- 1Shenzhen Branch, Guangdong Laboratory for Lingnan Modern Agriculture, Agricultural Genomics Institute at Shenzhen, Chinese Academy of Agricultural Sciences, Shenzhen, China
- 2Institute of Crop Sciences, National Key Facility for Crop Gene Resources and Genetic Improvement, Chinese Academy of Agricultural Sciences, Beijing, China
- 3College of Agronomy, Hebei Agricultural University, Baoding, China
- 4International Rice Research Institute, Los Baños, Philippines
Nitrogen is one of the most important nutrients for rice growth and development. Breeding of nitrogen deficiency tolerance (NDT) variety is considered to be the most economic measure to solve the constrain of low nitrogen stress on grain yield in rice. An interconnected breeding (IB) population of 497 lines developed using Huanghuazhan (HHZ) as the recurrent parent and eight elite lines as the donor parents were tested for five traits including grain yield, biomass, harvest index, thousand grain weight, and spikelet fertility under two nitrogen treatments in three growing seasons. Association analysis using 7,388 bins generated by sequencing identified a total of 14, 14, and 12 QTLs for the five traits under low nitrogen (LN), normal nitrogen (NN), and LN/NN conditions, respectively, across three seasons. Favorable alleles were dissected for the 40 QTLs at the 10 NDT regions, and OM1723 was considered as the most important parent with the highest frequency of favorable alleles contributing to NDT-related traits. Six superior lines all showed significantly higher GY in LN environments and similar GY under NN environments except for H10. Substitution mapping using near-isogenic introgression lines delimited the qTGW2-1, which was identified on chromosome 2 under LN, NN, and LN/NN conditions into two QTLs, which were located in the two regions of about 200 and 350 kb with different favorable alleles. The bins 16, 1301, 1465, 1486, 3464, and 6249 harbored the QTLs for NDT detected in this study, and the QTLs/genes previously identified for NDT or nitrogen use efficiency (NUE) could be used for enhancing NDT and NUE by marker-assisted selection (MAS).
Introduction
Rice (Oryza sativa L.) is one of the most important staple crops in the world and also the main calorie source for more than 65% of the population in China. Nitrogen is one of the most important nutrients in the growth stage of crops (Yoshida, 1981). In China, about 70% of the rice paddy fields have low or moderate nitrogen supply, which limits yield potential of rice production (Feng et al., 2018). Therefore, development of rice varieties with good nitrogen deficiency tolerance (NDT) is considered as a key method of sustainable agriculture for food security.
In recent years, many QTLs/genes for NDT, measured as the relative trait values under low nitrogen (LN) stress to normal nitrogen (NN) conditions have been reported by QTL mapping and gene expression methods. QTLs were detected for the traits or the relative trait values of seedling height, shoot dry weight, chlorophyll content (Tong et al., 2011), maximum root length, root dry weight, plant dry weight (Lv et al., 2010; Zhao et al., 2014) at seedling stage, spikelet fertility percentage (Shan et al., 2005), grain yield (Tong et al., 2006), panicle number and total panicle weight (Wang et al., 2009), grain number per panicle, spikelet fertility percentage and 100-grain weight (Tong et al., 2011), and nitrogen efficiency (Tang et al., 2011) at maturity stage using populations such as backcross, chromosome segment substitution line, and recombinant inbred lines under LN stress or different nitrogen level conditions. A total of 14 QTLs for NDT-related traits (relative shoot and root biomass, and relative plant height) were identified by Lian et al. (2005) in an RIL population derived from Zhenshan97 × Minghui63. Fifteen QTLs were identified for the four NDT traits including relative grain yield, relative biomass, relative grain nitrogen, and relative biomass nitrogen (Wei et al., 2012), and some of which are close to genes controlling nitrogen cycle.
Most of NDT QTLs reported in rice were conducted using populations derived from two parents and sparse linkage maps constructed using restriction fragment length polymorphism (RFLP) or simple sequence repeat (SSR). It is very hard to obtain precise information about the QTLs using a small number of markers, which were coarsely located. Fine mapping using a large secondary population and new markers are needed (Wang et al., 2014). With the development of sequencing technology, high-density single-nucleotide polymorphism (SNP) markers can be easily and quickly generated, which has been widely applied to genome-wide association studies (GWAS) and QTL mapping in rice and many other crops (Yan et al., 2010; Wang et al., 2014; Chen et al., 2020).
The past decade has seen the rise of multiparental populations as a study design offering great advantages for genetic studies in plants. Multiparent mapping populations such as nested association mapping (NAM) population (Yu et al., 2008) and multiparent advanced generation inter-cross (MAGIC) population (Cavanagh et al., 2008) have been developed for many crops. A NAM population is usually generated by crossing multiple genotypes with a single genotype. Using multiparental population for QTL mapping provides an opportunity to test pleiotropy, genetic background effect, and the genetic overlap between different complex traits (Qu et al., 2020). Buckler et al. (2009) developed a NAM population that was consisted of 25 families with 200 lines per family in maize. The NAM population has been used for studying the complex traits in maize, such as pathogen resistances (Kump et al., 2011), morphological traits (Tian et al., 2015), and kernel composition (Cook et al., 2012). Jordan et al. (2011) developed a NAM population containing 56 families for detecting QTLs in sorghum. However, the application of NAM population for QTL mapping in rice is rarely reported.
A backcross (BC)-based breeding strategy has been adopted by our team to improve multiple abiotic stress tolerance for many years (Ali et al., 2006; He et al., 2010; Meng et al., 2013; Wang et al., 2013; Feng et al., 2018). A few outstanding varieties were used as recurrent parents, and many varieties and landraces were used as donor parents in combination with a selection of tolerance to multiple stresses and grain yield. As a result, many small-to-medium size populations sharing a common parent were developed (Ali et al., 2017). Mapping QTL for a range of complex traits using these populations has been carried out (Li et al., 2005; Cui et al., 2015; Feng et al., 2018). However, the interconnectedness between the populations has not been well exploited. A set of breeding populations linked together by a common parent can be regarded as a single interconnected breeding (IB) population for mapping purpose, which is similar to a NAM population that consists of subpopulations sharing a common parent. It is expected that IB population could significantly increase the mapping resolution and power by exploring multiple populations simultaneously. An IB population consisting of highly selected introgression lines derived using HHZ as recurrent parent and eight elite lines as donors has been successfully used in identifying QTL for cold tolerance at the booting stage (Zhu et al., 2015). The fact that a QTL for cold tolerance was fine mapped to a 192-kb region encouraged us to explore this IB population for other traits.
The current study was to identify QTL and favorable alleles for NDT at the reproductive stage. The objectives of this study were (1) to identify the QTLs affecting NDT under different nitrogen conditions; and (2) to gain a better understanding of the genetic relationships between NDT and GY at the QTL level. The results will provide a good example of QTL mapping using breeding population and useful information for rice breeding of NDT by marker-assisted selection (MAS).
Materials and Methods
Plant Materials and Field Experiments
The HHZ IB population used in the study was derived from recurrent parent Huanghuazhan (HHZ) crossed with eight donor parents IR50, IR64, Teqing, PSBRc28, PSBRc66, CDR22, OM1723, and Phalguna. Eight F1s were backcrossed once with HHZ to harvest the BC1F1. Through multiple generations selfing and screenings for yield and abiotic stresses (drought, salinity, and submergence), a total of 496 BC1F5 lines were produced (Ali et al., 2017). A total of 496 lines of the IB population plus the HHZ were field tested under different nitrogen conditions in the early and late seasons of 2013 (2013E and 2013L) and the early season of 2014 (2014E) in Shenzhen (22°52′N 113°46′E), Guangdong province, China. Two nitrogen conditions were applied, they were (1) low nitrogen condition (LN), no chemical nitrogen fertilizer was applied to the LN paddy since 2012; (2) normal nitrogen condition (NN), 150 kg N ha–1 was applied with two splits. About 70% nitrogen fertilizers were used as basal, and 30% nitrogen fertilizers were applied at 15 days after transplanting under the normal nitrogen condition. Phosphorus (40 kg ha–1) and potassium (40 kg ha–1) were applied as basal under two nitrogen conditions. All fertilizers were broadcast by hand. The LN paddy soil had the following properties: pH 6.18, organic matter of 4.32 g kg–1, total N of 380 mg kg–1, available P of 59.2 mg kg–1, and available K of 165 mg kg–1. The key properties of the NN paddy soil were pH 5.97, organic matter of 10.9 g kg–1, total N of 920 mg kg–1, available P of 88.8 mg kg–1, and available K of 155 mg kg–1.
The experiments were conducted using a randomized block design with two replications. The seeds were sowed on March 5 in 2013E and 2014E, and on July 13 in 2013L. The seedlings were transplanted into three rows with eight plants per row at a planting density of 20 cm × 16.5 cm on April 5 in 2013E and 2014E, and on August 5 in 2013L. The field management was according to the local rice production, and the pests, diseases, birds, weeds, and rats were intensively controlled during the whole growth duration.
Sampling and Trait Measurement
At maturity stage, six uniform plants from the middle of each plot were harvested. The plants were cut at ground level, and grains were separated from other parts (leaves and sheath, and stems and panicle branches). All samples were oven-dried at 80°C until a constant weight was achieved. Grain yield per plant (GY), biomass per plant (BM), thousand grain weight (TGW), and spikelet fertility (SF) were measured. Harvest index (HI) was calculated by GY/BM. Also, specific parameters were calculated using the following equations:
Relative harvest index (RHI) = HILN/HINN,
Relative grain yield (RGW) = GYLN/GYNN,
Relative biomass yield (RBM) = BMLN/BMNN,
Relative thousand grain weight (RTGW) = TGWLN/TGWNN,
Relative spikelet fertility (RSF) = TSFLN/TSFNN.
SNP Genotyping and Bin Markers
To get the consensus sequence that can be used to compare the differential bases between the HHZ and the donor parent, all reads from each parent with 30× re-sequencing were first aligned to the Nipponbare reference genome. Based on the differential SNP results, the pseudo-molecules of both parents were generated in pairs by using Perl script for eight mapping populations, respectively. A total of 400 k high-quality SNPs was developed based on whole-genome re-sequencing of 2× in IB population. To improve analytical accuracy and the resolution of re-sequencing data, we used the sliding window approach to determine the recombination breakpoint based on the SNP ratios (Huang et al., 2009). Based on the recombination breakpoint, the 496 lines were aligned to all the chromosomes and compared for the minimal of 50-kb intervals. Adjacent 50-kb intervals with the same genotype across the entire IB population were recognized as a single recombination bin. A total of 7,388 bins covered in all of 12 chromosomes were obtained for association analysis.
Statistical and Association Analyses
We used the R project for statistical analysis. The basic statistics of phenotypic values were calculated using the R package named EnvStats. Correlation coefficients were obtained from the R function called corr. Two-way ANOVA was conducted by the R function called ANOVA. Based on the adjusted means and the trait ratios of LN to NN of ILs, association analyses were conducted by R-package MAGICqtl1. This package implemented random model-based methods proposed by Wei and Xu (2016) for QTL detection using a multi-parental population. The initial threshold to declare a significant association was set at p = 1.0 × 10–4. The threshold value of the main effect QTL was LOD > 3.2. The gene effects of multiple alleles in each QTL were estimated by the difference between the mean phenotypic value of one allele and mean phenotypic value of all the alleles.
Results
Phenotypic Performance
Grain yield and BM were much higher in the NN than in the LN, while HI and SF were similar in the two nitrogen treatments (Table 1). TGW was slightly higher in the NN. The average GY, BM, HI, SF, and TGW of the IB population were similar to those of HHZ. The estimates of skewness ranged from −2.873 to 1.783, and the estimates of kurtosis ranged from 0.059 to 16.65. The heritability in the NN was similar to or higher than that in the LN in all three seasons, ranging from 0.647 of BM under LN in 2013E to 0.929 of HI under NN in 2014E.
Two-way ANOVA (genotype and season) indicated that season was highly significant for GY, BM, HI, TGW, and SF in the two nitrogen treatments (Supplementary Table 1). Genotype was highly significant for GY, HI, TGW, and SF in the two nitrogen treatments. Genotype-by-season interaction was highly significant for HI and SF in the two nitrogen treatments. For the five traits, the main source of the variation was the season. The effect of genotype was significant for all five traits in the two conditions except for BM in the NN treatment.
In the LN, GY was highly, significantly, and positively correlated with other traits in three seasons except TGW in 2014E (Supplementary Table 2). In the NN, GY was highly significantly correlated with BM, HI, and SF, and not significantly correlated with TGW in the three seasons.
QTL Mapping
A total of 40 QTLs were identified for the five NDT-related traits across the six environments (nitrogen–season combinations) (Table 2 and Figure 1).
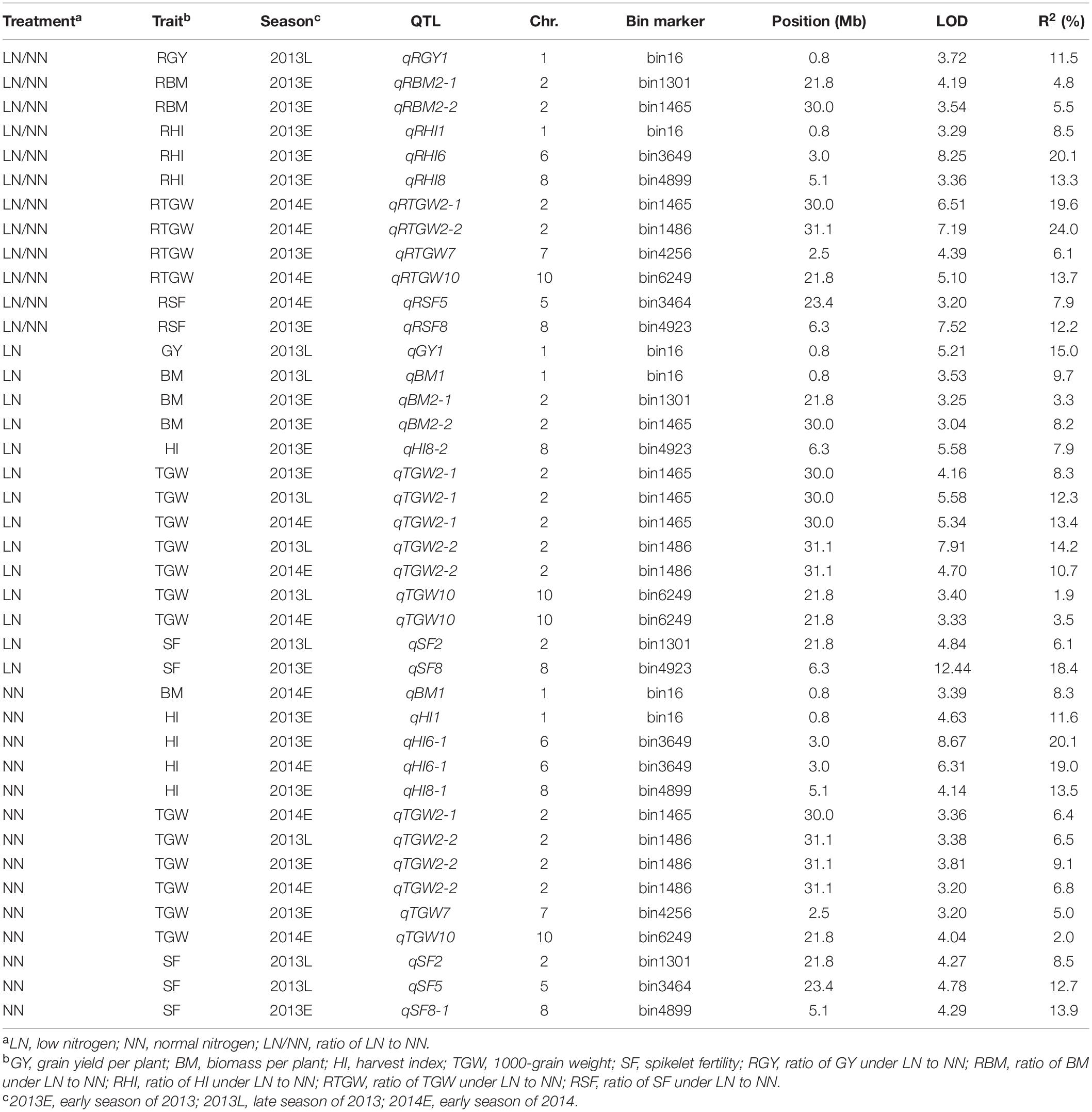
Table 2. Putative QTLs for five NDT-related traits identified under LN, NN, and LN/NN conditions in three seasons.
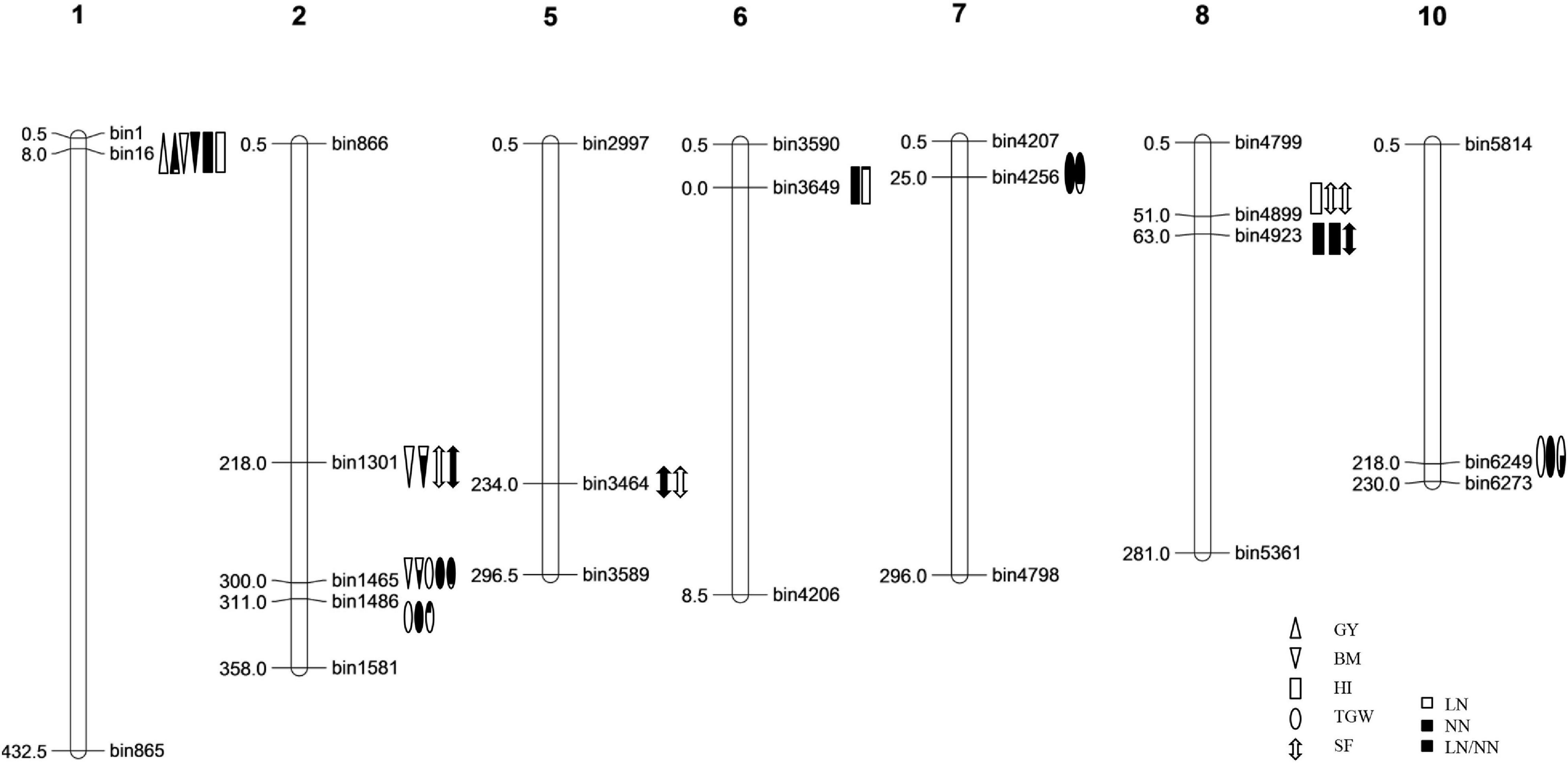
Figure 1. The genetic linkage map and the locations of QTLs for nitrogen deficiency tolerance (NDT)-related traits identified in the IB population.
QTLs Detected for Trait Ratios of LN to NN (LN/NN)
For RGY, one QTL (qRGY1) was detected on chromosome 1 in 2013L and accounted for 11.5% of phenotypic variation (Table 2). For RBM, two QTLs (qRBM2-1 and qRBM2-2) were identified on chromosome 2 in 2013E and explained 4.8% and 5.5% of the phenotypic variation, respectively. For RHI, three QTLs (qRHI1, qRHI6, and qRHI8) were identified in 2013E and distributed on chromosomes 1, 6, and 8 with the phenotypic variations of 8.5%, 20.1%, and 13.3%, respectively. Four QTLs for RTGW, including qRTGW7 in 2013E, and qRTGW2-1, qRTGW2-2, and qRTGW10 in 2014E, were detected on chromosomes 7, 2, 2, and 10, respectively, and explained 6.1%, 19.6%, 24.0%, and 13.7% of the phenotypic variations, respectively. For RSF, two QTLs (qRSF5 and qRSF8) were identified on chromosomes 5 and 8 in 2014E and 2013E with phenotypic variations of 7.9% and 12.2%, respectively.
QTLs Detected for Traits in Low Nitrogen (LN) Condition
One QTL for GY, qGY1 was detected on chromosome 1 in 2013L and explained 15.0% of the phenotypic variation (Table 2). For BM, three QTLs (qBM1, qBM2-1, and qBM2-2) were identified on chromosomes 1, 2, and 2 in 2013L and 2013E, and accounted for phenotypic variations of 9.7%, 3.3%, and 8.2%, respectively. One QTL named qHI8-2 was identified for HI, which explained a phenotypic variation of 7.9%. Three QTLs were identified for TGW. Specifically, qTGW2-1 was simultaneously detected on chromosome 2 in all three conditions with phenotypic variations from 8.3 to 13.4%. qTGW2-2 was detected on chromosome 2 in 2013L and 2014E with phenotypic variations of 14.2% and 10.7%, while qTGW10 was detected on chromosome 10 in 2013L and 2014E with phenotypic variations of 1.9–3.5%. Two QTLs (qSF2, qSF8) were mapped to chromosomes 2 and 8 for SF in 2013L and 2013E, which accounted for phenotypic variations of 6.1% and 18.4%, respectively.
QTLs Detected for Traits in Normal Nitrogen (NN) Condition
One QTL named qBM1 was detected on chromosome 1 for BM in 2014E (Table 2), which explained 8.3% of the phenotypic variation. For HI, three QTLs (qHI1, qHI6-1, and qHI8-1) were identified. The QTL on chromosome 1, qHI1, was identified in 2013E and explained 11.6% of the phenotypic variation. The QTL, qHI6-1, on chromosome 6 was simultaneously identified in 2013E and 2014E and explained 20.1% and 19.0% of the phenotypic variation. The QTL qHI8-1 on chromosome 8 was detected in 2013E and explained 13.5% of the phenotypic variation. Four QTLs were identified for TGW. qTGW2-2 was simultaneously identified on chromosome 2 in all three conditions and explained 6.5–9.1% of phenotypic variation. qTGW2-1 and qTGW10 were mapped to chromosomes 2 and 10 in 2014E and explained 6.4% and 2.0% of the phenotypic variation, respectively. qTGW7 was detected on chromosome 7 in 2013E with a phenotypic variation of 5.0%. For SF, two QTLs (qSF2 and qSF5) were mapped on chromosomes 2 and 5 in 2013L with phenotypic variations of 8.5% and 12.7%, respectively, while another one (qSF8-1) was mapped on chromosome 8 in 2013E with phenotypic variations of 13.9%.
Favorable Allele Mining of Important QTLs
Ten regions were detected for NDT-related traits in multiple seasons (Table 3). Among them, five were reported near the cloned genes related to nitrogen use efficiency (NUE) and/or functional ammonium transporter. The region bin16 on chromosome 1 was detected and associated with multiple traits, such as GY, RGY, BM, HI, and RHI, and the best favorable alleles all came from the donor parent OM1723 except the HI in 2013E NN environment. The region bin1301 was identified in relation to the traits BM, RBM, and SF, which were located very close to the cloned gene LOC_Os02g38230 with functional annotation of partner protein for high-affinity nitrate transport (Liu et al., 2014). The best favorable alleles for BM and RBM came from the donor Teqing, while the best favorable alleles for SF came from OM1723 and Phalguna in 2013L LN and 2013L NN environments, respectively. Even though the best favorable alleles came from different donors, they are all carrying higher positive effects than the recurrent parent HHZ. The bin1465 and bin1486 were very near the cloned genes LOC_Os02g47280 or GRF4 (Hu et al., 2015; Li et al., 2018a) and LOC_Os02g53130 (Gao et al., 2019) on chromosome 2 for NUE, respectively. At the region of bin1465, the favorable PSBRc28 alleles increased the traits RBM in 2013E LN/NN, BM in 2013E LN, and RTGW in 2014E LN/NN environments, whereas the favorable OM1723 alleles increased TGW in 2013E LN, 2013L LN, and 2014E NN environments. The bin1486 was detected in relation to TGW in both LN and NN environments with the favorable alleles coming from the donor OM1723. Bin3464, bin3649, bin4256, and bin4899 were detected for SF, HI, TGW, etc., in both NN and LN/NN environments with the favorable alleles coming from various donors. At the region of bin3464 harboring LOC_Os05g39240 for functional ammonium transporter (Gaur et al., 2012), the favorable allele was from IR64 for RSF in 2014E LN/NN environment, while another one was from PSBRc28 for SF in 2013L NN environment. Similarly, at the region of bin3649, the favorable allele was from OM1723 for RHI in 2013E LN/NN, while the other ones were from PSBRc66 for HI in 2013E and 2014E NN environments. At the region of bin4256, the favorable OM1723 allele increased RTGW in 2013E LN/NN environment, while the favorable CDR22 allele increased TGW in 2013E NN environment. At the region of bin4899, the favorable alleles were from IR64, Teqing, and Phalguna for the traits RHI, HI, and SF in 2013E LN/NN, 2013E NN, and 2013E NN environments, respectively. The favorable PSBRc28 alleles at the bin4923 increased RSF in 2013E LN/NN and SF in 2013E LN environments, while the favorable Phalguna allele increased HI in 2013E LN environment. The bin6249 was detected for TGW and RTGW in LN, NN, and LN/NN environments of 2014E, with the favorable alleles all from Phalguna, which is located in the same region as the cloned gene LOC_Os10g40600 with the functional annotation of nitrogen use efficiency (Hu et al., 2019; Zhang et al., 2019).
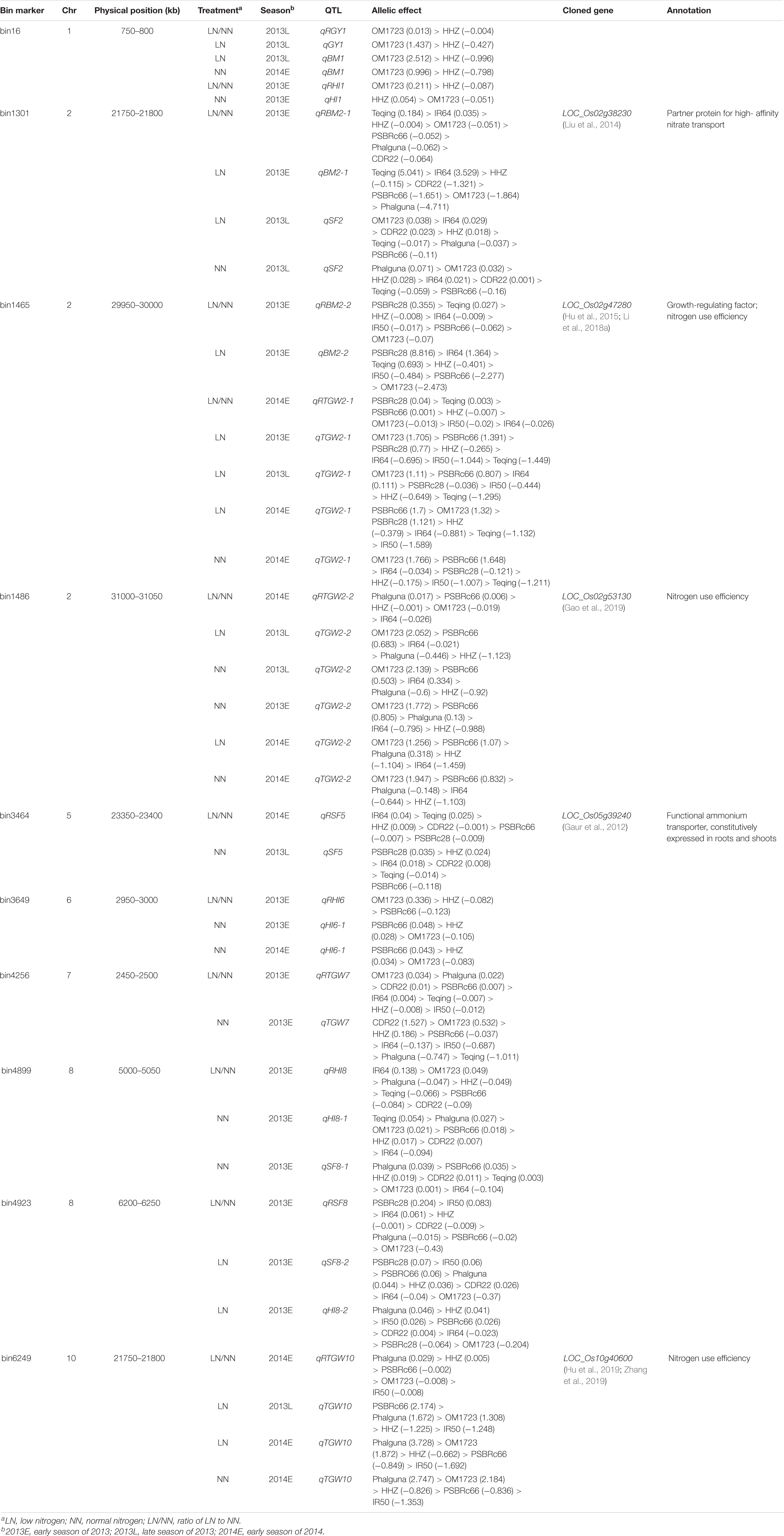
Table 3. Effects of putative QTLs identified in this study and the previously cloned genes related to nitrogen deficiency tolerance.
Selecting Super Nitrogen Deficiency Tolerant Lines
Based on the favorable allele dissection, six superior lines with four favorable introgressed alleles were selected (Table 4). Compared with the recurrent parent HHZ, the six lines all showed significantly higher GY in LN environments and similar GY under NN environments except for H10. Out of the six lines, Line H11 carrying simultaneously three detected QTLs for GY and TGW with the best favorable alleles all coming from OM1723 performed the highest GY, TGW, and BM in LN environments. Lines H10 and H12, separately carrying QTLs underlying GY and TGW, were superior to HHZ in terms of GY, TGW, and BM in LN environments. Three lines (H289, H456, and H53) harbored different QTLs affecting BM wherein the best favorable alleles were from Teqing, PSBRc28, and OM1723, respectively. The above six lines carrying different favorable introgressed alleles for NDT-related traits from various donors are beneficial for pyramid breeding for improved GY under LN environment.
Fine Mapping of qTGW2-1
The qTGW2-1, which was simultaneously detected in 2014E in three conditions (LN, NN, and LN/NN) and also in 2013E and 2013L in LN conditions, most likely associated with NDT and the favorable alleles mainly came from OM1723 and PSBRc28 in different conditions. To fine map the stable qTGW2-1 in the region of bin1456–bin1466 on chromosome 2 by means of substitution mapping, ILs with overlapped introgressed segments and covered the genomic region of qTGW2-1 were selected from the IB population. Seven selected ILs had the recurrent genome percentage higher than 94.0%. The graphic genotypes of these seven ILs are given in Figure 2. Three, three, and one lines had the alleles from the donor parents PSBRc66, IR50, and Phalguna, respectively. The lines of H129, H342, H358, and H359 had significantly higher TGW than the recipient parent HHZ, while H180 and H181 had significantly lower TGW than the recipient parent. The TGW of H165 was very similar to that of HHZ. Therefore, the qTGW2-1 was separated into two QTLs for TGW, one was located in about the 200-kb region flanked by bin1456 and bin1460 with the positive alleles for increased TGW from the donor parent PSBRc66 and Phalguna, and the other one was located in about the 350-kb region delimited by bin1459 and 1466 with the negative allele for decreased TGW from the donor parent IR50. The GRF4 for NUE (Hu et al., 2015; Li et al., 2018a) was around 633-kb apart from the QTL flanked by bin1456 and bin1460 (Figure 2), suggesting the two QTLs separated from qTGW2-1 were new loci for NDT.
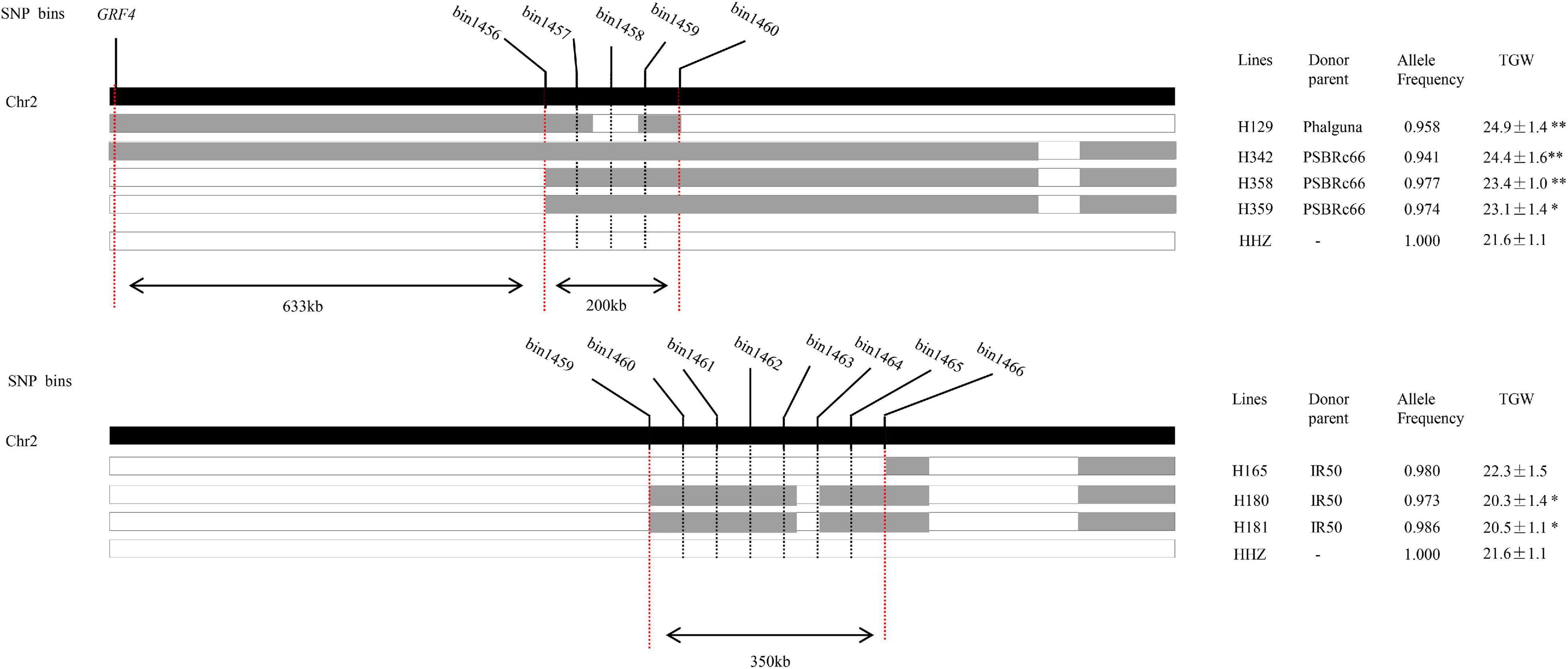
Figure 2. The physical location of qTGW2-1 delimited by graphical genotype mapping. The black-colored strip indicates chromosome 2. The gray and white strips show the homozygous introgressed segment derived from the donor and HHZ, respectively. The allele frequency is the percentage of the alleles of HHZ. * and ** represent significant differences at P ≤ 0.05 and 0.01, respectively.
Discussion
Comparisons of the NDT QTLs Identified in This Study With Previously Reported QTLs and Cloned Genes
Nitrogen-deficiency tolerance (NDT) traits were measured by the ratios of the trait values under LN to those under NN and could be used as a criterion for selecting genotypes for the tolerance of low nitrogen. Many agronomic traits such as effective tiller number, biomass, and grain yield have been studied under low-N stress in rice, and some QTLs associated with N translocation were found to locate in the same chromosomal regions (Shan et al., 2005; Tong et al., 2006; Senapathy et al., 2008). Among 10 QTL regions identified for the NDT-related traits in this study (Table 3), the region bin16 on chromosome 1 harboring QTLs for BM, GY, and HI was associated with the QTLs affecting shoot dry weight and plant dry weight at seedling stage under low nitrogen condition identified by Lian et al. (2005), and the QTLs for relative shoot weight, relative plant height, and relative biomass yield at seedling stage (Feng et al., 2010). QTLs affecting BM and SF in the bin1301 identified in this study were mapped together with the QTLs for dry shoot weight under low nitrogen condition (Lian et al., 2005), grain nitrogen use efficiency and grain yield (Wei et al., 2011), soluble protein content detected in the backcross inbred lines of Nipponbare/Kasalath (Ishimaru et al., 2001), and the cloned gene LOC_Os02g38230 for partner protein for high-affinity nitrate transport (Liu et al., 2014). The region bin1465 harboring QTLs for BM and for TGW on chromosome 2 identified in the present study were mapped together with the QTL affecting root dry weight under low nitrogen condition (Lian et al., 2005) and near the LOC_Os02g47280 for nitrogen use efficiency and growth-regulating factor (Hu et al., 2015; Li et al., 2018a), and the gy2b affecting GY under low nitrogen condition (Cho et al., 2007). The bin1486 harboring QTLs for TGW and RTGW on chromosome 2 in this study were mapped together to the QTL for the ratio of plant height under low nitrogen stress to normal conditions (Feng et al., 2010) and the LOC_Os02g53130, a nitrate reductase gene for nitrogen use efficiency (Gao et al., 2019). The bin3464 harboring QTLs for RSF and SF on chromosome 5 in this study was mapped near the qHGW-5b for grain weight under low nitrogen input (Tong et al., 2011) and the LOC_Os05g39240 for functional ammonium transporter, which was constitutively expressed in roots and shoots (Gaur et al., 2012). The bin4899 harboring QTLs for HI and SF on chromosome 8 in this study was associated with the QTL for plant dry weight under low nitrogen condition (Lian et al., 2005). The bin6249 on chromosome 10 affecting TGW detected under LN, NN, and LN/NN conditions in this study harbored the QTL (qFGPP-10b) for filled grains per panicle under low nitrogen input (Tong et al., 2011), the qNR10 for nitrogen response (Wei et al., 2012), and the LOC_Os10g40600, a nitrate-transporter gene for nitrogen use efficiency (Hu et al., 2019; Zhang et al., 2019). Allelisms of the above QTLs for NDT-related traits identified in this study with the previously reported QTLs or cloned genes for NDT or NUE require further verification via fine mapping and QTL cloning.
Nitrogen deficiency tolerance is defined as the plants’ ability to maintain normal growth and good yield when the soil N content is low. Thus, NDT could have something to do with NUE to some extent. Based on comparisons of QTLs affecting NDT detected in this study with those previously reported, five regions underlying NDT at bins 1301, 1465, 1486, 3464, and 6249 on chromosomes 2, 2, 2, 5, and 10, respectively, were found to map together with or near the five cloned NUE-related genes. Using 127 recombinant inbred lines (RILs) derived from the cross of Zhenshan97/Minghui63, Wei et al. (2012) also identified four genomic regions containing QTLs for NDT and NUE traits with the same additive effect directions. It was suggested that partial genetic overlaps at least exist between NDT and NUE, which is beneficial for rice breeding for simultaneously improving NDT and NUE.
Mining Favorable Alleles for NDT
From the breeding point of view, the major use of identified QTL or cloned gene is to mine favorable alleles for efficient molecular breeding. So far, over 2,000 genes controlling important agronomic traits have been cloned in rice (Li et al., 2018b). Unfortunately, the phenotypic effects of different alleles in rice germplasm accessions at these cloned rice genes and their values in rice breeding remain largely unknown. This is one of the most important reasons why so many cloned genes have never been exploited in breeding through MAS so far. Using eight BC1 populations derived from a widely adaptable recipient and eight donors plus three rounds of phenotypic selection, 496 trait-specific introgression lines (ILs) in Huanghuazhan (HHZ) background have been developed (Ali et al., 2017), forming a material platform for discovery of QTLs underlying the target and non-target traits (Zhu et al., 2015; Feng et al., 2018). The IL population has characteristics of similar developmental stage and plant height in elite rice background, thus very suitable for evaluation of abiotic stress tolerances such as drought, salt, and cold whose tolerance performances largely depend on developmental stage. In this study, favorable alleles at QTLs for different NDT-related traits in the 10 NDT-related regions were dissected (Table 3). Among the total 40 loci affecting different NDT-related traits at the 10 bins under different conditions (Table 3), frequencies of favorable alleles were 16 for OM1723, seven for Phalguna, six for PSBRc28, four for PSBRc66, three for Teqing, two for IR64, and one for each of CDR22 and HHZ. Obviously, OM1723, an indica variety from Vietnam is an outstanding parent with many favorable alleles for improving NDT. For instance, the OM1723 alleles simultaneously increased GY under LN and LN/NN, BM under LN and NN, and RHI under LN/NN for all QTLs except qHI1 at bin16 under NN, and TGW at almost all QTLs except qRTGW2-2 at bin1486 under LN/NN. Similarly, the Phalguna alleles improved TGW for all QTLs except qTGW10 at bin6249 under LN in 2013L. So, the bin16 is one of the most important regions for rice breeding of NDT. Information of favorable allele dissection for different NDT QTLs will be beneficial for introgressing and pyramiding breeding to improve NDT by MAS.
Implications for Breeding
Historically, many breeding programs took yield potential as a primary target, particularly in China in the past few decades. In China, pursuing high yield of super rice cultivar has been accompanied with excess uses of chemical fertilizers and pesticides in rice production, resulting in serious environmental pollutions and largely reduced rate of fertilizer utilization by the crops (Zhang, 2007). Nevertheless, modern semidwarf rice cultivars have rarely achieved their yield potentials in farmers’ fields because of many abiotic and biotic stresses. The main reason for this huge yield gap is primarily due to the fact that most super rice cultivars developed under the high input conditions do not perform well in more than 70% of the moderate- and low-yielding fields in China, much of which suffer more frequently inadequate fertilizers and other abiotic stresses (Pandey, 1997; Feng et al., 2018). Consequently, developing crops that are less dependent on the heavy application of N fertilizers is essential for the sustainability of agriculture. NDT traits have been considered as indirect selection criteria for the improvement of NUE (Lian et al., 2005; Namai et al., 2009; Feng et al., 2010; Wei et al., 2012). As a matter of experience, the lines with superior yield and yield-related traits under both LN and NN conditions, or superior under LN and normal or average under NN, could be preferentially selected because such lines can use the nitrogen efficiently to produce BM and GY under limited nitrogen supply (Lafitte and Edmeades, 1994).
Nitrogen deficiency tolerance and NUE are complex quantitative traits controlled by multiple genes, which makes it difficult to improve these complex traits using conventional breeding approaches. Recently, high-throughput SNP genotyping based on re-sequencing and gene chips promises to greatly accelerate QTL mapping and pyramiding on the whole genome (Thomson, 2014; Feng et al., 2018). Ten important NDT regions were identified in the breeding population with elite variety background in this study. Some of them were co-located with the previously reported NUE-related QTLs or genes, which are useful for rice breeding for high NDT or both for NDT and NUE by introgressing or pyramiding of favorable alleles at those important loci by MAS. Specifically, the Bin16 affecting GY, RGY, BM, and RHI under different nitrogen conditions, and other five bins 1301, 1465, 1486, 3464, and 6249 shared with the previously reported QTLs, or genes underlying NDT- and NUE-related traits could be used in MAS-based or QTL-designed breeding for enhancing NDT and/or NUE in rice. As indicated in Table 4, two promising NDT lines, H10 carrying favorable alleles from OM1723 at bins 16 and 1465, and H11 with favorable alleles from OM1723 at bins 16, 1465, and 1486, can be used as donor parents to improve NDT of an elite variety by marker-assisted selection against the flanking markers linked to respective bins. Of course, important NDT loci identified herein could be also pyramided with other previously identified QTLs or genes controlling NDT and NUE as most of the NDT and NUE QTLs identified previously under different environmental conditions were genetically independent (Lian et al., 2005; Senapathy et al., 2008).
Conclusion
A total of 14, 14, and 12 QTLs for the five traits was identified under LN, NN, and LN/NN conditions, respectively, in an interconnected breeding population across three seasons. Among them, 10 NDT-bin regions were identified, and the favorable alleles contributing to NDT-related traits were primarily from OM1723, secondarily from Phalguna. Six superior lines showed significantly higher GY in LN environments and similar GY under NN environments due to introgressing the favorable alleles at the related NDT-QTLs. The bins 16, 1301, 1465, 1486, 3464, and 6249 shared the QTLs or genes for NDT identified in this study and NUE previously reported could be used for improvement of NDT and NUE by MAS.
Data Availability Statement
All datasets presented in this study are included in the article/Supplementary Material.
Author Contributions
JX and ZL designed the experiment. CS, KC, JC, SZ, and YZ performed all the phenotypic evaluation. XM and YC performed the analysis and interpretation of the data. JX, XM, and YC drafted the manuscript. GY and JA revised the manuscript. All authors contributed to the article and approved the submitted version.
Funding
This study was supported by the National Key R&D Program of China to KC (2017YFD0100100) and YZ (2017YFD0102002), the 863 Key Project from the Chinese Ministry of Science and Technology to JX (2014AA10A601), and the Agricultural Science and Technology Innovation Program and the Cooperation and Innovation Mission to JX (CAAS-ZDXT202001).
Conflict of Interest
The authors declare that the research was conducted in the absence of any commercial or financial relationships that could be construed as a potential conflict of interest.
Acknowledgments
We would like to thank the English editor for editing our manuscript.
Supplementary Material
The Supplementary Material for this article can be found online at: https://www.frontiersin.org/articles/10.3389/fgene.2021.616428/full#supplementary-material
Footnotes
References
Ali, A. J., Xu, J. L., Ismail, A. M., Fu, B. Y., Vijayakumar, C. H. M., Gao, Y. M., et al. (2006). Hidden diversity for abiotic and biotic stress tolerances in the primary gene pool of rice revealed by a large backcross breeding program. Field Crop Res. 97, 66–76. doi: 10.1016/j.fcr.2005.08.016
Ali, J., Xu, J. L., Gao, Y. M., Ma, X. F., Meng, L. J., Wang, Y., et al. (2017). Harnessing the hidden genetic diversity for improving multiple abiotic stress tolerance in rice (Oryza sativa L.). PLoS One 12:e0172515.
Buckler, E. S. (2009). The genetic architecture of maize flowering time. Science 325, 714–718. doi: 10.1126/science.1174276
Cavanagh, C. R., Morell, M. K., Mackay, I., and Powell, W. (2008). From mutations to MAGIC: resources for gene discovery, validation and delivery in crop plants. Curr. Opin. Plant Biol. 11, 215–221. doi: 10.1016/j.pbi.2008.01.002
Chen, H. W., Zhao, X. Q., Zhai, L. Y., Shao, K. T., Jiang, K. J., Shen, C. C., et al. (2020). Genetic bases of the stomata-related traits revealed by a genome-wide association analysis in rice (Oryza sativa L.). Front. Genet. 11:611. doi: 10.3389/fgene.2020.00611
Cho, Y. I., Jiang, W. Z., Chin, J. H., Piao, Z. Z., Cho, Y. G., McCouch, S. R., et al. (2007). Identification of QTLs associated with physiological nitrogen use efficiency in rice. Mol. Cells. 23, 72–79.
Cook, J. P., McMullen, M. D., Holland, J. B., Tian, F., Bradbury, P. J., Ross-Ibarra, J., et al. (2012). Genetic architecture of maize kernel composition in the nested association mapping and inbred association panels. Plant Physiol. 158, 824–834. doi: 10.1104/pp.111.185033
Cui, Y. R., Zhang, F., Xu, J. L., Li, Z. K., and Xu, S. Z. (2015). Mapping quantitative trait loci in selected breeding populations: a segregation distortion approach. Heredity 115, 538–546. doi: 10.1038/hdy.2015.56
Feng, B., Chen, K., Cui, Y. R., Wu, Z. C., Zheng, T. Q., Zhu, Y. J., et al. (2018). Genetic dissection and simultaneous improvement of drought and low nitrogen tolerances by designed QTL pyramiding in rice. Front. Plant Sci. 9:306. doi: 10.3389/fpls.2018.00306
Feng, Y., Cao, L. Y., Wu, W. M., Shen, X. H., Zhan, X. D., Zhai, R. R., et al. (2010). Mapping QTLs for nitrogen-deficiency tolerance at seedling stage in rice (Oryza sativa L.). Plant Breed. 129, 652–656. doi: 10.1111/j.1439-0523.2009.01728.x
Gao, Z. Y., Wang, Y. F., Chen, G., Zhang, A., Yang, S. L., Shang, L. G., et al. (2019). The indica nitrate reductase gene OsNR2 allele enhances rice yield potential and nitrogen use efficiency. Nat. Commun. 10:5207. doi: 10.1038/s41467-019-13110-8
Gaur, V. S., Singh, U. S., Gupta, A. K., and Kumar, A. (2012). Influence of different nitrogen inputs on the members of ammonium transporter and glutamine synthetase genes in two rice genotypes having differential responsiveness to nitrogen. Mol. Biol. Rep. 39, 8035–8044. doi: 10.1007/s11033-012-1650-8
He, Y. X., Zheng, T. Q., Hao, X. B., Wang, L. F., Gao, Y. M., Hua, Z. T., et al. (2010). Yield performances of japonica introgression lines selected for drought tolerance in a BC breeding programme. Plant Breed. 129, 167–175. doi: 10.1111/j.1439-0523.2009.01683.x
Hu, B., Jiang, Z., Wang, W., Qiu, Y., Zhang, Z., Liu, Y., et al. (2019). Nitrate–NRT1.1B–SPX4 cascade integrates nitrogen and phosphorus signaling networks in plants. Nat. Plants 5, 401–413.
Hu, J., Wang, Y. X., Fang, Y. X., Zeng, L. J., Xu, J., Yu, H. P., et al. (2015). A rare allele of GS2 enhances grain size and grain yield in rice. Mol. Plan. 8, 1146–1155. doi: 10.1016/j.molp.2015.07.002
Huang, X., Feng, Q., Qian, Q., Zhao, Q., Wang, L., Wang, A., et al. (2009). High-throughput genotyping by whole-genome resequencing. Genome Res. 19, 1068–1076.
Ishimaru, K., Yano, M., Aoki, N., Ono, K., Hirose, T., Lin, S. Y., et al. (2001). Toward the mapping of physiological and agronomic characters on a rice function map. Theor. Appl. Genet. 102, 793–800. doi: 10.1007/s001220000467
Jordan, D. R., Mace, E. S., Cruickshank, A. W., Hunt, C. H., and Henzell, R. G. (2011). Exploring and exploiting genetic variation from unadapted sorghum germplasm in a breeding program. Crop Sci. 51, 1444–1457. doi: 10.2135/cropsci2010.06.0326
Kump, K. L., Bradbury, P. J., Wisser, R. J., Buckler, E. S., Belcher, A. R., Oropeza-Rosas, M. A., et al. (2011). Genome-wide association study of quantitative resistance to southern leaf blight in the maize nested association mapping population. Nat. Genet. 43, 163–168. doi: 10.1038/ng.747
Lafitte, H. R., and Edmeades, G. O. (1994). Improvement for tolerance to low soil nitrogen in tropical maize. II. Grain yield, biomass production, and N accumulation. Field Crops Res. 39, 15–25. doi: 10.1016/0378-4290(94)90067-1
Li, S., Tian, Y., Wu, K., Ye, Y., Yu, J., Zhang, J., et al. (2018a). Modulating plant growth–metabolism coordination for sustainable agriculture. Nature 560, 595–600.
Li, Y., Xiao, J. H., Chen, L. L., Huang, X. H., Cheng, Z. K., Han, B., et al. (2018b). Rice functional genomics research: past decade and future. Mol. Plant 11, 359–380.
Li, Z. K., Fu, B. Y., Gao, Y. M., Xu, J. L., Ali, J., Lafitte, H. R., et al. (2005). Genome-wide introgression lines and their use in genetic and molecular dissection of complex phenotypes in rice (Oryza sativa L.). Plant Mol. Biol. 59, 33–52. doi: 10.1007/s11103-005-8519-3
Lian, X. M., Xing, Y. Z., Yan, H., Xu, C. G., Li, X. H., and Zhang, Q. F. (2005). QTLs for low nitrogen tolerance at seedling stage identified using a recombinant inbred line population derived from an elite rice hybrid. Theor. Appl. Genet. 112, 85–96.
Liu, X. Q., Huang, D. M., Tao, J. Y., Miller, A. J., Fan, X. R., and Xu, G. H. (2014). Identification and functional assay of the interaction motifs in the partner protein OsNAR2.1 of the two-component system for high-affinity nitrate transport. New Phytol. 204, 74–80.
Lv, H. X., Zhou, G. S., Ding, Z. H., Sun, Y. J., and Yu, S. B. (2010). QTL Identification for nitrogen responses in rice chromosomal segment substitution lines. Mol. Plant Breed. 8, 1074–1081.
Meng, L. J., Lin, X. Y., Wang, J. M., Chen, K., Cui, Y. R., Xu, J., et al. (2013). Simultaneous improvement of cold tolerance and yield of temperate japonica rice (Oryza sativa L.) by introgression breeding. Plant Breed. 132, 604–612. doi: 10.1111/pbr.12097
Namai, S., Toriyama, K., and Fukuta, Y. (2009). Genetic variation in dry matter production and physiological nitrogen use efficien-cy in rice (Oryza sativa L.) varieties. Breed Sci. 59, 269–276.
Pandey, S. (1997). “Rainfed lowland rice research: challenges and priorities for the 21st century,” in Breeding Strategies for Rainfed Lowland Rice in Drought-prone Environments, eds S. Fukai, M. D. Cooper, and J. Salisbury (Canberra: Australian Centre for International Agricultural Research), 1–12.
Qu, P. P., Shi, J. H., Chen, T. X., Chen, K., Shen, C. C., Wang, J. K., et al. (2020). Construction and integration of genetic linkage maps from three multi-parent advanced generation inter-cross populations in rice. Rice 13:13. doi: 10.1186/s12284-020-0373-z
Senapathy, S., Kunnummal, K. V., Palaniappan, M., and Marappa, M. (2008). QTL and QTL× environment effects on agronomic and nitrogen acquisition traits in rice. J. Integr. Plant Biol. 50, 1108–1117. doi: 10.1111/j.1744-7909 2008.00713.x
Shan, Y. H., Wang, Y. L., and Pan, X. B. (2005). Mapping of QTLs for nitrogen use efficiency and related traits in rice. Sci. Agric. Sinica 4, 721–727.
Tang, J. Y., Zhang, T., Jiang, K. F., Yang, L., Yang, Q. H., Wan, X. Q., et al. (2011). Identification of QTL for yield traits of low nitrogen stress by using introgression lines of rice. J. Agric. Biotech. 19, 996–1002.
Thomson, M. J. (2014). High-throughput SNP genotyping to accelerate crop improvement. Plant Breed. Biotechnol. 2, 195–212. doi: 10.9787/PBB.2014.2.3.195
Tian, F., Bradbury, P. J., Brown, P. J., Hung, H. Y., Sun, Q., Flintgarcia, S., et al. (2015). Genome-wide association study of leaf architecture in the maize nested association mapping population. Nat. Genet. 43, 159–162. doi: 10.1038/ng.746
Tong, H. H., Chen, L., Li, W. P., Xing, Y. Z., Yu, X. Q., Xu, X. Y., et al. (2011). Identification and characterization of quantitative trait loci for grain yield and its components under different nitrogen fertilization levels in rice (Oryza sativa L.). Sci. Agric. Sinica 28, 495–509. doi: 10.1007/s11032-010-9499-9
Tong, H. H., Mei, H. W., Yu, X. Q., Xu, X. Y., Li, M. S., Zhang, S. Q., et al. (2006). Identification of related QTLs at late developmental stage in rice (Oryza sativa L.) under two nitrogen levels. Acta Genet. Sin. 33, 458–467. doi: 10.1016/S0379-4172(06)60073-5
Wang, K., Cui, K. H., Liu, G. L., Xie, W. B., Yu, H. H., Pan, J., et al. (2014). Identification of quantitative trait loci for phosphorus use efficiency traits in rice using a high density SNP map. BMC Genet. 15:155. doi: 10.1186/s12863-014-0155-y
Wang, Y., Sun, Y. J., Chen, D. Y., and Yu, S. B. (2009). Analysis of quantitative trait loci in response to nitrogen and phosphorus deficiency in rice using chromosomal segment substitution lines. Acta. Agron. Sin. 35, 580–587. doi: 10.1016/S1875-2780(08)60072-3
Wang, Y., Zhang, L. B., Nafisah, A., Zhu, L. H., Xu, J. L., and Li, Z. K. (2013). Selection efficiencies for improving drought/salt tolerances and yield using introgression breeding in rice (Oryza sativa L.). Crop J. 1, 134–142. doi: 10.1016/j.cj.2013.07.006
Wei, D., Cui, K. H., Pan, J. F., Ye, G. Y., Xiang, J., Nie, L., et al. (2011). Genetic dissection of grain nitrogen use efficiency and grain yield and their relationship in rice. Field Crops Res. 124, 340–346. doi: 10.1016/j.fcr.2011.07.003
Wei, D., Cui, K. H., Ye, G. Y., Pan, J. F., Xiang, J., Huang, J., et al. (2012). QTL mapping for nitrogen-use efficiency and nitrogen deficiency tolerance traits in rice. Plant Soil. 359, 281–295. doi: 10.1007/s11104-012-1142-6
Wei, J. L., and Xu, S. Z. (2016). A random model approach to QTL mapping in multi-parent advanced generation inter-cross (MAGIC) populations. Genetics 202, 471–486.
Yan, J. B., Yang, X. H., Shah, T., Sánchez, H. V., Li, J. S., Warburton, M., et al. (2010). High-through put SNP genotyping with the golden gate assay in maize. Mol. Breed. 25, 441–451. doi: 10.1007/s11032-009-9343-2
Yoshida, S. (1981). Fundamentals of rice crop science. Philippines: International Rice Research Institute, 135–147.
Yu, J. M., Holland, J. B., McMullen, M. D., and Buckler, E. S. (2008). Genetic design and statistical power of nested association mapping in maize. Genetics 178, 539–551. doi: 10.1534/genetics.107.074245
Zhang, J., Liu, Y., Zhang, N., Hu, B., Jin, T., Xu, H., et al. (2019). NRT1.1B is associated with root microbiota composition and nitrogen use in field-grown rice. Nat. Biotechnol. 37, 676–684.
Zhang, Q. F. (2007). Strategies for developing green super rice. Proc. Natl. Acad. Sci. USA. 104, 16402–16409. doi: 10.1073/pnas.0708013104
Zhao, C. F., Zhou, L. H., Zhang, Y. D., Zhu, Z., Chen, T., Zhao, Q. Y., et al. (2014). QTL mapping for seedling traits associated with low-nitrogen tolerance using a set of advanced backcross introgression lines of rice. Plant Breed. 133, 189–195. doi: 10.1111/pbr.12123
Keywords: GWAS, interconnected breeding population, nitrogen deficiency tolerance, favorable allele mining, pyramiding breeding
Citation: Shen C, Chen K, Cui Y, Chen J, Mi X, Zhu S, Zhu Y, Ali J, Ye G, Li Z and Xu J (2021) QTL Mapping and Favorable Allele Mining of Nitrogen Deficiency Tolerance Using an Interconnected Breeding Population in Rice. Front. Genet. 12:616428. doi: 10.3389/fgene.2021.616428
Received: 12 October 2020; Accepted: 04 March 2021;
Published: 06 April 2021.
Edited by:
Genlou Sun, Saint Mary’s University, CanadaReviewed by:
Te-Ming (Paul) Tseng, Mississippi State University, United StatesShaoqing Li, Wuhan University, China
Copyright © 2021 Shen, Chen, Cui, Chen, Mi, Zhu, Zhu, Ali, Ye, Li and Xu. This is an open-access article distributed under the terms of the Creative Commons Attribution License (CC BY). The use, distribution or reproduction in other forums is permitted, provided the original author(s) and the copyright owner(s) are credited and that the original publication in this journal is cited, in accordance with accepted academic practice. No use, distribution or reproduction is permitted which does not comply with these terms.
*Correspondence: Jianlong Xu, xujlcaas@126.com; Yanru Cui, yanrucui0427@163.com
†These authors have contributed equally to this work