- 1College of Animal Science and National Engineering Research Center for Breeding Swine Industry, South China Agricultural University, Guangzhou, China
- 2National Engineering Research Center for Breeding Swine Industry, Wens Foodstuff Group Co., Ltd., Yunfu, China
Body length, body height, and total teat number are economically important traits in pig breeding, as these traits are usually associated with the growth, reproductivity, and longevity potential of piglets. Here, we report a genetic analysis of these traits using a population comprising 2,068 Large White pigs. A genotyping-by-sequencing (GBS) approach was used to provide high-density genome-wide SNP discovery and genotyping. Univariate and bivariate animal models were used to estimate heritability and genetic correlations. The results showed that heritability estimates for body length, body height, and total teat number were 0.25 ± 0.04, 0.11 ± 0.03, and 0.22 ± 0.04, respectively. The genetic correlation between body length and body height exhibited a strongly positive correlation (0.63 ± 0.15), while a positive but low genetic correlation was observed between total teat number and body length. Furthermore, we used two different genome-wide association study (GWAS) approaches: single-locus GWAS and weighted single-step GWAS (WssGWAS), to identify candidate genes for these traits. Single-locus GWAS detected 76, 13, and 29 significant single-nucleotide polymorphisms (SNPs) associated with body length, body height, and total teat number. Notably, the most significant SNP (S17_15781294), which is located 20 kb downstream of the BMP2 gene, explained 9.09% of the genetic variance for body length traits, and it also explained 9.57% of the genetic variance for body height traits. In addition, another significant SNP (S7_97595973), which is located in the ABCD4 gene, explained 8.92% of the genetic variance for total teat number traits. GWAS results for these traits identified some candidate genomic regions, such as SSC6: 14.96–15.02 Mb, SSC7: 97.18–98.18 Mb, SSC14: 128.29–131.15 Mb, SSC17: 15.39–17.27 Mb, and SSC17: 22.04–24.15 Mb, providing a starting point for further inheritance research. Most quantitative trait loci were detected by single-locus GWAS and WssGWAS. These findings reveal the complexity of the genetic mechanism of the three traits and provide guidance for subsequent genetic improvement through genome selection.
Introduction
External characteristics such as body length, body height, and total teat number are very important in the pig industry. Body length and body height are not directly considered as pig breeding target traits. However, body length and other morphological characteristics often affect the value of the animal when purchasing a sow. Besides, the growth potential of pigs has a negative effect on the longevity of sows (Hong et al., 2019). A large body length, flat rib shape, narrow body width, and upright leg posture might be adverse features for sow longevity (Nikkila et al., 2013). An increase in the number of offspring leads to the need for more nipples; therefore, selecting the total teat number is an effective strategy to improve the reproductive performance of sows (Rohrer and Nonneman, 2017). Genome-wide association studies (GWAS) have been widely used to analyze the genetic architecture of reproduction, meat quality, and production traits in the pig population (Verardo et al., 2016; Schmid et al., 2018; Ding et al., 2019). Previous GWAS found that several single nucleotide polymorphisms (SNPs) in or near the bone morphogenetic protein 2 (BMP2) gene were associated with body length and body height, and many reported that the vertebrae development-associated (VRTN) gene was significantly associated with total teat number in pigs (Lee et al., 2014, 2019; Arakawa et al., 2015; Wang et al., 2017). Almost all GWAS for body length, body height, and total teat number were performed by single-locus GWAS. Weighted single-step GWAS (WssGWAS) is widely used in association analysis to increase the number of genotyped animals in livestock (Suwannasing et al., 2018; Lee et al., 2019; Fu et al., 2020). WssGWAS is a promising method allowing utilization of phenotypes from ungenotyped animals based on pedigree information, so we compared the result from two methods on body length, body height, and total teat number in pigs. On one hand, we can obtain more candidate genes. On the other hand, we can verify the reliability of GWAS result based on two methods.
High-density genetic markers can identify linkage disequilibrium (LD) in large populations (Marchini et al., 2007). In many scenarios, although there is a downtrend in sequencing cost, it is still expensive to use whole-genome sequencing in agricultural breeding. By simplifying genome sequencing, the cost of genotyping animals can be greatly reduced, further enabling the application of genome-wide selection (Wen et al., 2017). Imputation-based strategies are key to simplifying genome sequencing, because it increases the density of genetic markers (Howie et al., 2012). The accuracy of imputation influences the results of the analysis.
As one of the most commonly used pig breeds, Large White pigs are often used as the standard in modern commercial settings. This breed has the characteristics of large litters, heavy milk production, and strong maternal instincts. It can be used in a crossbreeding program in different ways, such as terminal sire on rare breeds, maternal sire in Landrace breeds, and commercial sire. Large White pigs are meek and less fat, which is in line with market demand1.
In this study, we used genotyping-by-sequencing (GBS) technology to genotype Large White pigs and analyzed the genetic correlation between body length, body height, and total teat number. We combined single-locus and weighted single-step GWAS for these traits in the Large White population.
Materials and Methods
Ethics Statement
All procedures related to animals in this study were performed strictly in line with the guidelines for the care and use of experimental animals established by the Ministry of Science and Technology of the People’s Republic of China (Approval Number: 2006-398). All the scheme design experiments using animals were approved by the ethics committee of South China Agricultural University (SCAU, Guangzhou, China). The experimental animals were not anesthetized or euthanized for the purpose of this study.
Sample Collection and Phenotyping
The Large White sows used for this study were born between January 2012 and November 2016. All 2,068 sows were subjected to the same growth and feeding conditions and raised in a single nuclear farm in Wen’s Foodstuff Group (Guangdong, China). The following phenotypic data were used in this study: body length, body height, and total teat number. Body length was measured from the base of the pig’s ears to the base of its tail when the weight of the pigs reached 100 ± 5 kg. Body height was measured from the shoulders to the ground when the pig’s weight reached 100 ± 5 kg. Tapes were used to measure body length and height. The total teat number in this study was the sum of normal left and right teats and was recorded within 48 h after birth.
DNA Extraction, Genotyping, and Quality Control
Genomic DNA was extracted from the ear tissue using the QIAamp DNA Mini kit (QIAGEN, Hilden, Germany) and checked using agarose gel electrophoresis. The genomic DNA concentration was determined by a NanoDrop 2000 spectrophotometer (Thermo Fisher Scientific, Waltham, MA, United States), and then diluted to 40 ng/μL in 96-well plates. Genome-wide SNP discovery and genotyping was performed using GBS technology. DNA samples (160 ng) were digested with two restriction endonucleases EcoRI and MspI, then ligated to the digested adapters. Following adapter ligation, samples were pooled in 96-plex and size-selected using two cycles of purification with Agencourt AMPure XP Beads (Beckman Coulter, Pasadena, CA, United States). The Agilent 2100 Bioanalyzer was used to assess the library inserts’ fragment size. The qualified libraries were sequenced on the Illumina Hiseq platform by 150-bp paired-end sequencing. Raw reads were pre-processed using Trimmomatic (version 0.33) to remove the adapter sequences and low quality reads. SNP genotyping were called according to the pipeline implemented in TASSEL 5.0 with default parameters (Bradbury et al., 2007), and Beagle 5.1 (Browning et al., 2018) was used to impute missing SNP genotypes. SNP quality control was conducted by Plink (version 1.07) with the parameters of Hardy-Weinberg equilibrium P > 10–6, minor allele frequency >0.01, individuals call rate >0.95, and SNPs call rate >0.99 (Purcell et al., 2007). Finally, a total of 195,895 SNP markers passed quality control in all 2,068 Large White pigs.
Principal Components Analysis and Linkage Disequilibrium Analysis
Principal component analysis (PCA) was performed using GCTA software (Yang et al., 2011). LD analysis was performed with PopLDdency software (Zhang et al., 2019). The average LD decay distance (r2 = 0.2) across the whole genome and each chromosome genome was analyzed.
Genetic Parameters Estimation
Genetic parameters were estimated using AIREMLK90 in BLUPF90 software packages (Legarra et al., 2014). A univariate animal model was used to calculate heritability, and a bivariate animal model was used to calculate genetic correlation. The models used were as follows: Y = Xb + Za + Wt + e, where Y is the vector of phenotypic records, b is the vector of fixed effects (including year-season), a is the vector of additive genetic variation, t is the vector of litter effect, e is the vector of residuals, and X, Z, and W are incidence matrices for b, a, and e, respectively.
Single-Locus GWAS
In the current study, association tests with univariate linear mixed models were performed using GEMMA software (Zhou and Stephens, 2012, 2014). The statistical model was as follows: Y = Zβ + Wa + u + e, where Y is the vector of phenotypic records, β is the vector of the regression coefficients, including the intercept, a is the effect size of the marker, u is the vector of random effects, e is the vector of random errors, Z is a matrix of covariates (fixed effect such as year-season), W is the vector of SNP genotypes (u∼MVNn(0,λτ–1K),e ∼MVNn(0,τ–1In), where λ is the ratio between the two variance components, K is a known relatedness matrix, In is the identity matrix, and MVNn denotes the dimensional multivariate normal distribution). Considering that the Bonferroni correction is a stringent criterion, we used Plink (version 1.07) to generate a refined subset of SNPs that were in approximate linkage equilibrium with each other. The genome-wide significant threshold was set as 0.05/N, and the chromosome-wide significant threshold was set as 1/N, where N is the number of the independent SNPs. To further confirm whether the signal in the candidate region was caused by the top SNP, conditional analysis was performed by adding the genotypes of these variants in the model as fixed effects.
WssGWAS
WssGWAS was performed using the programs implemented in the BLUPF90 family. Firstly, RENUMF90, which is a renumbering program, was run to create an input file for BLUPF90 programs and provide basic statistics. Then, we ran BLUPF90 with genomic information and saved A22–1 and G–1, where A22 is a numerator relationship matrix for genotyped animals, and G is a genomic relationship matrix. Finally, we ran POSTGSF90 to obtain the effect of SNP on the additive variance explained by segments. The results were presented in terms of the additive genetic variance contribution explained by each window of 50 SNPs.
Search for Genes and Functional Enrichment Analysis
In the single-locus GWAS method, we searched for candidate genes that were the nearest to the SNPs that reached a potentially significant level according to their physical position in the genome, as published on the EnsEMBL website2. In the GWAS method, several peaks explaining >0.5% of the direct additive genetic variance were discovered for these traits. Functional enrichment analysis was performed using the Gene Ontology, Kyoto Encyclopedia of Genes and Genomes, and UniProt databases. Furthermore, a functional enrichment analysis was performed using Metascape3 (Zhou et al., 2019), which is based on a Homo sapiens (human) database and using the default parameters.
Results
Summary of Phenotype, Genotype, and Genetic Parameter Statistics
The descriptive statistics of body length, body height, and total teat number for the Large White sows are described in Table 1. Briefly, the average value for body length, body height, and total teat numbers were 121.11 ± 3.55, 61.41 ± 2.57, and 13.88 ± 1.04, respectively. The heritability of body length, body height, and total teat number were 0.25 ± 0.04, 0.11 ± 0.03, and 0.22 ± 0.04, respectively. The genetic correlation and phenotypic correlation of body length, body height, and total teat number for the Large White sows were shown in Table 2. The phenotypic and genetic correlations between body length and body height were high and positive, which were 0.47 ± 0.02 and 0.63 ± 0.15, respectively. There was a positive but low genetic correlation between body length and total teat number. However, a very low genetic correlation was observed between total teat number and body height. Genetic correlation among these traits showed that selected body length traits also indirectly selected body height traits, and selected total teat number traits did not influence the body length and body height traits in pigs.
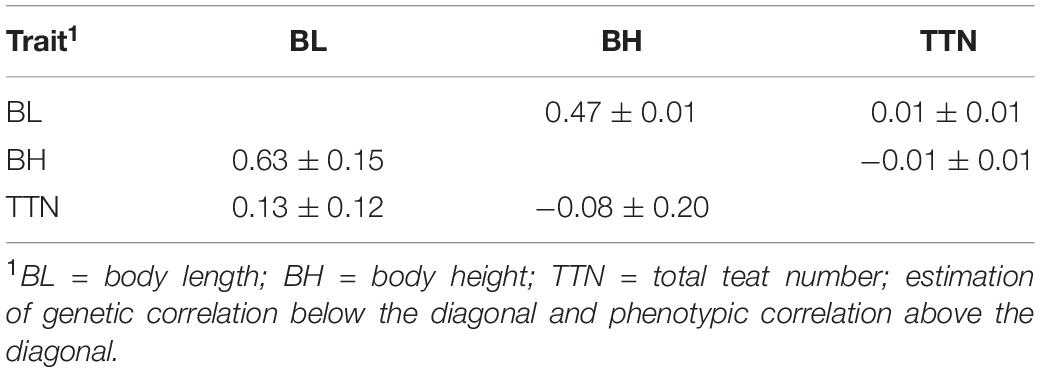
Table 2. Genetic correlation and phenotypic correlation of three traits in Large White pig population.
Genetic Architecture and LD Decay
The PCA plot is shown in Supplementary Figure 1B. The figure shows that our population did not have population stratification. The average LD decay distance was different among the chromosomes, and the fastest and the slowest LD decay occurred in SSC8 and 13, respectively. The average LD decay distance was approximately 300 kb, where the r2 values fell to 0.20 (Supplementary Figure 1A).
Single-Locus GWAS for Three Traits
The number of independent SNPs was 71,220, the genome-wide significant threshold and chromosome-wide significant threshold were equal to 7.03E-7 and 1.41E-5, respectively, Significant SNPs (P < 1.41E-5) were detected for body length, body height, and total teat number, and are shown in Supplementary Table 1 and Figure 1. A total of 40, 1, and 13 SNPs reached extremely significant levels in body length, body height, and total teat number traits (P = 7.03E-7). Besides, 76, 13, and 29 SNPs reached a suggestive significance level (P = 1.41E-5). In terms of body length traits, 27 SNPs on SSC7, 3 SNPs on SSC15, and 46 SNPs on SSC17 exceeded the chromosome-wide significance level (P = 1.41E-5). The lowest P-value of SNPs was 3.89E-21, located on SSC17. In the body height traits, there were 4, 6, and 3 SNPs that exceeded the chromosome-wide significance level (P = 1.41E-5) on SSC8, SSC15, and SSC17, respectively. In the total teat number, 28 SNPs were located on SSC7, and 1 SNP located on SSC15 exceeded the threshold (P = 1.41E-5). The SNP with the lowest P-value (P = 1.10E-10) was S7_97595973 on SSC7. Significantly, a total of 24 SNPs that exceeded the chromosome-wide significance level (P = 1.41E-5) were all exhibited in the traits of body length and total teat number.
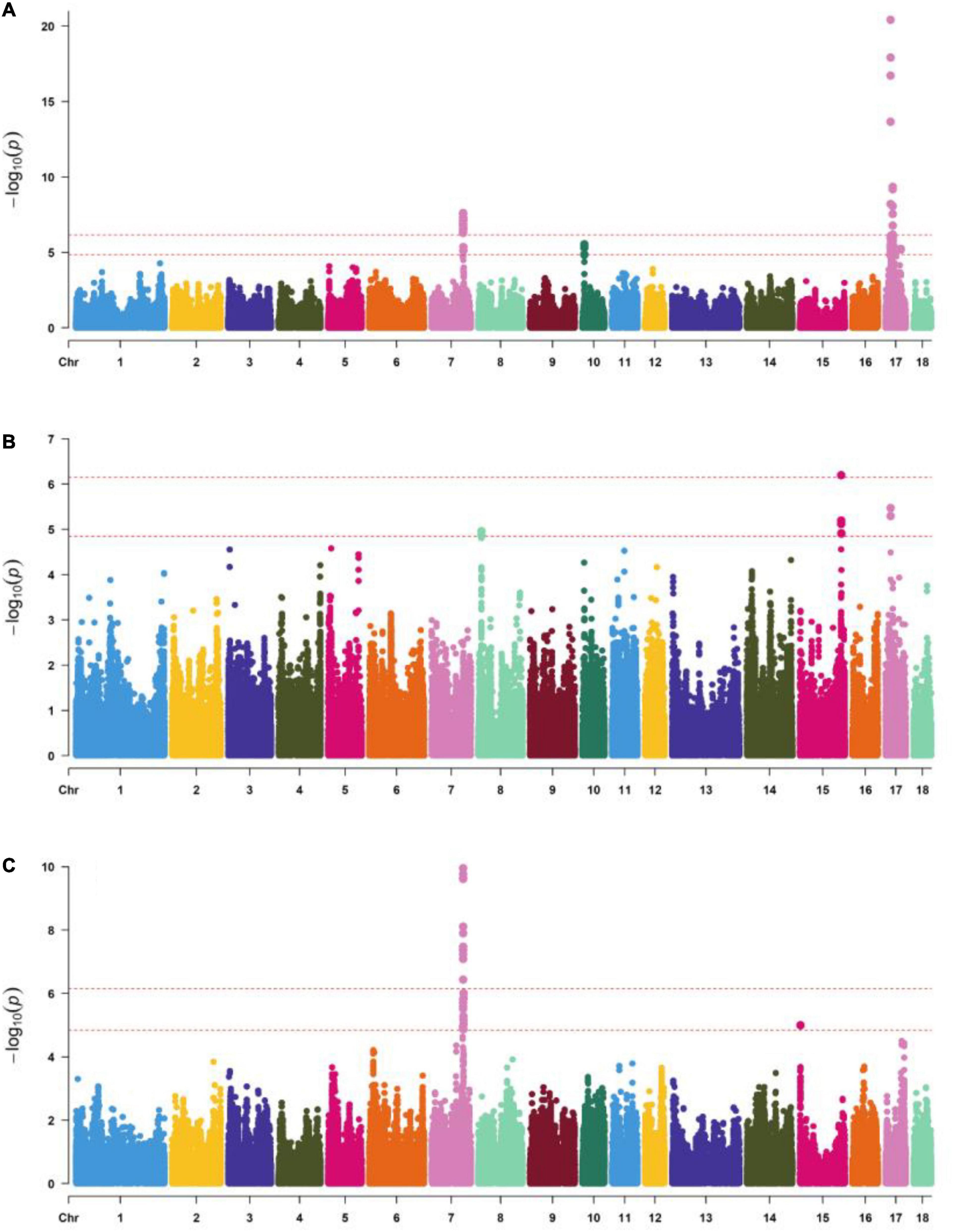
Figure 1. Manhattan plots of genome-wide SNP significance for (A) body length, (B) body height, and (C) total number teats. The X axis shows SNPs across chromosomes SSC1 to SSC18, and the Y axis represents the –log10(P). The top line indicates the genome-wide significant thresholds (P < 7.03E-7) and the bottom line indicates the suggestive genome-wide significant thresholds (P < 1.41E-5).
WssGWAS for Three Traits
The results of WssGWAS for body length, body height, and total teat number were grouped by the proportion of genetic variance, explained by windows of 50 successive SNPs in Figure 2. The genomic windows that explained more than 0.5% of the additive genetic variance are shown in Supplementary Table 2. For the body length trait, three genomic windows that were located on SSC7, 14, and 17 were detected. For the body height trait, a series of successive genomic windows of a 3 Mb region in SSC14 was detected. For the trait of the total teat number, two genomic windows were discovered in SSC6 and SSC7. Importantly, a region (SSC7: 97.18–97.87 Mb) explains more than 0.5% of the additive genetic variance relevant to the traits of body length and total teat number. In addition, the genetic windows at SSC14: 128.29–128.86 Mb were also associated with body length and height traits. The results of single-locus GWAS and WssGWAS showed the same quantitative trait loci (QTL) region at the traits of body length and total teat number, and almost significant SNPs were mapped to different LD blocks in the region of SSC7: 97.38–98.04 Mb (Figure 3). We also analyzed the LD pattern of the significant SNPs for the body length trait in SSC17: 15.30–17.75 Mb and SSC17: 21.96–22.36 Mb.
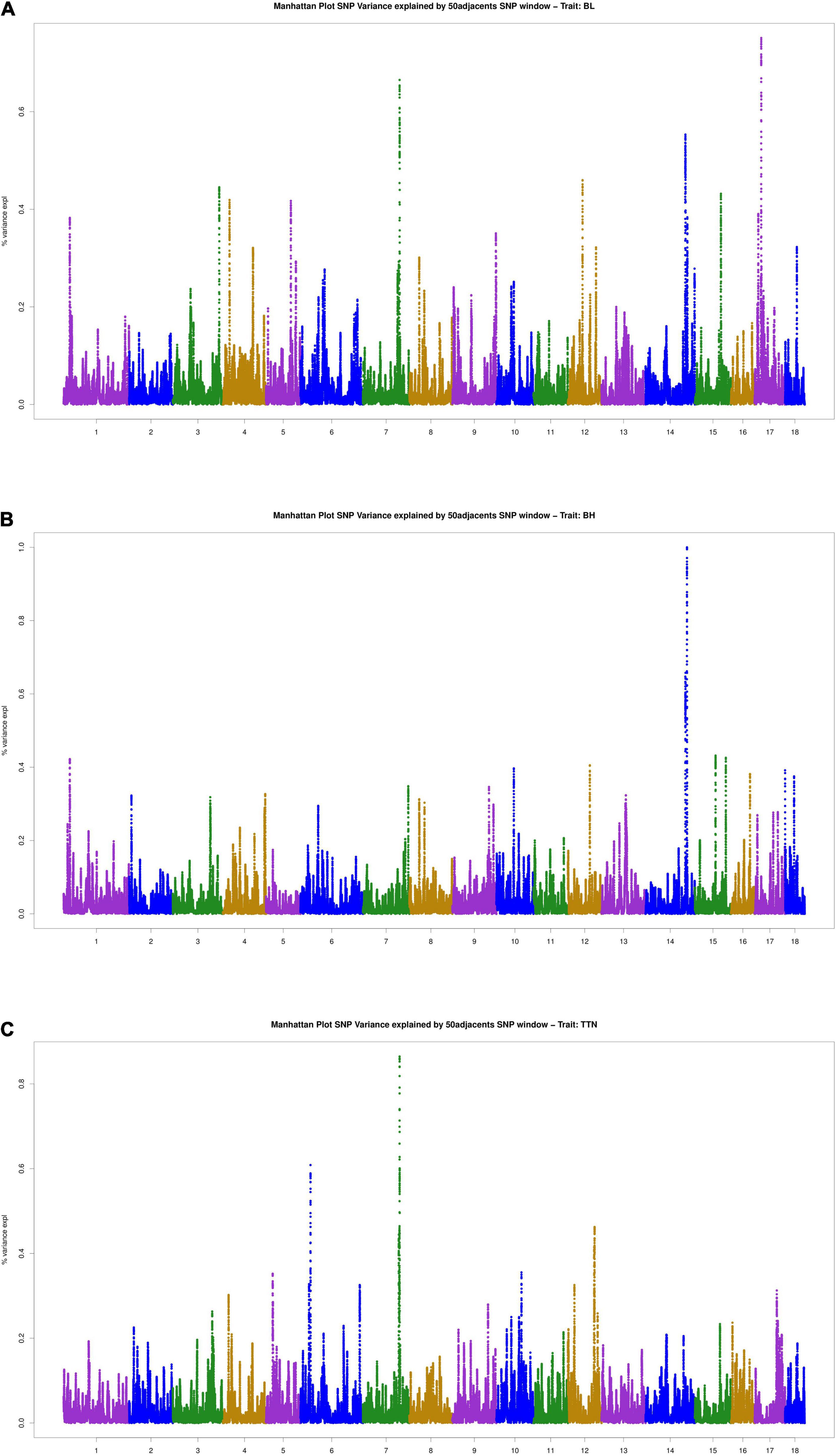
Figure 2. Manhattan plots for the percentage of genetic variance by 50 adjacent SNP windows for (A) body length, (B) body height, and (C) total teat number. Variance expl (%) represents the proportion of genetic variance explained by 50 adjacent SNPs.
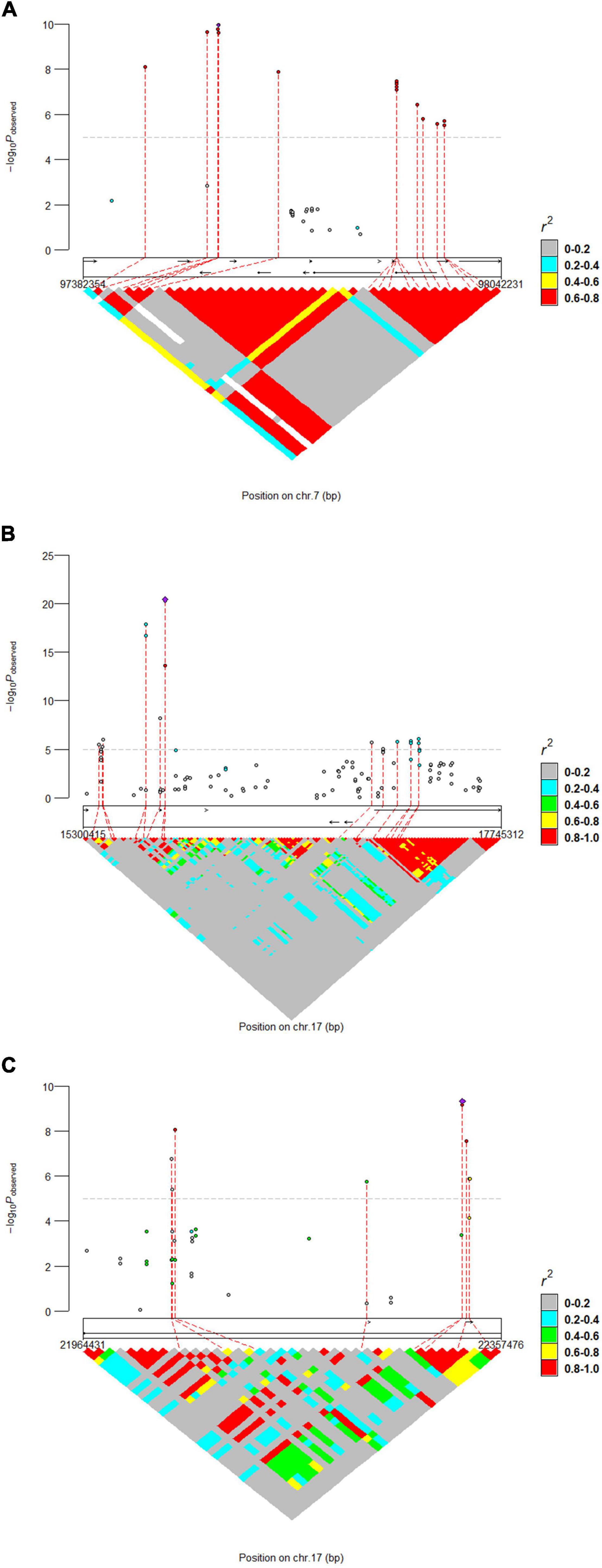
Figure 3. Locus-specific Manhattan plot and linkage disequilibrium analysis for (A) total teat number and (B,C) body length. The horizontal dashed line indicates the significance thresholds (P-value = 1.41E-5). The most significant SNP within each region was annotated with corresponding candidate genes.
Search for Candidate Genes and Functional Enrichment Analysis
The result of single-locus GWAS in the body length trait discovered an SNP associated with the BMP2 gene, located on the 15.75 Mb of SSC17. In WssGWAS, the most significant SNPs related to the PLCB1 gene were discovered at position 17.05 Mb on SSC17. In addition, a significant SNP in SSC7 related to the AREL1 gene was detected at position 97.88 Mb. In the result of the body height trait, we also discovered BMP2 as a candidate gene, and the most significant SNP was discovered at position 127.85 Mb on SSC15, near the ENSSSCG00000043546 gene. In WssGWAS, we discovered a region of approximately 2.86 Mb on SSC14, which contained approximately 23 candidate genes, and the region explained 1% genetic variance. For the total teat number trait, the most significant SNPs were located in the ABCD4 gene on SSC7. In WssGWAS, we also discovered that this region was associated with the total teat number trait. However, the most significant SNP was related to the ENTPD5 and COQ6 genes. After combining the SNP sets from the two GWAS results, a total of 35, 20, and 27 functional genes were identified for body length, body height, and total teat number, respectively. The result of GO analysis showed that these genes were enriched in 10 functional categories, including fat cell differentiation, cofactor metabolic process, behavior, cilium organization, regulation of intracellular transport, negative regulation of phosphorylation, dephosphorylation, developmental process involved in reproduction, cofactor metabolic process, and small molecule catabolic process (P < 0.01, Supplementary Table 3).
Discussion
Population Structure and Genetic Parameters Statistics
In this study, we used GBS technology to obtain 195,895 high-quality SNPs. Despite the genomic selection (GS) and GWAS methods, the advantage of GBS has been proven in previous studies (Meuwissen and Goddard, 2010; Elshire et al., 2011). GBS can obtain more recombinant fragments than SNP chips and provide high SNP coverage at a low cost. Population stratification is the main factor that influences the accuracy of GWAS, and a great number of the Large White pigs had no population stratification which supports our result. The average LD decay was very fast, it may be that the Large White population was strongly selected. We used these SNPs to calculate genetic parameters for body length, body height, and total teat number traits. The heritability of body length and total teat number was 0.25 ± 0.04 and 0.22 ± 0.04, respectively. Other studies have reported that the heritability of body length and total teat number was 0.16 to 0.32 and 0.19 to 0.365, respectively (Johnson and Nugent, 2003; Tan et al., 2017; Zhuang et al., 2020). The heritability of body height was lower than that reported in other literature (Zhou et al., 2019). The genetic correlation between body length and body height was 0.63 ± 0.15, there was a strong positive genetic correlation between body length and body height.
Candidates Regions and Genes
In both single-locus GWAS and WssGWAS, many SNPs found to be located on SSC7 between the 97.18 and 99.07 Mb regions reached a suggestive significance level for body length and total teat number traits. Though similar, these QTLs are not identical copies of one another (Supplementary Table 1). The most significant SNPs of these two traits were located in different genes. One was located upstream of the VRTN gene and another was located downstream of the VRTN gene. In a previous study, the VRTN gene was associated with carcass length, vertebral number, and teat number (Lopes et al., 2014; Yang et al., 2016; Falker-Gieske et al., 2019). In a recent study, conditional analyses, LD pattern assessment, and the phenotype distribution pattern of the VRTN alleles showed that the VRTN gene may not be a strong or the only candidate causal gene for teat number (Zhuang et al., 2020). In the trait of total teat number, the most significant SNP was near the ABCD4 gene, which is expected to have a negative effect on protein function (Zhao et al., 2020). We also performed conditional analyses and found that no signal remained when we used an SNP (S7_97595288) as a fixed effect. Two missense mutations in the ABCD4 gene were discovered; moreover, both of these two variants and lots of non-coding variants were present in all Landrace wt/wt animals (van Son et al., 2019). Thus, ABCD4 is more likely to be a causal gene.
In the body length traits, at SSC7, the most significant SNP within AREL1 was located 250.95 kb downstream of VRTN. It may be a high LD with the true causal mutation in this QTL region, causing the VRTN to be affected. BMP2 and PLCB1 genes were more likely to be true candidate genes in the body length trait. The SNP (S17_15781294) explained 9.09% of the genetic variance and neared the BMP2 gene. Zhou et al. reported the BMP2 gene as a candidate gene associated with body length and body height traits in Chinese Shushan pigs (Zhou et al., 2019). Moreover, BMP2 as a candidate gene was discovered and associated with carcass length in pigs (Falker-Gieske et al., 2019). BMP2 was also associated with the distal midpiece reflex for sperm traits in a Duroc boar population (Zhao et al., 2020). BMP2 plays an important role in the regulation of ovarian folliculogenesis and luteal formation in humans (Bai et al., 2020). We also discovered that the SNP (S17_15781304) explained 9.57% genetic variance and neared the BMP2 gene for body height trait. In the WssGWAS method, the continuous SNP explained that the top genetic variance was located in the PLCB1 gene. Meng et al. (2017) reported that the PLCB1 gene contributes to growth at the onset of puberty in a Yorkshire purebred pig population.
In WssGWAS, the QTL region SSC14: 128.29–128.86 Mb was associated with body length and body height traits. This QTL segment has not been reported as a GWAS result for these traits in pigs previously. The CACUL1 gene was located in this region and has been reported to be associated with anthropometric traits such as height and weight in humans (Galvan-Femenia et al., 2018). Interestingly, the BMP2 gene was also related to body length and body height in the single-locus GWAS result, and the QTL region on SSC14: 128.29 – 128.86 Mb was also associated with body length and body height. These findings were the same as the WssGWAS result. Both body length and height belonged to growth traits, and there was a high genetic correlation between them. From this, it is easy to understand that some QTLs or QTL regions were found to be associated with body length and body height, and these can also reflect the accuracy of our results. The 122 SNPs associated with body height were located on SSC14 between 128.29 and 131.15 Mb. This was a relatively large region, which contained approximately 20 genes and explained about 1% of the genomic variation. Nevertheless, according to the pig QTL databases, there was no body height-related gene discovered in this region. Although some SNPs showed high LD; it was still difficult to determine which gene significantly affected body height. In this region, the WDR11 gene has been associated with congenital hypogonadotropic hypogonadism and Kallmann syndrome, which are human developmental genetic disorders defined by delayed puberty and infertility (Kim et al., 2018). A search for GRK5 as a putative candidate determined that it is associated with residual feed intake in pigs (Do et al., 2014).
In another candidate gene, CPEB2 is necessary for proper porcine meiotic maturation and embryonic development (Prochazkova et al., 2018). In the WssGWAS method, the SNPs associated with total teat number were located on SSC6: 14.96 – 15.02 Mb. This region contains DHODH, ENSSSCG00000002749, TXNL4B, DHX3B, and PMFBP1 genes. Mutations in PMFBP1 cause acephalic spermatozoa syndrome that leads to infertility in male mice (Zhu et al., 2018; Li et al., 2019).
Comparison Using Two Methods
In our study, we used two methods to perform GWAS. Compared with single-locus GWAS, WssGWAS has the following advantages. Firstly, it can analyze individuals with phenotypes and pedigrees but no genotyped data (Zhang et al., 2016). Secondly, it can be adapted to complex animal models, such as the repeatability model. Wu et al. (2018) used WssGWAS to analyze reproductive traits such as total number born and the number of born alive traits in pigs. Gao et al. (2019) utilized a WssGWAS procedure to detect genetic regions and further candidate genes associated with semen traits in a Duroc boar population. Lastly, WssGWAS can reduce noise and highlight the most significant peaks (Wang et al., 2014). In our study, two approaches were mutually verified and supplemented. WssGWAS can target QTL regions associated with traits, but it is difficult to target some true candidate genes. A small effective population size and a small number of independent chromosome segments could affect the variance of the SNP windows. A few segments explained 1% of the total variance in our study, but it still not enough to determine whether we discovered a significant QTL region. This is likely because we had a large number of SNPs or possibly because of the specific characteristics of this dataset. Here, we reported the chromosome segments that explained more than 0.5% of the additive genetic variance as the significant QTL region. Compared with single-locus GWAS, WssGWAS is a powerful and potentially useful tool, especially when statistical models of analysis are complex.
Conclusion
In conclusion, we used two methods to perform GWAS for body length, body length, and total teat number traits. GWAS results for three important agricultural traits identified a series of candidate genes and showed their genetic architecture. These findings revealed the complexity of the genetic mechanism of the three traits, providing guidance for subsequent genetic improvement through genome selection.
Data Availability Statement
The original contributions presented in the study are included in the article/Supplementary Material, further inquiries can be directed to the corresponding authors.
Ethics Statement
The animal study was reviewed and approved by the Ethics Committee of South China Agricultural University.
Author Contributions
ZW and CT: conceptualization. CT: methodology. JY, LD, and CT: validation. YH: formal analysis and writing – original draft preparation. GC and DL: investigation. JY and CT: resources. YL and LY: data curation. YH and CT: writing, review, and editing. ZW: supervision, project administration, and funding acquisition. All authors have read and agreed to the published version of the manuscript.
Funding
This study was supported by the Natural Science Foundation of Guangdong Province, China (Grant No. 2018B030313011) and the Science and Technology Innovation Strategy projects of Guangdong Province, China (Grant No. 2018B020203002).
Conflict of Interest
YH, JY, LD, YL, LY, GC, CT, and ZW were employed by company Wens Foodstuff Group Co., Ltd.
The remaining author declares that the research was conducted in the absence of any commercial or financial relationships that could be construed as a potential conflict of interest.
Publisher’s Note
All claims expressed in this article are solely those of the authors and do not necessarily represent those of their affiliated organizations, or those of the publisher, the editors and the reviewers. Any product that may be evaluated in this article, or claim that may be made by its manufacturer, is not guaranteed or endorsed by the publisher.
Acknowledgments
Many thanks to all staff at the pig core breeding farms of Wens Foodstuff Group Co., Ltd. (Guangdong, China) for sample collection.
Supplementary Material
The Supplementary Material for this article can be found online at: https://www.frontiersin.org/articles/10.3389/fgene.2021.650370/full#supplementary-material
Footnotes
References
Arakawa, A., Okumura, N., Taniguchi, M., Hayashi, T., Hirose, K., Fukawa, K., et al. (2015). Genome-wide association qtl mapping for teat number in a purebred population of duroc pigs. Anim. Genet. 46, 571–575. doi: 10.1111/age.12331
Bai, L., Chang, H. M., Zhang, L., Zhu, Y. M., and Leung, P. C. K. (2020). Bmp2 increases the production of bdnf through the upregulation of probdnf and furin expression in human granulosa-lutein cells. FASEB J. 34, 16129–16143. doi: 10.1096/fj.202000940r
Bradbury, P. J., Zhang, Z., Kroon, D. E., Casstevens, T. M., Ramdoss, Y., and Buckler, E. S. (2007). Tassel: software for association mapping of complex traits in diverse samples. Bioinformatics 23, 2633–2635. doi: 10.1093/bioinformatics/btm308
Browning, B. L., Zhou, Y., and Browning, S. R. (2018). A one-penny imputed genome from next-generation reference panels. Am. J. of Hum. Genet. 103, 338–348. doi: 10.1016/j.ajhg.2018.07.015
Ding, R., Yang, M., Quan, J., Li, S., Zhuang, Z., Zhou, S., et al. (2019). Single-locus and multi-locus genome-wide association studies for intramuscular fat in duroc pigs. Front. Genet. 10:619. doi: 10.3389/fgene.2019.00619
Do, D. N., Strathe, A. B., Ostersen, T., Pant, S. D., and Kadarmideen, H. N. (2014). Genome-wide association and pathway analysis of feed efficiency in pigs reveal candidate genes and pathways for residual feed intake. Front. Genet. 5:307. doi: 10.3389/fgene.2014.00307
Elshire, R. J., Glaubitz, J. C., Sun, Q., Poland, J. A., Kawamoto, K., Buckler, E. S., et al. (2011). A Robust, simple genotyping-by-sequencing (Gbs) approach for high diversity species. PLoS One 6:e19379. doi: 10.1371/journal.pone.0019379
Falker-Gieske, C., Blaj, I., Preuss, S., Bennewitz, J., Thaller, G., and Tetens, J. (2019). Gwas for meat and carcass traits using imputed sequence level genotypes in pooled F2-Designs in pigs. G3 (Bethesda) 9, 2823–2834. doi: 10.1534/g3.119.400452
Fu, L., Jiang, Y., Wang, C., Mei, M., Zhou, Z., Jiang, Y., et al. (2020). A Genome-wide association study on feed efficiency related traits in landrace pigs. Front. Genet. 11:692. doi: 10.3389/fgene.2020.00692
Galvan-Femenia, I., Obon-Santacana, M., Pineyro, D., Guindo-Martinez, M., Duran, X., Carreras, A., et al. (2018). Multitrait genome association analysis identifies new susceptibility genes for human anthropometric variation in the gcat cohort. J. of Med. Genet. 55, 765–778. doi: 10.1136/jmedgenet-2018-105437
Gao, N., Chen, Y., Liu, X., Zhao, Y., Zhu, L., Liu, A., et al. (2019). Weighted single-step gwas identified candidate genes associated with semen traits in a duroc boar population. BMC Genomics 20:797. doi: 10.1186/s12864-019-6164-5
Hong, J. K., Kim, Y. M., Cho, K. H., Cho, E. S., Lee, D. H., and Choi, T. J. (2019). Genetic association between sow longevity and social genetic effects on growth in pigs. Asian-Australas J. Anim. Sci. 32, 1077–1083. doi: 10.5713/ajas.18.0789
Howie, B., Fuchsberger, C., Stephens, M., Marchini, J., and Abecasis, G. R. (2012). Fast and accurate genotype imputation in genome-wide association studies through pre-phasing. Nat. Genet. 44, 955–959. doi: 10.1038/ng.2354
Johnson, Z. B., and Nugent, R. A. III. (2003). Heritability of body length and measures of body density and their relationship to backfat thickness and loin muscle area in swine. J. of Anim. Sci. 81, 1943–1949. doi: 10.2527/2003.8181943x
Kim, Y. J., Osborn, D. P., Lee, J. Y., Araki, M., Araki, K., Mohun, T., et al. (2018). Wdr11-Mediated hedgehog signalling defects underlie a new ciliopathy related to kallmann syndrome. EMBO Rep. 19, 269–289. doi: 10.15252/embr.201744632
Lee, J. B., Jung, E. J., Park, H. B., Jin, S., Seo, D. W., Ko, M. S., et al. (2014). Genome-Wide association analysis to identify snp markers affecting teat numbers in an F2 intercross population between landrace and korean native pigs. Mol. Biol. Rep. 41, 7167–7173. doi: 10.1007/s11033-014-3599-2
Lee, S., Dang, C., Choy, Y., Do, C., Cho, K., Kim, J., et al. (2019). Comparison of genome-wide association and genomic prediction methods for milk production traits in Korean holstein cattle. Asian-Australas J. Anim. Sci. 32, 913–921. doi: 10.5713/ajas.18.0847
Legarra, A., Christensen, O. F., Aguilar, I., and Misztal, I. (2014). Single Step, a general approach for genomic selection. Livestock Sci. 166, 54–65. doi: 10.1016/j.livsci.2014.04.029
Li, L., Wang, J. S., Yin, C. H., and Yue, W. T. (2019). Advances in the molecular genetic studies of acephalic spermatozoa syndrome. Zhonghua Nan Ke Xue 25, 838–842.
Lopes, M. S., Bastiaansen, J. W., Harlizius, B., Knol, E. F., and Bovenhuis, H. (2014). A Genome-Wide association study reveals dominance effects on number of teats in pigs. PLoS One 9:e105867. doi: 10.1371/journal.pone.0105867
Marchini, J., Howie, B., Myers, S., McVean, G., and Donnelly, P. (2007). A new multipoint method for genome-wide association studies by imputation of genotypes. Nat. Genet. 39, 906–913. doi: 10.1038/ng2088
Meng, Q., Wang, K., Liu, X., Zhou, H., Xu, L., Wang, Z., et al. (2017). Identification of growth trait related genes in a yorkshire purebred pig population by genome-wide association studies. Asian-Australas J. Anim. Sci. 30, 462–469. doi: 10.5713/ajas.16.0548
Meuwissen, T., and Goddard, M. (2010). Accurate prediction of genetic values for complex traits by whole-genome resequencing. Genetics 185, 623–631. doi: 10.1534/genetics.110.116590
Nikkila, M. T., Stalder, K. J., Mote, B. E., Rothschild, M. F., Gunsett, F. C., Johnson, A. K., et al. (2013). Genetic associations for gilt growth, compositional, and structural soundness traits with sow longevity and lifetime reproductive performance. J. of Anim. Sci. 91, 1570–1579. doi: 10.2527/jas.2012-5723
Prochazkova, B., Komrskova, P., and Kubelka, M. (2018). Cpeb2 is necessary for proper porcine meiotic maturation and embryonic development. Int. J. of Mol. Sci. 19:3138. doi: 10.3390/ijms19103138
Purcell, S., Neale, B., Todd-Brown, K., Thomas, L., Ferreira, M. A., Bender, D., et al. (2007). Plink: a tool set for whole-genome association and population-based linkage analyses. Am. J. of Hum. Genet. 81, 559–575. doi: 10.1086/519795
Rohrer, G. A., and Nonneman, D. J. (2017). Genetic analysis of teat number in pigs reveals some developmental pathways independent of vertebra number and several loci which only affect a specific side. Genet. Select. Evol. 49:4.
Schmid, M., Maushammer, M., Preuss, S., and Bennewitz, J. (2018). Mapping Qtl for production traits in segregating pietrain pig populations using genome-wide association study results of F2 crosses. Anim. Genet. 49, 317–320. doi: 10.1111/age.12663
Suwannasing, R., Duangjinda, M., Boonkum, W., Taharnklaew, R., and Tuangsithtanon, K. (2018). The identification of novel regions for reproduction trait in landrace and large white pigs using a single step genome-wide association study. Asian-Australas J. Anim. Sci. 31, 1852–1862. doi: 10.5713/ajas.18.0072
Tan, C., Wu, Z., Ren, J., Huang, Z., Liu, D., He, X., et al. (2017). Genome-Wide association study and accuracy of genomic prediction for teat number in duroc pigs using genotyping-by-sequencing. Genetics Selection Evolution 49:35.
van Son, M., Lopes, M. S., Martell, H. J., Derks, M. F. L., Gangsei, L. E., and Kongsro, J. (2019). A Qtl for number of teats shows breed specific effects on number of vertebrae in pigs: bridging the gap between molecular and quantitative genetics. Front. Genet. 10:272. doi: 10.3389/fgene.2019.00272
Verardo, L. L., Silva, F. F., Lopes, M. S., Madsen, O., Bastiaansen, J. W., Knol, E. F., et al. (2016). Revealing new candidate genes for reproductive traits in pigs: combining bayesian gwas and functional pathways. Genet. Select. Evol. 48:9.
Wang, H., Misztal, I., Aguilar, I., Legarra, A., Fernando, R. L., Vitezica, Z., et al. (2014). Genome-Wide association mapping including phenotypes from relatives without genotypes in a single-step (Ssgwas) for 6-week body weight in broiler chickens. Front. Genet. 5:134. doi: 10.3389/fgene.2014.00134
Wang, L., Zhang, Y., Zhang, T., Zhang, L., Yan, H., Liu, X., et al. (2017). Genotyping by sequencing reveals a new locus for pig teat number. Anim. Genet. 48, 470–472. doi: 10.1111/age.12547
Wen, W., He, Z., Gao, F., Liu, J., Jin, H., Zhai, S., et al. (2017). A high-density consensus map of common wheat integrating four mapping populations scanned by the 90K Snp array. Front. Plant Sci. 8:1389. doi: 10.3389/fpls.2017.01389
Wu, P., Yang, Q., Wang, K., Zhou, J., Ma, J., Tang, Q., et al. (2018). Single step genome-wide association studies based on genotyping by sequence data reveals novel loci for the litter traits of domestic pigs. Genomics 110, 171–179. doi: 10.1016/j.ygeno.2017.09.009
Yang, J., Huang, L., Yang, M., Fan, Y., Li, L., Fang, S., et al. (2016). Possible introgression of the Vrtn mutation increasing vertebral number, carcass length and teat number from Chinese pigs into European pigs. Sci. Rep. 6:19240.
Yang, J., Lee, S. H., Goddard, M. E., and Visscher, P. M. (2011). Gcta: a tool for genome-wide complex trait analysis. Am. J. of Hum. Genet. 88, 76–82. doi: 10.1016/j.ajhg.2010.11.011
Zhang, C., Dong, S. S., Xu, J. Y., He, W. M., and Yang, T. L. (2019). Poplddecay: a fast and effective tool for linkage disequilibrium decay analysis based on variant call format files. Bioinformatics 35, 1786–1788. doi: 10.1093/bioinformatics/bty875
Zhang, X., Lourenco, D., Aguilar, I., Legarra, A., and Misztal, I. (2016). Weighting strategies for single-step genomic blup: an iterative approach for accurate calculation of Gebv and Gwas. Front. Genet. 7:151. doi: 10.3389/fgene.2016.00151
Zhao, Y., Gao, N., Li, X., El-Ashram, S., Wang, Z., Zhu, L., et al. (2020). Identifying candidate genes associated with sperm morphology abnormalities using weighted single-step Gwas in a Duroc Boar population. Theriogenology 141, 9–15. doi: 10.1016/j.theriogenology.2019.08.031
Zhou, L., Zhao, W., Fu, Y., Fang, X., Ren, S., and Ren, J. (2019). Genome-Wide detection of genetic loci and candidate genes for teat number and body conformation traits at birth in Chinese sushan pigs. Anim. Genet. 50, 753–756. doi: 10.1111/age.12844
Zhou, X., and Stephens, M. (2012). Genome-wide efficient mixed-model analysis for association studies. Nat. Genet. 44, 821–824. doi: 10.1038/ng.2310
Zhou, X., and Stephens, M. (2014). Efficient multivariate linear mixed model algorithms for genome-wide association studies. Nat. Methods 11, 407–409. doi: 10.1038/nmeth.2848
Zhu, F., Liu, C., Wang, F., Yang, X., Zhang, J., Wu, H., et al. (2018). Mutations in Pmfbp1 cause Acephalic spermatozoa syndrome. Am. J. of Hum. Genet. 103, 188–199.
Keywords: genome-wide association study, body length, body height, total teat number, Large white pigs
Citation: Hong Y, Ye J, Dong L, Li Y, Yan L, Cai G, Liu D, Tan C and Wu Z (2021) Genome-Wide Association Study for Body Length, Body Height, and Total Teat Number in Large White Pigs. Front. Genet. 12:650370. doi: 10.3389/fgene.2021.650370
Received: 07 January 2021; Accepted: 15 June 2021;
Published: 02 August 2021.
Edited by:
Fabyano Fonseca Silva, Universidade Federal de Viçosa, BrazilReviewed by:
Diana Acosta, Consejo Nacional de Investigaciones Científicas y Técnicas (CONICET), ArgentinaLucas Lima Verardo, Universidade Federal dos Vales do Jequitinhonha e Mucuri, Brazil
Copyright © 2021 Hong, Ye, Dong, Li, Yan, Cai, Liu, Tan and Wu. This is an open-access article distributed under the terms of the Creative Commons Attribution License (CC BY). The use, distribution or reproduction in other forums is permitted, provided the original author(s) and the copyright owner(s) are credited and that the original publication in this journal is cited, in accordance with accepted academic practice. No use, distribution or reproduction is permitted which does not comply with these terms.
*Correspondence: Zhenfang Wu, d3pmZW1haWxAMTYzLmNvbQ==; Cheng Tan, dGFuY2hlbmcyMDA1MDhAMTYzLmNvbQ==
†These authors have contributed equally to this work