- 1Department of Female Plastic Surgery, Gansu Provincial Maternity and Child-Care Hospital, Lanzhou, China
- 2Department of Pediatric Surgery, Gansu Provincial Maternity and Child-Care Hospital, Lanzhou, China
We aim to find a biomarker that can effectively predict the prognosis of patients with cutaneous melanoma (CM). The RNA sequencing data of CM was downloaded from The Cancer Genome Atlas (TCGA) database and randomly divided into training group and test group. Survival statistical analysis and machine-learning approaches were performed on the RNA sequencing data of CM to develop a prognostic signature. Using univariable Cox proportional hazards regression, random survival forest algorithm, and receiver operating characteristic (ROC) in the training group, the four-mRNA signature including CD276, UQCRFS1, HAPLN3, and PIP4P1 was screened out. The four-mRNA signature could divide patients into low-risk and high-risk groups with different survival outcomes (log-rank p < 0.001). The predictive efficacy of the four-mRNA signature was confirmed in the test group, the whole TCGA group, and the independent GSE65904 (log-rank p < 0.05). The independence of the four-mRNA signature in prognostic prediction was demonstrated by multivariate Cox analysis. ROC and timeROC analyses showed that the efficiency of the signature in survival prediction was better than other clinical variables such as melanoma Clark level and tumor stage. This study highlights that the four-mRNA model could be used as a prognostic signature for CM patients with potential clinical application value.
Introduction
Cutaneous melanoma (CM) is a highly aggressive and heterogeneous skin malignancy. In recent years, the morbidity and mortality of CM have increased significantly (Dimitriou et al., 2018), with approximately 232,100 new cases and 55,500 death each year (Schadendorf et al., 2018). Although CM is usually detected in T1 stage and the corresponding patients’ 5-year survival exceeds 90%, the rapid progression of melanoma and the failure to detect thin melanoma in time lead to the progression and metastasis, which worsen the prognosis of CM patients [the 5-year survival rate is reduced to 62.9% (Dinnes et al., 2018) for regional lymph nodes spread and 19% (Miller et al., 2019) for distant metastasis]. Classic prognostic factors, including age and American Joint Committee on Cancer (AJCC) stage, have been proven to be effective indicators for melanoma (Kretschmer et al., 2011; Shields et al., 2012, 2015). Melanoma-specific indicators, including Clark level and Breslow thickness (Morton et al., 1993; Panda et al., 2018), are also used to assess the survival of CM patients. However, these clinicopathological indicators cannot reflect the molecular heterogeneity of melanoma (Palmieri et al., 2018), nor can they accurately predict the clinical outcome. Thus, novel prognostic biomarkers are extremely necessary for CM patients.
The development of sequencing technology and bioinformatics tools has promoted the discovery of new tumor biomarkers and the study of tumor molecular mechanisms. Based on the analysis of public messenger RNA (mRNA) expression data, studies have shown that gene signature could be prognostic marker for different types of tumors. For instance, a nine-gene signature can reliably predict the overall survival of patients with pancreatic cancer (Wu et al., 2019). An eight-gene signature showed a robust prognostic performance in early-stage non-small cell lung cancer (He et al., 2019). A 15-gene signature has been found to divide colon cancer patients into two groups with different prognosis (Xu et al., 2017). The prognostic two-gene signature has presented good predictive ability in the prognosis of GBM patients (Pan et al., 2020). For melanoma, Wang et al. (2020) identified that an eight-gene signature could independently predict the poor clinical outcome of melanoma patients.
It is well known that signatures with fewer genes have better clinical significance. In this study, through mining the mRNA expression profile and clinical information of 385 CM patients by statistical and machine-learning analyses, we aim to evaluate the prognostic significance of all expressed mRNAs and construct an effective prognostic signature for CM patients.
Materials and Methods
Expression Profile of CM Patients
The clinical parameters and mRNA expression data of CM in The Cancer Genome Atlas (TCGA) database1 were from UCSC Xena2. The CM cases with clinical survival follow-up information were selected to establish a prognostic model, which were randomly divided into training and test groups. The independent validation set (GSE65904) was obtained from Gene Expression Omnibus (GEO) database. The clinical details of CM patients including age; gender; pathological T, N, and M stage; and tumor stage are displayed and summarized in Supplementary Table 1. We discarded genes whose expression values were missing in more than 20% of CM samples. All expression values were log2 transformed.
The Process of Developing Prognostic Models Through Statistics and Machine-Learning Methods
Univariable Cox analysis was applied to seek mRNAs significantly correlated with CM patients’ OS in the training group. Then, random survival forest (RSFVH, a machine learning approach) was performed. In RSFVH, an iteration procedure was implemented to reduce the node set in which the one-third least important mRNAs were discarded at each iteration step (Li et al., 2014). As a result, we obtained a set of prognosis-related mRNAs that contained a relatively small number. Subsequently, we constructed prognostic combination models as the following formula:
where N is the number of prognosis-related mRNAs, mRNAExp is the expression value of prognosis-related mRNA, and the coefficient of prognosis-related mRNA is derived from Cox regression. The prognostic RS model was selected for its largest area under the curve (AUC) value from all the combinations (Zhang et al., 2020).
Statistical Analysis
Log rank test and Kaplan–Meier (KM) analysis were performed to analyze the difference in survival between the two groups separated by the median risk score. Chi-square test was performed to test the association of the selected signature with other clinical parameters. The predictive performance of the signature in survival was tested by receiver operating characteristic (ROC) and time-dependent ROC. The R program (⁇) performed the above analyses using R packages named pROC, randomForestSRC, and survival. The prognostic mRNAs were analyzed by Gene Ontology (GO) and Kyoto Encyclopedia of Genes and Genomes (KEGG) analysis through the Cluego plug-in of Cytoscape software (Bindea et al., 2009).
Results
Development of the Prognostic Four-mRNA Signature
From the TCGA database, we obtained 385 patients diagnosed with CM and their mRNA expression profiles including a total of 18,496 expressed mRNAs. After summarizing the clinical characteristics of CM patients, we found that the median age was 58 years (15–87 years), and a large proportion of patients were men, indicating that CM was more common in male adults. Additionally, we found that the median survival time of CM patients was 37.5 months, and only a small number of people were still alive, confirming the poor prognosis of CM (Supplementary Table 1).
After univariable Cox regression, we discovered 3,058 mRNAs that were significantly related to survival (red and blue dots in Figure 1A, p < 0.05). Subsequently, we screened out 12 prognostic mRNAs based on the importance score of RSFVH analysis (Figure 1B). When these 12 prognostic mRNAs were incorporated into the risk prediction model in different combinations, we got a total of 212–1 = 4,095 possible signatures. After performing ROC analysis on all 4,095 signatures, we found a four-mRNA signature with the largest AUC value (AUCsignature = 0.708; Figures 1C,D and Table 1). The prognostic four mRNAs from the signature were CD276, HAPLN3, PIP4P1, and UQCRFS1. The selected RS model formula was as follows: RS = (0.35 × CD276 expression level) + (0.75 × UQCRFS1 expression level) + (-0.41 × HAPLN3 expression level) + (-0.75 × PIP4P1 expression level).
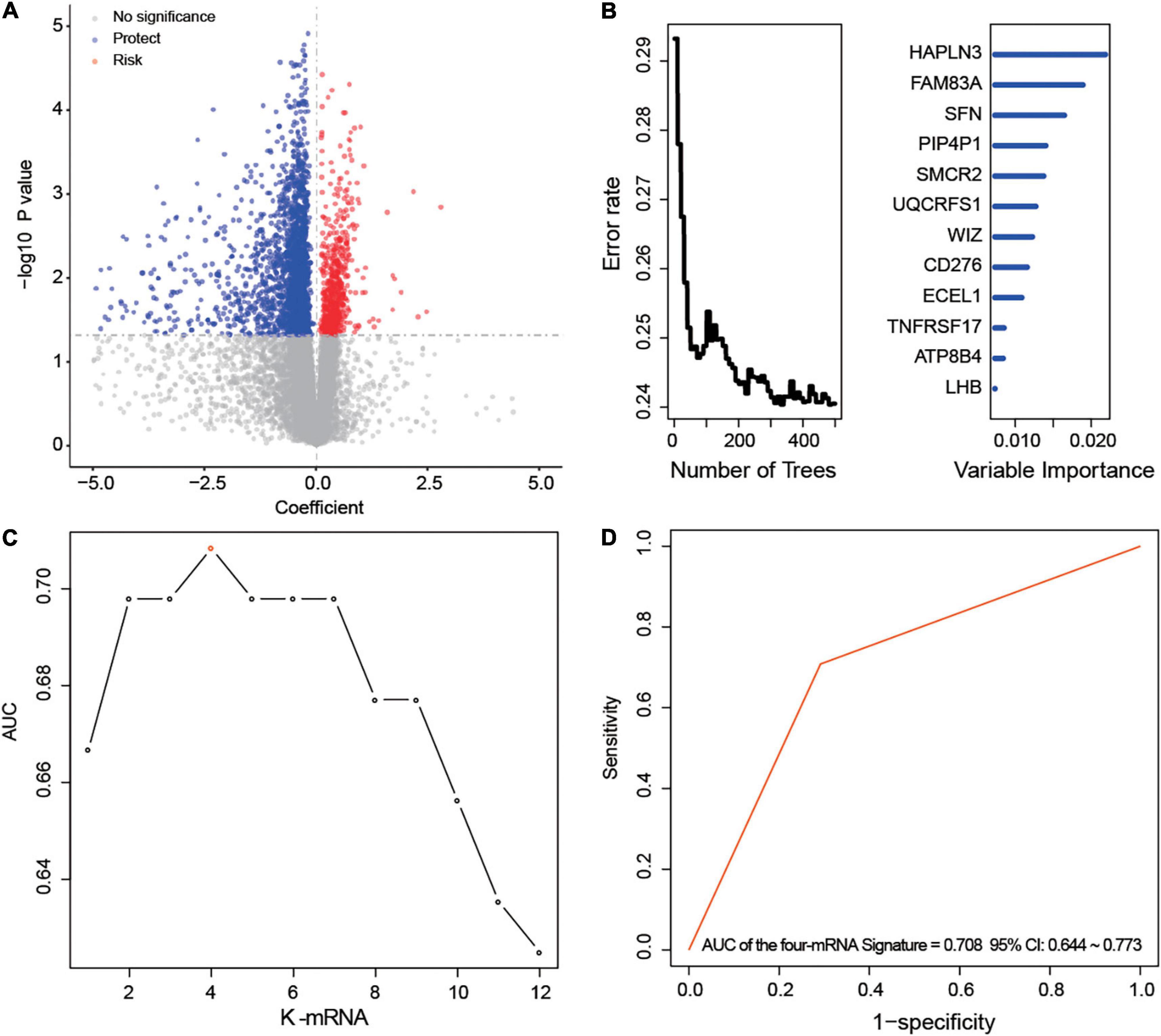
Figure 1. Development of the prognostic messenger RNA (mRNA) signature. (A) The survival-associated mRNAs obtained from Cox analysis are displayed on the volcano plot. (B) After random forest classification algorithm, the prognosis-associated mRNAs were decreased to 12. (C,D) The prognostic four-mRNA signature was selected because its area under the curve (AUC) was the largest (AUC = 0.708) among the 212–1 = 4,095 signatures.
The Survival Prediction Performance and Validation of the Four-mRNA Signature
Based on the model constructed above, the risk scores of CM patients were calculated, and the median risk score was obtained as the cutoff. In the training dataset, the median RS divided patients into a high-risk group (n = 96) or a low-risk group (n = 96). Kaplan–Meier analysis showed that there were a significant difference in survival time between patients in the high- and the low-risk group (median survival time: 29.2 vs. 104.7 months, p < 0.001; Figure 2A). Then, Kaplan–Meier analysis was performed in the test (n = 193) and entire TCGA datasets (n = 385). The four-mRNA signature could distinguish the CM patients into two risk groups with different survival outcomes in the test group (median survival time: 43.8 vs. 70.0 months, p < 0.001; Figure 2B). The same performance for survival prediction was shown in the entire TCGA dataset (median survival time: 38.5 vs. 86.3 months, p < 0.001; Figure 2C). In addition, we also verified its survival prediction performance in an independent set (GSE65904, n = 150) from GEO database. The median RS value also classified patients from GSE65904 into high- or low-risk group significantly (p = 0.017, Figure 2D). Moreover, when the patient’s mRNA expression, survival time, and risk score were displayed on the same chart, we found that CM patients with higher risk mRNAs expression and higher risk scores had poorer survival outcomes in the training (Figure 3A), test (Figure 3B), entire TCGA datasets (Figure 3C), and GSE65904 (Figure 3D).
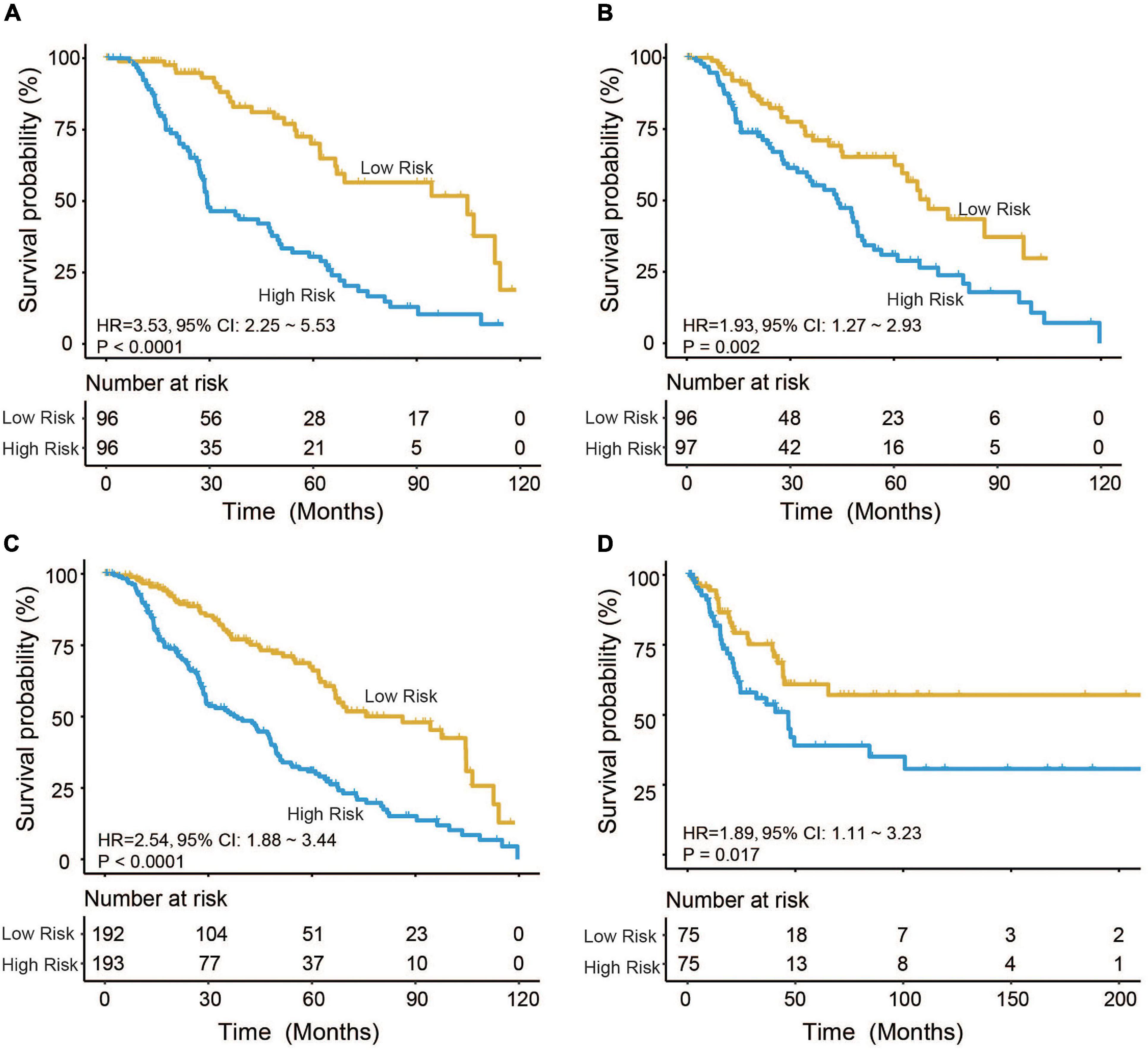
Figure 2. Cutaneous melanoma patients were divided by the four-messenger RNA (four-mRNA) signature into two risk groups with significantly different survival outcomes in the (A) training, (B) test, (C) entire The Cancer Genome Atlas (TCGA), and (D) GSE65904 datasets.
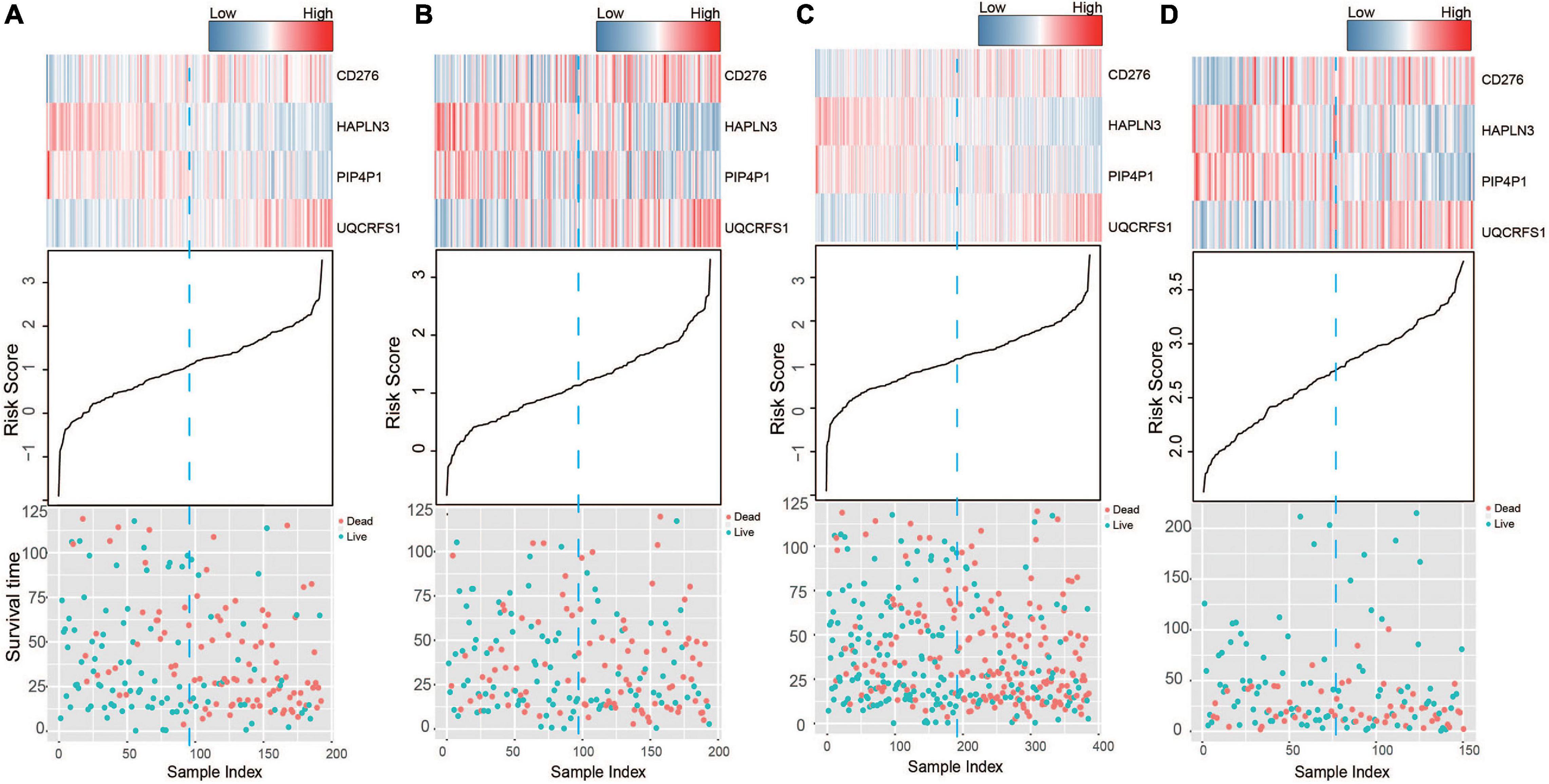
Figure 3. The risk score distribution, survival status, and messenger RNA (mRNA) expression patterns of cutaneous melanoma patients in the (A) training, (B) test, (C) entire The Cancer Genome Atlas (TCGA), and (D) GSE65904 datasets.
Independent Prognostic Value of the Four-mRNA Signature
Chi-square test did not detect the relationship between the four-mRNA signature and other clinical variables (Table 2). Then, we tested the independence of the four-mRNA model by performing Cox regression analysis (including univariate and multivariable Cox, Table 3). Multivariable Cox regression confirmed the independence of four-mRNA signature in prognosis prediction in the training, test, entire TCGA, or GSE65904 datasets (HR training = 3.00, p < 0.001, n = 192; HR test = 1.72, p < 0.05, n = 193; HR entire = 2.00, p < 0.001, n = 385; HRGSE65904 = 2.25, p < 0.001, n = 150, Table 3).
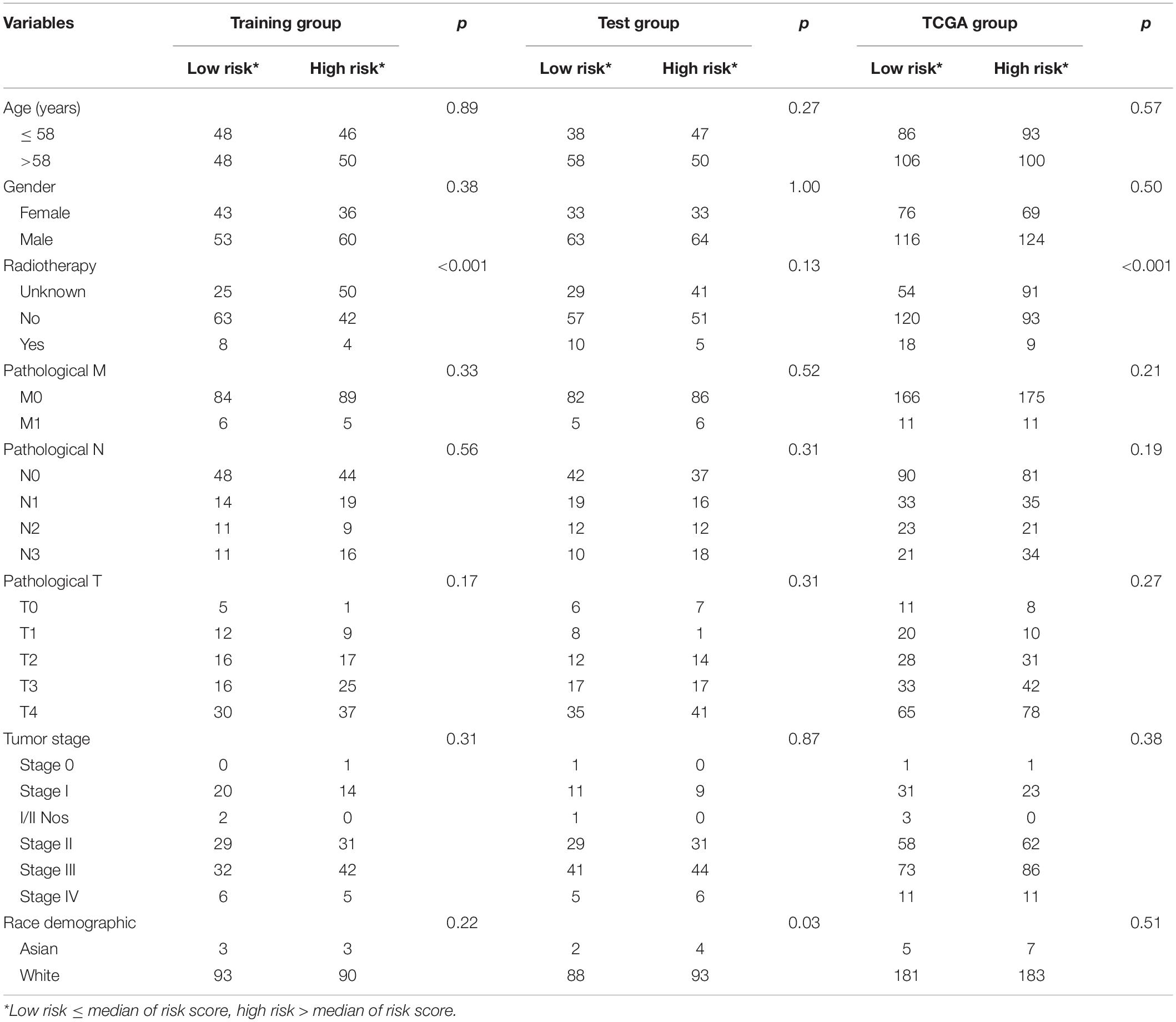
Table 2. Association of the messenger RNA (mRNA) signature with clinical characteristics in cutaneous melanoma (CM) patients.
The Comparison of the Performance in Survival Prediction Between the Four-mRNA Signature With Melanoma Clark Level and Tumor Stage
We compared the performance of the four-mRNA signature with other clinical prognostic markers (including melanoma Clark level and tumor stage) in predicting survival. Figure 4A shows that the four-mRNA signature was better than other clinical variables in survival prediction of the entire TCGA set (AUC signature = 0.67 vs. AUCtumor stage = 0.52 vs. AUCmelanoma Clark level = 0.55). TimeROC analysis found that the AUC values of the four-mRNA signature within 1–12 years were greater than that of the Clark level or tumor stage (Figure 4B). All these suggest that the four-mRNA signature have better performance in survival prediction of CM.
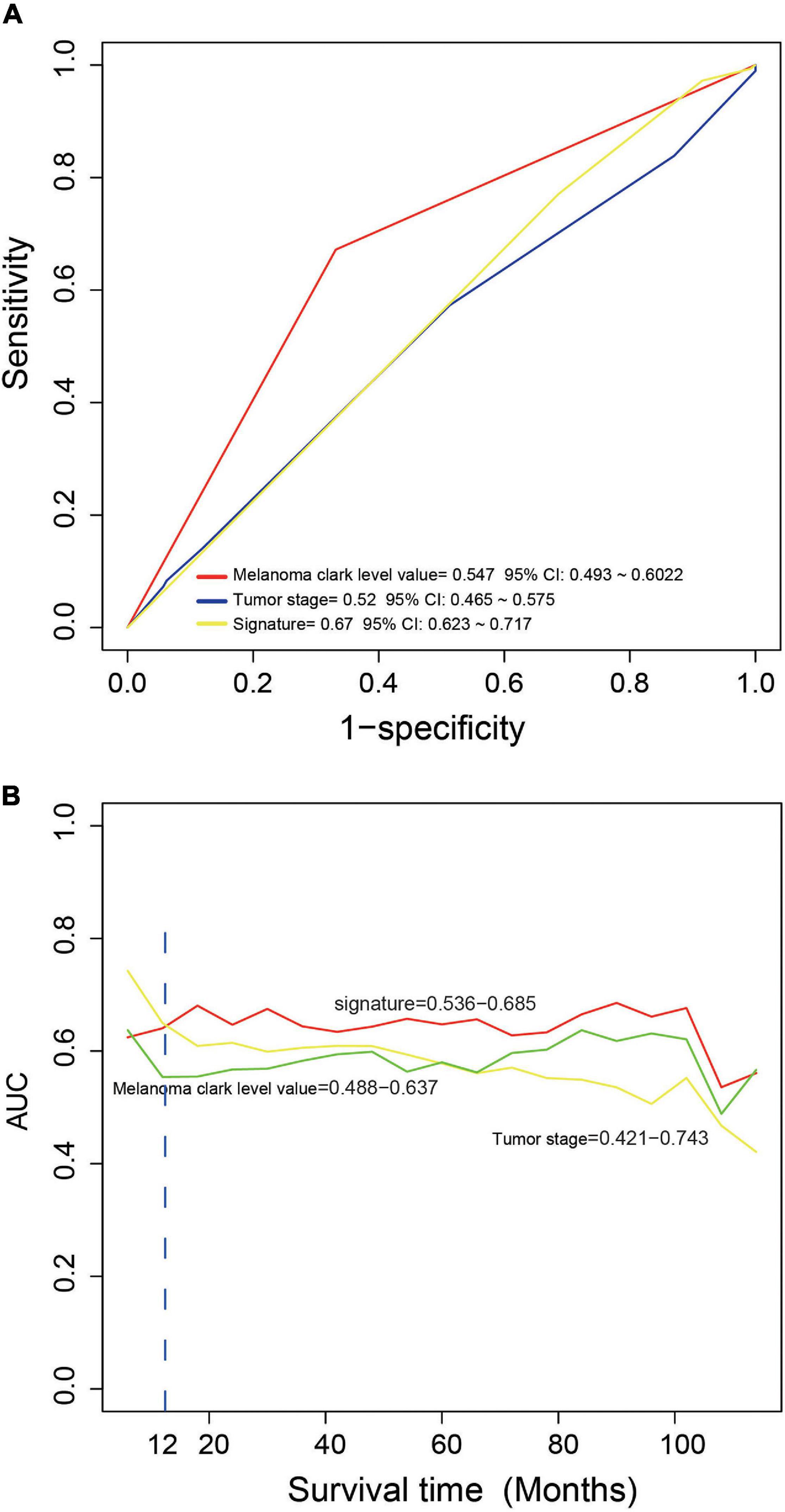
Figure 4. The comparison of the performance in survival prediction between the four-messenger RNA (four-mRNA) signature with tumor stage and Clark level. (A) ROC analysis was performed to compare the performance of the four-mRNA signature with that of tumor stage and Clark level. (B) TimeROC analysis was conduct to compare the performance of the four-mRNA signature with that of tumor stage and Clark level.
Function Enrichment Analysis of the Four Selected mRNAs
To explore the biological roles of the four selected mRNAs from the signature in the CM, we conducted Pearson correlation test and obtained a total of 533 coexpressed genes in the TCGA dataset (coefficient > 0.5/ < -0.5, p < 0.001). Then, KEGG and GO analysis were performed on the above 533 coexpressed genes. We found that these coexpressed genes were significantly enriched in 739 GO terms and 45 KEGG pathways (p < 0.05), such as regulation of immune system process, leukocyte activation, T-cell activation, Toll-like receptor signaling pathway, Jak-STAT signaling pathway, nuclear factor (NF)-kappa B signaling pathway, suggesting the four mRNAs may influence the immune function of CM patients (top 30 are shown, Figure 5).
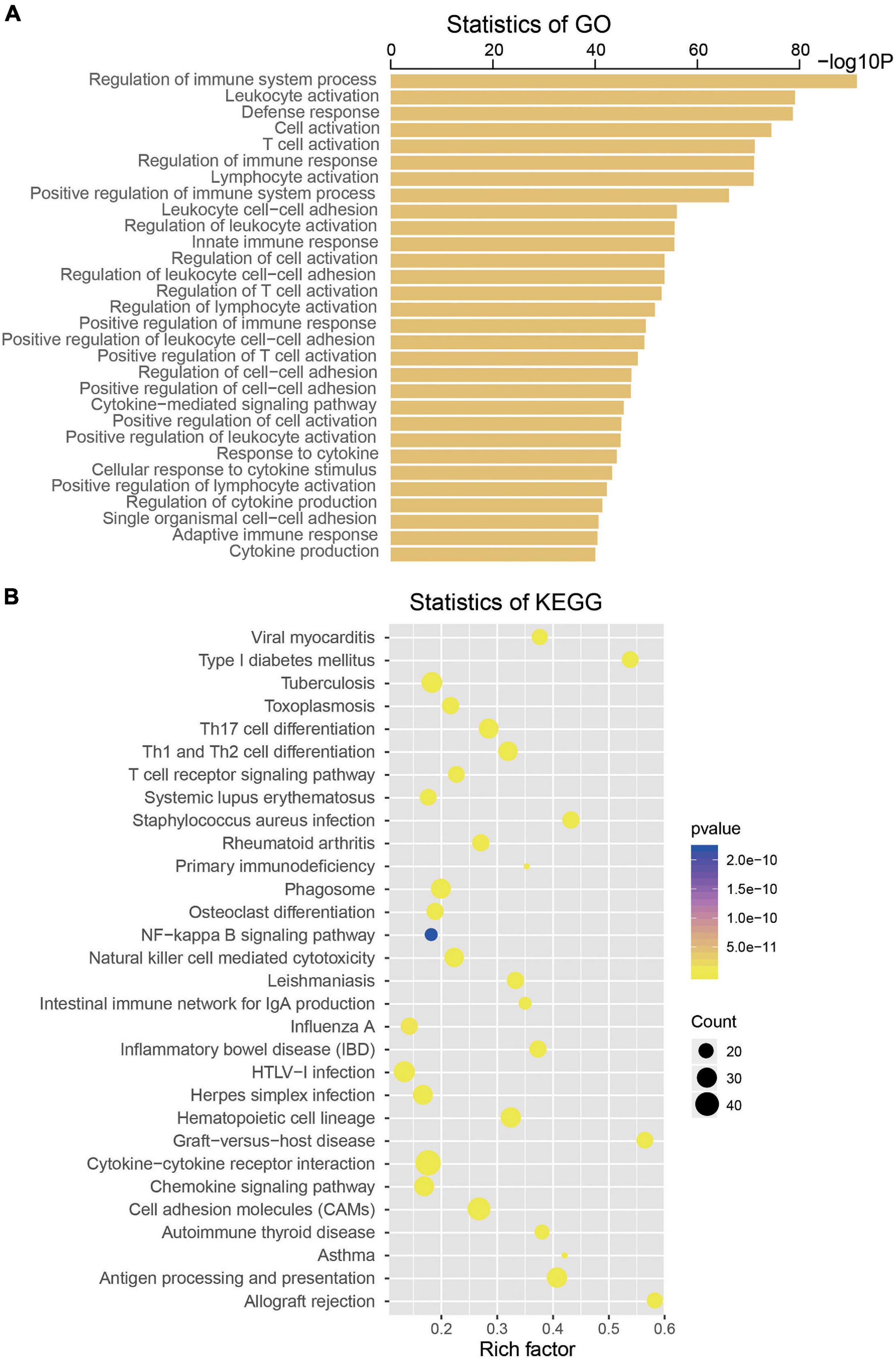
Figure 5. Functional enrichment analysis of the four messenger RNAs (mRNAs) in the signature by Gene Ontology (A) and Kyoto Encyclopedia of Genes and Genomes (B).
Discussion
Cutaneous melanoma is a highly malignant disease with large difference in prognosis, lacking effective biomarkers for accurate survival prediction or reliable prognostic indicators. The application of precision medicine in the field of oncology highlights the prediction of individual prognosis based on gene expression profiles. Through analyzing gene expression profiles, gene expression signatures have been used to predict the prognosis of patients in different types of cancer (Burska et al., 2014), such as glioblastoma, esophageal squamous cell carcinoma, breast cancer, lung cancer, hepatocellular carcinoma, and bladder carcinoma. Therefore, using statistics and machine learning approaches, we analyzed the clinical survival information and mRNA expression of 385 melanoma patients and developed the four-mRNA signature, which could be a good prognostic biomarker for patients with CM.
After performing a variety of bioinformatics analysis methods in the TCGA group, a prognostic risk model based on the expression of four mRNAs was established to distinguish CM patients with different prognosis. This model has two advantages in predicting the prognosis of CM patients: first, it is an independent prognostic biomarker, which means that known clinical predicators such as melanoma Clark level and tumor grade will not affect its prognosis prediction. Second, the AUC value of the four-mRNA signature is greater than that of melanoma Clark and tumor grade, indicating that the four-mRNA signature has the best survival predictive performance.
The high expression of CD276 and UQCRFS1 in the four-mRNA signature was associated with short OS (Cox regression coefficient > 0), suggesting that they were risk factors for prognostic. Meanwhile, the high expression of HAPLN3 and PIP4P1 were associated with long OS (Cox regression coefficient < 0), which indicates that these two genes were beneficial factors for prognosis. Among the candidate genes, CD276 (B7-H3) is an important component of the B7 family, which can provide stimulus or inhibitory signals to enhance or weaken T-cell immune response. CD276 at the mRNA level is widely expressed in normal tissues. In tumors, CD276 or B7-H3 is reported to be highly expressed in tumors of various tissue types including melanoma, which is closely related to the poor clinical outcome of tumor patients. Studies have shown that B7-H3 plays an important role in tumor immune escape and can also affect tumor proliferation, invasion, and migration (Castellanos et al., 2017; Dong et al., 2018). In accordance with the results of this article, researchers found that B7-H3 is a significant factor in tumor progression and poor prognosis for CM patients (Tekle et al., 2012; Wang et al., 2013). HAPLN3 is a member of the hyaluronan and proteoglycan binding link protein gene family with a length of 2.1 kb and is widely expressed in various tissues (Spicer et al., 2003). HAPLN3 has been reported to play an important role in maintaining the stability of the extracellular matrix, thereby regulating the mobility and migration of tumor cells. Kuo et al. (2010) found that HAPLN3 was significantly overexpressed in breast cancer tissues, but there was no correlation between HAPLN3 gene expression and overall survival. Ubiquinol cytochrome c reductase (UQCRFS1, also named as Rieske Fe–S protein) is a catalytic submit of complex III and plays an important role in the mitochondrial respiratory chain. Researchers have found that UQCRFS1 is overexpressed in ovarian carcinoma and gastric cancer and may promote tumor development (Kaneko et al., 2003; Jun et al., 2012). Phosphatidylinositol-4, 5-bisphosphate 4-phosphatase (PIP4P1, known as TMEM55B) is an enzyme that influences cholesterol homeostasis (Medina et al., 2014) and regulates lysosomal positioning (Willett et al., 2017). However, there is no report about the role of TMEM55B in tumors.
In recent years, the role of immunity and inflammation in tumor progression has been gradually discovered (Coussens and Werb, 2002; Keibel et al., 2009). To explore the function of genes in the four-mRNA signature, GO and KEGG analyses were performed and identified that these genes were enriched in several immune and inflammation-related pathways, such as regulation of immune system process, regulation of inflammatory response and Toll-like receptor signaling pathway, Jak-STAT signaling pathway, and NF-kappa B signaling pathway, which suggests that the four-mRNA signature might influence the survival of patients with CM through regulating immune and inflammation-related pathways (Pansky et al., 2000; Janostiak et al., 2017; Luo et al., 2018; Rathore et al., 2019).
In summary, our study developed a prognostic four-mRNA signature (CD276, HAPLN3, PIP4P1, UQCRFS1) for CM, which can predict the clinical outcome of patients. Since its prognostic ability is better than the current markers (Clark level or tumor stage), the four-mRNA signature would have stronger clinical application value.
Data Availability Statement
Publicly available datasets analyzed in this study can be found here: https://xenabrowser.net/datapages/?cohort=TCGA%20Melanoma%20(SKCM)&removeHub=https%3A%2F%2Fxena.treehouse.gi.ucsc.edu%3A443; https://www.ncbi.nlm.nih.gov/geo/query/acc.cgi?acc=GSE65904.
Author Contributions
JL designed the study. HB and YW performed all analyses. HL checked the final manuscript. All authors reviewed the manuscript and approved the manuscript for publication.
Conflict of Interest
The authors declare that the research was conducted in the absence of any commercial or financial relationships that could be construed as a potential conflict of interest.
Supplementary Material
The Supplementary Material for this article can be found online at: https://www.frontiersin.org/articles/10.3389/fgene.2021.680617/full#supplementary-material
Footnotes
References
Bindea, G., Mlecnik, B., Hackl, H., Charoentong, P., Tosolini, M., Kirilovsky, A., et al. (2009). ClueGO: a Cytoscape plug-in to decipher functionally grouped gene ontology and pathway annotation networks. Bioinformatics 25, 1091–1093. doi: 10.1093/bioinformatics/btp101
Burska, A. N., Roget, K., Blits, M., Soto Gomez, L., van de Loo, F., Hazelwood, L. D., et al. (2014). Gene expression analysis in RA: towards personalized medicine. Pharmacogenomics J. 14, 93–106. doi: 10.1038/tpj.2013.48
Castellanos, J. R., Purvis, I. J., Labak, C. M., Guda, M. R., Tsung, A. J., Velpula, K. K., et al. (2017). B7-H3 role in the immune landscape of cancer. Am. J. Clin. Exp. Immunol. 6, 66–75.
Coussens, L. M., and Werb, Z. (2002). Inflammation and cancer. Nature 420, 860–867. doi: 10.1038/nature01322
Dimitriou, F., Krattinger, R., Ramelyte, E., Barysch, M. J., Micaletto, S., Dummer, R., et al. (2018). The world of Melanoma: epidemiologic, genetic, and anatomic differences of Melanoma across the Globe. Curr. Oncol. Rep. 20:87. doi: 10.1007/s11912-018-0732-8
Dinnes, J., Deeks, J. J., Saleh, D., Chuchu, N., Bayliss, S. E., Patel, L., et al. (2018). Skin Cancer Diagnostic Test Accuracy, Reflectance confocal microscopy for diagnosing cutaneous melanoma in adults. Cochrane Database Syst. Rev. 12:CD013190. doi: 10.1002/14651858.CD013190
Dong, P., Xiong, Y., Yue, J., Hanley, S. J. B., and Watari, H. (2018). B7H3 As a Promoter of Metastasis and Promising Therapeutic Target. Front. Oncol. 8:264. doi: 10.3389/fonc.2018.00264
He, R., Zuo, S., and Robust, A. (2019). 8-Gene Prognostic Signature for Early-Stage Non-small Cell Lung Cancer. Front. Oncol. 9:693. doi: 10.3389/fonc.2019.00693
Janostiak, R., Rauniyar, N., Lam, T. T., Ou, J., Zhu, L. J., Green, M. R., et al. (2017). Growth by Stimulating the NF-kappaB Pathway. Cell Rep. 21, 2829–2841. doi: 10.1016/j.celrep.2017.11.033
Jun, K. H., Kim, S. Y., Yoon, J. H., Song, J. H., and Park, W. S. (2012). Amplification of the UQCRFS1 Gene in Gastric Cancers. J. Gastric Cancer 12, 73–80. doi: 10.5230/jgc.2012.12.2.73
Kaneko, S. J., Gerasimova, T., Smith, S. T., Lloyd, K. O., Suzumori, K., and Young, S. R. (2003). CA125 and UQCRFS1 FISH studies of ovarian carcinoma. Gynecol. Oncol. 90, 29–36. doi: 10.1016/S0090-8258(03)00144-6
Keibel, A., Singh, V., and Sharma, M. C. (2009). Inflammation, microenvironment, and the immune system in cancer progression. Curr. Pharm. Des. 15, 1949–1955. doi: 10.2174/138161209788453167
Kretschmer, L., Starz, H., Thoms, K. M., Satzger, I., Volker, B., Jung, K., et al. (2011). Age as a key factor influencing metastasizing patterns and disease-specific survival after sentinel lymph node biopsy for cutaneous melanoma. Int. J. Cancer 129, 1435–1442. doi: 10.1002/ijc.25747
Kuo, S. J., Chien, S. Y., Lin, C., Chan, S. E., Tsai, H. T., and Chen, D. R. (2010). Significant elevation of CLDN16 and HAPLN3 gene expression in human breast cancer. Oncol. Rep. 24, 759–766. doi: 10.3892/or_00000918
Li, J., Chen, Z., Tian, L., Zhou, C., He, M. Y., Gao, Y., et al. (2014). LncRNA profile study reveals a three-lncRNA signature associated with the survival of patients with oesophageal squamous cell carcinoma. Gut 63, 1700–1710. doi: 10.1136/gutjnl-2013-305806
Luo, N., Formisano, L., Gonzalez-Ericsson, P. I., Sanchez, V., Dean, P. T., Opalenik, S. R., et al. (2018). Melanoma response to anti-PD-L1 immunotherapy requires JAK1 signaling, but not JAK2. Oncoimmunology 7:e1438106. doi: 10.1080/2162402X.2018.1438106
Medina, M. W., Bauzon, F., Naidoo, D., Theusch, E., Stevens, K., Schilde, J., et al. (2014). Transmembrane protein 55B is a novel regulator of cellular cholesterol metabolism. Arterioscler. Thromb. Vasc. Biol. 34, 1917–1923. doi: 10.1161/ATVBAHA.113.302806
Miller, K. D., Nogueira, L., Mariotto, A. B., Rowland, J. H., Yabroff, K. R., Alfano, C. M., et al. (2019). Cancer treatment and survivorship statistics, 2019. CA Cancer J. Clin. 69, 363–385. doi: 10.3322/caac.21565
Morton, D. L., Davtyan, D. G., Wanek, L. A., Foshag, L. J., and Cochran, A. J. (1993). Multivariate analysis of the relationship between survival and the microstage of primary melanoma by Clark level and Breslow thickness. Cancer 71, 3737–3743. doi: 10.1002/1097-0142(19930601)71:11<3737::AID-CNCR2820711143>3.0.CO;2-7
Palmieri, G., Colombino, M., Casula, M., Manca, A., Mandala, M., Cossu, A., et al. (2018). Molecular pathways in Melanomagenesis: what we learned from Next-Generation sequencing approaches. Curr. Oncol. Rep. 20:86. doi: 10.1007/s11912-018-0733-7
Pan, Y., Zhang, J. H., Zhao, L., Guo, J. C., Wang, S., Zhao, Y., et al. (2020). A robust two-gene signature for glioblastoma survival prediction. J. Cell. Biochem. 121, 3593–3605. doi: 10.1002/jcb.29653
Panda, S., Dash, S., Besra, K., Samantaray, S., Pathy, P. C., and Rout, N. (2018). Clinicopathological study of malignant melanoma in a regional cancer center. Indian J. Cancer 55, 292–296. doi: 10.4103/ijc.IJC_612_17
Pansky, A., Hildebrand, P., Fasler-Kan, E., Baselgia, L., Ketterer, S., Beglinger, C., et al. (2000). Defective Jak-STAT signal transduction pathway in melanoma cells resistant to growth inhibition by interferon-alpha. Int. J. Cancer 85, 720–725. doi: 10.1002/(SICI)1097-0215(20000301)85:5<720::AID-IJC20>3.0.CO;2-O
Rathore, M., Girard, C., Ohanna, M., Tichet, M., Ben Jouira, R., Garcia, E., et al. (2019). Cancer cell-derived long pentraxin 3 (PTX3) promotes melanoma migration through a toll-like receptor 4 (TLR4)/NF-kappaB signaling pathway. Oncogene 38, 5873–5889. doi: 10.1038/s41388-019-0848-9
Schadendorf, D., van Akkooi, A. C. J., Berking, C., Griewank, K. G., Gutzmer, R., Hauschild, A., et al. (2018). Melanoma. Lancet 392, 971–984. doi: 10.1016/S0140-6736(18)31559-9
Shields, C. L., Kaliki, S., Furuta, M., Fulco, E., Alarcon, C., and Shields, J. A. (2015). American Joint Committee on Cancer Classification of Uveal Melanoma (Anatomic Stage) Predicts Prognosis in 7,731 Patients: the 2013 Zimmerman Lecture. Ophthalmology 122, 1180–1186. doi: 10.1016/j.ophtha.2015.01.026
Shields, C. L., Kaliki, S., Furuta, M., Mashayekhi, A., and Shields, J. A. (2012). Clinical spectrum and prognosis of uveal melanoma based on age at presentation in 8,033 cases. Retina 32, 1363–1372. doi: 10.1097/IAE.0b013e31824d09a8
Spicer, A. P., Joo, A., and Bowling, R. A. Jr. (2003). A hyaluronan binding link protein gene family whose members are physically linked adjacent to chondroitin sulfate proteoglycan core protein genes: the missing links. J. Biol. Chem. 278, 21083–21091. doi: 10.1074/jbc.M213100200
Tekle, C., Nygren, M. K., Chen, Y. W., Dybsjord, I., Nesland, J. M., Maelandsmo, G. M., et al. (2012). B7-H3 contributes to the metastatic capacity of melanoma cells by modulation of known metastasis-associated genes. Int. J. Cancer 130, 2282–2290. doi: 10.1002/ijc.26238
Wang, J., Chong, K. K., Nakamura, Y., Nguyen, L., Huang, S. K., Kuo, C., et al. (2013). B7-H3 associated with tumor progression and epigenetic regulatory activity in cutaneous melanoma. J. Investig. Dermatol. 133, 2050–2058. doi: 10.1038/jid.2013.114
Wang, J., Kong, P. F., Wang, H. Y., Song, D., Wu, W. Q., Zhou, H. C., et al. (2020). Identification of a Gene-Related Risk Signature in Melanoma Patients Using Bioinformatic Profiling. J. Oncol. 2020:7526204. doi: 10.1155/2020/7526204
Willett, R., Martina, J. A., Zewe, J. P., Wills, R., Hammond, G. R. V., and Puertollano, R. (2017). TFEB regulates lysosomal positioning by modulating TMEM55B expression and JIP4 recruitment to lysosomes. Nat. Commun. 8:1580. doi: 10.1038/s41467-017-01871-z
Wu, M., Li, X., Zhang, T., Liu, Z., and Zhao, Y. (2019). Identification of a Nine-Gene Signature and Establishment of a Prognostic Nomogram Predicting Overall Survival of Pancreatic Cancer. Front. Oncol. 9:996. doi: 10.3389/fonc.2019.00996
Xu, G., Zhang, M., Zhu, H., and Xu, J. (2017). A 15-gene signature for prediction of colon cancer recurrence and prognosis based on SVM. Gene 604, 33–40.
Keywords: cutaneous melanoma, prognostic signature, random survival forest, MRNA expression data, machine learning
Citation: Bai H, Wang Y, Liu H and Lu J (2021) Development of a Four-mRNA Expression-Based Prognostic Signature for Cutaneous Melanoma. Front. Genet. 12:680617. doi: 10.3389/fgene.2021.680617
Received: 15 March 2021; Accepted: 17 May 2021;
Published: 15 July 2021.
Edited by:
Lihong Peng, Hunan University of Technology, ChinaReviewed by:
Xinyi Liu, University of Illinois at Chicago, United StatesYuhua Yin, Shanghai Jiao Tong University, China
Copyright © 2021 Bai, Wang, Liu and Lu. This is an open-access article distributed under the terms of the Creative Commons Attribution License (CC BY). The use, distribution or reproduction in other forums is permitted, provided the original author(s) and the copyright owner(s) are credited and that the original publication in this journal is cited, in accordance with accepted academic practice. No use, distribution or reproduction is permitted which does not comply with these terms.
*Correspondence: Junyang Lu, lujy10@lzu.edu.cn
†These authors have contributed equally to this work