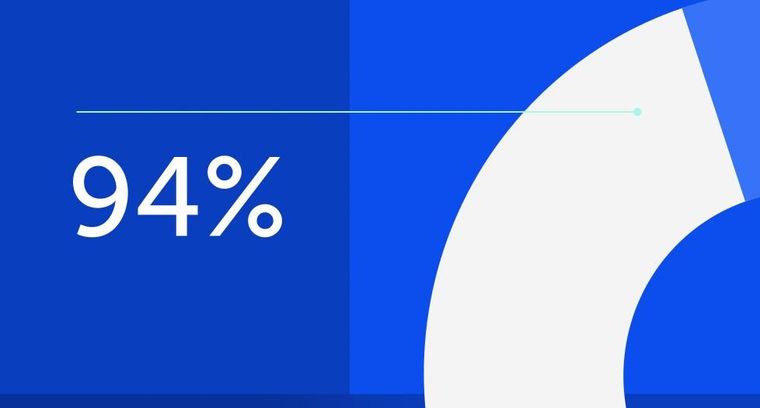
94% of researchers rate our articles as excellent or good
Learn more about the work of our research integrity team to safeguard the quality of each article we publish.
Find out more
ORIGINAL RESEARCH article
Front. Genet., 28 May 2021
Sec. Systems Biology Archive
Volume 12 - 2021 | https://doi.org/10.3389/fgene.2021.640951
Adult patients with atopic dermatitis (AD) present relatively higher rates of major depressive disorder (MDD). However, the underlying mechanism is largely unknown. Here, we first conducted a systematic literature-based data mining to identify entities linking AD and MDD, including proteins, cells, functional classes, and small molecules. Then we conducted an AD-RNA expression data-based mega-analysis to test the expression variance of the genes that were regulators of MDD. After that, a Fisher Exact test-based pathway enrichment analysis (PEA) was performed to explore the AD-driven MDD-genetic regulators’ functionality. We identified 22 AD-driven entities that were up-stream MDD regulators, including 11 genes, seven small molecules, three functional classes, and one cell. AD could exert a promoting effect on the development of MDD. Four of the 11 genes demonstrated significant expression changes in AD patients in favor of the development of MDD. PEA results showed that AD mainly drives cytokine/chemokine regulation and neuroinflammatory response-related pathways to influence the pathological development of MDD. Our results supported the promotion role of AD in the pathological development of MDD, including the regulation of multiple genetic regulators of MDD involved in cytokine/chemokine regulation and inflammatory response.
Atopic dermatitis (AD), also known as atopic eczema, is long-term inflammation of the skin. AD is characterized by pruritic erythema lesions, commonly located in curved areas, face, and hands (Tollefson et al., 2014). AD is not fatal, but the accompanying irritation, itching, and other symptoms can cause sleep disturbance and heat sensation, thereby increasing AD patients’ psychological burden (Absolon et al., 1997). For example, a case and control study conducted in school-aged children indicated that AD cases are at high risk of developing psychological difficulties (Absolon et al., 1997).
The relationship between AD symptoms and the prevalence of depression was reported, such as major depressive disorder (Hashiro and Okumura, 1997; Gupta and Gupta, 1998; Sanna et al., 2014; Cheng et al., 2015; Lee and Shin, 2017). Major depressive disorder (MDD), also known as depression, is a mental disorder characterized by at least 2 weeks of pervasive low mood. It has been reported that adult patients with AD had relatively higher rates of clinical depression, antidepressant use, and suicidality (Cheng and Silverberg, 2019). Approximately 1 in 3 adults with AD met diagnostic criteria for MDD (Blaiss, 2019). Besides, the associations’ strengths varied depending on the severity of AD symptoms (Hashiro and Okumura, 1994; Sanna et al., 2014). Hashiro et al. reported that patients with AD increased the scores according to the severity of their AD on a psychological disturbances scale (Hashiro and Okumura, 1994). Other cross-sectional, population-based studies found consistent results (Sanna et al., 2014).
Although there have been concerns about MDD among patients with AD, the underlying mechanism that AD promotes the development of MDD is largely unknown. Here, we hypothesize that the AD-driven molecule changes in patients may promote the development of MDD. We first conducted a systematic literature data mining to uncover connections between AD and MDD at different levels. Then, we performed a mega-analysis to test the expression levels of MDD regulators (genes) in the case of AD. At last, a gene set enrichment analysis was conducted to explore the functional profile of the genetic regulators of MDD that was also driven by AD. Our results enabled us to uncover functional networks and pathways that may partially explain AD’s influences on MDD.
To explore the underlying mechanism of increased MDD risk for patients with AD, we first conducted a systematic literature-based data mining to identify entities linking AD and MDD, including proteins, cells, functional classes, and small molecules. Then we conducted an AD-RNA expression data-based mega-analysis to test the expression variance of the genes that were regulators of MDD. After that, we performed pathway analysis to explore the functionality of the AD-driven MDD-genetic regulators. We provide the workflow diagram of this study in Figure 1.
Predominant molecular pathways and networks are becoming a common aid in inferring novel pathophysiological insights from changes in the levels of various biomolecules profiled in a high-throughput fashion (Ochs, 2010). Here, assisted by the tool Pathway Studio1 (version 12.3.0.16), we conducted systematic literature-data mining to uncover biological factors linking AD to MDD. Pathway Studio contains a literature-based database covering over 24 million PubMed abstracts and over 3.5 million Elsevier and 3rd part full-text papers. It contains 13 types of entities, including 138,106 genes, 1,053,259 small molecules, 5,489 functional classes, and 4,155 cells. There were about 12.8 million relations identified among these entities, with each of them being supported by one or more references. Here, we identified the biological factors influencing AD and also were regulators of MDD, and provided the relevant information for the references supporting each of these relations in Supplementary Material AD_MDD→Ref4AD_MDD. Specifically, we first identified the cells, functional classes, small molecules, and genes that regulate MDD by employing the network building function of Pathway Studio. These molecules were identified through literature data mining to show the relationship with MDD. Details regarding the network building function of Pathway Studio were described in https://supportcontent.elsevier.com/Support%20Hub/Pathway%20Studio/7680_Network_Builder.pdf. Then, following the same process, we identified the items that were regulated by AD. All relationships without polarity (positive or negative) were filtered out. Then the overlapped items were used to construct the network that connects AD and MDD. The definition of different entities and relationships within the network were presented in https://supportcontent.elsevier.com/RightNow%20Next%20Gen/Pathway%20Studio/2683_PS_QuickStartGuide_2020.pdf.
Following the initial search with ‘atopic dermatitis’, 103 microarray expression datasets were identified on gene expression omnibus (GEO).2Subsequently, the following criteria were applied:
1) The organism used in the study was Homo sapiens.
2) The data type was microarray expression profiling.
3) The studies were limited to a comparison between AD and healthy controls.
4) The original data and the corresponding format file were downloadable.
A total of nine datasets satisfied the mega-analysis’ inclusion criteria. We provided the information of these datasets in Table 1.
For the 203 genes that were upstream regulators of MDD (see Supplementary Material AD_MDD→MDD Regulators), we performed a partial mega-analysis (Dong et al., 2020) using the nine RNA expression data presented in Table 1. The log2 fold-change (LFC) of the gene expression level was used to indicate the effect size. Both fixed-effect and random-effects models were employed to investigate and compare the effect size (Borenstein et al., 2010). Mega-analysis is a one-stage approach that analyzes all individual datasets in one statistical model to estimate an overall effect, and meta-analysis is a two-stage approach, analyzing the individual datasets from each study separately to obtain summary data and then using standard meta-analytical techniques, such as a random-effects meta-analysis model (Boedhoe et al., 2019). In this study, the term “mega-analysis” instead of “meta-analysis” was used to reflect the fact that the effect size of each gene was calculated from the original data using one statistical analysis workflow rather than extracted summary results from previous studies.
The heterogeneity of the mega-analysis was analyzed to study the variance within and between different studies. In the case that the total variance (Q) was equal to or smaller than the expected between-study variance (df), the within-study variance percentage (ISq) = 100% * (Q-df)/Q was set at 0, and a fixed-effect model was selected for the mega-analysis. Otherwise, a random-effects model was selected. Q-p represents the probability that the total variance was only due to within-study variance. The current study presented all the mega-analysis results identified in AD_MDD→Mega-analysis. All analyses were performed using Matlab (version R2017a3). Mega-analysis package was developed according to the algorithm developed in (Borenstein et al., 2010).
Among the 203 genes tested in mega-analysis, those showing significant expression changes in AD patients were identified, and their relationship with MDD was studied through Pathway Studio-assisted literature data mining. The results will be used to construct an additional functional network that may add more insights into the ADMDD regulation mechanism.
A multiple linear regression (MLR) model was employed to investigate the possible influence of sample size, country of origin, and study date on the gene expression in the case of AD. p-values were reported for each of these factors.
To test the functional profile of the genes involved in the AD-driven MDD genetic regulators, a Pathway Enrichment Analysis (PEA) was conducted using Pathway Studio (version 12.1.0.16)4 against Gene Ontology (GO).5 Fisher Exact test p-value was used to determine the overlap between an input gene list and a GO term.6 The PEA results were reported with enrichment p-value corrected using the original Benjamini and Hochberg false discovery ratio (FDR) procedure (Benjamini and Hochberg, 1995). Based on the PEA results, a protein-protein interaction (PPI) network was constructed. Two genes were recognized as connected if they were identified to play roles within at least one common pathway or functional group. We also employed Human Reference Interactome (HuRI) to explore the physical interactions of proteins we found relevant with mega-analysis.
Through large-scale literature data mining, we identified 18 AD-driven entities that were up-stream MDD regulators, as shown in Figure 2. These 18 entities include seven genes, seven small molecules, three functional classes, and one cell. Three of these entities were MDD inhibitors, including vitamin D3, Mg2+, and ascorbic acid. AD could promote the development of MDD by deactivating these AD inhibitors. On the other hand, AD could activate 15 MDD promoters, which may exert stronger promoting effects on the development of MDD. In the Supplementary Material AD_MDD→Ref4AD_MDD, the reference-related information was provided for each of these relationships in Figure 2, including titles and sentences where these relationships were identified.
Figure 2. Atopic dermatitis driven biology entities that promote the development of major depressive disorder.
Mega-analysis showed six genes presented significant expression changes in AD patients, as shown in Table 2. Moreover, the MLR results in Table 2 suggested that the sample size was a significant factor to influence the expression levels of one gene (ISG15). However, the three parameters (country, sample size, and study age) presented no significant influence on most of these six genes.
Pathway Studio assisted literature data mining results showed that, among these genes that presented the significant expression variations in AD, the expression changes of four genes favored the development of MDD, as shown in Figure 3. The references supporting the relation between MDD and the four genes in Figure 3 were presented in AD_MDD→Ref4AD_MDD1. The relationships between AD and these four genes were built based on mega-analysis results.
Figure 3. Mega-analysis uncovered four genes showed significant expression changes in atopic dermatitis in favor of the development of major depressive disorder.
The PEA was conducted to test the functional profile of the 11 genes identified in the AD-driven pathways in Figures 2, 3. The 10 most significantly enriched pathways (p-value < 5.18E-5, q = 0.001 for FDR) are presented in Figure 4A. The full 38 pathways/gene sets enriched with nine genes (p-value < 0.001) have been listed in Supplementary Material AD_MDD→PEA.
Figure 4. Gene set enrichment analysis results and gene–gene interaction network. (A) The top 10 GO terms enriched by 11 atopic dermatitis-driven genes promoting the development of major depressive disorder. (B) The pathway-based gene-gene interaction network. The number within each cell represents the number of pathways two genes shared.
As shown in Figure 4A, most of these pathways/gene sets were related to cytokine/chemokine regulation and inflammatory response. Moreover, five genes were enriched in three immune system-related pathways [p-value: (0.00011, 0.00096); GO ID: 0002822; 0002819; and 0002700]. These pathways were previously implicated in the development of MDD (Kim et al., 2012; Miller and Raison, 2016; Money et al., 2016). For detailed information on these significantly enriched pathways, please refer to Supplementary Material AD_MDD→PEA.
Based on the PEA results, we build a gene-gene interaction (GGI) network, as shown in Figure 4B. Results showed that most of these genes play roles in multiple common pathways, indicating the functional linkage among them in the regulations of MDD.
We also used HuRI (Human Reference Interactome) to explore the physical interactions of genes identified in both function networks (Figures 2, 3), as shown in Figure 5. Results showed that genes CRH, NPPB, and BMP7 were physically linked to common targets, which supported the potential functional connection among the three genes.
In this study, we integrated literature data mining results and RNA expression data-based mega-analysis results to construct functional networks revealing potential mechanisms underlying the MDD promoting-effect of AD. Our results showed that AD could influence multiple MDD regulators at different levels and drive cytokine/chemokine regulation and inflammatory response-related pathways that play important roles in the development of MDD.
Literature data mining showed that AD could impact seven genes, seven small molecules, three functional classes, and one cell, which are upstream regulators of MDD. AD patients present accumulated inflammatory cells (Sung et al., 2011), which may induce depression (Qian et al., 2019). At the functional class level, AD has been shown to present over-expressed cytokines, serotonin receptors, and inflammatory cytokines (Schmid-Ott et al., 2001; Kitaichi et al., 2006; Kim et al., 2013), which play critical roles in the pathogenesis of MDD (González-Maeso et al., 2002; Miller, 2009). The top 10 GO terms enriched by 11 atopic dermatitis-driven genes promote major depressive disorder development. By the pathway-based gene-gene interaction network, the number within each cell represents the number of pathways two genes shared.
AD patients demonstrated increased acetylcholine levels (Profita et al., 2008), while clinical studies showed that increased central acetylcholine could lead to a depressed mood (Dulawa and Janowsky, 2019). AD patients also present increased levels of reactive oxygen species (ROS; Choi et al., 2010), catecholamine (Buske-Kirschbaum et al., 2002), and arabinosyladenine triphosphate (ATP; Blunder et al., 2018), which have pivotal roles in the biological mechanisms of MDD (Xu et al., 2011; Cao et al., 2013; Dhingra and Bansal, 2015). On the other hand, AD patients have lower levels of vitamin C, vitamin D3, and Mg2+, which were shown to have therapeutic value in MDD (Bodnar and Wisner, 2005; Ghasemi et al., 2014; Smolders et al., 2017). These findings suggested that AD might promote the development of MDD by regulating multiple small molecules in the human body.
At the genetic level, there were 11 genes which have been shown as AD-driven regulators of MDD, including eight MDD promoters (TNF, IL33, NPPB, CRH, IL6, IL4, AGER, and ISG15) and three MDD inhibitors (BMP7, USP46, and AR), as shown in Figures 2, 3. Specifically, four of these 11 genes presented significant expression changes in AD cases (mega-analysis using nine AD RNA expression datasets; see Table 2), including ISG15, BMP7, USP46, and AR. These results support the relationship between these four genes, AD, and MDD, suggesting that AD might regulate the expression levels of these four genes to influence the pathological development of MDD. However, considering the expression data sources were from skin and blood, a direct experiment should be conducted to test the expression level connection between AD and MDD (e.g., only using data collected from peripheral blood).
We also noted that the expression changes of the other two genes (CCL22 and CC2D1A) in AD patients might not favor the development of MDD. Therefore, further study is needed to test the genetic regulators of MDD in AD patients.
PEA results showed that most of the AD-driven genes regulating MDD were enriched within cytokine/chemokine regulation and neuroinflammatory response-related pathways, as shown in Figure 4 and Supplementary Material AD_MDD→PEA. AD has been shown to present over-expressed cytokines/chemokine (Schmid-Ott et al., 2001), which play critical roles in the pathogenesis of MDD (Miller, 2009). Several main findings supported the neuroinflammatory theory of MDD, which hypothesizes that cerebral inflammation is crucial to the development of depression and other neuropsychiatric diseases (Duque and Fricchione, 2019). Elevated microglia activation and neuroinflammatory response have been observed in the brains of AD by using a mice model (Park et al., 2020), which may add to the explanation of the AD-promoting effect on the development of MDD. Moreover, three out of the 11 AD-driven MDD regulating genes (Figures 2, 3) presented physical linkage to common targets, supporting their functional connection.
Our study indicated that AD might drive multiple molecules that were implicated as upstream regulators of MDD, including genes involved in cytokine/chemokine regulation and inflammatory response.
The original contributions presented in the study are included in the article/Supplementary Material; further inquiries can be directed to the corresponding author.
TY and XH developed the study design, analyzed the data, and wrote the original paper. All authors contributed to the article and approved the submitted version.
This work was supported by the Sichuan Science and Technology Support Program (Grant No. 2019YFS0218).
The authors declare that the research was conducted in the absence of any commercial or financial relationships that could be construed as a potential conflict of interest.
The Supplementary Material for this article can be found online at: https://www.frontiersin.org/articles/10.3389/fgene.2021.640951/full#supplementary-material
2. ^https://www.ncbi.nlm.nih.gov/geo/
3. ^https://www.mathworks.com/products/matlab.html
6. ^https://www.pathwaycommons.org/guide/primers/statistics/fishers_exact_test/
Absolon, C. M., Cottrell, D., Eldridge, S. M., and Glover, M. T. (1997). Psychological disturbance in atopic eczema: the extent of the problem in school-aged children. Br. J. Dermatol. 137, 241–245. doi: 10.1046/j.1365-2133.1997.18121896.x
Benjamini, Y., and Hochberg, Y. (1995). Controlling the false discovery rate: a practical andpowerful approach to multiple testing. J. Roy. Statist. Soc. Ser. B57, 289–300. doi: 10.2307/2346101
Blaiss, M. S. (2019). Socioeconomics of atopic dermatitis-can we afford new treatments? Ann. Allergy Asthma Immunol. 122, 355–357. doi: 10.1016/j.anai.2018.07.021
Blunder, S., Kõks, S., Kõks, G., Reimann, E., Hackl, H., Gruber, R., et al. (2018). Enhanced expression of genes related to xenobiotic metabolism in the skin of patients with atopic dermatitis but not with Ichthyosis vulgaris. J. Invest. Dermatol. 138, 98–108. doi: 10.1016/j.jid.2017.08.036
Bodnar, L. M., and Wisner, K. L. (2005). Nutrition and depression: implications for improving mental health among childbearing-aged women. Biol. Psychiatry 58, 679–685. doi: 10.1016/j.biopsych.2005.05.009
Boedhoe, P., Heymans, M., Schmaal, L., Abe, Y., Alonso, P., Ameis, S., et al. (2019). An empirical comparison of meta‐ and mega-analysis with data from the ENIGMA obsessive-compulsive disorder working group. Front. Neuroinform. 12:102. doi: 10.3389/fninf.2018.00102
Borenstein, M., Hedges, L. V., Higgins, J. P., and Rothstein, H. R. (2010). A basic introduction to fixed-effect and random-effects models for meta-analysis. Res. Synth. Methods 1, 97–111. doi: 10.1002/jrsm.12
Buske-Kirschbaum, A., Geiben, A., Höllig, H., Morschhäuser, E., and Hellhammer, D. (2002). Altered responsiveness of the hypothalamus-pituitary-adrenal axis and the sympathetic adrenomedullary system to stress in patients with atopic dermatitis. J. Clin. Endocrinol. Metab. 87, 4245–4251. doi: 10.1210/jc.2001-010872
Cao, X., Li, L. P., Wang, Q., Wu, Q., Hu, H. H., Zhang, M., et al. (2013). Astrocyte-derived ATP modulates depressive-like behaviors. Nat. Med. 19, 773–777. doi: 10.1038/nm.3162
Cheng, C. M., Hsu, J. W., Huang, K. L., Bai, Y. M., Su, T. P., Li, C. T., et al. (2015). Risk of developing major depressive disorder and anxiety disorders among adolescents and adults with atopic dermatitis: a nationwide longitudinal study. J. Affect. Disord. 178, 60–65. doi: 10.1016/j.jad.2015.02.025
Cheng, B. T., and Silverberg, J. I. (2019). Depression and psychological distress in US adults with atopic dermatitis. Ann. Allergy Asthma Immunol. 123, 179–185. doi: 10.1016/j.anai.2019.06.002
Choi, H., Kim, S., Kim, H. J., Kim, K. M., Lee, C. H., Shin, J. H., et al. (2010). Sphingosylphosphorylcholine down-regulates filaggrin gene transcription through NOX5-based NADPH oxidase and cyclooxygenase-2 in human keratinocytes. Biochem. Pharmacol. 80, 95–103. doi: 10.1016/j.bcp.2010.03.009
Dhingra, D., and Bansal, S. (2015). Antidepressant-like activity of plumbagin in unstressed and stressed mice. Pharmacol. Rep. 67, 1024–1032. doi: 10.1016/j.pharep.2015.03.001
Dong, Y., Cao, H., Cao, R., and Baranova, A. (2020). TNFRSF12A and CD38 contribute to a vicious circle for chronic obstructive pulmonary disease by engaging senescence pathways. Front. Cell Dev. Biol. 8:330. doi: 10.3389/fcell.2020.00330
Dulawa, S. C., and Janowsky, D. S. (2019). Cholinergic regulation of mood: from basic and clinical studies to emerging therapeutics. Mol. Psychiatry 24, 694–709. doi: 10.1038/s41380-018-0219-x
Duque, L., and Fricchione, G. (2019). Fibromyalgia and its new lessons for neuropsychiatry. Med. Sci. Monit. Basic Res. 25, 169–178. doi: 10.12659/MSMBR.915962
Ghasemi, M., Phillips, C., Trillo, L., De Miguel, Z., Das, D., and Salehi, A. (2014). The role of NMDA receptors in the pathophysiology and treatment of mood disorders. Neurosci. Biobehav. Rev. 47, 336–358. doi: 10.1016/j.neubiorev.2014.08.017
González-Maeso, J., Rodríguez-Puertas, R., Meana, J. J., García-Sevilla, J. A., and Guimón, J. (2002). Neurotransmitter receptor-mediated activation of G-proteins in brains of suicide victims with mood disorders: selective supersensitivity of alpha (2A)-adrenoceptors. Mol. Psychiatry 7, 755–767. doi: 10.1038/sj.mp.4001067
Gupta, M. A., and Gupta, A. K. (1998). Depression and suicidal ideation in dermatology patients with acne, alopecia areata, atopic dermatitis and psoriasis. Br. J. Dermatol. 139, 846–850. doi: 10.1046/j.1365-2133.1998.02511.x
Hashiro, M., and Okumura, M. (1994). Anxiety, depression, psychosomatic symptoms and autonomic nervous function in patients with chronic urticaria. J. Dermatol. Sci. 8, 129–135. doi: 10.1016/0923-1811(94)90007-8
Hashiro, M., and Okumura, M. (1997). Anxiety, depression and psychosomatic symptoms in patients with atopic dermatitis: comparison with normal controls and among groups of different degrees of severity. J. Dermatol. Sci. 14, 63–67. doi: 10.1016/S0923-1811(96)00553-1
Kim, J. E., Cho, B. K., Cho, D. H., and Park, H. J. (2013). Expression of hypothalamic-pituitary-adrenal axis in common skin diseases: evidence of its association with stress-related disease activity. Acta Derm. Venereol. 93, 387–393. doi: 10.2340/00015555-1557
Kim, S. J., Lee, H., Lee, G., Oh, S. J., Shin, M. K., Shim, I., et al. (2012). CD4+CD25+ regulatory T cell depletion modulates anxiety and depression-like behaviors in mice. PLoS One 7:e42054. doi: 10.1371/journal.pone.0042054
Kitaichi, N., Shimizu, T., Honda, A., Abe, R., Ohgami, K., Shiratori, K., et al. (2006). Increase in macrophage migration inhibitory factor levels in lacrimal fluid of patients with severe atopic dermatitis. Graefes Arch. Clin. Exp. Ophthalmol. 244, 825–828. doi: 10.1007/s00417-005-0168-3
Lee, S., and Shin, A. (2017). Association of atopic dermatitis with depressive symptoms and suicidal behaviors among adolescents in Korea: the 2013 Korean youth risk behavior survey. BMC Psychiatry 17:3. doi: 10.1186/s12888-016-1160-7
Miller, A. H. (2009). Norman cousins lecture. Mechanisms of cytokine-induced behavioral changes: psychoneuroimmunology at the translational interface. Brain Behav. Immun. 23, 149–158. doi: 10.1016/j.bbi.2008.08.006
Miller, A. H., and Raison, C. L. (2016). The role of inflammation in depression: from evolutionary imperative to modern treatment target. Nat. Rev. Immunol. 16, 22–34. doi: 10.1038/nri.2015.5
Money, K. M., Olah, Z., Korade, Z., Garbett, K. A., Shelton, R. C., and Mirnics, K. (2016). An altered peripheral IL6 response in major depressive disorder. Neurobiol. Dis. 89, 46–54. doi: 10.1016/j.nbd.2016.01.015
Ochs, M. F. (2010). Knowledge-based data analysis comes of age. Brief. Bioinform. 11, 30–39. doi: 10.1093/bib/bbp044
Park, S. H., Jang, S., An, J. E., Choo, B. K., and Kim, H. K. (2020). I. inflexus (Thunb.) kudo extract improves atopic dermatitis and depressive-like behavior in DfE-induced atopic dermatitis-like disease. Phytomedicine 67:153137. doi: 10.1016/j.phymed.2019.153137
Profita, M., Bonanno, A., Siena, L., Ferraro, M., Montalbano, A. M., Pompeo, F., et al. (2008). Acetylcholine mediates the release of IL-8 in human bronchial epithelial cells by a NFkB/ERK-dependent mechanism. Eur. J. Pharmacol. 582, 145–153. doi: 10.1016/j.ejphar.2007.12.029
Qian, L., Jiang, Y., and Lin, F. (2019). Mechanism of the acupoints of the governor vessel in treatment of post-stroke depression on the base of the specificity of meridian points. World J. Acup. 29, 244–248. doi: 10.1016/j.wjam.2019.07.004
Sanna, L., Stuart, A. L., Pasco, J. A., Jacka, F. N., Berk, M., Maes, M., et al. (2014). Atopic disorders and depression: findings from a large, population-based study. J. Affect. Disord. 155, 261–265. doi: 10.1016/j.jad.2013.11.009
Schmid-Ott, G., Jaeger, B., Meyer, S., Stephan, E., Kapp, A., and Werfel, T. (2001). Different expression of cytokine and membrane molecules by circulating lymphocytes on acute mental stress in patients with atopic dermatitis in comparison with healthy controls. J. Allergy Clin. Immunol. 108, 455–462. doi: 10.1067/mai.2001.117800
Smolders, J., Rolf, L., Damoiseaux, J., and Hupperts, R. (2017). On the ethics of not supplementing low 25-hydroxyvitamin D levels in a controlled study in relapsing remitting multiple sclerosis. J. Neurol. Sci. 379:331. doi: 10.1016/j.jns.2017.06.009
Sung, Y. Y., Yoon, T., Jang, J. Y., Park, S. J., Jeong, G. H., and Kim, H. K. (2011). Inhibitory effects of Cinnamomum cassia extract on atopic dermatitis-like skin lesions induced by mite antigen in NC/Nga mice. J. Ethnopharmacol. 133, 621–628. doi: 10.1016/j.jep.2010.10.043
Tollefson, M. M., and Bruckner, A. L., Section On Dermatology (2014). Atopic dermatitis: skin-directed management. Pediatrics 134, e1735–e1744. doi: 10.1542/peds.2014-2812
Keywords: atopic dermatitis, major depressive disorder, literature-based data mining, mega-analysis, multiple linear regression analysis, gene set enrichment analysis, protein-protein interaction analysis
Citation: Yang T, Huang X, Xu J, Situ M, Xiao Q, Kural KC and Kang Y (2021) Explore the Underlying Mechanism Between Atopic Dermatitis and Major Depressive Disorder. Front. Genet. 12:640951. doi: 10.3389/fgene.2021.640951
Received: 14 December 2020; Accepted: 06 April 2021;
Published: 28 May 2021.
Edited by:
Xiaogang Wu, University of Texas MD Anderson Cancer Center, United StatesReviewed by:
Elisa Domínguez-Hüttinger, National Autonomous University of Mexico, MexicoCopyright © 2021 Yang, Huang, Xu, Situ, Xiao, Kural and Kang. This is an open-access article distributed under the terms of the Creative Commons Attribution License (CC BY). The use, distribution or reproduction in other forums is permitted, provided the original author(s) and the copyright owner(s) are credited and that the original publication in this journal is cited, in accordance with accepted academic practice. No use, distribution or reproduction is permitted which does not comply with these terms.
*Correspondence: Xuehua Huang, aHVhbmd4dWVodWExMEAxNjMuY29t; aHVhbmd4dWVodWExMEBnb3VzaW5mby5jb20=
Disclaimer: All claims expressed in this article are solely those of the authors and do not necessarily represent those of their affiliated organizations, or those of the publisher, the editors and the reviewers. Any product that may be evaluated in this article or claim that may be made by its manufacturer is not guaranteed or endorsed by the publisher.
Research integrity at Frontiers
Learn more about the work of our research integrity team to safeguard the quality of each article we publish.