Wapinski, O., and Chang, H. Y. (2011). Long noncoding RNAs and human disease. Trends Cell Biol. 21, 354–361. doi: 10.1016/j.tcb.2011.04.001
Weikard, R., Demasius, W., and Kuehn, C. (2017). Mining long noncoding RNA in livestock. Anim. Genet. 48, 3–18. doi: 10.1111/age.12493
Weikard, R., Hadlich, F., Hammon, H. M., Frieten, D., Gerbert, C., Koch, C., et al. (2018). Long noncoding RNAs are associated with metabolic and cellular processes in the jejunum mucosa of pre-weaning calves in response to different diets. Oncotarget 9, 21052–21069. doi: 10.18632/oncotarget.24898
Wilusz, J. E., Sunwoo, H., and Spector, D. L. (2009). Long noncoding RNAs: functional surprises from the RNA world. Genes Dev. 23, 1494–1504. doi: 10.1101/gad.1800909
Wolfe, C. J., Kohane, I. S., and Butte, A. J. (2005). Systematic survey reveals general applicability of “guilt-by-association” within gene coexpression networks. BMC Bioinform. 6:227. doi: 10.1186/1471-2105-6-227
Wucher, V., Legeai, F., Hedan, B., Rizk, G., Lagoutte, L., Leeb, T., et al. (2017). FEELnc: a tool for long non-coding RNA annotation and its application to the dog transcriptome. Nucleic Acids Res. 45:e57. doi: 10.1093/nar/gkw1306
Youle, R. J., and Strasser, A. (2008). The BCL-2 protein family: opposing activities that mediate cell death. Nat. Rev. Mol. Cell Biol. 9, 47–59. doi: 10.1038/nrm2308
Zhang, R., Liu, F., Hunt, P., Li, C., Zhang, L., Ingham, A., et al. (2019). Transcriptome analysis unraveled potential mechanisms of resistance to Haemonchus contortus infection in Merino sheep populations bred for parasite resistance. Vet. Res. 50:7. doi: 10.1186/s13567-019-0622-6
Zhang, T., Zhang, X., Han, K., Zhang, G., Wang, J., Xie, K., et al. (2017). Analysis of long noncoding RNA and mRNA using RNA sequencing during the differentiation of intramuscular preadipocytes in chicken. PLoS One 12:e0172389. doi: 10.1371/journal.pone.0172389
Zhao, X. Y., and Lin, J. D. (2015). Long Noncoding RNAs: a new regulatory code in metabolic control. Trends Biochem. Sci. 40, 586–596. doi: 10.1016/j.tibs.2015.08.002
Zhao, Y., Li, H., Fang, S., Kang, Y., Wu, W., Hao, Y., et al. (2016). NONCODE 2016: an informative and valuable data source of long non-coding RNAs. Nucleic Acids Res. 44, D203–D208. doi: 10.1093/nar/gkv1252
Zheng, K., Kitazato, K., Wang, Y., and He, Z. (2016). Pathogenic microbes manipulate cofilin activity to subvert actin cytoskeleton. Crit. Rev. Microbiol. 42, 677–695. doi: 10.3109/1040841X.2015.1010139
Keywords: adult sheep, gastrointestinal infection, nematode, abomasal lymph node, long non-coding RNA, functional annotation, gene co-expression, pathways
Citation: Chitneedi PK, Weikard R, Arranz JJ, Martínez-Valladares M, Kuehn C and Gutiérrez-Gil B (2021) Identification of Regulatory Functions of LncRNAs Associated With T. circumcincta Infection in Adult Sheep. Front. Genet. 12:685341. doi: 10.3389/fgene.2021.685341
Received: 25 March 2021; Accepted: 20 May 2021;
Published: 14 June 2021.
Edited by:
Aline Silva Mello Cesar, University of São Paulo, BrazilReviewed by:
Rodrigo Baptista, University of Georgia, United StatesRobert W. Li, United States Department of Agriculture, United States
Copyright © 2021 Chitneedi, Weikard, Arranz, Martínez-Valladares, Kuehn and Gutiérrez-Gil. This is an open-access article distributed under the terms of the Creative Commons Attribution License (CC BY). The use, distribution or reproduction in other forums is permitted, provided the original author(s) and the copyright owner(s) are credited and that the original publication in this journal is cited, in accordance with accepted academic practice. No use, distribution or reproduction is permitted which does not comply with these terms.
*Correspondence: Beatriz Gutiérrez-Gil, beatriz.gutierrez@unileon.es