- 1Department of Epidemiology and Biostatistics, School of Public Health, Xuzhou Medical University, Xuzhou, China
- 2Center for Medical Statistics and Data Analysis, School of Public Health, Xuzhou Medical University, Xuzhou, China
- 3Department of Pediatrics, Affiliated Hospital of Xuzhou Medical University, Xuzhou, China
Background: Multiple genes were previously identified to be associated with cervical cancer; however, the genetic architecture of cervical cancer remains unknown and many potential causal genes are yet to be discovered.
Methods: To explore potential causal genes related to cervical cancer, a two-stage causal inference approach was proposed within the framework of Mendelian randomization, where the gene expression was treated as exposure, with methylations located within the promoter regions of genes serving as instrumental variables. Five prediction models were first utilized to characterize the relationship between the expression and methylations for each gene; then, the methylation-regulated gene expression (MReX) was obtained and the association was evaluated via Cox mixed-effect model based on MReX. We further implemented the aggregated Cauchy association test (ACAT) combination to take advantage of respective strengths of these prediction models while accounting for dependency among the p-values.
Results: A total of 14 potential causal genes were discovered to be associated with the survival risk of cervical cancer in TCGA when the five prediction models were separately employed. The total number of potential causal genes was brought to 23 when conducting ACAT. Some of the newly discovered genes may be novel (e.g., YJEFN3, SPATA5L1, IMMP1L, C5orf55, PPIP5K2, ZNF330, CRYZL1, PPM1A, ESCO2, ZNF605, ZNF225, ZNF266, FICD, and OSTC). Functional analyses showed that these genes were enriched in tumor-associated pathways. Additionally, four genes (i.e., COL6A1, SYDE1, ESCO2, and GIPC1) were differentially expressed between tumor and normal tissues.
Conclusion: Our study discovered promising candidate genes that were causally associated with the survival risk of cervical cancer and thus provided new insights into the genetic etiology of cervical cancer.
Introduction
Cervical cancer is one of the most common malignancies in the female population, mostly caused by infection with human papillomavirus (HPV) (Šarenac and Mikov, 2019). In terms of cancer statistics in 2018, cervical cancer is the fourth most common malignancy and the fourth leading cause of cancer death among women worldwide, with an estimate of 570,000 cases and 311,000 deaths globally (Bray et al., 2018). Moreover, cervical cancer is the second primary cause of cancer death in women aged 20–39 years (Siegel et al., 2019). Although great advances have been achieved for cervical cancer, there is still a lack of reliable diagnostic biomarkers for early identification and screening (Chen and Gyllensten, 2015). In addition, despite the utilization of HPV vaccines for prevention and chemoradiotherapy as well as radical surgery offering satisfactory survival rate for early-stage cervical cancer patients, effective treatments for advanced patients are rarely available, especially in developing countries and regions (Chen and Gyllensten, 2015).
Therefore, it is an urgent demand in clinical practice that valuable biomarkers should be well discerned and validated to be a sign of the early stage or to provide profile of cervical cancer progression (Nahand et al., 2019). As an effort to understand the genetic foundation of susceptibility to cervical cancer, in the past decade multiple genome-wide association studies (GWASs) were undertaken and discovered a group of cervical-cancer-associated genetic variants (see Chen and Gyllensten, 2015), where a large number of associated germline genetic variants and genes were described for cervical cancer. These findings imply that the development of cervical cancer relies to a significant extent on inherited genetic components and genetic predisposing factors may affect the probability and persistence of, or sensitivity to, HPV infection and the rate of tumor development as well as progression (Chen and Gyllensten, 2015). However, like many complex human traits and diseases (Visscher et al., 2017), the genome-wide SNP-based heritability of cervical cancer estimated in GWAS is smaller than expected. For example, the heritability is 11.7% (se = 9.8%) in a Japanese population (Masuda et al., 2020) and 24.0% (se = 2.9%) in a Swedish population (Chen et al., 2015), both of which are lower than that observed in family studies (Magnusson et al., 2000). The remaining missing heritability suggests that a large number of causal genes and genetic variants have yet been discovered and that continuous efforts to identify causative genes for cervical cancer are worthwhile (Chen and Gyllensten, 2015).
As also demonstrated in many studies (Zhao et al., 2006, 2014; Wang et al., 2013; Zhu et al., 2017; Kim et al., 2018; Yu et al., 2020), mRNA-gene expression measured at the transcript level influences the progression of complex diseases more directly than other omic measurements. However, the establishment of the potential causal relationship between altered gene expressions and the survival of cervical cancer patients is not straightforward in observation studies due to unknown confounders and possible reverse causation. The latter is of particular concern because we cannot determine whether the regulated gene expressions are the causal factors or the consequence of the development or progression of cervical cancer due to the considerably complicated biological network and interaction. Due to this reason, previous studies often aimed to examine association rather than causality between gene expression and cervical cancer.
In statistical genetics, a powerful statistical tool to determine causal relationship and estimate causal effect of the exposure on the outcome in observational studies is Mendelian randomization (MR), which is built based on commonly used instrumental variable approaches developed in the field of causal inference (Angrist et al., 1996; Greenland, 2000; Sheehan et al., 2008). Under some certain assumptions, the results of MR analysis are less susceptible to reverse causation and confounding factors (Davey Smith and Ebrahim, 2003). A key point in MR is to select valid instrumental variables for the exposure (i.e., expression level). Biologically, methylation CpG sites of a specific gene within the transcript start site can downregulate its expression level, which can in turn further affect the survival of cancer patients (Glinsky, 2006; de Tayrac et al., 2009; Fabiani et al., 2010; Wang et al., 2013), indicating that methylation alterations play a central role in cancers by regulating expression profile. This motivates us to propose a two-stage causal inference approach with methylations as instrumental variables of expression to detect potential causal genes for the survival of cervical cancer patients. The two-stage instrumental variable inference is widely employed in many research fields, such as sociology, economics (Angrist and Keueger, 1991), and genetic medicine (Xue et al., 2020). In addition, applying methylations as instruments for causal inference is also commonly seen in recent genomic integrative analyses (Hannon et al., 2018; Qi et al., 2018; Wu et al., 2018).
Methodologically, our proposed approach follows the similar principle of prediXcan (Gamazon et al., 2015) that was developed recently to identify causal genes for complex diseases with genetic variants serving as instrumental variables in the framework of MR and transcriptome-wide association studies (TWAS) (Gamazon et al., 2015; Gusev et al., 2016; Zeng and Zhou, 2017; Barbeira et al., 2019; Hu et al., 2019; Wainberg et al., 2019). Specifically, in our context we implement a two-stage inference procedure (Figure 1): in the first stage, the weights (i.e., effect sizes on expression) of DNA methylation alterations within the promoter region and gene body for individual genes are estimated via genetic prediction models; in the second stage, the methylation-regulated gene expression (MReX) is imputed based on the corresponding prediction model and the potential causal association between the gene and the survival risk of cervical cancer is examined using MReX. More importantly, the two-stage based causal inference can be viewed as a special case of MR analysis from a statistical perspective (Zhu and Zhou, 2020). Furthermore, we consider five commonly used prediction models in the first stage of our two-stage inference procedure and exploit the aggregated Cauchy association test (ACAT) method (Liu et al., 2019; Liu and Xie, 2020; Xiao et al., 2020)—a novel combination strategy that is robust against positive correlation—to take advantage of respective strengths of these models while accounting for dependency among the p-values of various models.
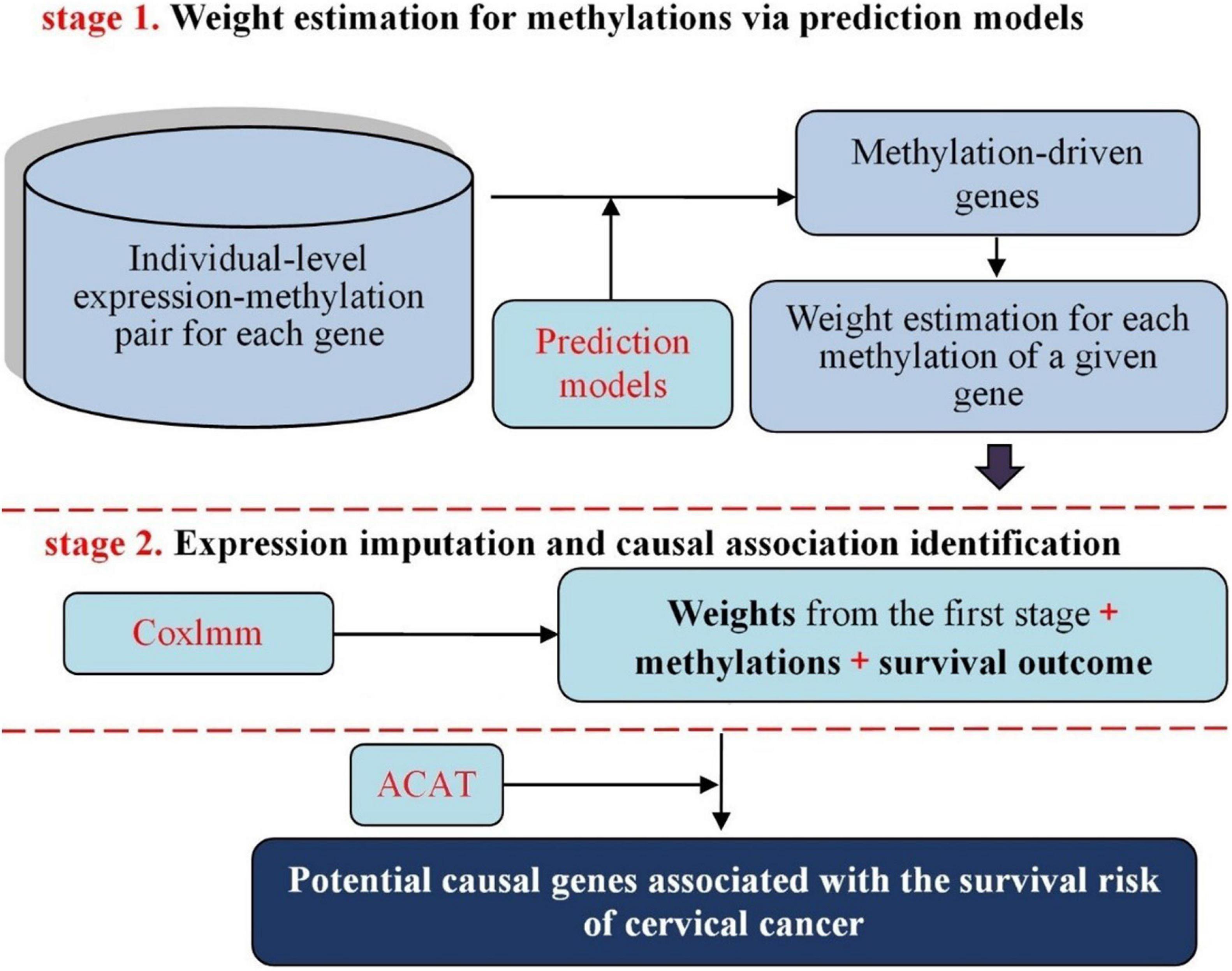
Figure 1. Schematic framework of our proposed two-stage causal inference approach. Top: estimate the weight of each methylation site based on the methylation-expression pair of a given gene with various prediction models; Bottom: evaluate the association between methylation-regulated gene expression (MReX) and the survival of cervical cancer using the Cox linear mixed-effects model and then discover causal genes for cervical cancer in TCGA.
We finally apply the proposed approach to the cervical cancer dataset in The Cancer Genome Atlas (TCGA) project (Hoadley et al., 2018). A total of 14 potential causal genes were discovered to be associated with the survival risk of cervical cancer when the five prediction models were separately implemented. The total number of potential causal genes was brought to 23 when conducting the combination test with ACAT. Some of the newly discovered genes were reported in previous literature and differentially expressed between tumor and normal tissues. In addition, functional analyses showed that these genes were enriched in tumor-associated pathways.
Materials and Methods
TCGA Cervical Cancer Data Sets and Quality Control
Our analysis mainly relied on publicly available datasets of cervical cancer in TCGA (Hoadley et al., 2018). From https://xenabrowser.net/hub/, we obtained clinical features on 317 samples, 20,530 RSEM normalized expressions on 308 samples, and 485,577 DNA methylation alterations on 312 samples. To avoid racial heterogeneity in survival, expressions, and methylations, when carrying out quality control before the formal analysis, we only reserved 216 white patients. Afterward, we further deleted eight patients for whom gene expressions or methylations cannot be available. We also removed another eight patients who had incomplete clinical covariates. The description of important characteristics of this cervical cancer dataset after filtering is summarized in Table 1. According to the TCGA annotation mapping file, we only considered protein-coding genes and defined in our analysis methylations as those within the gene body and an extended region before the transcription starting site so that the promoter can be included. Then, each gene expression was quantile-transformed so that it followed a standard normal distribution and each methylation was standardized. The missing DNA methylations values and gene expressions (no more than 10%) were simply imputed with median. The flowchart for our study is shown in Figure 2.
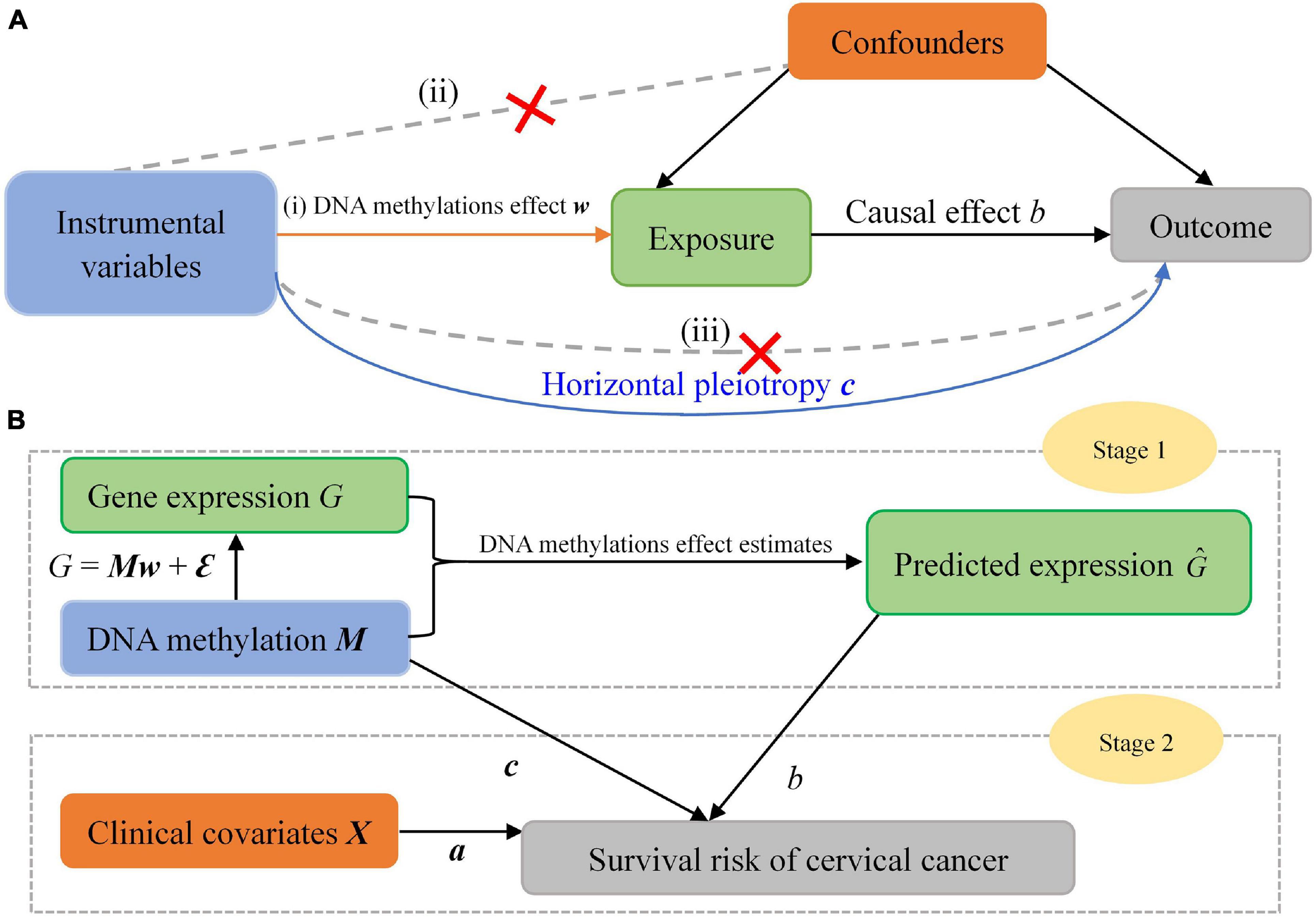
Figure 2. (A) Mendelian randomization framework for the two-stage association analysis. The three assumptions for valid instrumental variables (IV) are indicated by arrows or the absence of arrows: (i) the relevance assumption: the IV is robustly associated with the exposure; (ii) the independence assumption: the IV is not associated with confounding factors; (iii) the exclusion restriction assumption: there is no alternative way that the IV affects the outcome other than via the exposure. The blue solid line represents direct effects of DNA methylations. (B) Statistical scheme of our two-stage inference approach.
A Two-Stage Inference Approach
Linear Models Predicting Gene Expression With DNA Methylation Alterations
We now explain our two-stage inference model (Figure 1). Let G be an n-vector of gene expression levels for the ith gene measured on n individuals, and M be an n × p matrix for a group of DNA methylations that are located within this gene; note that p varies gene by gene. In the first stage, we apply the following linear model to link G and M
where w is a p-vector for effect sizes of DNA methylations, ε is an n-vector of residual errors following an independent and identical normal distribution with mean zero and variance , and In denotes the n-dimensional identity matrix. Because of the possible high-dimensional issue where the number of DNA methylations p is larger than the sample size n, the commonly used least-squares method is no longer applicable for estimating w. We instead employ several novel prediction models which are specially designed for high-dimensional datasets and particularly consider five regressions including linear mixed-effects model (LMM) (Yang et al., 2010; Makowsky et al., 2011; Zeng et al., 2017), Bayesian sparse linear mixed-effects model (BSLMM) (Zhou et al., 2013), latent Dirichlet process regression (DPR) (Zeng and Zhou, 2017), Lasso (Tibshirani, 1996), and elastic net (ENET) (Zou and Hastie, 2005). Among these methods, LMM, BSLMM, and DPR explicitly incorporate all DNA methylations into the model by assuming diverse prior distributions for the effect sizes, while Lasso and ENET only include some most important DNA methylations with the way of regularization based on variable selection. The details of these models are described in Zhu and Zhou (2020) and Zeng et al. (2021). We implement LMM and BSLMM with the GEMMA software (version 0.94), DPR with the DPR software (Zeng and Zhou, 2017), and Lasso and ENET with the R glmnet package (version 2.0-18) (Friedman et al., 2010). Using these models, we can obtain the estimate of effect sizes of DNA methylations (denoted by ) as well as the MReX level = for each gene.
Cox Mixed-Effect Regression Discovering Methylation-Regulated Genes
In the second stage, we investigate the association between the gene and the survival risk of cervical cancer using the Cox model (Cox, 1972). Besides the direct gene effect based on MReX , we also incorporate the impact of DNA methylation alterations into the survival model to explain possible horizontal pleiotropy (Bowden et al., 2015, 2016; Burgess and Thompson, 2017; Slob et al., 2017; Barfield et al., 2018; Verbanck et al., 2018)
where t is the observed survival time, h0(t) is an arbitrary baseline hazard function, and a = (a1, a2, …, am) is an m-vector of effect sizes for available covariates X, such as age of onset, clinical stage, and tumor status (Table 1); note that, as methylation is highly associated with age, we here explicitly adjust for age as a covariate; b is the effect size for the given gene and is of our primary interest, and c = (c1, c2, …, cp) is a p-vector of effect sizes for DNA methylations. Because of the same reason of high-dimensional problem mentioned before, we assume c’s are random effects following a normal distribution with mean zero and variance , leading to the Cox linear mixed-effects regression model (denoted by coxlmm) (Therneau et al., 2003). When c = 0, or equivalently = 0, coxlmm shown in (2) reduces into the general Cox model where only the influence of the methylation-driven gene exists. We fit coxlmm with the R coxme (version 2.2-10) package (Therneau, 2019) via the Laplace approximation algorithm based on the second-order Taylor series expansion (Therneau et al., 2003). The significance of MReX is examined through the Wald test (H0: b = 0): , where is the estimate of the effect size b, with the variance of the estimate . The p-values of the Z statistic can be easily obtained because it asymptotically follows a standard normal distribution.
Three Remarks for the Proposed Two-Stage Causal Inference Method
First, it needs to highlight that instrumental variables are often obtained from external independent datasets in traditional MR studies, leading to the so-called two-sample analysis. However, sometimes, if we have only one sample dataset with individual-level methylations, expressions, and survival outcomes, we can still perform a one-sample MR analysis. In brief, there are two ways to conduct such analysis. First, as done in the present work, one can estimate effect sizes of instrumental variables and examine the association with all individuals. Second, one can split the dataset into two parts, with one part for estimating effect sizes of instrumental variables and the other part for analyzing association between the gene and the outcome. Both the ways have advantages and limitations. Specifically, the major advantage of the first way is that no random split is needed and it has relatively higher power because of larger samples employed. The limitation is that it might suffer from inflation in controlling type I error; however, the resulting inflation is acceptable in terms of recent simulations (Xue et al., 2020). The advantage of the second way is that it can maintain calibrated type I error control, but its power might be limited as smaller samples applied in the estimation stage and the association stage. Another limitation of the second way is that its performance may greatly rely on how to split the dataset. Therefore, in our work we perform our one-sample analysis using all available cervical cancer patients in both stages.
Second, in our analysis we apply a group of local methylations serving as instrumental variables, which has the potential of higher power because of more variation of expression explained compared to the strategy of applying only a few significantly gene-associated ones (Zeng and Zhou, 2017; Zeng et al., 2021). In addition, utilizing local methylation CpG sites for a given gene is also widely seen in gene-based statistical genomics analysis when involving methylations (Kingsley et al., 2016; Loucks et al., 2016; Chu and Huang, 2017; Huang, 2019; Liu et al., 2020). However, it has been widely warned in MR studies that incorporating more instrumental variables (e.g., methylations) may have higher risk in violating the third MR assumption (the exclusion restriction assumption; Figure 3) due to unknown biological pathways (Zeng and Zhou, 2019a,b; Zeng et al., 2019; Yuan et al., 2020; Liu et al., 2021). More specifically, methylations themselves might have substantial impact on survival risk through horizontal pleiotropy besides the indirect influence via the pathway of gene. To handle this problem, we attempt to remove possible pleiotropic effects of methylations by adding a random-effect term of methylations in the Cox model. It has been shown that doing this is an effective manner to account for instrumental pleiotropy (Yuan et al., 2020; Liu et al., 2021).
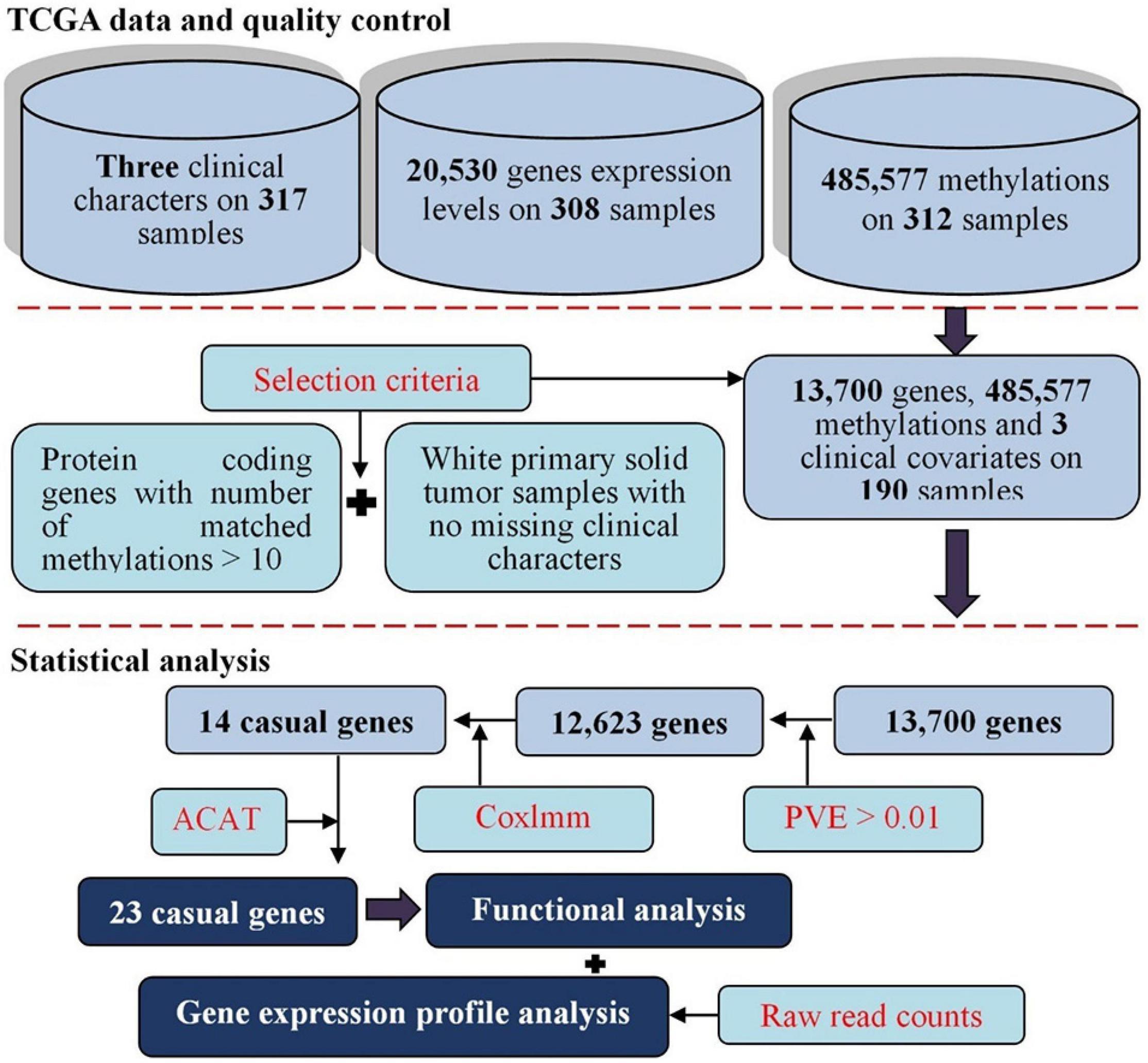
Figure 3. Flowchart for the present study with datasets of cervical cancer available from TCGA. First, various levels of raw datasets were included for cervical cancer; we conducted a series of quality control for those raw datasets. Second, gene expressions predicted with methylations were generated with diverse prediction models, the Cox linear mixed-effects model was applied to identify methylation-driven genes based on predicted expression levels; we aggregated the p-values of genes from different prediction models through a p-values combination manner to find significant genes that were related to the survival of cervical cancer. Finally, we further implemented functional and differential expression analyses for newly identified associated genes.
Third, as mentioned before and shown in Figure 3, we construct our two-stage-based causal inference under the framework of MR and TWAS (Yuan et al., 2020; Zhu and Zhou, 2020; Liu et al., 2021; Zeng et al., 2021); therefore, in terms of the principles of the two methods, we possess the potential for identifying putatively causal genes associated with the survival risk of cervical cancer.
Aggregated Cauchy Association Test
Because multiple prediction models are applied, for each gene we thus yield a set of p-values pk (k = 1, 2, …, K; with K the number of the prediction models) according to (2). Unfortunately, the simple and commonly used Fisher’s method for aggregating mutually independent multiple tests cannot be exploited due to highly positive correlation among individual tests since they are implemented for the same data set with the similar logic (Fisher, 1934; Rice, 2010). To effectively address the aforementioned difficulty of dependency, we apply the recently developed aggregated Cauchy association test (ACAT) (Liu et al., 2019; Liu and Xie, 2020). Specifically, suppose there are a set of p-values for each gene and each pk is uniformly distributed between 0 and 1 under the null; we have
where ωk represents the nonnegative weight for each pk with and K = 5; in the absence of prior knowledge, the equal weights are adapted, and assume that ωk is not related to pk. It has been theoretically demonstrated that the dependency among p-values imposes little influence on the final pooled p-values in ACAT, especially on exceedingly small p-values which are of particular interest for practitioners (Li et al., 2019; Liu et al., 2019). Therefore, ACAT renders the potential to allow us to aggregate correlated p-values obtained from multiple tests into a single well-calibrated p-value that can maintain the type I error control correctly.
Functional Analysis and Differential Expression Analysis for Newly Identified Associated Genes
Using the proposed two-stage causal inference model, we identified multiple candidate causal genes associated with the survival risk of cervical cancer. We here implemented additional bioinformatics analyses to study their biological functions. First, gene ontology (GO) and Kyoto Encyclopedia of Genes and Genomes (KEGG) pathway analyses were conducted using the R clusterProfiler package (version 3.16.0) (Yu et al., 2012). In addition, to further evaluate the expression profiles of these newly discovered genes, we performed differential expression analysis with 190 cervical tumors and three normal tissues that were also available from TCGA. After normalization with the trimmed mean of M values (TMM) method, differential expressed genes (DEGs) were screened via the exact test based on quantile-adjusted conditional maximum likelihood estimation (Robinson and Smyth, 2008; Li et al., 2013) implemented in the edgeR package (version 3.30.3) (Robinson et al., 2010; McCarthy et al., 2012). Following previous work (Deng et al., 2019), DEGs were defined if false discovery rate (FDR) < 0.05 and | log2FC| ≥ 1.0.
Results
Cervical Cancer Datasets in TCGA and Methylation-Regulated Genes
After quality control, we reserved 485,577 DNA methylation CpG sites, three clinical covariates (i.e., age of onset, clinical stage, and tumor status) and up to 190 cervical cancer patients of European ancestry. To avoid numerical instability, we focused on protein-coding genes which had at least 10 methylations within the promoter region and the total gene body (Liu et al., 2020). We also first performed the LMM analysis (Visscher et al., 2008; Yang et al., 2011; Zhou et al., 2013) for each protein-coding gene based on its methylations and selected genes with the phenotypic variance explained by methylations larger than 1% (corresponding to a correlation coefficient of 10%). The remaining 12,623 genes are referred to as methylation-regulated genes and included in our subsequent analyses (Figure 2). Most of the genes analyzed (92.0%) have the number of DNA methylation CpG sites less than 50 (Supplementary Figure 1).
Identification of Potential Causal Genes With Cox Linear Mixed-Effect Regression
We employed the coxlmm (Therneau et al., 2003) with various prediction models to examine the relationship between MReX and the survival risk of cervical cancer patients while adjusting for the direct effect of methylations and the confounding effect of clinical covariates. First, we observe that these prediction models display varying performances across genes (Figure 4A). Specifically, some prediction models have higher prediction accuracy for some genes but behave less satisfactorily for others. For example, in terms of R2, BSLMM behaves well for 38.3% genes (=4,834/12,623), while Lasso, ENET, LMM, and DPR have higher R2 for 26.82% (=3,386/12,623), 14.79% (=1,867/12,623), 10.92% (=1,378/12,623), and 9.17% (=1,158/12,623) genes, respectively. As expected, the resulting p-values of these prediction methods in coxlmm are highly correlated (Figure 4B). For example, the Pearson’s correlation of the p-values (in a scale of -log10) ranges from 0.63 between DPR-coxlmm and Lasso-coxlmm to 0.96 between LMM-coxlmm and BSLMM-coxlmm.
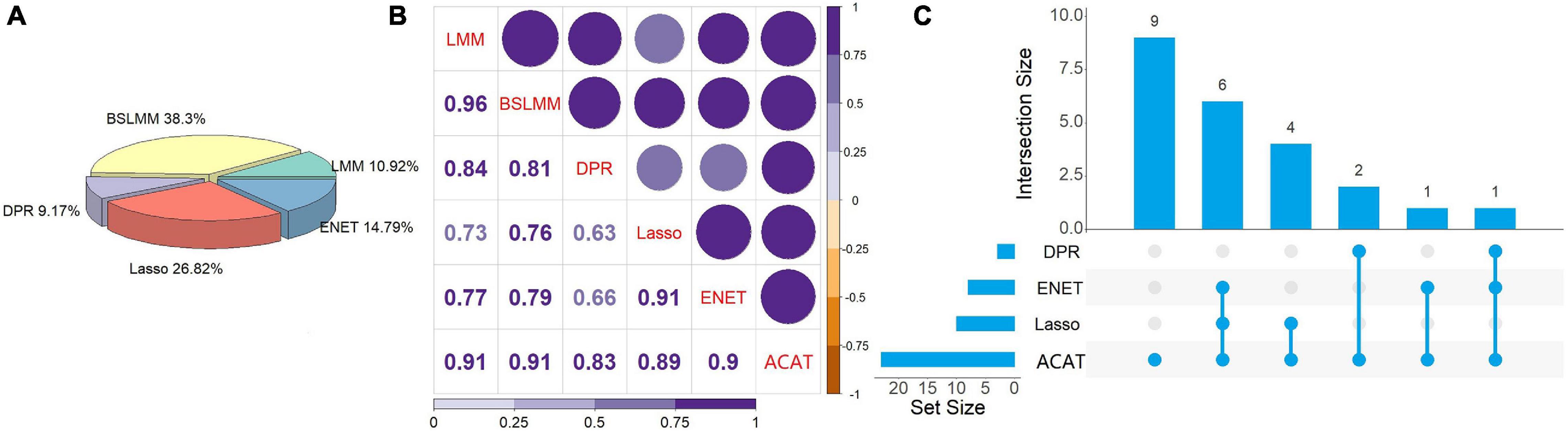
Figure 4. (A) The number of prediction models that have maximum R2 across all the genes analyzed when predicting expression level with using methylations. (B) Pearson’s correlation of the p-values (in a scale of -log10) obtained via in the Cox linear mixed-effects model with five different prediction models. In the plot the intensity of the color and the size of the circle represent the magnitude of the correlation. (C) UpSet plot to illustrate the intersection of associated genes identified by tests with five prediction models. LMM, Linear mixed model; BSLMM, Bayesian sparse linear mixed model; DPR, Latent Dirichlet Process Regression; ENET, elastic net; ACAT, aggregated Cauchy association test.
Based on the results of coxlmm, a total of 14 unique potential associated genes (FDR < 0.05) are identified (Table 2). Specifically, we detect three genes with DPR-coxlmm, 10 genes via Lasso-coxlmm, and eight genes through ENET-coxlmm but do not discover any potential associated genes using LMM-coxlmm or BSLMM-coxlmm (Figure 5). Among these, six genes (i.e., YJEFN3, SPATA5L1, C5orf55, PPIP5K2, ESCO2, and ZNF225) are simultaneously found by Lasso-coxlmm and ENET-coxlmm, while only one gene (i.e., VPS4B) is simultaneously discovered by DPR-coxlmm and ENET-coxlmm (Figure 4C).
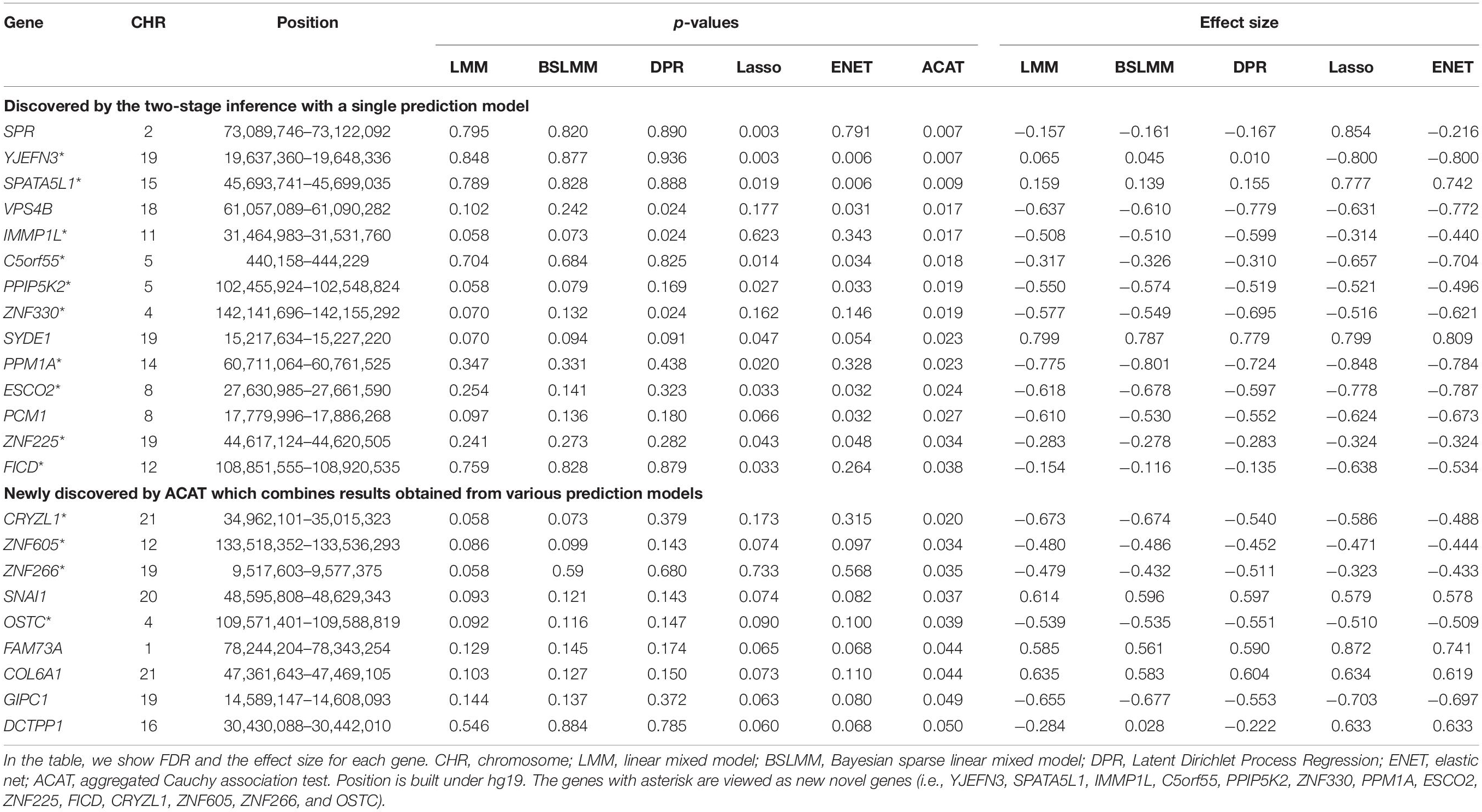
Table 2. A total of 23 associated genes identified via the proposed two-stage causal inference approach.
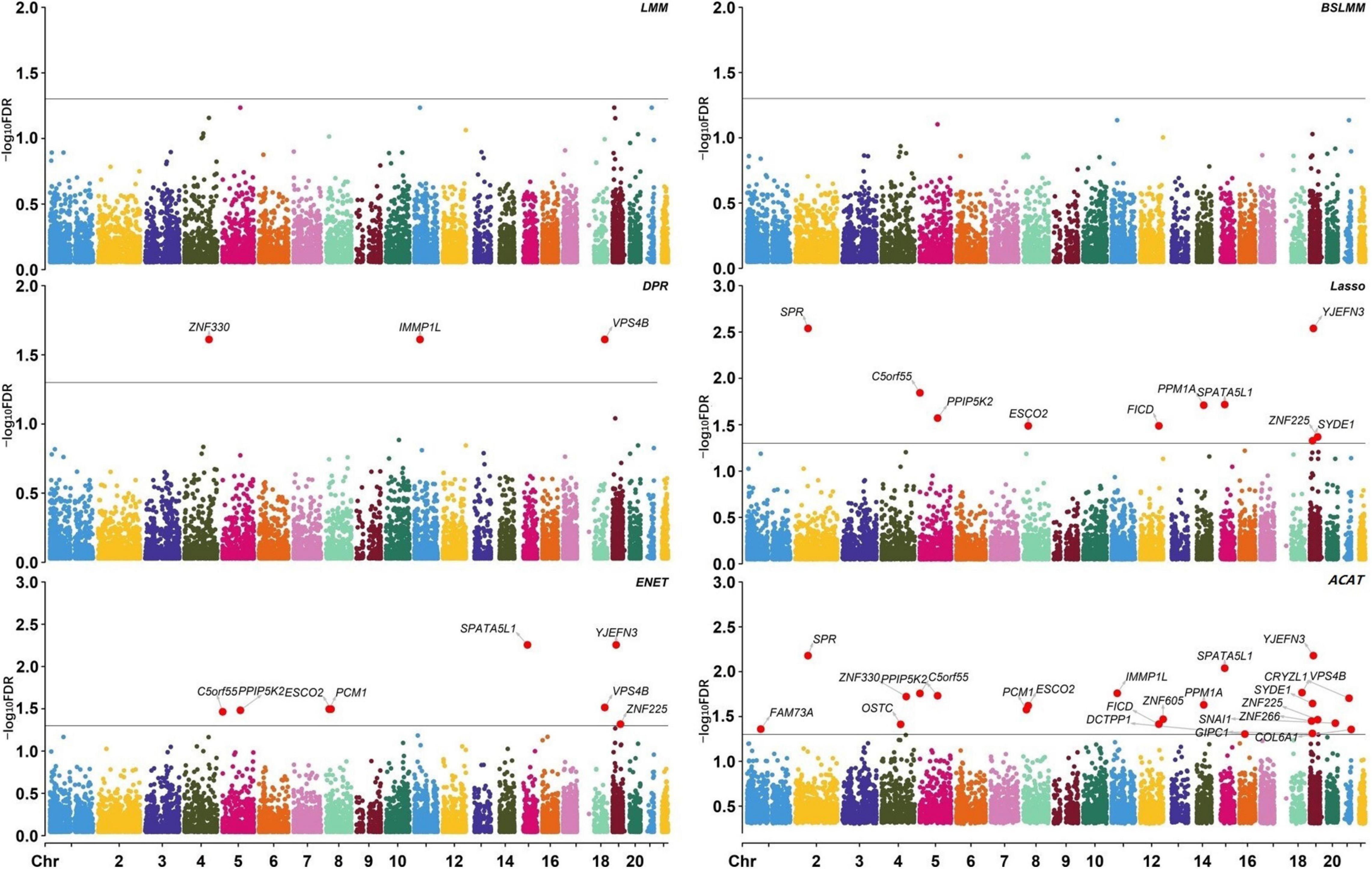
Figure 5. Manhattan plot showing the significance of all genes. Each plot is in a -log10 [false discovery rate (FDR)] scale. Genes with -log10 FDR > 1.3 (i.e. FDR < 0.05) are highlighted. DPR, Latent Dirichlet Process Regression; ENET, elastic net; ACAT, aggregated Cauchy association test.
Among these potential associated genes, we find that PCM1 (FDRENET = 0.032), classified to the cell cycle control network, was previously discovered to be associated with the early stage of cervical cancer (Güzel et al., 2018). SPR (FDRLasso = 0.003) is located within the 1-Mb genetic region of previous GWAS-identified gene ALMS1 (Masuda et al., 2020). In addition, VPS4B (FDRDPR = 0.024 and FDRENET = 0.031) is a subtype of VPS4 which is the component of the ESCRT machinery and plays an essential role in HPV infectious entry and capsid disassembly (Broniarczyk et al., 2017). The remaining 10 genes (i.e., YJEFN3, SPATA5L1, C5orf55, PPM1A, IMMP1L, ZNF330, PPIP5K2, ESCO2, FICD, and ZNF225) are not directly reported to be related to the survival risk of cervical cancer in previous literature. However, for these genes we find suggestive indirect evidence that may support their association with the survival risk of cervical cancer. Specifically, for example, YJEFN3 is a member of the human YJEFN domain-containing protein family strongly expressing in Leydig cell tumors and in the fibromas and participates in cholesterol processing and steroid hormone metabolism (Rudolph et al., 2007). SPATA5L1 might play a key role in inhibiting ATP hydrolysis and four-way junction helicase activity and further influence DNA replication and pathogenesis (White et al., 2005; Rudolph et al., 2007). Smac/DIABLO was expressed de novo in a certain subset of cervical tumors (Martinez-Ruiz et al., 2008), while mature Smac/DIABLO was produced on the mitochondrial inner membrane via IMMP1L (Burri et al., 2005). PPIP5 kinases (e.g., PPIP5K2) mediate PP-IP binding, activate casein kinase 2 (CK2), and promote the phosphorylation of the TTT complex, which stimulates DNA-PK/ATM to activate p53 on the cancer cells (Fridy et al., 2007; Lee et al., 2020). There exists evidence that miR-135b leads to cervical cancer cell transformation (Leung et al., 2014) and downregulated miR-135b expression could inhibit the proliferation and invasion of tumor cells by upregulating PPM1A (Gao et al., 2019).
ACAT Combining p-Values From Different Prediction Models
As mentioned before, because the p-values obtained from coxlmm with diverse prediction models are highly dependent (Figure 4B), we effectively apply ACAT to combine the five p-values and generate an overall significance for each gene (Figure 3 and Table 2). Nine associated genes are additionally discovered (Figure 4C), including CRYZL1, ZNF605, ZNF266, SNAI1, OSTC, FAM73A, COL6A1, GIPC1, and DCTPP1. We found 87.0% (=20/23; except SPR, YJEFN3, and DCTPP1) directions of gene effect consistent across the five genetic prediction models (Table 2). In addition, for these genes it seems that the association signals are mostly driven by LASSO and ENET (Table 2). This observation might imply that there may be only a few of methylations implicated in regulating the expression levels of these genes. As a result, sparse prediction models (i.e., LASSO and ENET) lead to higher power in subsequent association analysis due to better accuracy (Zeng et al., 2021). Among these genes, five (i.e., SNAI1, COL6A1, GIPC1, DCTPP1, and FAM73A) were identified in prior work and SYDE1 locates within the 1-Mb generic region of GIPC1 (Chen et al., 2013a, 2016; Shi et al., 2013; Miura et al., 2014; Leo et al., 2017; Takeuchi et al., 2019; Masuda et al., 2020). Specifically, it is shown that SNAI1, along with ZEB1, regulated the epithelial–mesenchymal transition and was then involved in the metastasis of cervical cancer (Chen et al., 2013b). The upregulated COL6A1 expression in the tissues of cervical cancer was related to poor clinical prognosis and treated as an important biomarker of cervical cancer progression (Hou et al., 2016). The downregulation of GIPC1 in cervical cancer with HPV-18 infection can lead to the resistance to cytostatic transforming growth factor β signaling through TGFβR3 destabilization (Katoh, 2013).
In addition, DCTPP1 was found to be differentially expressed in normal and cancerous tissues and it was significantly accumulated in the nucleus of cervical carcinoma, implying the important role of DCTPP1 under malignant pathology (Zhang et al., 2013). Family with sequence similarity 73, member A (FAM73A) is the downregulated gene of DNA from exfoliated cervical cells in terms of the HPV-16 variant analysis (Green, 2019; Meng et al., 2020). CRYZL1 contains a reduced nicotinamide adenine dinucleotide (phosphate) (NAD(P)H) binding site which is involved in cellular metabolism, while cervical lesions are associated with cellular metabolic abnormalities (Wang et al., 2020). It is previously found that the members of the ZNF family interact with nucleic acids, proteins, and small molecules and are involved in a variety of crucial molecular processes in cervical tumor cells at replication, transcriptional, and translational levels. Thus, ZNF605 and ZNF266 may be potentially targetable (Das et al., 2016; The Cancer Genome Atlas Research Network, 2017; Li et al., 2018). OSTC can regulate gamma-secretase (Wilson et al., 2011) while this secretase affects the ability of HPV pseudo-viruses infection in both human HaCat cells and mouse cells (Huang et al., 2010).
In summary, compared with the tests via individual prediction methods, it is demonstrated that ACAT greatly improves statistical power by combining dependent tests and thus identifies more potential prognosis-associated genes for the survival risk of cervical cancer. Totally, 23 genes are discovered to be related to the survival risk of cervical cancer, among which 14 genes are likely newly novel genes (i.e., YJEFN3, SPATA5L1, IMMP1L, C5orf55, PPIP5K2, ZNF330, CRYZL1, PPM1A, ESCO2, ZNF605, ZNF225, ZNF266, FICD, and OSTC).
Identification of DEGs, GO, and KEGG Pathway Annotation
In terms of the differential expression analysis, four DEGs are detected among the 23 new potential causal genes identified above (Figure 6A). COL6A1 and SYDE1 are upregulated genes, while ESCO2 and GIPC1 are downregulated genes (Figure 6B). To explore the potential functions of these genes that may be associated with the tumorigenesis and development of cervical cancer, we performed functional enrichment analysis with GO and KEGG using the R package clusterProfiler (version 3.16.0) (Yu et al., 2012). The top five GO terms of three parts and two KEGG pathways are shown in Figure 6C.
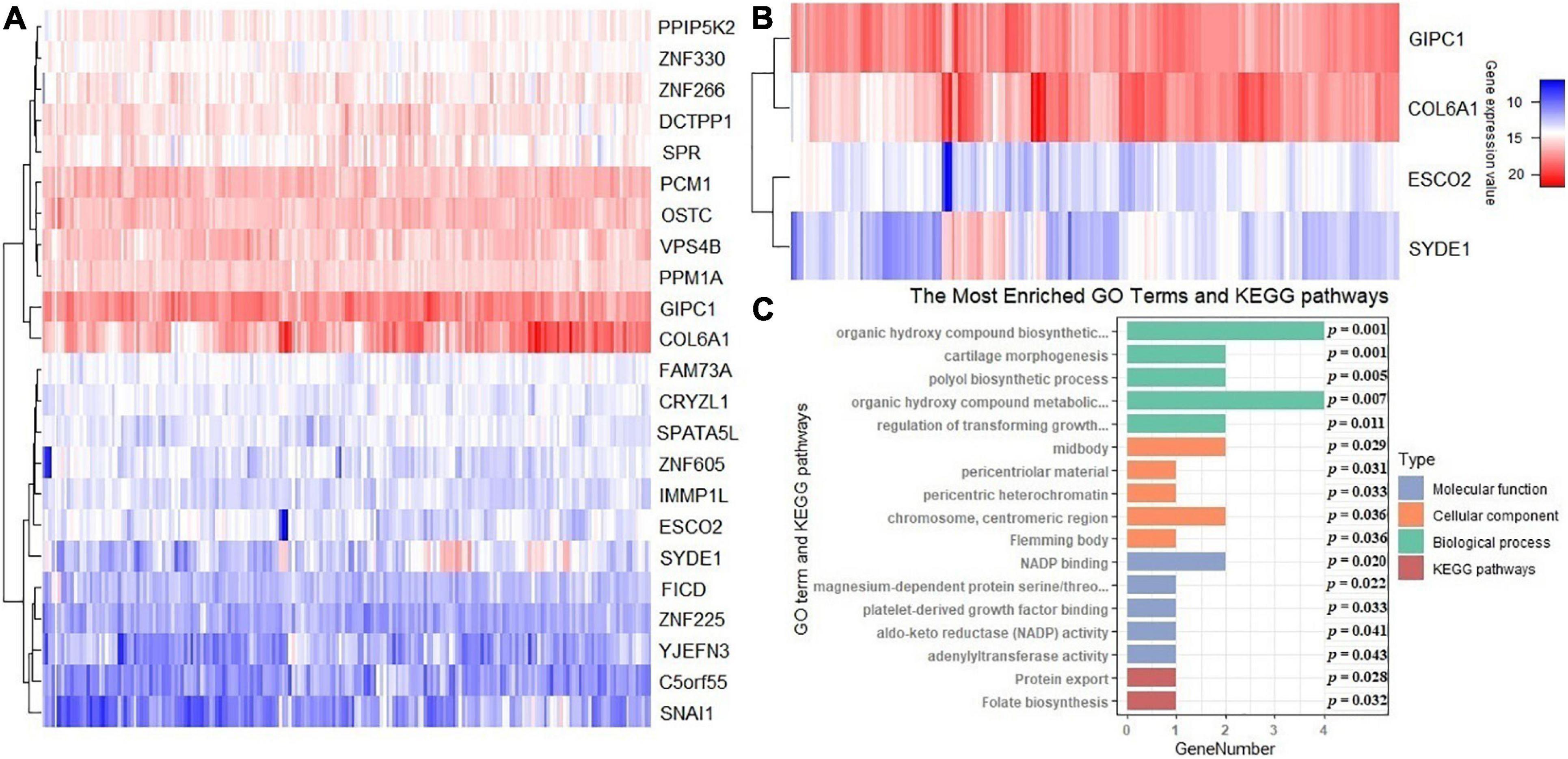
Figure 6. (A) Heatmap of expression levels for these 23 newly identified causal genes of cervical cancer. (B) Heatmap for differentially expressed genes. (C) Gene ontology (GO) and Kyoto Encyclopedia of Genes and Genomes (KEGG) pathway enrichment analyses for the 23 genes; the p-values are also shown. Count Number denotes the number of genes related to the enriched GO or KEGG pathway.
The GO biological process (BP) terms are remarkably enriched in the polyol metabolic process, regulation of biosynthetic process, and signaling pathway and chondrocyte differentiation. For the GO cellular component (CC) terms, the target genes are concentrated in the midbody, pericentriolar material, and so on. The molecular function (MF) category was focused on NADP binding, platelet-derived growth factor binding (Supplementary Table 1). The KEGG enrichment analysis indicates that these genes are remarkably enriched in tumor-associated pathways, including protein export (P = 0.028) and folate biosynthesis (P = 0.032) (Figure 6C). The combined action of folate biosynthesis and graft-vs.-host disease was demonstrated to be significantly associated with cervical cancer in suit: HLA-DPB1 (Ivansson et al., 2011). The upregulated differentially expressed genes are mostly associated with cartilage morphogenesis (ontology: BP), collagen trimer (ontology: CC), and extracellular matrix structural constituent conferring tensile strength (ontology: MF). The downregulated differentially expressed genes are mostly associated with organic hydroxy compound biosynthetic process (ontology: BP), organic hydroxy compound metabolic process (ontology: BP), and dendritic shaft (ontology: CC) (Supplementary Table 1). The functional enrichment results suggest that these newly discovered potential causal genes may participate in oncogenicity and tumor progression in cervical cancer through regulating relevant biological processes and critical pathways.
Discussion
Given the severe health threat among women and little knowledge of genetic basis for cervical cancer, persistent work should be done to discover genes that are causally related to cervical cancer (Chen and Gyllensten, 2015). The present study is one of such efforts with the aim to detect newly possible causal genes for the survival risk of cervical cancer through integrative genomic methods. The two-stage inference analysis pipeline applied in this work can be considered as a gene-centered integration approach by aggregating omics datasets and clinical information. With the growing high-throughput omics datasets in cancer research over recent years (Hoadley et al., 2018), it is well-recognized that the utilization of only one single level of genomic measurements is insufficient to completely untangle the etiology of cancer prognosis (Zhao et al., 2014; Hoadley et al., 2018). Based on the omics datasets of TCGA measured from multiple platforms, we treated the gene expression as the exposure and the survival time as the outcome to explore the possible causal genes of the survival risk of cervical cancer within the framework of the two-stage MR study to avoid the reverse causation.
One critical step in our two-stage inference is to evaluate the effect relationship between a group of DNA methylation CpG sites and the expression level for each gene. The power of the subsequent association performed in coxlmm would greatly depend on how well the prediction model utilized can capture the underlying genetic architecture of the transcriptome (Gamazon et al., 2015; Gusev et al., 2016; Zeng and Zhou, 2017; Barbeira et al., 2019; Hu et al., 2019; Wainberg et al., 2019), which can differ in the numbers, effect sizes, and effect directions of causal methylation alterations in diverse genes. Therefore, a powerful two-stage inference approach should in the first stage choose a prediction model whose prior effect distribution closely matches the true effect distribution so that it can approximate well the genetic architecture of the gene (Zhou et al., 2013; Zeng and Zhou, 2017; Zhu and Zhou, 2020). For example, if DNA methylation alterations have effect sizes following a normal distribution, then LMM-coxlmm would be more powerful; on the other hand, if only a very small fraction of DNA methylation alterations may be predictive for the gene expression, then the test with sparse prediction models (e.g., Lasso-coxlmm and ENET-coxlmm) would be superior. Due to unknown true association patterns, there is no uniformly most powerful test. As a result, the two-stage association test may perform well for one gene, but not necessarily for another.
To leverage the advantage of distinct prediction models to improve power, instead selecting an optimal prediction model, in the present study we considered a wide range of prediction models in our two-stage inference procedure. It can be imaged that the resulting p-values would be highly correlated because they are generated with the same data set following the similar logic (Figure 3). The correlation structure of these p-values also depends on the true architecture of gene expression, which however, is rarely known in advance and is likely to vary from one gene to another across the genome. Therefore, it is desirable to construct an omnibus test that integrates the advantage of multiple prediction approaches and is robust against distinct transcriptomic architectures. To achieve this aim, we exploited ACAT (Liu et al., 2019; Liu and Xie, 2020) to combine these correlated p-values and integrating individual strengths of various tests. As illustrated in our empirical application, ACAT achieves relatively higher power since it aggregates genetic association information across different tests.
Compared to previous similar methods, the proposed two-stage inference approach differs in three aspects. First, unlike prediXcan (Gamazon et al., 2015) we constructed the two-stage inference procedure in one sample, leading to the so-called one-sample two-stage regression (Xue et al., 2020). Second, multiple competing prediction models rather than a single model were utilized and combined with ACAT which was p-value calibrated (Liu et al., 2019; Sun et al., 2020; Xiao et al., 2020). Thus, our strategy often has higher power compared to the test with the single prediction model. Third, due to widespread pleiotropic effects in omics (Bowden et al., 2015, 2016; Burgess and Thompson, 2017; Slob et al., 2017; Barfield et al., 2018; Verbanck et al., 2018), we also considered the direct influence of methylations. Therefore, our results would be robust against the bias of pleiotropy of instrumental variables that are commonly encountered in MR.
However, the present study is not without limitation. First, these newly identified methylation-regulated genes were detected only in TCGA; no external relevant expression profiles were applied for validation. Second, we only employed methylations as instrumental variables; other omic measurements that regulate gene expression (e.g., genetic variants; Manor and Segal, 2013, 2015; Zeng et al., 2017) can be also simultaneously incorporated to further improve power. Third, we only utilized local methylation CpG sites of a gene as candidate instruments. It is not known whether the power can be further enhanced if the global methylation CpG sites are exploited. Fourth, the present study assumed a linear relationship for each methylation–expression pair. While a linear relationship can be methodologically interpreted as a first-order approximation to nonlinear relationship (Zhou et al., 2013), modeling a linear relationship may be suboptimal and suffer from power loss if the true relationship is nonlinear. Fifth, due to the complicated standard error structures for those prediction models, in terms of the assumption of no measurement error (NOME) (Bowden et al., 2016), we did not incorporate the uncertainty in the estimated effect sizes of methylations into our two-stage approach, although such uncertainty may be important in integrative genomic causal inference (Yeung et al., 2019; Yuan et al., 2020). Actually, we note that many previous two-stage MR studies or TWAS approaches followed this NOME principle (Gamazon et al., 2015; Bowden et al., 2016; Gusev et al., 2016; Zeng and Zhou, 2017).
Conclusion
In summary, using the proposed two-stage causal inference approach within the framework of MR analysis, we discovered a total of 14 potential causal genes which were associated with the survival risk of cervical cancer patients when separately applying five commonly used prediction models. The number of possible causal genes was brought to 23 when employing the combination method of ACAT. Some of these genes were differentially expressed between tumor and normal tissue and were enriched in tumor-associated pathways. Our findings provide new insights into the genetic etiology of the survival risk of cervical cancer and suggest possibly potential therapeutic targets for cervical cancer in the future.
Data Availability Statement
The original contributions presented in the study are included in the article/Supplementary Material, further inquiries can be directed to the corresponding author/s.
Author Contributions
PZ conceived the idea for the study. PZ, JZ, HL, FG, TW, and SZ obtained the data and performed the data analyses. PZ, JZ, HL, HZ, and SZ interpreted the results of the data analyses. PZ, FG, JZ, and HL wrote the manuscript with the participation of all authors.
Funding
This work was supported by the Youth Foundation of Humanity and Social Science funded by the Ministry of Education of China (18YJC910002), the Natural Science Foundation of Jiangsu Province of China (BK20181472), the China Postdoctoral Science Foundation (2018M630607 and 2019T120465), the QingLan Research Project of Jiangsu Province for Outstanding Young Teachers, the Six-Talent Peaks Project in Jiangsu Province of China (WSN-087), the Training Project for Youth Teams of Science and Technology Innovation at Xuzhou Medical University (TD202008), the Postdoctoral Science Foundation of Xuzhou Medical University, the National Natural Science Foundation of China (81402765), and the Statistical Science Research Project from National Bureau of Statistics of China (2014LY112). The research of TW was supported in part by the Social Development Project of Xuzhou City (KC20062).
Conflict of Interest
The authors declare that the research was conducted in the absence of any commercial or financial relationships that could be construed as a potential conflict of interest.
Acknowledgments
TCGA datasets were publicly available from https://xenabrowser.net/. The data analyses in the present study were carried out with the high-performance computing cluster that was supported by the special central finance project of local universities for Xuzhou Medical University. We are also very grateful to two reviewers for their constructive comments which improved our manuscript substantially.
Supplementary Material
The Supplementary Material for this article can be found online at: https://www.frontiersin.org/articles/10.3389/fgene.2021.667877/full#supplementary-material
Abbreviations
BP, biological process; BSLMM, Bayesian sparse linear mixed model; Coxlmm, Cox linear mixed-effect model; CC, cellular component; DEG, differential expressed genes; DPR, latent Dirichlet Process Regression; EBI, the European Bioinformatics Institute; ENET, elastic net; FDR, false discovery rate; GO, Gene Ontology; GWAS, genome-wide association study; ACAT, aggregated Cauchy association test; HPV, human papillomavirus; KGEE, Kyoto Encyclopedia of Genes and Genomes; LMM, Linear mixed model; MF, molecular function; MR, Mendelian randomization; TCGA, The Cancer Genome Atlas; TWAS, transcriptome-wide association study.
References
Angrist, J. D., Imbens, G. W., and Rubin, D. B. (1996). Identification of causal effects using instrumental variables. J. Am. Stat. Assoc. 91, 444–455. doi: 10.1080/01621459.1996.10476902
Angrist, J. D., and Keueger, A. B. (1991). Does compulsory school attendance affect schooling and earnings? Q. J. Econ. 106, 979–1014. doi: 10.2307/2937954
Barbeira, A. N., Pividori, M., Zheng, J., Wheeler, H. E., Nicolae, D. L., and Im, H. K. (2019). Integrating predicted transcriptome from multiple tissues improves association detection. PLoS Genet. 15:e1007889. doi: 10.1371/journal.pgen.1007889
Barfield, R., Feng, H., Gusev, A., Wu, L., Zheng, W., Pasaniuc, B., et al. (2018). Transcriptome-wide association studies accounting for colocalization using Egger regression. Genet. Epidemiol. 42, 418–433. doi: 10.1002/gepi.22131
Bowden, J., Davey Smith, G., and Burgess, S. (2015). Mendelian randomization with invalid instruments: effect estimation and bias detection through Egger regression. Int. J. Epidemiol. 44, 512–525. doi: 10.1093/ije/dyv080
Bowden, J., Del Greco, M. F., Minelli, C., Davey Smith, G., Sheehan, N. A., and Thompson, J. R. (2016). Assessing the suitability of summary data for two-sample Mendelian randomization analyses using MR-Egger regression: the role of the I2 statistic. Int. J. Epidemiol. 45, 1961–1974. doi: 10.1093/ije/dyw220
Bray, F., Ferlay, J., Soerjomataram, I., Siegel, R. L., Torre, L. A., and Jemal, A. (2018). Global cancer statistics 2018: GLOBOCAN estimates of incidence and mortality worldwide for 36 cancers in 185 countries. CA Cancer J. Clin. 68, 394–424. doi: 10.3322/caac.21492
Broniarczyk, J., Pim, D., Massimi, P., Bergant, M., Goździcka-Józefiak, A., Crump, C., et al. (2017). The VPS4 component of the ESCRT machinery plays an essential role in HPV infectious entry and capsid disassembly. Sci. Rep. 7:45159. doi: 10.1038/srep45159
Burgess, S., and Thompson, S. G. (2017). Interpreting findings from Mendelian randomization using the MR-Egger method. Eur. J. Epidemiol. 32, 377–389. doi: 10.1007/s10654-017-0255-x
Burri, L., Strahm, Y., Hawkins, C. J., Gentle, I. E., Puryer, M. A., Verhagen, A., et al. (2005). Mature DIABLO/Smac is produced by the IMP protease complex on the mitochondrial inner membrane. Mol. Biol. Cell 16, 2926–2933. doi: 10.1091/mbc.e04-12-1086
Chen, D., Cui, T., Ek, W. E., Liu, H., Wang, H., and Gyllensten, U. (2015). Analysis of the genetic architecture of susceptibility to cervical cancer indicates that common SNPs explain a large proportion of the heritability. Carcinogenesis 36, 992–998. doi: 10.1093/carcin/bgv083
Chen, D., Enroth, S., Liu, H., Sun, Y., Wang, H. B., Yu, M., et al. (2016). Pooled analysis of genome-wide association studies of cervical intraepithelial neoplasia 3 (CIN3) identifies a new susceptibility locus. Oncotarget 7, 42216–42224. doi: 10.18632/oncotarget.9916
Chen, D., and Gyllensten, U. (2015). Lessons and implications from association studies and post-GWAS analyses of cervical cancer. Trends Genet. 31, 41–54. doi: 10.1016/j.tig.2014.10.005
Chen, D., Juko-Pecirep, I., Hammer, J., Ivansson, E., Enroth, S., Gustavsson, I., et al. (2013a). Genome-wide association study of susceptibility loci for cervical cancer. J. Natl. Cancer Inst. 105, 624–633. doi: 10.1093/jnci/djt051
Chen, Z., Li, S., Huang, K., Zhang, Q., Wang, J., Li, X., et al. (2013b). The nuclear protein expression levels of SNAI1 and ZEB1 are involved in the progression and lymph node metastasis of cervical cancer via the epithelial-mesenchymal transition pathway. Hum. Pathol. 44, 2097–2105. doi: 10.1016/j.humpath.2013.04.001
Chu, S. H., and Huang, Y. T. (2017). Integrated genomic analysis of biological gene sets with applications in lung cancer prognosis. BMC Bioinformatics 18:336. doi: 10.1186/s12859-017-1737-2
Das, P., Bansal, A., Rao, S. N., Deodhar, K., Mahantshetty, U., Shrivastava, S. K., et al. (2016). Somatic variations in cervical cancers in Indian patients. PLoS One 11:e0165878. doi: 10.1371/journal.pone.0165878
Davey Smith, G., and Ebrahim, S. (2003). ‘Mendelian randomization’: can genetic epidemiology contribute to understanding environmental determinants of disease? Int. J. Epidemiol. 32, 1–22. doi: 10.1093/ije/dyg070
de Tayrac, M., Lê, S., Aubry, M., Mosser, J., and Husson, F. (2009). Simultaneous analysis of distinct Omics data sets with integration of biological knowledge: multiple factor analysis approach. BMC Genomics 10:32. doi: 10.1186/1471-2164-10-32
Deng, S., Ma, J., Zhang, L., Chen, F., Sang, Z., Jia, Z., et al. (2019). De novo transcriptome sequencing and gene expression profiling of Magnolia wufengensis in response to cold stress. BMC Plant Biol. 19:321. doi: 10.1186/s12870-019-1933-5
Fabiani, E., Leone, G., Giachelia, M., D’Alo, F., Greco, M., Criscuolo, M., et al. (2010). Analysis of genome-wide methylation and gene expression induced by 5-aza-2’-deoxycytidine identifies BCL2L10 as a frequent methylation target in acute myeloid leukemia. Leuk. Lymphoma 51, 2275–2284. doi: 10.3109/10428194.2010.528093
Fisher, R. A. (1934). Statistical Methods for Research Workers: Biological Monographs and Manuals, 5th Edn. Edinburgh: Oliver and Boyd Ltd.
Fridy, P. C., Otto, J. C., Dollins, D. E., and York, J. D. (2007). Cloning and characterization of two human VIP1-like inositol hexakisphosphate and diphosphoinositol pentakisphosphate kinases. J. Biol. Chem. 282, 30754–30762. doi: 10.1074/jbc.M704656200
Friedman, J., Hastie, T., and Tibshirani, R. (2010). Regularization paths for generalized linear models via coordinate descent. J. Stat. Softw. 33, 1–22.
Gamazon, E. R., Wheeler, H. E., Shah, K. P., Mozaffari, S. V., Aquino-Michaels, K., Carroll, R. J., et al. (2015). A gene-based association method for mapping traits using reference transcriptome data. Nat. Genet. 47, 1091–1098. doi: 10.1038/ng.3367
Gao, J., Zhang, L., Liu, Z., Yao, S., Gao, S., and Wang, L. (2019). Effect of miR-135b inhibitor on biological characteristics of osteosarcoma cells through up-regulating PPM1A. Int. J. Clin. Exp. Pathol. 12, 689–699.
Glinsky, G. V. (2006). Integration of HapMap-based SNP pattern analysis and gene expression profiling reveals common SNP profiles for cancer therapy outcome predictor genes∗. Cell Cycle 5, 2613–2625. doi: 10.4161/cc.5.22.3498
Green, E. S. (2019). Analysis of HPV16 Variants in the Carolina Women’s Care Study and a Comparison of Gene Expression Profiles of Exfoliated Cervical Cells From Women Who Either Clear or Do Not Clear an HPV16 Infection. Doctoral dissertation. Columbia, SC: University of South Carolina.
Greenland, S. (2000). An introduction to instrumental variables for epidemiologists. Int. J. Epidemiol. 29, 722–729. doi: 10.1093/ije/29.4.722
Gusev, A., Ko, A., Shi, H., Bhatia, G., Chung, W., Penninx, B. W., et al. (2016). Integrative approaches for large-scale transcriptome-wide association studies. Nat. Genet. 48, 245–252. doi: 10.1038/ng.3506
Güzel, C., Govorukhina, N. I., Wisman, G. B. A., Stingl, C., Dekker, L. J. M., Klip, H. G., et al. (2018). Proteomic alterations in early stage cervical cancer. Oncotarget 9, 18128–18147. doi: 10.18632/oncotarget.24773
Hannon, E., Gorrie-Stone, T. J., Smart, M. C., Burrage, J., Hughes, A., Bao, Y., et al. (2018). Leveraging DNA-methylation quantitative-trait loci to characterize the relationship between methylomic variation, gene expression, and complex traits. Am. J. Hum. Genet. 103, 654–665. doi: 10.1016/j.ajhg.2018.09.007
Hoadley, K. A., Yau, C., Hinoue, T., Wolf, D. M., Lazar, A. J., Drill, E., et al. (2018). Cell-of-origin patterns dominate the molecular classification of 10,000 tumors from 33 types of cancer. Cell 173, 291–304.e6. doi: 10.1016/j.cell.2018.03.022
Hou, T., Tong, C., Kazobinka, G., Zhang, W., Huang, X., Huang, Y., et al. (2016). Expression of COL6A1 predicts prognosis in cervical cancer patients. Am. J. Transl. Res. 8, 2838–2844.
Hu, Y., Li, M., Lu, Q., Weng, H., Wang, J., Zekavat, S. M., et al. (2019). A statistical framework for cross-tissue transcriptome-wide association analysis. Nat. Genet. 51, 568–576. doi: 10.1038/s41588-019-0345-7
Huang, H. S., Buck, C. B., and Lambert, P. F. (2010). Inhibition of gamma secretase blocks HPV infection. Virology 407, 391–396. doi: 10.1016/j.virol.2010.09.002
Huang, Y. T. (2019). Variance component tests of multivariate mediation effects under composite null hypotheses. Biometrics 75, 1191–1204. doi: 10.1111/biom.13073
Ivansson, E. L., Juko-Pecirep, I., Erlich, H. A., and Gyllensten, U. B. (2011). Pathway-based analysis of genetic susceptibility to cervical cancer in situ: HLA-DPB1 affects risk in Swedish women. Genes Immun. 12, 605–614. doi: 10.1038/gene.2011.40
Katoh, M. (2013). Functional proteomics, human genetics and cancer biology of GIPC family members. Exp. Mol. Med. 45:e26. doi: 10.1038/emm.2013.49
Kim, Y., Kang, Y. S., and Seok, J. (2018). GAIT: gene expression analysis for interval time. Bioinformatics 34, 2305–2307. doi: 10.1093/bioinformatics/bty111
Kingsley, S. L., Eliot, M. N., Whitsel, E. A., Huang, Y. T., Kelsey, K. T., Marsit, C. J., et al. (2016). Maternal residential proximity to major roadways, birth weight, and placental DNA methylation. Environ. Int. 9, 43–49. doi: 10.1016/j.envint.2016.03.020
Lee, S., Kim, M. G., Ahn, H., and Kim, S. (2020). Inositol pyrophosphates: signaling molecules with pleiotropic actions in mammals. Molecules 25:2208. doi: 10.3390/molecules25092208
Leo, P. J., Madeleine, M. M., Wang, S., Schwartz, S. M., Newell, F., Pettersson-Kymmer, U., et al. (2017). Defining the genetic susceptibility to cervical neoplasia- a genome-wide association study. PLoS Genet. 13:e1006866. doi: 10.1371/journal.pgen.1006866
Leung, C. O., Deng, W., Ye, T. M., Ngan, H. Y., Tsao, S. W., Cheung, A. N., et al. (2014). miR-135a leads to cervical cancer cell transformation through regulation of β-catenin via a SIAH1-dependent ubiquitin proteosomal pathway. Carcinogenesis 35, 1931–1940. doi: 10.1093/carcin/bgu032
Li, C. I., Su, P. F., and Shyr, Y. (2013). Sample size calculation based on exact test for assessing differential expression analysis in RNA-seq data. BMC Bioinformatics 14:357. doi: 10.1186/1471-2105-14-357
Li, P., Guo, H., Zhou, G., Shi, H., Li, Z., Guan, X., et al. (2018). Increased ZNF84 expression in cervical cancer. Arch. Gynecol. Obstet. 297, 1525–1532. doi: 10.1007/s00404-018-4770-0
Li, Z., Li, X., Liu, Y., Shen, J., Chen, H., Zhou, H., et al. (2019). Dynamic scan procedure for detecting rare-variant association regions in whole-genome sequencing studies. Am. J. Hum. Genet. 104, 802–814. doi: 10.1016/j.ajhg.2019.03.002
Liu, L., Zeng, P., Xue, F., Yuan, Z., and Zhou, X. (2021). Multi-trait transcriptome-wide association studies with probabilistic Mendelian randomization. Am. J. Hum. Genet. 108, 240–256. doi: 10.1016/j.ajhg.2020.12.006
Liu, L., Zeng, P., Yang, S., and Yuan, Z. (2020). Leveraging methylation to identify the potential causal genes associated with survival in lung adenocarcinoma and lung squamous cell carcinoma. Oncol. Lett. 20, 193–200. doi: 10.3892/ol.2020.11564
Liu, Y., Chen, S., Li, Z., Morrison, A. C., Boerwinkle, E., and Lin, X. (2019). ACAT: a fast and powerful p value combination method for rare-variant analysis in sequencing studies. Am. J. Hum. Genet. 104, 410–421. doi: 10.1016/j.ajhg.2019.01.002
Liu, Y., and Xie, J. (2020). Cauchy combination test: a powerful test with analytic p-value calculation under arbitrary dependency structures. J. Am. Stat. Assoc. 115, 393–402. doi: 10.1080/01621459.2018.1554485
Loucks, E. B., Huang, Y. T., Agha, G., Chu, S., Eaton, C. B., Gilman, S. E., et al. (2016). Epigenetic mediators between childhood socioeconomic disadvantage and mid-life body mass index: the New England family study. Psychosom. Med. 78, 1053–1065. doi: 10.1097/psy.0000000000000411
Magnusson, P. K. E., Lichtenstein, P., and Gyllensten, U. B. (2000). Heritability of cervical tumours. Int. J. Cancer 88, 698–701. doi: 10.1002/1097-0215(20001201)88:5<698::aid-ijc3<3.0.co;2-j
Makowsky, R., Pajewski, N. M., Klimentidis, Y. C., Vazquez, A. I., Duarte, C. W., Allison, D. B., et al. (2011). Beyond missing heritability: prediction of complex traits. PLoS Genet. 7:e1002051. doi: 10.1371/journal.pgen.1002051
Manor, O., and Segal, E. (2013). Robust prediction of expression differences among human individuals using only genotype information. PLoS Genet. 9:e1003396. doi: 10.1371/journal.pgen.1003396
Manor, O., and Segal, E. (2015). GenoExp: a web tool for predicting gene expression levels from single nucleotide polymorphisms. Bioinformatics 31, 1848–1850. doi: 10.1093/bioinformatics/btv050
Martinez-Ruiz, G., Maldonado, V., Ceballos-Cancino, G., Grajeda, J. P., and Melendez-Zajgla, J. (2008). Role of Smac/DIABLO in cancer progression. J. Exp. Clin. Cancer Res. 27:48. doi: 10.1186/1756-9966-27-48
Masuda, T., Low, S. K., Akiyama, M., Hirata, M., Ueda, Y., Matsuda, K., et al. (2020). GWAS of five gynecologic diseases and cross-trait analysis in Japanese. Eur. J. Hum. Genet. 28, 95–107. doi: 10.1038/s41431-019-0495-1
McCarthy, D. J., Chen, Y., and Smyth, G. K. (2012). Differential expression analysis of multifactor RNA-Seq experiments with respect to biological variation. Nucleic Acids Res. 40, 4288–4297. doi: 10.1093/nar/gks042
Meng, L., Dian, F., Yinan, X., Hao, Z., and Dingyue, Z. (2020). Anticancer effect of natural product sulforaphane by targeting MAPK signal through miRNA-1247-3p in human cervical cancer cells. Biointerface Res. Appl. Chem. 11, 7943–7972. doi: 10.33263/BRIAC111.79437972
Miura, K., Mishima, H., Kinoshita, A., Hayashida, C., Abe, S., Tokunaga, K., et al. (2014). Genome-wide association study of HPV-associated cervical cancer in Japanese women. J. Med. Virol. 86, 1153–1158. doi: 10.1002/jmv.23943
Nahand, J. S., Taghizadeh-Boroujeni, S., Karimzadeh, M., Borran, S., Pourhanifeh, M. H., Moghoofei, M., et al. (2019). microRNAs: new prognostic, diagnostic, and therapeutic biomarkers in cervical cancer. J. Cell. Physiol. 234, 17064–17099. doi: 10.1002/jcp.28457
Qi, T., Wu, Y., Zeng, J., Zhang, F., Xue, A., Jiang, L., et al. (2018). Identifying gene targets for brain-related traits using transcriptomic and methylomic data from blood. Nat. Commun. 9:2282. doi: 10.1038/s41467-018-04558-1
Rice, K. (2010). A decision-theoretic formulation of Fisher’s approach to testing. Am. Stat. 64, 345–349. doi: 10.1198/tast.2010.09060
Robinson, M. D., McCarthy, D. J., and Smyth, G. K. (2010). edgeR: a Bioconductor package for differential expression analysis of digital gene expression data. Bioinformatics 26, 139–140. doi: 10.1093/bioinformatics/btp616
Robinson, M. D., and Smyth, G. K. (2008). Small-sample estimation of negative binomial dispersion, with applications to SAGE data. Biostatistics 9, 321–332. doi: 10.1093/biostatistics/kxm030
Rudolph, C., Sigruener, A., Hartmann, A., Orso, E., Bals-Pratsch, M., Gronwald, W., et al. (2007). ApoA-I-binding protein (AI-BP) and its homologues hYjeF_N2 and hYjeF_N3 comprise the YjeF_N domain protein family in humans with a role in spermiogenesis and oogenesis. Horm. Metab. Res. 39, 322–335. doi: 10.1055/s-2007-977699
Šarenac, T., and Mikov, M. (2019). Cervical cancer, different treatments and importance of bile acids as therapeutic agents in this disease. Front. Pharmacol. 10:484. doi: 10.3389/fphar.2019.00484
Sheehan, N. A., Didelez, V., Burton, P. R., and Tobin, M. D. (2008). Mendelian randomisation and causal inference in observational epidemiology. PLoS Med. 5:e177. doi: 10.1371/journal.pmed.0050177
Shi, Y., Li, L., Hu, Z., Li, S., Wang, S., Liu, J., et al. (2013). A genome-wide association study identifies two new cervical cancer susceptibility loci at 4q12 and 17q12. Nat. Genet. 45, 918–922. doi: 10.1038/ng.2687
Siegel, R. L., Miller, K. D., and Jemal, A. (2019). Cancer statistics, 2019. CA Cancer J. Clin. 69, 7–34. doi: 10.3322/caac.21551
Slob, E. A. W., Groenen, P. J. F., Thurik, A. R., and Rietveld, C. A. (2017). A note on the use of Egger regression in Mendelian randomization studies. Int. J. Epidemiol. 46, 2094–2097. doi: 10.1093/ije/dyx191
Sun, S., Zhu, J., and Zhou, X. (2020). Statistical analysis of spatial expression patterns for spatially resolved transcriptomic studies. Nat. Methods 17, 193–200. doi: 10.1038/s41592-019-0701-7
Takeuchi, F., Kukimoto, I., Li, Z., Li, S., Li, N., Hu, Z., et al. (2019). Genome-wide association study of cervical cancer suggests a role for ARRDC3 gene in human papillomavirus infection. Hum. Mol. Genet. 28, 341–348. doi: 10.1093/hmg/ddy390
The Cancer Genome Atlas Research Network (2017). Integrated genomic and molecular characterization of cervical cancer. Nature 543, 378–384. doi: 10.1038/nature21386
Therneau, T. M. (2019). coxme: Mixed Effects Cox Models. R Package Version 2.2-14. Available online at: https://CRAN.R-project.org/package=coxme
Therneau, T. M., Grambsch, P. M., and Pankratz, V. S. (2003). Penalized survival models and frailty. J. Comput. Graph. Stat. 12, 156–175.
Tibshirani, R. (1996). Regression shrinkage and selection via the lasso. J. R. Stat. Soc. B 58, 267–288. doi: 10.1111/j.2517-6161.1996.tb02080.x
Verbanck, M., Chen, C.-Y., Neale, B., and Do, R. (2018). Detection of widespread horizontal pleiotropy in causal relationships inferred from Mendelian randomization between complex traits and diseases. Nat. Genet. 50, 693–698. doi: 10.1038/s41588-018-0099-7
Visscher, P. M., Hill, W. G., and Wray, N. R. (2008). Heritability in the genomics era–concepts and misconceptions. Nat. Rev. Genet. 9, 255–266. doi: 10.1038/nrg2322
Visscher, P. M., Wray, N. R., Zhang, Q., Sklar, P., McCarthy, M. I., Brown, M. A., et al. (2017). 10 years of GWAS discovery: biology, function, and translation. Am. J. Hum. Genet. 101, 5–22. doi: 10.1016/j.ajhg.2017.06.005
Wainberg, M., Sinnott-Armstrong, N., Mancuso, N., Barbeira, A. N., Knowles, D. A., Golan, D., et al. (2019). Opportunities and challenges for transcriptome-wide association studies. Nat. Genet. 51, 592–599. doi: 10.1038/s41588-019-0385-z
Wang, W., Baladandayuthapani, V., Morris, J. S., Broom, B. M., Manyam, G., and Do, K. A. (2013). iBAG: integrative Bayesian analysis of high-dimensional multiplatform genomics data. Bioinformatics 29, 149–159. doi: 10.1093/bioinformatics/bts655
Wang, X., Wang, Y., Zhang, Z., Huang, M., Fei, Y., Ma, J., et al. (2020). Discriminating different grades of cervical intraepithelial neoplasia based on label-free phasor fluorescence lifetime imaging microscopy. Biomed. Opt. Express 11, 1977–1990. doi: 10.1364/boe.386999
White, P. W., Faucher, A. M., Massariol, M. J., Welchner, E., Rancourt, J., Cartier, M., et al. (2005). Biphenylsulfonacetic acid inhibitors of the human papillomavirus type 6 E1 helicase inhibit ATP hydrolysis by an allosteric mechanism involving tyrosine 486. Antimicrob. Agents Chemother. 49, 4834–4842. doi: 10.1128/aac.49.12.4834-4842.2005
Wilson, C. M., Magnaudeix, A., Yardin, C., and Terro, F. (2011). DC2 and keratinocyte-associated protein 2 (KCP2), subunits of the oligosaccharyltransferase complex, are regulators of the gamma-secretase-directed processing of amyloid precursor protein (APP). J. Biol. Chem. 286, 31080–31091. doi: 10.1074/jbc.M111.249748
Wu, Y., Zeng, J., Zhang, F., Zhu, Z., Qi, T., Zheng, Z., et al. (2018). Integrative analysis of omics summary data reveals putative mechanisms underlying complex traits. Nat. Commun. 9:918. doi: 10.1038/s41467-018-03371-0
Xiao, L., Yuan, Z., Jin, S., Wang, T., Huang, S., and Zeng, P. (2020). Multiple-tissue integrative transcriptome-wide association studies discovered new genes associated with amyotrophic lateral sclerosis. Front. Genet. 11:587243. doi: 10.3389/fgene.2020.587243
Xue, H., Pan, W., and Alzheimer’s Disease Neuroimaging Initiative (2020). Some statistical consideration in transcriptome-wide association studies. Genet. Epidemiol. 44, 221–232. doi: 10.1002/gepi.22274
Yang, J., Benyamin, B., McEvoy, B. P., Gordon, S., Henders, A. K., Nyholt, D. R., et al. (2010). Common SNPs explain a large proportion of the heritability for human height. Nat. Genet. 42, 565–569. doi: 10.1038/ng.608
Yang, J., Manolio, T. A., Pasquale, L. R., Boerwinkle, E., Caporaso, N., Cunningham, J. M., et al. (2011). Genome partitioning of genetic variation for complex traits using common SNPs. Nat. Genet. 43, 519–525. doi: 10.1038/ng.823
Yeung, K.-F., Yang, Y., Yang, C., and Liu, J. (2019). CoMM: a collaborative mixed model that integrates GWAS and eQTL data sets to investigate the genetic architecture of complex traits. Bioinform. Biol. Insights 13:1177932219881435. doi: 10.1177/1177932219881435
Yu, G., Wang, L. G., Han, Y., and He, Q. Y. (2012). clusterProfiler: an R package for comparing biological themes among gene clusters. OMICS 16, 284–287. doi: 10.1089/omi.2011.0118
Yu, X., Wang, T., Huang, S., and Zeng, P. (2020). How can gene expression information improve prognostic prediction in TCGA cancers: an empirical comparison study on regularization and mixed-effect survival models. Front. Genet. 11:920. doi: 10.3389/fgene.2020.00920
Yuan, Z., Zhu, H., Zeng, P., Yang, S., Sun, S., Yang, C., et al. (2020). Testing and controlling for horizontal pleiotropy with probabilistic Mendelian randomization in transcriptome-wide association studies. Nat. Commun. 11:3861. doi: 10.1038/s41467-020-17668-6
Zeng, P., Dai, J., Jin, S., and Zhou, X. (2021). Aggregating multiple expression prediction models improves the power of transcriptome wide association studies. Hum. Mol. Genet. ddab056. doi: 10.1093/hmg/ddab056
Zeng, P., Wang, T., Zheng, J., and Zhou, X. (2019). Causal association of type 2 diabetes with amyotrophic lateral sclerosis: new evidence from Mendelian randomization using GWAS summary statistics. BMC Med. 17:225. doi: 10.1186/s12916-019-1448-9
Zeng, P., and Zhou, X. (2017). Non-parametric genetic prediction of complex traits with latent Dirichlet process regression models. Nat. Commun. 8:456. doi: 10.1038/s41467-017-00470-2
Zeng, P., and Zhou, X. (2019a). Causal association between birth weight and adult diseases: evidence from a Mendelian randomization analysis. Front. Genet. 10:618. doi: 10.3389/fgene.2019.00618
Zeng, P., and Zhou, X. (2019b). Causal effects of blood lipids on amyotrophic lateral sclerosis: a Mendelian randomization study. Hum. Mol. Genet. 28, 688–697. doi: 10.1093/hmg/ddy384
Zeng, P., Zhou, X., and Huang, S. (2017). Prediction of gene expression with cis-SNPs using mixed models and regularization methods. BMC Genomics 18:368. doi: 10.1186/s12864-017-3759-6
Zhang, Y., Ye, W. Y., Wang, J. Q., Wang, S. J., Ji, P., Zhou, G. Y., et al. (2013). dCTP pyrophosphohydrase exhibits nucleic accumulation in multiple carcinomas. Eur. J. Histochem. 57:e29. doi: 10.4081/ejh.2013.e29
Zhao, H., Ljungberg, B., Grankvist, K., Rasmuson, T., Tibshirani, R., and Brooks, J. D. (2006). Gene expression profiling predicts survival in conventional renal cell carcinoma. PLoS Med. 3:e13. doi: 10.1371/journal.pmed.0030013
Zhao, Q., Shi, X., Xie, Y., Huang, J., Shia, B., and Ma, S. (2014). Combining multidimensional genomic measurements for predicting cancer prognosis: observations from TCGA. Brief. Bioinform. 16, 291–303. doi: 10.1093/bib/bbu003
Zhou, X., Carbonetto, P., and Stephens, M. (2013). Polygenic modeling with bayesian sparse linear mixed models. PLoS Genet. 9:e1003264. doi: 10.1371/journal.pgen.1003264
Zhu, B., Song, N., Shen, R., Arora, A., Machiela, M. J., Song, L., et al. (2017). Integrating clinical and multiple Omics data for prognostic assessment across human cancers. Sci. Rep. 7:16954. doi: 10.1038/s41598-017-17031-8
Zhu, H., and Zhou, X. (2020). Transcriptome-wide association studies: a view from Mendelian randomization. Quant. Biol. 1–15.
Keywords: Cox linear mixed-effects model, prediction model, gene expression, DNA methylation, two-stage inference, potential causal gene, cervical cancer, aggregated Cauchy association test
Citation: Zhang J, Lu H, Zhang S, Wang T, Zhao H, Guan F and Zeng P (2021) Leveraging Methylation Alterations to Discover Potential Causal Genes Associated With the Survival Risk of Cervical Cancer in TCGA Through a Two-Stage Inference Approach. Front. Genet. 12:667877. doi: 10.3389/fgene.2021.667877
Received: 15 February 2021; Accepted: 19 April 2021;
Published: 02 June 2021.
Edited by:
Tao Wang, Medical College of Wisconsin, United StatesReviewed by:
Xiaoqing Pan, Shanghai Normal University, ChinaHanfei Xu, Boston University, United States
Copyright © 2021 Zhang, Lu, Zhang, Wang, Zhao, Guan and Zeng. This is an open-access article distributed under the terms of the Creative Commons Attribution License (CC BY). The use, distribution or reproduction in other forums is permitted, provided the original author(s) and the copyright owner(s) are credited and that the original publication in this journal is cited, in accordance with accepted academic practice. No use, distribution or reproduction is permitted which does not comply with these terms.
*Correspondence: Huashuo Zhao, aHN6aGFvQHh6aG11LmVkdS5jbg==; Fengjun Guan, Z3VhbnhpYW9tdUBzaW5hLmNvbQ==; Ping Zeng, enBzdGF0QHh6aG11LmVkdS5jbg==
†These authors have contributed equally to this work and share first authorship