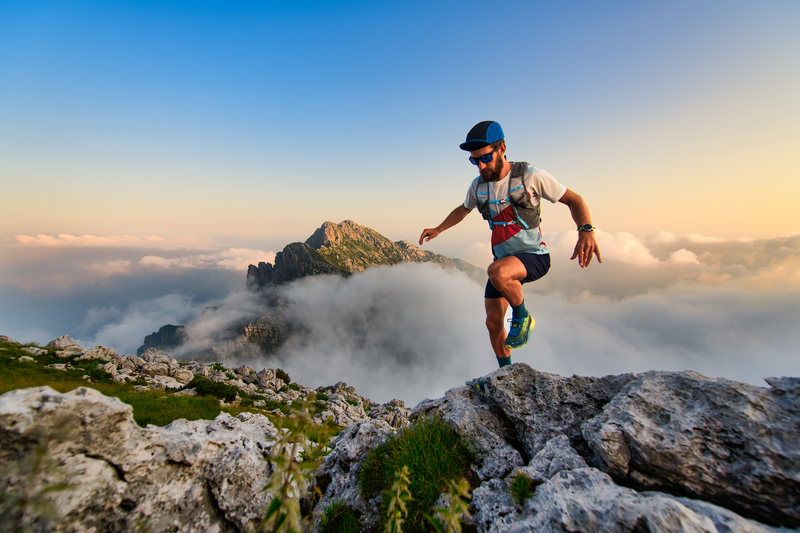
95% of researchers rate our articles as excellent or good
Learn more about the work of our research integrity team to safeguard the quality of each article we publish.
Find out more
REVIEW article
Front. Genet. , 12 August 2021
Sec. Statistical Genetics and Methodology
Volume 12 - 2021 | https://doi.org/10.3389/fgene.2021.683082
This article is part of the Research Topic Mendelian Randomization: Approach and Applications View all 15 articles
Stroke is a leading cause of death and disability worldwide. However, our understanding of its underlying biology and the number of available treatment options remain limited. Mendelian randomization (MR) offers a powerful approach to identify novel biological pathways and therapeutic targets for this disease. Around ~100 MR studies have been conducted so far to explore, confirm, and quantify causal relationships between several exposures and risk of stroke. In this review, we summarize the current evidence arising from these studies, including those investigating ischemic stroke, hemorrhagic stroke, or both. We highlight the different types of exposures that are currently under study, ranging from well-known cardiovascular risk factors to less established inflammation-related mechanisms. Finally, we provide an overview of future avenues of research and novel approaches, including drug target validation MR, which is poised to have a substantial impact on drug development and drug repurposing.
Stroke is a leading cause of mortality and disability worldwide. While the overall incidence of stroke is decreasing, the global burden of this disease remains high because the absolute number of disability-adjusted life years lost due to stroke is increasing as the population grows and ages (Johnson et al., 2019). Thus, there is an urgent need for novel preventive, therapeutic, and rehabilitation strategies. Among these three, new acute treatments are particularly needed, as the few alternatives available thus far include thrombolytic therapy and mechanical thrombectomy for ischemic stroke (Powers et al., 2019), targeted blood pressure reduction for intracerebral hemorrhage (ICH; Hemphill et al., 2015), and early securing of the aneurysm in subarachnoid hemorrhage (Connolly et al., 2012).
In this setting, the field of stroke research can greatly benefit from the tools that population genetics has to offer. Mendelian randomization (MR) is a statistical method aimed at determining and quantifying causal relationships between genetically determined exposures and outcomes of interest (Davies et al., 2018). Importantly, in contrast to randomized clinical trials (the most frequently used tool to evaluate causality), MR can be performed using already available open-access data from different sources, allowing the evaluation of larger numbers of possible mechanisms and accelerating the speed of the translational cycle.
In this review, we introduced the concept of MR and provide an overview of the existing MR studies focused on stroke. To identify these studies, we conducted a systematic search of the medical literature using PubMed and the keywords “Mendelian randomization stroke,” “Mendelian randomization intracerebral hemorrhage,” and “Mendelian randomization subarachnoid hemorrhage” in the MEDLINE database. We also explored some analytical possibilities beyond classical MR, including the use of multiomics data and drug target validation MR.
MR constitutes a special case of instrumental variable analysis, a widely used analytical framework for causal inference. When the MR assumptions are met, it is possible to identify and quantify causal relationships between exposures and outcomes of interest (Figure 1). MR is best explained using an example. For this section, we will use the link between cholesterol levels and systolic blood pressure as our starting point. In randomized clinical trials, investigators randomly assign study participants to an intervention or placebo to study causal relationships between the intervention and the outcome of interest. In our example, researchers would randomly assign participants to receive a cholesterol-lowering drug or placebo and then measure systolic blood pressure levels in both groups. In MR analysis, we would use genetic variants strongly associated with the exposure of interest as the instrument. In this case, we would choose genetic variants strongly associated with cholesterol levels. As these variants are randomly segregated before birth, one could then separate groups according to the number of risk alleles, and the resulting analyses would not be confounded by environmental exposures happening after birth. Thus, we can measure systolic blood pressure levels in those groups and implement the necessary comparisons.
Figure 1. Mendelian randomization (MR) as instrument variable analysis. MR is a type of instrumental variable analysis, similar to randomized clinical trials. Exposed individuals in MR are those carrying risk alleles for determined genetic variants known to associate with an exposure of interest.
The implementation of MR analyses requires important assumptions (Figure 2). First, the genetic variants used as instruments must be strongly associated with the exposure or risk factor of interest. This is generally easily accomplished, since these variants are often derived from large-scale genome-wide association studies (GWAS) of the exposure of interest. Second, there should be no confounders affecting the association between genetic variants and the outcome of interest. This assumption is not trivial, and, at the moment, there is no method to definitively confirm this assumption. Although not always performed, a practical way to tackle this problem is to test the genetic variants used as instruments against an array of different other potential covariates that could lead to bias. Third, the genetic variants used as instruments affect the outcome of interest only via the exposure of interest, with no other alternative pathways coming into play. These alternative pathways are part of what is called horizontal pleiotropy. There are several statistical methods to test and correct for horizontal pleiotropy, including the Mendelian randomization–Egger (MR–Egger) intercept, MR Pleiotropy RESidual Sum and Outlier (MR-PRESSO), and Causal Analysis Using Summary Effect Estimates (CAUSE; Bowden et al., 2015; Verbanck et al., 2018; Morrison et al., 2020).
Figure 2. Mendelian randomization paradigm and assumptions. Mendelian randomization assumptions: (1) valid instrument, (2) no association with confounders, and (3) no horizontal pleiotropy.
Crucial to MR studies is the online availability of open-access resources, including tools to rapidly test hypotheses such as MR-Base (Hemani et al., 2018); large biobanks such as the UK Biobank (Bycroft et al., 2018), the China Kadoorie Biobank (CKB; Chen et al., 2011), Biobank Japan (Nagai et al., 2017), and the All of Us Research Program (Denny et al., 2019); and repositories of summary statistics from large GWAS like the GWAS catalog (Buniello et al., 2019), the GWAS atlas (Tian et al., 2020), and specifically for stroke, the Cerebrovascular Disease Knowledge Portal (Crawford et al., 2018). These and other helpful resources are summarized in Table 1.
Cardiovascular risk factors represent the most important determinants of risk of stroke. While several observational studies have shown associations between traditional vascular risk factors and risk of different types of stroke, deriving causality from observational data is problematic due to the possibility of bias. In the last 5 years, several MR studies have been conducted to explore, confirm, and quantify causality behind these already described associations (Table 2).
Robust existing evidence indicates that high blood pressure is one of the main risk factors for stroke. However, several questions remain, including how early and sustained changes in blood pressure affect outcomes later in life and whether specific pharmacological interventions provide additional benefits over others. Georgakis et al. (2020b) utilized MR analyses to assess the relationship between genetically proxied blood pressure levels and the risk of any stroke as well as its subtypes. They demonstrated that blood pressure levels were causally associated with the risk of any stroke and most subtypes, with the exception of lobar ICH. The effect of blood pressure appeared stronger in large artery stroke (LAS) and small vessel stroke (SVS). Additionally, the authors explored genetic proxies for two different antihypertensive drugs classes, calcium channel blockers and beta-blockers, finding that a 10 mmHg reduction in systolic blood pressure using genetic proxies for calcium channel blockers was protective for any stroke and its subtypes, especially for SVS (40% reduction). Furthermore, the same reduction in blood pressure through calcium channel blockers variants was also associated with lower white matter hyperintensity (WMH) volumes, a well-established neuroimaging biomarker for cerebral small vessel disease. A study from the same group also evaluated whether blood pressure pulsatility (i.e., pulse pressure) affects stroke risk independently of the mean arterial pressure. Using multivariable MR within the UK Biobank, they found that pulse pressure was independently associated with ischemic stroke risk (but not ICH) in participants older than 55 years, being particularly important for LAS (Georgakis et al., 2020a). Beyond ischemic stroke, MR analyses have confirmed observational evidence that indicate that high blood pressure is associated with higher risks of intracranial aneurysms and subarachnoid hemorrhage (Bakker et al., 2020).
The role of lipids in cardiovascular disease (CVD) has been extensively studied. However, the effect of circulating lipid levels on stroke appear to be heterogeneous and depend on the specific subtypes being evaluated. Hindy et al. (2018) investigated the effect of blood lipids on the risk of ischemic stroke and its subtypes using summary statistics from the GWAS completed by the Stroke Genetics Network (SiGN), which included 17,000 ischemic stroke cases and more than 32,000 controls of European ancestry. They found that low-density lipoprotein cholesterol (LDL-C) levels were associated with risk of ischemic stroke, especially LAS. HDL-C levels were inversely associated with the risk of SVS. There were no consistent associations for triglycerides. More recently, Allara et al. (2019) conducted a two-sample MR study to identify relationships between lipid levels and the risk of several cardiovascular outcomes, including stroke. In their study, higher HDL-C levels were associated with lower risk of ICH, while higher LDL-C levels were associated with higher risk of ischemic cerebrovascular disease. Interestingly, they also found an inverse association between triglycerides and the risk of ICH. A rigorous study performed by Valdes-Marquez et al. (2019) investigated the effect of LDL-C on the risk of ischemic stroke and its subtypes and did not find any significant associations with these conditions. Georgakis et al. (2020c) also looked at the association between lipid fractions and small vessel disease, a broad phenotype that includes SVS, white matter disease, and ICH. They found that higher HDL-C was associated with lower risk of SVS and lower WMH volume. Interestingly, they also found a relationship between higher HDL-C and higher risk of ICH. Along this lines, Sun et al. (2019) found a strong positive association between LDL-C and ischemic stroke and a strong inverse association with ICH in participants of the China Kadoorie Biobank. This inverse association between genetically determined LDL-C levels and the risk of ICH was confirmed in cohorts of European descent by Falcone et al. (2020). The latter two studies confirmed previous observational results that suggested that very low LDL-C levels increase the risk of ICH (Wang et al., 2013; Saliba et al., 2018; Ma et al., 2019), pointing to novel pathways in ICH.
Another study recently explored association pertains to lipoprotein(a) [Lp(a)] levels and ischemic stroke. Using summary statistics from MEGASTROKE and the International Genomics of Alzheimer’s Project (IGAP), Pan et al. (2019) found a causal positive association between Lp(a) levels and LAS and, remarkably, an inverse association with SVS and Alzheimer disease (AD). Using individual-level data from the UK Biobank, Larsson et al. (2020b) confirmed a positive association with any ischemic stroke but could not replicate the protective association with AD. The latter result, however, could have been due to lack of statistical power, as the investigators did find a protective association with parental AD or dementia.
Extensive data point to a deleterious effect of diabetes on ischemic stroke, but there is no such evidence for hemorrhagic stroke. Larsson et al. (2017) used MR analyses to confirm a causal relationship between type 2 diabetes mellitus (T2DM) and risk of ischemic stroke, especially LAS, but found null associations between other metabolic markers such as glucose, insulin levels, and body mass index (BMI). Gan et al. (2019) replicated this causal association between T2DM and ischemic stroke in the CKB, and Liu et al. (2018) found an association between T2DM and lacunar stroke. Similarly, using a combined exposure comprising phenotypes related to insulin resistance (fasting insulin adjusted for BMI, HLD-C and triglycerides, and insulin sensitivity), Chen et al. (2020) found a causal relationship between insulin resistance and ischemic stroke, particularly small vessel stroke. On the other hand, Yeung et al. (2018) did not find an association between glycated hemoglobin (HbA1c) and ischemic stroke in an MR study within the UK Biobank. More recently, Georgakis et al. (2021) investigated the effects of T2DM, hyperglycemia, insulin resistance, and β-cell dysfunction on the risk of stroke and related traits, finding that type 2 diabetes and higher HbA1c levels are associated with higher risk stroke, and particularly of LAS and SVS, with similar associations found for insulin resistance and β-cell dysfunction. β-Cell dysfunction was also associated with the risk of ICH. Furthermore, a study focused on neuroimaging markers of cerebrovascular disease found that type 2 diabetes was also positively associated with fractional anisotropy (a measure of white matter integrity) and inversely associated with gray matter volume and total brain volume, with similar inverse associations seen for HbA1c and β-cell dysfunction.
A large body of literature indicate that smoking is associated with both ischemic stroke and aneurysmal subarachnoid hemorrhage (Peters et al., 2013). Qian et al. (2019) reported a causal association between smoking and any ischemic stroke and LAS. In other MR studies, Larsson et al. (2019a, 2020c) confirmed the association between smoking initiation and the risk of any ischemic stroke, LAS and SVS, but did not find an association with cardioembolic stroke (CES) or ICH. In a one-sample MR study using the UK Biobank, Acosta et al. (2021) confirmed an association between genetic propensity to smoke and risk of nontraumatic subarachnoid hemorrhage. Similarly, in a large GWAS of intracranial aneurysms and subarachnoid hemorrhage, smoking was shown to be a powerful risk factor for this disease (Bakker et al., 2020).
Observational evidence suggests that obesity is a risk factor for stroke. However, MR studies have yielded heterogeneous results. One study indicated that while both general and central adiposity had causal effects on coronary heart disease, only central adiposity appeared to be associated with ischemic stroke (Dale et al., 2017). Further evidence supporting this hypothesis was provided by a more recent MR study by Marini et al. (2020) which found that higher waist-to-hip ratio, but not higher BMI, was causally associated with all-cause ischemic stroke, LAS, SVS, non-lobar ICH, and WMH volume.
Physical activity, especially moderate-to-vigorous physical activity, has been associated with lower risk of stroke in several observational studies. To date, only one MR has investigated this relationship, finding null results for this association (Zhuang et al., 2020). Therefore, further research is warranted in order to fully understand these conflicting results.
Atrial fibrillation (AF) is considered a major risk factor of cardioembolic ischemic stroke. Remarkably, patients with stroke are also at a higher risk of developing AF (Sposato et al., 2015, 2018). Only one MR study has looked at this relationship and, as expected given the overwhelming amount of observational evidence, found causal evidence to support this link using bidirectional MR (Hou et al., 2020).
A number of studies have explored nontraditional risk factors that had mixed or inconclusive evidence when evaluated in nongenetic, observational studies. Prominent among these are studies that focused on inflammation, coagulation factors, sleep health, and nutrition. MR studies have evaluated several of these factors in search of causal associations (Table 3).
Observational studies have demonstrated that patients with underlying inflammation have a higher risk of stroke (Anrather and Iadecola, 2016), although this association could be the result of confounding. Therefore, genetically determined inflammatory biomarkers have been targeted by many studies investigating the risk of stroke. Zhang et al. (2020) examined whether genetically raised plasma C-reactive protein (CRP) concentration levels were associated with ischemic stroke without finding a significant association. In line with these findings, Lin et al. (2020) evaluated numerous inflammatory biomarkers and found no association between genetically elevated levels of these biomarkers and ischemic stroke. Yuan et al. (2020b) analyzed genetically determined circulating interleukins in relation to coronary artery disease (CAD), atrial fibrillation, and ischemic stroke, and its subtypes. There was a suggestive (p < 0.05 but not statistically significant after correction for multiple testing) positive association between interleukin-1 receptor antagonist and cardioembolic stroke and a suggestive inverse association between interleukin-6 and ischemic stroke, CES, and SVS, and of interleukin-16 with CAD. Georgakis et al. (2020d) reported the results of an MR study focused on interleukin-6 signaling effects on ischemic stroke and other cardiovascular outcomes and demonstrated that genetically downregulated interleukin-6 signaling was associated with lower risk of ischemic stroke. The same group published a study investigating genetically determined levels of circulating cytokines and risk of stroke (Georgakis et al., 2019). They showed that genetic predisposition to elevated circulating levels of monocyte chemoattractant protein-1 was associated with higher risk of stroke, in particular with LAS and CES. Another study by Yuan et al. (2020a) investigated causal associations of increased tumor necrosis factor levels and several highly prevalent CVDs. Genetically elevated tumor necrosis factor levels were positively associated with both CAD and ischemic stroke. In addition, Wang et al. (2020) investigated the impact of growth differentiation factor 15 on the risk of CVDs using an MR approach. They found evidence for a relationship between circulating of growth differentiation factor 15 levels and increased risk of CES, atrial fibrillation, CAD, and myocardial infarction. Finally, Song et al. (2020) published results of their study investigating association of genetically determined T-cell immunoglobulin and mucin domain 1 with incidence of stroke, which found a causal effect of TIM-1 on any stroke and ischemic stroke.
The role of hematological traits and pathways in the occurrence of stroke has also been extensively evaluated using MR. Gill et al. (2018b) investigated genetically determined platelet count and risk of different CVDs, finding that higher genetically determined platelet count is associated with higher risk of ischemic stroke. The same group released results of an MR study focused on genetically determined factor XI levels that found causal evidence supporting factor XI as a possible target to reduce the risk of cardioembolic stroke (Gill et al., 2018a). Harshfield et al. (2020) reported a study that, rather than focusing on a single target, evaluated several different hematological traits in connection to risk of ischemic stroke and its subtypes. Several factors in the intrinsic coagulation pathway were significantly associated with CES and LAS but not with SVS. Specifically, gamma fibrinogen, a component of the common pathway, was associated with CES, plateletcrit was associated with CES, eosinophil percentage of white cells was associated with LAS, and thrombin-activatable fibrinolysis inhibitor activation peptide antigen was associated with any ischemic stroke. Follow-up analyses in the UK Biobank showed that among individuals with atrial fibrillation, those with genetically lower versus normal levels of factor XI have a reduced risk of ischemic stroke. Finally, one group investigated circulating vitamin K1 levels in connection to cerebrovascular disease and found that genetic predisposition to higher circulating vitamin K₁ levels was associated with an increased risk of LAS (Larsson et al., 2018a).
A number of MR studies also investigated the role of nutritional factors in the occurrence of stroke, another research avenue extensively explored from an observational perspective. Larsson et al. (2020a) examined whether genetically determined predisposition to alcohol consumption had influence on risk of CVD. This study provided evidence for a causal relationship between higher alcohol consumption and increased risk of any stroke and peripheral artery disease.
Beyond alcohol, several other nutritional factors have been investigated. One research group examined the role of plasma phospholipid fatty acids in risk of 15 CVD-related phenotypes. Genetically higher plasma α-linolenic, linoleic, and oleic acid levels were inversely associated with LAS and venous thromboembolism, whereas arachidonic and stearic acid levels are positively associated with these two CVD-related outcomess (Yuan et al., 2019).
Iron metabolism, including anemia and polycythemia, have long been postulated to play a role in cerebrovascular disease. Gill et al. (2018c) published results of an MR study investigating this question, finding that higher iron levels were associated with increased risk of stroke and, in particular, CES.
Two groups reported on genetically predicted levels of vitamin D and the risk of stroke. Huang et al. investigated whether vitamin D played a role in risk and mortality of several vascular diseases by conducting an MR study that included both Asian and European participants (Huang et al., 2019). They found no evidence to support that genetically increased vitamin D was associated with a lower risk of ischemic stroke, ICH, SAH, and lipid levels in neither Chinese nor European. Similarly, Larsson et al. (2018b) reported the results of a study on genetically determined vitamin D concentrations and ischemic stroke and its subtypes that failed to find significant associations.
Among other investigated risk factors, it is worth mentioning two studies investigating endocrine changes. Schooling et al. (2018) analyzed genetic predictors of testosterone and their associations with different CVD phenotypes. They confirmed prior results from observational studies showing a significant association between genetically proxied testosterone and risk of ischemic stroke. Another study by Marouli et al. (2020) used MR analyses to analyze whether thyroid function affects the risk of stroke via atrial fibrillation, finding that a 1 SD increase in TSH was associated with a 5% decrease in the risk of ischemic stroke.
Finally, another four studies are also worth mentioning. Larsson et al. (2019b) examined serum magnesium and calcium levels in relation to ischemic stroke using MR. They found that genetically higher serum magnesium concentrations were associated with a reduced risk of CES but not with other stroke subtypes. Au Yeung and Schooling (2020) investigated the impact of urinary sodium on CVD. Higher genetically determined log-transformed urinary sodium was associated with higher risk of stroke. Choi et al. (2020) used MR tools to describe a causal association between serum bilirubin levels and decreased stroke risk. Lastly, Jia et al. (2019) investigated the role of gut microbiota-dependent metabolites and risk of several CVDs, finding no evidence of a causal link between these metabolites (betaine, carnitine, choline, and trimethylamine N-oxide) and the risk of stroke.
Beyond hypothesis-driven MR studies, population genetics offers powerful tools to accelerate the discovery of novel biological pathways by agnostically evaluating several biological targets and/or reevaluating targets for drug repurposing (Nelson et al., 2015; Schmidt et al., 2020). This approach is significantly potentiated by the growing culture of open-access research and the increasing availability of high-throughput genomic and proteomic technologies.
Drug target MR studies use genetic variants that lie within or near the genes coding for these targets (the latter called cis-variants) as instruments, which either have an effect on the actual serum levels of the target protein or other endpoint, such as an intermediate biomarker, gene expression levels, or metabolite levels. This distinction is important because drug target MR aims to answer a different question than conventional MR. While conventional MR establishes causal relationships between biomarkers or traits and an outcome, drug target MR addresses whether modifications of a specific drug target or protein will have an effect on the outcome (Gill et al., 2021).
Drug target MR overcomes problems related to pleiotropy, which are especially relevant when looking at possible targets for intervention. In addition, by testing these drug targets phenome-wide, investigators can also pinpoint possible adverse effects. While drug target MR is a robust methodology, it also has limitations. While new high-throughput technologies have increased the amount of proteome-wide data available, publicly available summary statistics and proteomic studies with adequate sample sizes are still limited. This relative paucity of proteomic data leads to the utilization of cis-variants associated with a biomarker in the causal pathway, which could in turn lead to some problems. For example, circulating levels of a biomarker could not represent accurately its cellular concentration, which is often the value of interest, and this could limit the ability to detect causality. Additionally, the biomarker of interest could be relevant only in certain physiological or disease states, or during a critical period of time, which could also limit results. Lastly, not all proteins are druggable (i.e., able to be pharmacologically manipulated), which of course would defeat the purpose of doing drug target MR (Mokry et al., 2015).
Gill et al. (2019a) compared the results of drug target MR and clinical trials for three antihypertensive drug classes (angiotensin-converting-enzyme inhibitors, β-blockers, and calcium channel blockers) in coronary heart disease and stroke, finding comparable estimates. Along these lines, Chong et al. (2019) conducted a proteome-wide MR study to investigate potential therapeutic targets in ischemic stroke. The authors analyzed 653 circulating proteins as possible causal factors for the three main subtypes of ischemic strokes (LAS, SVS, and CES) and hemorrhagic stroke. In their analyses, they found eight biomarker–stroke associations encompassing seven unique targets. Of these biomarkers, five had already been associated with CVDs, including the coagulation factor 11, Lp(a), ABO, CD40 (a member of the tumor necrosis factor superfamily), and MMP12 (a member of the matrix metalloproteinase family, implicated in vascular remodeling). Novel biomarkers included SCARA5, a protein with a role in iron homeostasis, and TNFS12, a pleiotropic tumor necrosis factor-like cytokine linked to atrial fibrillation, a potential mediating mechanism.
Drug repurposing is another potentially useful approach, as previously approved drugs can be more easily brought to clinical practice if a beneficial effect is found. There are no clear examples of MR studies specifically focused on drug repurposing for stroke. However, we bring the reader’s attention to two such studies that explored the potential of repurposing medications to treat Alzheimer’s disease, unfortunately with null results (Walker et al., 2020; Wu et al., 2021). These studies provide an appropriate example and analytical framework for future studies applying this approach to stroke and cerebrovascular diseases.
Stroke constitutes an increasingly prevalent condition worldwide and remains one of the leading causes of death and disability. MR has proved to be a powerful methodology to confirm or refute associations described by observational studies and identify novel therapeutic targets for stroke. The ability of MR studies to add valuable scientific evidence to the field of cerebrovascular disease research will be greatly increased by the utilization of novel tools, including proteome-wide and drug target validation analyses.
JA, NS, and GF reviewed the literature, drafted the manuscript, and revised the manuscript for intellectual content. All authors contributed to the article and approved the submitted version.
GF was supported by the National Institute on Aging (K76AG59992), the National Institute of Neurological Disorders and Stroke (R03NS112859), the American Heart Association (18IDDG34280056 and 817874), the Yale Pepper Scholar Award (P30AG021342), and the Neurocritical Care Society Research Fellowship. JNA is supported by the American Heart Association’s Bugher Fellowship in Hemorrhagic Stroke Research.
The authors declare that the research was conducted in the absence of any commercial or financial relationships that could be construed as a potential conflict of interest.
All claims expressed in this article are solely those of the authors and do not necessarily represent those of their affiliated organizations, or those of the publisher, the editors and the reviewers. Any product that may be evaluated in this article, or claim that may be made by its manufacturer, is not guaranteed or endorsed by the publisher.
Acosta, J. N., Szejko, N., Both, C. P., Vanent, K., and Noche, R. B. (2021). Genetically determined smoking behavior and nontraumatic subarachnoid hemorrhage. Stroke 52, 582–587. doi: 10.1161/STROKEAHA.120.031622
Allara, E., Morani, G., Carter, P., Gkatzionis, A., Zuber, V., Foley, C. N., et al. (2019). Genetic determinants of lipids and cardiovascular disease outcomes: a wide-angled Mendelian randomization investigation. Circ. Genom. Precis. Med. 12, 543–551. doi: 10.1161/CIRCGEN.119.002711
Anrather, J., and Iadecola, C. (2016). Inflammation and stroke: an overview. Neurotherapeutics 13, 661–670. doi: 10.1007/s13311-016-0483-x
Au Yeung, S. L., and Schooling, C. M. (2020). Impact of urinary sodium on cardiovascular disease and risk factors: a 2 sample Mendelian randomization study. Clin. Nutr. 40, 1990–1996. doi: 10.1016/j.clnu.2020.09.018
Bakker, M. K., van der Spek, R. A. A., van Rheenen, W., Morel, S., Bourcier, R., Hostettler, I. C., et al. (2020). Genome-wide association study of intracranial aneurysms identifies 17 risk loci and genetic overlap with clinical risk factors. Nat. Genet. 52, 1303–1313. doi: 10.1038/s41588-020-00725-7
Bowden, J., Smith, G. D., and Burgess, S. (2015). Mendelian randomization with invalid instruments: effect estimation and bias detection through egger regression. Int. J. Epidemiol. 44, 512–525. doi: 10.1093/ije/dyv080
Buniello, A., MacArthur, J. A. L., Cerezo, M., Harris, L. W., Hayhurst, J., Malangone, C., et al. (2019). The NHGRI-EBI GWAS catalog of published genome-wide association studies, targeted arrays and summary statistics 2019. Nucleic Acids Res. 47, D1005–D1012. doi: 10.1093/nar/gky1120
Bycroft, C., Freeman, C., Petkova, D., Band, G., Elliott, L. T., Sharp, K., et al. (2018). The UK biobank resource with deep phenotyping and genomic data. Nature 562, 203–209. doi: 10.1038/s41586-018-0579-z
Cai, H., Cai, B., Zhang, H., Sun, W., Wang, Y., Zhou, S., et al. (2019). Major depression and small vessel stroke: a Mendelian randomization analysis. J. Neurol. 266, 2859–2866. doi: 10.1007/s00415-019-09511-w
Cai, H., Liang, J., Liu, Z., Fang, L., Zheng, J., Xu, J., et al. (2020). Causal effects of sleep traits on ischemic stroke and its subtypes: a Mendelian randomization study. Nat. Sci. Sleep 12, 783–790. doi: 10.2147/NSS.S265946
Chen, Z., Chen, J., Collins, R., Guo, Y., Peto, R., Wu, F., et al. (2011). China Kadoorie biobank of 0.5 million people: survey methods, baseline characteristics and long-term follow-up. Int. J. Epidemiol. 40, 1652–1666. doi: 10.1093/ije/dyr120
Chen, W., Wang, S., Lv, W., and Pan, Y. (2020). Causal associations of insulin resistance with coronary artery disease and ischemic stroke: a Mendelian randomization analysis. BMJ Open Diabetes Res. Care 8:e001214. doi: 10.1136/bmjdrc-2020-001217
Choi, Y., Lee, S. J., Spiller, W., Jung, K. J., Lee, J. Y., Kimm, H., et al. (2020). Causal associations between serum bilirubin levels and decreased stroke risk a two-sample Mendelian randomization study. Arterioscler. Thromb. Vasc. Biol. 40, 437–445. doi: 10.1161/ATVBAHA.119.313055
Chong, M., Sjaarda, J., Pigeyre, M., Mohammadi-Shemirani, P., Lali, R., Shoamanesh, A., et al. (2019). Novel drug targets for ischemic stroke identified through Mendelian randomization analysis of the blood proteome. Circulation 140, 819–830. doi: 10.1161/CIRCULATIONAHA.119.040180
Connolly, E. S., Rabinstein, A. A., Carhuapoma, J. R., Derdeyn, C. P., Dion, J., Higashida, R. T., et al. (2012). Guidelines for the management of aneurysmal subarachnoid hemorrhage: a guideline for healthcare professionals from the American heart association/American stroke association. Stroke 43, 1711–1737. doi: 10.1161/STR.0b013e3182587839
Crawford, K. M., Gallego-Fabrega, C., Kourkoulis, C., Miyares, L., Marini, S., Flannick, J., et al. (2018). Cerebrovascular disease knowledge portal an open-access data resource to accelerate genomic discoveries in stroke. Stroke 49, 470–475. doi: 10.1161/STROKEAHA.117.018922
Dale, C. E., Fatemifar, G., Palmer, T. M., White, J., Prieto-Merino, D., Zabaneh, D., et al. (2017). Causal associations of adiposity and body fat distribution with coronary heart disease, stroke subtypes, and type 2 diabetes mellitus: a Mendelian randomization analysis. Circulation 135, 2373–2388. doi: 10.1161/CIRCULATIONAHA.116.026560
Davies, N. M., Holmes, M. V., and Smith, D. G. (2018). Reading Mendelian randomisation studies: a guide, glossary, and checklist for clinicians. BMJ 362:k601. doi: 10.1136/bmj.k601
Denny, J. C., Rutter, J. L., Goldstein, D. B., Philippakis, A., Smoller, J. W., Jenkins, G., et al. (2019). The “All of Us” research program. N. Engl. J. Med. 381, 668–676. doi: 10.1056/NEJMsr1809937
Falcone, G. J., Kirsch, E., Acosta, J. N., Noche, R. B., Leasure, A., Marini, S., et al. (2020). Genetically elevated LDL associates with lower risk of intracerebral hemorrhage. Ann. Neurol. 88, 56–66. doi: 10.1002/ana.25740
Gan, W., Bragg, F., Walters, R. G., Millwood, I. Y., Lin, K., Chen, Y., et al. (2019). Genetic predisposition to type 2 diabetes and risk of subclinical atherosclerosis and cardiovascular diseases among 160,000 Chinese adults. Diabetes 68, 2155–2164. doi: 10.2337/db19-0224
Gaziano, J. M., Concato, J., Brophy, M., Fiore, L., Pyarajan, S., Breeling, J., et al. (2016). Million veteran program: a mega-biobank to study genetic influences on health and disease. J. Clin. Epidemiol. 70, 214–223. doi: 10.1016/j.jclinepi.2015.09.016
Georgakis, M. K., Gill, D., Malik, R., Protogerou, A. D., Webb, A. J. S., and Dichgans, M. (2020a). Genetically predicted blood pressure across the lifespan differential effects of mean and pulse pressure on stroke risk. Hypertension 76, 953–961. doi: 10.1161/HYPERTENSIONAHA.120.15136
Georgakis, M. K., Gill, D., Rannikmäe, K., Traylor, M., Anderson, C. D., Lee, J. M., et al. (2019). Genetically determined levels of circulating cytokines and risk of stroke: role of monocyte chemoattractant protein-1. Circulation 139, 256–268. doi: 10.1161/CIRCULATIONAHA.118.035905
Georgakis, M. K., Gill, D., Webb, A., Evangelou, E., Elliott, P., Sudlow, C., et al. (2020b). Genetically determined blood pressure, antihypertensive drug classes and risk of stroke subtypes. Neurology 95, e353–e361. doi: 10.1212/WNL.0000000000009814
Georgakis, M. K., Harshfield, E. L., Malik, R., Franceschini, N., Langenberg, C., Wareham, N. J., et al. (2021). Diabetes mellitus, glycemic traits, and cerebrovascular disease: a Mendelian randomization study. Neurology 96, e1732–e1742. doi: 10.1212/WNL.0000000000011555
Georgakis, M. K., Malik, R., Anderson, C. D., Parhofer, K. G., Hopewell, J. C., and Dichgans, M. (2020c). Genetic determinants of blood lipids and cerebral small vessel disease: role of high-density lipoprotein cholesterol. Brain 143, 597–610. doi: 10.1093/brain/awz413
Georgakis, M. K., Malik, R., Gill, D., Franceschini, N., Sudlow, C. L. M., and Dichgans, M. (2020d). Interleukin-6 signaling effects on ischemic stroke and other cardiovascular outcomes: a Mendelian randomization study. Circ. Genom. Precis. Med. 13:e002872. doi: 10.1161/CIRCGEN.119.002872
Gill, D., Georgakis, M. K., Koskeridis, F., Jiang, L., Feng, Q., Wei, W. Q., et al. (2019a). Use of genetic variants related to antihypertensive drugs to inform on efficacy and side effects. Circulation 140, 270–279. doi: 10.1161/CIRCULATIONAHA.118.038814
Gill, D., Georgakis, M. K., Laffan, M., Sabater-Lleal, M., Malik, R., Tzoulaki, I., et al. (2018a). Genetically determined FXI (factor XI) levels and risk of stroke. Stroke 49, 2761–2763. doi: 10.1161/STROKEAHA.118.022792
Gill, D., Georgakis, M. K., Walker, V. M., Schmidt, A. F., Gkatzionis, A., Freitag, D. F., et al. (2021). Mendelian randomization for studying the effects of perturbing drug targets. Wellcome Open Res. 6:16. doi: 10.12688/wellcomeopenres.16544.2
Gill, D., James, N. E., Monori, G., Lorentzen, E., Fernandez-Cadenas, I., Lemmens, R., et al. (2019b). Genetically determined risk of depression and functional outcome after ischemic stroke: Mendelian randomization study. Stroke 50, 2219–2222. doi: 10.1161/STROKEAHA.119.026089
Gill, D., Monori, G., Georgakis, M. K., Tzoulaki, I., and Laffan, M. (2018b). Genetically determined platelet count and risk of cardiovascular disease: Mendelian randomization study. Arterioscler. Thromb. Vasc. Biol. 38, 2862–2869. doi: 10.1161/ATVBAHA.118.311804
Gill, D., Monori, G., Tzoulaki, I., and Dehghan, A. (2018c). Iron status and risk of stroke: a Mendelian randomization study. Stroke 49, 2815–2821. doi: 10.1161/STROKEAHA.118.022701
Harshfield, E. L., Sims, M. C., Traylor, M., Ouwehand, W. H., and Markus, H. S. (2020). The role of haematological traits in risk of ischaemic stroke and its subtypes. Brain 143, 210–221. doi: 10.1093/brain/awz362
Hemani, G., Zheng, J., Elsworth, B., Wade, K. H., Haberland, V., Baird, D., et al. (2018). The MR-base platform supports systematic causal inference across the human phenome. eLife 7, 1–29. doi: 10.7554/eLife.34408
Hemphill, J. C., Greenberg, S. M., Anderson, C. S., Becker, K., Bendok, B. R., Cushman, M., et al. (2015). Guidelines for the management of spontaneous intracerebral hemorrhage: a guideline for healthcare professionals from the American heart association/American stroke association. Stroke 46, 2032–2060. doi: 10.1161/STR.0000000000000069
Hindy, G., Engström, G., Larsson, S. C., Traylor, M., Markus, H. S., Melander, O., et al. (2018). Role of blood lipids in the development of ischemic stroke and its subtypes: a Mendelian randomization study. Stroke 49, 820–827. doi: 10.1161/STROKEAHA.117.019653
Hou, L., Xu, M., Yu, Y., Sun, X., Liu, X., Liu, L., et al. (2020). Exploring the causal pathway from ischemic stroke to atrial fibrillation: a network Mendelian randomization study. Mol. Med. 26, 1–9. doi: 10.1186/s10020-019-0133-y
Huang, T., Afzal, S., Yu, C., Guo, Y., Bian, Z., Yang, L., et al. (2019). Vitamin D and cause-specific vascular disease and mortality: a Mendelian randomisation study involving 99,012 Chinese and 106,911 European adults. BMC Med. 17:160. doi: 10.1186/s12916-019-1401-y
Jia, J., Dou, P., Gao, M., Kong, X., Li, C., Liu, Z., et al. (2019). Assessment of causal direction between gut microbiota- dependent metabolites and cardiometabolic health: a bidirectional Mendelian randomization analysis. Diabetes 68, 1747–1755. doi: 10.2337/db19-0153
Johnson, C. O., Nguyen, M., Roth, G. A., Nichols, E., Alam, T., Abate, D., et al. (2019). Global, regional, and national burden of stroke, 1990–2016: a systematic analysis for the global burden of disease study 2016. Lancet Neurol. 18, 439–458. doi: 10.1016/S1474-4422(19)30034-1
Lambert, S. A., Jupp, S., Abraham, G., Parkinson, H., Danesh, J., MacArthur, J. A. L., et al. (2019). The Polygenic Score (pgs) Catalog: A Database of Published PGS to Enable Reproducibility and Uniform Evaluation. Available at: https://www.pgscatalog.org (Accessed February 1, 2021).
Lappalainen, I., Almeida-King, J., Kumanduri, V., Senf, A., Spalding, J. D., Ur-Rehman, S., et al. (2015). The European genome-phenome archive of human data consented for biomedical research. Nat. Genet. 47, 692–695. doi: 10.1038/ng.3312
Larsson, S. C., Burgess, S., Mason, A. M., and Michaëlsson, K. (2020a). Alcohol consumption and cardiovascular disease: a Mendelian randomization study. Circ. Genom. Precis. Med. 13:e002814. doi: 10.1161/CIRCGEN.119.002814
Larsson, S. C., Burgess, S., and Michaëlsson, K. (2019a). Smoking and stroke: a Mendelian randomization study. Ann. Neurol. 86, 468–471. doi: 10.1002/ana.25534
Larsson, S. C., Gill, D., Mason, A. M., Jiang, T., Bäck, M., Butterworth, A. S., et al. (2020b). Lipoprotein(a) in Alzheimer, atherosclerotic, cerebrovascular, thrombotic, and valvular disease: Mendelian randomization investigation. Circulation 141, 1826–1828. doi: 10.1161/CIRCULATIONAHA.120.045826
Larsson, S. C., and Markus, H. S. (2019). Genetic liability to insomnia and cardiovascular disease risk. Circulation 140, 796–798. doi: 10.1161/CIRCULATIONAHA.119.041830
Larsson, S. C., Mason, A. M., Bäck, M., Klarin, D., Damrauer, S. M., Michaëlsson, K., et al. (2020c). Genetic predisposition to smoking in relation to 14 cardiovascular diseases. Eur. Heart J. 41, 3304–3310. doi: 10.1093/eurheartj/ehaa193
Larsson, S. C., Scott, R. A., Traylor, M., Langenberg, C. C., Hindy, G., Melander, O., et al. (2017). Type 2 diabetes, glucose, insulin, BMI, and ischemic stroke subtypes: Mendelian randomization study. Neurology 89, 454–460. doi: 10.1212/WNL.0000000000004173
Larsson, S. C., Traylor, M., Burgess, S., Boncoraglio, G. B., Jern, C., Michaëlsson, K., et al. (2019b). Serum magnesium and calcium levels in relation to ischemic stroke: Mendelian randomization study. Neurology 92, E944–E950. doi: 10.1212/WNL.0000000000007001
Larsson, S. C., Traylor, M., and Markus, H. S. (2018a). Circulating vitamin K1 levels in relation to ischemic stroke and its subtypes: a Mendelian randomization study. Nutrients 10:1575. doi: 10.3390/nu10111575
Larsson, S. C., Traylor, M., Mishra, A., Howson, J. M. M., Michaëlsson, K., and Markus, H. S. (2018b). Serum 25-hydroxyvitamin D concentrations and ischemic stroke and its subtypes a Mendelian randomization study. Stroke 49, 2508–2511. doi: 10.1161/STROKEAHA.118.022242
Lin, J., Wang, Y., Wang, Y., and Pan, Y. (2020). Inflammatory biomarkers and risk of ischemic stroke and subtypes: a 2-sample Mendelian randomization study. Neurol. Res. 42, 118–125. doi: 10.1080/01616412.2019.1710404
Liu, J., Rutten-Jacobs, L., Liu, M., Markus, H. S., and Traylor, M. (2018). Causal impact of type 2 diabetes mellitus on cerebral small vessel disease: a Mendelian randomization analysis. Stroke 49, 1325–1331. doi: 10.1161/STROKEAHA.117.020536
Lu, H., Wu, P. F., Li, R. Z., Zhang, W., and Huang, G. X. (2020). Sleep duration and stroke: a Mendelian randomization study. Front. Neurol. 11:976. doi: 10.3389/fneur.2020.00976
Ma, C., Gurol, M. E., Huang, Z., Lichtenstein, A. H., Wang, X., Wang, Y., et al. (2019). Low-density lipoprotein cholesterol and risk of intracerebral hemorrhage. Neurology 93, E445–E457. doi: 10.1212/WNL.0000000000007853
Marini, S., Merino, J., Montgomery, B. E., Malik, R., Sudlow, C. L., Dichgans, M., et al. (2020). Mendelian randomization study of obesity and cerebrovascular disease. Ann. Neurol. 87, 516–524. doi: 10.1002/ana.25686
Marouli, E., Kus, A., Del Greco, M. F., Chaker, L., Peeters, R., Teumer, A., et al. (2020). Thyroid function affects the risk of stroke via atrial fibrillation: a Mendelian randomization study. J. Clin. Endocrinol. Metab. 105, 2634–2641. doi: 10.1210/clinem/dgaa239
Mokry, L. E., Ahmad, O., Forgetta, V., Thanassoulis, G., and Richards, J. B. (2015). Mendelian randomisation applied to drug development in cardiovascular disease: a review. J. Med. Genet. 52, 71–79. doi: 10.1136/jmedgenet-2014-102438
Morrison, J., Knoblauch, N., Marcus, J. H., Stephens, M., and He, X. (2020). Mendelian randomization accounting for correlated and uncorrelated pleiotropic effects using genome-wide summary statistics. Nat. Genet. 52, 740–747. doi: 10.1038/s41588-020-0631-4
Nagai, A., Hirata, M., Kamatani, Y., Muto, K., Matsuda, K., Kiyohara, Y., et al. (2017). Overview of the BioBank Japan project: study design and profile. J. Epidemiol. 27, S2–S8. doi: 10.1016/j.je.2016.12.005
Nelson, M. R., Tipney, H., Painter, J. L., Shen, J., Nicoletti, P., Shen, Y., et al. (2015). The support of human genetic evidence for approved drug indications. Nat. Genet. 47, 856–860. doi: 10.1038/ng.3314
Pan, Y., Li, H., Wang, Y., Meng, X., and Wang, Y. (2019). Causal effect of Lp(a) [lipoprotein(a)] level on ischemic stroke and alzheimer disease a Mendelian randomization study. Stroke 50, 3532–3539. doi: 10.1161/STROKEAHA.119.026872
Peters, S. A. E., Huxley, R. R., and Woodward, M. (2013). Smoking as a risk factor for stroke in women compared with men: a systematic review and meta-analysis of 81 cohorts, including 3 980 359 individuals and 42 401 strokes. Stroke 44, 2821–2828. doi: 10.1161/STROKEAHA.113.002342
Powers, W. J., Rabinstein, A. A., Ackerson, T., Adeoye, O. M., Bambakidis, N. C., Becker, K., et al. (2019). Guidelines for the early management of patients with acute ischemic stroke: 2019 update to the 2018 guidelines for the early management of acute ischemic stroke a guideline for healthcare professionals from the American heart association/American stroke association. Stroke 50, e344–e418. doi: 10.1161/STR.0000000000000211
Qian, Y., Ye, D., Huang, H., Wu, D. J. H., Zhuang, Y., Jiang, X., et al. (2020). Coffee consumption and risk of stroke: a Mendelian randomization study. Ann. Neurol. 87, 525–532. doi: 10.1002/ana.25693
Qian, Y., Ye, D., Wu, D. J. H., Feng, C., Zeng, Z., Ye, L., et al. (2019). Role of cigarette smoking in the development of ischemic stroke and its subtypes: a Mendelian randomization study. Clin. Epidemiol. 11, 725–731. doi: 10.2147/CLEP.S215933
Saliba, W., Rennert, H. S., Barnett-Griness, O., Gronich, N., Molad, J., Rennert, G., et al. (2018). Association of statin use with spontaneous intracerebral hemorrhage: a cohort study. Neurology 91, e400–e409. doi: 10.1212/WNL.0000000000005907
Schmidt, A. F., Finan, C., Gordillo-Marañón, M., Asselbergs, F. W., Freitag, D. F., Patel, R. S., et al. (2020). Genetic drug target validation using Mendelian randomisation. Nat. Commun. 11:3255. doi: 10.1038/s41467-020-16969-0
Schooling, C. M., Luo, S., Au Yeung, S. L., Thompson, D. J., Karthikeyan, S., Bolton, T. R., et al. (2018). Genetic predictors of testosterone and their associations with cardiovascular disease and risk factors: a Mendelian randomization investigation. Int. J. Cardiol. 267, 171–176. doi: 10.1016/j.ijcard.2018.05.051
Song, L., Sun, J., Söderholm, M., Melander, O., Orho-Melander, M., Nilsson, J., et al. (2020). Association of TIM-1 (T-cell immunoglobulin and mucin domain 1) with incidence of stroke. Arterioscler. Thromb. Vasc. Biol. 40, 1777–1786. doi: 10.1161/ATVBAHA.120.314269
Sposato, L. A., Cerasuolo, J. O., Cipriano, L. E., Fang, J., Fridman, S., Paquet, M., et al. (2018). Atrial fibrillation detected after stroke is related to a low risk of ischemic stroke recurrence. Neurology 90, e924–e931. doi: 10.1212/WNL.0000000000005126
Sposato, L. A., Cipriano, L. E., Saposnik, G., Vargas, E. R., Riccio, P. M., and Hachinski, V. (2015). Diagnosis of atrial fibrillation after stroke and transient ischaemic attack: a systematic review and meta-analysis. Lancet Neurol. 14, 377–387. doi: 10.1016/S1474-4422(15)70027-X
Sun, L., Clarke, R., Bennett, D., Guo, Y., Walters, R. G., Hill, M., et al. (2019). Causal associations of blood lipids with risk of ischemic stroke and intracerebral hemorrhage in Chinese adults. Nat. Med. 25, 569–574. doi: 10.1038/s41591-019-0366-x
Tian, D., Wang, P., Tang, B., Teng, X., Li, C., Liu, X., et al. (2020). GWAS atlas: a curated resource of genome-wide variant-trait associations in plants and animals. Nucleic Acids Res. 48, D927–D932. doi: 10.1093/nar/gkz828
Titova, O. E., Michaëlsson, K., and Larsson, S. C. (2020). Sleep duration and stroke: prospective cohort study and Mendelian randomization analysis. Stroke 51, 3279–3285. doi: 10.1161/STROKEAHA.120.029902
Valdes-Marquez, E., Parish, S., Clarke, R., Stari, T., Worrall, B. B., and Hopewell, J. C. (2019). Relative effects of LDL-C on ischemic stroke and coronary disease: a Mendelian randomization study. Neurology 92, E1176–E1187. doi: 10.1212/WNL.0000000000007091
Verbanck, M., Chen, C. Y., Neale, B., and Do, R. (2018). Detection of widespread horizontal pleiotropy in causal relationships inferred from Mendelian randomization between complex traits and diseases. Nat. Genet. 50, 693–698. doi: 10.1038/s41588-018-0099-7
Walker, V. M., Kehoe, P. G., Martin, R. M., and Davies, N. M. (2020). Repurposing antihypertensive drugs for the prevention of Alzheimer’s disease: a Mendelian randomization study. Int. J. Epidemiol. 49, 1132–1140. doi: 10.1093/ije/dyz155
Wang, M., Bai, Y., Wang, Z., Zhang, Z., Liu, D., and Lian, X. (2021). Higher tea consumption is associated with decreased risk of small vessel stroke. Clin. Nutr. 40, 1430–1435. doi: 10.1016/j.clnu.2020.08.039
Wang, X., Dong, Y., Qi, X., Huang, C., and Hou, L. (2013). Cholesterol levels and risk of hemorrhagic stroke: a systematic review and meta-analysis. Stroke 44, 1833–1839. doi: 10.1161/STROKEAHA.113.001326
Wang, Z., Yang, F., Ma, M., Bao, Q., Shen, J., Ye, F., et al. (2020). The impact of growth differentiation factor 15 on the risk of cardiovascular diseases: two-sample Mendelian randomization study. BMC Cardiovasc. Disord. 20:462. doi: 10.1186/s12872-020-01744-2
Wong, K. M., Langlais, K., Tobias, G. S., Fletcher-Hoppe, C., Krasnewich, D., Leeds, H. S., et al. (2017). The dbGaP data browser: a new tool for browsing dbGaP controlled-access genomic data. Nucleic Acids Res. 45, D819–D826. doi: 10.1093/nar/gkw1139
Wu, C., Wu, L., Wang, J., Lin, L., Li, Y., Lu, Q., et al. (2021). Systematic identification of risk factors and drug repurposing options for Alzheimer’s disease. Alzheimer's Dement. 7:e12148. doi: 10.1002/trc2.12148
Yeung, S. L. A., Luo, S., and Schooling, C. M. (2018). The impact of glycated hemoglobin (HbA1c) on cardiovascular disease risk: a Mendelian randomization study using UK biobank. Diabetes Care 41, 1991–1997. doi: 10.2337/dc18-0289
Yuan, S., Bäck, M., Bruzelius, M., Mason, A. M., Burgess, S., and Larsson, S. (2019). Plasma phospholipid fatty acids, FADS1 and risk of 15 cardiovascular diseases: a Mendelian randomisation study. Nutrients 11:3001. doi: 10.3390/nu11123001
Yuan, S., Carter, P., Bruzelius, M., Vithayathil, M., Kar, S., Mason, A. M., et al. (2020a). Effects of tumour necrosis factor on cardiovascular disease and cancer: a two-sample Mendelian randomization study. EBioMedicine 59:102956. doi: 10.1016/j.ebiom.2020.102956
Yuan, S., Lin, A., He, Q.-Q., Burgess, S., and Larsson, S. C. (2020b). Circulating interleukins in relation to coronary artery disease, atrial fibrillation and ischemic stroke and its subtypes: a two-sample Mendelian randomization study. Int. J. Cardiol. 313, 99–104. doi: 10.1016/j.ijcard.2020.03.053
Yuan, T., Si, S., Li, Y., Li, W., Chen, X., Liu, C., et al. (2020c). Roles for circulating polyunsaturated fatty acids in ischemic stroke and modifiable factors: a Mendelian randomization study. Nutr. J. 19:70. doi: 10.1186/s12937-020-00582-4
Zhang, X., Wang, A., Zhang, J., Singh, M., Liu, D., Zuo, Y., et al. (2020). Association of plasma C-reactive protein with ischaemic stroke: a Mendelian randomization study. Eur. J. Neurol. 27, 565–571. doi: 10.1111/ene.14113
Keywords: stroke, genetics, Mendelian randomization, polygenic risk scores, drug target validation
Citation: Acosta JN, Szejko N and Falcone GJ (2021) Mendelian Randomization in Stroke: A Powerful Approach to Causal Inference and Drug Target Validation. Front. Genet. 12:683082. doi: 10.3389/fgene.2021.683082
Received: 19 March 2021; Accepted: 28 June 2021;
Published: 12 August 2021.
Edited by:
Lei Zhang, Soochow University, ChinaReviewed by:
Chaolong Wang, Huazhong University of Science and Technology, ChinaCopyright © 2021 Acosta, Szejko and Falcone. This is an open-access article distributed under the terms of the Creative Commons Attribution License (CC BY). The use, distribution or reproduction in other forums is permitted, provided the original author(s) and the copyright owner(s) are credited and that the original publication in this journal is cited, in accordance with accepted academic practice. No use, distribution or reproduction is permitted which does not comply with these terms.
*Correspondence: Guido J. Falcone, Z3VpZG8uZmFsY29uZUB5YWxlLmVkdQ==
Disclaimer: All claims expressed in this article are solely those of the authors and do not necessarily represent those of their affiliated organizations, or those of the publisher, the editors and the reviewers. Any product that may be evaluated in this article or claim that may be made by its manufacturer is not guaranteed or endorsed by the publisher.
Research integrity at Frontiers
Learn more about the work of our research integrity team to safeguard the quality of each article we publish.