- 1Guangdong Provincial Key Laboratory of Stomatology, Department of Preventive Dentistry, Guanghua School of Stomatology, Hospital of Stomatology, Sun Yat-sen University, Guangzhou, China
- 2Foshan Stomatology Hospital, School of Stomatology and Medicine, Foshan University, Foshan, China
Dental caries is a multifactorial disease that can be caused by interactions between genetic and environmental risk factors. Despite the availability of caries risk assessment tools, caries risk prediction models incorporating new factors, such as human genetic markers, have not yet been reported. The aim of this study was to construct a new model for caries risk prediction in teenagers, based on environmental and genetic factors, using a machine learning algorithm. We performed a prospective longitudinal study of 1,055 teenagers (710 teenagers for cohort 1 and 345 teenagers for cohort 2) aged 13 years, of whom 953 (633 teenagers for cohort 1 and 320 teenagers for cohort 2) were followed for 21 months. All participants completed an oral health questionnaire, an oral examination, biological (salivary and cariostate) tests, and single nucleotide polymorphism sequencing analysis. We constructed a caries risk prediction model based on these data using a random forest with an AUC of 0.78 in cohort 1 (training cohort). We further verified the discrimination and calibration abilities of this caries risk prediction model using cohort 2. The AUC of the caries risk prediction model in cohort 2 (testing cohort) was 0.73, indicating high discrimination ability. Risk stratification revealed that our caries risk prediction model could accurately identify individuals at high and very high caries risk but underestimated risks for individuals at low and very low caries risk. Thus, our caries risk prediction model has the potential for use as a powerful community-level tool to identify individuals at high caries risk.
Introduction
Permanent teeth caries was the most common chronic disease worldwide in 2016. A previous study reported that the global cost of dental diseases exceeded 540 billion dollars in 2015 and resulted in major health and financial burdens (Righolt et al., 2018). Therefore, there is an urgent need for effective caries control.
Accumulating evidence has shown a skewed distribution of caries; the majority of the disease was suffered by the minority teenagers in the population (Kaste et al., 1996). The conference of National Institutes of Health Consensus Development Conference Statement (2001) concluded that a focus on high-risk individuals was required for the prevention and control of dental caries (2001). Since caries is largely preventable, risk prediction models for early and accurate identification of teenagers at high risk of caries would be useful tools for designing more cost-effective caries control measures.
As a prerequisite for implementing minimally invasive treatment programs, caries risk prediction models (CRPMs) have huge potential in improving patient care because they allow individuals to choose appropriate non-invasive or invasive interventions (Domejean et al., 2017). There are four commonly used standardized caries risk assessment models at present: ADA (American Dental Association), CAT (Caries-Risk Assessment Tool), CAMBRA (Caries Management by Risk Assessment), and Cariogram. All these models included only environmental factors such as socio-demographic indicators, behavioral factors, plaque index, the number of Streptococcus mutans, and Lactobacillus, saliva flow, and salivary buffer capacity (Petersson and Twetman, 2015). Cariogram, one of the better CRPMs, has provided reliable results for few tests in children, but there is not enough evidence to prove its effectiveness in caries assessment and prediction. Cagetti et al. (2018) reported that the sensitivity of Cariogram in different samples ranged from 41.0 to 75.0%, while the specificity ranged from 65.8 to 88.0%.
Dental caries is a multifactorial disease caused by complex interactions between genetic and environmental risk factors. Environmental risk factors for caries included sugar-rich diet, poor oral hygiene, dental plaque, high numbers of cariogenic bacteria, inadequate salivary flow and so on (Selwitz et al., 2007). Genetic contribution to caries risk score variation has been reported to be 49.1–62.7% (Haworth et al., 2020). As a genetically complex phenotype, caries risk may be influenced by many loci with small contributions individually. These genetic factors that contribute to caries may include variants in loci for enamel formation, immune response, saliva, taste, and dietary habits (Vieira et al., 2014). Enamel formation was tested as being potentially involved in caries susceptibility. Patir et al. (2008) reported an association between enamelin (ENAM) and higher caries experience. Additionally, a relationship between the genetic variation of tuftelin (TUFT1) and caries could be detected only when the Streptococcus mutans levels were high (Slayton et al., 2005).
Therefore, CRPMs based on environmental factors alone may lead to the loss of useful information. Previous studies have suggested that constructing a disease risk prediction model with both environmental and genetic factors can stratify the disease risk more accurately than either of these factors alone (Li et al., 2019; Okubo et al., 2020). Accordingly, research is needed to construct CRPMs based on both genetic and environmental risk factors and evaluate their abilities to predict caries risk better. Thus, this prospective study aimed to construct a new CRPM including both genetic and environmental risk factors in teenagers of the Chinese population.
Materials and Methods
Study Population
This study was approved by the Ethics Committee of the Guanghua School of Stomatology, Sun Yat-sen University (ERC- [2018]01). The analysis consisted of two cohorts that began from March to April 2018 and were followed up for 21 months until the end, from December 2019 to January 2020, in Foshan, southern China. The two cohorts included 710 and 345 teenagers aged 13–14 years. Cohort 1 was used to construct the model, which included teenagers from two urban and two rural schools. Cohort 2 was used to evaluate the caries risk prediction model and included teenagers from one urban and one rural school. All participants completed an oral health questionnaire, clinical examination, and donated saliva samples at baseline. Written informed consent was obtained from the guardians of every participant before the study.
Oral Health Questionnaire
Under the guidance of their guardians, the adolescents completed a well-designed oral health questionnaire consisting of three parts: Part 1 was mainly about demographic information, Part 2 was mainly about socioeconomic information, and Part 3 was mainly about oral health-related behaviors (Wang et al., 2020a). The specific variables are as follows:
The variables in part 1: sex, age, residence, whether the child is an only child in his/her family, and his/her primary caregiver.
The variables in part 2: family income, caregivers’ education levels, and whether they have dental insurance.
The variables in part 3: frequency of tooth brushing, flossing or mouthwash habits, toothpaste containing fluoride or not, professional application of fluoride, frequency of snack consumption, sweet drink consumption, and attendance in a dental clinic in the past 6 months.
Clinical Examination
Plaque index (PlI) was evaluated using Silness and Löe’s scale (Loe, 1967), and six dental indices were recorded. Plaque samples were collected with sterile swabs, according to the procedural instructions of the cariostat kit (GangDa Medical Technology Co. Ltd., Beijing, China). The swabs were then immersed in culture media in ampules and incubated at 37°C for 48 h. Finally, the color of the medium was compared with the reference colors in the color chart provided by the cariostat kit.
After air drying, each tooth was examined and recorded as decayed, missing, or filled (DMFT). The caries status was evaluated according to the International Caries Detection and Assessment System (ICDAS) criteria (Pitts and Ekstrand, 2013). Codes 3–6 in the ICDAS system were recorded as decayed teeth. We also recorded filled and missing teeth due to caries. Oral examinations were conducted at both the baseline and after 21 months in the classrooms.
The students rinsed their mouths before the collection of unstimulated saliva. Unstimulated saliva was collected for 15 min. Students were first asked to swallow all the saliva in the mouth, then spit all the saliva into the scaled tube every 3 min and five times in total. The saliva flow rate (ml/min) was calculated, and saliva buffering capability was measured according to the Ericsson method. One milliliter of saliva was added to 3 ml of 3.3 mmol HCl within 5 min after collection and then allowed to stand for 20 mins. The final pH of the saliva was evaluated by an electrical pH meter (Wang et al., 2020b).
Selection of Candidate Genetic Markers and DNA Analysis
Single nucleotide polymorphisms (SNPs) were selected based on the results of previous studies on caries susceptibility (n = 4) and screening of tag SNPs (n = 19). We used a candidate gene approach or related-pathway strategies to screen tag SNPs. Caries-related pathway genes, such as those involved in enamel formation, immune responses, saliva secretion, and taste, were identified based on the pathogenesis of caries. The tag SNPs were screened as described in our previous study (Wang et al., 2020b). Thus, 23 target SNPs were detected in all study participants (Table 1).
From each participant, 2 ml of unstimulated saliva samples were collected and stored in Oragene DNA Self-Collection kits (Lang Fu, China) at room temperature until they were processed. Genomic DNA was extracted from saliva samples according to the manufacturer’s instructions. DNA samples were first purified using MassARRAY Nanodispenser (Sequenom, United States) and then transferred to a SpectroCHIP (Sequenom, United States) chip. Finally, the SNP markers were sequenced by matrix-assisted laser desorption/ionization time-of-flight mass spectrometry (MALDI-TOF MS) (Pang et al., 2017). First, 10 ng of genomic DNA were amplified by PCR in a final volume of 0.5 μL containing locus-specific primers at a final concentration of 10 μmol/L using 0.1-unit HotStarTaq DNA polymerase (Qiagen, Hilden, Germany). PCR conditions were 94°C for 3 min for hot start followed by 40 cycles of denaturation at 94°C for 30 s, annealing at 56°C for 25 s, and extension for 30 s at 72°C, and, finally, incubation at 72°C for 3 min. Then, PCR products were treated with shrimp alkaline phosphatase (Amersham, Freiburg, Germany) for 40 min at 37°C to remove excess deoxynucleotide triphosphates followed by 5 min at 85°C to inactivate shrimp alkaline phosphatase. Base extension reaction conditions were 94°C for 30 s followed by 40 cycles of 94°C for 5 s, 52°C for 5 s, and 80°C for 5 s, and, finally, incubation at 72°C for 3 min. The final base extension products were treated with SpectroCLEAN resin (Sequenom) to remove salts from the reaction buffer. A total of 10 nl of the reaction solution was dispensed onto a 384 format SpectroCHIP microarray (Sequenom, SanDiego, CA). The MassARRAY Analyzer Compac was used for data acquisitions from the MassARRAY SpectroCHIP. Genotyping calls were made in real-time with the Mass Array RT software (Sequenom) (Pang et al., 2020).
Statistical Analysis
Data of all teenagers in cohort 1 were used to construct a CRPM with random forest, and those of teenagers from cohort 2 were used to verify this newly constructed model. The logistic regression model was used as a reference for performance evaluation. When we analyzed the variables associated with the occurrence and development of caries, the independent variable included the environmental variables and SNPs. The dependent variable was DMFT increment (ΔDMFT) over 21 months of follow-up, which is the outcome of this study. A previous study conducted by Chaffee BW (Chaffee et al., 2015) found that the DMFT increment was about 1.01 in the low caries risk groups after 18 months of follow-up. Remember that individuals with DMFT increments of no more than one caries after 21 months of follow-up should be classified in the low caries risk group. Chi-square tests were used to identify SNPs associated with increased risk of caries, and univariate logistic analysis was used to select environmental factors associated with caries. Variables with P < 0.1 were considered statistically significant and used as predictors in the caries risk prediction model. R 3.6.1 software was used to construct the model. Using the data of the training cohort (cohort1), the random forest package was used to train the random forest model, and the nTree and mtry parameters were debugged. The random forest prediction model was the most effective when nTree = 300 and mtry = 2. In the model constructed with cohort 1, we segmented the population into five different caries risk layers based on the 5-quantiles: very low, low, moderate, high, and very high caries risk. Then, we stratified the caries risk in the cohort 2 (testing cohort) population based on the cutoff value in cohort 1. The discrimination ability of the model was evaluated using receiver operator characteristic (ROC) curve analysis. The calibration ability of the model was measured via a risk stratification plot, which was used to demonstrate the similarity of the predicted absolute risk to the absolute observed risk at different risk levels.
Results
Characteristics of Study Samples
In total, 1,055 teenagers (710 in cohort 1 and 345 in cohort 2) were recruited. The average age at baseline was 13.19 ± 0.40 years (Wang et al., 2020a). The questionnaire was completed by all teenagers. After 21 months, 953 teenagers (including 633 teenagers in cohort 1 and 320 teenagers in cohort 2) were followed up. During these 21 months, follow-up was lost for only 102 (9.66%) teenagers. The main reasons for loss of follow-up were absence in school or transfer from schools. The flow chart of the prospective longitudinal study is shown in Figure 1.
At baseline, 34.37% of the teenagers in cohort 1 and 39.88% of those in cohort 2 were affected by caries, and the mean (SD) DMFTs were 0.67 ± 1.25 and 0.84 ± 1.38, respectively. After 21 months, 57.66% of the teenagers in cohort 1 and 63.13% of those in cohort 2 developed more than one caries (ΔDMFT > 1). The mean (SD) increases in DMFTs after 21 months were 2.40 ± 2.97 in cohort 1 and 2.73 ± 3.21 in cohort 2.
Caries Risk Prediction Factors
Table 2 shows the results of a logistic analysis of the association between environmental variation and caries. Among the environmental variations, we found that “sex,” “dental attendance in the past 6 months,” “cariostat score,” and “past caries experience” were significantly associated with the caries risk (all P < 0.05).
Table 3 shows the results of the chi-square tests on the association between SNPs and caries. Among all the SNPs, rs1996315 (AQP5), and rs3790506 (TUFT1) were significantly associated with caries risk (all P < 0.05).
CRPM Training and Validation
The CRPM has been developed using logistic regression and random forest. The performance of CRPM developed using logistic regression was 0.70 (0.66–0.74) for the training cohort (Figure 2A) and 0.74 (0.68–0.79) for the test cohort (Figure 2B). The performance of the random forest was 0.78 (0.75–0.82) for the training cohort (Figure 3A) and 0.73 (0.67–0.78) for the test cohort (Figure 3B). The results showed that the prediction performance of the CRPM constructed using Random Forest was stable.
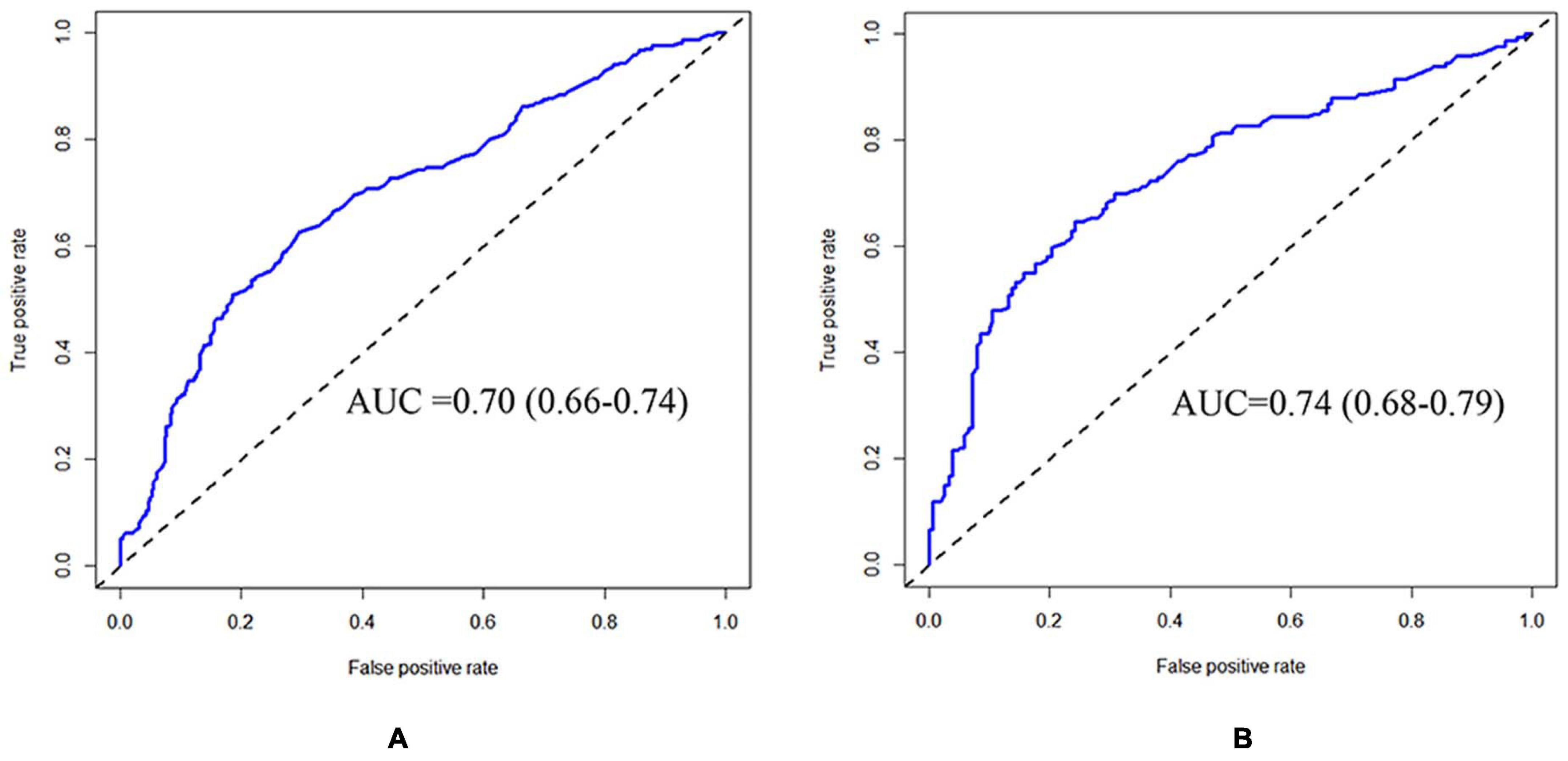
Figure 2. ROC curve of training and testing cohort (Logistic Regression Model). Measurement of the discrimination ability of the caries risk prediction model (Logistic Regression) with ROC curve. The AUC (95%CI) of the training cohort was 0.70 (0.66–0.74) (A), and the AUC (95% CI) of the testing cohort was 0.74 (0.68–0.79) (B).
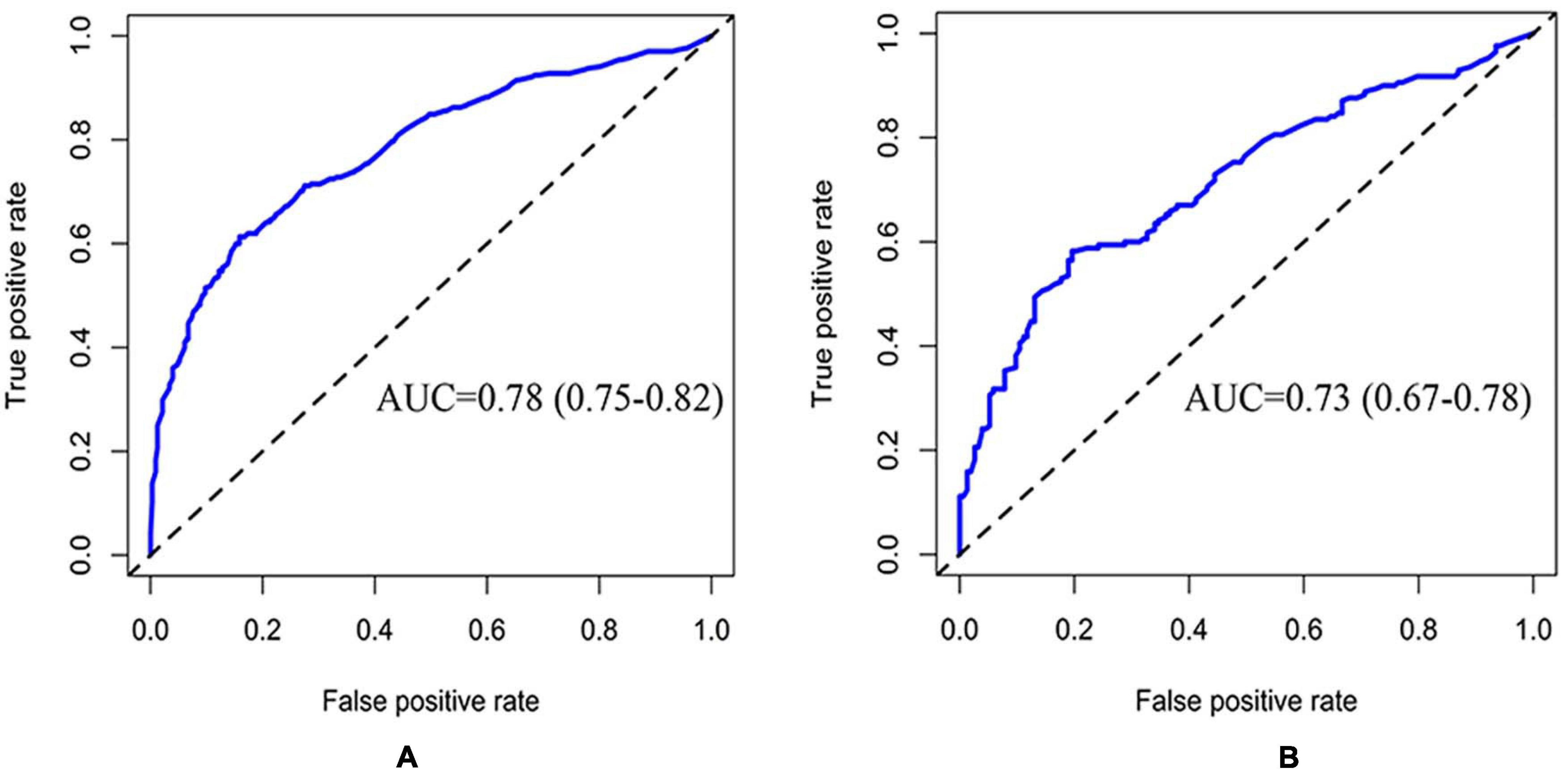
Figure 3. ROC curve of training and testing cohort (Random Forest Model). Measurement of the discrimination ability of the caries risk prediction model (Random Forest) with ROC curves. The AUC of the training cohort was 0.78 (0.75–0.82) (A), and the AUC of the testing cohort was 0.73 (0.67–0.78) (B).
The Gini coefficient of the random forest suggested that the selected variables in this prediction model could be arranged as follows according to their importance: “past caries experience,” “cariostate score,” “plaque index,” “rs3790506,” “rs1996315,” “gender,” and “whether they were only teenagers” (Figure 4).
The ability of the CRPM to identify caries risk in individuals was examined further. A risk stratification plot was created, in which the data from 320 patients in cohort 2 were sorted by increasing the predicted risk and separated into five risk layers: very low, low, medium, high, and very high. Then, the actual rate of caries incidence after 21 months was calculated for each risk layer. Figure 5 shows the degree of discrepancy between the actual and predicted risks for each of the five risk layers.
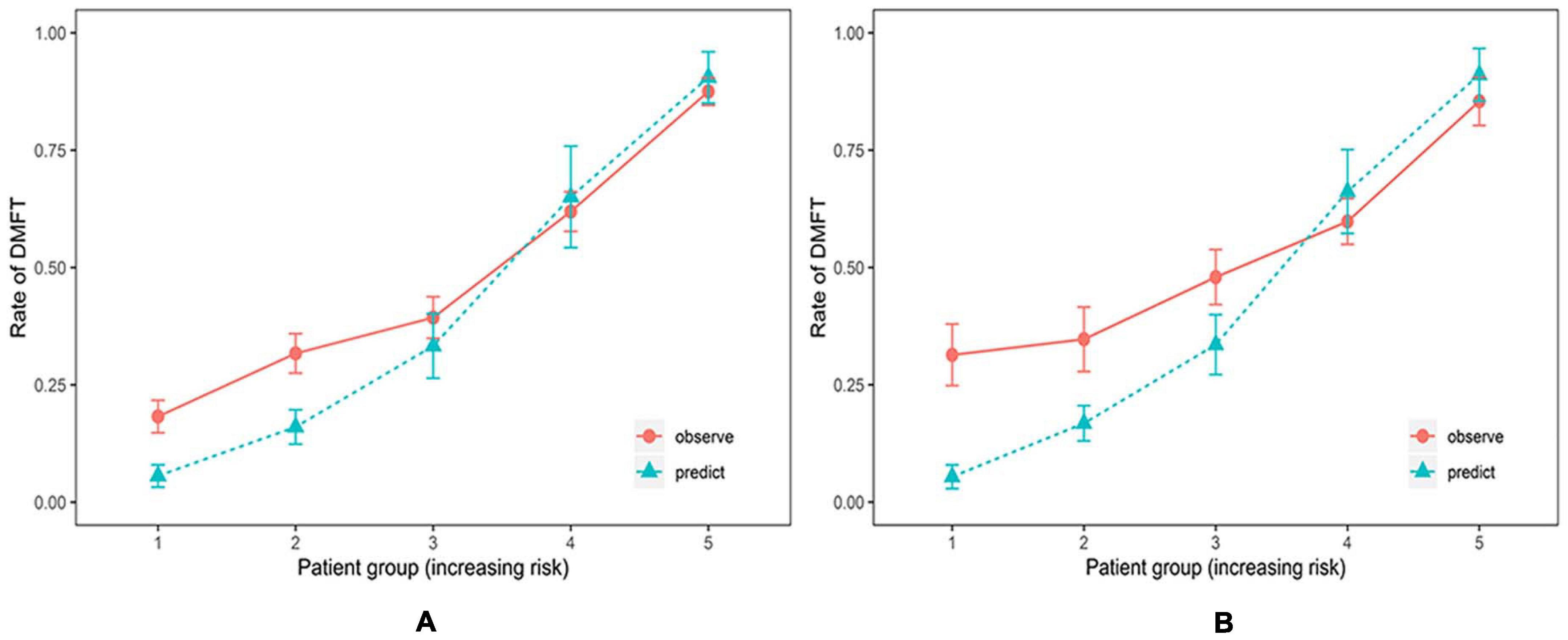
Figure 5. Risk stratification plot for the training and testing cohort (Random Forest Model). Relationship between observed (orange, 95% confidence intervals) and predicted (green) scores of new carious lesions for 21 months for the training cohort (A) and the testing cohort (B). The prediction model could accurately estimate risk for individuals at high and very high caries risk but underestimated risks for individuals at low and very low caries risk.
Using the CRPM constructed with the training cohort, we assigned the participants in cohort 1 into five risk groups based on the 5-quantiles of the predicted incidence probabilities as follows: very low, low, medium, high, and very high. The predicted incidence rates of caries after 21 months in cohort 1 for each risk layer were 5.60, 16.02, 33.29, 65.06, and 90.51%, respectively, and the actual incidence rates of caries after 21 months in cohort 1 for each risk layer were 18.25, 31. 71, 39. 34, 61. 94, and 87.50%, respectively (Table 4). The numbers of individuals in the caries layers of cohort 2, i.e., very low, low, medium, high, and very high, were 48,49,73,102, and 48, respectively, and the mean DMFT increment in each risk layer are shown in Table 5; the predicted incidence rates of caries after 21 months in each risk layer of cohort 2 were 5.41, 16.79, 33.56, 66.20, and 91.07%, respectively, and the actual incidence rates of caries after 21 months in each risk layer of cohort 2 were 27.08, 34.69, 47.95, 59.80, and 85.42%, respectively (Table 5). The risk of new caries was consistently reduced from the extremely high-risk category to the extremely low-risk category, reflecting the ability of our newly constructed CRPM to estimate future caries accurately.

Table 4. Actual number of new caries after 21 months: actual and predicted caries incidences in cohort 1.

Table 5. Actual number of new caries after 21 months: actual and predicted caries incidences in cohort 2.
The sensitivity, specificity, positive predictive value, and negative predictive value of cohorts 1 and 2 are displayed in Table 6. The positive predictive value was high (>73%) for those stratified into very high and high caries risk categories. When the “moderate caries risk” and “low caries risk “categories were used as a cutoff level, the negative predictive values were low.
Discussion
In this study, a new caries risk prediction model was constructed, using both environmental risk factors, such as cariostate score, plaque index, and past caries experience, and genetic factors as predictors. To our knowledge, this is the first CRPM constructed with both environmental and genetic factors, using machine learning algorithms. We further verified the accuracy of this prediction model using another independent cohort, and the results demonstrated that this CRPM could effectively identify high caries-risk individuals.
It is well recognized that dental caries is a multifactorial disease. Environmental and genetic factors play important roles in the occurrence and development of caries (Yildiz et al., 2016). Combining genetic factors with environmental factors to explain the incidence of caries is both reasonable and necessary. Being a polygenetic disease, caries is difficult to predict based on a single SNP or SNPs of individual genes. Hence, it is necessary to select SNPs from different candidate genes. In this study, SNPs were selected based on the results of previous studies, combining tag SNP screening via related-pathway strategies and candidate gene approach (Opal et al., 2015). Finally, 23 SNPs from 16 candidate genes were included in this study. After analyzing the correlation of each SNP, two SNPs were found to be associated with caries in the Chinese population.
The SNPs included in the final CRPM described here were rs3790506 and rs1996315. Of these, rs3790506 is an SNP of TUFT1, which is involved in enamel development and mineralization. Previous studies have reported a relationship between TUFT1 and caries incidence in both children and adults. Slayton et al. suggested that rs3790506 in TUFT1 interacts with the Streptococcus mutans present in the oral cavity and further explained over a quarter of the factors affecting the variability of caries conditions in teenagers from Iowa, United States (Slayton et al., 2005). rs1996315 is a SNP of AQP5, which encodes a water channel protein expressed in lacrimal and salivary glands and epithelial cells. Aquaporins play a role in the generation of tears, saliva, and pulmonary secretions. AQP5 protein also plays an important role in extracellular matrix hydration during tooth development (Felszeghy et al., 2004). It has been reported that variations in AQP5 could contribute to the occurrence and development of caries (Wang et al., 2012; Anjomshoaa et al., 2015). Our previous study showed that gene-gene interaction between rs1996315 and rs923911 was significantly associated with molar-incisor hypomineralization (Pang et al., 2020). Both SNPs included in the CRPM constructed in this study were associated with enamel development. The etiological theory of dental caries states that enamel characteristics also affect the pathogenesis of dental caries, although it is not feasible to detect the physical and chemical characteristics of enamel in vivo. The identification of variations in enamel-related genes can indirectly reflect enamel characteristics associated with the occurrence of dental caries. Although genetic factors were included in this CRPM, it should be noted that environmental factors were more dominant than genetic factors. Silva et al. revealed that, compared to environmental factors, genetic factors have relatively little influence on the risk of dental caries, which is consistent with the results of our study (Silva et al., 2019).
In accordance with the results of traditional CRPMs, such as the Cariogram model, the CRPM constructed in this study using a machine learning algorithm identified “past caries experience” as the strongest predictor of individual risk. Besides the “past caries experience,” “cariostate score,” “plaque index,” “gender,” and “whether they were only teenagers in the family” were also included in this new CRPM. Unlike the Cariogram model, we used the “cariostate score” instead of “bacterial counts” to evaluate the cariogenic ability of the dental plaque. Cariostat uses a colorimetric test to evaluate the acid produced by bacteria in the plaque (Ramesh et al., 2013). The occurrence of carious lesions is a dynamic process in which acids produced by bacteria impact the demineralization of dental tissues (Richards et al., 2017). When the pH of the tooth surface decreases to a level < 5.5, the hydroxyapatite (HA) matrix of the tooth starts to demineralize; Cariostat can assess the activity of the caries microbiology. Unlike other cariogenic microbiology tests, such as Dentocult SM, Cariostat assesses bacteria in plaque instead of saliva, leading to higher accuracy because cariogenic bacteria act on tooth surfaces in the form of plaque.
An ideal but possibly unrealistic model will correctly distinguish individuals at risk of a caries event from those who are not at risk, without any instance of misdiagnosis (Alba et al., 2017). The extent to which a model can achieve this goal is represented by two related properties of discrimination and calibration (Alba et al., 2017). Discrimination refers to the extent to which the model distinguishes between high-risk and low-risk participants of an event, usually described by the receiver operating characteristic (ROC) curve. It is well recognized that an AUC < 0.6 represents poor discrimination, while an AUC ≥ 0.7 indicates high discrimination ability (Fontana et al., 2020). The training set resulted in an AUC of 0.78 in cohort 1 and 0.73 in cohort 2, indicating high discrimination ability.
Discrimination alone is not sufficient to evaluate the performance of a prediction model. The second essential characteristic of a prediction model is demonstrating the similarity of the predicted absolute risk to the absolute observed risk at different risk levels. Calibration is usually considered the most important characteristic of a prediction model because it reflects the extent to which a model correctly predicts the absolute risk (Alba et al., 2017). In terms of accurate estimation, the model is well-calibrated. The relationship between predicted and observed risk could be visually represented, allowing efficient evaluation of the calibration (Alba et al., 2017). We found that the CRPM constructed in this study can accurately estimate the risks of individuals at high and very high caries risks but underestimates those for individuals at low and very low caries risks. However, this poor calibration may not pose a problem for low-risk individuals because the purpose of this CRPM is to identify teenagers at high risk of developing caries for better prevention and intervention, and the underestimation of patients at lower risk would be rather irrelevant. Hence, our CRPM can be considered a useful tool for selecting high caries risk population in China.
Our study has several limitations. First, although the SNPs were selected based on the results of previous studies on caries susceptibility and through screening of tag SNPs from multiple genes, it cannot be ruled out that some key loci with powerful diagnostic performance were missed. As an infectious disease, caries risk will certainly be affected by microorganisms. Even if we use “cariostate score” to evaluate the cariogenic ability of the dental plaque, the prediction performance might be influenced by microbiome markers. Although the ICDAS system was used to record caries, earlier signs (ICDAS code 1 or 2) of caries were not detected in our study. In addition, despite external verification with an independent cohort, further multicenter research is also highly needed.
In conclusion, we constructed a CRPM based on both environmental and genetic factors using a machine learning algorithm. We also estimated the discrimination and calibration abilities of this CRPM using a separate independent cohort for validation, demonstrating that this CRPM can accurately identify a high caries risk population. Our CRPM included specific patient characteristics, such as SNPs, gender, and whether the participants were the only child of the respective families, to provide an estimate of the absolute risk of a specific caries outcome. Thus, our CRPM can be utilized as a powerful tool at the community level for identifying high caries risk groups, enabling policymakers to plan necessary preventive measures for the future.
Data Availability Statement
The data presented in the study are deposited in the European Variation Archive (EVA) repository, accession number PRJEB43233. The data will first be made available to download here: https://www.ebi.ac.uk/ena/data/view/PRJEB43233.
Ethics Statement
The studies involving human participants were reviewed and approved by the Ethics Committee of the Guanghua School of Stomatology, Sun Yat-sen University (ERC- [2018]01). Written informed consent to participate in this study was provided by the participants’ legal guardian/next of kin.
Author Contributions
LP contributed to conception, design, and drafted the manuscript. KW contributed to data acquisition, analysis, and critically revised manuscript. YT contributed to design and critically revised manuscript. QZ contributed to conception and drafted manuscript. JZ contributed to design and critically revised manuscript. HL contributed to conception, design, and critically revised manuscript. All authors gave final approval and agreed to be accountable for all aspects of the work.
Funding
This research was supported by the National Natural Science Foundation of China (Grant No. 81903345).
Conflict of Interest
The authors declare that the research was conducted in the absence of any commercial or financial relationships that could be construed as a potential conflict of interest.
The reviewer SZ declared a past co-authorship with one of the authors HL to the handling editor.
References
Alba, A. C., Agoritsas, T., Walsh, M., Hanna, S., Iorio, A., Devereaux, P. J., et al. (2017). Discrimination and calibration of clinical prediction models: users’ guides to the medical literature. JAMA 318, 1377–1384. doi: 10.1001/jama.2017.12126
Anjomshoaa, I., Briseño-Ruiz, J., Deeley, K., Poletta, F. A., Mereb, J. C., Leite, A. L., et al. (2015). Aquaporin 5 interacts with fluoride and possibly protects against caries. PLoS One 10:e143068. doi: 10.1371/journal.pone.0143068
Cagetti, M. G., Bonta, G., Cocco, F., Lingstrom, P., Strohmenger, L., and Campus, G. (2018). Are standardized caries risk assessment models effective in assessing actual caries status and future caries increment? A systematic review. BMC Oral Health 18:123. doi: 10.1186/s12903-018-0585-4
Chaffee, B. W., Cheng, J., and Featherstone, J. (2015). Baseline caries risk assessment as a predictor of caries incidence. J. Dent. 43, 518–524. doi: 10.1016/j.jdent.2015.02.013
Domejean, S., Banerjee, A., and Featherstone, J. (2017). Caries risk/susceptibility assessment: its value in minimum intervention oral healthcare. Br. Dent. J. 223, 191–197. doi: 10.1038/sj.bdj.2017.665
Felszeghy, S., Módis, L., Németh, P., Nagy, G., Zelles, T., Agre, P., et al. (2004). Expression of aquaporin isoforms during human and mouse tooth development. Arch. Oral Biol. 49, 247–257. doi: 10.1016/j.archoralbio.2003.09.011
Fontana, M., Carrasco-Labra, A., Spallek, H., Eckert, G., and Katz, B. (2020). Improving caries risk prediction modeling: a call for action. J. Dent. Res. 99, 1215–1220. doi: 10.1177/0022034520934808
Haworth, S., Esberg, A., Lif Holgerson, P., Kuja-Halkola, R., Timpson, N. J., Magnusson, P. K. E., et al. (2020). Heritability of caries scores, trajectories, and disease subtypes. J. Dent. Res. 99, 264–270. doi: 10.1177/0022034519897910
Kaste, L. M., Selwitz, R. H., Oldakowski, R. J., Brunelle, J. A., Winn, D. M., and Brown, L. J. (1996). Coronal caries in the primary and permanent dentition of children and adolescents 1-17 years of age: united states, 1988-1991. J. Dent. Res. 75, 631–641. doi: 10.1177/002203459607502S03
Li, C., Sun, D., Liu, J., Li, M., Zhang, B., Liu, Y., et al. (2019). A prediction model of essential hypertension based on genetic and environmental risk factors in northern han chinese. Int. J. Med. Sci. 16, 793–799. doi: 10.7150/ijms.33967
Loe, H. (1967). The gingival index, the plaque index, and the retention index systems. J. Periodontol. 38, 610–616. doi: 10.1902/jop.1967.38.6.610
National Institutes of Health Consensus Development Conference Statement (2001). Diagnosis and management of dental caries throughout life, March 26-28, 2001. J. Am. Dent. Assoc.132, 1153–1161. doi: 10.14219/jada.archive.2001.0343
Okubo, Y., Nakano, Y., Ochi, H., Onohara, Y., Tokuyama, T., Motoda, C., et al. (2020). Predicting atrial fibrillation using a combination of genetic risk score and clinical risk factors. Heart Rhythm 17, 699–705. doi: 10.1016/j.hrthm.2020.01.006
Opal, S., Garg, S., Jain, J., and Walia, I. (2015). Genetic factors affecting dental caries risk. Aust. Dent. J. 60, 2–11. doi: 10.1111/adj.12262
Pang, L., Li, X., Wang, K., Tao, Y., Cui, T., Xu, Q., et al. (2020). Interactions with the aquaporin 5 gene increase the susceptibility to molar-incisor hypomineralization. Arch. Oral Biol. 111:104637. doi: 10.1016/j.archoralbio.2019.104637
Pang, L., Zhi, Q., Zhuang, P., Yu, L., Tao, Y., and Lin, H. (2017). Variation in enamel formation genes influences enamel demineralization in vitro in a Streptococcus mutans biofilm model. Front. Physiol. 8:851. doi: 10.3389/fphys.2017.00851
Patir, A., Seymen, F., Yildirim, M., Deeley, K., Cooper, M. E., and Marazita, M. L. (2008). Enamel formation genes are associated with high caries experience in Turkish children. Caries Res. 42, 394–400. doi: 10.1159/000154785
Petersson, G., and Twetman, S. (2015). Caries risk assessment in young adults: a 3 year validation of the Cariogram model. BMC Oral Health 27:17. doi: 10.1186/1472-6831-15-17
Pitts, N. B., and Ekstrand, K. R. (2013). International caries detection and assessment system (icdas) and its international caries classification and management system (iccms) – methods for staging of the caries process and enabling dentists to manage caries. Community Dent. Oral Epidemiol. 41, e41–e52. doi: 10.1111/cdoe.12025
Ramesh, K., Kunjappan, S., Ramesh, M., Shankar, S., and Reddy, S. (2013). Comparative evaluation of predictive value of three caries activity tests-snyder, lactobacillus count and cariostat in mixed dentition children with and without caries. J. Pharm. Bioallied Sci. 5, S63–S68. doi: 10.4103/0975-7406.113299
Richards, V. P., Alvarez, A. J., Luce, A. R., Bedenbaugh, M., Mitchell, M. L., Burne, R. A., et al. (2017). Microbiomes of site-specific dental plaques from children with different caries status. Infect. Immun. 85, e00106-17. doi: 10.1128/IAI.00106-17
Righolt, A. J., Jevdjevic, M., Marcenes, W., and Listl, S. (2018). Global-, regional-, and country-level economic impacts of dental diseases in 2015. J. Dent. Res. 97, 501–507. doi: 10.1177/0022034517750572
Selwitz, R. H., Ismail, A. I., and Pitts, N. B. (2007). Dental caries. Lancet 369, 51–59. doi: 10.1016/S0140-6736(07)60031-2
Silva, M. J., Kilpatrick, N. M., Craig, J. M., Manton, D. J., Leong, P., Burgner, D. P., et al. (2019). Genetic and early-life environmental influences on dental caries risk: a twin study. Pediatrics 143:e20183499. doi: 10.1542/peds.2018-3499
Slayton, R. L., Cooper, M. E., and Marazita, M. L. (2005). Tuftelin, mutans streptococci, and dental caries susceptibility. J. Dent. Res. 84, 711–714. doi: 10.1177/154405910508400805
Vieira, A. R., Modesto, A., and Marazita, M. L. (2014). Caries: review of human genetics research. Caries Res. 48, 491–506. doi: 10.1159/000358333
Wang, K., Pang, L., Fan, C., Cui, T., Yu, L., and Lin, H. (2020b). Enamel and dentin caries risk factors of adolescents in the context of the International Caries Detection and Assessment System (ICDAS): a longitudinal study. Front. Pediatr. 8:419. doi: 10.3389/fped.2020.00419
Wang, K., Pang, L., Tao, Y., Li, X., Zhang, J., Cui, T., et al. (2020a). Association of genetic and environmental factors with dental caries among adolescents in south china: a cross-sectional study. Eur. J. Paediatr. Dent. 21, 129–136. doi: 10.23804/ejpd.2020.21.02.07
Wang, X., Willing, M. C., Marazita, M. L., Wendell, S., Warren, J. J., Broffitt, B., et al. (2012). Genetic and environmental factors associated with dental caries in children: the Iowa fluoride study. Caries Res. 46, 177–184. doi: 10.1159/000337282
Keywords: caries, risk prediction model, preventive dentistry, biomarkers, biomedical informatics
Citation: Pang L, Wang K, Tao Y, Zhi Q, Zhang J and Lin H (2021) A New Model for Caries Risk Prediction in Teenagers Using a Machine Learning Algorithm Based on Environmental and Genetic Factors. Front. Genet. 12:636867. doi: 10.3389/fgene.2021.636867
Received: 02 December 2020; Accepted: 19 February 2021;
Published: 11 March 2021.
Edited by:
Lu Zhang, Hong Kong Baptist University, Hong KongReviewed by:
Erika Kuchler, Universidade Positivo, BrazilAlexandre Rezende Vieira, University of Pittsburgh, United States
Shuguo Zheng, Peking University School and Hospital of Stomatology, China
Xingyu Zhang, University of Michigan, United States
Copyright © 2021 Pang, Wang, Tao, Zhi, Zhang and Lin. This is an open-access article distributed under the terms of the Creative Commons Attribution License (CC BY). The use, distribution or reproduction in other forums is permitted, provided the original author(s) and the copyright owner(s) are credited and that the original publication in this journal is cited, in accordance with accepted academic practice. No use, distribution or reproduction is permitted which does not comply with these terms.
*Correspondence: Huancai Lin, linhc@mail.sysu.edu.cn