- 1Center for Biotechnology, Khalifa University of Science and Technology, Abu Dhabi, United Arab Emirates
- 2Department of Electrical Engineering and Computer Science, Khalifa University of Science and Technology, Abu Dhabi, United Arab Emirates
- 3Department of Biomedical Engineering, Khalifa University of Science and Technology, Abu Dhabi, United Arab Emirates
- 4Division of Psychiatry, Faculty of Health and Medical Sciences, The University of Western Australia, Crawley, WA, Australia
- 5School of Medical and Health Sciences, Edith Cowan University, Joondalup, WA, Australia
- 6Department of Genetics and Molecular Biology, College of Medicine and Health Sciences, Khalifa University of Science and Technology, Abu Dhabi, United Arab Emirates
The ethnic composition of the population of a country contributes to the uniqueness of each national DNA sequencing project and, ideally, individual reference genomes are required to reduce the confounding nature of ethnic bias. This work represents a representative Whole Genome Sequencing effort of an understudied population. Specifically, high coverage consensus sequences from 120 whole genomes and 33 whole exomes were used to construct the first ever population specific major allele reference genome for the United Arab Emirates (UAE). When this was applied and compared to the archetype hg19 reference, assembly of local Emirati genomes was reduced by ∼19% (i.e., some 1 million fewer calls). In compiling the United Arab Emirates Reference Genome (UAERG), sets of annotated 23,038,090 short (novel: 1,790,171) and 137,713 structural (novel: 8,462) variants; their allele frequencies (AFs) and distribution across the genome were identified. Population-specific genetic characteristics including loss-of-function variants, admixture, and ancestral haplogroup distribution were identified and reported here. We also detect a strong correlation between FST and admixture components in the UAE. This baseline study was conceived to establish a high-quality reference genome and a genetic variations resource to enable the development of regional population specific initiatives and thus inform the application of population studies and precision medicine in the UAE.
Introduction
The lack of diversity in genome sequencing projects and Genome Wide Association Studies (GWAS) have led to a disproportionate representation of ethnicities in DNA sequence repositories. In non-European populations, individual whole genome sequencing efforts have been completed for the Yoruba-Nigerian (Bentley et al., 2008), Chinese (Wang et al., 2008), Korean (Kim et al., 2009; Cho et al., 2016; Seo et al., 2016), Japanese (Fujimoto et al., 2010), and Indian (Gupta et al., 2012; Almal et al., 2019) populations. Within the Arabian Peninsula, Qatar (Fakhro et al., 2016), Kuwait (John et al., 2015; Thareja et al., 2015), Saudi Arabia (Ibrahim Alabdulkareem et al., 2015), and most recently the genomes of the United Arab Emirates (UAE) (AlSafar et al., 2019; Daw Elbait et al., 2020) have been described. The significant reduction in the costs of DNA sequencing has enabled the upscaling of sequencing projects by several orders of magnitude. This has led to a proliferation of a number of national genome projects including the UK-100K project (Genomics England, 2019), the genome of the Netherlands using 750 Dutch genomes (Boomsma et al., 2014), and the 100 Southeast Asian Malays (Wong et al., 2013) project, among others. These large scales are necessary to provide the necessary statistical power to assess associations with genetic diseases as well as for identifying genetic diversity of a population [i.e., to detect rare and common variants and their population specific allele frequencies (AFs)].
However, despite all these efforts, genome science is failing in considering the diversity of the humans (Popejoy and Fullerton, 2016). For instance, the combined amount of all non-European samples in the GWAS catalog is only around 19% (Mills and Rahal, 2019). As a consequence, biomarkers in individuals of Asian and African descent could be potentially misclassified due to inaccurate statistical information on each variant (Petrovski and Goldstein, 2016). The situation is particularly dire for populations of the Middle East and Emirati people, who are represented by only 0.08 and 0.05% of samples in the GWAS catalog, respectively (Popejoy and Fullerton, 2016). Efforts to study the Arabian population genetics are recent, e.g., (Almarri et al., 2020; Tay et al., 2020), and their main focus has been directed toward genomic history and admixture events in the Middle East. However, to the best of our knowledge, no dedicated constructions of UAE population specific reference genomes have been conducted to date.
It is common practice to map sequences to an existing reference genome to map genetic variants. To date, the two versions of the human reference genome, namely the NCBI GRCh37 (hg19) and GRCh38 (Lander et al., 2001; NCBI, 2009), have been primarily relied upon. These reference genomes were constructed from a few individuals of mainly Caucasian backgrounds. A number of studies have revealed the limitation of these reference genomes when used for the identification of genetic variations that are population-specific (Hugo Pan-Asian Snp Consortium Abdulla et al., 2009; Li et al., 2010; Rosenfeld et al., 2012; Lu and Xu, 2013; Popejoy and Fullerton, 2016; Levy-Sakin et al., 2019; Sherman et al., 2019) which are not from the ethnic groups that are used to construct the reference genome, such as populations of the Arabian Peninsula. This is especially true among highly admixed and understudied populations such as those of the Arabian Peninsula. In the re-sequencing efforts of these groups, the suitable analyses of DNA reads will strongly depend on the suitability of the reference genomes that are used, to uncover the true nature of the genetic heritage of these populations.
Reference genomes will only represent the major variant of the individuals that were used to construct that reference. The low number of reference samples as well as the selection bias impacts variant mapping efforts, and thus subsequent genome analysis steps negatively. As a result, many national genome initiatives have tried to remedy the ethnic bias of the reference genome by producing population-specific reference genomes (Stark et al., 2019). For example, the Danish reference genome was constructed from sequencing and “de novo” assembly of 150 genomes of Danes (Maretty et al., 2017), the Qatari genome from 1,005 individuals from Qatar (88 whole genomes, 917 exomes) (Fakhro et al., 2016), and the Vietnamese reference from 50 genomes collected from the 1,000 genome project (Thanh et al., 2015).
The underrepresentation of genomes from the people of the UAE in global genome databases (Popejoy and Fullerton, 2016) makes analysis problematic. The information gleaned using genome references and AFs from other populations will impact negatively on the nation’s desire to implement precision medicine tools and individualized therapeutic strategies. This challenge led to the inception of the 1,000 UAE genome project (Al-Ali et al., 2018), an initiative with the goal to establish a high quality UAE specific Reference Genome (UAERG) that accounts for the unique and diverse genetic information of the UAE population. The knowledge generated from the analysis of the reference genome would support the accurate classification of genome variants and subsequently the development of personalized medicine strategies which are expected to contribute to improvements in the local healthcare system. In addition, the availability of a representative reference panel of genomes is likely to improve haplotype imputation accuracy of genotype arrays and hence increases their power in GWAS.
This study has established a baseline of the highest quality for future genome sequencing efforts in the UAE. A comprehensive catalog of short and structural variants (SVs) in particular novel variants found in the UAE population has been prepared and listed herewithin. These are fundamental to our understanding of human genetic variations in the UAE, in the context of the people who reside in the Middle East as well as global populations. This contribution is also a timely effort to counter the disproportionate representation of the genetic variation of Middle Eastern representatives, which if unaddressed, will lead to healthcare inequality in the application of precision medicine.
Materials and Methods
Sampling and DNA Extraction
A total of 1,028 UAE nationals were recruited for this study as part of the 1,000 Arab genome project (Al-Ali et al., 2018). One thousand of the samples were genotyped using the Illumina OmniExome genotype arrays (San Diego, CA, United States). Of these samples, 129 samples – 125 for the UAE genome reference construction and four for testing- were selected as the most representative individuals among the sampled set of the population (see section “Selection of Unrelated Samples and Admixture Calculation”). These 129 samples were sequenced using whole genome sequencing (WGS) and another 33 samples were sequenced using whole exome sequencing (WES) using Illumina paired-end sequencing technology.
All sequenced subjects provided their written informed consent. This study has been approved by the Institutional Ethics Committee of Mafraq Hospital in Abu Dhabi (MAF-REC_07). The inclusion criteria of the studied subjects were as follows: UAE nationals, >18 years old, capable to understand their contribution to the study, and the ability to provide informed consent. Saliva samples were collected from all subjects using the Oragene OGR 500 kit. The prepIT®L2P system was used to extract genomic DNA (gDNA) from the saliva samples. All experiments were performed in accordance with relevant guidelines and regulations.
Library Preparation
Libraries for each individual were prepared from the cleaned and sheared gDNA using the protocol provided and recommended by the manufacturer of the Illumina TruSeq® DNA PCR Free Library Prep kit (Illumina Inc., San Diego, CA, United States) and TruSeq Exome Library Prep kit in case of WES. The indexed paired-end libraries were then quantified using the Denovix DS 11 FX Fluorometer and sizes were confirmed using the Advanced Analytical Fragment Analyzer (Advanced Analytical Technologies Inc. where). The libraries were then sequenced using the Illumina Platform for paired-end WGS.
Selection of Unrelated Samples and Admixture Calculation
For the selection of the most representative subjects among the recruited individuals from the UAE population, a phylogenetic tree from the genotype arrays of the 1,000 samples, each holding 2.3M variants after cleaning was constructed. The phylogenetic tree was generated using the identity-by-state distance measure from PLINK for creating the distance matrix and BioPython’s Phylo module to construct a neighbor joining tree. We select every eighth sample from the ordered list of tree leaves, to ensure that representatives were picked across the entire span of the phylogenetic tree. Furthermore, the KING (Manichaikul et al., 2010) tool was used to test for inferred relationships among the selected sample. The KING tool takes as input the. bed file compiled by PLINK from the genotype arrays.
As outlined in Figure 1, the joint variant calling workflow is designed to run on our in-house high performance computing (HPC) center which comprises 92 compute nodes, each with 24 cores and 256 GB of memory, featuring the IBM LSF queuing system for parallel job processing. The workflow started with quality control (QC) processes that are applied to the raw data. Firstly, the FastQC tool (Andrews, 2010) was used, and by scanning the result files, only samples that passed the QC are used in the downstream analysis. The FastQC results also guided the parameter selection for the subsequent trimming step, where the Trimmomatic tool (Bolger et al., 2014), which removes low quality and short reads, was used. The Admixture calculation is described in (Tay et al., 2020).
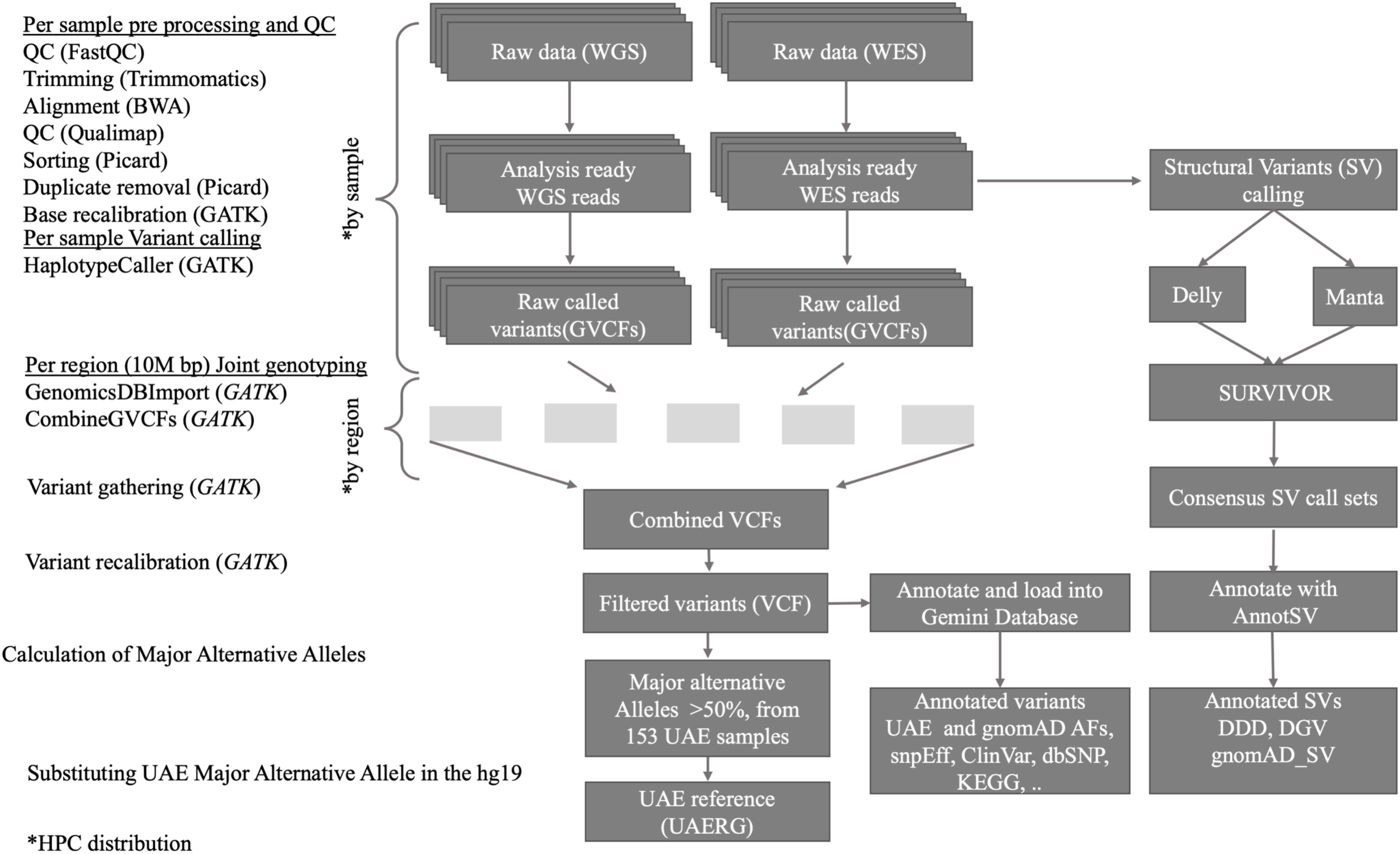
Figure 1. Overview of the data processing workflow, resources, and tools used for the joint short and structural variant calling and reference genome construction from the 153 UAE samples.
Variant Calling and Joint Genotyping Workflow
The raw reads from each of the 162 UAE nationals were mapped in parallel against the standard reference genome hg19 (Lander et al., 2001) using BWA-MEM v0.7.12 (Li and Durbin, 2010). The mapping quality and mean coverage per sample were calculated using Qualimap v2.2.1 (Garcia-Alcalde et al., 2012), where five genomic samples with coverage <10X were discarded, thus retaining 157 samples. Then, for each sample, the duplicate reads were marked and removed and the resulting BAM files were sorted by applying Picard v2.9.4 tools (Picard toolkit, 2018). The output files – one BAM file per sample – contain the analysis-ready reads for the subsequent processes.
Variants were called using the Genome Analysis Toolkit (GATK) version 4.0.6.0 Genomic Variant Call Format (GVCF) workflow (McKenna et al., 2010; DePristo et al., 2011). Variants from all samples were called individually using the GATK HaplotypeCaller with the – ERC GVCF parameter to produce GVCFs files. GVCF files were beneficial for joint genotype calling in the downstream analysis as they maintain sequencing depth information for non-variant loci which then facilitates the distinction of variant, reference, and no-call states for any site of interest.
The GVCF workflow enables us to operate on a population-wide scale by performing joint genotype calling from a cohort of multiple samples, thus improving variant calls in terms of accuracy. It inherits the advantages of classic joint variant calling over single and batch calling while being computationally efficient. The latter is owed to the fact that GATK’s Haplotype caller in GVCF mode can be run individually, and thus in parallel, on all samples (as shown in Figure 1, upper part). Subsequently, joint genotype calling on all 120 genomes and 33 exomes simultaneously were then performed. GATK’s new features Genomics DB Import and Genotype GVCFs were utilized. These steps were computationally expensive and were facilitated by parallel processing of GVCFs files split into 10M base pair sized regions (see Figure 1).
The Genotype GVCF step produces multiple VCF files that are then combined into one file that contains the integrated variants from all samples. The resulting VCF file is then subjected to filtering using the GATK VQSR which produces high-quality variants [single nucleotide polymorphisms (SNPs) and INDELs] that passed the VQSR filter.
United Arab Emirates Reference Genome Construction and Testing
To identify the major AFs within the UAE samples with reference to the hg19, the AF for each SNP and INDEL’s positions were calculated. Then the positions where the reference alleles were different from the majority alleles on the UAE genomes were identified. Finally, to construct the UAERG, the hg19 reference genome was modified at these positions by replacing at each site in the hg19 with the major allele in UAE making it the new reference allele in the UAERG.
To assess the advantages of using the UAERG over the hg19 reference for variant detection in the UAE individuals, four extra whole genome sequenced samples were selected (samples not used in the reference genome construction). The variant calling pipeline was run using the raw data of each of the four samples where the mapping step was performed twice, one time against the standard genome (hg19) and another against the newly constructed reference genome (UAERG), for the four samples. The process resulted in two sets of called variants that were evaluated by calculating the difference of called variants from the two reference genomes.
The Python scripts for the reference genome construction have been made available at https://github.com/henschellab/ReferenceGenome. The repository also contains a reference genome construction script that works in parallel (by chromosome) on the previously mentioned HPC infrastructure.
The study by Fakhro et al. (2016) generated a similar dataset for 1,005 individuals from Qatar (88 genomes, 917 exomes). Each SNP in the UAE dataset was checked against the variant calling file from the Qatar dataset. Due to the nature of the dataset, the comparison to the UAE SNPs data was limited to biallelic SNPs (see script overlap.py).
Annotation of Variants
In an effort to facilitate downstream variant analysis, the variant toolset, Vt (Tan et al., 2015) was used for decomposing (multi allelic variants) and normalizing the final variants call set (vcf file). The GEMINI tool was then used to annotate each variant by integrating several clinical and functional genome annotations including vcftools (Danecek et al., 2011), dbSNP, ClinVar (Landrum et al., 2018), snpEff (Cingolani et al., 2012), KEGG (Kanehisa and Goto, 2000), and gnomAD’s exons AFs. We additionally added the gnomAD’s genomes AFs to the database.
Characterization of the UAE Ancestry Using Y and Mitochondrial DNA Haplogroups
The mitochondrial DNA (mtDNA) variants were extracted from vcf files generated from all samples. The resulting mtDNA vcf files were then lifted over to the revised Cambridge Reference Sequence of human mitochondrial DNA (rCRS) (Andrews et al., 1999) using the PICARD “LiftoverVcf” tool before using Haplogrep (Weissensteiner et al., 2016) to assign the respective mitochondrial haplogroups.
As for the identification of the Y-DNA haplogroups, the Y chromosome was extracted from the vcf files for each male sample using the bcftools. The Yhaplo (Poznik, 2016) python module yhaplo.callHaplogroups was then used to detect the haplogroups from the provided vcf files.
Structural Variant Calculation
The SVs for the 120 WGS samples were generated using Manta (Chen et al., 2016) and Delly (Rausch et al., 2012) joint genotyping germline SV calling workflows parallelized on our in-house HPC. For consensus SV call sets from the results of the two callers, the SURVIVOR (Jeffares et al., 2017) tool was used to merge across SV callers and individuals and generate a union call set and an intersection call set, for which the SVs frequency was calculated. The tool AnnotSV (Geoffroy et al., 2018), an integrated tool for structural variations annotation; including annotations from DDD and DGV, and gnomAD_SV; was used to annotate the SV calls as illustrated in Figure 1.
Visualization and Pathway Enrichment Analysis
Visual representation of the spatial variability of SNVs and SVs across the UAE genomes has been generated using Circos (Krzywinski et al., 2009). Furthermore, the variants of the highest peaks of the visualization, has been selected to perform Reactome Pathways (Croft et al., 2011) Enrichment analysis using SNPnexus (Dayem Ullah et al., 2012).
Results
Sequences from 153 (120 WGS and 33 WES) UAE nationals were used to comprehensively describe the genetic make-up of the local population. Observed genotypes and AFs were defined. The collective variant calls were used to construct the UAERG. The suitability of such a bespoke reference genome was assessed by calling variants on the four test samples. One motivation for building the UAERG was to exclude variant calls that are predominant in the UAE population and are thus very unlikely to be disease relevant. In addition, several informative statistics on genome variants and population metrics were calculated.
Sample Selection and Information on Subjects and Alignment Statistics
Sample selection was performed to maximize diversity according to the method in section “Selection of Unrelated Samples and Admixture Calculation.” Figure 2 shows our unique representation of diversity using principal component analysis (PCA) that incorporates admixture plot data as a pie chart used for selecting samples of interest for sequencing. The zoomed insets in Figure 2, clearly reflects the genetic diversity of the UAE population. Although around 40% of the samples have a major Middle Eastern admixture component (>70%), the rest of the samples shows significant admixture with other world populations predominantly with Central/South Asia and Sub-Saharan Africa.
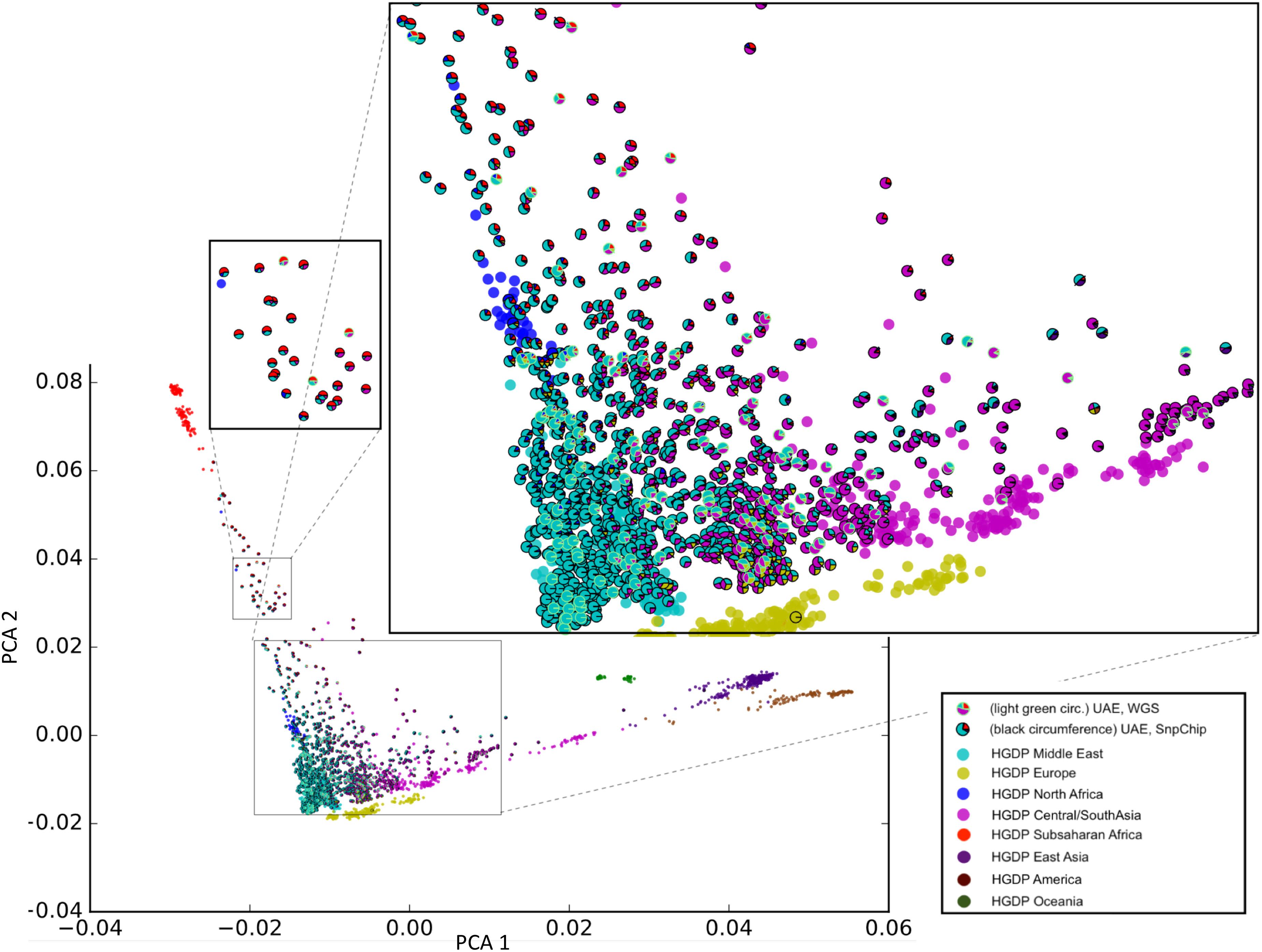
Figure 2. Principal component analysis (PCA)/Admixture plot of 1,000 UAE nationals and 1,043 samples from the human genome diversity project (HGDP). From the phylogenetic tree of 1,000 genotype arrays, 120 UAE samples (predominantly cyan color with outline) were selected for whole genome sequencing. The admixture of UAE samples is shown as pie charts, with sector coloring consistent with HGDP population colors. The zoomed-in views display the genetic diversity of the UAE population with admixtures predominated by Middle Eastern, Central/South Asia (large zoomed inset), and Sub-Saharan Africa (small zoomed inset).
The phylogenetic selection method (see sections “Materials and Methods” and “Selection of Unrelated Samples and Admixture Calculation”) included samples across the entire plane spanned by the first principal components (X-axis). Samples with different admixture proportions were selected. Specifically, samples with nearly 100% Middle-eastern component, as well as representatives with Sub-Saharan African and Central/South Asian components were selected. This shows that the selection method was capable of capturing the genetic diversity that was determined by the array-based genotype pre-analysis.
The mapping statistics for the 153 raw sequencing reads that were mapped to the hg19 reference genome were calculated and shown in Table 1. The quality of the mapping data affects the quality of the downstream processing of the data. The results were consistent with high-quality mapping scores with an average mapped read percentage of 87 and 99% and a mean mapping quality of 49 and 57 (with 60 being the highest possible score) for the WGS and WES, respectively. Furthermore, average coverages of 28.8X for the WGS and 44.9X for the WES were established.

Table 1. The average alignment and genome coverage for the 153 WGS/WES from mapping the raw reads of each sample to the hg19 reference genome.
Joint Genotyping and UAE Specific AF Calculation
Genotyping and variant calling of the 153 genomes of the UAE nationals resulted in 29,165,331 million variants of which 23,038,090 passed the GATK VQSR filter. Of the total number of variants, 92% (21,247,919) were “known” with respect to dbSNP (Sherry et al., 2001) (Build151) and 8% were “novel” (Table 2). The number of variants with major alternative allele frequencies (MAAF) was calculated by considering each AF from the 153 samples and then selecting those with AFs that were different from the reference by greater than 50%. The MAAF for each chromosome is shown in Table 2.
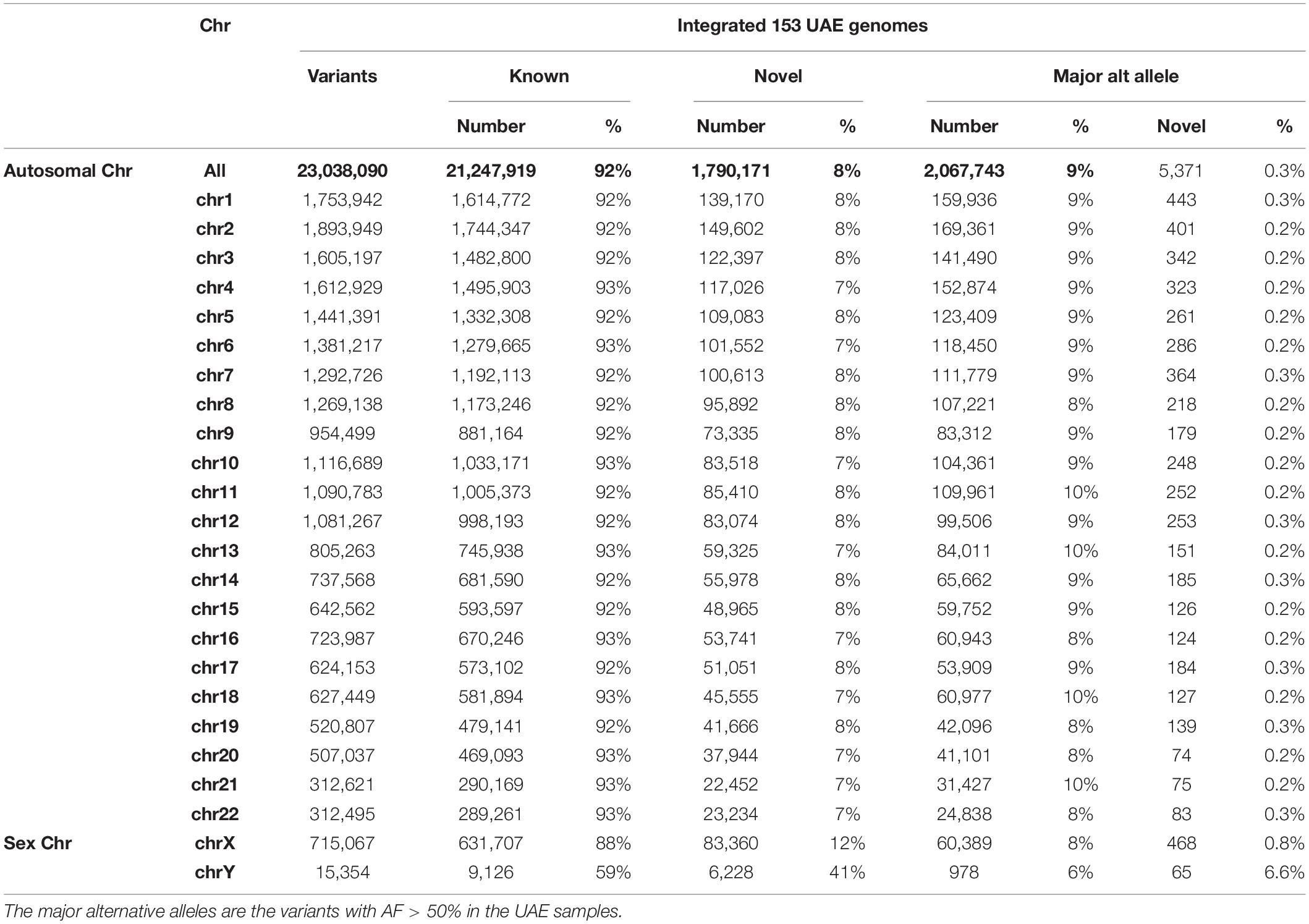
Table 2. The summary of autosomal and sex chromosomes’ variants called by genotyping of the 153 UAE nationals showing the known and novel number of variants based on their overlap with dbSNP (Build151).
The 23,038,090 variants were categorized into two main groups (major reference allele and major alternate allele). These two groups were further subcategorized into four groups based on its AF: (1) the UAE rare alternate allele for those with an alternate AF of up to 5%, (2) common alternate allele (between 5 and 50% AF), (3) common reference allele (from 50 to 95% alternate AF), and (4) rare and unobserved reference allele (from 95 to 100% alternate AF) (Figure 3).
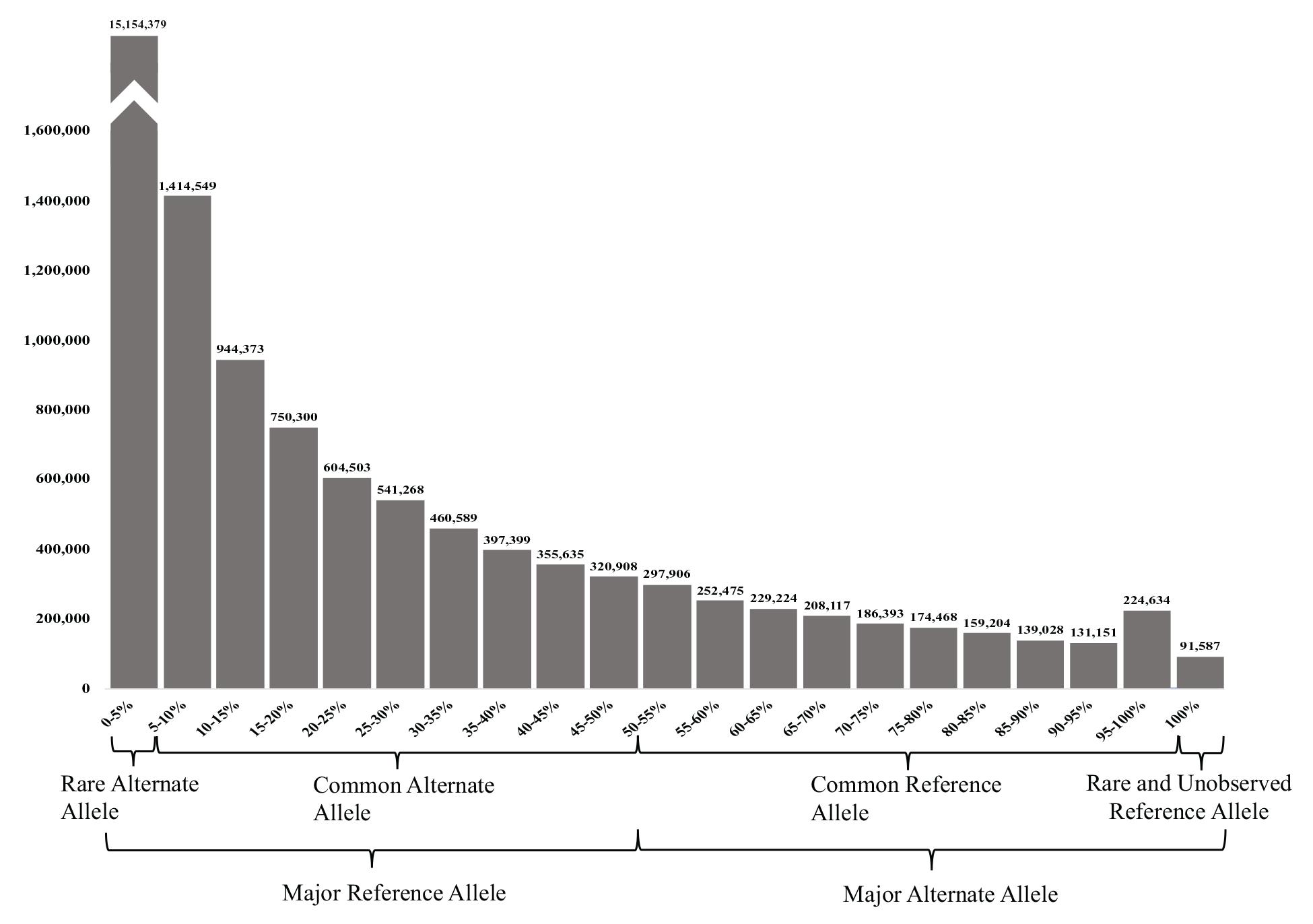
Figure 3. Allele frequency (AF) histogram of all filtered variants identified in the 153 samples. The histogram shows the number of variants against their AFs in (5% intervals). The highest peak represents the number of variants with rare alternate AFs of <5%, while the less common (lowest number of variants) had the rare or unobserved reference allele with 100% alternate AFs.
Predictably, the majority of the variants (65.78%) had a major reference allele with rare alternate AFs of <5, and 25.13% had the common alternate allele. Less common were the variants with MAAF, where 7.71% of the variants had a common reference allele of between 50–95 and 0.98% of the variants had a rare or unobserved reference allele with 95–100 and 0.40% had 100% alternate AFs (see Figure 3).
United Arab Emirates Reference Genome Calculation and Testing
The UAERG was generated by integrating variants with MAAF frequencies of greater than 50% that were identified in the cohort of 153 UAE samples, into the hg19 reference genome. This resulted in the construction of the UAERG genome by replacing 2,067,743 sites in the hg19 reference genomes with the Major Alleles calculated for the UAE for both SNPs and INDELs.
The UAERG was subsequently tested using four genomes from samples that were not used to construct the reference genome. The variants for each of the four test samples were called by running the same variant calling pipeline twice using the two reference genomes hg19 and UAERG separately. Although no significant change in read per locus coverage was observed, the alignment quality improved with an increase of up to 5.3 million base pairs that were mapped to the UAERG in comparison to the hg19 reference. This, in turn, provided greater statistical power to variant calling where a reduction of up to 990,664 called variants per genome when using the UAERG in comparison to hg19 (see Table 3).
Comparison With Similar Data From the Region
A similar study conducted by Fakhro et al. (2016) compiled a catalog of around 24 million variants from 1,005 samples from the population of Qatar. For comparability purposes, the SNPs from this UAE effort were reduced to only contain biallelic data, resulting in 18,894,377 SNPs. When comparing the integrated (WGS and WES) autosomal biallelic SNPs from the UAE with the data from Qatar (20,159,695 SNPs), an overlap of 12,286,745 SNPs was found (Supplementary Figure S1).
While the overlap of 65% with the Qatari population was substantial, the distinct variants from the UAE population could be of added value to research efforts that seek to catalog global and local variants (Tadmouri et al., 2006; Scott et al., 2016). The frequencies of the UAE-specific variants were further analyzed. From the comparison with the Qatar dataset, 1,261,482 variants that occurred with an allele count (AC) >1 were unique to the UAE dataset. Moreover, in terms of AFs thresholds above 1, 5, 10, and 50%, 423,486; 23,974; 17,648; and 4,025 UAE specific variants were observed, respectively.
Annotation of Variants
The resulting UAE database comprises 25,754,157 variants (19,929,600 SNPs, 2,827,383 short deletion, 2,906,803 short insertions, and 89,971 unknown). Additionally, all variants were annotated with AFs derived from this UAE study as well as AFs from gnomAD’s (Karczewski and Francioli, 2017) different populations. A total of 8,726,217 variants were found to be common in the UAE as they had AFs of more than 0.05, of which 611 variants were found to be UAE population-specific being rare (AF < 0.01) when compared to exons AFs from all gnomAD’s populations. When using genomes AFs instead of exons AFs from gnomAD, 13,112 UAE population-specific variants were identified. Moreover, the GEMINI (Paila et al., 2013) tool impact annotations were used to describe the functional consequence of the called variants. A total of 7,429, 98,288 and 25,645,131 variants were of high, medium, and low impact, respectively. Of greater interest, were the variants that were not found in repeat regions (using GEMINI Repeatmasker annotation) and were novel in contrast to dbSNP build 151 (Table 4).
Table 5 shows the variants with high and medium functional effects that are specific to the UAE population. These were common in the UAE cohort (MAF > 0.05) but were rare or missing in all gnomAD populations (MAF < 0.01).
Loss of Function Analysis
A total of 1,669 variants with an AF of >1% that cause a loss of function (LoF) in the genes according to GEMINI were identified. There were 1,033 indels, 625 SNPs, and 11 of unknown variant types. If we further narrow the search for LoF variants to those common in the UAE samples (AF > 5%) and rare elsewhere (i.e., less than 1% in all gnomAD populations when using AFs from the gnomAD exon catalog), 15 variants with AFs between 0.052 and 0.345 were detected. Four of the 11 affected genes were associated with different cancer types: MUC6 and ZNF717 (prostate), SPEN (breast), and STK33 (pancreas). While 3 other genes were associated with inborn genetic diseases: NRP2 (Hirschsprung disease 1), STAG (stag2 related disorder), and HTT (Huntington’s Chorea) (Supplementary Table S3).
Heterozygosity/Homozygosity Ratios
The heterozygosity to homozygosity (het/hom) ratio is not only a tool for QC, it also provides an indication of ancestry (Wang et al., 2015). We found that UAE nationals with high Sub-Saharan African admixture also had a high het/hom ratio. Specifically, the individual (UAE_S120) with the highest Sub-Saharan African admixture (58.2%) had a het/hom ratio of 2.135 and was also the individual with the lowest inbreeding coefficient FST, at 0.405. On the other hand, the sample (UAE_S116) with the lowest het/hom ratio (1.25) had an inbreeding coefficient of 0.562 (Supplementary Figures S2, S3) and has 99.9% Middle Eastern ancestry (Supplementary Table S1). Throughout the entire cohort, we observe a strong negative correlation between FST and Sub-Saharan African admixture, with Pearson coefficient of −0.745. Likewise, we observe a weak positive correlation between FST and Middle Eastern component with Pearson coefficient 0.406 (Supplementary Figure S3).
Mitochondrial and Y-DNA Ancestry Haplogroups of the UAE Population
The distribution of the mtDNA haplogroups within the UAE population showed influences from populations in neighboring countries as well as from remote geographical regions. The haplogroup analysis revealed the major influence of H, J, K, T, and U. Fifty five percent of the study group carried these haplogroups that predominant in Europe and West Asia. The next most common haplogroups were the R and M (21%) haplogroups that are mainly found in Central/South Asia followed by the African haplogroups L at 17%. Finally, the N, G, D, and B haplogroups together were found in 7% of the study group, confirming a weaker influence from East Asia (Supplementary Figure 4a).
The analysis of the Y haplogroups in male subjects showed major influences from the Middle East and Central/South Asia. The most common J haplogroup was found in 52% of the study group, a lineage that is mainly found in the Middle East. The next largest group at 21% was the E haplogroup, primarily found in West and East Africa. Fourteen percent of the study group carried the R haplogroup that is primarily found in Central and South Asia as well as in Eastern Europe. There were minor influences from the G, L, T, and Q haplogroups at 5, 4, 2, and 1%, respectively, haplogroups that are native to Central South Asia and the Middle East (Supplementary Figure 4b).
Structural Variants Call Set Generation and Evaluation
We generated SV call sets using the Manta (Chen et al., 2016) and Delly (Rausch et al., 2012) tools. The rationale and choice of integrating multiple SV-callers as an effective approach for SV discovery was based on previous analyses (Werling et al., 2018; Chaisson et al., 2019; Collins, 2019). This approach has been shown to provide a balanced sensitivity and specificity due to combining the benefits from the used SV calling tools. Specifically, Manta was selected for its leading performance among all paired-end/split reads algorithms and DELLY for its maximization of sensitivity for small and balanced SV.
Depending on whether recall or precision was prioritized in subsequent analysis steps, results on both the intersection and union of the call sets were reported. For the union call set, 137,713 SVs (present in at least five individuals), including 8,845 inversions, 11,818 insertions, 27,620 duplications, 40,582 deletions, and 48,902 transitions (Supplementary Table S2) were identified. The AF distribution is shown in Supplementary Figure S5. A genome overview of the short and SVs sets using Circos (Krzywinski et al., 2009) is shown in Figure 4.

Figure 4. Circos plot of the spatial distribution of short variants and structural variants (SVs) across all chromosomes (outer ring). From outer to the inner rings: (A) short variants called from the 153 UAE samples (light red), novel (red), and novel UAE population-specific (dark red) variations which indicate regional variability characteristics (note that scales are modified for visibility), (B) loss of function (yellow) and UAE population specific variants (black line), (C) SVs consensus set (dark purple), of which the (D) through (G) rings show insertions (dark green), deletions (blue), duplications (gray), translocation (dark yellow), and inversions (orange), respectively. The heatmap in the innermost plot of the figure displays the frequency of SVs.
The figure shows spatial variability characteristics and allows for the identification of chromosomal positions with remarkable regional features. The variants at prominent peaks were used to perform Reactome Pathways (Croft et al., 2011) Enrichment analysis. The analysis showed that the peaks of the UAE population specific variation (black line, ring B) in chromosomes 1, 3, 4, and 13 corresponds to significant increase in variations in Glycosaminoglycan metabolism (p-value = 4.8 × 10–5), chondroitin sulfate/dermatan sulfate metabolism (p-value = 8 × 10–5), HS-GAG degradation (p-value = 6.05 × 10–4), Cristae formation (p-value = 0.001), Metabolism of carbohydrates (p-value = 0.001) and MPS I – Hurler syndrome (p-value = 0.002) pathways (Supplementary Figure S6). The LoF variations (yellow bars, ring B) peaks in chromosomes 1, at the end of 8, at the start of 11 and in 12 contained enriched variation in PTK6 promotes HIF1A stabilization (p-value = 0.031), signaling by high-kinase activity BRAF mutants (p-value = 0.016), transcriptional regulation of pluripotent stem cells (p-value = 0.016), and MAP2K and MAPK activation (p-value = 0.018) pathways (Supplementary Figure S7). Similarly, the peaks from the overall SV (purple bars, ring C) in chromosomes Y, 4, 10, 16, and 21 contained enriched variations that correspond with synthesis of PI (p-value = 0.004), TP53 regulated transcription of death receptors and ligands (p-value = 0.008), hemostasis (p-value = 0.009), and Cytosolic sulfonation of small molecules (p-value = 0.017) pathways (Supplementary Figure S8). In-depth analysis of these peaks could be of interest for further investigation when studying genomes from the UAE and those from populations of neighboring countries.
Discussion
Using best practices described by Van der Auwera et al. (2013) for genome data QC, alignment, variant discovery techniques, and High-Performance Computing, the first UAERG was constructed. It was based on 153 high coverage samples from healthy subjects selected to represent the UAE nationals. This was achieved by using an in-house pipeline that mapped sequenced reads to the hg19 reference genome and then used the GATK4 (GVCF) genome analysis workflow (Figure 1) that in turn deployed joint genotype calling of the UAE genomes.
To avoid sampling bias, the WGS samples were carefully chosen from a cohort of 1,000 samples for which genotype array data was available. The choice of the representative samples was guided by a systematic phylogenetic analysis that selected samples from different parts of the tree to include samples with different ethnic admixtures that better represented the current UAE population.
The analysis of Y-DNA showed strong paternal influences from the Middle East and West Asia. The mtDNA analysis revealed a more diverse maternal origin with possible influences from North Africa, the Middle East; West, East, and South Asia as well as Europe, which could suggest that the diversity of the UAE population is influenced to a greater extent by the maternal ancestry.
The frequencies of all variants in the UAE genomes were calculated and their position relative to the NCBI reference genome hg19 were identified. Over 2 million alleles on hg19 were altered by replacing all the positions with the UAE major alternative alleles with frequencies of 50% or higher, a strategy that was used in the construction of the Qatar and Vietnam reference genomes (Thanh et al., 2015; Fakhro et al., 2016). From this effort, a reference genome for the UAE was generated for the first time. The UAERG should be more compatible with UAE genomes than the reference genomes that have been widely used to date. The UAERG is expected to be a fundamental tool that will advance genome and public health research in the UAE.
The benefit of the new reference genome was established by testing four WGS from UAE individuals by showing significant enhancement of the genotype calling quality in the form of a reduction of approximately 19% called variants which corresponds to approximately one million less called variants.
The UAE-specific AFs will decrease the rates of misdiagnosis of genetic diseases that mainly depend on values from populations with genetic compositions that differ from the UAE and that, for example, by ruling out known disease-causing variants with high AFs in the UAE population. The variant reduction; in the magnitude of hundreds of thousands due to the use of the UAERG; is deemed helpful in the variant filtration step when searching for disease causing variants by reducing the number of variants that need to be considered in the analysis. Furthermore, records of variants with MAAF > 50% were cataloged as well as their associated pathogenicity whenever available (Supplementary Table S4).
Although the use of data from similar populations in the region could be a beneficial resource, as one could see in the comparison with the Qatari variants (65% of the generated UAE variants were overlapping with the Qatari data), there was still a significant difference (more than 6 million) variants between the two populations. These results show, that the diversity of the Arabian Peninsula has not been exhaustively covered by previous studies and that our dataset provides a substantial contribution toward that goal.
Furthermore, this reference panel is an inevitable component for high accuracy genotype imputation, which enriches datasets from cost efficient genome sequences from SNP arrays.
The sequencing was based on short-read technology, which limits SV discovery as well as de novo assembly. However, as demonstrated by Collins (2019), the use of various SV callers can significantly reduce the number of undetected variants.
In addition, the integration of population specific variants into a reference genome avoids coordinate lift-over inaccuracies derived from de novo assemblies, which remain a challenge for subsequent annotation procedures. Moreover, the homogeneous quality of samples enables the extraction of population characteristics from a population more evenly, in contrast, to study designs with emphasis on the high quality of a single or few sample(s). Therefore, samples with low coverage are likely to contribute less to a consensus genome due to their smaller call sets. As it is unlikely that quality/coverage reflects representativeness, we here avoid this study-based representation bias.
Data Availability Statement
The VCF file including the variants and allele frequencies produced by this study is available at the European Genome Archive (EGA) under the accession number “EGAS00001004537”.
Ethics Statement
The studies involving human participants were reviewed and approved by the Institutional Ethics Committee of Mafraq Hospital in Abu Dhabi (MAF-REC_07). The patients/participants provided their written informed consent to participate in this study.
Author Contributions
HA and GT conceived the 1,000 UAE genome project and received funding that contributed toward sequencing the individuals in this publication. GD and AH performed the analyses and prepared the first draft of the manuscript. All authors developed the manuscript further and contributed to the final version.
Funding
Genome sequencing was funded from internal research awards from the Khalifa University awarded to HA.
Conflict of Interest
The authors declare that the research was conducted in the absence of any commercial or financial relationships that could be construed as a potential conflict of interest.
Acknowledgments
We acknowledge the participants, whose contributions made the construction of the UAERG possible. We would also like to thank the numerous technical staff of the Center for Biotechnology who over the years have assisted in the compilation of the genome sequences from these volunteers.
Supplementary Material
The Supplementary Material for this article can be found online at: https://www.frontiersin.org/articles/10.3389/fgene.2021.660428/full#supplementary-material
References
Al-Ali, M., Osman, W., Tay, G. K., and AlSafar, H. S. (2018). A 1000 Arab genome project to study the Emirati population. J. Hum. Genet. 63, 533–536. doi: 10.1038/s10038-017-0402-y
Almal, S., Jeon, S., Agarwal, M., Patel, S., Patel, S., Bhak, Y., et al. (2019). Sequencing and analysis of the whole genome of Indian Gujarati male. Genomics 111, 196–204. doi: 10.1016/j.ygeno.2018.02.003
Almarri, M. A., Haber, M., Lootah, R. A., Hallast, P., Turki, S. Al, Martin, H. C., et al. (2020). The genomic history of the middle east. bioRxiv [Preprint]. doi: 10.1101/2020.10.18.342816
AlSafar, H. S., Al-Ali, M., Elbait, G. D., Al-Maini, M. H., Ruta, D., Moya, B. P., et al. (2019). Introducing the first whole genomes of nationals from the United Arab Emirates. Sci. Rep. 9:14725. doi: 10.1038/s41598-019-50876-9
Andrews, R. M., Kubacka, I., Chinnery, P. F., Lightowlers, R. N., Turnbull, D. M., and Howell, N. (1999). Reanalysis and revision of the Cambridge reference sequence for human mitochondrial DNA. Nat. Genet. 23:147. doi: 10.1038/13779
Andrews, S. (2010). FastQC A Quality Control tool for High Throughput Sequence Data. Berlin: Springer.
Bentley, D. R., Balasubramanian, S., Swerdlow, H. P., Smith, G. P., Milton, J., Brown, C. G., et al. (2008). Accurate whole human genome sequencing using reversible terminator chemistry. Nature 456, 53–59. doi: 10.1038/nature07517
Bolger, A. M., Lohse, M., and Usadel, B. (2014). Trimmomatic: a flexible trimmer for Illumina sequence data. Bioinformatics 30, 2114–2120. doi: 10.1093/bioinformatics/btu170
Boomsma, D. I., Wijmenga, C., Slagboom, E. P., Swertz, M. A., Karssen, L. C., Abdellaoui, A., et al. (2014). The Genome of the Netherlands: design, and project goals. Eur. J. Hum. Genet. 22, 221–227. doi: 10.1038/ejhg.2013.118
Chaisson, M. J. P., Sanders, A. D., Zhao, X., Malhotra, A., Porubsky, D., Rausch, T., et al. (2019). Multi-platform discovery of haplotype-resolved structural variation in human genomes. Nat. Commun. 10:1784. doi: 10.1038/s41467-018-08148-z
Chen, X., Schulz-Trieglaff, O., Shaw, R., Barnes, B., Schlesinger, F., Källberg, M., et al. (2016). Manta: rapid detection of structural variants and indels for germline and cancer sequencing applications. Bioinformatics 32, 1220–1222. doi: 10.1093/bioinformatics/btv710
Cho, Y. S., Kim, H., Kim, H. M., Jho, S., Jun, J., Lee, Y. J., et al. (2016). An ethnically relevant consensus Korean reference genome is a step towards personal reference genomes. Nat. Commun. 7:13637. doi: 10.1038/ncomms13637
Cingolani, P., Platts, A., Wang le, L., Coon, M., Nguyen, T., Wang, L., et al. (2012). A program for annotating and predicting the effects of single nucleotide polymorphisms, SnpEff: SNPs in the genome of Drosophila melanogaster strain w1118; iso-2; iso-3. Fly 6, 80–92. doi: 10.4161/fly.19695
Collins, R. L. (2019). An open resource of structural variation for medical and population genetics. bioRxiv [Prprint]. doi: 10.1101/578674v1
Croft, D., O’Kelly, G., Wu, G., Haw, R., Gillespie, M., Matthews, L., et al. (2011). Reactome: a database of reactions, pathways and biological processes. Nucleic Acids Res. 39, D691–D697. doi: 10.1093/nar/gkq1018
Danecek, P., Auton, A., Abecasis, G., Albers, C. A., Banks, E., DePristo, M. A., et al. (2011). The variant call format and VCFtools. Bioinformatics 27, 2156–2158. doi: 10.1093/bioinformatics/btr330
Daw Elbait, G., Henschel, A., Tay, G. K., and Al Safar, H. S. (2020). Whole genome sequencing of four representatives from the admixed population of the United Arab Emirates. Front. Genet. 11:681. doi: 10.3389/fgene.2020.00681
Dayem Ullah, A. Z., Lemoine, N. R., and Chelala, C. (2012). SNPnexus: a web server for functional annotation of novel and publicly known genetic variants (2012 update). Nucleic Acids Res. 40, W65–W70. doi: 10.1093/nar/gks364
DePristo, M. A., Banks, E., Poplin, R., Garimella, K. V., Maguire, J. R., Hartl, C., et al. (2011). A framework for variation discovery and genotyping using next-generation DNA sequencing data. Nat. Genet. 43, 491–498. doi: 10.1038/ng.806
Fakhro, K. A., Staudt, M. R., Ramstetter, M. D., Robay, A., Malek, J. A., Badii, R., et al. (2016). The Qatar genome: a population-specific tool for precision medicine in the Middle East. Hum. Genome Var. 3:16016. doi: 10.1038/hgv.2016.16
Fujimoto, A., Nakagawa, H., Hosono, N., Nakano, K., Abe, T., Boroevich, K. A., et al. (2010). Whole-genome sequencing and comprehensive variant analysis of a Japanese individual using massively parallel sequencing. Nat. Genet. 42, 931–936. doi: 10.1038/ng.691
Garcia-Alcalde, F., Okonechnikov, K., Carbonell, J., Cruz, L. M., Götz, S., Tarazona, S., et al. (2012). Qualimap: evaluating next-generation sequencing alignment data. Bioinformatics 28, 2678–2679. doi: 10.1093/bioinformatics/bts503
Genomics England (2019). The National Genomics Research and Healthcare Knowledgebase v5. London: Genomics England.
Geoffroy, V., Herenger, Y., Kress, A., Stoetzel, C., Piton, A., Dollfus, H., et al. (2018). AnnotSV: an integrated tool for structural variations annotation. Bioinformatics 34, 3572–3574. doi: 10.1093/bioinformatics/bty304
Gupta, R., Ratan, A., Rajesh, C., Chen, R., Kim, H. L., Burhans, R., et al. (2012). Sequencing and analysis of a South Asian-Indian personal genome. BMC Genomics 13:440. doi: 10.1186/1471-2164-13-440
Hugo Pan-Asian Snp Consortium Abdulla, M. A., Ahmed, I., Assawamakin, A., Bhak, J., Brahmachari, S. K., et al. (2009). Mapping human genetic diversity in Asia. Science 326, 1541–1545. doi: 10.1126/science.1177074
Ibrahim Alabdulkareem, A. J. R., Garawi, S. Al, Aljumah, M., Al-Turki, S., and AlBalwi, M. A. (2015). Distinctive features of a saudi genome. bioRxiv [Preprint]. doi: 10.1101/015909
Jeffares, D. C., Jolly, C., Hoti, M., Speed, D., Shaw, L., Rallis, C., et al. (2017). Transient structural variations have strong effects on quantitative traits and reproductive isolation in fission yeast. Nat. Commun. 8:14061. doi: 10.1038/ncomms14061
John, S. E., Thareja, G., Hebbar, P., Behbehani, K., Thanaraj, T. A., Alsmadi, O., et al. (2015). Kuwaiti population subgroup of nomadic Bedouin ancestry—whole genome sequence and analysis. Genomics Data 3, 116–127. doi: 10.1016/j.gdata.2014.11.016
Kanehisa, M., and Goto, S. (2000). KEGG: kyoto encyclopedia of genes and genomes. Nucleic Acids Res. 28, 27–30. doi: 10.1093/nar/28.1.27
Karczewski, K., and Francioli, L. (2017). The Genome Aggregation Database (gnomAD). Toronto: McArthur & Company Publishing.
Kim, J. I., Ju, Y. S., Park, H., Kim, S., Lee, S., Yi, J. H., et al. (2009). A highly annotated whole-genome sequence of a Korean individual. Nature 460, 1011–1015. doi: 10.1038/nature08211
Krzywinski, M., Schein, J., Birol, I., Connors, J., Gascoyne, R., Horsman, D., et al. (2009). Circos: an information aesthetic for comparative genomics. Genome Res. 19, 1639–1645. doi: 10.1101/gr.092759.109
Lander, E. S., Linton, L. M., Birren, B., Nusbaum, C., Zody, M. C., Baldwin, J., et al. (2001). Initial sequencing and analysis of the human genome. Nature 409, 860–921. doi: 10.1038/35057062
Landrum, M. J., Lee, J. M., Benson, M., Brown, G. R., Chao, C., Chitipiralla, S., et al. (2018). ClinVar: improving access to variant interpretations and supporting evidence. Nucleic Acids Res. 46, D1062–D1067. doi: 10.1093/nar/gkx1153
Levy-Sakin, M., Pastor, S., Mostovoy, Y., Li, L., Leung, A. K. Y., McCaffrey, J., et al. (2019). Genome maps across 26 human populations reveal population-specific patterns of structural variation. Nat. Commun. 10:1025. doi: 10.1038/s41467-019-08992-7
Li, H., and Durbin, R. (2010). Fast and accurate long-read alignment with Burrows–Wheeler transform. Bioinformatics 26, 589–595. doi: 10.1093/bioinformatics/btp698
Li, R., Li, Y., Zheng, H., Luo, R., Zhu, H., Li, Q., et al. (2010). Building the sequence map of the human pan-genome. Nat. Biotechnol. 28, 57–63. doi: 10.1038/nbt.1596
Lu, D., and Xu, S. (2013). Principal component analysis reveals the 1000 Genomes Project does not sufficiently cover the human genetic diversity in Asia. Front. Genet. 4:127. doi: 10.3389/fgene.2013.00127
Manichaikul, A., Mychaleckyj, J. C., Rich, S. S., Daly, K., Sale, M., Chen, W. M., et al. (2010). Robust relationship inference in genome-wide association studies. Bioinformatics 26, 2867–2873. doi: 10.1093/bioinformatics/btq559
Maretty, L., Jensen, J., Petersen, B., Sibbesen, J. A., Liu, S., Villesen, P., et al. (2017). Sequencing and de novo assembly of 150 genomes from Denmark as a population reference. Nature 548, 87–91. doi: 10.1038/nature23264
McKenna, A., Hanna, M., Banks, E., Sivachenko, A., Cibulskis, K., Kernytsky, A., et al. (2010). The genome analysis Toolkit: a MapReduce framework for analyzing next-generation DNA sequencing data. Genome Res. 20, 1297–1303. doi: 10.1101/gr.107524.110
Mills, M. C., and Rahal, C. (2019). A scientometric review of genome-wide association studies. Commun. Biol. 2:9. doi: 10.1038/s42003-018-0261-x
NCBI (2009). GRCh37 - hg19 - Genome - Assembly. Available online at: https://www.ncbi.nlm.nih.gov/assembly/GCF_000001405.13/ (Accessed January 7, 2018).
Paila, U., Chapman, B. A., Kirchner, R., and Quinlan, A. R. (2013). GEMINI: integrative exploration of genetic variation and genome annotations. PLoS Comput. Biol. 9:e1003153. doi: 10.1371/journal.pcbi.1003153
Petrovski, S., and Goldstein, D. B. (2016). Unequal representation of genetic variation across ancestry groups creates healthcare inequality in the application of precision medicine. Genome Biol. 17:157. doi: 10.1186/s13059-016-1016-y
Picard toolkit (2018). Available online at: http://broadinstitute.github.io/picard/ (Accessed January 15, 2018).
Popejoy, A. B., and Fullerton, S. M. (2016). Genomics is failing on diversity. Nature 538, 161–164. doi: 10.1038/538161a
Poznik, G. D. (2016). Identifying Y-chromosome haplogroups in arbitrarily large samples of sequenced or genotyped men. bioRxiv [Preprint]. doi: 10.1101/088716v1
Rausch, T., Zichner, T., Schlattl, A., Stütz, A. M., Benes, V., and Korbel, J. O. (2012). DELLY: structural variant discovery by integrated paired-end and split-read analysis. Bioinformatics 28, i333–i339. doi: 10.1093/bioinformatics/bts378
Rosenfeld, J. A., Mason, C. E., and Smith, T. M. (2012). Limitations of the human reference genome for personalized genomics. PLoS One 7:e40294. doi: 10.1371/journal.pone.0040294
Scott, E. M., Halees, A., Itan, Y., Spencer, E. G., He, Y., Azab, M. A., et al. (2016). Characterization of Greater Middle Eastern genetic variation for enhanced disease gene discovery. Nat. Genet. 48, 1071–1076. doi: 10.1038/ng.3592
Seo, J. S., Rhie, A., Kim, J., Lee, S., Sohn, M. H., Kim, C. U., et al. (2016). De novo assembly and phasing of a Korean human genome. Nature 538, 243–247. doi: 10.1038/nature20098
Sherman, R. M., Forman, J., Antonescu, V., Puiu, D., Daya, M., Rafaels, N., et al. (2019). Assembly of a pan-genome from deep sequencing of 910 humans of African descent. Nat. Genet. 51, 30–35. doi: 10.1038/s41588-018-0273-y
Sherry, S. T., Ward, M. H., Kholodov, M., Baker, J., Phan, L., Smigielski, E. M., et al. (2001). dbSNP: the NCBI database of genetic variation. Nucleic Acids Res. 29, 308–311. doi: 10.1093/nar/29.1.308
Stark, Z., Dolman, L., Manolio, T. A., Ozenberger, B., Hill, S. L., Caulfied, M. J., et al. (2019). Integrating genomics into healthcare: a global responsibility. Am. J. Hum. Genet. 104, 13–20. doi: 10.1016/j.ajhg.2018.11.014
Tadmouri, G. O., Al Ali, M. T., Al-Haj Ali, S., and Al Khaja, N. (2006). CTGA: the database for genetic disorders in Arab populations. Nucleic Acids Res. 34, D602–D606. doi: 10.1093/nar/gkj015
Tan, A., Abecasis, G. R., and Kang, H. M. (2015). Unified representation of genetic variants. Bioinformatics 31, 2202–2204. doi: 10.1093/bioinformatics/btv112
Tay, G. K., Henschel, A., and AlSafar, H. S. (2020). Genetic diversity and low stratification of the population of the United Arab Emirates. Front. Genet. 11:608. doi: 10.3389/fgene.2020.00608
Thanh, N. D., Trang, P. T. M., Hai, D. T., Tuan, N. H. A., Quang, L. S., Minh, B. Q., et al. (2015). “Building population-specific reference genomes: a case study of vietnamese reference genome,” in Proceesings of the Seventh International Conference on Knowledge and Systems Engineering (KSE) (Piscataway, NJ: IEEE), 97–102.
Thareja, G., John, S. E., Hebbar, P., Behbehani, K., Thanaraj, A. T., Alsmadi, O., et al. (2015). Sequence and analysis of a whole genome from Kuwaiti population subgroup of Persian ancestry. BMC Genomics 16:92. doi: 10.1186/s12864-015-1233-x
Van der Auwera, G. A., Carneiro, M. O., Hartl, C., Poplin, R., Del Angel, G., Levy-Moonshine, A., et al. (2013). From FastQ data to high confidence variant calls: the Genome Analysis Toolkit best practices pipeline. Curr. Protoc. Bioinformatics 43, 11.10.11–11.10.33. doi: 10.1002/0471250953.bi1110s43
Wang, J., Raskin, L., Samuels, D. C., Shyr, Y., and Guo, Y. (2015). Genome measures used for quality control are dependent on gene function and ancestry. Bioinformatics 31, 318–323. doi: 10.1093/bioinformatics/btu668
Wang, J., Wang, W., Li, R., Li, Y., Tian, G., Goodman, L., et al. (2008). The diploid genome sequence of an Asian individual. Nature 456, 60–65. doi: 10.1038/nature07484
Weissensteiner, H., Pacher, D., Kloss-Brandstätter, A., Forer, L., Specht, G., Bandelt, H. J., et al. (2016). HaploGrep 2: mitochondrial haplogroup classification in the era of high-throughput sequencing. Nucleic Acids Res. 44, W58–W63.
Werling, D. M., Brand, H., An, J. Y., Stone, M. R., Zhu, L., Glessner, J. T., et al. (2018). An analytical framework for whole-genome sequence association studies and its implications for autism spectrum disorder. Nat. Genet. 50, 727–736. doi: 10.1038/s41588-018-0107-y
Keywords: UAE reference genome, next generation sequencing, structural variants, population representative sampling, population genetics, Arab genome, reference genome
Citation: Daw Elbait G, Henschel A, Tay GK and Al Safar HS (2021) A Population-Specific Major Allele Reference Genome From The United Arab Emirates Population. Front. Genet. 12:660428. doi: 10.3389/fgene.2021.660428
Received: 01 February 2021; Accepted: 19 March 2021;
Published: 23 April 2021.
Edited by:
Fulvio Cruciani, Sapienza University of Rome, ItalyReviewed by:
Jijun Tang, University of South Carolina, United StatesRashed Alghafri, Dubai Police, United Arab Emirates
Copyright © 2021 Daw Elbait, Henschel, Tay and Al Safar. This is an open-access article distributed under the terms of the Creative Commons Attribution License (CC BY). The use, distribution or reproduction in other forums is permitted, provided the original author(s) and the copyright owner(s) are credited and that the original publication in this journal is cited, in accordance with accepted academic practice. No use, distribution or reproduction is permitted which does not comply with these terms.
*Correspondence: Habiba S. Al Safar, aGFiaWJhLmFsc2FmYXJAa3UuYWMuYWU=
†These authors have contributed equally to this work and share first authorship