- Department of Radiology, Shengjing Hospital of China Medical University, Shenyang, China
N6-methyladenosine [m(6)A/m6A] methylation is one of the most common RNA modifications in eukaryotic cell mRNA and plays an important regulatory role in mRNA metabolism, splicing, translocation, stability, and translation. Previous studies have demonstrated that the m6A modification is highly associated with tumor cell proliferation, migration, and invasion. In the present study, five m6A regulatory factors have been revealed, namely heterogeneous nuclear ribonucleoprotein A2/B1(HNRNPA2B1), heterogeneous nuclear ribonucleoprotein C (HNRNPC), Vir like m6A methyltransferase associated protein (KIAA1429/VIRMA), RNA binding motif protein 15 (RBM15) and methyltransferase like 3 (METTL3), which are closely related to the overall survival (OS) of patients with lung adenocarcinoma (LUAD). These five m6A regulatory factors exhibited potential prognostic value for the 1, 3, and 5-years survival outcomes of LUAD patients. Our findings revealed that several signaling pathways, such as cell cycle, DNA replication, RNA degradation, RNA polymerase, nucleotide excision repair and basal transcription factors, are activated in the high-risk group of LUAD patients.
Introduction
Lung cancer is currently one of the most common malignant tumors presenting the highest fatality rate among all malignancies (Siegel et al., 2020). In opposite to the surgical resection of early lung cancer, advanced lung cancer is mainly treated with radiotherapy and/or chemotherapy, while adjuvant immunotherapy and targeted therapy are also administered (Hirsch et al., 2017). Most patients have already advanced lung cancer by the time of their diagnosis mainly due to the limited knowledge in the pathogenesis of lung cancer, and the 5-year survival rate does not exceed 20% (Siegel et al., 2020). The clinical prognosis of patients is mainly based on tumor stage and other clinical indicators such as tumor node metastasis (TNM) stage. However, huge variation is observed in the final prognosis of the same tumor stage as a result of patients' heterogeneity. Therefore, relying on simple tumor staging may lead to poor prognosis accuracy, greatly affecting patients' further treatment and reducing the overall survival rate (OSR) (Razzouk, 2014; Perakis et al., 2016). The identification of accurate prognostic markers can contribute to the improvement of the treatment of lung cancer patients.
N6-methyladenosine (m6A) refers to the N6 terminal methylation of adenosine, which is a ubiquitous post-transcriptional modification mechanism of RNA in eukaryotic cells (Chen et al., 2019). m6A is involved in the RNA metabolism, and more specifically in mRNA translation, degradation, splicing, export, and folding (Liu et al., 2017; Chen et al., 2019; Liu and Gregory, 2019). The completion of m6A modification requires the binding of methyltransferase with the conservative motif RRACH (R=A/G, H=U/A/C) in RNA (Kane and Beemon, 1985; Narayan et al., 1994; Balacco and Soller, 2019). m6A often occurs in the stop codons of the 3′ untranslated (3′UTRs) and exon regions, respectively (Dominissini et al., 2012; Meyer et al., 2012). m6A modification is usually a reversible process that is regulated by various related factors (Jia et al., 2011, 2013). The m6A regulators reported so far can be divided into three types. The first type is called Writers including METTL3, METTL14, METTL16, WTAP, KIAA1429, RBM15, and ZC3H13, which are able to recognize RNA and modify m6A (Dai et al., 2018; Balacco and Soller, 2019). The second type is Erasers that include fat mass- and obesity-associated protein (FTO) and alk B homolog 5 (ALKBH5). These regulators are mainly responsible for removing m6A modifications (Liu et al., 2018; Pan et al., 2018). The third type is Readers that consist of YTHDF1, YTHDF2, YTHDF3, YTHDC1, YTHDC2, HNRNPC, and HNRNPA2B1. Readers can recognize RNA methylation modifications and further regulate RNA processing, translation, and degradation (Wang et al., 2018; Ma et al., 2019).
Functional analysis has shown that m6A is crucial for cell proliferation, cell self-renewal, and apoptosis as it affects many important life processes (Zhou et al., 2019). A large number of studies have confirmed that the aberrant m6A modification plays a key role in the occurrence and progression of various tumors including LUAD (Zhou et al., 2019; Yi et al., 2020; Zhang et al., 2020). For instance, m6A Reader YTHDF2 can promote the non-small cell lung cancer (NSCLC) progression (Sheng et al., 2019), while the Eraser ALKBH5 can inhibit the metastasis of NSCLC by inhibiting the miR-107/LATS2-mediated YAP activity (Jin et al., 2020). In addition, m6A status can also affect the sensitivity of NSCLC to Afatinib treatment (Meng et al., 2020). However, the potential value of m6A for the prognosis of lung cancer treatment still remains unexplored, especially for the prognosis of LUAD. The present study initially confirmed that the expression levels of five m6A regulators, including HNRNPA2B1, HNRNPC, KIAA1429, RBM15, and METTL3 were correlated with OS of LUAD patients. m6A Writers regulatory factors were also suggested as potential prognostic biomarkers for LUAD.
Materials and Methods
Data Acquisition
The LUAD gene expression data and the corresponding clinical data were downloaded from The Cancer Genome Atlas database (TCGA) (https://cancergenome.nih.gov/) by using TCGA-assembler in February 20201. Gene expression in the downloaded files was normalized using the Fragments Per Kilobase of exon model per Million mapped fragments (FPKM) metric. Data for the m6A regulators including METTL3, METTL14, METTL16, WTAP, KIAA1429, RBM15, ZC3H13, FTO, ALKBH5, YTHDF1, YTHDF2, YTHDF3, YTHDC1, YTHDC2, HNRNPC, and HNRNPA2B1, were retrieved by mining the transcriptomics data of LUAD and para-carcinoma tissues. The human tissue expression levels in Genotype-tissue expression (GTEx) database were downloaded in May 2020. The GTEx dataset contains more than 900 organs and tissues of healthy people, with a total of more than 17,000 samples, covering 54 types of tissues in the human body.
Bioinformatics Analysis
Gene expression data of the tumor and control sample were separately sorted. Gene expression data for the 16 m6A related genes were then extracted and data with incomplete information were deleted. R software (Version 3.6.1) was used to perform differential expression analysis on the m6A regulatory factors in lung tissue samples in a comparison between 497 LUAD tissues (from 467 LUAD patients) and 54 para-carcinoma tissues. Vioplot tool was used to plot violin graphs to visualize the results of the differential expression analysis between LUAD patients and control samples. The Spearman correlation analysis was deployed to study the associations between the expression levels of the 16 m6A-related regulatory factors.
The Least Absolute Shrinkage and Selection Operator (LASSO) model is a dimensionality reduction method, which can reduce the number of variables through a penalty mechanism and ultimately achieve the goal of reducing bias. The LASSO model analysis was performed using the glmnet R package. Gene Set Enrichment Analysis (GSEA) software (Version 4.0.3 from Broad Institute official website homepage) was used to analyze the enrichment analysis between high-risk and low-risk groups. A total of 55,268 genes were included in the analysis. Moreover, the “c2.cp.kegg.V7.0.symbols.gmt” analysis package was used to study the pathway enrichment. All the LUAD samples were divided into two groups (high-risk and low-risk groups) by median risk score. P-value and False Discovery Rate (FDR) thresholds of 0.05 and 0.25, respectively, were used to infer significant findings.
Statistical Analysis
One-way analysis of variance (Anova) test was used to compare the 16 m6A regulatory factors in between 497 LUAD tissues and 54 para-carcinoma tissues. The Spearman correlation was used to clarify the relationship between the m6A gene expression level and the basic clinical information (such as age, gender, TMN stage) of LUAD patients. The OSR is defined as the time period from diagnosis to death. Univariate and multivariate COX logistic regression models were used to analyze the prognostic potential of each factor and their ability to predict the survival outcome of LUAD. Kaplan-Meier and receiver operating characteristic (ROC) curves were plotted to demonstrate the prognostic performance of the explored m6A related regulators. A prognostic model was established by drawing a Nomogram plot, and calibration curves were used to verify it. P-value threshold of 0.05 was used to infer statistical significance.
Results
m6A Regulator mRNA Levels
After screening the mRNA expression levels of the m6A regulators in 497 LUAD and 54 normal control samples, respectively, we analyzed the expressions of 16 m6A related regulators: METTL3, METTL14, METTL16, WTAP, KIAA1429, RBM15, ZC3H13, FTO, ALKBH5, YTHDF1, YTHDF2, YTHDF3, YTHDC1, YTHDC2, HNRNPC and HNRNPA2B1. Among them, METTL3, METTL14, KIAA1429, RBM15, ZC3H13, FTO, YTHDF1, YTHDF2, HNRNPC, HNRNPA2B1, WTAP, YTHDF3 and METTL16, were significantly overexpressed in LUAD tissues. The expression of ALKBH5, YTHDC1, and YTHDC2 presented no statistically significant differences (Figures 1A,B). Moreover, Pearson correlation analysis was conducted between the 16 m6A related regulators. We found that the positive correlation between the expression levels of YTHDF3 and KIAA1429 was the highest one. Furthermore, significant positive correlations were revealed between YTHDC1, YTHDC2, RBM15, and METTL14 from the same analysis (Figure 1C).
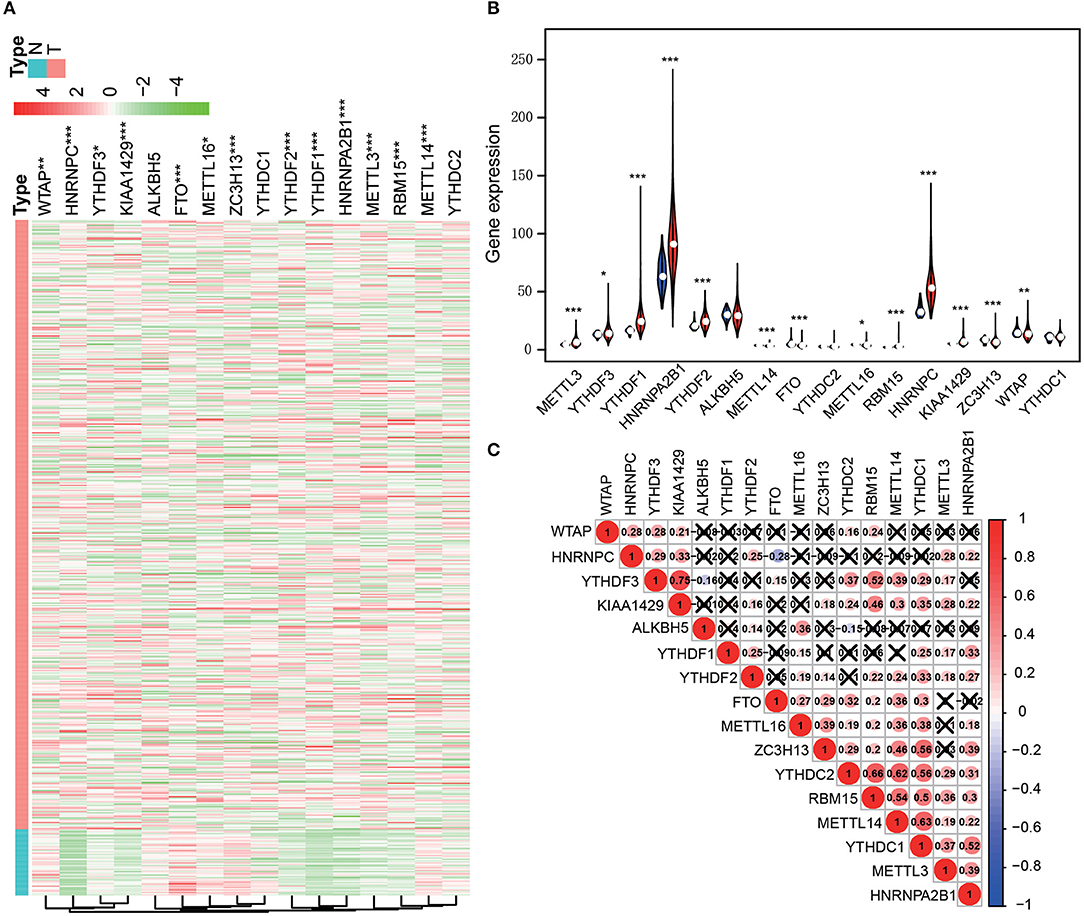
Figure 1. The 16 m6A-related genes were differentially expressed in the comparison of LUAD with normal tissues. (A) Heatmap (***p < 0.001; **p < 0.01; *p < 0.05; Red means high expression and green means low expression). (B) Violet plots (***p < 0.001; **p < 0.01; *p < 0.05; Blue represents normal tissue and red represents cancer tissue). (C) The correlation between the expression levels of each m6A-related gene.
m6A-Related Gene Expression and LUAD Prognosis
Clinical data of the 467 patients were further analyzed to explore the prognostic potential of the expression levels of the m6A regulatory factors in LUAD (Table 1). Survival analysis showed that the expression levels of four regulatory factors (HNRNPA2B1, HNRNPC, KIAA1429, and RBM15) were significantly positively associated with the patient's death risk, while METTL3 was negatively associated with it without reaching statistical significance (0.05<p<0.1) (Figure 2A). Then, a Nomogram prediction model based on the expression levels of the above five genes and patient's outcomes data were established (Figure 2B). Four hundred and twenty cases were randomly selected from all cases and divided into three groups with each one of them having 140 cases. The results of the calibration curves indicated that the Nomogram prediction model presented good predictive potential for 1, 3, and 5-year OS of LUAD patients (Figures 2C–E).
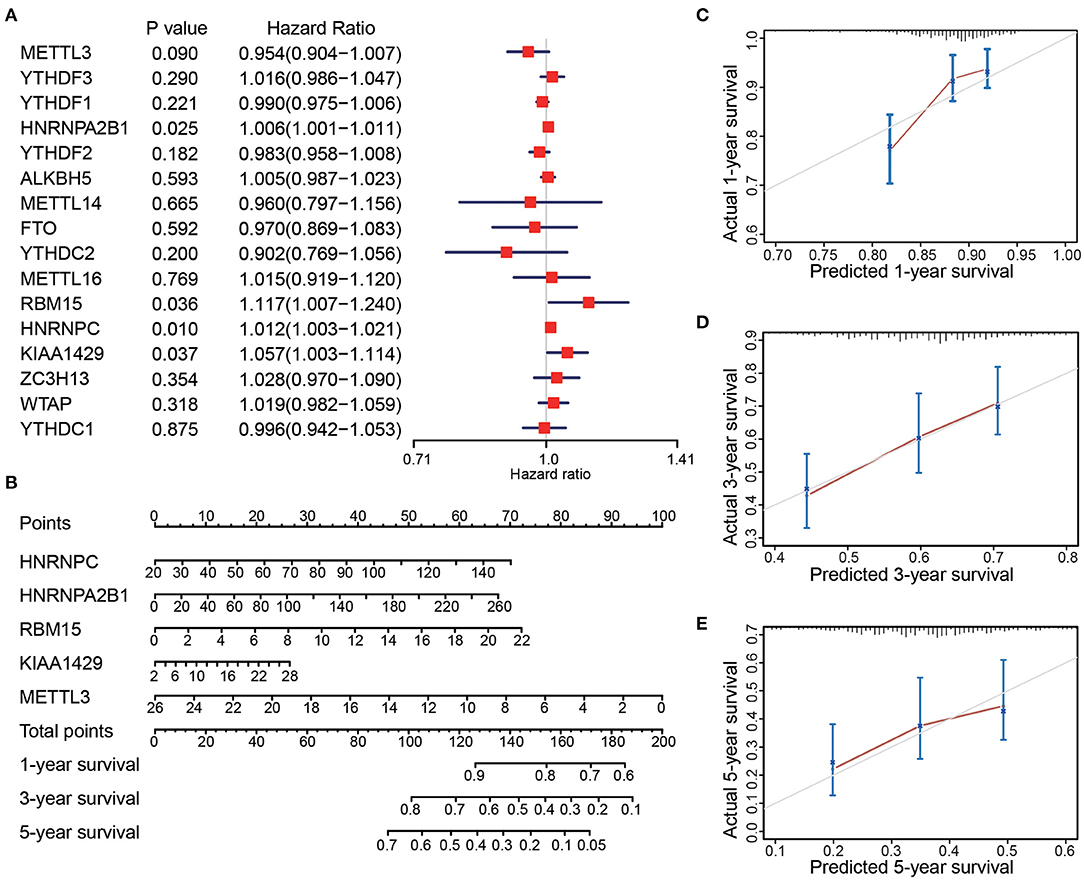
Figure 2. The expression levels of 16 m6A-related genes were correlated with the survival time of LUAD patients. (A) Forest plot depicting Hazard ratios (HR) and 95% intervals of trust of the m6A-related genes. The expression levels of HNRNPA2B1, HNRNPC, KIAA1429, RBM15, and METTL3 were associated to the prognosis of patients. (B) Combining the levels of these five m6A-related genes to establish a Nomogram chart to predict 1, 3, and 5-year survival patients with LUAD. (C–E) Validation curve corresponding to patient 1, 3, and 5-year survival prediction model (Calibrated curve).
LASSO Regression and Risk Co-Efficient
LASSO regression models were used to analyze the risk coefficient and risk value of the expression of the five m6A regulators (HNRNPA2B1, HNRNPC, KIAA1429, RBM15, METTL3) in OS prediction (Figures 3A,B). The patients of the present study were divided into high and low-risk groups based on their predicted risk scores. HNRNPA2B1, HNRNPC, KIAA1429, and RBM15 were found to be overexpressed in the high-risk group, while METTL3 was overexpressed in the low-risk group (Figure 3C). Furthermore, survival analysis was conducted using the combined risk value. Results confirmed that the prognosis of patients in the high-risk group was significantly worse than the one in the low-risk group (P < 0.01) (Figure 3D). ROC curves for the 1, 3, and 5-year survival prediction demonstrated that the risk value possesses high prognostic accuracy with area under the curve (AUC) of 0.60–0.71 (Figures 3E–G).
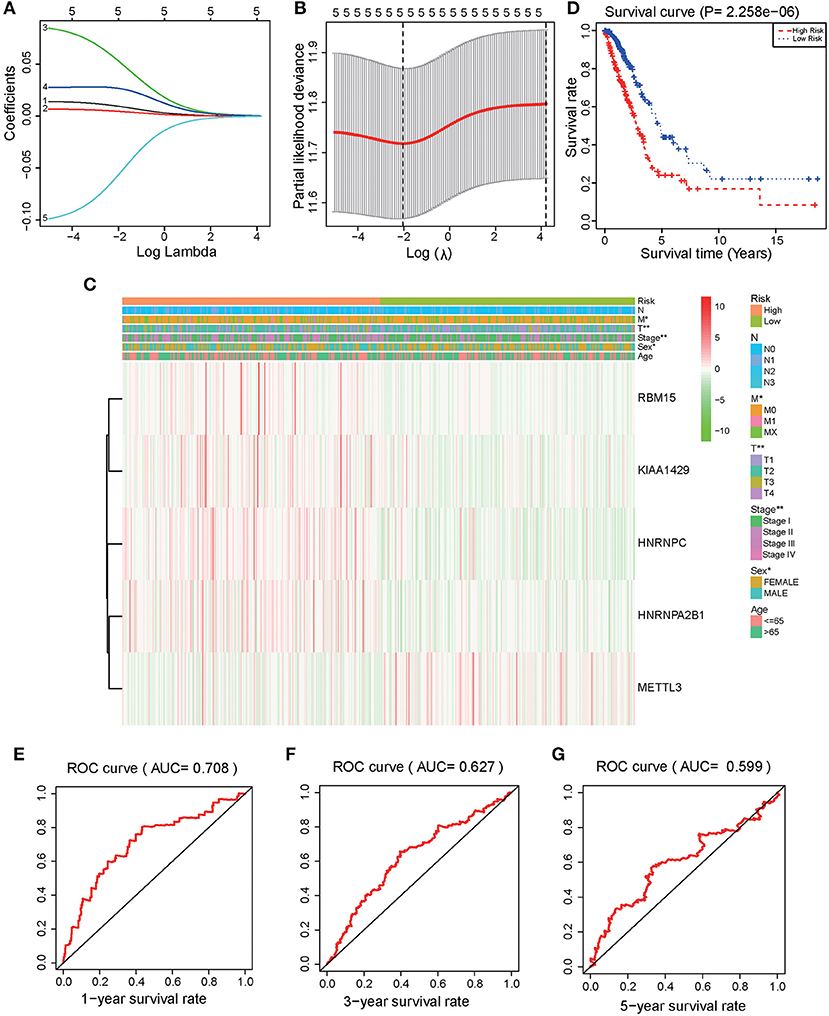
Figure 3. Identification and validation of a risk model to prognose LUAD patients. (A) The LASSO analysis model was verified by repeated calculation of the 16 m6A genes. (B) LASSO coefficient profiles. (C) According to LASSO risk factors, LUAD patients were divided into high-risk group and low-risk group. Heatmap demonstrated the expression levels of HNRNPA2B1, HNRNPC, KIAA1429, RBM15, and METTL3 in the two assessed groups. (D) Kaplan Meier analysis for the survival of LUAD patients. (E) 1-year (P < 0.001), (F) 3-year (P < 0.001), (G) 5-year OS ROC curves (P < 0.001).
KEGG and Multi-Factor Analysis
GSEA software was used to analyze pathway enrichment for the genes that are differentially expressed between high and low-risk patients. The cell pathway was found to be enriched in the set of deregulated factors with a p-value threshold of 0.05 and a FDR threshold of 0.25. The pathway enrichment analysis using KEGG database pathways revealed that Cell cycle (P < 0.001, FDR< 0001, Normalized Enrichment Score (NES)=2.56), DNA replication (P < 0.001, FDR< 0001, NES = 2.17), RNA degradation (P < 0.001, FDR< 0001, NES = 2.49), RNA polymerase (P = 0.006, FDR=0.024, NES=1.85), Nucleotide excision repair (P < 0.001, FDR< 0001, NES=2.29), Basal transcription factors (P < 0.001, FDR < 0001, NES=2.29), and other related signaling pathways are significantly activated in the high-risk group (P < 0.05; FDR <0.25) (Figure 4). Accordingly, a heatmap of the most enriched genes for each identified KEGG pathway by GSEA between high and low-risk groups was presented in Supplementary Figures 1–6 and Supplementary Tables 1–6. Univariate and multivariate COX regression analyses were performed based on existing risk factors and patient clinical information (such as age, gender, and tumor and TMN staging) to evaluate their prognostic potential in LUAD (Table 2). The results of univariate analysis suggested that the stage (P < 0.001), T stage (P < 0.001), lymph node metastasis stage (P < 0.001) and risk score (P < 0.001) of LUAD patients are significantly negatively associated with the patient's OS. The results of multivariate analysis suggested that the tumor stage (P = 0.015), lymph node metastasis stage (P = 0.022) and risk score (P < 0.001) are significantly negatively associated with the patient's OS.
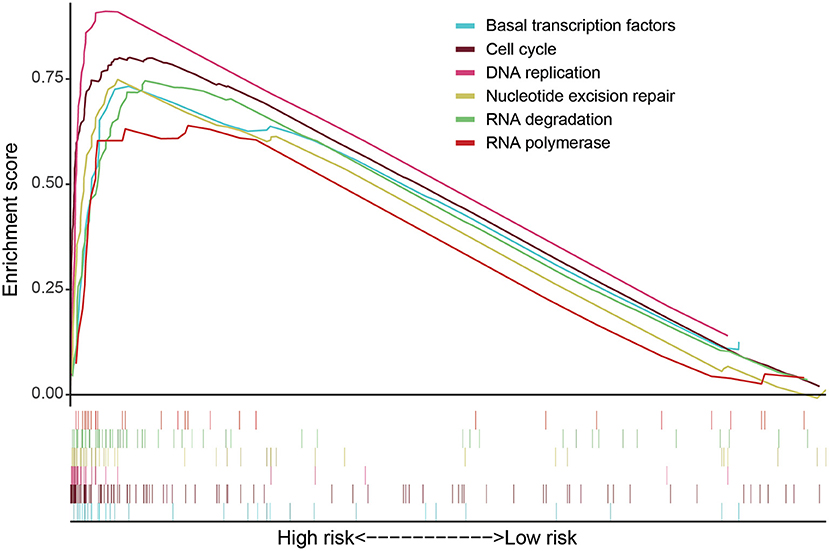
Figure 4. GSEA software was used to analyze the expression differences of internal signal pathways between the two subgroups, including Cell cycle (P < 0.001, FDR < 0.001, NES = 2.56), DNA replication (P < 0.001, FDR < 0.001, NES = 2.17), RNA degradation (P < 0.001, FDR<0.001, NES = 2.49), RNA polymerase (P = 0.006, FDR = 0.024, NES = 1.85), Nucleotide excision repair (P < 0.001, FDR < 0.001, NES = 2.29), and Basal transcription factors (P < 0.001, FDR < 0.001, NES = 2.29) as well as other related signaling pathways (P < 0.05, FDR < 0.25).
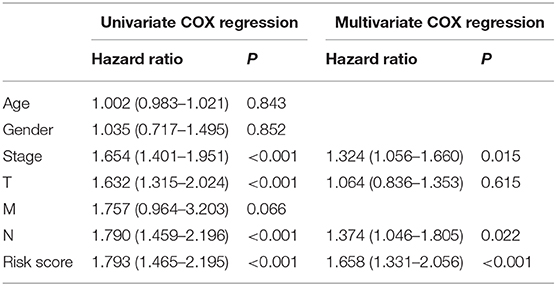
Table 2. Univariate and multivariate COX regression analyses were used to assess the association between the clinical data and risk score of LUAD patients and the prognosis.
A Nomogram prognostic analysis model was established based on clinical data such as age, gender, stage, lymph node metastasis and risk value (Figure 5A). The analysis of the 1, 3 and 5-year OS of patients through the Nomogram prediction model has demonstrated that the risk value contributes most to the prediction model followed by lymph node metastasis, age and tumor stage. Three hundred cases were randomly selected from all cases and were then divided into three groups with each one of them having 100 cases. Calibration curves showed that the established Nomogram prediction model presented good predictive potential for the 1, 3, and 5-year OS of LUAD patients (Figures 5B–D).
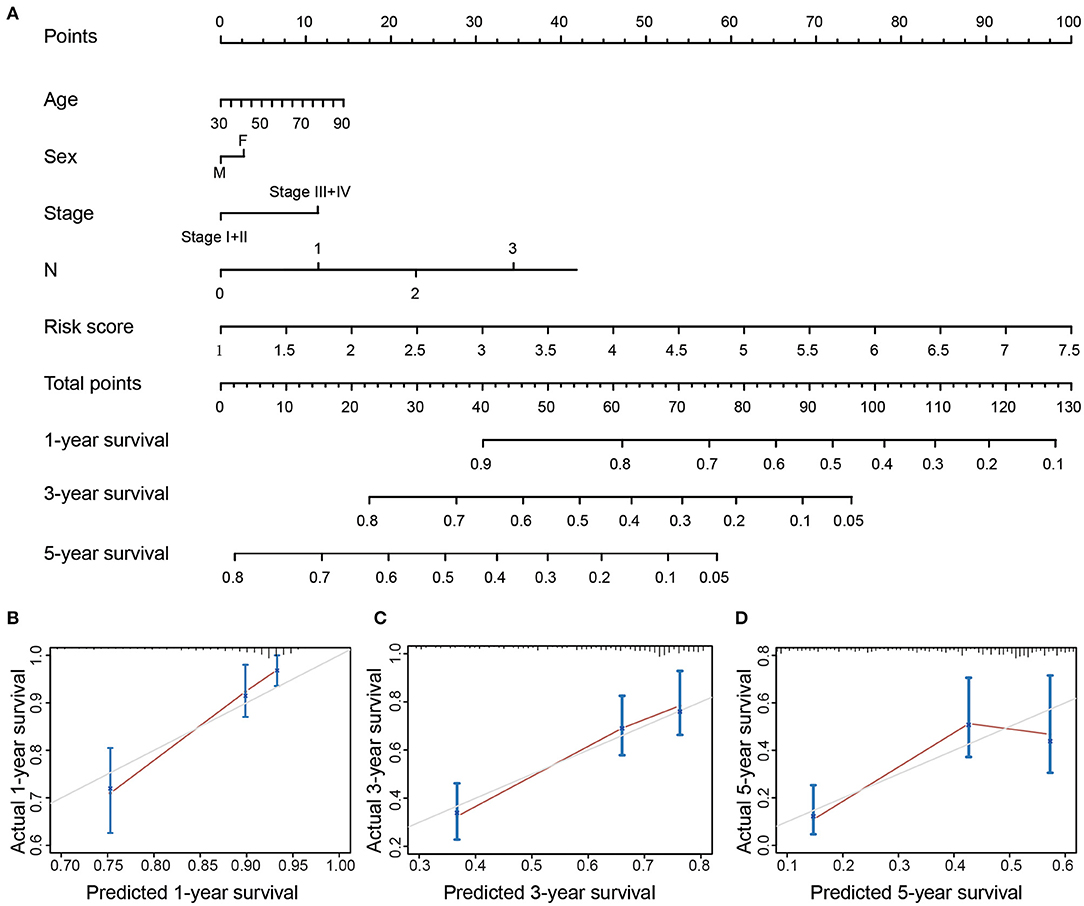
Figure 5. (A) Nomogram of OS in LUAD patients. (B–D) Corresponding 1/3/5-year survival time verification curve (Calibrated curve).
m6A Writers in Normal Human Organ Tissues
METTL3, RBM15, and KIAA1429 have been grouped to the m6A Writers type (Wang et al., 2018; Chen et al., 2019; Chen and Wong, 2020). LASSO regression analysis showed that the m6A Writers, METTL3, RBM15 and KIAA1429, present higher weight co-efficients (Co-ef) (METTL3: −0.0576562669796008; KIAA1429: 0.0269410278179687; RBM15: 0.0539704827385957) than those of HNRNPA2B1 (0.00473147643475602) and HNRNPC (0.00964499370244368). The expression levels of three genes (METTL3, RBM15, and KIAA1429) in 54 human normal organ tissues were compared and analyzed using the GTEx database. No significant difference was found in the comparison between the expression levels of METTL3 and KIAA1429 in various tissues of the human body (Figures 6A,B), while RBM15 was overexpressed in bone marrow and testis compared to other organs in the human body (Figure 6C).
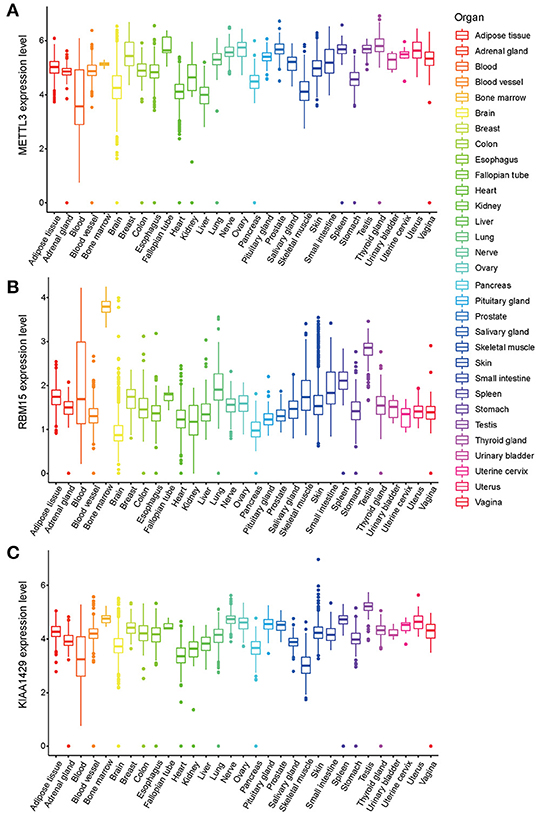
Figure 6. The expression levels of three m6A-related genes METTL3 (A), RBM15 (B), and KIAA1429 (C) in various organs in the human body.
Discussion
The present study has discussed the m6A regulatory factor-related genes are associated to the overall prognosis of LUAD patients, and the newly introduced prognostic model is proven to accurately prognose the outcomes of LUAD patients. Meanwhile, the m6A regulatory factor-related genes are associated with the occurrence and development of LUAD.
m6A is one of the most common RNA modifications discovered so far. Accumulating studies have recently shown that the deregulation of the m6A RNA modification plays an important role in the occurrence and progression of tumors (Cui et al., 2017; Yang et al., 2017; Dai et al., 2018). For instance, the overexpression of FTO in acute myeloid leukemia (AML) can inhibit the m6A levels of Ankyrin repeat and SOCS box containing 2 (ASB2) and of retinoic acid receptor α (RARα) mRNA, resulting in the occurrence and progression of AML (Li et al., 2017). The low expression of m6A regulators METTL14 in HCC (Ma et al., 2017) and the overexpression of the m6A regulator ALKBH5 in glioblastoma (Zhang et al., 2017) have been both associated with poor prognosis. Thus, the abnormal modification of m6A is closely related to tumor progress, metastasis and survival prognosis.
The expression levels of a variety of genes involved in RNA methylation mechanisms are closely related to the prognosis of lung cancer patients (Sun et al., 2020). m6A methylation is an important RNA modification that occurs in various RNA types such as microRNAs (miRs), circRNAs, and lncRNAs. At the same time, a large number of studies have confirmed that m6A and tumor progression are strongly correlated (Ma et al., 2019). The levels of m6A-related genes are also tightly associated with the prognosis of lung cancer patients. For instance, Liu and colleagues have confirmed that the expression level of m6A is weakly correlated with the prognosis of patients with lung squamous cell carcinoma, while it has a strong correlation with the prognosis of patients with LUAD (Liu et al., 2020). A most recent report from zhuang et al. have reported that the differences in the expression of m6A regulators not only have certain diagnostic significance for early lung cancer, but are also closely related to the prognosis of LUAD patients (Zhuang et al., 2020).
In the present study, we have further expanded the number of m6A regulatory factors and explored their gene expression levels are related to the prognosis of patients with LUAD. Five m6A modification regulators, namely HNRNPA2B1, HNRNPC, KIAA1429, RBM15, and METTL3 are found to be closely related to the prognosis of LUAD patients. LASSO analysis reveals that the outcomes of patients in the high-risk group are significantly worse than the ones in the low-risk group. Both univariate and multivariate analyses conclude that the risk value, stage, and lymph node metastasis are closely related to the patient's prognosis. Finally, a newly developed Nomogram model is able to improve the accuracy of the prognosis of patients compared to conventional risk factors.
The m6A writers, METTL3, RBM15, and KIAA1429, were found to be linked with a higher risk in the LASSO regression analysis. Therefore, the abnormal expression of m6A writer regulatory factors may affect the prognosis of LUAD. Among them, METTL3 belongs to the class I methyltransferases family and is a predominantly catalytic enzyme in m6A modification. METTL3 has been confirmed to be abnormally expressed in a variety of tumors and is believed to be involved in carcinogenesis (Zheng et al., 2019). Prior studies have validated that METTL3 is overexpressed in lung cancer. METTL3 can interact with the transcription factor eIF3h to promote the translation of oncogenes and ultimately to catalyze and accelerate tumor growth and metastasis (Lin et al., 2016; Choe et al., 2018). METTL3 can promote the splicing of the miR-143-3p precursor, which in turn activates the miR-143-3p/VASH1 axis and ultimately leads to the progression and metastasis of lung cancer (Wang et al., 2019). METTL3 has also been shown to increase the expression level of JUNB and promote the occurrence of epithelial-mesenchymal transition (Wanna-Udom et al., 2020). miR-600 (Wei et al., 2019) and miR-33a (Du et al., 2017) is able to inhibit the expression of METTL3, thereby ablating the progression of NSCLC. Thus, METTL3 plays an important role in the occurrence and development of lung cancer. One of the main contributions of the present study is the validation of the hypothesis that the overexpression of METTL3 is negatively correlated with the prognosis of LUAD. This is consistent with the results of previous studies suggesting that the overexpression of METTL3 often indicates poor prognosis in patients with primary liver cancer (Chen et al., 2018).
m6A modification regulatory RBM15 is a member of the split end protein (SPEN) family and can bind with METTL3 and WTAP (Wang et al., 2020). Studies have confirmed that m6A plays an important regulatory role in the lncRNA XIST-mediated gene transcription silencing. RBM15 catalyzes the recognition of the m6A site on lncRNA XIST by METTL3. This m6A methylation process can be blocked by the inhibition of RBM15 (Patil et al., 2016). Recent studies have reported that RBM15 assists ZC3H13 in regulating m6A methylation, which is essential for speeding up the progress of glioblastoma multiforme (GBM) (Chow et al., 2017; Knuckles et al., 2018). It has been confirmed that RBM15 can regulate the differentiation of megakaryocytes by modulating the alternative splicing of RNA (Jin et al., 2018). Our results have showed that the overexpression of RBM15 may be related to the prognosis of LUAD by affecting m6A.
KIAA1429 can upregulate c-Jun mRNA via m6A by increasing its stability and by promoting the proliferation of gastric cancer cells (Miao et al., 2020). KIAA1429 increases the expression of cyclin-dependent kinase 1 (CDK1) mRNA to increase the invasion ability of breast cancer cells (Qian et al., 2019). KIAA1429 has been shown to regulate the m6A modification of GATA3 precursor mRNA (Lan et al., 2019) and ID2 mRNA (Cheng et al., 2019) in HCC, thereby promoting the progression and metastasis of HCC. In the present study, we have revealed that the expression level of m6A Writer KIAA1429 may act as a prognostic marker for LUAD patients.
In conclusion, our findings provide bioinformatics evidence to trigger and support further research on the important role of m6A in LUAD. Toward this direction, the validation of the molecular mechanism of m6A underlying LUAD occurrence and its association with LUAD prognosis can be further explored by mechanistic experiments with animal models and/or cancer cell lines.
Data Availability Statement
Requests to access the datasets should be directed to Xiangxuan Zhao, xiangxuanzhao@163.com.
Author Contributions
XZ and ZL: study design. HW and XZ: data collection. HW, XZ, and ZL: data analysis. XZ and HW: manuscript preparation. All authors read and approved the final manuscript.
Funding
This work was partially supported by National Natural Science Foundation of China [81771947] to ZL and [31371425] to XZ; Liaoning Provincial Natural Science Foundation of China [20180551061] to XZ.
Conflict of Interest
The authors declare that the research was conducted in the absence of any commercial or financial relationships that could be construed as a potential conflict of interest.
Supplementary Material
The Supplementary Material for this article can be found online at: https://www.frontiersin.org/articles/10.3389/fgene.2021.622233/full#supplementary-material
Footnotes
1. ^The cancer genome atlas (TCGA) (2020). https://portal.gdc.cancer.gov/
References
Balacco, D. L., and Soller, M. (2019). The m(6)a writer: rise of a machine for growing tasks. Biochemistry 58, 363–378. doi: 10.1021/acs.biochem.8b01166
Chen, M., Wei, L., Law, C. T., Tsang, F. H., Shen, J., Cheng, C. L., et al. (2018). RNA N6-methyladenosine methyltransferase-like 3 promotes liver cancer progression through YTHDF2-dependent posttranscriptional silencing of SOCS2. Hepatology 67, 2254–2270. doi: 10.1002/hep.29683
Chen, M., and Wong, C. M. (2020). The emerging roles of N6-methyladenosine (m6A) deregulation in liver carcinogenesis. Mol. Cancer 19:44. doi: 10.1186/s12943-020-01172-y
Chen, X. Y., Zhang, J., and Zhu, J. S. (2019). The role of m(6)A RNA methylation in human cancer. Mol. Cancer 18:103. doi: 10.1186/s12943-019-1033-z
Cheng, X., Li, M., Rao, X., Zhang, W., Li, X., Wang, L., et al. (2019). KIAA1429 regulates the migration and invasion of hepatocellular carcinoma by altering m6A modification of ID2 mRNA. Onco. Targets Ther. 12, 3421–3428. doi: 10.2147/OTT.S180954
Choe, J., Lin, S., Zhang, W., Liu, Q., Wang, L., Ramirez-Moya, J., et al. (2018). mRNA circularization by METTL3-eIF3h enhances translation and promotes oncogenesis. Nature 561, 556–560. doi: 10.1038/s41586-018-0538-8
Chow, R. D., Guzman, C. D., Wang, G., Schmidt, F., Youngblood, M. W., Ye, L., et al. (2017). AAV-mediated direct in vivo CRISPR screen identifies functional suppressors in glioblastoma. Nat. Neurosci. 20, 1329–1341. doi: 10.1038/nn.4620
Cui, Q., Shi, H., Ye, P., Li, L., Qu, Q., Sun, G., et al. (2017). m(6)A RNA methylation regulates the self-renewal and tumorigenesis of glioblastoma stem cells. Cell Rep. 18, 2622–2634. doi: 10.1016/j.celrep.2017.02.059
Dai, D., Wang, H., Zhu, L., Jin, H., and Wang, X. (2018). N6-methyladenosine links RNA metabolism to cancer progression. Cell Death Dis. 9:124. doi: 10.1038/s41419-017-0129-x
Dominissini, D., Moshitch-Moshkovitz, S., Schwartz, S., Salmon-Divon, M., Ungar, L., Osenberg, S., et al. (2012). Topology of the human and mouse m6A RNA methylomes revealed by m6A-seq. Nature 485, 201–206. doi: 10.1038/nature11112
Du, M., Zhang, Y., Mao, Y., Mou, J., Zhao, J., Xue, Q., et al. (2017). MiR-33a suppresses proliferation of NSCLC cells via targeting METTL3 mRNA. Biochem. Biophys. Res. Commun. 482, 582–589. doi: 10.1016/j.bbrc.2016.11.077
Hirsch, F. R., Scagliotti, G. V., Mulshine, J. L., Kwon, R., Curran, W. J. Jr., Wu, Y. L., et al. (2017). Lung cancer: current therapies and new targeted treatments. Lancet 389, 299–311. doi: 10.1016/S0140-6736(16)30958-8
Jia, G., Fu, Y., and He, C. (2013). Reversible RNA adenosine methylation in biological regulation. Trends Genet. 29, 108–115. doi: 10.1016/j.tig.2012.11.003
Jia, G., Fu, Y., Zhao, X., Dai, Q., Zheng, G., Yang, Y., et al. (2011). N6-methyladenosine in nuclear RNA is a major substrate of the obesity-associated FTO. Nat. Chem. Biol. 7, 885–887. doi: 10.1038/nchembio.687
Jin, D., Guo, J., Wu, Y., Yang, L., Wang, X., Du, J., et al. (2020). m(6)A demethylase ALKBH5 inhibits tumor growth and metastasis by reducing YTHDFs-mediated YAP expression and inhibiting miR-107/LATS2-mediated YAP activity in NSCLC. Mol. Cancer 19:40. doi: 10.1186/s12943-020-01161-1
Jin, S., Mi, Y., Song, J., Zhang, P., and Liu, Y. (2018). PRMT1-RBM15 axis regulates megakaryocytic differentiation of human umbilical cord blood CD34+ cells. Exp. Therapeut. Med. 15:2563–2568. doi: 10.3892/etm.2018.5693
Kane, S. E., and Beemon, K. (1985). Precise localization of m6A in Rous sarcoma virus RNA reveals clustering of methylation sites: implications for RNA processing. Mol. Cell Biol. 5, 2298–2306. doi: 10.1128/MCB.5.9.2298
Knuckles, P., Lence, T., Haussmann, I. U., Jacob, D., Kreim, N., Carl, S. H., et al. (2018). Zc3h13/Flacc is required for adenosine methylation by bridging the mRNA-binding factor Rbm15/Spenito to the m6A machinery component Wtap/Fl(2)d. Genes Dev. 32, 415–429. doi: 10.1101/gad.309146.117
Lan, T., Li, H., Zhang, D., Xu, L., Liu, H., Hao, X., et al. (2019). KIAA1429 contributes to liver cancer progression through N6-methyladenosine-dependent post-transcriptional modification of GATA3. Mol. Cancer 18:186. doi: 10.1186/s12943-019-1106-z
Li, Z., Weng, H., Su, R., Weng, X., Zuo, Z., Li, C., et al. (2017). FTO plays an oncogenic role in acute myeloid leukemia as a N(6)-methyladenosine RNA demethylase. Cancer Cell 31, 127–141. doi: 10.1016/j.ccell.2016.11.017
Lin, S., Choe, J., Du, P., Triboulet, R., and Gregory, R. I. (2016). The m(6)A methyltransferase METTL3 promotes translation in human cancer cells. Mol. Cell 62, 335–345. doi: 10.1016/j.molcel.2016.03.021
Liu, N., Zhou, K. I., Parisien, M., Dai, Q., Diatchenko, L., and Pan, T. (2017). N6-methyladenosine alters RNA structure to regulate binding of a low-complexity protein. Nucleic Acids Res. 45, 6051–6063. doi: 10.1093/nar/gkx141
Liu, Q., and Gregory, R. I. (2019). RNAmod: an integrated system for the annotation of mRNA modifications. Nucleic Acids Res. 47, W548–W555. doi: 10.1093/nar/gkz479
Liu, Y., Guo, X., Zhao, M., Ao, H., Leng, X., Liu, M., et al. (2020). Contributions and prognostic values of m(6) A RNA methylation regulators in non-small-cell lung cancer. J. Cell Physiol. 235, 6043–6057. doi: 10.1002/jcp.29531
Liu, Z.-X., Li, L.-M., Sun, H.-L., and Liu, S.-M. (2018). Link Between m6A modification and cancers. Front. Bioeng. Biotechnol. 6:89. doi: 10.3389/fbioe.2018.00089
Ma, J. Z., Yang, F., Zhou, C. C., Liu, F., Yuan, J. H., Wang, F., et al. (2017). METTL14 suppresses the metastatic potential of hepatocellular carcinoma by modulating N(6) -methyladenosine-dependent primary MicroRNA processing. Hepatology 65, 529–543. doi: 10.1002/hep.28885
Ma, S., Chen, C., Ji, X., Liu, J., Zhou, Q., Wang, G., et al. (2019). The interplay between m6A RNA methylation and noncoding RNA in cancer. J. Hematol. Oncol. 12:121. doi: 10.1186/s13045-019-0805-7
Meng, Q., Wang, S., Zhou, S., Liu, H., Ma, X., Zhou, X., et al. (2020). Dissecting the m(6)A methylation affection on afatinib resistance in non-small cell lung cancer. Pharmacogenomics J. 20, 227–234. doi: 10.1038/s41397-019-0110-4
Meyer, K. D., Saletore, Y., Zumbo, P., Elemento, O., Mason, C. E., and Jaffrey, S. R. (2012). Comprehensive analysis of mRNA methylation reveals enrichment in 3' UTRs and near stop codons. Cell 149, 1635–1646. doi: 10.1016/j.cell.2012.05.003
Miao, R., Dai, C. C., Mei, L., Xu, J., Sun, S. W., Xing, Y. L., et al. (2020). KIAA1429 regulates cell proliferation by targeting c-Jun messenger RNA directly in gastric cancer. J. Cell Physiol. 235:7420–32. doi: 10.1002/jcp.29645
Narayan, P., Ludwiczak, R. L., Goodwin, E. C., and Rottman, F. M. (1994). Context effects on N6-adenosine methylation sites in prolactin mRNA. Nucleic Acids Res. 22, 419–426. doi: 10.1093/nar/22.3.419
Pan, Y., Ma, P., Liu, Y., Li, W., and Shu, Y. (2018). Multiple functions of m(6)A RNA methylation in cancer. J. Hematol. Oncol. 11:48. doi: 10.1186/s13045-018-0590-8
Patil, D. P., Chen, C. K., Pickering, B. F., Chow, A., Jackson, C., Guttman, M., et al. (2016). m(6)A RNA methylation promotes XIST-mediated transcriptional repression. Nature 537, 369–373. doi: 10.1038/nature19342
Perakis, S. O., Thomas, J. E., and Pichler, M. (2016). Non-coding RNAs enabling prognostic stratification and prediction of therapeutic response in colorectal cancer patients. Adv. Exp. Med. Biol. 937, 183–204. doi: 10.1007/978-3-319-42059-2_10
Qian, J. Y., Gao, J., Sun, X., Cao, M. D., Shi, L., Xia, T. S., et al. (2019). KIAA1429 acts as an oncogenic factor in breast cancer by regulating CDK1 in an N6-methyladenosine-independent manner. Oncogene 38, 6123–6141. doi: 10.1038/s41388-019-0861-z
Razzouk, S. (2014). Translational genomics and head and neck cancer: toward precision medicine. Clin. Genet. 86, 412–421. doi: 10.1111/cge.12487
Sheng, H., Li, Z., Su, S., Sun, W., Zhang, X., Li, L., et al. (2019). YTH domain family 2 promotes lung cancer cell growth by facilitating 6-phosphogluconate dehydrogenase mRNA translation. Carcinogenesis 41:541–50. doi: 10.1093/carcin/bgz152
Siegel, R. L., Miller, K. D., and Jemal, A. (2020). Cancer statistics, 2020. CA Cancer J. Clin. 70, 7–30. doi: 10.3322/caac.21590
Sun, L., Liu, W. K., Du, X. W., Liu, X. L., Li, G., Yao, Y., et al. (2020). Large-scale transcriptome analysis identified RNA methylation regulators as novel prognostic signatures for lung adenocarcinoma. Ann. Transl. Med. 8:751. doi: 10.21037/atm-20-3744
Wang, H., Deng, Q., Lv, Z., Ling, Y., Hou, X., Chen, Z., et al. (2019). N6-methyladenosine induced miR-143-3p promotes the brain metastasis of lung cancer via regulation of VASH1. Mol. Cancer 18:181. doi: 10.1186/s12943-019-1108-x
Wang, S., Chai, P., Jia, R., and Jia, R. (2018). Novel insights on m(6)A RNA methylation in tumorigenesis: a double-edged sword. Mol. Cancer 17:101. doi: 10.1186/s12943-018-0847-4
Wang, T., Kong, S., Tao, M., and Ju, S. (2020). The potential role of RNA N6-methyladenosine in Cancer progression. Mol. Cancer 19:88. doi: 10.1186/s12943-020-01204-7
Wanna-Udom, S., Terashima, M., Lyu, H., Ishimura, A., Takino, T., Sakari, M., et al. (2020). The m6A methyltransferase METTL3 contributes to transforming growth factor-beta-induced epithelial-mesenchymal transition of lung cancer cells through the regulation of JUNB. Biochem. Biophys. Res. Commun. 524, 150–155. doi: 10.1016/j.bbrc.2020.01.042
Wei, W., Huo, B., and Shi, X. (2019). miR-600 inhibits lung cancer via downregulating the expression of METTL3. Cancer Manag. Res. 11, 1177–1187. doi: 10.2147/CMAR.S181058
Yang, Z., Li, J., Feng, G., Gao, S., Wang, Y., Zhang, S., et al. (2017). MicroRNA-145 modulates N(6)-methyladenosine levels by targeting the 3'-untranslated mRNA region of the N(6)-methyladenosine binding YTH domain family 2 protein. J. Biol. Chem. 292, 3614–3623. doi: 10.1074/jbc.M116.749689
Yi, D., Wang, R., Shi, X., Xu, L., Yilihamu, Y., and Sang, J. (2020). METTL14 promotes the migration and invasion of breast cancer cells by modulating N6methyladenosine and hsamiR146a5p expression. Oncol. Rep. 43, 1375–1386. doi: 10.3892/or.2020.7515
Zhang, C., Huang, S., Zhuang, H., Ruan, S., Zhou, Z., Huang, K., et al. (2020). YTHDF2 promotes the liver cancer stem cell phenotype and cancer metastasis by regulating OCT4 expression via m6A RNA methylation. Oncogene 39, 4507–4518. doi: 10.1038/s41388-020-1303-7
Zhang, S., Zhao, B. S., Zhou, A., Lin, K., Zheng, S., Lu, Z., et al. (2017). m(6)A demethylase ALKBH5 maintains tumorigenicity of glioblastoma stem-like cells by sustaining FOXM1 expression and cell proliferation program. Cancer Cell 31:e596. doi: 10.1016/j.ccell.2017.02.013
Zheng, W., Dong, X., Zhao, Y., Wang, S., Jiang, H., Zhang, M., et al. (2019). Multiple functions and mechanisms underlying the role of METTL3 in human cancers. Front. Oncol. 9:1403. doi: 10.3389/fonc.2019.01403
Zhou, J., Wang, J., Hong, B., Ma, K., Xie, H., Li, L., et al. (2019). Gene signatures and prognostic values of m6A regulators in clear cell renal cell carcinoma - a retrospective study using TCGA database. Aging 11, 1633–1647. doi: 10.18632/aging.101856
Keywords: lung cancer, N6-methylAdenosine (m6A), prognosis, epitranscriptomics, cancer biomarker
Citation: Wang H, Zhao X and Lu Z (2021) m6A RNA Methylation Regulators Act as Potential Prognostic Biomarkers in Lung Adenocarcinoma. Front. Genet. 12:622233. doi: 10.3389/fgene.2021.622233
Received: 28 October 2020; Accepted: 15 January 2021;
Published: 10 February 2021.
Edited by:
Giovanni Nigita, The Ohio State University, United StatesReviewed by:
Borhane Guezguez, German Cancer Research Center (DKFZ), GermanyXiaoli Ping, Beckman Research Institute, City of Hope, United States
Copyright © 2021 Wang, Zhao and Lu. This is an open-access article distributed under the terms of the Creative Commons Attribution License (CC BY). The use, distribution or reproduction in other forums is permitted, provided the original author(s) and the copyright owner(s) are credited and that the original publication in this journal is cited, in accordance with accepted academic practice. No use, distribution or reproduction is permitted which does not comply with these terms.
*Correspondence: Xiangxuan Zhao, xiangxuanzhao@163.com; Zaiming Lu, luzaiming@sina.com
†These authors have contributed equally to this work