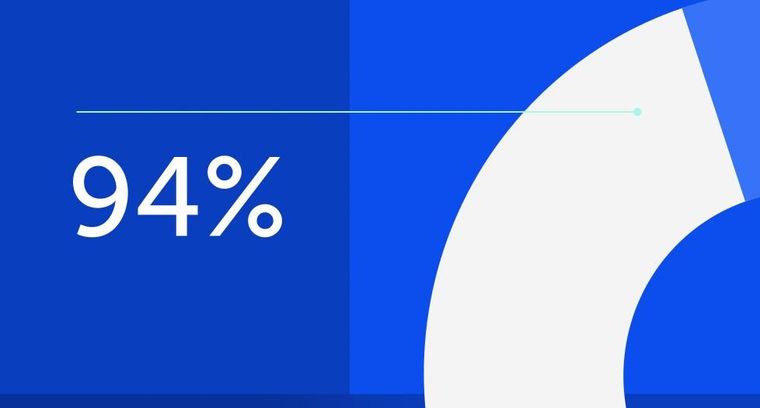
94% of researchers rate our articles as excellent or good
Learn more about the work of our research integrity team to safeguard the quality of each article we publish.
Find out more
ORIGINAL RESEARCH article
Front. Genet., 29 July 2021
Sec. Livestock Genomics
Volume 12 - 2021 | https://doi.org/10.3389/fgene.2021.662143
This article is part of the Research TopicSheep and Goat Gene ExplorationView all 19 articles
Many local sheep breeds in China have poor meat quality. Increasing intramuscular fat (IMF) content can significantly improve the quality of mutton. However, the molecular mechanisms of intramuscular adipocyte formation and differentiation remain unclear. This study compared differences between preadipocytes and mature adipocytes by whole-transcriptome sequencing and constructed systematically regulatory networks according to the relationship predicted among the differentially expressed RNAs (DERs). Sequencing results showed that in this process, there were 1,196, 754, 100, and 17 differentially expressed messenger RNAs (mRNAs), long non-coding RNAs (lncRNAs), microRNAs (miRNAs), and circular RNAs (circRNAs), respectively. Gene Ontology analysis showed that most DERs enriched in Cell Part, Cellular Process, Biological Regulation, and Binding terms. Kyoto Encyclopedia of Genes and Genomes (KEGG) analysis found that the DERs primarily focused on Focal adhesion, phosphoinositide 3-kinase (PI3K)-Akt, mitogen-activated protein kinase (MAPK), peroxisome proliferator-activated receptor (PPAR) signaling pathways. Forty (40) DERs were randomly selected from the core regulatory network to verify the accuracy of the sequence data. The results of qPCR showed that the DER expression trend was consistent with sequence data. Four novel promising candidate miRNAs (miR-336, miR-422, miR-578, and miR-722) played crucial roles in adipocyte differentiation, and they also participated in multiple and important regulatory networks. We verified the expression pattern of the miRNAs and related pathways’ members at five time points in the adipocyte differentiation process (0, 2, 4, 6, 8, 10 days) by qPCR, including miR-336/ACSL4/LncRNA-MSTRG71379/circRNA0002331, miR-422/FOXO4/LncRNA-MSTRG54995/circRNA0000520, miR-578/IGF1/LncRNA-MSTRG102235/circRNA0002971, and miR-722/PDK4/LncRNA-MSTRG107440/circ RNA0002909. In this study, our data provided plenty of valuable candidate DERs and regulatory networks for researching the molecular mechanisms of sheep adipocyte differentiation and will assist studies in improving the IMF.
Small Tail Han sheep, a Chinese endemic breed, possesses high fecundity and strong resistance (Miao et al., 2016). Farmers and breeding enterprises widely raise Small Tail Han sheep to provide for the local consumers in Northeast China. Unfortunately, Small Tail Han sheep can no longer adapt to the current market because it has a slow growth rate and low meat quality (Kashan et al., 2005). Therefore, it is urgent to improve the quality of mutton. Intramuscular fat (IMF) content is closely related to meat quality traits and affects the taste and tenderness of mutton, changes the nutritional composition, and directly affects consumers’ purchasing decisions (Zhao et al., 2015). Therefore, increasing the content of IMF is the key to improving meat quality. Many factors affect the accumulation of IMF content including nutrition (Scollan et al., 2017), environment (Lambe et al., 2021), animal breed (Martins et al., 2020), age (Rahemi et al., 2015), gender (Gálvez et al., 2018), and genetic factors (Park et al., 2018). Although excessive caloric intake without a rise in energy expenditure increases IMF (Tang and Lane, 2012), different individuals possess the diverse ability to increase IMF in the same diet condition. The accumulation of IMF is the result of de novo lipogenesis and adipocyte differentiation, so when increasing IMF, we need to explore the mechanism of adipocyte differentiation.
Intramuscular adipose contains a high percentage of mature adipocytes, some preadipocytes, and a few other cells (Géloën et al., 1989). Adipocyte differentiation is a process that the preadipocyte differentiates into the mature adipocyte. The regulatory mechanisms of adipocyte differentiation are complex, and many genes (Ntambi and Young-Cheul, 2000), transcription factors (Liu et al., 2020), proteins (Gregoire et al., 1998), and hormones are involved (Obregon, 2008). Genetic factors can affect the accumulation of IMF by regulating de novo lipogenesis and adipocyte differentiation (Koutnikova and Auwerx, 2001). Recently, non-coding RNAs that contain long non-coding RNA (lncRNA), circular RNA (circRNA), and microRNA (miRNA) may play a core role in lipid metabolism and adipocyte differentiation (Zeng et al., 2018). LncRNA has more tissue specificity, binds to target genes by cis or trans methods (Tafer and Hofacker, 2008), and develops into pre-miRNA (Friedlander et al., 2012). LncRNA participates in various physiological functions, such as dose compensation effect, epigenetic regulation, cell cycle, and differentiation regulation (Xiao et al., 2015; Xiong et al., 2018). Some studies have reported that lncRNA plays a core role in adipocyte differentiation (Lopez-Pajares, 2016). circRNA, covalently closed by 3-terminal and 5-terminal RNAs, is more stable and conserved than other RNAs (Jeck and Sharpless, 2014). A part of circRNAs can transcribe as the protein; other positions are in intron sequences of the cell nucleus (Li Z. et al., 2015). circRNA may become potential biological markers involved in adipocyte differentiation (Yu et al., 2021). miRNA is a crucial regulatory molecule and can bind to the untranslated region (UTR) of messenger RNAs (mRNAs) to regulate their expression (Ali et al., 2020). miRNA participates in many biological functions, such as cellular growth and differentiation (Lu and Rothenberg, 2018). Many reports have identified that miRNA acts as a vital factor affecting adipocyte differentiation (Shi et al., 2016). Compete endogenous RNA (ceRNA) is a common physiological mechanism that lncRNA and circRNA competitively combine with miRNA (Thomson and Dinger, 2016). circRNA acts as a sponge to absorb miRNA competing with other RNAs (Memczak et al., 2013). Some studies have proven that ceRNA widely exists in adipocyte differentiation and forms the crucial regulatory networks (Xu et al., 2015; Chen et al., 2018). Although many studies related to adipocyte differentiation were published, the detailed molecular mechanism remains unclear, especially non-coding RNA and ceRNA in sheep.
To explore the molecular mechanisms and identify the candidate RNAs in sheep adipocyte differentiation, we compared the changes between preadipocytes and mature adipocytes by whole-transcriptome analysis. Our data provided many novel candidate RNAs, ceRNA networks, and, at the same time, research directions and theoretical basis for related research. This work is meaningful for excavating the molecular mechanism of sheep adipocyte differentiation and increasing IMF.
The Small-Tailed Han sheep was raised as experimental animals in the Jilin Academy of Agricultural Sciences. A 2-month-old healthy male sheep’s groin adipose tissue was isolated to extract the preadipocytes. The tissue was washed with ice-cold phosphate-buffered saline (PBS; Sigma-Aldrich, St. Louis, MO, United States) containing 1% penicillin/streptomycin (Sigma) and cut into small pieces, then the tissue blocks were digested for 1 h using collagenase Type II (Sigma) and 0.25% trypsin (Sigma) to collect preadipocytes. The cells were cultured with a complete culture medium containing 10% fetal bovine serum (Gemini Bio-Products, Woodland, CA, United States), 1% penicillin/streptomycin (Sigma), and DMEM-F12 medium (Sigma) in a 37°C and 5% CO2 incubator (Thermo Fisher Scientific, Waltham, MA, United States) and replaced with new culture medium every 48 h. When the cells are overgrown in the culture dish, they are induced to differentiate into mature adipocytes by the exogenous inducer. The cells were treated with inducer I solution containing 10 mg/ml insulin (Sigma), 1.0 mM dexamethasone (Sigma), 0.5 mM IBMX (Sigma), and complete culture medium for 48 h, inducer II solution containing 10 mg/ml insulin (Sigma) and complete culture medium for 48 h, and then fresh complete culture medium to continue cell culture. The whole process of cell differentiation underwent about 12 days.
Oil red O staining solution can identify adipocytes because the lipid droplets within the cell can be stained red, but other cells cannot. Oil red O staining occurs on the 12th day when the cells are growing, which is the eighth day when the cells start to differentiate. The adipocytes were washed with PBS buffer three times and fixed with 4% paraformaldehyde solution (Sangon Biotech Co., Ltd., Shanghai, China) in a 37°C and 5% CO2 incubator for 30 min. The cells were washed with PBS buffer three times, then cells were treated with Oil red O (Sigma) for 30 min in the incubator. After this, the cells were washed with PBS buffer three times, the staining was observed with a microscope, and pictures were taken.
The fourth-generation preadipocytes possess fast and stable growth and can be used as experimental cells based on previous experimental experience. Some preadipocytes are regarded as group P, and the remaining cells differentiate into adipocytes as group M. Each group has three repetitions (technical repetition); they are P1, P2, P3 and M1, M2, M3. The cells’ total RNA was extracted using TRIzol reagent (Thermo Fisher Scientific, Waltham, MA, United States) according to the instructions, then RNA degradation and contamination were monitored using 1% agarose gels. NanoPhotometer spectrophotometer (IMPLEN, CA, United States) assesses the purity of RNA. Qubit RNA Assay Kit in a Qubit 2.0 Fluorometer (Life Technologies, CA, United States) measured the concentration of RNA. Nano 6000 Assay Kit in a Bioanalyzer 2100 system (Agilent Technologies, CA, United States) evaluated the integrity of RNA (Ma et al., 2019).
Here, 3 μg total RNA of each sample was used to construct the lncRNA library. The total RNA was digested by DNase I, and ribosomal RNA (rRNA) was removed using Ribo-ZeroTM Gold Kit (Illumina, San Diego, United States); at this time, the lncRNA library contains mRNA. Since mRNA contains a specific Poly-A tail, oligo(dT) magnetic beads can specifically bind to the Poly-A tail to separate mRNA. Fragmentation Buffer was added to the reaction system to fragment RNA into short fragments (about 200–500 nt), and then the fragments were used as templates to synthesize the first strand of cDNA with six-base random hexamer primers, and then buffer, dNTPs, RNase H, and DNA Polymerase I were used to synthesize the second strand of cDNA. QiaQuick PCR kit purified cDNA and EB buffer resolved end reparation and single nucleotide A (adenine) addition and adaptor. The target fragment is recovered by agarose gel electrophoresis, the uracil-N-glycosylase (UNG) enzyme degraded the second strand of cDNA, and the suitable fragments were selected as templates for PCR amplification. Finally, agarose gel electrophoresis recovers the target fragments to construct the library. To construct a circRNA library, it is necessary to add an RNase enzyme to remove linear RNA after rRNA is removed, so that circRNA can be obtained. After the RNA of the sample is qualified, the Small RNA Sample Pre Kit constructs the miRNA library; small RNA sequence length is small and has a special structure at the 3′ and 5′ ends (the 5′ end has a complete phosphate group, and the 3′ end has a hydroxyl group). The small RNA is directly connected to both ends with an adaptor, and then reverse transcription is used to synthesize cDNA. Then, after PCR amplification, polyacrylamide gel electrophoresis (PAGE) was performed to separate the target DNA fragments; the cDNA library is recovered by cutting the gel. The constructed library was sequenced using an Illumina system (Agilent Technologies Inc.) by Annoroad Gene Technology Co., Ltd. (Beijing, China).
The original results of Illumina high-throughput sequencing are image data files, which are converted into Raw Reads after Base Calling by bcl2fastq2 software, and the results are stored in the FASTQ (referred to as fq) file format. In the FASTQ format file, each base corresponds to a base quality character, and the ASCII code value corresponding to each base quality character minus 33 (Sanger quality value system) is the sequencing quality score of the base (Phred Quality Score). Different Phred Quality Scores represent different base sequencing error rates. For example, Phred Quality Score values of 20 and 30 indicate base sequencing error rates of 1 and 0.1%, respectively. Raw data contain sequencing adapter sequences and low-quality sequences. To ensure the quality of the information analysis data, we filter raw data sequences and use Cutadapt and fastx_toolkit to remove the adaptor, low-quality reads, reads with an N content greater than 5%, and reads that match ribosomal RNA.
To better identify lncRNAs and mRNAs, clean RNA sequencing (RNA-Seq) reads were mapped to the genome using Hisat2. To identify circRNAs, clean RNA-Seq reads were mapped to the genome using the BWA-MEM algorithm. BWA-MEM can quickly and efficiently compare reads with the genome. For the accuracy of subsequent miRNA analysis, clean miRNA-Seq reads were mapped to the genome using Bowtie software (setting allows one mismatch). The reference genome and gene annotation files were downloaded from the NCBI database. Version: Ovis_aries.NCBI.GCF_002742125.1.v1.0. GCF_002742125.1_Oar_rambouillet_v1.0_genomic. gff.gz. StringTie can quickly assemble transcripts using RNA-Seq alignment results. Reads were divided into different categories, and built splice graph to determine the transcript. Each transcript build flow network for path of heaviest coverage to compute maximum flow to evaluate abundance, and combine the complex data set assemble into a transcript.
We mainly screen for three types: long intergenic non-coding RNA (lincRNA), intronic lncRNA, and antisense lncRNA. The basic screening conditions are as follows: (1) The length of the transcript is greater than or equal to 200 bp, and the number of exons is greater than or equal to 2; (2) Calculating the read coverage of each transcript, and transcripts with less than five reads in all samples were removed; (3) gffcompare1 compares the annotation files of the species to screen out the known mRNA and other non-coding RNA (rRNA, tRNA, snoRNA, snRNA, etc.); (4) Identifying potential lincRNA, intronic lncRNA, and antisense lncRNA according to the class_code information (“u,” “i,” “x”) in the comparison result. Through the initial screening of lncRNA in the previous step, a variety of coding potential analysis software is used to screen, mainly Coding-Non-Coding Index (CNCI), Coding Potential Calculator (CPC), Coding Potential Assessment Tool (CPAT for animals only), and PFAM protein domain analysis. Several analysis methods all distinguish the non-coding transcript as the final novel lncRNA data set.
The current research mainly is on exonic circular RNA (ecircRNA) and the cyclization mechanism: (1) lariat-driven circularization (2) intron pairing-driven circularization. The difference between the two models is whether the first step of cyclization is to form a lariat-driven structure or the introns on both sides of the exon are complementarily paired first. No matter which form it is, it is formed by trans-splicing the splice donor (SD) of the downstream exon of the circRNA and the splice acceptor (SA) of the upstream exon. The main idea of recognizing circRNA: looking for the GT-AG signal next to the junction site. CIRI (Gao et al., 2015) is an efficient and fast circRNA identification tool. BWA-MEM algorithm is used to split and compare the sequences, and then the resulting SAM file is scanned to find paired chiastic clipping (PCC) and paired-end mapping (PEM) sites, as well as GT-AG splicing signals. The sequence with junction site was realigned with a dynamic programming algorithm to ensure the reliability of identifying circRNA.
According to the mapped reads, it is compared with the specified species sequence in the miRBase database to identify known miRNAs. Clean Reads that are not annotated as known miRNAs are compared with the non-coding RNA sequence in Rfam (13.0) to realize the annotation of rRNA, tRNA, snRNA, snoRNA, and other non-coding RNAs. For Clean Reads that are not annotated as known miRNA, non-coding RNA, and Repeat, the small RNA derived from mRNA is annotated by matching with the location information of gene exons and introns (100% position overlap). There are still some features of miRNAs, but no sequences have been discovered so far, so novel miRNA predictive analysis is needed. The software miRDeep2 is used to predict novel miRNAs, obtain the matched Clean Reads information of each novel miRNA, and obtain the structure and expression information of each novel miRNA.
The expression levels of the protein-coding genes and lncRNAs were estimated by FPKM (Fragments Per Kilobase of transcript per Million mapped reads), which is a very effective tool for quantitatively estimating gene expression and can eliminate the influence of the difference in gene length and sequencing amount on the calculated gene expression, and the results can be directly used to compare the expressed difference between different samples. Due to the particularity of circRNA, it is difficult to accurately obtain the information of circRNA Reads on all alignments (linear RNA interference), so the usual expression estimation method is to use the number of Junction reads to estimate the expression of circRNA. We selected SRPBM (Spliced Reads per Billion Mapping) (Li Y. et al., 2015) normalization method to quantify the expression of circRNA. TPM (Transcripts Per Million) is used to estimate the expression level of miRNA, which is calculated based on the reads (incompletely mature or degraded) that are aligned to the miRNA precursor and slide in a certain area of the mature body. We selected DEseq2 to analyze the differential expression of RNAs, comparing the treatment group and the reference group. |log2Ratio| ≥ 1 and p < 0.05 were set as the threshold in the differential expression analysis.
LncRNA binds to target genes in Cis or Trans mode. Cis binding method prediction: the protein-coding genes adjacent to lncRNA (upstream and downstream 50 kb) will be screened out as target genes. Trans combination method prediction: when the number of samples is greater than or equal to 6, the target gene is screened according to the correlation coefficient between the expression of lncRNA and mRNA (correlation coefficient ≥ 0.9). The forecasting software is implemented using Annoroad’s in-house scripts. There are multiple binding sites of miRNA on the circRNA sequence. When miRNA is adsorbed, it cannot regulate its corresponding target gene, thus acting as a sponge of miRNA molecules, and then we selected miRanda (3.3a) (Enright et al., 2003) for target prediction. MiRanda (Enright et al., 2003) and TargetScan (Agarwal et al., 2015), two target prediction software, were selected to predict miRNA target genes, and the intersection of the two target prediction results is taken as miRNA target genes.
GOseq R package (ver. 2.12) conducted Gene Ontology (GO) analysis according to the following rules: differentially expressed genes (DEGs), differentially expressed circular RNA (DEcircRNA)-deriving genes, target genes of differentially expressed long non-coding RNAs (DElncRNAs), and differentially expressed miRNAs (DEmiRNAs). GO analysis was used to annotate the genes with terms under biological process, cellular component, and molecular function categories. KOBAS software (2.0) conducted Kyoto Encyclopedia of Genes and Genomes (KEGG) pathways analysis according to the same rules. GO and KEGG terms featuring p < 0.05 were considered significantly enriched.
According to the prediction results of target RNAs, we systematically constructed various regulatory networks of the differentially expressed RNAs (DERs) related to sheep adipocyte differentiation. The two-element network was based on the following relationships: target mRNA of lncRNA, target mRNA of miRNA, lncRNA can become miRNA, circRNA adsorbs miRNA. Then, we constructed ceRNA networks, including the three-element network and four-element network based on various RNAs that can competitively bind the same miRNA response elements (MREs). Cytoscape 3.6.1 visualized the networks.
We randomly selected 40 DERs from the regulatory networks to identify the accuracy of sequencing results using qPCR and also explored the expression trend of the RNAs at five time points during the preadipocyte to mature adipocyte period (2, 4, 6, 8, 10 days). The glyceraldehyde-3-phosphate dehydrogenase gene (Gapdh) and U6 were internal controls. The total RNA transcribed into the cDNA using a reverse transcription kit (TaKaRa, Japan) as qPCR template, and the glyceraldehyde-3-phosphate dehydrogenase gene (Gapdh) and U6 were internal controls for qPCR. The primer sequences were synthesized by Genewiz Company as Supplementary Tables 1–4. qPCR was performed on LightCycler Roche 480 (Roche) with SuperMix Real PreMix Plus (SYBR green) (Roche). The delta-delta CT method calculated the relative expression level of RNAs.
The sequencing data of samples were compared according to the following three groups: M1 vs. P1, M2 vs. P2, and M3 vs. P3. The commonly owned data among the three groups were the final results of the adipocyte differentiation process. R software calculated parameters for the high-throughput sequencing-related data and differential expression analyses. All data representation using means ± SD and a p-value threshold of 0.05 was to infer statistically significant expression changes.
Adipocytes’ differentiation process mainly undergoes two stages: proliferation and differentiation. As shown in Figure 1, the non-adherent preadipocytes are spherical with a diameter of 10–30 μm. The cells begin to adhere to the wall and become irregular fusiform after 6 h. When the cells are overgrown in the culture dish, the exogenous inducer promotes the differentiation of the cells into mature adipocytes. Under the microscope, it can be seen that there are lipid droplets inside the cells, and the lipid droplets can be stained by Oil Red O, which indicates that the cells are successfully differentiated.
Figure 1. Growth morphology of adipocytes in different periods. (A) Non-adherent cells are spherical. (B) The adherent cells are irregularly shaped, and the cells are photographed on the fourth day of culture. (C) The lipid droplets in differentiated adipocytes can be stained red by Oil Red O, and the cells are photographed on the 12th day of culture. Scales bar: 50 μm.
As shown in Table 1, the quality of the sequencing data is high, the proportion of high-quality clean reads exceeds 95%, and the average percentage of Q30 is above 93%. The clean reads mapping rates of lncRNA and mRNA were more than 93%. We obtained 18,968 RNA transcripts, of which 1,713 known and 5,640 novel lncRNAs were in the P1 group, 1,705 known and 5,640 novel lncRNAs were in the P2 group, 1,680 known and 5,637 novel lncRNAs were in the P3 group, 1,569 known and 5,548 novel lncRNAs were in the M1 group, 1,611 known and 5,537 novel lncRNAs were in the M2 group, and 1,666 known and 5,602 novel lncRNAs were in the M3 group. The mapping rates of circRNA surpass 99% (Table 1). We identified 3,480 circRNAs, of which the CLASSIC circRNAs account for more than 80%. The mapping rates of miRNA exceed 97% (Table 2). We identified 150 known and 326 novel miRNAs in the P1 group, 150 known and 378 novel miRNAs in the P2 group, 150 known and 375 novel miRNAs in the P3 group, 151 known and 372 novel miRNAs in the M1 group, 149 known and 361 novel miRNAs in the M2 group, and 150 known and 342 novel miRNAs in the M3 group.
Using |log2Ratio| ≥ 1 and p < 0.05 as the criteria for screening DERs, the volcano chart shows the number of DERs in each group (Supplementary Figure 1). R language draws a cluster map to reflect the expression changes of DERs under different conditions (Supplementary Figure 2). DERs of each group are different, and we use the three groups of shared DERs as the final data. Figure 2 showed that we identified 1,196 DEGs, of which 711 genes were upregulated, and 485 were downregulated. There were 754 DElncRNAs in the process, of which 529 lncRNAs were upregulated, and 225 lncRNAs were downregulated. There were 17 DEcircRNAs in the process, of which 9 circRNAs were upregulated, and 8 circRNAs were downregulated. In addition, 100 DEmiRNAs dysregulated, 46 miRNAs were upregulated, and 54 miRNAs were downregulated between the P and M groups. The DERs’ detailed information is in Supplementary Tables 5–8.
Figure 2. Histogram of differentially expressed RNA shared by three groups. From preadipocytes to mature adipocytes, brown column is the number of total differentially expressed RNAs, red column is RNAs with increased expression, and blue column is RNAs with decreased expression.
GO analysis showed that the DERs were mainly involved in the cell part of the cellular component classification. Many DERs belonged to cellular processes and biological regulation on the physiological process classification. The molecular function assessment showed that the DERs associated with binding (Supplementary Figure 3). KEGG pathway analysis found that DEGs mainly enriched in the Focal adhesion, Axon guidance, and phosphoinositide 3-kinase (PI3K)-Akt signaling pathway. Peroxisome proliferator-activated receptor (PPAR), adipocytokine, and mitogen-activated protein kinase (MAPK) signaling pathways were also significantly enriched, but there was no significant enrichment in Fatty acid biosynthesis, Insulin signaling pathway, fat digestion and absorption, and fatty acid metabolism. The top three lncRNAs were MAPK signaling pathway, endocytosis, and pathways in cancer. Insulin and adipocytokine signaling pathway and fatty acid metabolism enriched significantly, but there was no significant enrichment in fat digestion and absorption, fatty acid biosynthesis, and PPAR signaling pathways. Most circRNAs focused on focal adhesion, FoxO signaling pathway, longevity regulating pathway-worm, axon guidance, and PI3K–Akt signaling pathways. A large number of miRNAs were involved in focal adhesion and PPAR signaling pathways (Supplementary Figure 4).
A lncRNA combines with multiple target genes, at the same time, a gene can also be targeted by multiple lncRNAs in the lncRNA–mRNA networks. There were 729 DElncRNAs and 1,844 DE target genes in the three groups. There were 46 DElncRNAs and 24 target miRNAs in the lncRNA–miRNA networks. The lncRNA–mRNA and lncRNA–miRNA regulatory networks were too complex and huge, so the networks were shown clearly in a chart on the manuscript. Detailed information is shown in Supplementary Tables 9, 10. Figures 3A,B showed circRNA–miRNA and miRNA–mRNA regulatory networks. Detailed information is shown in Supplementary Tables 11, 12.
Figure 3. Regulatory networks from preadipocytes to mature adipocytes. (A) Circular RNA (circRNA)–microRNA (miRNA) network regulation diagram; the red graphic is miRNA, and the green graphic is circRNA. (B) miRNA–messenger RNA (mRNA) network regulation diagram; the yellow graphic is mRNA. (C) Long non-coding RNA (lncRNA)–miRNA–mRNA network regulation diagram; the blue graphic is mRNA. (D) circRNA–miRNA–mRNA network regulation diagram between M1 vs. P1 and M2 vs. P2 groups. (E) circRNA–miRNA–mRNA network regulation diagram between M2 vs. P2 and M3 vs. P3 groups. (F) LncRNA–circRNA–miRNA–mRNA network regulation diagram between M1 vs. P1 and M2 vs. P2 groups. (G) LncRNA–circRNA–miRNA–mRNA network regulation diagram between M2 vs. P2 and M3 vs. P3 groups.
As shown in Figure 3C, 13 novel miRNAs play a central role in the mRNA–miRNA–lncRNA networks. Detailed information is shown in Supplementary Table 13. In miRNA–mRNA–circRNA networks, we did not find shared networks in the three groups, but there are shared networks in M1 vs. P1 and M2 vs. P2, and there are other shared networks in M2 vs. P2 and M3 vs. P3. There are six novel miRNAs (miR-336, miR-422, miR-578, miR-722, miR-748, and miR-831) that may play a core role in the regulatory networks (Figures 3D,E). Detailed information is shown in Supplementary Table 14.
In miRNA–mRNA–circRNA–lncRNA networks, we did not find shared networks in the three groups, but there are shared networks in M1 vs. P1 and M2 vs. P2, and there are other shared networks in M2 vs. P2 and M3 vs. P3 (Figures 3F,G). Interestingly, six novel miRNAs (miR-336, miR-422, miR-578, miR-722, miR-748, and miR-831) can simultaneously form three-element and four-element networks. Detailed information is shown in Supplementary Table 15.
Because the number of DERs and networks was large and complex, we screened out the highest expression DERs and core networks. The highest expression of DEGs was screened out based on | logFC| > 5. The highest expression of lncRNAs and circRNAs was chosen based on | logFC| > 2 because the | logFC| value of DElncRNAs and microRNAs is low (Table 3). The | logFC| value of DEmiRNAs is high, so the highest expression of miRNAs was selected based on | logFC| > 10. miRNAs’ detailed information is shown in Table 4. The raw data of sequencing have been in the public database (Sequence Read Archive): SUB7601005 and SUB76001332,3.
The core networks were screened out based on three- and four-element networks, and they all contained four crucial novel miRNAs (miR-336, miR-422, miR-578, and miR-722). The miRNAs can form various networks because they had many targets, such as the following: miR-336 has 402 target RNAs (58 genes, 328 lncRNAs, 16 circRNAs); miR-422 has 24 target RNAs (11 genes, 11 lncRNAs, 2 circRNAs); miR-578 has 335 targets (46 genes, 273 lncRNAs, 16 circRNAs); miR-722 has 201 target RNAs (24 genes, 163 lncRNAs, 14 circRNAs). We considered the miRNAs as the candidate miRNAs. Detailed information is shown in Table 5.
To validate the accuracy of RNA-Seq data, we selected 40 DERs from the highest expression DERs and the core regulatory networks, including four well-known genes that affect the adipocyte differentiation, including Lipoprotein lipase (LPL) (Ailhaud et al., 1985), Fatty acid-binding protein 4 (FABP4) (Moseti et al., 2016), Insulin-like growth factor 1 (IGF1) (Chun et al., 2019), Carnitine palmitoyltransferase 1A (CPT1A) (Calderon et al., 2016), and novel crucial miRNAs (miR-336, miR-422, miR-578, miR-722). The qPCR results were highly consistent with the RNA-Seq data (Figure 4). It suggested that RNA-Seq data were credible and accurate. DERs’ detailed information is shown in Tables 6, 7.
Figure 4. Comparison of sequencing results and qPCR results. The abscissa is differentially expressed RNAs (DERs), the ordinate is Log2(Fold Change), the gray column is the sequencing result, and the black column is the qPCR result. (A) Validation of messenger RNA (mRNA) results by qPCR. (B) Validation of long non-coding RNA (lncRNA) results by qPCR. (C) Validation of circular RNA (circRNA) results by qPCR. (D) Validation of microRNA (miRNA) results by qPCR.
Although we saw the expression alteration of the DERs between the P and M groups, it still cannot indicate that the RNAs were indeed involved in every period of adipocyte differentiation. We detected the expression trend of the DERs in the adipocyte differentiation period (2–10 days) by qPCR. The results showed that the RNAs had significant changes in the whole process of adipocyte differentiation (Figure 5). The RNAs also contained some pathways, such as miR-336/ACSL4/LncRNA-MSTRG71379/circRNA0002331, miR-422/FOXO4/LncRNA-MSTRG54995/circRNA0000520, miR-578/IGF1/LncRNA-MSTRG102235/circRNA0002971, and miR-722/PDK4/LncRNA-MSTRG107440/circRNA0002909. The DERs and networks were indeed involved in the process of sheep adipocyte differentiation. The core regulatory networks of four miRNAs are worthy of attention and in-depth studying.
Figure 5. The change trend of differentially expressed RNA during adipocyte differentiation. The abscissa is the different days of cell culture, the ordinate is relative expression level, different colored lines represent different RNAs. (A) Messenger RNA (mRNA) results by qPCR. (B) Long non-coding RNA (lncRNA) results by qPCR. (C) Circular RNA (circRNA) results by qPCR. (D) MicroRNA (miRNA) results by qPCR.
Marbling is a vital standard to measure the quality of meat (Pethick et al., 2004). IMF content can significantly affect a marbling state. Increasing the accumulation of fat in intramuscular can promote the formation of meat marbling and improve meat properties such as taste, flavor, color, etc. (Dodson et al., 2010). The genetic factor leading to the speed of individual accumulating IMF content was different in the same breed and feeding conditions. The reasons may be which genetic factor determines the number of mammalian initial fat cells and the speed rate of adipocyte differentiation (Hocquette et al., 2010). Therefore, it is crucial to explore the potential molecular mechanisms of adipocyte differentiation. At present, a few studies provided candidate RNAs related to meat traits and were used for actual production (Hong et al., 2012; Yuan et al., 2013; Pertea et al., 2016). However, other non-coding RNAs and ceRNA remain less reported in sheep. In this study, we used high-throughput sequencing to compare RNA changes from preadipocytes to mature adipocytes. We obtained plenty of candidate genes and core regulatory networks affecting adipocyte differentiation by qPCR validation. These findings will enable us to understand the molecular mechanisms involved in adipocyte differentiation and expand new researching directions or biomarkers for improving the IMF and quality of mutton.
We found plenty of novel RNA transcripts in the study, especially non-coding RNAs. The number of novel miRNAs and lncRNAs dramatically surpassed that of the known ones. These results deserve attention. The main reasons may be that the reports on non-coding RNAs in sheep adipocyte differentiation were less and leading to lots of novel miRNAs and lncRNAs were not found. At the same time, there is lately no complete genome reference. In this study, a large number of genes involved in adipocyte differentiation were identified, especially well-known genes. The expression trend of the genes was consistent with what others reported (Prosdocimo et al., 2014; Olivecrona, 2016; Li et al., 2017; Kineman et al., 2018; Rodríguez-Calvo et al., 2019), such as LPL, FABP4, Forkhead box-O1 (FoxO1) (Chen et al., 2019), IGF1, and KLF family members. Some studies found that the selective knockdown of Matrix metalloproteinase 2 (MMP2) in mouse adipose cell lines led to a significant reduction (Bauters et al., 2015). Our sequencing data indicated that the MMP2 gene was also significantly increased in sheep adipocyte differentiation. Some reports suggested that Sirtuin 3 (SIRT3) promotes age-related adipogenesis and osteoclast production related to bone loss (Ho et al., 2017). The changing trend of the SIRT3 gene in these studies was consistent with our data. Reported miRNAs related to sheep lipid metabolism were less, especially only a few DEmiRNAs were found in the present study. miR-143 could be associated with obesity and could promote adipocyte differentiation in mice fed with a high-fat diet. We also found that miR-143 was significantly expressed during adipocyte differentiation (Bae et al., 2017). Some studies suggested that miR-181a promoted preadipocyte differentiation in porcine by directly targeting transforming growth factor-beta receptor 1 (TGFBR1) (Zhang et al., 2019). In this study, we also identified miR-181a and TGFBR1, and the expression trends were consistent with previous reports and findings. miR-27a can promote the proliferation of sheep preadipocytes and inhibit cell differentiation by regulating the expression of RXRα (Deng et al., 2020). Our experiment also identified the expression changes of miR-27a, but the trend was not statistically significant. One potential explanation for this is that our study missed the probability of the period of higher expression of miR-27a. To the best of our knowledge, only a limited number of reports have previously studied the expression profiles of lncRNAs and circRNAs concerning sheep lipid metabolism by searching the NCBI website. Although in the present study we found most lncRNAs and circRNAs affecting adipocyte differentiation, the DERs do not have too much literature for reference. The underlying function of DERs can provide a new research direction. In this study, some known genes affecting adipocyte differentiation had significant changes, such as PPARγ, CCAAT/enhancer-binding proteins (C/EBPα), and sterol regulatory element-binding protein 1 (SREBP1) (Sarjeant and Stephens, 2012). The main reasons may be what genes are only significantly expressed in the early stage of adipocyte differentiation. These trial samples were in preadipocyte and mature adipocyte stages, and the interval time is too long. Therefore, the experiment did not identify these well-known genes’ significant expression.
GO enrichment analysis showed that the results of four varieties of RNAs were similar; GO terms all enriched in cell part, cellular process, biological regulation, and binding. Non-coding RNAs made GO enrichment analysis based on targets or related to genes, so the final results were similar. KEGG pathway analysis results for the four varieties of RNAs showed that DERs mostly enriched in focal adhesion, PPAR signaling pathway, cancer, adipocytokine signaling pathway, MAPK signaling pathway, FoxO signal pathway, PI3K/Akt signaling pathways, and so on. A potential explanation for these findings could be that the non-coding RNAs may present different functions and spatiotemporal specific activities. However, we only studied the RNA expression in two periods of cell differentiation. Interestingly, we found many significantly dysregulated genes involved in cancer that participated in adipocyte differentiation.
We systematically constructed regulatory networks affecting adipocyte differentiation. The regulatory networks were complex; therefore, constructed various networks were necessary. In this study, IGF1 can participate in all regulatory networks, and it suggested that IGF1 played a vital role in adipocyte differentiation. We also noticed that miR-336, miR-422, miR-578, and miR-722 played core roles in all ceRNA regulatory networks. In this study, although the expression differences of the four new miRNAs (miR-336, miR-422, miR-578, and miR-722) did not seem to be significant in the qPCR results, we can see that the expressions of other network factors related to these four miRNAs are all significant, and these four genes also have significant changes at different stages of the adipocyte differentiation process. Interestingly, we found that all core miRNAs of the circRNA–miRNA–mRNA network participated in the four-element network. However, none of the core miRNAs of the lncRNA–miRNA–mRNA participated in the four-element network, and the reasons were unclear. We chose 40 DERs to detect the expression pattern in the process adipocyte differentiation, from which form many regulatory networks: miR-336/ACSL4/LncRNA-MSTRG71379/circRNA0002331, miR-422/FOXO4/LncRNA-MSTRG54995/circRNA0000520, miR-578/IGF1/LncRNA-MSTRG102235/circRNA0002971, miR-722/PDK4/LncRNA-MSTRG107440/circRNA0002909, and so on. The four networks and their core miRNAs were worthy of the next study. There were some problems encountered during the experiment. For example, the interval time from the P group to the M group was too long. Sequencing results may miss some highly expressed genes or non-coding RNAs in this period. We plan to add a new group of experiments between P and M groups to deal with the problems. In this study, trial data provided the DERs and pathways as practical value and reference for exploring the molecular mechanisms of adipocyte differentiation. This study not only adapts to livestock but also has valuable reference significance for human fat metabolism.
We did whole-transcriptome sequencing in the sheep preadipocyte and the mature adipocyte group. We constructed for the first time comprehensive and systematic regulatory networks affecting sheep adipocyte differentiation. Sequencing data contained plenty of well-known and novel genes and non-coding RNAs; some of the DERs may be candidate RNAs, and they played a vital role in sheep adipocyte differentiation. The study identified that the four miRNAs (miR-336, miR-422, miR-578, and miR-722) were valuable for researching the molecular mechanisms of adipocyte differentiation. The four miRNAs involved in the networks also play crucial roles in the process and may be new biomarkers for improving IMF content and meat quality in sheep.
The datasets presented in this study can be found in online repositories. The names of the repository/repositories and accession number(s) are Sequence Read Archive—PRJNA639304, https://www.ncbi.nlm.nih.gov/search/all/?term=PRJNA639304.
The animal study was reviewed and approved by AWEC 2019A05, 16 May 2019. Written informed consent was obtained from the owners for the participation of their animals in this study.
LZ and YC: conceptualization. YC: methodology and writing—review and editing. TW: software. CX: validation, formal analysis, writing—original draft preparation, and visualization. JL: investigation. HM: resources. LL: data curation. CW and ZY: supervision. HJ: project administration. HJ and HM: funding acquisition. All authors have read and agreed to the published version of the manuscript.
This study was supported by the Scientific and Technological Developing Scheme of Jilin Province (20190301006NY), the Jilin Scientific and Technological Development Program (20190301005NY), and the China Agriculture Research System of MOF and MARA (CARS-38 and CARS-39-17).
The authors declare that the research was conducted in the absence of any commercial or financial relationships that could be construed as a potential conflict of interest.
All claims expressed in this article are solely those of the authors and do not necessarily represent those of their affiliated organizations, or those of the publisher, the editors and the reviewers. Any product that may be evaluated in this article, or claim that may be made by its manufacturer, is not guaranteed or endorsed by the publisher.
We thank all the researchers who contributed to this work.
The Supplementary Material for this article can be found online at: https://www.frontiersin.org/articles/10.3389/fgene.2021.662143/full#supplementary-material
Supplementary Figure 1 | Volcano map of different groups of differential RNAs. (A–C) Expression profiles of mRNAs and LncRNAs (M1 vs. P1, M2 vs. P2, M3 vs. P3). (D–F) Expression profiles of circRNAs (M1 vs. P1, M2 vs. P2, M3 vs. P3). Green points represent down-regulated RNAs; red points represent up-regulated RNAs; gray points represent not significantly expressed RNAs in the volcano plots. (G–I) Expression profiles of miRNAs (M1 vs. P1, M2 vs. P2, M3 vs. P3). Blue points represent down-regulated RNAs; yellow points represent up-regulated RNAs; gray points represent not significantly expressed RNAs in the volcano plots. X-axis: Fold change log2 ratio of RNAs. Y-axis: false discovery rate values (-log10 transformed).
Supplementary Figure 2 | Cluster map of different groups of differential RNAs. (A) Cluster analysis of mRNAs. (B) Cluster analysis of LncRNAs. (C) Cluster analysis of circRNAs. (D) Cluster analysis of miRNAs. The yellow and blue regions represent increased and decreased RNAs.
Supplementary Figure 3 | Gene ontology (GO) analysis of different groups of differential RNAs. (A) GO annotations of DE mRNAs. (B) GO annotations of DE LncRNAs. (C) GO annotations of DE circRNAs. (D) GO annotations of DE miRNAs.
Supplementary Figure 4 | KEGG pathway analysis of different groups of differential RNAs. (A) KEGG pathway of DE mRNAs. (B) KEGG pathway of DE LncRNAs. (C) KEGG pathway of DE circRNAs. (D) KEGG pathway of DE miRNAs.
Supplementary Table 1 | Primer sequences of mRNAs.
Supplementary Table 2 | Primer sequences of LncRNAs.
Supplementary Table 3 | Primer sequences of circRNAs.
Supplementary Table 4 | Primer sequences of miRNAs.
Supplementary Table 5 | List of 1,196 DEmRNAs.
Supplementary Table 6 | List of 754 DELncRNAs.
Supplementary Table 7 | List of 17 DEcircRNAs.
Supplementary Table 8 | List of 100 DEmiRNAs.
Supplementary Table 9 | Data of LncRNA binds to miRNA.
Supplementary Table 10 | Data of LncRNA binds to mRNA.
Supplementary Table 11 | Data of circRNA absorbs miRNA.
Supplementary Table 12 | Data of miRNA bind to mRNA.
Supplementary Table 13 | LncRNA-mRNA-miRNA correlation data.
Supplementary Table 14 | circRNA-mRNA-miRNA correlation data.
Supplementary Table 15 | circRNA-LncRNA-mRNA-miRNA correlation data.
Agarwal, V., Bell, G. W., Nam, J. W., and Bartel, D. P. (2015). Predicting effective microRNA target sites in mammalian mRNAs. Elife 4:e05005. doi: 10.7554/eLife.05005
Ailhaud, G., Amri, E., Czerucka, D., Forest, C., Gaillard, D., Grimaldi, P., et al. (1985). Lipoprotein lipase and adipocyte differentiation. Reprod. Nutr. Dev. 25, 153–158.
Ali, S. Z., Langden, S. S., Munkhzul, C., Lee, M., and Song, S. J. (2020). Regulatory mechanism of MicroRNA expression in cancer. Int. J. Mol. Sci. 21:1723. doi: 10.3390/ijms21051723
Bae, I., Park, P. J., Lee, J. H., Cho, E. G., Lee, T. R., and Kim, S. H. (2017). PPARγ-mediated G-protein coupled receptor 120 signaling pathway promotes transcriptional activation of miR-143 in adipocytes. Gene 626, 64–69. doi: 10.1016/j.gene.2017.05.016
Bauters, D., Scroyen, I., Van, H. M., and Lijnen, H. R. (2015). Gelatinase A (MMP-2) promotes murine adipogenesis. Biochim. Biophys. Acta 1850, 1449–1456. doi: 10.1016/j.bbagen.2015.04.003
Calderon, D. M., Sebastián, D., Fucho, R., Weber, M., Mir, J. F., García-Casarrubios, E., et al. (2016). Carnitine palmitoyltransferase 1 increases lipolysis, UCP1 protein expression and mitochondrial activity in brown adipocytes. PLoS One 11:e0159399. doi: 10.1371/journal.pone.0159399
Chen, C., Cui, Q., Zhang, X., Luo, X., Liu, Y., Zuo, J., et al. (2018). Long non-coding RNAs regulation in adipogenesis and lipid metabolism: emerging insights in obesity. Cell Signal. 51, 47–58. doi: 10.1016/j.cellsig.2018.07.012
Chen, J., Lu, Yi, Tian, M., and Huang, Q. (2019). Molecular mechanisms of FOXO1 in adipocyte differentiation.[J]. J. Mol. Endocrinol. 62, R239–R253. doi: 10.1530/JME-18-0178
Chun, S. Y., Lim, J., Lee, E. H., Han, M. H., Ha, Y. S., Lee, N. J., et al. (2019). Preparation and characterization of human adipose tissue-derived extracellular matrix, growth factors, and stem cells: a concise review. Tissue Eng. Regen. Med. 16, 385–393. doi: 10.1007/s13770-019-00199-7
Deng, K., Ren, C., Fan, Y., Liu, Z., Zhang, G., Zhang, Y., et al. (2020). miR-27a is an important adipogenesis regulator associated with differential lipid accumulation between intramuscular and subcutaneous adipose tissues of sheep. Domest. Anim. Endocrinol. 71:106393. doi: 10.1016/j.domaniend.2019.106393
Dodson, M. V., Jiang, Z., Chen, J., Hausman, G. J., Guan, L. L., Novakofski, J., et al. (2010). Allied industry approaches to alter intramuscular fat content and composition in beef animals. J. Food Sci. 75, R1–R8. doi: 10.1111/j.1750-3841.2009.01396.x
Enright, A. J., John, B., Gaul, U., Tuschl, T., Sander, C., and Marks, D. S. (2003). MicroRNA targets in Drosophila. Genome Biol. 5:R1. doi: 10.1186/Gb-2003-5-1-r1
Friedlander, M. R., Mackowiak, S. D., Li, N., Chen, W., and Rajewsky, N. (2012). miRDeep2 accurately identifies known and hundreds of novel microRNA genes in seven animal clades. Nucleic Acids Res. 40, 37–52. doi: 10.1093/var/gkr688
Gálvez, F., Domínguez, R., Pateiro, M., Carballo, J., Tomasevic, I., and Lorenzo, J. M. (2018). Effect of gender on breast and thigh turkey meat quality. Br. Poult. Sci. 59, 408–415. doi: 10.1080/00071668.2018.1465177
Gao, Y., Wang, J., and Zhao, F. (2015). CIRI: an efficient and unbiased algorithm for de novo circular RNA identification. Genome Biol. 16:4.
Géloën, A., Roy, P. E., and Bukowiecki, L. J. (1989). Regression of white adipose tissue in diabetic rats. Am. J. Physiol. 257, E547–E553. doi: 10.1152/append.1989.257.4.E547
Gregoire, F. M., Smas, C. M., and Sul, H. S. (1998). Understanding adipocyte differentiation. Physiol. Rev. 78, 783–809. doi: 10.1152/physrev.1998.78.3.783
Ho, L., Wang, L. P., Roth, T. M., Pan, Y., Verdin, E. M., Hsiao, E. C., et al. (2017). Sirtuin-3 promotes adipogenesis, osteoclastogenesis, and bone loss in aging male mice. Endocrinology 158, 2741–2753. doi: 10.1210/en.2016-1739
Hocquette, J. F., Gondret, F., Baéza, E., Médale, F., Jurie, C., Pethick, D. W., et al. (2010). Intramuscular fat content in meat-producing animals: development, genetic and nutritional control, and identification of putative markers. Animal 4, 303–319. doi: 10.1017/S1751731109991091
Hong, J. S., Noh, S. H., Lee, J. S., Kim, J. M., Hong, K. C., and Lee, Y. S. (2012). Effects of polymorphisms in the porcine microRNA miR-1 locus on muscle fiber type composition and miR-1 expression[J]. Gene 506, 211–216. doi: 10.1016/j.gene.2012.06.050
Jeck, W. R., and Sharpless, N. E. (2014). Detecting and characterizing circular RNAs. Nat. Biotechnol. 32, 453–461. doi: 10.1038/nbt.2890
Kashan, N., Azar, G., Afzalzadeh, A., Afzalzadeh, A., and Salehi, A. (2005). Growth performance and carcass quality of fattening lambs from fat-tailed and tailed sheep breeds. Small Rumin Res 60, 267–271. doi: 10.1016/j.smallrumres.2005.01.001
Kineman, R. D., Del, R. M., and Sarmento, C. A. (2018). 40 YEARS of IGF1: understanding the tissue-specific roles of IGF1/IGF1R in regulating metabolism using the Cre/loxP system.[J]. J. Mol. Endocrinol. 61, T187–T198. doi: 10.1530/JME-18-0076
Koutnikova, H., and Auwerx, J. (2001). Regulation of adipocyte differentiation. Ann. Med. 33, 556–561. doi: 10.3109/07853890108995966
Lambe, N. R., Clelland, N., Draper, J., Smith, E. M., Yates, J., and Bunger, L. (2021). Prediction of intramuscular fat in lamb by visible and near-infrared spectroscopy in an abattoir environmen. Meat Sci. 171:108286. doi: 10.1016/j.meatsci.2020.108286
Li, Y., Ma, Z., Jiang, S., Hu, W., Li, T., Di, S., et al. (2017). A global perspective on FOXO1 in lipid metabolism and lipid-related diseases. Prog. Lipid Res. 66, 42–49. doi: 10.1016/j.plipres
Li, Y., Zheng, Q., Bao, C., Li, S., Guo, W., Zhao, J., et al. (2015). Circular RNA is enriched and stable in exosomes: a promising biomarker for cancer diagnosis. Cell Res. 25, 981–984. doi: 10.1038/cr.2015.82
Li, Z., Huang, C., Bao, C., Chen, L., Lin, M., Wang, X., et al. (2015). Exon-intron circular RNAs regulate transcription in the nucleus. Nat. Struct. Mol. Biol. 22, 256–264. doi: 10.1038/nsmb.2959
Liu, S., Huang, J., Wang, X., and Ma, Y. (2020). Transcription factors regulate adipocyte differentiation in beef cattle. Anim. Genet. 51, 351–357. doi: 10.1111/age.12931
Lu, T. X., and Rothenberg, M. E. (2018). MicroRNA. J. Allergy Clin. Immunol. 141, 1202–1207. doi: 10.1016/j.jaci.2017.08.034
Lopez-Pajares, V. (2016). Long non-coding RNA regulation of gene expression during differentiation. Pflugers Arch. 468, 971–981. doi: 10.1007/s00424-016-1809-6
Ma, N., Pan, J., Ye, X., Yu, B., Zhang, W., and Wan, J. (2019). Whole-transcriptome analysis of APP/PS1 mouse brain and identification of circRNA-miRNA-mRNA networks to investigate AD pathogenesis. Mol. Ther. Nucleic Acids 18, 1049–1062. doi: 10.1016/j.omtn.2019.10.030
Martins, J. M., Fialho, R., Albuquerque, A., Neves, J., Freitas, A., Tirapicos Nunes, J., et al. (2020). Portuguese local pig breeds: genotype effects on meat and fat quality traits. Animals 10:905. doi: 10.3390/ani10050905
Memczak, S., Jens, M., Elefsinioti, A., Torti, F., Krueger, J., Rybak, A., et al. (2013). Circular RNAs are a large class of animal RNAs with regulatory potency. Nature 495, 333–338. doi: 10.1038/nature11928
Miao, X., Luo, Q., Zhao, H., and Qin, X. (2016). Ovarian proteomic study reveals the possible molecular mechanism for hyperprolificacy of Small Tail Han sheep. Sci. Rep. 6:27606. doi: 10.1038/srep27606
Moseti, D., Regassa, A., and Kim, W. K. (2016). Molecular regulation of adipogenesis and potential anti-adipogenic bioactive molecules. Int. J. Mol. Sci. 17:124. doi: 10.3390/ijms17010124
Ntambi, J. M., and Young-Cheul, K. (2000). Adipocyte differentiation and gene expression. J. Nutr. 130, 3122S–3126S. doi: 10.1093/jn/130.12.3122S
Obregon, M. J. (2008). Thyroid hormone and adipocyte differentiation. Thyroid 18, 185–195. doi: 10.1089/thy.2007.0254
Olivecrona, G. (2016). Role of lipoprotein lipase in lipid metabolism. Curr. Opin. Lipidol. 27, 233–241. doi: 10.1097/MOL.0000000000000297
Park, S. J., Beak, S. H., Jung, D. J. S., Kim, S. Y., Jeong, I. H., Piao, M. Y., et al. (2018). Genetic, management, and nutritional factors affecting intramuscular fat deposition in beef cattle–A review. Asian Austr. J. Anim. Sci. 31, 1043–1061. doi: 10.5713/ajas.18.0310
Pertea, M., Kim, D., Pertea, G. M., Leek, J. T., and Salzberg, S. L. (2016). Transcript-level expression analysis of RNA-seq experiments with HISAT, StringTie and Ballgown. Nat. Protoc. 11, 1650–1667. doi: 10.1038/nprot.2016.095
Pethick, D. W., Harper, G. S., and Oddy, V. H. (2004). Growth, development and nutritional mainpulation of marbling in cattle. Aust. J. Exp. Agr. 44, 705–715. doi: 10.1071/ea02165
Prosdocimo, D. A., Anand, P., Liao, X., Zhu, H., Shelkay, S., Artero-Calderon, P., et al. (2014). Kruppel-like factor 15 is a critical regulator of cardiac lipid metabolism. J. Biol. Chem. 289, 5914–5924. doi: 10.1074/jbc.M113.531384
Rahemi, H., Nigam, N., and Wakeling, J. M. (2015). The effect of intramuscular fat on skeletal muscle mechanics: implications for the elderly and obese. J. R. Soc. Interface 12:20150365. doi: 10.1098/rsif.2015.0365
Rodríguez-Calvo, R., Girona, J., Rodríguez, M., Samino, S., Barroso, E., de Gonzalo-Calvo, D., et al. (2019). Fatty acid binding protein 4 (FABP4) as a potential biomarker reflecting myocardial lipid storage in type 2 diabetes. Metabolism 96, 12–21. doi: 10.1016/j.metabol.2019.04.007
Sarjeant, K., and Stephens, J. M. (2012). Adipogenesis. Cold Spring Harb. Perspect. Biol. 4:a008417. doi: 10.1101/cshperspect.a008417
Scollan, N. D., Price, E. M., Morgan, S. A., Huws, S. A., and Shingfield, K. J. (2017). Can we improve the nutritional quality of meat? Proc. Nutr. Soc. 76, 603–618. doi: 10.1017/S0029665117001112
Shi, C., Huang, F., Gu, X., Zhang, M., Wen, J., Wang, X., et al. (2016). Adipogenic miRNA and meta-signature miRNAs involved in human adipocyte differentiation and obesity. Oncotarget 7, 40830–40845. doi: 10.18632/oncotarget.8518
Tafer, H., and Hofacker, I. L. (2008). RNAplex: a fast tool for RNA-RNA interaction search. Bioinformatics 24, 2657–2663. doi: 10.1093/bioinformatics/btn193
Tang, Q. Q., and Lane, M. D. (2012). Adipogenesis: from stem cell to adipocyte. Annu. Rev. Biochem. 81, 715–736. doi: 10.1146/annurev-biochem-052110-115718
Thomson, D. W., and Dinger, M. E. (2016). Endogenous microRNA sponges: evidence and controversy. Nat. Rev. Genet. 17, 272–283. doi: 10.1038/nrg.2016.20
Xiao, T., Liu, L., Li, H., Sun, Y., Luo, H., Li, T., et al. (2015). Long noncoding rna adinr regulates adipogenesis by transcriptionally activating C/EBP alpha. Stem Cell Rep. 5, 856–865. doi: 10.1016/j.stemcr.2015.09.007
Xiong, Y., Yue, F., Jia, Z., Gao, Y., Jin, W., Hu, K., et al. (2018). A novel brown adipocyte-enriched long non-coding RNA that is required for brown adipocyte differentiation and sufficient to drive thermogenic gene program in white adipocytes. Biochim. Biophys. Acta Mol. Cell Biol. Lipids. 1863, 409–419. doi: 10.1016/j.bbalip.2018.01.008
Xu, S., Chen, P., and Sun, L. (2015). Regulatory networks of non-coding RNAs in brown/beige adipogenesis. Biosci. Rep. 35:e00262. doi: 10.1042/BSR20150155
Yu, G., Yang, Z., Peng, T., and Lv, Y. (2021). Circular RNAs: rising stars in lipid metabolism and lipid disorders. J. Cell Physiol. 236, 4797–4806. doi: 10.1002/jcp.30200
Yuan, Z., Li, J., Li, J., Gao, X., Gao, H., and Xu, S. (2013). Effects of DGAT1 gene on meat and carcass fatness quality in Chinese commercial cattle. Mol. Biol. Rep. 40, 1947–1954. doi: 10.1007/s11033-012-2251-2
Zhang, Z., Gao, Y., Xu, M. Q., Wang, C. J., Fu, X. H., Liu, J. B., et al. (2019). miR-181a regulate porcine preadipocyte differentiation by targeting TGFBR1. Gene 681, 45–51. doi: 10.1016/j.gene.2018.09.046
Zhao, X. H., Yang, Z. Q., Bao, L. B., Wang, C. Y., Zhou, S., Gong, J. M., et al. (2015). Daidzein enhances intramuscular fat deposition and improves meat quality in finishing steers. Exp. Biol. Med. 240, 1152–1157. doi: 10.1177/1535370214564755
Keywords: whole-transcriptome analysis, adipocyte differentiation, preadipocyte, mature adipocyte, differentially expressed RNAs, regulatory networks, sheep
Citation: Xiao C, Wei T, Liu LX, Liu JQ, Wang CX, Yuan ZY, Ma HH, Jin HG, Zhang LC and Cao Y (2021) Whole-Transcriptome Analysis of Preadipocyte and Adipocyte and Construction of Regulatory Networks to Investigate Lipid Metabolism in Sheep. Front. Genet. 12:662143. doi: 10.3389/fgene.2021.662143
Received: 31 January 2021; Accepted: 09 June 2021;
Published: 29 July 2021.
Edited by:
Rui Su, Inner Mongolia Agricultural University, ChinaReviewed by:
Bao Yuan, Jilin University, ChinaCopyright © 2021 Xiao, Wei, Liu, Liu, Wang, Yuan, Ma, Jin, Zhang and Cao. This is an open-access article distributed under the terms of the Creative Commons Attribution License (CC BY). The use, distribution or reproduction in other forums is permitted, provided the original author(s) and the copyright owner(s) are credited and that the original publication in this journal is cited, in accordance with accepted academic practice. No use, distribution or reproduction is permitted which does not comply with these terms.
*Correspondence: Li Chun Zhang, MjgwNzc0OTgxQHFxLmNvbQ==; Yang Cao, Y2FveWFuZzAwM0AxNjMuY29t
†These authors have contributed equally to this work
Disclaimer: All claims expressed in this article are solely those of the authors and do not necessarily represent those of their affiliated organizations, or those of the publisher, the editors and the reviewers. Any product that may be evaluated in this article or claim that may be made by its manufacturer is not guaranteed or endorsed by the publisher.
Research integrity at Frontiers
Learn more about the work of our research integrity team to safeguard the quality of each article we publish.