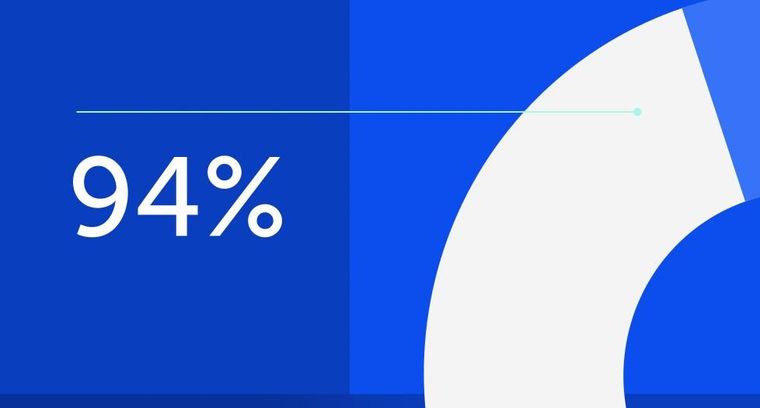
94% of researchers rate our articles as excellent or good
Learn more about the work of our research integrity team to safeguard the quality of each article we publish.
Find out more
REVIEW article
Front. Genet., 27 July 2021
Sec. Computational Genomics
Volume 12 - 2021 | https://doi.org/10.3389/fgene.2021.671057
This article is part of the Research TopicComputational Approaches for Biomarker Detection and Precision Therapeutics in CancersView all 21 articles
Detection of cellular changes in tissue biopsies has been the basis for cancer diagnostics. However, tissue biopsies are invasive and limited by inaccuracies due to sampling locations, restricted sampling frequency, and poor representation of tissue heterogeneity. Liquid biopsies are emerging as a complementary approach to traditional tissue biopsies to detect dynamic changes in specific cell populations. Cell-free DNA (cfDNA) fragments released into the circulation from dying cells can be traced back to the tissues and cell types they originated from using DNA methylation, an epigenetic regulatory mechanism that is highly cell-type specific. Decoding changes in the cellular origins of cfDNA over time can reveal altered host tissue homeostasis due to local cancer invasion and metastatic spread to distant organs as well as treatment responses. In addition to host-derived cfDNA, changes in cancer cells can be detected from cell-free, circulating tumor DNA (ctDNA) by monitoring DNA mutations carried by cancer cells. Here, we will discuss computational approaches to identify and validate robust biomarkers of changed tissue homeostasis using cell-free, methylated DNA in the circulation. We highlight studies performing genome-wide profiling of cfDNA methylation and those that combine genetic and epigenetic markers to further identify cell-type specific signatures. Finally, we discuss opportunities and current limitations of these approaches for implementation in clinical oncology.
Liquid biopsies are emerging as a minimally invasive approach to complement and potentially advance the traditional standards of care in oncology (Bronkhorst et al., 2019). Tissue biopsies are taken as part of routine clinical care for most solid cancers and used to identify the molecular determinants of disease that can inform both diagnosis and prognosis. However, tissue biopsies are invasive and limited by inaccuracies due to sampling locations, restricted sampling frequency, and poor representation of local tumor heterogeneity as well as dispersed cancerous lesions. To address these limitations, liquid biopsy technologies are rapidly advancing to provide analysis of tumors using circulating biomarkers in fluids such as the blood. One of the main advantages of liquid biopsies is its capacity for serial sampling by simple blood draws. The increased sampling frequency is helpful to monitor clonal evolution of tumor subpopulations as well as to assess evolutionary dynamics influencing treatment response and resistance as well as disease recurrence (Corcoran and Chabner, 2018). Also, liquid biopsies are capable of capturing systemic changes to provide an organism-wide picture of disease progression including the local primary tumor as well as distant metastatic sites and treatment responses across different sites. Finally, liquid biopsies are uniquely able to capture tumor heterogeneity over time, and thus complement traditional tissue biopsies that can only sample locally and at accessible sites (Figure 1).
Figure 1. Complementary role of tissue and liquid biopsies in oncology. Localized solid tissue biopsies are invasive and provide a snapshot of limited representational heterogeneity based on the small piece of tissue that is excised. In comparison, liquid biopsies are minimally invasive and allow for serial sampling to provide systemic information about the primary tumor as well as distant metastatic sites indicated in different colors. Thus, liquid biopsies complement tissue biopsies and increase representation of heterogeneity supporting the tracking of clonal evolution over time.
Similar to tissue biopsies, the major purpose of liquid biopsies in oncology is to identify circulating analytes that provide molecular information about the cancer. In this context, there are a multitude of molecules that may be isolated from biological fluids and targeted for analysis. Until recently, the main focus has been on circulating molecules that can be directly tied back to the primary tumor, including circulating tumor cells (CTCs), cell-free tumor DNA (ctDNA), tumor-educated platelets (TEPs), and tumor secreted vesicles (exosomes, oncosomes, apoptotic bodies) (Best et al., 2015; Rapisuwon et al., 2016). However, as comprehensive approaches gain traction, there has been an expansion to include molecules reflective of dynamic changes to the host, tumor microenvironment and distant metastatic sites as well. Both tumor cells and normal host-derived cells release cell-free DNA (cfDNA) into the circulation as a result of physiological processes. cfDNA is thought to originate from the genomes of dying cells, including cells within tumors, and is reflective of cell turnover rates at steady state as well as altered homeostasis throughout the body with disease (Kustanovich et al., 2019; Heitzer et al., 2020; Rostami et al., 2020). Thus, circulating tumor DNA (ctDNA) is a subset of cfDNA that has different biological characteristics (Table 1). There is still much to be learned about the biology of cfDNA release, distribution, and elimination mechanisms leading to differential stability and circulation half-life in healthy compared to diseased states (Jiang and Lo, 2016; Heitzer and Speicher, 2018; Sanchez et al., 2018; Serpas et al., 2018; Han et al., 2020; Barefoot et al., 2021). The focus of this review will be on methylated cell-free DNA and its utility and applications in cancer diagnosis and management.
There are still many challenges to overcome before liquid biopsies may be routinely implemented in the clinic. Signal abundance (fraction of target cfDNA relative to total cfDNA), sequencing depth, and breadth of genomic regions assayed by sequencing are factors that must be considered to detect signals in the circulation of cancer patients relevant to inform care (Figure 2B; Im et al., 2020). Strategies aimed at increasing any of these factors will improve the odds that informative signals can be detected. Signal abundance is largely a byproduct of the biology of the disease in question and therefore little can be done to modify this variable (Heitzer et al., 2019). For instance, ctDNA is highly correlated with tumor burden, with larger amounts of ctDNA found in the circulation of individuals at advanced stages of tumor progression. For this reason, mutation analysis of ctDNA is limited in its capacity to detect cancer-related signals, especially with low-volume tumors at early stage and relapse (Im et al., 2020). However, signal abundance can be increased by leveraging signals from all cfDNA molecules rather than the smaller subset of fragments containing specific tumor-related mutations (Figures 2A,C). This can be accomplished by targeting tumor-specific epigenetic changes that occur early on during carcinogenesis and thus are found at higher abundance in early stage cancers than tumor-related mutations (Snyder et al., 2016; Ulz et al., 2016; Wong et al., 2016; Leygo et al., 2017; Jiang et al., 2018, 2019; Cristiano et al., 2019; Gai and Sun, 2019; Ivanov et al., 2019; Panagopoulou et al., 2019; Sun et al., 2019; Van der pol and Mouliere, 2019; Sadeh et al., 2021). Further, combining tumor-cell derived signals with those from the surrounding host microenvironment can increase signal abundance (Hoadley et al., 2018; Liu et al., 2018; Haigis et al., 2019; Lam et al., 2019). Tumor DNA identified by genetic or epigenetic markers circulates admixed with non-tumor DNA. The same DNA sequence is found in all non-tumor cells and simple sequence analysis cannot be used to distinguish its cell-type origin. However, covalent and non-covalent epigenetic marks are pivotal to cell-type identity and can be used to distinguish tumor as well as non-tumor DNA, expanding the reach of molecules targeted to reflect disease-pertinent changes (Barefoot et al., 2021).
Figure 2. Factors contributing to probability of signal detection. (A) Tumors release mutant genomic and epigenomic cfDNA into the circulation. Normal cell types from the surrounding microenvironment and other somatic cells also release cfDNA into the circulation that can be identified through cell-type specific epigenetic markers. Combining tumor cell- and normal host cell-derived signals can increase target abundance in the circulation to increase sensitivity, while maintaining specificity. (B) Target abundance, sequencing depth, and breadth of genomic regions assayed are factors that determine signal detection probability in the circulation of cancer patients. (C) Relative abundance of cfDNA populations and associated specificity.
Despite its highly fragmented nature, advances in sequencing technologies have made comprehensive profiling of low integrity cfDNA possible. At a fixed target abundance and coverage, detection probabilities can be increased by broader sequencing, increasing the number of potential markers assayed (Im et al., 2020). Genomic analysis of ctDNA has decreased sensitivity relative to epigenetic approaches because of lower abundance at any one given marker (Leygo et al., 2017). Increasing the number of potential mutations assayed with whole-genome Next-Generation-Sequencing (NGS) applications has been shown to increase sensitivity, but there can still be a lack of sufficient markers when limited to tumor-specific mutations alone (Im et al., 2020). Comprehensive epigenetic profiling of tumor and non-tumor cfDNA has led to advances in detection of brain cancers, including gliomas, which “hide” behind the blood-brain barrier and have restricted access to release ctDNA into the circulation (Li et al., 2020; Nassiri et al., 2020). In these cases, integration across multiple markers allows for unparalleled sensitivity that cannot be achieved from low numbers of select targeted loci, despite high specificity and deep sequencing. Detection methods trending toward broader sequencing have been termed “third-generation” liquid biopsies and are emerging to allow for more comprehensive assessment of a multitude of signals.
Comprehensive sequencing approaches have unleashed the potential of liquid biopsies to achieve optimal sensitivity; however, there is still a need to improve the specificity and biological relevance of these assays. With the transition from targeted to comprehensive approaches come decreasing signal-to-noise ratios and new challenges to separate true biological signals from background sources of error (Ko et al., 2018). Physiological flux due to clonal hematopoiesis, inflammation, exercise, and other biological factors may dilute out relevant signals and calls for an increased understanding of the mechanisms of cell-free DNA release into the circulation and the distinct processing (Kustanovich et al., 2019; Barefoot et al., 2021). The predominating hematopoietic origins of cfDNA in healthy individuals makes it essential to identify markers separating cell-types of interest from peripheral immune cells (Barefoot et al., 2021). Machine-learning algorithms and data-science-driven approaches are being developed in tandem to reduce dimensionality and make sense of the data available to identify applicable information that may better inform clinical courses of action (Ko et al., 2018). As these approaches become increasingly complex, prior knowledge about the relevance of the features selected will be imperative to maintain biological interpretability.
DNA methylation functions as an epigenetic regulatory mechanism and involves covalent addition of a methyl-group to the 5-carbon of cytosine (5mc). DNA methylation occurs most commonly in the context of CpG dinucleotides (Greenberg and Bourc’his, 2019). One of the main benefits to harnessing cell-free methylated DNA for liquid biopsy applications in cancer is the potential to exploit prior knowledge about the biological relevance of these marks. DNA methylation is an intrinsic mark of cell identity and pathologic alterations of DNA methylation are hallmarks of cancer (Greenberg and Bourc’his, 2019). The DNA methylation landscape changes in a highly regulated manner throughout development. Before embryo implantation, there is a global erasure of DNA methylation that is reset in multiple stages leading to the creation of cell-type specific methylation patterns, paralleling ongoing cell differentiation and organogenesis (Dor and Cedar, 2018). Once established, this pattern of DNA methylation is highly stable and conserved across DNA replication, making DNA methylation the predominant mechanism for inherited cellular memory during cell growth (Daniūnaitė et al., 2019). DNA methylation patterns may be selected as features that are relatively hyper- or hypo-methylated in specific cell types or in the context of specific cancers. Therefore, while there has been extensive characterization of DNA methylation changes that occur with disease and physiological aging, these changes occur only at specific locations throughout the epigenome allowing methylation states at regions critical to cell-type identity to remain constant over time (Michalak et al., 2019). This stability allows methylated cfDNA to serve as a robust biomarker in the face of patient heterogeneity, capable of being generalized across diverse patient populations (Dor and Cedar, 2018). There are many areas where liquid biopsies can be applied in clinical oncology. These include, but are not limited to, efforts aimed at early detection, assessment of prognosis, detection of minimal residual disease, metastasis, targeted-therapy selection, and treatment response monitoring (Sina et al., 2019; Luo et al., 2021). Both cancer and cell-type specific cell-free DNA methylation markers have been employed in each of these applications; however, there are important distinctions based on using disease-specific or normal cell-type specific markers that are worth noting. Specifically, cell-type specific DNA methylation markers have unique applications to localize cancers of unknown primary (CUP) as well as to detect metastases (Figure 3; Gai et al., 2018; Moss et al., 2018). In addition, systemic therapy-related adverse event monitoring remains one of the most promising applications.
Figure 3. Applications for detection and localization of metastasis and Cancer of Unknown Primary (CUP). (A) CfDNA in healthy individuals is mostly of hematopoietic origin. (B) The composition of cell-free DNA changes with disease. In this example, primary lung cancer results in increased levels of ctDNA identified by tumor-specific genomic and epigenomic markers, as well as increased levels of cfDNA from the surrounding lung microenvironment identified by normal cell-specific epigenetic markers. (C) Genomic mutations occur independently in primary and metastatic tumor sites. Liquid biopsies are capable of capturing this heterogeneity; however, mutations alone cannot localize these clonal populations to their tissue origins at the primary tumor site and distant metastatic site. As a complementary approach, normal tissue- and cell-type epigenetic markers can be used for detection and localization of metastasis and Cancers of Unknown Primary (CUP).
There are many techniques that can be used to study DNA methylation as well as different strategies that can be applied to classify and quantify methylation status (Olkhov-Mitsel and Bapat, 2012; Kurdyukov and Bullock, 2016; Galardi et al., 2020; Zhao et al., 2020). These methodologies must be able to distinguish between methylated and unmethylated cytosines. This review mainly focuses on 5mc as it is the most commonly characterized epigenetic mark in cancer. However, other DNA modifications, including 5-hydroxymethylcytosine (5hmc), are thought to be more dynamic, reflecting active demethylation events, and may be complementary to characterize as well (Song et al., 2017). The different DNA methylation detection technologies and platforms are categorized in Figure 4. The main methods are restriction enzyme digestion, affinity enrichment, bisulfite-conversion, and enzymatic modification approaches. To date, several of these approaches have been successfully implemented to study genome-wide cfDNA methylation, highlighted in Table 2. Restriction enzyme-based methods cleave DNA at enzyme specific CpG sites. However, the highly fragmented nature of cfDNA and limited frequency of CpG-containing recognition sites make this approach challenging for comprehensive profiling of cfDNA (Huang and Wang, 2019). cfMeDIP-seq is an affinity-based approach that enriches for methylated DNA using 5mc-specific antibodies (Shen et al., 2018). As such, it is capable of characterizing overall methylation levels across a region, but not at single CpG sites. In addition, the majority of cell-type specific methylation markers in the human body are hypomethylated as a result of methylation resetting that takes place throughout tissue differentiation and development. These methods that specifically enrich for hypermethylated DNA may have limited detection potential at these regions of interest.
Figure 4. DNA methylation technologies and platforms for signal detection. (A) Scale representation of DNA methylation technologies from targeted First- and Second-Generation toward comprehensive Third-Generation applications in liquid biopsies. (B) Methods for detection of DNA methylation. The same DNA sequence is found in all non-tumor cells, and simple sequence analysis cannot be used to distinguish its cell-type identity. However, these methods can be used to detect DNA methylation (5mc) and DNA hydroxymethylation (5hmc) levels in tumor and non-tumor cells. MeDIP-seq, Methylated DNA immunoprecipitation sequencing; MBD, methyl-CpG-binding domain sequencing; WGBS, Whole Genome Bisulfite Sequencing; BSAS, Bisulfite Amplicon Sequencing; RRBS, Reduced Representation Bisulfite Sequencing; MCTA-seq, methylated CpG tandem amplification and sequencing; MSP, Methylation Specific PCR; MRE-seq, methylation-sensitive restriction enzyme sequencing; HELP, Hpall-tiny fragment enrichment by ligation-mediated PCR; MSCC, Methyl-sensitive Cut Counting; EM-seq, Enzymatic Methyl-Sequencing; TAPS, TET-assisted pyridine borane sequencing; TAB-seq, TET-assisted bisulfite sequencing; ACE-seq, APOBEC-coupled epigenetic sequencing; hmc-CATCH, chemical-assistant C-to-T conversion of 5hmC sequencing; oxBS-seq, oxidative bisulfite sequencing.
Table 2. Feasibility of tissue-of-origin analysis in oncology using cell-free DNA methylation markers.
Bisulfite conversion chemically modifies DNA so that unmethylated cytosines (C) are deaminated to uracil (U) to be later replaced by thymine (T) via PCR, while unmethylated cytosines are protected and remain cytosine (C) (Olova et al., 2018). The majority of comprehensive cfDNA methylation profiling has been done using bisulfite conversion methods, including Whole Genome Bisulfite Sequencing (WGBS), Reduced Representation Bisulfite Sequencing (RRBS), Methylated CpG Tandem Amplification and Sequencing (MCTA-seq), and Methylation Arrays. WGBS and RRBS are capable of detecting DNA methylation at single-base resolution. More importantly, these methods are capable of detecting read-specific DNA methylation patterns (Scott et al., 2020). WGBS is the most comprehensive approach, but it can be costly to sequence the whole genome to an informative depth. However, sequencing costs are decreasing, making this approach more attractive. RRBS has been optimized in a few instances for accommodating highly fragmented cfDNA molecules (Guo et al., 2017; De Koker et al., 2019). Despite these modifications, the use of restriction enzymes in this sequencing approach give rise to the same limitations as restriction-enzyme based methods. MCTA-seq uses primers to preferentially amplify methylated CpG islands (CpG tandem regions) and, while being more targeted, this approach is also biased toward hypermethylated regions (Liu X. et al., 2019). Methylation hybridization arrays allow for single-base resolution but do not allow for pattern analysis of multiple CpG sites from the same molecule and have reduced genome-wide coverage of CpG sites compared to NGS approaches (Moss et al., 2018).
While bisulfite conversion has long been considered the gold standard of methylation detection, there are major limitations that recent advances in enzymatic approaches show promise in overcoming (Schutsky et al., 2018; Liu Y. et al., 2019). For instance, sodium bisulfite is a harsh chemical treatment that causes unwanted DNA degradation and fragmentation, resulting in uneven genome coverage. Enzymatic Methyl-seq (EM-seq) uses the enzyme APOBEC to deaminate unmethylated cytosines and protects methylated cytosines from conversion by utilizing TET2 as an oxidative enhancer (Vaisvila et al., 2019). This results in the same base conversions as bisulfite sequencing, but this method has been shown to cause less DNA damage and as a result is more sensitive, requiring smaller amounts of input DNA. This method is used in a recent publication to profile cytosine methylation and nucleosome occupancy at the same time, a feat made possible from retention of the original cfDNA structure without fragmentation or degradation (Erger et al., 2020).
The nuances of these different methodologies to detect DNA methylation make choosing the right method and accounting for its limitations essential toward accurate interpretation of results. Methylation detection technologies are rapidly evolving, leading to expanded potential applications. For instance, one such advancement involves the direct detection of methylation without treatment of DNA, possible with nanopore-sequencing from Oxford Nanopore Technologies (ONT) and single molecule real-time (SMRT) sequencing from Pacific Biosciences (PacBio) (Flusberg et al., 2010; Liu Q. et al., 2019; Ewing et al., 2020; Yuen et al., 2020; Tse et al., 2021). Although direct detection of methylation is not currently possible with cfDNA inputs, these advances point toward new possibilities in the future.
Tissue-of-origin (TOO) analysis takes each individual cell-free DNA molecule in the circulation and routes it back to its tissue and cellular origins as a non-invasive monitoring tool for tissue damages (Figure 5). At steady state, cfDNA is released into the circulation reflective of cellular turnover happening throughout the human body, resulting in a complex mixture of fragments (Barefoot et al., 2021). On average, the plasma from healthy individuals has 1,500 genome equivalents or roughly 10 ng/mL cfDNA concentration (Moss et al., 2018). With cancer, cfDNA levels are thought to increase in parallel with disease progression as a result of increased proliferation and death rates of tumor cells (Kustanovich et al., 2019). However, relying on concentration of cfDNA alone to diagnose disease is too simplistic of an approach, as concentration is not an absolute indicator of disease and changes can result from a plethora of factors, including exercise, inflammation, and induction of cellular senescence. Detection of changing cell-type proportions from alterations in cfDNA composition is a more reliable approach. Shifting cfDNA makeup has been used for monitoring altered death rates of cells in different tissues, applicable to a broad spectrum of physiological and pathological conditions as well as therapeutic interventions. These include non-invasive prenatal testing, solid organ transplant, cancer, neurodegenerative and autoimmune pathologies, among many others (Sun et al., 2015; Zemmour et al., 2018; Chatterton et al., 2019; Cheng A. P. et al., 2019). To demonstrate feasibility, DNA methylation patterns specific to a variety of epithelial, endothelial, nervous, stromal, muscle, fat, and immune cell-types have been discovered and successfully applied using TOO analysis of cfDNA (Lehmann-Werman et al., 2016, 2018; Moss et al., 2018). In addition to tumor-derived DNA, changes to the host microenvironment can contribute to altered cell-type proportions of cfDNA in the circulation through cancer-related changes to normal tissue architecture. Although normal cell-specific DNA methylation markers are used, relevance to disease is inferred through abnormal detection in the circulation as a result of aberrant cell death and tissue damages (Heitzer et al., 2020). Thus, the changing proportion of normal cell types found in the circulation can be used to inform about disease states (Houseman et al., 2012; Teschendorff et al., 2017; Zheng et al., 2018; Huang et al., 2019; Barefoot et al., 2021). Recent studies demonstrating the feasibility of using cell-type specific cfDNA methylation marks for TOO analysis in cancer are described below (Table 2). This methodology is useful to detect damage to specific cell types in tissues and has many applications to inform diagnostics in the clinic as well as to reveal complexities of cancer pathophysiology at the cellular level.
Figure 5. Tissue-of-origin deconvolution analysis. CfDNA is a mixture of fragments released from healthy and diseased cells in different tissue types throughout the human body into the circulation. DNA methylation is highly cell-type specific and can be used to identify the cellular origins of cfDNA at specific markers. Tissue-of-origin (TOO) analysis traces cfDNA molecules back to the tissues and cell types they originated from and use changing tissue proportions to reveal altered tissue homeostasis in diseased states or during therapy.
Advances in liquid biopsy technology have led to the generation of massive amounts of methylation sequencing data that can be difficult to analyze due to the extensive number of possible features in the human methylome. Computational methods, including many machine learning techniques, have been developed to better handle such data by isolating specific signals and discriminative features, thereby reducing the dimensions of the data so that it is easier to interpret (Ko et al., 2018). In this dimension reduction approach, the data is projected into lower-dimensional spaces, ultimately with the aim to improve prediction accuracy through increasing the signal-to-noise ratio. As previously described, the total makeup of cfDNA can be modeled as a complex mixture with TOO deconvolution analysis aiming to trace each individual cfDNA molecule back to its cellular origins as a non-invasive measure of tissue damage.
There are many computational methods that have been successfully applied to facilitate TOO deconvolution of cfDNA (Table 2). These include reference-based supervised learning models, which utilize labeled training and test datasets for classification tasks (Teschendorff et al., 2017). Commonly used methods include linear or logistic regression and random forests. In addition, one study uses Particle Swarm Optimization as a supervised global optimization method. While global optimization methods may be supervised, semi-supervised, or unsupervised, in this case the method is applied as a supervised learning model (Liu Y. et al., 2019). There are also several unsupervised learning models, including clustering and density estimation methods, in which the goal is to learn the inherent structure and relations of unlabeled data (Houseman et al., 2016). One advantage of unsupervised, reference-free algorithms is the ability to estimate contributions from unknown cell types, or cell types for which reference methylation data is not available. However, the biological meaning of the features selected in these models is often lost or difficult to interpret, making it more challenging to explain the relevance of results. Recently, deep learning has also been applied as a powerful modeling technique for deconvolution of DNA methylation data as these methods perform simultaneous feature extraction and classification (Levy et al., 2020; Menden et al., 2020). As a high-level overview, the computational methods for cell-mixture deconvolution can be generalized as adhering to the following format that is consistent across liquid biopsy applications. Initially, features are selected or extracted that can characterize variation among cell-type contributors in the circulation. Then, statistical models are built to estimate the mixing proportions of each cell type based on the reduced number of discriminative DNA methylation features selected. Typically, these models are trained using reference data where the mixing proportions are already known and then tested on datasets where the mixing proportions are unknown for evaluation (Feng et al., 2019). As a final step, predictive models can be developed after deconvolution, using the inferred cell-mixture proportions as predictors to estimate disease phenotypes.
Despite demonstrated success applying these computational models to cfDNA methylation deconvolution, these algorithms were originally designed to be learned from very large training datasets (Ko et al., 2018). In order to maintain predictive capabilities, modifications are necessary to optimize these models for working with smaller and often more diverse datasets, typical to the field of liquid biopsy. With this in mind, there are important biological properties of cell-free DNA methylation that can be leveraged toward this goal. First, in comparison to DNA in tissues that is artificially sheared for introduction to standard library preparation methods, the fragmentation patterns of cfDNA are biologically derived (Lo et al., 2021). The majority of cfDNA fragments are ∼167 bp, representing the length of DNA wrapped around a nucleosome and reflective of degradation by nucleases as a by-product of cell death. This fragmented nature of cfDNA lends itself to methods developed for characterizing cfDNA at the level of single molecules as opposed to population-level averages at single CpG sites (Li et al., 2018). Read-specific analysis allows for each read-pair to be modeled as an independent sample reflective of each individual cfDNA molecule. This allows the depth of sequencing to be utilized toward increasing sample size (Scott et al., 2020). In addition, the density of neighboring CpG sites varies across the human genome with highly dense organization defined as CpG islands. Methylation status at adjacent CpG sites is co-regulated in CpG islands due to the expanse of methylating and demethylating enzymes acting in the area (Marzese and Hoon, 2015). This co-dependency can be leveraged to increase specificity through modeling the methylation features selected with pattern analysis. DNA methylation detection technologies and computational approaches that take advantage of pattern analysis of individual cfDNA molecules have demonstrated to be more robust and to increase the sensitivity and specificity of cell-type proportion estimations (Lehmann-Werman et al., 2016; Guo et al., 2017; Li et al., 2018; Zemmour et al., 2018; Chatterton et al., 2019). Overall, the biological relevance of selected DNA methylation markers and derived tissue proportions to disease can be utilized to inform analysis and model optimization.
There is a need to identify predictive biomarkers for real-time monitoring of therapy-related adverse events relative to therapeutic efficacy. Combining changes to mutant ctDNA with altered proportions of cell-type specific cfDNA can reflect intervention-based changes (Figure 6; Erger et al., 2020; Guo et al., 2020; Wu et al., 2020). Therapy regimens for many cancers involve surgery, chemotherapy, radiotherapy, targeted therapy, and immunotherapy (Hofman et al., 2019). Each of these interventions can have a different systemic effect, and the ability to distinguish different cell types participating and potentially contributing to toxicities with cfDNA in serially drawn blood samples could significantly impact therapeutic decision making. Although imaging modalities can be used as an indirect way to gauge therapeutic efficacy, these results are often unreliable and difficult to interpret. Imaging results can be clouded by depictions of pseudoprogression, making them ineffective or crude instruments to monitor for concurrent changes necessary to guide therapy decisions (Maia et al., 2020). In contrast, the half-life of cfDNA is between 15 min and 2 h (Khier and Lohan, 2018). The rapid clearance allows for serial analysis of disease evolution over time, especially under selective pressures from ongoing therapy (O’Leary et al., 2018; Oellerich et al., 2019; Nabet et al., 2020; Peter et al., 2020). This technology allows for serial sampling to include a baseline comparison from which therapy-related relative changes may be assessed, taking into account patient specific co-morbidities at an individualized level.
Figure 6. Predicting treatment response and therapy-related toxicities from combined genetic and epigenetic analyses of cfDNA. The minimally invasive nature of liquid biopsies allows for serial sampling to monitor changes over time, especially under selective pressures from ongoing therapy. CtDNA can be used to track clonal heterogeneity over time to assess treatment response and detect treatment-resistant clones. Normal cell-specific cfDNA methylation patterns can be used in combination with ctDNA to assess the impact of treatment to the surrounding tumor microenvironment and to monitor for therapy-related toxicities in somatic cell-types. Acronyms: ctDNA, (circulating tumor DNA); cme-DNA, (circulating methylated cell-free DNA).
Combining genetic and epigenetic analyses of cell-free DNA has many unique advantages when applied to precision therapeutics in cancer (Cheng T. H. et al., 2019; Zhang et al., 2019). Liquid biopsies have been shown to accurately characterize tumor genotypes and allow for molecular subtype classification to provide a comprehensive view of intratumor heterogeneity (Cohen et al., 2018; Christensen et al., 2019; Heitzer et al., 2019). High sampling frequency allows for modeling of evolutionary dynamics of tumor progression. Also, molecular changes identified after initiation of therapy can provide insight into therapy response as well as track tumor subclones that may lead to emergence of therapy resistance (Ahronian and Corcoran, 2017; Zhou et al., 2020). The systemic view provided by serial liquid biopsies is ideal to monitor widespread changes that may better inform clinical decision making in the face of uncertainty. For example, in the case of surgical removal of the tumor or therapeutic success, liquid biopsies can be used to monitor for minimal residual disease and recurrence. While ctDNA can be used to track molecular changes in the circulation, there is a benefit to monitoring the cancer-related changes to the host microenvironment in tandem requiring a combined genetic and epigenetic analysis. Cell-specific cfDNA methylation patterns of normal cells can be used in combination with ctDNA to assess the impact of treatment also on the surrounding tumor microenvironment. This is particularly useful to surveil for metastatic disease in distant tissue types from the primary tumor as well as to monitor for therapy-related toxicities in somatic cell types (Zhang et al., 2019). Further, liquid biopsies can help delineate factors that underlie clinical outcomes, providing a basis for recommending different treatments based on anticipated benefit to the patient. Liquid biopsies can identify predictive biomarkers to guide selection of treatment, recognize off-target effects, and develop individualized treatment plans for patients (Hofman et al., 2019). These applications provide a more complete picture of therapeutic response as well as tissue-specific cellular toxicity to better inform clinical care and management throughout the treatment process.
Liquid biopsies are rapidly emerging as an alternative and complementary approach to traditional solid tissue biopsies and have high utility for many applications in clinical oncology. Technology advances have made genome-wide profiling of circulating analytes possible and allow for transition from targeted to comprehensive approaches. With transition to “third-generation” liquid biopsies, DNA methylation patterns can be used to leverage signals from both tumor and non-tumor cells to increase signal abundance and discern biological relevance (Im et al., 2020). Despite great potential, comprehensive applications of liquid biopsy in oncology are still in their infancy. Additional large-scale, stratified, and randomized longitudinal studies are needed to begin to understand the complex interactions and biological significance of the comprehensive data identified from NGS technologies. Future work aimed at elucidating the biology of cell-free DNA release is needed to begin to control for co-morbidities and other confounding variables. Efforts aimed at assessing the effect of therapy regimens (chemo, radiation, immunotherapy, etc.) on tumor and non-tumor signals will become essential to determine what signals can be derived from the circulation. Tissue-of-origin analysis can be used to localize signals and generation of cell-type specific reference methylomes can improve specificity of features selected for application of TOO analysis in cancer (Moss et al., 2018; Barefoot et al., 2021). In addition, combining genetic and epigenetic markers may improve targeted-therapy selection and treatment response monitoring. These approaches are potentially synergistic, and future integration of signals across multiple genetic and epigenetic omics levels could fine-tune these applications for optimal use in precision oncology.
MB and AW wrote the first draft of the manuscript. All authors contributed to manuscript revision, read, and approved the submitted version.
Supported in part by funding from the National Institutes of Health United States: T32 CA009686 (MB), F30 CA250307 (MB), R01 CA231291 (AW), and P30 CA51008 (AW).
Georgetown University filed a patent related to some of the approaches described in this manuscript. MB and AW are named as inventors on this application and declare that as a potential conflict of interest.
The remaining authors declare that the research was conducted in the absence of any commercial or financial relationships that could be construed as a potential conflict of interest.
All claims expressed in this article are solely those of the authors and do not necessarily represent those of their affiliated organizations, or those of the publisher, the editors and the reviewers. Any product that may be evaluated in this article, or claim that may be made by its manufacturer, is not guaranteed or endorsed by the publisher.
Valuable discussions and suggestions were contributed by Anne Deslattes Mays (Science and Technology Consulting LLC and Georgetown University), Habtom Ressom, Michael R. Lindberg, and Marcel O. Schmidt (all at Georgetown University). All figures in this manuscript were created with BioRender.com.
Ahronian, L. G., and Corcoran, R. B. (2017). Strategies for monitoring and combating resistance to combination kinase inhibitors for cancer therapy. Genome Med. 9:37. doi: 10.1186/s13073-017-0431-3
Barefoot, M. E., Lindberg, M. R., and Wellstein, A. (2021). Decoding the tissue of origin of cellular damage from cell-free dna in liquid biopsies. Syst. Med. 2, 365–378. doi: 10.1016/b978-0-12-801238-3.11669-1
Best, M., Sol, N., Kooi, I., Tannous, J., Westerman, B., Rustenburg, F., et al. (2015). RNA-seq of tumor-educated platelets enables blood-based pan-cancer, multiclass, and molecular pathway cancer diagnostics. Cancer Cell 28, 666–676. doi: 10.1016/j.ccell.2015.09.018
Bronkhorst, A. J., Ungerer, V., and Holdenrieder, S. (2019). The emerging role of cell-free DNA as a molecular marker for cancer management. Biomol. Detect. Quantif. 17:100087. doi: 10.1016/j.bdq.2019.100087
Caggiano, C., Celona, B., Garton, F., Mefford, J., Black, B., Lomen-Hoerth, C., et al. (2020). Estimating the rate of cell type degeneration from epigenetic sequencing of cell-free DNA. bioRxiv [Preprint]. doi: 10.1101/2020.01.15.907022
Chatterton, Z., Mendelev, N., Chen, S., Raj, T., Walker, R., Carr, W., et al. (2019). Brain-derived circulating cell-free DNA defines the brain region and cell specific origins associated with neuronal atrophy. bioRxiv [Preprint]. doi: 10.1101/538827
Cheng, A. P., Burnham, P., Lee, J. R., Cheng, M. P., Suthanthiran, M., Dadhania, D., et al. (2019). A cell-free DNA metagenomic sequencing assay that integrates the host injury response to infection. Proc. Natl. Acad. Sci. U.S.A. 116, 18738–18744. doi: 10.1073/pnas.1906320116
Cheng, A. P., Cheng, M. P., Gu, W., Sesing Lenz, J., Hsu, E., Schurr, E., et al. (2021). Cell-free DNA tissues of origin by methylation profiling reveals significant cell, tissue, and organ-specific injury related to COVID-19 severity. Medicine 2, 411.e5–422.e5. doi: 10.1016/j.medj.2021.01.001
Cheng, A. P., Cheng, M. P., Lenz, J. S., Chen, K., Burnham, P., Timblin, K. M., et al. (2020). Cell-free DNA Tissues-of-origin profiling to predict graft versus host disease and detect infection after hematopoietic cell transplantation. bioRxiv [Preprint]. doi: 10.1101/2020.04.25.061580
Cheng, T. H., Jiang, P., Tam, J. C., Sun, X., Lee, W., Yu, S. C., et al. (2017). Genomewide bisulfite sequencing reveals the origin and time-dependent fragmentation of urinary cfdna. Clin. Biochem. 50, 496–501. doi: 10.1016/j.clinbiochem.2017.02.017
Cheng, T. H., Jiang, P., Teoh, J. Y., Heung, M. M., Tam, J. C., Sun, X., et al. (2019). Noninvasive detection of bladder cancer by shallow-depth Genome-Wide Bisulfite sequencing of urinary Cell-Free DNA For methylation and copy number profiling. Clin. Chem. 65, 927–936. doi: 10.1373/clinchem.2018.301341
Christensen, E., Birkenkamp-Demtröder, K., Sethi, H., Shchegrova, S., Salari, R., Nordentoft, I., et al. (2019). Early detection of metastatic relapse and monitoring of therapeutic efficacy by ultra-deep sequencing of plasma cell-free DNA in patients with urothelial bladder carcinoma. J. Clin. Oncol. 37, 1547–1557. doi: 10.1200/jco.18.02052
Cohen, J. D., Li, L., Wang, Y., Thoburn, C., Afsari, B., Danilova, L., et al. (2018). Detection and localization of surgically resectable cancers with a multi-analyte blood test. Science 359, 926–930. doi: 10.1126/science.aar3247
Corcoran, R. B., and Chabner, B. A. (2018). Application of cell-free DNA analysis to cancer treatment. N. Engl. J. Med. 379, 1754–1765. doi: 10.1056/nejmra1706174
Cristiano, S., Leal, A., Phallen, J., Fiksel, J., Adleff, V., Bruhm, D. C., et al. (2019). Genome-wide cell-free DNA fragmentation in patients with cancer. Nature 570, 385–389. doi: 10.1038/s41586-019-1272-6
Daniūnaitė, K., Jarmalaite, S., and Kriukiene, E. (2019). Epigenomic technologies for deciphering circulating tumor DNA. Curr. Opin. Biotechnol. 55, 23–29. doi: 10.1016/j.copbio.2018.07.002
De Koker, A., Van Paemel, R., De Wilde, B., De Preter, K., and Callewaert, N. (2019). A versatile method for circulating cell-free DNA methylome profiling by reduced representation bisulfite sequencing. bioRxiv [Preprint]. doi: 10.1101/663195
Dor, Y., and Cedar, H. (2018). Principles of DNA methylation and their implications for biology and medicine. Lancet 392, 777–786. doi: 10.1016/s0140-6736(18)31268-6
Erger, F., Nörling, D., Borchert, D., Leenen, E., Habbig, S., Wiesener, M. S., et al. (2020). CfNOMe — a single assay for comprehensive Epigenetic analyses Of cell-free DNA. Genome Med. 12:54. doi: 10.1186/s13073-020-00750-5
Ewing, A. D., Smits, N., Sanchez-Luque, F. J., Faivre, J., Brennan, P. M., Richardson, S. R., et al. (2020). Nanopore sequencing enables comprehensive transposable element epigenomic profiling. Mol. Cell 80, 915.e5–928.e5. doi: 10.1016/j.molcel.2020.10.024
Feng, H., Jin, P., and Wu, H. (2019). Disease prediction by cell-free DNA methylation. Brief Bioinform. 20, 585–597. doi: 10.1093/bib/bby029
Flusberg, B. A., Webster, D. R., Lee, J. H., Travers, K. J., Olivares, E. C., Clark, T. A., et al. (2010). Direct detection of DNA methylation during single-molecule, real-time sequencing. Nat. Methods 7, 461–465. doi: 10.1038/nmeth.1459
Gai, W., Ji, L., Lam, W. K., Sun, K., Jiang, P., Chan, A. W., et al. (2018). Liver- and colon-specific DNA methylation markers in plasma for investigation of colorectal cancers with or without Liver Metastases. Clin. Chem. 64, 1239–1249. doi: 10.1373/clinchem.2018.290304
Gai, W., and Sun, K. (2019). Epigenetic Biomarkers in Cell-Free DNA and applications in liquid biopsy. Genes 10:32. doi: 10.3390/genes10010032
Galardi, F., De Luca, F., Romagnoli, D., Biagioni, C., Moretti, E., Biganzoli, L., et al. (2020). Cell-Free DNA-Methylation-Based methods and applications in oncology. Biomolecules 10:1677. doi: 10.3390/biom10121677
Greenberg, M. V. C., and Bourc’his, D. (2019). The diverse roles of DNA methylation in mammalian development and disease. Nat. Rev. Mol. Cell Biol. 20, 590–607. doi: 10.1038/s41580-019-0159-6
Guo, D., Yang, L., Yang, J., and Shi, K. (2020). Plasma cell-free DNA methylation combined with tumor mutation detection in prognostic prediction of patients with non-small cell lung cancer (Nsclc). Medicine 99:e20431. doi: 10.1097/md.0000000000020431
Guo, S., Diep, D., Plongthongkum, N., Fung, H. L., Zhang, K., and Zhang, K. (2017). Identification of methylation haplotype blocks aids in deconvolution of heterogeneous tissue samples and tumor tissue-of- origin mapping from plasma DNA. Nat. Genet. 49, 635–642. doi: 10.1038/ng.3805
Haigis, K. M., Cichowski, K., and Elledge, S. J. (2019). Tissue-specificity in cancer: the rule, not the exception. Science 363, 1150–1151. doi: 10.1126/science.aaw3472
Han, D. S., Ni, M., Chan, R. W., Chan, V. W., Lui, K. O., Chiu, R. W., et al. (2020). The biology of cell-free DNA fragmentation and the roles of dnase1, dnase1l3, and dffb. Am. J. Hum. Genet. 106, 202–214. doi: 10.1016/j.ajhg.2020.01.008
Heitzer, E., Auinger, L., and Speicher, M. R. (2020). Cell-free DNA and apoptosis: how dead cells inform about the living. Trends Mol. Med. 26, 519–528. doi: 10.1016/j.molmed.2020.01.012
Heitzer, E., Haque, I. S., Roberts, C. E. S., and Speicher, M. R. (2019). Current and future perspectives of liquid biopsies in genomics-driven oncology. Nat. Rev. Genet. 20, 71–88. doi: 10.1038/s41576-018-0071-5
Heitzer, E., and Speicher, M. R. (2018). One size does not fit all: size-based plasma DNA diagnostics. Sci. Transl. Med. 10:eaav3873. doi: 10.1126/scitranslmed.aav3873
Hoadley, K. A., Yau, C., Hinoue, T., Wolf, D. M., Lazar, A. J., Drill, E., et al. (2018). Cell-of-Origin patterns dominate the molecular classification of 10,000 tumors from 33 types of cancer. Cell 173, 291.e6–304.e6. doi: 10.1016/j.cell.2018.03.022
Hofman, P., Heeke, S., Alix-Panabières, C., and Pantel, K. (2019). Liquid biopsy in the era of immuno-oncology: is it ready for prime-time use for cancer patients? Ann. Oncol. 30, 1448–1459. doi: 10.1093/annonc/mdz196
Houseman, E. A., Accomando, W. P., Koestler, D. C., Christensen, B. C., Marsit, C. J., Nelson, H. H., et al. (2012). DNA methylation arrays as surrogate measures of cell mixture distribution. BMC Bioinformatics 13:86. doi: 10.1186/1471-2105-13-86
Houseman, E. A., Kile, M. L., Christiani, D. C., Ince, T. A., Kelsey, K. T., and Marsit, C. J. (2016). Reference-free deconvolution of DNA methylation data and mediation by cell composition effects. BMC Bioinformatics 17:259. doi: 10.1186/s12859-016-1140-4
Huang, C. C., Du, M., and Wang, L. (2019). Bioinformatics analysis for circulating cell-free DNA in cancer. Cancers 11:805. doi: 10.3390/cancers11060805
Huang, J., and Wang, L. (2019). Cell-free DNA methylation profiling analysis-technologies and bioinformatics. Cancers 11:1741. doi: 10.3390/cancers11111741
Im, Y. R., Tsui, D. W. Y., Diaz, L. A., and Wan, J. C. M. (2020). Next-generation liquid biopsies: embracing data science in oncology. Trends Cancer 7, 283–292. doi: 10.1016/j.trecan.2020.11.001
Ivanov, M., Chernenko, P., Breder, V., Laktionov, K., Rozhavskaya, E., Musienko, S., et al. (2019). Utility of cfdna fragmentation patterns in designing the liquid biopsy profiling panels to improve their sensitivity. Front. Genet. 10:194. doi: 10.3389/fgene.2019.00194
Jiang, P., Chan, K. C. A., and Lo, Y. M. D. (2019). Liver-derived cell-free nucleic acids in plasma: biology and applications in liquid biopsies. J. Hepatol. 71, 409–421. doi: 10.1016/j.jhep.2019.04.003
Jiang, P., and Lo, Y. M. D. (2016). The long and short of circulating cell-free DNA and the ins and outs of molecular diagnostics. Trends Genet. 32, 360–371. doi: 10.1016/j.tig.2016.03.009
Jiang, P., Sun, K., Tong, Y. K., Cheng, S. H., Cheng, T. H., Heung, M. M., et al. (2018). Preferred end coordinates and somatic variants as signatures of circulating tumor DNA associated with hepatocellular carcinoma. Proc. Natl. Acad. Sci. U.S.A. 115, E10925–E10933. doi: 10.1073/pnas.1814616115
Kang, S., Li, Q., Chen, Q., Zhou, Y., Park, S., Lee, G., et al. (2017). CancerLocator: non-invasive cancer diagnosis and tissue-of-origin prediction using methylation profiles of cell-free DNA. Genome Biol. 18:53. doi: 10.1186/s13059-017-1191-5
Khier, S., and Lohan, L. (2018). Kinetics of circulating cell-free DNA for biomedical applications: critical appraisal of the literature. Future Sci. OA 4:FSO295.
Ko, J., Baldassano, S. N., Loh, P. L., Kording, K., Litt, B., and Issadore, D. (2018). Machine learning to detect signatures of disease in liquid biopsies - a user’s guide. Lab Chip 18, 395–405. doi: 10.1039/c7lc00955k
Kurdyukov, S., and Bullock, M. (2016). DNA methylation analysis: choosing the right method. Biology 5:3. doi: 10.3390/biology5010003
Kustanovich, A., Schwartz, R., Peretz, T., and Grinshpun, A. (2019). Life and death of circulating cell-free DNA. Cancer Biol. Ther. 20, 1057–1067. doi: 10.1080/15384047.2019.1598759
Lam, W. K., Jiang, P., Chan, K. C., Peng, W., Shang, H., Heung, M. M., et al. (2019). Methylation analysis of plasma DNA informs etiologies of Epstein-barr virus-associated diseases. Nat. Commun. 10:3256. doi: 10.1038/s41467-019-11226-5
Lehmann-Werman, R., Magenheim, J., Moss, J., Neiman, D., Abraham, O., Piyanzin, S., et al. (2018). Monitoring liver damage using hepatocyte-specific methylation markers in cell-free circulating DNA. JCI Insight 3:e120687. doi: 10.1172/jci.insight.120687
Lehmann-Werman, R., Neiman, D., Zemmour, H., Moss, J., Magenheim, J., Vaknin-Dembinsky, A., et al. (2016). Identification of tissue-specific cell death using methylation patterns of circulating DNA. Proc. Natl. Acad. Sci. U.S.A. 113, E1826–E1834. doi: 10.1073/pnas.1519286113
Levy, J. J., Titus, A. J., Petersen, C. L., Chen, Y., Salas, L. A., and Christensen, B. C. (2020). MethylNet: an automated and modular deep learning approach for dna methylation analysis. BMC Bioinformatics 21:108. doi: 10.1186/s12859-020-3443-8
Leygo, C., Williams, M., Jin, H. C., Chan, M. W., Chu, W. K., Grusch, M., et al. (2017). DNA methylation as a Noninvasive Epigenetic biomarker for the detection of cancer. Dis. Markers 2017, 1–13. doi: 10.1155/2017/3726595
Li, J., Zhao, S., Lee, M., Yin, Y., Li, J., Zhou, Y., et al. (2020). Reliable tumor detection by whole-genome methylation sequencing of cell-free DNA in cerebrospinal fluid of pediatric medulloblastoma. Sci. Adv. 6:eabb5427. doi: 10.1126/sciadv.abb5427
Li, W., Li, Q., Kang, S., Same, M., Zhou, Y., Sun, C., et al. (2018). CancerDetector: ultrasensitive and non-invasive cancer detection at the resolution of individual reads Using cell-free DNA methylation sequencing data. Nucleic Acids Res. 46:e89. doi: 10.1093/nar/gky423
Liu, L., Toung, J., Jassowicz, A., Vijayaraghavan, R., Kang, H., Zhang, R., et al. (2018). Targeted methylation sequencing of plasma cell-free DNA for cancer detection and classification. Ann. Oncol. 29, 1445–1453. doi: 10.1093/annonc/mdy119
Liu, M., Oxnard, G., Klein, E., Swanton, C., Seiden, M., Liu, M. C., et al. (2020). Sensitive and specific multi-cancer detection and localization using methylation signatures in cell-free DNA. Ann. Oncol. 31, 745–759. doi: 10.1016/j.annonc.2020.02.011
Liu, Q., Fang, L., Yu, G., Wang, D., Xiao, C., and Wang, K. (2019). Detection of DNA base modifications by deep recurrent neural network on Oxford nanopore sequencing data. Nat. Commun. 10:20. doi: 10.1038/s41467-019-10168-2
Liu, X., Ren, J., Luo, N., Guo, H., Zheng, Y., Li, J., et al. (2019). Comprehensive DNA methylation analysis of tissue of origin of plasma cell-free DNA by Methylated CpG Tandem amplification and sequencing (MCTA-Seq). Clin. Epigenet. 11:93. doi: 10.1186/s13148-019-0689-y
Liu, Y., Siejka-Zieliñska, P., Velikova, G., Bi, Y., Yuan, F., Tomkova, M., et al. (2019). Bisulfite-free direct detection of 5-methylcytosine and 5-hydroxymethylcytosine at base resolution. Nat. Biotechnol. 37, 424–429. doi: 10.1038/s41587-019-0041-2
Lo, Y. M. D., Han, D. S. C., Jiang, P., and Chiu, R. W. K. (2021). Epigenetics, fragmentomics, and topology of cell-free DNA in liquid biopsies. Science 372:eaaw3616. doi: 10.1126/science.aaw3616
Luo, H., Wei, W., Ye, Z., Zheng, J., and Xu, R.-H. (2021). Liquid biopsy of methylation biomarkers in cell-free DNA. Trends Mol. Med. 27, 482–500. doi: 10.1016/j.molmed.2020.12.011
Maia, M. C., Salgia, M., and Pal, S. K. (2020). Harnessing cell-free DNA: plasma circulating tumour DNA for liquid biopsy in genitourinary cancers. Nat. Rev. Urol. 17, 271–291. doi: 10.1038/s41585-020-0297-9
Marzese, D. M., and Hoon, D. S. (2015). Emerging technologies for studying DNA methylation for the molecular diagnosis of cancer. Expert Rev. Mol. Diagn. 15, 647–664. doi: 10.1586/14737159.2015.1027194
Menden, K., Marouf, M., Oller, S., Dalmia, A., Magruder, D. S., Kloiber, K., et al. (2020). Deep learning–based cell composition analysis from tissue expression profiles. Sci. Adv. 6:eaba2619. doi: 10.1126/sciadv.aba2619
Michalak, E. M., Burr, M. L., Bannister, A. J., and Dawson, M. A. (2019). The roles of dna, rna and histone methylation in ageing and cancer. Nat. Rev. Mol. Cell Biol. 20, 573–589. doi: 10.1038/s41580-019-0143-1
Moss, J., Magenheim, J., Neiman, D., Zemmour, H., Loyfer, N., Korach, A., et al. (2018). Comprehensive human cell-type methylation atlas reveals origins of circulating cell-free DNA in health and disease. Nat. Commun. 9:5068. doi: 10.1038/s41467-018-07466-6
Moss, J., Zick, A., Grinshpun, A., Carmon, E., Maoz, M., Ochana, B., et al. (2020). Circulating breast-derived DNA allows universal detection and monitoring of localized breast cancer. Ann. Oncol. 31, 395–403. doi: 10.1016/j.annonc.2019.11.014
Nabet, B. Y., Esfahani, M. S., Moding, E. J., Hamilton, E. G., Chabon, J. J., Rizvi, H., et al. (2020). Noninvasive early identification of therapeutic benefit from immune checkpoint inhibition. Cell 183, 363.e13–376.e13. doi: 10.1016/j.cell.2020.09.001
Nassiri, F., Chakravarthy, A., Feng, S., Shen, S. Y., Nejad, R., Zuccato, J. A., et al. (2020). Detection and discrimination of intracranial tumors using plasma cell-free dna methylomes. Nat. Med. 26, 1044–1047. doi: 10.1038/s41591-020-0932-2
Oellerich, M., Schütz, E., Beck, J., and Walson, P. D. (2019). Circulating cell-free DNA—diagnostic and prognostic applications in personalized cancer therapy. Ther. Drug Monit. 41, 115–120. doi: 10.1097/ftd.0000000000000566
O’Leary, B., Hrebien, S., Morden, J. P., Beaney, M., Fribbens, C., Huang, X., et al. (2018). Early circulating tumor DNA dynamics and clonal selection with palbociclib and fulvestrant for breast cancer. Nat. Commun. 9:896. doi: 10.1038/s41467-018-03215-x
Olkhov-Mitsel, E., and Bapat, B. (2012). Strategies for discovery and validation of methylated and hydroxymethylated DNA biomarkers. Cancer Med. 1, 237–260. doi: 10.1002/cam4.22
Olova, N., Krueger, F., Andrews, S., Oxley, D., Berrens, R. V., Branco, M. R., et al. (2018). Comparison of whole-genome bisulfite sequencing library preparation strategies identifies sources of biases affecting DNA methylation data. Genome Biol. 19:33. doi: 10.1186/s13059-018-1408-2
Panagopoulou, M., Karaglani, M., Balgkouranidou, I., Biziota, E., Koukaki, T., Karamitrousis, E., et al. (2019). Circulating cell-free DNA in breast cancer: size profiling, levels, and methylation patterns lead to prognostic and predictive classifiers. Oncogene 38, 3387–3401. doi: 10.1038/s41388-018-0660-y
Peter, M. R., Bilenky, M., Isserlin, R., Bader, G. D., Shen, S. Y., De Carvalho, D. D., et al. (2020). Dynamics of the cell-free DNA methylome of metastatic prostate cancer during androgen-targeting treatment. Epigenomics 12, 1317–1332. doi: 10.2217/epi-2020-0173
Rapisuwon, S., Vietsch, E. E., and Wellstein, A. (2016). Circulating biomarkers to monitor cancer progression and treatment. Comput. Struct. Biotechnol. J. 14, 211–222. doi: 10.1016/j.csbj.2016.05.004
Rostami, A., Lambie, M., Yu, C. W., Stambolic, V., Waldron, J. N., and Bratman, S. V. (2020). Senescence, necrosis, and apoptosis govern circulating cell-free DNA release kinetics. Cell Rep. 31:107830. doi: 10.1016/j.celrep.2020.107830
Sadeh, R., Sharkia, I., Fialkoff, G., Rahat, A., Gutin, J., Chappleboim, A., et al. (2021). Chip-seq of plasma cell-free nucleosomes identifies gene expression programs of the cells of origin. Nat. Biotechnol. 39, 586–598. doi: 10.1038/s41587-020-00775-6
Sanchez, C., Snyder, M. W., Tanos, R., Shendure, J., and Thierry, A. R. (2018). New insights into structural features and optimal detection of circulating tumor DNA determined by single-strand DNA analysis. NPJ Genom. Med. 3:31.
Schutsky, E. K., DeNizio, J. E., Hu, P., Liu, M. Y., Nabel, C. S., Fabyanic, E. B., et al. (2018). Nondestructive, base-resolution sequencing of 5-hydroxymethylcytosine using a dna deaminase. Nat. Biotechnol. 36, 1083–1090. doi: 10.1038/nbt.4204
Scott, C. A., Duryea, J. D., MacKay, H., Baker, M. S., Laritsky, E., Gunasekara, C. J., et al. (2020). Identification of cell type-specific methylation signals in bulk whole genome bisulfite sequencing data. Genome Biol. 21:156. doi: 10.1186/s13059-020-02065-5
Serpas, L., Chan, R. W., Jiang, P., Ni, M., Sun, K., Rashidfarrokhi, A., et al. (2018). Dnase1l3 deletion causes aberrations in length and end-motif frequencies in plasma DNA. Proc. Natl. Acad. Sci. U.S.A. 116, 641–649. doi: 10.1073/pnas.1815031116
Shen, S. Y., Singhania, R., Fehringer, G., Chakravarthy, A., Roehrl, M. H., Chadwick, D., et al. (2018). Sensitive tumour detection and classification using plasma cell-free DNA methylomes. Nature 563, 579–583. doi: 10.1038/s41586-018-0703-0
Sina, A. A. I., Carrascosa, L. G., and Trau, M. (2019). DNA methylation-based point-of-care cancer detection: challenges and possibilities. Trends Mol. Med. 25, 955–966. doi: 10.1016/j.molmed.2019.05.014
Snyder, M. W., Kircher, M., Hill, A. J., Daza, R. M., and Shendure, J. (2016). Cell-free DNA comprises an in vivo nucleosome footprint that informs its tissues-of-origin. Cell 164, 57–68. doi: 10.1016/j.cell.2015.11.050
Song, C., Yin, S., Ma, L., Wheeler, A., Chen, Y., Zhang, Y., et al. (2017). 5-Hydroxymethylcytosine signatures in cell-free dna provide information about tumor types and stages. Cell Res. 27, 1231–1242. doi: 10.1038/cr.2017.106
Sun, K., Jiang, P., Chan, K. C., Wong, J., Cheng, Y. K., Liang, R. H., et al. (2015). Plasma DNA tissue mapping by genome-wide methylation sequencing for noninvasive prenatal, cancer, and transplantation assessments. Proc. Natl. Acad. Sci. U.S.A. 112, E5503–E5512. doi: 10.1073/pnas.1508736112
Sun, K., Jiang, P., Cheng, S. H., Cheng, T. H., Wong, J., Wong, V. W., et al. (2019). Orientation-aware plasma cell-free DNA fragmentation analysis in open chromatin regions informs tissue of origin. Genome Res. 29, 418–427. doi: 10.1101/gr.242719.11
Teschendorff, A. E., Breeze, C. E., Zheng, S. C., and Beck, S. (2017). A comparison of reference-based algorithms for correcting cell-type heterogeneity in Epigenome-Wide Association Studies. BMC Bioinformatics 18:105. doi: 10.1186/s12859-017-1511-5
Tse, O. Y., Jiang, P., Cheng, S. H., Peng, W., Shang, H., Wong, J., et al. (2021). Genome-wide detection of cytosine methylation by single molecule real-time sequencing. Proc. Natl. Acad. Sci. U.S.A. 118:e2019768118. doi: 10.1073/pnas.2019768118
Ulz, P., Thallinger, G. G., Auer, M., Graf, R., Kashofer, K., Jahn, S. W., et al. (2016). Inferring expressed genes by whole-genome sequencing of plasma DNA. Nat. Genet. 48, 1273–1278. doi: 10.1038/ng.3648
Vaisvila, R., Ponnaluri, V. K., Sun, Z., Langhorst, B. W., Saleh, L., Guan, S., et al. (2019). EM-seq: detection of DNA methylation at single Base resolution from picograms of DNA. bioRxiv [Preprint]. doi: 10.1101/2019.12.20.884692
Van der pol, Y., and Mouliere, F. (2019). Toward the early detection of cancer by decoding the epigenetic and environmental fingerprints of cell-free DNA. Cancer Cell 36, 350–368. doi: 10.1016/j.ccell.2019.09.003
Wong, F. C., Sun, K., Jiang, P., Cheng, Y. K., Chan, K. A., Leung, T. Y., et al. (2016). Cell-free DNA in maternal plasma and serum: a comparison of quantity, quality and tissue origin using genomic and epigenomic approaches. Clin. Biochem. 49, 1379–1386. doi: 10.1016/j.clinbiochem.2016.09.009
Wu, A., Cremaschi, P., Wetterskog, D., Conteduca, V., Franceschini, G. M., Kleftogiannis, D., et al. (2020). Genome-wide plasma DNA methylation features of metastatic prostate cancer. J. Clin. Investig. 130, 1991–2000. doi: 10.1172/jci130887
Yuen, Z. W.-S., Srivastava, A., Daniel, R., McNevin, D., Jack, C., and Eyras, E. (2020). Systematic benchmarking of tools for CpG methylation detection from nanopore sequencing. Bioinformatics 12:3438.
Zemmour, H., Planer, D., Magenheim, J., Moss, J., Neiman, D., Gilon, D., et al. (2018). Non-invasive detection of human cardiomyocyte death using methylation patterns of circulating DNA. Nat. Commun. 9:1443. doi: 10.1038/s41467-018-03961-y
Zhang, L., Liang, Y., Li, S., Zeng, F., Meng, Y., Chen, Z., et al. (2019). The interplay of circulating tumor DNA and chromatin modification, therapeutic resistance, and metastasis. Mol. Cancer 18:36. doi: 10.1186/s12943-019-0989-z
Zhao, L.-Y., Song, J., Liu, Y., Song, C.-X., and Yi, C. (2020). Mapping the epigenetic modifications of DNA and RNA. Protein Cell 11, 792–808. doi: 10.1007/s13238-020-00733-7
Zheng, S. C., Webster, A. P., Dong, D., Feber, A., Graham, D. G., Sullivan, R., et al. (2018). A novel cell-type deconvolution algorithm reveals substantial contamination by immune cells in saliva, buccal and cervix. Epigenomics 10, 925–940. doi: 10.2217/epi-2018-0037
Keywords: Cell-free DNA (cfDNA), cellular damage, circulating tumor DNA (ctDNA), deconvolution, liquid biopsy, tissue-of-origin, tumor microenvironment
Citation: Barefoot ME, Loyfer N, Kiliti AJ, McDeed AP IV, Kaplan T and Wellstein A (2021) Detection of Cell Types Contributing to Cancer From Circulating, Cell-Free Methylated DNA. Front. Genet. 12:671057. doi: 10.3389/fgene.2021.671057
Received: 22 February 2021; Accepted: 17 May 2021;
Published: 27 July 2021.
Edited by:
Suman Ghosal, National Institutes of Health (NIH), United StatesReviewed by:
Xiaobin Zheng, Carnegie Institution for Science (CIS), United StatesCopyright © 2021 Barefoot, Loyfer, Kiliti, McDeed, Kaplan and Wellstein. This is an open-access article distributed under the terms of the Creative Commons Attribution License (CC BY). The use, distribution or reproduction in other forums is permitted, provided the original author(s) and the copyright owner(s) are credited and that the original publication in this journal is cited, in accordance with accepted academic practice. No use, distribution or reproduction is permitted which does not comply with these terms.
*Correspondence: Anton Wellstein, d2VsbHN0ZWFAZ2VvcmdldG93bi5lZHU=
Disclaimer: All claims expressed in this article are solely those of the authors and do not necessarily represent those of their affiliated organizations, or those of the publisher, the editors and the reviewers. Any product that may be evaluated in this article or claim that may be made by its manufacturer is not guaranteed or endorsed by the publisher.
Research integrity at Frontiers
Learn more about the work of our research integrity team to safeguard the quality of each article we publish.