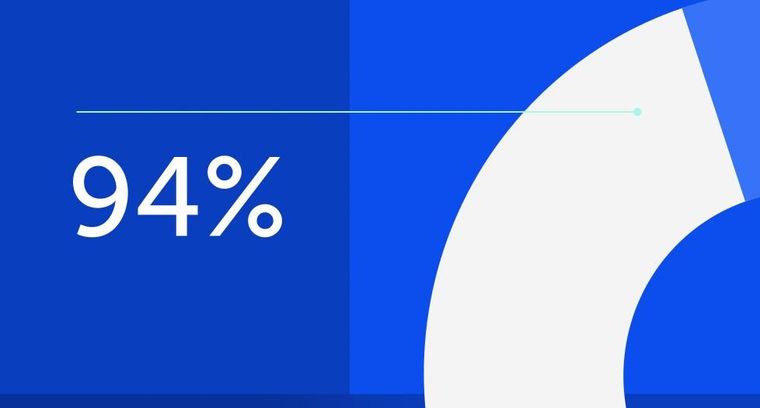
94% of researchers rate our articles as excellent or good
Learn more about the work of our research integrity team to safeguard the quality of each article we publish.
Find out more
ORIGINAL RESEARCH article
Front. Genet., 10 May 2021
Sec. Applied Genetic Epidemiology
Volume 12 - 2021 | https://doi.org/10.3389/fgene.2021.657499
This article is part of the Research TopicStatistical Approaches in Omics Data Association StudiesView all 15 articles
Cutaneous squamous cell carcinoma (cSCC) accounts for about 20% of all skin cancers, the most common type of malignancy in the United States. Genome-wide association studies (GWAS) have successfully identified multiple genetic variants associated with the risk of cSCC. Most of these studies were single-locus-based, testing genetic variants one-at-a-time. In this article, we performed gene-based association tests to evaluate the joint effect of multiple variants, especially rare variants, on the risk of cSCC by using a fast sequence kernel association test (fastSKAT). The study included 1,710 cSCC cases and 24,304 cancer-free controls from the Nurses’ Health Study, the Nurses’ Health Study II and the Health Professionals Follow-up Study. We used UCSC Genome Browser to define gene units as candidate loci, and further evaluated the association between all variants within each gene unit and disease outcome. Four genes HP1BP3, DAG1, SEPT7P2, and SLFN12 were identified using Bonferroni adjusted significance level. Our study is complementary to the existing GWASs, and our findings may provide additional insights into the etiology of cSCC. Further studies are needed to validate these findings.
Cutaneous squamous cell carcinoma (cSCC) is the second most common type of non-melanoma skin cancers, accounting for about 20% of all skin cancers and the majority of deaths attributable to non-melanoma skin cancers (Chitsazzadeh et al., 2016; Motaparthi et al., 2017; Parekh and Seykora, 2017; Que et al., 2018a). The incidence of cSCC in the United States has been increasing over the last few decades, with over 1 million annual cases in recent years (Nguyen et al., 2014; Muzic et al., 2017; Que et al., 2018a,b). The increase is also expected to continue because of the longer life expectancy, aging population and chronic ultraviolet exposure (Nguyen et al., 2014; Motaparthi et al., 2017; Waldman and Schmults, 2019). The growing mortality and morbidity of cSCC has posed immense economic burden on the national healthcare systems. Though the remission rate of cSCC cases has substantially improved, many cases were still associated with higher probability of recurrence, metastasis and poor prognosis after surgery (Motaparthi et al., 2017; Que et al., 2018a; Waldman and Schmults, 2019). It is of crucial importance to understand the pathogenesis of cSCC and to reduce the public health impact of the disease.
The etiology of cSCC has not been fully understood. However, the risk of the disease can be influenced by multiple environmental exposures. For example, higher risk of cSCC is found to be associated with increased age, fair skin color, male gender, exposure to ultraviolet radiation, immunosuppression and human papillomavirus (Chahal et al., 2016; Parekh and Seykora, 2017; Que et al., 2018a; Waldman and Schmults, 2019). Similar to all cancers, genetic susceptibility also plays an important role in the development of cSCC. Familial aggregation provides direct evidence for the heritability of cSCC (Hussain et al., 2009; Asgari et al., 2015). A few known cancer-related genes, such as TP53, CDKN2A, Ras, and NOTCH1 were also causal to skin cancers (Que et al., 2018a). Mutations with these genes may disrupt normal cell growth, cell circle and cellular signal transduction, leading to the development of the disease. In the past decade, genome-wide association studies (GWAS) have become a commonly used strategy to identify genetic variants for complex human diseases in the general population. A few GWASs have identified multiple genetic variants that are associated with the risk of cSCC, such as CADM1, AHR, SEC16A, and DEF8 (Nan et al., 2011; Asgari et al., 2016; Chahal et al., 2016; Siiskonen et al., 2016). Many findings were also successfully replicated in independent populations. These findings have provided valuable insights into the genetic etiology of cSCC.
Despite of these successes, it was estimated that the genetic variants identified by existing GWASs only account for ∼8.5% of the cSCC heritability (Sarin et al., 2020). The genetic causes of the disease remain largely unknown (Chahal et al., 2016). This challenge may be due to a number of limitations of the existing GWASs, such as insufficient statistical power to detect small to moderate genetic effects, burden of multiple testing adjustment, and overlooking potential interactions among variants (Mo et al., 2015; Nettiksimmons et al., 2016). As an alternative to the single-locus analysis, gene- or region-based analysis can be a complementary approach addressing some of those limitations. It may integrate effects of multiple genetic variants, especially rare variants, within a genetic region for improved power, reduce the computational intensities and alleviate the burden of multiple testing (Wu et al., 2010). In recent years, a number of statistical methods have been developed for conducting region-based association test. For example, a sequence kernel association test (SKAT) has been a commonly used method that evaluates the joint effects of genetic variants in a region on a disease outcome while adjusting for covariates (Wu et al., 2011). It uses flexible kernel functions to integrate the effects from multiple variants and allows the effect of causal variants to be bi-directional. Further, a fast sequencing kernel association test (fastSKAT) has been developed to implement SKAT in a computational efficient fashion, especially for large-scale studies with thousands of subjects (Lumley et al., 2018). In this article, we assessed the validity of region-based fastSKAT by replicating 18 GWAS-identified SNPs using single-locus testing. We further tested the association between approximately 23,000 gene regions and cSCC outcome in five independent study populations. The results from each population were further integrated by a Fisher’s combined probability test.
The study protocol was approved by the institutional review boards of the Brigham and Women’s Hospital and Harvard T.H. Chan School of Public Health, and those of participating registries as required.
Our study included 26,014 individuals from three large prospective cohort studies in the U.S., including the Nurses’ Health Study (NHS), the Nurses’ Health Study 2 (NHS2), and the Health Professionals Follow-up Study (HPFS). The subjects were selected under a nested case-control design based on cSCC status. Cases were defined as individuals diagnosed with invasive cSCC, while controls were individuals free of cSCC or any primary type of cancers. The individuals’ characteristics, genotypes and other covariates information were collected in the NHS, the NHS2 and the HPFS studies. In this study, we partitioned the subjects into five independent sub-populations based on their genotyping platforms, including “Affymetrix,” “Illumina,” “OmniExpress,” “OncoArray” and “HumanCore.” In the following, we used these platforms to represent five populations. After the quality control process, the five populations included a total of 5,533, 3,314, 5,354, 5,267, and 6,646 subjects, respectively. More details about the study design and data collection were described elsewhere (Chahal et al., 2016; Duffy et al., 2018).
The genomic datasets, imputation and quality control procedures were conducted separately in each population and were described with details in previous publications (Lindström et al., 2017; Duffy et al., 2018). Briefly, the participants from five sub-populations were genotyped at different times and by different genotyping platforms. The subjects in “Affymetrix” were genotyped by the Genome-wide Human SNP Array 6.0. The subjects in “Illumina” were genotyped by either Illumina HumanHap300 BeadChip, HumanHap550-Quad BeadChip, Human610-Quad BeadChip, or Human660W-Quad BeadChip. The subjects in “OmniExpress” were genotyped by Illumina HumanOmniExpress-12 BeadChip. The subjects in “OncoArray” were genotyped by Infinium OncoArray-550K BeadChip. The subjects in “HumanCore” were genotyped by Illumina HumanCoreExome-12v1-0 BeadChip.
Variants with low call rate (<95%) were removed. A pairwise identity-by-descent (IBD) analysis was conducted to identify duplicates. For individuals who may be genotyped for more than once using different genotyping platforms, one of the duplicated pair was excluded by the order of “Affymetrix,” “Illumina,” “OmniExpress,” “OncoArray,” and “HumanCore.” For individuals with different cohort IDs but a high genetic concordance rate, both of the pairs were removed. Genome imputation was further conducted in each population using the 1000 Genomes Project Phase 3 Integrated Release Version 5 as reference panels. Software ShapeIT (v2.r837) was used for genotype phasing, and the phased genotypes were further imputed to ∼ 47 million variants using Minimac3 (O’Connell et al., 2014; Das et al., 2016).
To evaluate the validity of fastSKAT, we used 18 SNPs identified in two previous GWAS as positive controls (Chahal et al., 2016; Sarin et al., 2020). In these previous GWASs, ten SNPs were identified involving 3 independent populations (i.e., “Affymetrix,” “Illumina,” and “OmniExpress”), and 8 SNPs were identified using all 5 populations. For comparison purpose, we first used fastSKAT to test the association between each of these SNPs and cSCC, and further conducted a Fisher’s combined probability test to evaluate the overall association across three or five populations consist with their analysis in the previous GWASs. For fair comparison, we calculated p-values by applying fastSKAT to the same NHS and HPFS populations used in previous publications. In particular, “Affymetrix,” “Illumina,” and “OmniExpress” were used in Chahal et al. (2016), while “Affymetrix,” “Illumina,” “OmniExpress,” “OncoArray,” and “HumanCore” were all used in Sarin et al. (2020). The p-values were compared to those of previous GWAS publications for consistency.
To identify biologically meaningful loci, we used UCSC Genome Browser (assembly GRCh37/hg19) to define gene units as candidate loci for region-based analysis. Software bedtools were used to merge the redundant and overlapping genomic regions based on the gene annotation (Kindlon ARQaN, 2009–2019; Quinlan and Hall, 2010). A candidate locus was then defined as 7.5KB upstream and downstream the corresponding gene region. Ultimately, a total of 25,437 regions were extracted. During the data processing, SNPs with an imputation quality score less than 0.3 were removed. We also extracted common and rare variants separately for each region using PLINK2.0 (Purcell et al., 2007; Purcell). Common and rare variants were defined based on whether the minor allele frequency (MAF) was larger than 5%. Because previous GWAS has comprehensively evaluated each single variant for association with cSCC, we only considered regions with two or more variants for region-based association analysis.
We evaluated the association between genomic regions and cSCC using the fastSKAT, a region-based association test that is computationally efficient for large-scale genomic datasets (Lumley et al., 2018). Similar to the SKAT method, it is a variance component score test that integrates the effect of multiple genetic variants within the same region (Wu et al., 2011). The improvement of computational speed over SKAT was achieved by accurately approximating the tail probability for the asymptotic distribution of the test statistics (Lumley et al., 2018). Instead of computing all the eigenvalues of the genotypic similarity matrix, only the top ones were computed through random projections (Halko et al., 2011; Tropp, 2011). The tail probability can then be approximated by the top eigenvalues and a reminder term computed using Satterthwaite approximation, which approximates the sum of weighted chi-square distributions with a single chi-square distribution. The fastSKAT has been implemented in R package “bigQF” (Lumley et al., 2018). For each gene region, the method was applied for rare variants (MAF < 5%) and common (MAF ≥ 5%) variants separately, and also for all variants together, adjusting for age, gender and the first five genetic principal components. A weighted linear kernel was used with each variant weighted by Beta(MAF,1,25), the beta distribution density function. After testing each region within each of the five sub-populations, we further adopted the Fisher’s combined probability test to integrate the p-values from sub-populations for an overall p-value.
The majority of variants identified by existing GWASs were located in the non-coding regions of the genome, and were therefore likely to be involved in gene regulation. One hypothesis is that that causal genetic variants for complex diseases may function through regulating the expression level of genes within specific tissues. To prioritize our findings, we further examined if the identified genes harbor any known expression quantitative trait locus (eQTL) in the database. We used the Genotype-Tissue Expression (GTEx) database (GTEx Consortium, 2013) for cross checking. There are two main types of skin tissues available in the GTEx, including sun-exposed skin at lower leg and sun-unexposed skin in suprapubic region. We summarized the number of eQTLs located within each identified region for either of skin tissue types.
Our study included a total of 1,710 cSCC cases and 24,304 controls, partitioned into five sub-populations based on genotyping platforms. The number of subjects and their characteristics by each population is summarized in Table 1. The case-control ratios ranged from 1:6 to 1:31 across five populations. Gender was statistically different between cases and controls in four populations (p < 0.05), which was consist with the fact that the incidence rate was higher in men than in women (Karagas et al., 1999; Nguyen et al., 2014). Age, a well-established risk factor, was associated with cSCC in all populations (p < 0.001).
For a total of 18 SNPs identified by previous GWASs, we used fastSKAT to test each variant for association with the disease outcome and compared the testing p-values with those reported in previous publications. The comparison is presented in Figure 1 and summarized in Table 2. We found that the Fisher’s p-values combining fastSKAT results of multiple populations were highly correlated with the reported p-values in previous publications. The Fisher’s combined p-values tend to be smaller, especially for variants with relatively small testing p-values (e.g., <0.01), leading to a higher level of statistical significance for the association. The results suggested that testing with fastSKAT in each population and combining with Fisher’s combined probability test was able to reliably identify the gene-disease association with improved statistical power.
Figure 1. Replication of 18 GWAS identified SNPs using fastSKAT. The p-values of fastSKAT were based on Fisher’s method combining its testing p-values from the same NHS and HPFS populations used in previous publications.
Approximately 23,000 candidate regions were extracted and tested in each population. The numbers differed slightly across populations and was listed in Table 3. For each candidate region, the rare variants, common variants and all variants were tested separately for association with cSCC outcome using fastSKAT. The distribution of testing p-values were examined against a uniform distribution via quantile-quantile plots (Supplementary Figures 1–3 for rare, common and all variants, respectively). The genomic inflation factors ranged between 0.974 and 1.07, suggesting well-controlled type I error rates. The Manhattan plots based on fastSKAT and Fisher’s method are provided in Figures 2–4.
Figure 2. The Manhattan plots by rare variants analysis in each population (A) Affymetrix. (B) Illumina. (C) OmniExpress. (D) OncoArray. (E) HumanCore. (F) Fisher.
Figure 3. The Manhattan plots by common variants analysis in each population (A) Affymetrix. (B) Illumina. (C) OmniExpress. (D) OncoArray. (E) HumanCore. (F) Fisher.
Figure 4. The Manhattan plots by all variants analysis in each population (A) Affymetrix. (B) Illumina. (C) OmniExpress. (D) OncoArray. (E) HumanCore. (F) Fisher.
A total of four genomic regions were identified by Fisher’s combined probability test at the Bonferroni adjusted significance level. The genomic regions and their testing p-values are listed in Table 4. Four regions were identified via rare variants association, and one of them was also identified via all variants analysis. No regions reached statistical significance after Bonferroni adjustment via common variants analysis. While the overall significant association was largely driven by one particular population for most of these regions, the association for one region was replicated by one additional population in the study. In particular, a region (gene SLFN12) was located on chromosome 17, BP: 33,737,940–33,760,195. The rare variant association test achieved statistical significance after Bonferroni correction (p = 2.40 × 10–8). The association was highly significant in “OncoArray” population (p = 5.05 × 10–9) and was replicated in “HumanCore” population (p = 3.73 × 10–3).
We further looked into the significant findings within each population. In Table 5, we summarized the regions that were identified in a particular population by both rare variants and all variants association test. In Table 6, we summarized the regions that were identified by rare variants association test only. The p-values computed in five populations for these regions were summarized in Supplementary Tables 1, 2. In particular, the results suggested that multiple gene regions on chromosome 12 and chromosomes 17 were identified for association with the disease outcome. For example, two regions close to each other on chromosome 17 (gene LOC101928000, BP: 5,015,229–5,017,677 and gene USP6, BP: 5,019,732–5,078,326) were identified for both rare and all variants association. A different region on chromosome 17 was identified for rare variants association. While the underlying genetic mechanism and causal SNPs were not clear, we think the rare variants association test may provide findings that are complementary to existing GWAS that usually are limited to relatively common variants. For common variants analysis, we were not able to identify any regions after Bonferroni adjustment. In Table 7, we summarized regions with suggestive significance (i.e., 10–5) in a particular population. In particular, the association for region SPATA2L was marginally significant in “OmniExpress” and was also nominally significant in both “Illumina” and “OncoArray.”
Table 5. Regions identified by both rare and all variants analysis in a particular population after Bonferroni adjustment.
Table 6. Regions identified by rare variants analysis in a particular population after Bonferroni adjustment.
To provide additional insights on the possible involvement of these identified regions in regulating gene expression, we summarized the number of known eQTLs within each region (Table 8). Most of those loci (15 out of 18) included at least one eQTL either in not-sun-exposed or sun-exposed skin tissues. Among 24,279 regions being tested, a total of 16,534 contained at least one eQTL in the GTEx database. To evaluate the overrepresentation of eQTL in the identified region, we calculated an exact p-value using a hyper-genomic distribution as:
Table 8. Number of eQTLs located within identified regions in skin tissues exposed or not exposed to sun.
It is also worthwhile to note that most of existing studies of eQTL were also based on single-locus association test between each genetic variants and gene expression data. Though the p-value was not statistically significant at 0.05 level, the large proportion of identified regions harboring known eQTL suggested their possible involvement of gene expression within skin tissues.
In this study, we identified 18 cSCC-associated genomic regions using gene-based fastSKAT method. One region (i.e., SLFN12) was statistically significant in one population and replicated in another population. The eQTL analysis further supported the possible biological contribution of those regions to the genetic susceptibility of cSCC. The replication of previous GWAS-identified SNPs also demonstrated the reliability of fastSKAT in identifying susceptibility loci with improved statistical power. To our knowledge, our study is among the first ones to investigate the region-based association for cSCC on a genome-wide level.
As an effective and powerful tool, GWAS has been commonly used to investigate the genetic architecture of complex diseases, including squamous cell carcinoma. The goal of our study is to provide a complementary strategy to address a few limitations of the GWAS, especially to evaluate the rare variants with low frequencies in the populations. In our study, although the total sample size was relatively large (∼26K), the number of cases were relatively small in each sub-population (<800). In such a situation, the single-locus-based GWAS is expected to be under-powered to identify rare variants (Tong et al., 2011; Mo et al., 2015). In addition, the highly unbalanced numbers of cases and controls may also present additional challenge to both conventional GWAS and rare-variants association tests. Recent studies have suggested that the number of cases and case to control ratio may both have an impact on the statistical power and type I errors, especially under large control group scenarios (Zhang et al., 2019). It was also found that SKAT can reach reasonably high power with well-controlled type I error if the number of cases is larger than 200. In our study, the number of cases ranged between ∼200 and 700 across five subpopulations, and the results appeared to be consistent with previous studies. The QQ-plot and estimated genomic inflation factors suggested well-controlled type I errors. While we expect the statistical power will improve with additional cases, the current results also suggested that region-based association test was able to identify genomic regions though rare variants association.
A number of gene units were identified to harbor genetic variants that may contribute to the susceptibility of cSCC. One gene was identified with replicated association in two sub-populations. Gene SLFN12, or Schlafen family member 12, belongs to a group of genes mediating growth-inhibition as cell cycle regulators (Katsoulidis et al., 2010). Many studies have found that SLFN12 played a key role in generating anti-tumor effects triggered by certain drugs or interventions (Katsoulidis et al., 2010; An et al., 2019; Lewis et al., 2019). For example, the drug Anagrelide (ANA) can only inhibits cancer cell growth when both PED3A and SLFN12 are expressed.
A number of other gene units were identified to be associated with cSCC in one population without replication. However, they have been reported in the literature for involvement with cancer development. For example, the identified gene units HP1BP1 and SEPT7P2 have been found to be involved in cancer growth and progression (Dutta et al., 2014; Wang et al., 2019). In addition, gene SPATA2L have been identified to be associated with vitiligo in a recent study (Cai et al., 2021), and the inverse relationship between vitiligo and NMSC was suggested in many research (Paradisi et al., 2014; Rodrigues, 2017; Wu et al., 2018; Wen et al., 2020).
A number of other methods were also available for region-based association test. For example, we and others have proposed a generalized genetic random field (GGRF) method for testing the association between a set of variants and a disease phenotype (Li et al., 2014). The proposed GGRF is a similarity-based method. It maps subjects to a Euclidean space using on their genotypes as coordinates, so that subjects who are close to each other in space would have similar phenotype if there is a gene-phenotype association (Li et al., 2014). GGRF used a Wald-type of test statistic and may achieve improved power over SKAT under various disease scenario. However, fastSKAT used a score test and is more computationally efficient with the approximation by random projection. In this study, we have used fastSKAT for analysis and we showed in Appendix, GGRF would be equivalent to SKAT if a generalized score test is used.
Our study must be considered in the light of certain limitations. First, none of the association was consistently replicated in all populations. This is partly due to the heterogeneous nature of rare variants and their low allele frequencies across populations. Multiple rare mutations within the same gene can independently influence the disease (i.e., allelic heterogeneity), and rare variants in different genes can also be involved in related pathways underlying complex human diseases (i.e., locus heterogeneity) (McClellan and King, 2010). Second, due to the nature of gene-based analysis, it is not straightforward to ascertain the causal SNPs or estimate their effect on cSCC risk. We also have not considered intergenic variants that were not within the gene regions (Mo et al., 2015). Third, the existing findings based on region-based association have been limited. For example, the eQTL variants available in GTEx database were mainly identified via single-locus analysis. Additional functional analysis is needed to validate the identified regions in the future. Forth, we are also aware that the results are subject to the strengths and limitations of fastSKAT due to its assumptions and implementation. For example, we have used a weight function that is inversely correlated with the MAF of each variant (i.e., probability density of beta distribution, default option of fastSKAT). It is often helpful to incorporate functional annotation of the variants to upweight those with potentially stronger effect on the disease (Kumar et al., 2009; Lee et al., 2015; Quick et al., 2019). Further, extensions of SKAT, such as SKAT-O, were able to effectively combine the test statistics of SKAT and burden test (Lee et al., 2012), which may have improved power when the causal variants have the same direction of effects. We have adopted fastSKAT mainly because of the computational advantage for studies with a very large number of subjects and variants. It can also be helpful to improve the power in other scenarios when SKAT-O becomes feasible for extremely large studies. Fifth, no genomic region was identified by common variants analysis after Bonferroni adjustment. It is partly because the weight function adopted gave more weight to variants with low MAF and regions with common variants receiving less weight may not be able to identify. Furthermore, region-based test would be less powerful when there are a few susceptible loci with effects in this region and the total number of tested SNPs is large.
The data analyzed in this study is subject to the following licenses/restrictions: GWAS data has not been publicly available. Further information including the procedures to obtain and access data from the Nurses’ Health Studies and Health Professionals Follow-up Study is described at https://www.nurseshealthstudy.org/researchers (contact email: bmhzYWNjZXNzQGNoYW5uaW5nLmhhcnZhcmQuZWR1) and https://sites.sph.harvard.edu/hpfs/for-collaborators/. The expression quantitative trait loci (eQTL) database are openly available from the Genotype-Tissue Expression (GTEx) project at https://www.gtexportal.org/home/.
The studies involving human participants were reviewed and approved by the institutional review boards of the Brigham and Women’s Hospital and Harvard T.H. Chan School of Public Health, and those of participating registries as required. The patients/participants provided their written informed consent to participate in this study.
MH and ML conceived and designed the analysis. JH and AQ collected the data. MH, CL, XL, AQ, JH, and ML contributed data and analysis tools and wrote the manuscript. MH, CL, and ML performed the analysis. All authors have read and approved the manuscript.
This study was supported, in part, by the National Heart, Lung and Blood Institute under award number K01HL140333 (ML), the Eunice Kennedy Shriver National Institute of Child Health and Human Development under award number R03HD092854 (ML), and the National Cancer Institute under award number UM1CA186107, P01CA87969, R01CA49449, U01CA176726, R01CA67262, and U01CA167552. The funders had no role in the design of the study and collection, analysis, and interpretation of data and in writing the manuscript. The contents are solely the responsibility of the authors and do not necessarily represent the official views of the National Institute of Health.
The authors declare that the research was conducted in the absence of any commercial or financial relationships that could be construed as a potential conflict of interest.
We would like to thank the participants and staff of the NHS, the NHS II and the HPFS, for their valuable contributions, as well as the following state cancer registries for their help: AL, AZ, AR, CA, CO, CT, DE, FL, GA, ID, IL, IN, IA, KY, LA, ME, MD, MA, MI, NE, NH, NJ, NY, NC, ND, OH, OK, OR, PA, RI, SC, TN, TX, VA, WA, WY. We also want to thank Channing Division of Network Medicine, Department of Medicine, Brigham and Women’s Hospital and Harvard Medical School, Boston, MA, United States for data sharing. We assume full responsibility for analyses and interpretation of these data.
The Supplementary Material for this article can be found online at: https://www.frontiersin.org/articles/10.3389/fgene.2021.657499/full#supplementary-material
An, R., Liu, J., He, J., Wang, F., Zhang, Q., and Yu, Q. (2019). PDE3A inhibitor anagrelide activates death signaling pathway genes and synergizes with cell death-inducing cytokines to selectively inhibit cancer cell growth. Am. J. Cancer Res. 9, 1905–1921.
Asgari, M. M., Wang, W., Ioannidis, N. M., Itnyre, J., Hoffmann, T., Jorgenson, E., et al. (2016). Identification of susceptibility loci for cutaneous squamous cell carcinoma. J. Investig. Dermatol. 136, 930–937. doi: 10.1016/j.jid.2016.01.013
Asgari, M. M., Warton, E. M., and Whittemore, A. S. (2015). Family history of skin cancer is associated with increased risk of cutaneous squamous cell carcinoma. Dermatol. Surg. 41, 481–486. doi: 10.1097/dss.0000000000000292
Cai, M., Yuan, T., Huang, H., Gui, L., Zhang, L., Meng, Z., et al. (2021). Integrative analysis of omics data reveals regulatory network of CDK10 in vitiligo risk. Front. Genet. 12:634553. doi: 10.3389/fgene.2021.634553
Chahal, H. S., Lin, Y., Ransohoff, K. J., Hinds, D. A., Wu, W., Dai, H. J., et al. (2016). Genome-wide association study identifies novel susceptibility loci for cutaneous squamous cell carcinoma. Nat. Commun. 7:12048. doi: 10.1038/ncomms12048
Chang, C. C., Chow, C. C., Tellier, L. C., Vattikuti, S., Purcell, S. M., and Lee, J. J. (2015). Second-generation PLINK: rising to the challenge of larger and richer datasets. Gigascience 4:7. doi: 10.1186/s13742-015-0047-8
Chitsazzadeh, V., Coarfa, C., Drummond, J. A., Nguyen, T., Joseph, A., Chilukuri, S., et al. (2016). Cross-species identification of genomic drivers of squamous cell carcinoma development across preneoplastic intermediates. Nat. Commun. 7:12601. doi: 10.1038/ncomms12601
Das, S., Forer, L., Schönherr, S., Sidore, C., Locke, A. E., Kwong, A., et al. (2016). Next-generation genotype imputation service and methods. Nat. Genet. 48, 1284–1287. doi: 10.1038/ng.3656
Duffy, D. L., Zhu, G., Li, X., Sanna, M., Iles, M. M., Jacobs, L. C., et al. (2018). Novel pleiotropic risk loci for melanoma and nevus density implicate multiple biological pathways. Nat. Commun. 9:4774. doi: 10.1038/s41467-018-06649-5
Dutta, B., Yan, R., Lim, S. K., Tam, J. P., and Sze, S. K. (2014). Quantitative profiling of chromatome dynamics reveals a novel role for HP1BP3 in hypoxia-induced oncogenesis. Mol. Cell. Proteom. 13, 3236–3249. doi: 10.1074/mcp.M114.038232
GTEx Consortium (2013). The genotype-tissue expression (GTEx) project. Nat. Genet. 45, 580–585. doi: 10.1038/ng.2653
Halko, N., Martinsson, P. G., and Tropp, J. A. (2011). Finding structure with randomness: probabilistic algorithms for constructing approximate matrix decompositions. SIAM Rev. 53, 217–288. doi: 10.1137/090771806
Hussain, S. K., Sundquist, J., and Hemminki, K. (2009). The effect of having an affected parent or sibling on invasive and in situ skin cancer risk in Sweden. J. Investig. Dermatol. 129, 2142–2147. doi: 10.1038/jid.2009.31
Karagas, M. R., Greenberg, E. R., Spencer, S. K., Stukel, T. A., and Mott, L. A. (1999). Increase in incidence rates of basal cell and squamous cell skin cancer in New Hampshire, USA. New Hampshire skin cancer study group. Int. J. Cancer 81, 555–559. doi: 10.1002/(sici)1097-0215(19990517)81:4<555::aid-ijc9<3.0.co;2-r
Katsoulidis, E., Mavrommatis, E., Woodard, J., Shields, M. A., Sassano, A., Carayol, N., et al. (2010). Role of interferon {alpha} (IFN{alpha})-inducible Schlafen-5 in regulation of anchorage-independent growth and invasion of malignant melanoma cells. J. Biol. Chem. 285, 40333–40341. doi: 10.1074/jbc.M110.151076
Kumar, P., Henikoff, S., and Ng, P. C. (2009). Predicting the effects of coding non-synonymous variants on protein function using the SIFT algorithm. Nat. Protoc. 4, 1073–1081. doi: 10.1038/nprot.2009.86
Lee, D., Gorkin, D. U., Baker, M., Strober, B. J., Asoni, A. L., McCallion, A. S., et al. (2015). A method to predict the impact of regulatory variants from DNA sequence. Nat. Genet. 47, 955–961. doi: 10.1038/ng.3331
Lee, S., Emond, M. J., Bamshad, M. J., Barnes, K. C., Rieder, M. J., Nickerson, D. A., et al. (2012). Optimal unified approach for rare-variant association testing with application to small-sample case-control whole-exome sequencing studies. Am. J. Hum. Genet. 91, 224–237. doi: 10.1016/j.ajhg.2012.06.007
Lewis, T. A., de Waal, L., Wu, X., Youngsaye, W., Wengner, A., Kopitz, C., et al. (2019). Optimization of PDE3A modulators for SLFN12-dependent cancer cell killing. ACS Med. Chem. Lett. 10, 1537–1542. doi: 10.1021/acsmedchemlett.9b00360
Li, M., He, Z., Zhang, M., Zhan, X., Wei, C., Elston, R. C., et al. (2014). A generalized genetic random field method for the genetic association analysis of sequencing data. Genet. Epidemiol. 38, 242–253. doi: 10.1002/gepi.21790
Liang, K.-Y., and Zeger, S. L. (1989). Longitudinal data analysis using generalized linear models. Biometrika 73, 13–22.
Lindström, S., Loomis, S., Turman, C., Huang, H., Huang, J., Aschard, H., et al. (2017). A comprehensive survey of genetic variation in 20,691 subjects from four large cohorts. PLoS One 12:e0173997. doi: 10.1371/journal.pone.0173997
Lumley, T., Brody, J., Peloso, G., Morrison, A., and Rice, K. (2018). FastSKAT: sequence kernel association tests for very large sets of markers. Genet. Epidemiol. 42, 516–527. doi: 10.1002/gepi.22136
McClellan, J., and King, M. C. (2010). Genetic heterogeneity in human disease. Cell 141, 210–217. doi: 10.1016/j.cell.2010.03.032
Mo, X. B., Lu, X., Zhang, Y. H., Zhang, Z. L., Deng, F. Y., and Lei, S. F. (2015). Gene-based association analysis identified novel genes associated with bone mineral density. PLoS One 10:e0121811. doi: 10.1371/journal.pone.0121811
Motaparthi, K., Kapil, J. P., and Velazquez, E. F. (2017). Cutaneous squamous cell carcinoma: review of the eighth edition of the American joint committee on cancer staging guidelines, prognostic factors, and histopathologic variants. Adv. Anat. Pathol. 24, 171–194. doi: 10.1097/pap.0000000000000157
Muzic, J. G., Schmitt, A. R., Wright, A. C., Alniemi, D. T., Zubair, A. S., Olazagasti Lourido, J. M., et al. (2017). Incidence and trends of basal cell carcinoma and cutaneous squamous cell carcinoma: a population-based study in Olmsted County, Minnesota, 2000 to 2010. Mayo Clin. Proc. 92, 890–898. doi: 10.1016/j.mayocp.2017.02.015
Nan, H., Xu, M., Kraft, P., Qureshi, A. A., Chen, C., Guo, Q., et al. (2011). Genome-wide association study identifies novel alleles associated with risk of cutaneous basal cell carcinoma and squamous cell carcinoma. Hum. Mol. Genet. 20, 3718–3724. doi: 10.1093/hmg/ddr287
Nettiksimmons, J., Tranah, G., Evans, D. S., Yokoyama, J. S., and Yaffe, K. (2016). Gene-based aggregate SNP associations between candidate AD genes and cognitive decline. Age (Dordrecht, Netherlands) 38:41. doi: 10.1007/s11357-016-9885-2
Nguyen, K. D., Han, J., Li, T., and Qureshi, A. A. (2014). Invasive cutaneous squamous cell carcinoma incidence in US health care workers. Arch. Dermatol. Res. 306, 555–560. doi: 10.1007/s00403-014-1469-3
O’Connell, J., Gurdasani, D., Delaneau, O., Pirastu, N., Ulivi, S., Cocca, M., et al. (2014). A general approach for haplotype phasing across the full spectrum of relatedness. PLoS Genet. 10:e1004234. doi: 10.1371/journal.pgen.1004234
Paradisi, A., Tabolli, S., Didona, B., Sobrino, L., Russo, N., and Abeni, D. (2014). Markedly reduced incidence of melanoma and nonmelanoma skin cancer in a nonconcurrent cohort of 10,040 patients with vitiligo. J. Am. Acad. Dermatol. 71, 1110–1116. doi: 10.1016/j.jaad.2014.07.050
Parekh, V., and Seykora, J. T. (2017). Cutaneous squamous cell carcinoma. Clin. Lab. Med. 37, 503–525. doi: 10.1016/j.cll.2017.06.003
Purcell, S., Neale, B., Todd-Brown, K., Thomas, L., Ferreira, M. A., Bender, D., et al. (2007). PLINK: a tool set for whole-genome association and population-based linkage analyses. Am. J. Human Genet. 81, 559–575. doi: 10.1086/519795
Que, S. K. T., Zwald, F. O., and Schmults, C. D. (2018b). Cutaneous squamous cell carcinoma: management of advanced and high-stage tumors. J. Am. Acad. Dermatol. 78, 249–261. doi: 10.1016/j.jaad.2017.08.058
Que, S. K. T., Zwald, F. O., and Schmults, C. D. (2018a). Cutaneous squamous cell carcinoma: incidence, risk factors, diagnosis, and staging. J. Am. Acad. Dermatol. 78, 237–247. doi: 10.1016/j.jaad.2017.08.059
Quick, C., Wen, X., Abecasis, G., Boehnke, M., and Kang, H. M. (2019). Integrating comprehensive functional annotations to boost power and accuracy in gene-based association analysis. bioRxiv [Preprint]. doi: 10.1101/732404 732404,
Quinlan, A. R., and Hall, I. M. (2010). BEDTools: a flexible suite of utilities for comparing genomic features. Bioinformatics (Oxf. Engl.) 26, 841–842. doi: 10.1093/bioinformatics/btq033
Rodrigues, M. (2017). Skin cancer risk (Nonmelanoma skin cancers/Melanoma) in vitiligo patients. Dermatol. Clin. 35, 129–134. doi: 10.1016/j.det.2016.11.003
Sarin, K. Y., Lin, Y., Daneshjou, R., Ziyatdinov, A., Thorleifsson, G., Rubin, A., et al. (2020). Genome-wide meta-analysis identifies eight new susceptibility loci for cutaneous squamous cell carcinoma. Nat. Commun. 11:820. doi: 10.1038/s41467-020-14594-5
Siiskonen, S. J., Zhang, M., Li, W. Q., Liang, L., Kraft, P., Nijsten, T., et al. (2016). A genome-wide association study of cutaneous squamous cell carcinoma among European descendants. Cancer Epidemiol. Biomark. Prevent. 25, 714–720. doi: 10.1158/1055-9965
Tong, L., Tayo, B., Yang, J., and Cooper, R. S. (2011). Comparison of SNP-based and gene-based association studies in detecting rare variants using unrelated individuals. BMC Proc. 5 Suppl. 9(Suppl. 9):S41. doi: 10.1186/1753-6561-5-s9-s41
Tropp, J. A. (2011). Improved analysis of the subsampled randomized hadamard transform. Adv. Adapt. Data Anal. 03, 115–116.
Waldman, A., and Schmults, C. (2019). Cutaneous squamous cell carcinoma. Hematol. Oncol. Clin. North Am. 33, 1–12. doi: 10.1016/j.hoc.2018.08.001
Wang, J., Xie, G. F., He, Y., Deng, L., Long, Y. K., Yang, X. H., et al. (2019). Interfering expression of chimeric transcript SEPT7P2-PSPH promotes cell proliferation in patients with nasopharyngeal carcinoma. J. Oncol. 2019, 1654724. doi: 10.1155/2019/1654724
Wen, Y., Wu, X., Peng, H., Li, C., Jiang, Y., Liang, H., et al. (2020). Cancer risks in patients with vitiligo: a Mendelian randomization study. J Cancer Res. Clin. Oncol. 146, 1933–1940. doi: 10.1007/s00432-020-03245-3
Wu, M. C., Kraft, P., Epstein, M. P., Taylor, D. M., Chanock, S. J., Hunter, D. J., et al. (2010). Powerful SNP-set analysis for case-control genome-wide association studies. Am. J. Hum. Genet. 86, 929–942. doi: 10.1016/j.ajhg.2010.05.002
Wu, M. C., Lee, S., Cai, T., Li, Y., Boehnke, M., and Lin, X. (2011). Rare-variant association testing for sequencing data with the sequence kernel association test. Am. J. Hum. Genet. 89, 82–93. doi: 10.1016/j.ajhg.2011.05.029
Wu, W., Amos, C. I., Lee, J. E., Wei, Q., Sarin, K. Y., and Han, J. (2018). Inverse relationship between vitiligo-related genes and skin cancer risk. J. Investig. Dermatol. 138, 2072–2075. doi: 10.1016/j.jid.2018.03.1511
Zhang, X., Basile, A. O., Pendergrass, S. A., and Ritchie, M. D. (2019). Real world scenarios in rare variant association analysis: the impact of imbalance and sample size on the power in silico. BMC Bioinformatics 20:46. doi: 10.1186/s12859-018-2591-6
In our study, a fastSKAT method was applied to test the association between each genomic region and disease outcome. A number of other methods were also available for region-based association test. For example, we and others have proposed a generalized genetic random field (GGRF) method for testing the association between a set of variants and a disease phenotype (Li et al., 2014), and compared its performance to that of SKAT. We described below that GGRF would have similar test statistic with SKAT if a generalized score test is used for inference.
Suppose the study include a total of N subjects, each with K variants in a region and M covariates. Let Y, G, X denotes the phenotype (N = 1), genotype (N = K), and covariates (N = M) matrix, respectively. The GGRF adopts a conditional autoregression model as:
Where the i-th element of Y− denotes the phenotype of all other subjects other than i-th subject, μ = f(Xβ) is used for covariants adjustment, and S is a matrix for pairwise genetic similarity among N subjects. To test the genotype-phenotype association (H0:γ = 0), a generalized score test can be used (Liang and Zeger, 1989), so that:
A generalized score statistic can thus be defined as (Boos, 1992)
where is estimated under the null hypothesis that γ = 0 via a generalized linear model. The score statistic takes the same format with that of SKAT, and follows asymptotically a mixture of Chi-square distributions (Wu et al., 2011).
Keywords: region-based association test, fast sequence kernel association test, cutaneous squamous cell carcinoma, rare variants, generalized genetic random field
Citation: Huang M, Lyu C, Li X, Qureshi AA, Han J and Li M (2021) Identifying Susceptibility Loci for Cutaneous Squamous Cell Carcinoma Using a Fast Sequence Kernel Association Test. Front. Genet. 12:657499. doi: 10.3389/fgene.2021.657499
Received: 23 January 2021; Accepted: 09 April 2021;
Published: 10 May 2021.
Edited by:
Qi Yan, Columbia University, United StatesCopyright © 2021 Huang, Lyu, Li, Qureshi, Han and Li. This is an open-access article distributed under the terms of the Creative Commons Attribution License (CC BY). The use, distribution or reproduction in other forums is permitted, provided the original author(s) and the copyright owner(s) are credited and that the original publication in this journal is cited, in accordance with accepted academic practice. No use, distribution or reproduction is permitted which does not comply with these terms.
*Correspondence: Ming Li, bGk0OThAaW5kaWFuYS5lZHU=
Disclaimer: All claims expressed in this article are solely those of the authors and do not necessarily represent those of their affiliated organizations, or those of the publisher, the editors and the reviewers. Any product that may be evaluated in this article or claim that may be made by its manufacturer is not guaranteed or endorsed by the publisher.
Research integrity at Frontiers
Learn more about the work of our research integrity team to safeguard the quality of each article we publish.