- 1Pramongkutklao Hospital and College of Medicine, Bangkok, Thailand
- 2Department of Tropical Pathology, Faculty of Tropical Medicine, Mahidol University, Bangkok, Thailand
- 3Center of Excellence for Antibody Research, Faculty of Tropical Medicine, Mahidol University, Bangkok, Thailand
Background: Beyond non-genetic risk factors, familial hypercholesterolemia (FH) plays a major role in the development of CHD. FH is a genetic disorder characterized by heritable and severely elevated levels of low-density lipoprotein (LDL) cholesterol, which can lead to premature cardiovascular disease, particularly familial coronary heart disease (FH-CHD).
Method: To explore genes indicating a risk of familial (premature) coronary heart disease (FH-CHD) development in FH, 30 Thai male volunteers were enrolled: 7 healthy controls (N), 6 patients with hypercholesterolemia (H), 4 with FH, 10 with CHD, and 3 with FH-CHD. Transcriptome data were investigated using next-generation sequencing analysis in whole blood (n = 3). Genes that were significantly expressed in both FH and FH-CHD, but not in N, H, and CHD groups, were selected and functionally analyzed.
Results: The findings revealed that 55 intersecting genes were differentially expressed between FH and FH-CHD groups. Ten of the 55 genes (MAPK14, TRPM2, STARD8, PDLIM5, BCL3, BLOC1S5, GBA, RBMS1, CD14, and CD36 were selected for validation. These 10 genes play potential roles in chronic inflammation and are involved in pathways related to pathogenesis of CHD. Using quantitative real-time PCR, we evaluated the mRNA expression of the selected genes in all 30 volunteers. TRPM2, PDLIM5, BCL3 were significantly upregulated and GBA was significantly downregulated in both FH and FH-CHD compared with the N, H, and CHD groups.
Conclusion: our preliminary investigation reveals that the TRPM2, PDLIM5, BCL3, and GBA genes may have potential for further development as predictive markers for FH-CHD.
Introduction
Atherogenesis and the complication of coronary heart disease (CHD) involve a long preclinical process and are poorly understood. Diverse risk factors have been for CHD, including behavioral, dietary, and lifestyle factors such as smoking, fatty dietary intake, physical activity level, infection (exogenous exposure), change of endogenous blood compositions such as lipid and lipoprotein, inflammation and coagulation factors, intermediary metabolites, and oxidant markers of stress, obesity, blood pressure, and diabetes mellitus (Anand et al., 2008; Weber and Noels, 2011). Beyond these non-genetic risk factors, familial hypercholesterolemia (FH) has a major role in development of CHD (Scheuner, 2003). FH is a genetic disorder characterized by heritable and severely increased levels of low-density lipoprotein (LDL) cholesterol, which can lead to premature cardiovascular disease, particularly familial coronary heart disease (FH-CHD) (Anand et al., 2008; Weber and Noels, 2011). Several previous investigations have reported that in FH, expression of at least four genes in sterol and lipoprotein pathways, including LDL receptors, apolipoprotein (apo) B, proprotein convertase subtilisin/kexin9, and the autosomal recessive hypercholesterolemia adaptor protein, are disordered in cholesterol metabolisms, e.g., in sterol and lipoprotein pathways (Defesche, 2001; Marais, 2004). Moreover, other roles of these receptors and related receptors and other genes associated with pathophysiology of disease are of interest in evaluating the risk of developing FH-CHD. In this study, we attempted to identify alternative biomarkers for risk of FH-CHD. We conducted a cross-sectional study using Thai male volunteers, including healthy controls (N) and patients with hypercholesterolemia (H), FH, CHD, and FH-CHD. Transcriptome data were analyzed by next-generation sequencing analysis in whole blood (n = 3/group) and validated by quantitative real-time PCR (qPCR). We selected genes that were significantly expressed in patients with FH and FH-CHD but not in the N, H, and CHD groups to create an intersecting gene profile and then functionally analyzed selected genes. We identified 55 intersecting genes between FH and FH-CHD groups. Similar to our previous study (Maneerat et al., 2017), the expressions of intersecting genes that shared between FH and FH-CHD groups are potential for further development as predictive markers for FH-CHD in FH patients. In this study, we selected that 10 of 55 genes, MAPK14, TRPM2, STARD8, PDLIM5, BCL3, BLOC1S5, GBA, RBMS1, CD14, and CD36 showed significant co-expression and potentially play roles in chronic inflammation and pathways related to pathogenesis of CHD. The selected genes were further validated in 30 volunteer samples using qPCR. The result revealed that TRMS2, PDLIM5, BCL3, CD14, and GBA genes were the most strongly associated with FH-CHD development and show potential for further application as inflammatory markers to predict the risk of FH-CHD development in Thai patients with FH.
Materials and Methods
Subjects
Thirty volunteers, males born to Thai parents, were enrolled in this study. Healthy volunteers who had no infections and underlying diseases or cardiovascular disease (CVD) risk factors were recruited as controls (N group; n = 7). Twenty-three patients were diagnosed, classified, treated and selected under supervision of a specialist (KP) at Pramongkutklao Hospital. They were classified into four groups based on their clinical manifestations according to the American College of Cardiology/American Heart Association criteria (2013) (Stone et al., 2014), and included 6 patients with high cholesterol levels [total cholesterol (TC), LDL, and high-density lipoprotein (HDL)], but with no evidence of vital organ dysfunction (H group) (Stone et al., 2014); 10 patients diagnosed with CHD (Stone et al., 2014) who were about to undergo coronary bypass grafting (CHD group); 3 patients with FH-CHD and 4 FH patients who were related to the 3 FH-CHD.
The workflow followed in the present study is illustrated in Figure 1. The study was performed at the Faculty of Tropical Medicine, Mahidol University. Approval for the study was considered from the Ethics Committees of the Faculty of Tropical Medicine, Mahidol University (MUTM2017-025-02), and Pramongkutklao Hospital (Q031b/59). Before enrollment, all participants were informed of the study aims, and filled an informed consent form.
Blood Sample Collection and Methods
Heparinized blood samples (5 mL) were obtained once from healthy controls and all patients before hyperlipidemia treatment or coronary bypass grafting. Sera from 1 mL of clotted blood were collected for lipid measurement.
Packed blood cells were resuspended in 5 mL of Dulbecco’s-PBS (Wisent Inc., Quebec, Canada). Approximately 1 mL of blood suspension was immediately used to extract total RNA using QIAamp RNA Blood Mini kit (Qiagen Inc., Germantown, MD, United States). RNA samples were kept at −70°C to investigate expression of genes profiled by next-generation sequencing (NGS) and further validated by qPCR.
Lipid profiles, TC, triglycerides (TG), LDL cholesterol (LDL-c), and HDL cholesterol (HDL-c), were analyzed enzymatically using kits (Randox Laboratories Ltd., Crumlin, United Kingdom) and a biochemistry analyzer (Architect CI 16200, Abbott Laboratories, Abbott Park, IL, United States).
cDNA Library Construction and Sequencing by NGS Technique
All total RNA samples were examined for amount and quality before analysis. Integrity of total RNA was assessed using Agilent 2100 Bioanalyzer (Agilent Technologies, Santa Clara, CA, United States). Approximately 500 ng of the total RNA from each sample was used to create individually indexed strand-specific RNA-seq libraries using TruSeq stranded mRNA library preparation kit (Illumina Inc., San Diego, CA, United States). Briefly, poly-A-containing mRNA molecules was captured using magnetic oligo (dT) beads, purified, and directed to cDNA synthesis. AMPure XP beads (Beckman Coulter Genomic, Atlanta, GA, United States) were used to separate the cDNA from reaction mix. Indexing adapters were ligated to the cDNA, and all cDNA libraries were checked for quality using an Agilent 2100 Bioanalyzer (Agilent Technologies) and quantified with DeNovix fluorometer (DeNovix Inc., Wilmington, DE, United States). The indexed sequencing libraries were pooled in equimolar quantities and subjected to cluster generation and paired-end 2 × 75 nucleotide read sequencing on an Illumina NextSeq 500 sequencer. The sequencing process was carried out at Omics Sciences and Bioinformatics Center (Bangkok, Thailand).
Differential Expression Analyses of RNA-Seq Data and Statistical Methods
Bioinformatics analyses comprised an initial quality check of the raw data files using FASTQC software (Bioinformatics Group, Babraham Institute, Cambridge, United Kingdom). Adapter and low-quality reads were removed using Trimmomatic1 (Bolger et al., 2014). The filtered reads were aligned to a human reference genome using HISAT2 aligner software (Center for Computational Biology, Johns Hopkins University, Baltimore, MD, United States). StringTie (Center for Computational Biology, Johns Hopkins University) was used to assemble transcripts from RNA-seq reads that were aligned to the genome, reconstructing all isoforms expressed from each gene as well as estimates of the relative abundance of those isoforms. Subsequently, differential isoform expression among five groups was performed using edgeR program (Robinson et al., 2010) via Cuffdiff 2.0 (Trapnell et al., 2013) (University of Maryland Center for Bioinformatics and Computational Biology)2. Fold change ≥ 1, p-value ≤ 0.05, and false discovery rate (FDR) ≤ 0.05 was interpreted statistically significant. In addition, the gffcompare utility (Pertea and Pertea, 2020) (Center for Computational Biology, Johns Hopkins University, Baltimore, MD, United States)3. StringTie (Center for Computational Biology, Johns Hopkins University) was used to discover a novel transcript. Gene Ontology (GO) and pathway enrichment analyses was conducted using a web-based bioinformatics tool DAVID (omicX, Seine Innopolis, Le-Petit-Quevilly, France).
Validation of Possible Marker Genes for Risk of FH-CHD by RT-qPCR
Fifty-five intersecting genes, significantly expressed in both FH and FH-CHD but not in N, H, and CHD groups, were selected for further validation (Figure 2). qPCR of the mRNA expression of 10 selected genes was performed in all volunteer samples. These genes were MAPK14, TRPM2, STARD8, PDLIM5, BCL3, BLOC1S5, GBA, RBMS1, CD14, and CD36, as described in Table 1 (in bold). Table 2 lists the primers designed to amplify these genes and their expected fragment lengths. qPCR was conducted in triplicate (Susomboon et al., 2006). Each 10-μL PCR reaction contained 5 μL of iTaq universal SYBR Green supermix (BioRad Laboratories Inc., Hercules, CA, United States) mixed with 100 ng of cDNA and 10 μM of each set of forward and reverse primers (Table 2). Amplification was run in a Bio-Rad CFX96 Real-time system (BioRad Laboratories Inc.). The qPCR conditions were 95°C for 3 min, followed by 35 cycles of denaturation at 95°C for 30 s, annealing at 60°C for 30 s, and melting curve analysis at 65°C for 5 min. β-Actin (ACTB) (primers: forward: 5′-TCACCCACACTGTGCCCATCTACGA-3′ and reverse: 5′-CAGCGGAACCGCTCATTGCCAATGG-3′) was used to normalize the relative expression of each gene (Heid et al., 1996; Kotepui et al., 2012) and the relative expression level was calculated using the 2–ΔΔCt method.
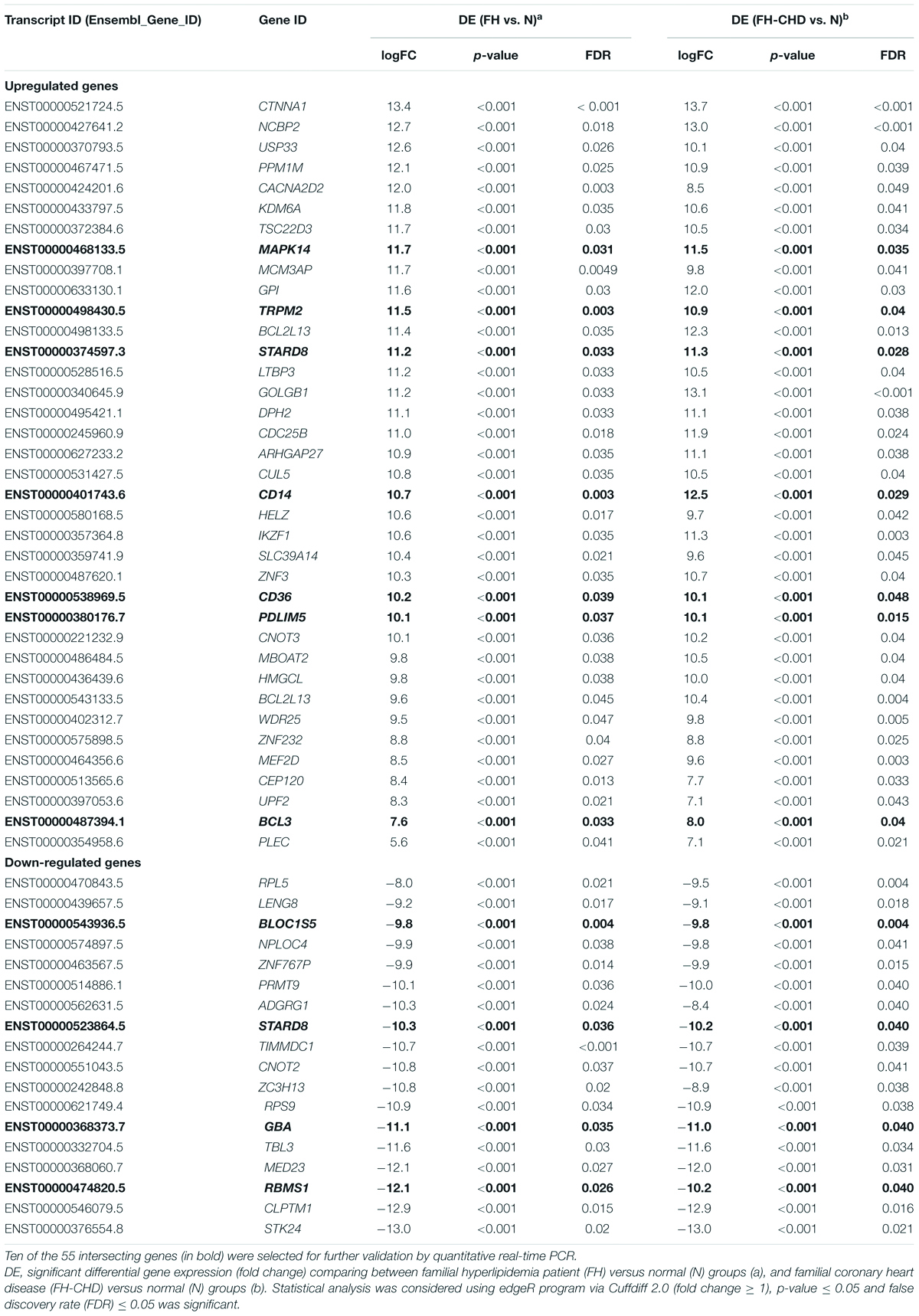
Table 1. Fifty-five intersecting genes expressed in patients in the FH and FH-CHD groups but not in the N, H, and CHD groups, including 37 significantly up-regulated and 18 down-regulated genes.
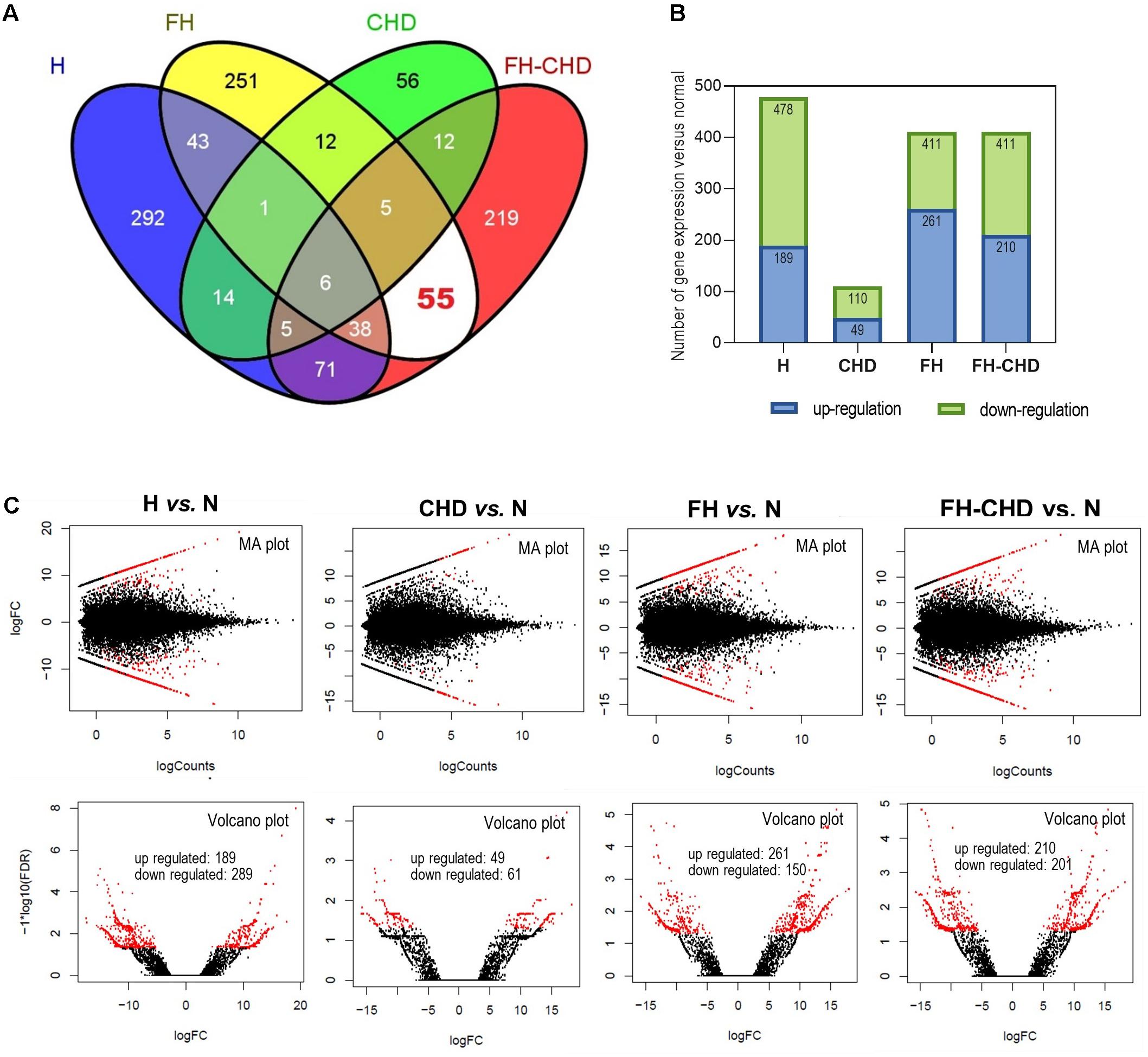
Figure 2. Transcriptome analysis by next-generation sequencing in four patient groups, hyperlipidemia (H), familial hyperlipidemia (FH), coronary heart disease (CHD), and familial CHD (FH-CHD) compared with healthy volunteers (N). Total RNA was extracted from blood (n = 3). Differentially expressed genes were performed using edgeR program via Cuffdiff 2.0 (fold change ≥ 1), p-value ≤ 0.05 and false discovery rate (FDR) ≤ 0.05. (A) Venn diagram illustrates all 1,080 differentially expressed genes, and (B) number of up- and downregulated genes expressed in the four patient groups compared with N. [(C), upper panels] MA plots for differential expression analysis in H, CHD, FH, or FH-CHD versus N samples. The y- and x-axes represent the log fold change (FC) of gene expression and log number of genes, respectively. The red and black points in the plot indicate significant and not significant differentially expressed genes, respectively. [(C), lower panels] Volcano plots of differentially expressed transcripts in H, CHD, FH, or FH-CHD versus N samples. The y- and x-axes represent the FDR value and log FC of gene expression, respectively. The filter threshold is FDR < 0.0001. The red and black points in the plot represent up- and downregulated transcripts, respectively.
Results and Discussion
Characteristics of Healthy Controls and Patients
General descriptions and clinical characteristics of the four patient groups and controls are presented as medians and ranges (Table 3).
Preselection of Regulatory Sequences
Transcriptome sequences of healthy volunteers (N) and patients in the H, FH, CHD, and FH-CHD were analyzed. We found approximately 500 differentially expressed genes in the patient groups compared with the healthy controls, as illustrated in a Venn diagram (Figure 2A). The number of significantly up- and down-regulated genes in the four patient groups compared with the N group are displayed in bar graphs (Figure 2B) and volcano plots (Figure 2C).
Intersecting and Selected Genes
To pick up sequence group to identify predictive markers for FH-CHD risk in the FH group, we focused on the 55 genes that intersected between FH and FH-CHD (white area in Figure 2A). The intersecting gene profiles include 37 up- and 18 down-regulated genes (Table 1). Gene Ontology and pathway enrichment analyses were conducted and the results are shown in Figure 3. The GO terms were in three categories: biological process, cellular component, and molecular functions (Figure 3A). The number of up- and down-regulated genes in each category is shown in Figure 3B.
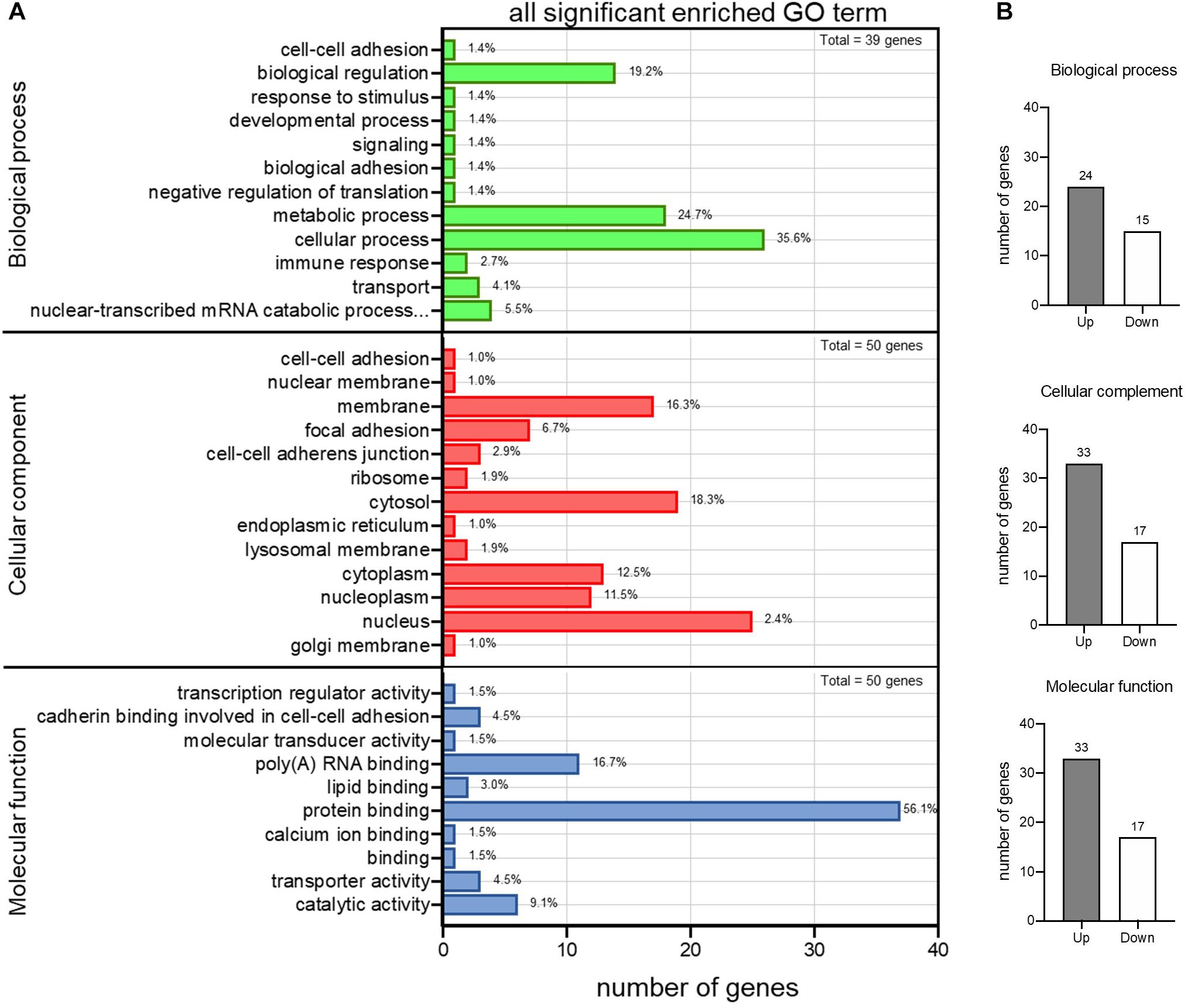
Figure 3. Gene Ontology (GO) enrichment analysis of differential expression of 55 intersecting genes in the familial hyperlipidemia (FH) and familial coronary heart disease (FH-CHD) versus N groups. (A) The ordinate represents the next level GO term of the three categories including biological process (upper panel), cellular component (middle panel), and molecular functions (lower panel). The abscissa represents the gene number under the term. (B) Bar graphs show number of upregulated (gray bar) and downregulated (white bar) genes in each category.
To investigate the importance of the intersecting genes, we conducted functional enrichment analyses of genes differentially expressed at the mRNA level analyzed by Metascape pathway4. We observed functional correlations between the top 11 clusters in the 55 intersecting genes between FH and FH-CHD; the genes were mainly involved in lipid metabolism, cellular process, oxidative stress, and inflammation (Figure 4A). Focusing on 10 differentially expressed genes, 5 clusters are displayed as the same network enrichment analysis of the 55 intersecting genes (Figure 4B). In addition, the expected protein–protein interaction networks in the 55 intersecting genes and the 10 selected genes analyzed by web-based bioinformatics tool are shown in Figures 5A,B, respectively.
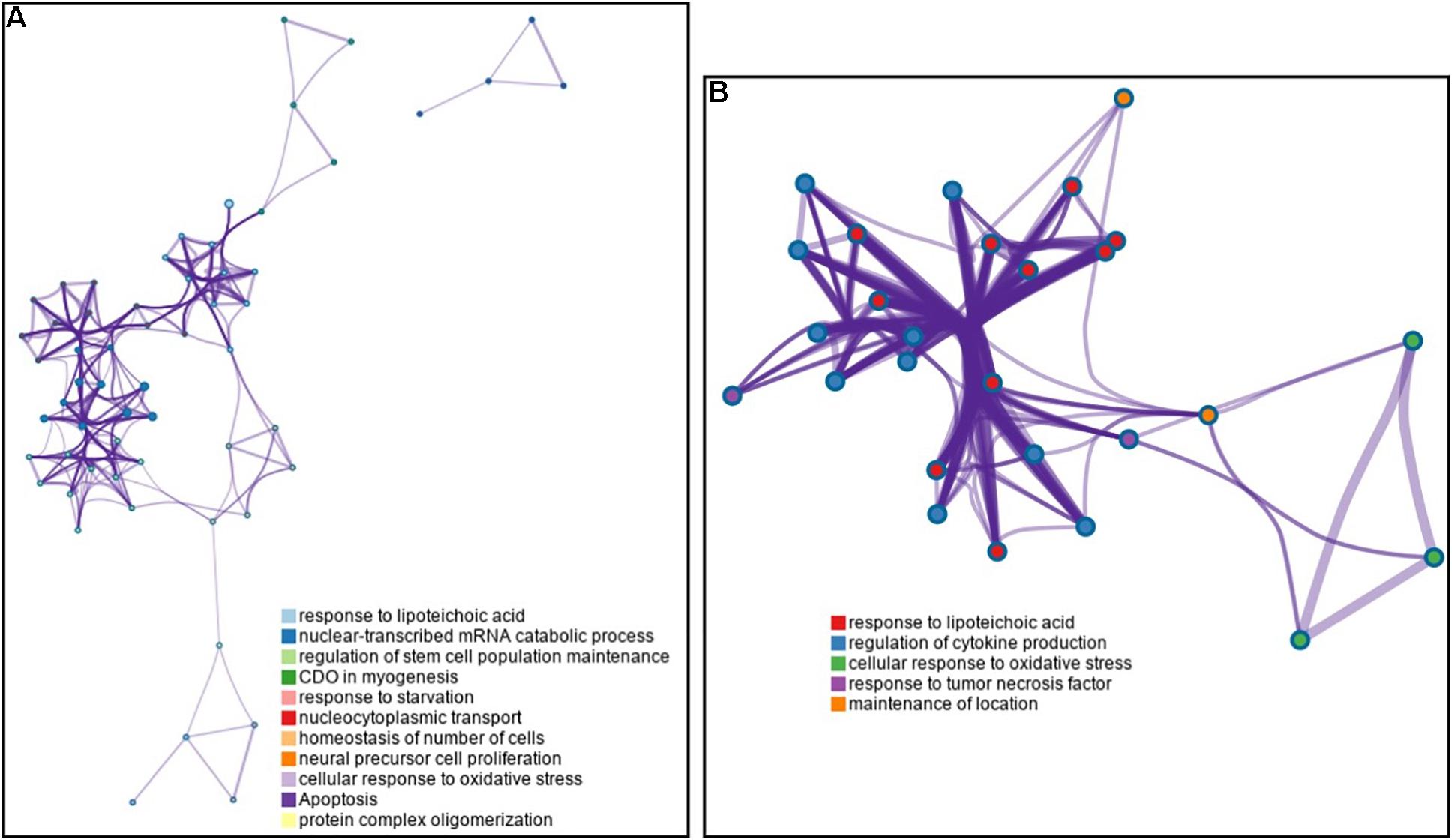
Figure 4. Functional enrichment analyses of differentially expressed genes at the mRNA level analyzed by Metascape pathway. (A) Association between the top 11 clusters and (B) 5 clusters of enriched terms displayed as a network enrichment analysis of 55 intersected and of 10 selected differentially expressed mRNAs, respectively. Nodes of the same color belong to the same cluster. Terms with a similarity score > 0.3 are linked by an edge. The network was visualized with Cytoscape with force-directed layout and edge bundled for clarity. The analysis was conducted using the web-based bioinformatics tool DAVID (omicX, Seine Innopolis, Le-Petit-Quevilly, France).
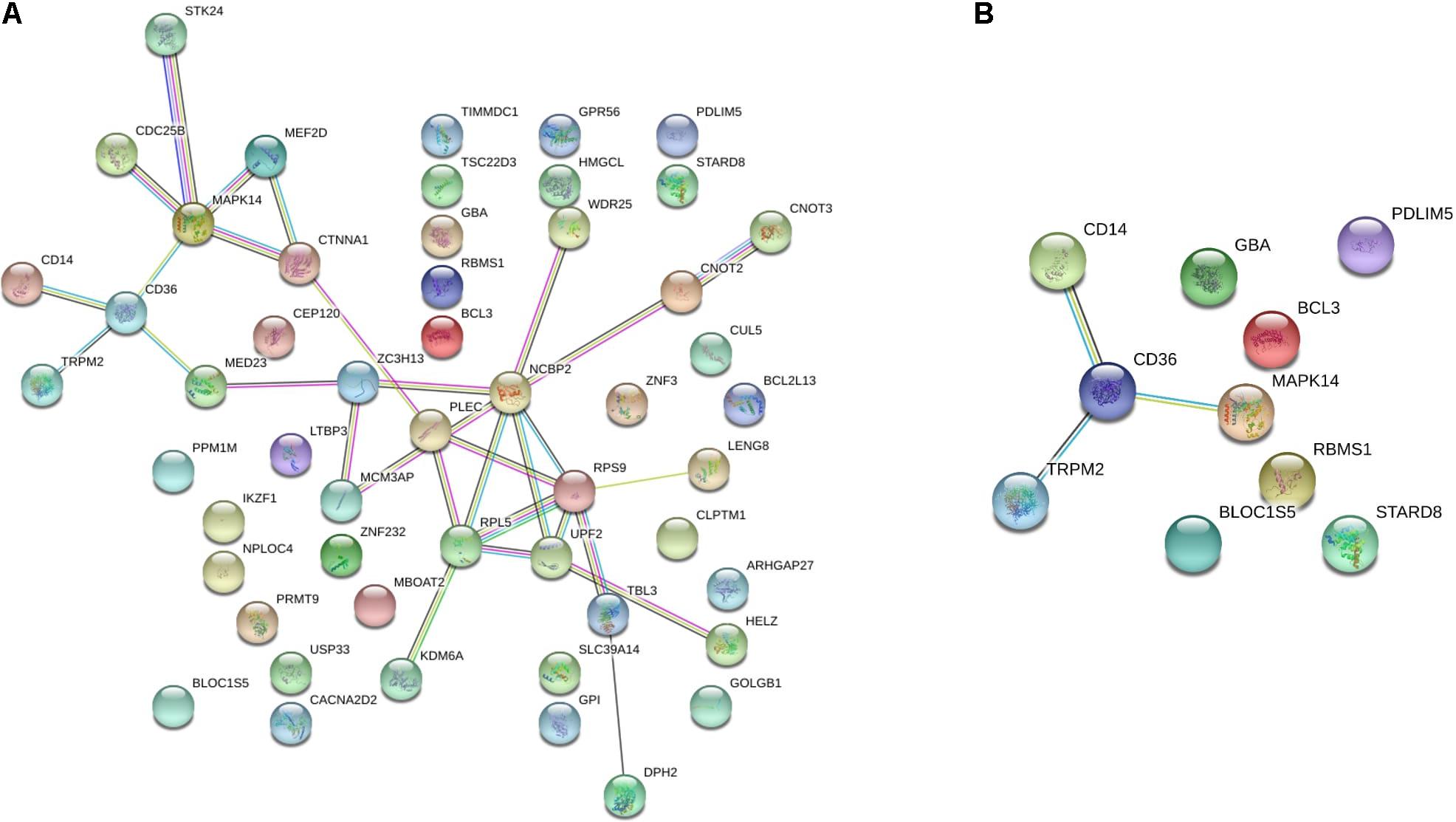
Figure 5. The protein–protein interaction network of (A) the 55 intersecting genes between familial hyperlipidemia (FH) and familial coronary heart disease (FH-CHD) groups, and (B) in 10 genes selected from the 55 intersecting genes using web-based bioinformatics tool: functional protein association networks analysis.
Verification of 10 Selected Genes Using RT-qPCR
Based on focusing regulatory and immune response roles of target genes, we selected 10 genes potentially involved in atherogenesis, chronic inflammation, or lipid metabolism. These genes include MAPK14, TRPM2, STARD8, PDLIM5, BCL3, BLOC1S5, GBA, RBMS1, CD14, and CD36. Table 4 summarizes the selected genes and their cardiovascular syndrome (CVS)-related functions. In Figure 6, we compared the expression of these genes among groups (Figure 6A) and detected high co-expression between CD14 and BCL3, between TRMP2 and CD14, and between CD14 and CD36 (Figure 6B), which may indicate a possible further extension of our indicative synergistic markers for risk of FH-CHD. The qPCR results of relative mRNA expression (mean two-fold changes) of the 10 genes in all groups using total RNA extracted from blood of volunteers are shown in Figure 7. The mRNA expression of these genes was compared among the groups. To identify ideal biomarkers, we selected only genes that were significantly up- or down-regulated in patients with FH and FH-CHD compared with healthy individuals (not differently expressed between FH and FH-CHD). The results reveal that TRPM2, PDLIM5, BCL3, and CD14 were up-regulated and GBA was down-regulated in both FH and FH-CHD compared with the N, H and CHD groups. These five target genes have potential as markers for risk of FH-CHD in FH.
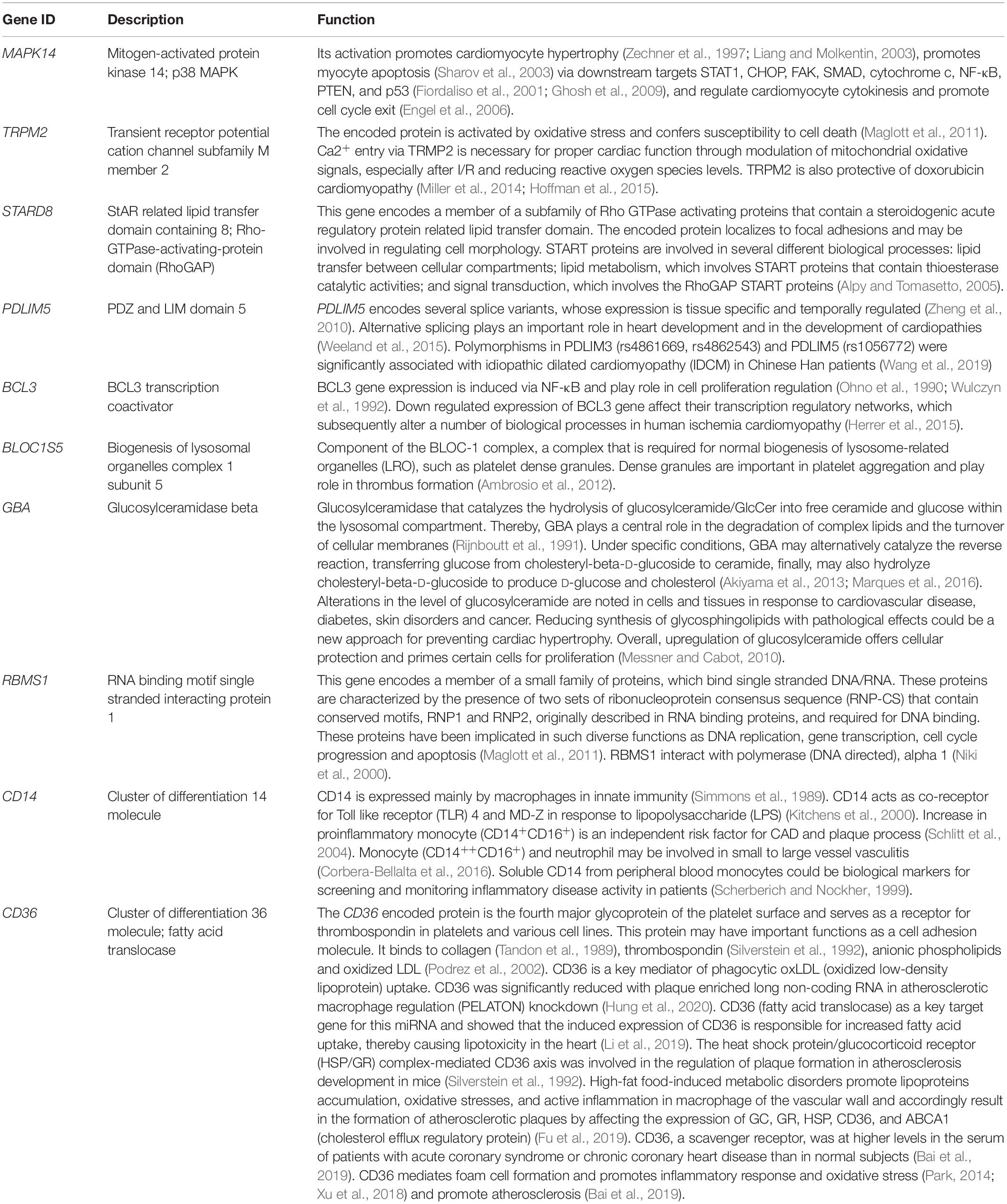
Table 4. Description and potential functions of 10 genes selected from the 55 intersecting genes expressed in both FH and FH-CHD but not in N, H, and CHD groups.
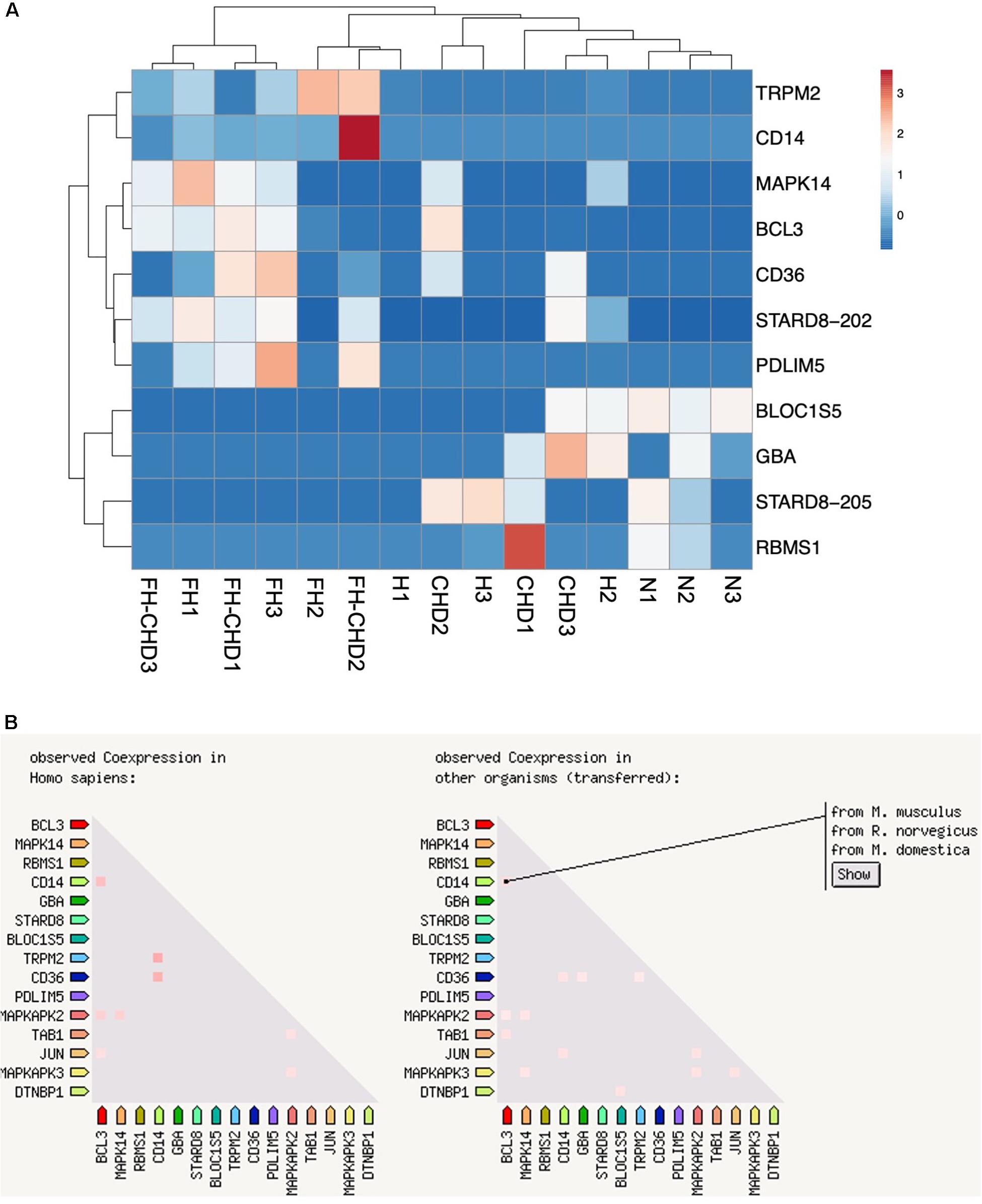
Figure 6. Ten selected genes from 55 intersecting genes between familial hyperlipidemia (FH) and familial coronary heart disease (FH-CHD) groups. (A) Heat maps of the 10 differentially expressed transcripts in the patient groups versus the normal control group (n = 3). (B) Co-expression of 10 selected genes. Note the high co-expression between CD14 and BCL3, between TRMP2 and CD14, and between CD14 and CD36 (left panel) in Homo sapiens and in other organisms (right panel).
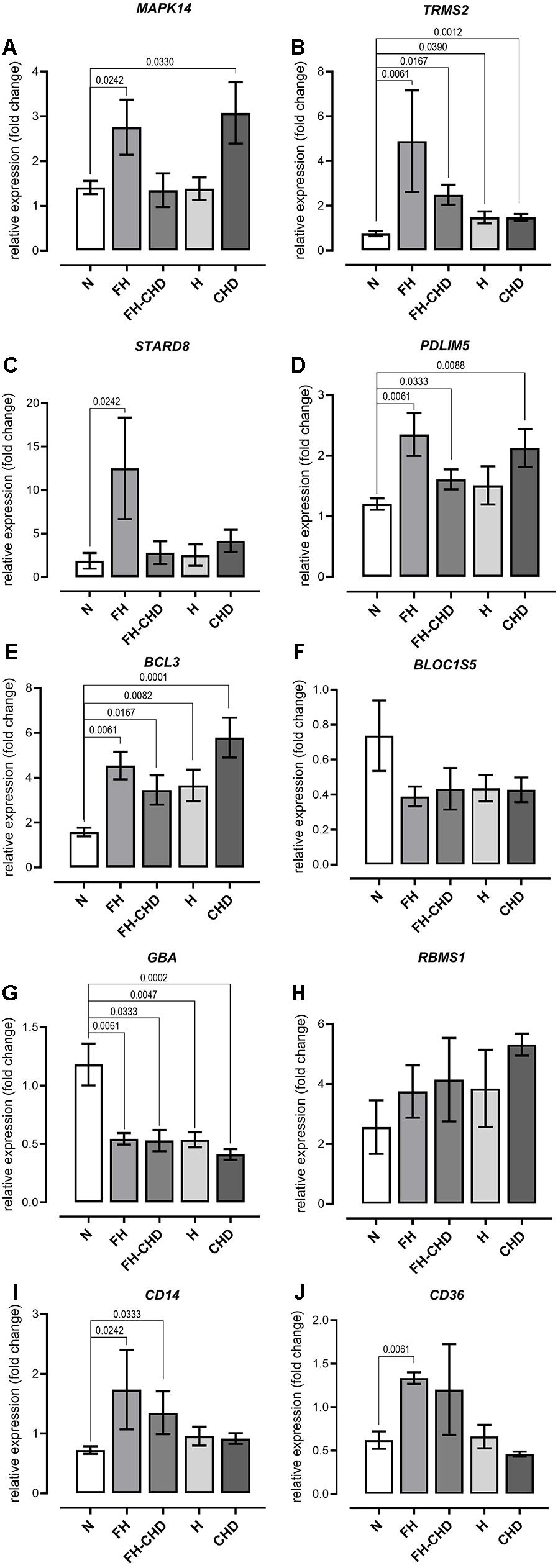
Figure 7. Quantitative reverse transcription PCR analysis of mRNA expression of (A) MAPK14, (B) TRPM2, (C) STARD8, (D) PDLIM5, (E) BCL3, (F) BLOC1S5, (G) GBA, (H) RBMS1, (I) CD14, and (J) CD36 genes, showing altered expression in patient groups vs. controls. mRNA expression (2.0-fold change) relative to ACTB mRNA in RNA samples obtained from the patient groups; familial hyperlipidemia (FH; n = 4), familial coronary heart disease (FH-CHD; n = 3), hyperlipidemia (H; n = 6), and coronary heart disease (CHD; n = 10) compared with the healthy (N) group (n = 7). Bracketed p-values indicate significant differences between groups.
The present study had some limitations; (1) We used whole blood to prepare transcriptome data. Therefore, our data lacks cardiac genes (reviewed in Saucerman et al., 2019) that play role in cardiovascular system but not mainly express in blood cells. In our opinion, atherosclerosis and its complication, coronary heart disease and stroke are consequence of chronic inflammation of vascular wall (Hansson, 2005). Blood contains inflammatory cells, which play an important role in atherogenesis. Therefore, blood is an accessible source particularly fitting surrogate for atherosclerotic tissue (Moore et al., 2005; Kang et al., 2006; Sharp et al., 2007). In consistence, Perisic et al., 2016 demonstrated similar gene expression profiles in carotid atherosclerosis compared between PBMC and vascular tissue sources (Perisic et al., 2016). These approaches contribute that our study using blood to investigate the transcriptome data is feasible; (2) we focused only on male volunteers to control the influence of the sex-hormone factor. Estrogens, in particular, are primary examples of female sex steroids. Previous studies in animal models, e.g., with rabbits, mice, and monkeys, have reported that estrogen has protective effects in CVD. Earlier evidence has also indicated that estrogen protects women against CHD pre-menopause (reviewed in Arnal et al., 2007). Estrogen ameliorates lipidaemia by up-regulating LDL-receptor production. In addition, estrogen attenuates inflammation in the atherosclerotic plaque. It is exerted via the sex hormone receptors on various inflammatory cells in the plaques, including reduced LDL oxidation, EC activation and the adhesion of neutrophils and monocytes to the endothelial lining, and impedes nitric oxide activity (Boese et al., 2017). We expect that our preliminary findings will help further studies to find appropriate biomarkers to predict CHD in both male and female Thai hyperlipidemia patients; (3) we illustrate our findings with low statistical power due to the small genetic sample size and single-center analysis. Further studies with a larger sample size and multi-center analysis in genotypic and phenotypic expressions of the 10 potential genes will be needed to clarify our current findings; (4) We conducted this study using a single ethnic background cohort. Ethnicity is a source of health inequalities. In particular, ethnic inheritance influences the occurrence rates of different cardiovascular disorders (Scarborough et al., 2010). In this study, we performed a small preliminary study only in Thai ethnicities to elucidate appropriate biomarkers to predict FH-CHD. We expect that further studies in larger sample sizes and multi-center Thai male and female populations will help to confirm the feasibility of using the FH-CHD biomarker in Thai ethnicities. Moreover, further meta-analysis studies using publicly available transcriptomic data from different ethnic populations to compare and share appropriate CHD biomarker is expected to be more reliable and advantageous; (5) Several current studies into the genetic basis of coronary heart disease have intensively reported the discovery and aggregation of genetic variants from multiple genes from lipid and other pathologic pathways (Yao et al., 2015; Glicksberg et al., 2019; Cohain et al., 2021). Based on our current NGS data, we are continuing to investigate the variant genes observed only in FH and FH-CHD patient groups, to identify potential predictive markers.
Taken together, based on previous knowledge and our findings, we suggest that among 10 potential markers of FH-CHD risk, TRMS2, PDLIM5, BCL3, CD14, and GBA genes were the most strongly correlated with development of FH-CHD. These show potential for further application as inflammatory markers for the risk of FH-CHD development in Thai patients with FH.
Data Availability Statement
The datasets presented in this study can be found in online repositories. The names of the repository/repositories and accession number(s) can be found below: NCBI BioProject, accession no: PRJNA663423.
Ethics Statement
The studies involving human participants were reviewed and approved by The Ethics Committees of the Faculty of Tropical Medicine, Mahidol University, Bangkok, Thailand and Pramongkutklao Hospital, Bangkok, Thailand. The patients/participants provided their written informed consent to participate in this study.
Author Contributions
KP chose healthy control, patients with hyperlipidemia, familial hyperlipidemia, coronary heart disease (CHD), familial CHD, and performed their coronary bypass grafting. YM and WD were responsible for laboratory work including blood collection and RNA extraction. YM and WD analyzed the NGS data. SB designed primers and conducted the qRT-PCR assays and analyses. WD worked on data analysis and statistical calculations. YM conceived the study and prepared the manuscript. All authors discussed the results, and read and approved the final manuscript.
Funding
This research project is supported by Mahidol University and the Faculty of Tropical Medicine, Mahidol University.
Conflict of Interest
The authors declare that the research was conducted in the absence of any commercial or financial relationships that could be construed as a potential conflict of interest.
Acknowledgments
We are grateful to the volunteers and patients who donated their blood and the staff at the Hospital for Tropical Diseases, Faculty of Tropical Medicine, Mahidol University taking consent from patients and filling out the questionnaire voluntarily. We appreciate Edanz Group Ltd. (www.edanzediting.com) and Mr. Paul Adams, who provided editorial assistance. We also thank Louise Adam, ELS(D), from Edanz Group (www.edanzediting.com/ac) for editing a draft of this manuscript.
Footnotes
- ^ http://www.usadellab.org/cms/?page=trimmomatic
- ^ https://www.genepattern.org/modules/docs/Cuffdiff/7
- ^ https://ccb.jhu.edu/software/stringtie/gffcompare.shtml
- ^ https://metascape.org/gp/index.html#/main/step1
References
Akiyama, H., Kobayashi, S., Hirabayashi, Y., and Murakami-Murofushi, K. (2013). Cholesterol glucosylation is catalyzed by transglucosylation reaction of beta-glucosidase 1. Biochem. Biophys. Res. Commun. 441, 838–843. doi: 10.1016/j.bbrc.2013.10.145
Alpy, F., and Tomasetto, C. (2005). Give lipids a START: the StAR-related lipid transfer (START) domain in mammals. J. Cell Sci. 118(Pt. 13), 2791–2801. doi: 10.1242/jcs.02485
Ambrosio, A. L., Boyle, J. A., and Di Pietro, S. M. (2012). Mechanism of platelet dense granule biogenesis: study of cargo transport and function of Rab32 and Rab38 in a model system. Blood 120, 4072–4081. doi: 10.1182/blood-2012-04-420745
Anand, S. S., Islam, S., Rosengren, A., Franzosi, M. G., Steyn, K., Yusufali, A. H., et al. (2008). Risk factors for myocardial infarction in women and men: insights from the INTERHEART study. Eur. Heart J. 29, 932–940. doi: 10.1093/eurheartj/ehn018
Arnal, J. F., Douin-Echinard, V., Tremollieres, F., Terrisse, A. D., Sie, P., Payrastre, B., et al. (2007). Understanding the controversy about hormonal replacement therapy: insights from estrogen effects on experimental and clinical atherosclerosis. Arch. Mal. Coeur Vaiss. 100, 554–562.
Bai, H. L., Lu, Z. F., Zhao, J. J., Ma, X., Li, X. H., Xu, H., et al. (2019). Microarray profiling analysis and validation of novel long noncoding RNAs and mRNAs as potential biomarkers and their functions in atherosclerosis. Physiol. Genomics 51, 644–656. doi: 10.1152/physiolgenomics.00077.2019
Boese, A. C., Kim, S. C., Yin, K. J., Lee, J. P., and Hamblin, M. H. (2017). Sex differences in vascular physiology and pathophysiology: estrogen and androgen signaling in health and disease. Am. J. Physiol. Heart Circ. Physiol. 313, H524–H545. doi: 10.1152/ajpheart.00217.2016
Bolger, A. M., Lohse, M., and Usadel, B. (2014). Trimmomatic: a flexible trimmer for Illumina sequence data. Bioinformatics 30, 2114–2120. doi: 10.1093/bioinformatics/btu170
Cohain, A. T., Barrington, W. T., Jordan, D. M., Beckmann, N. D., Argmann, C. A., Houten, S. M., et al. (2021). An integrative multiomic network model links lipid metabolism to glucose regulation in coronary artery disease. Nat. Commun. 12:547. doi: 10.1038/s41467-020-20750-8
Corbera-Bellalta, M., Planas-Rigol, E., Lozano, E., Terrades-Garcia, N., Alba, M. A., Prieto-Gonzalez, S., et al. (2016). Blocking interferon gamma reduces expression of chemokines CXCL9, CXCL10 and CXCL11 and decreases macrophage infiltration in ex vivo cultured arteries from patients with giant cell arteritis. Ann. Rheum. Dis. 75, 1177–1186. doi: 10.1136/annrheumdis-2015-208371
Defesche, J. (2001). World health organisation report on familial hypercholesterolemia. Atherosclerosis 154:242. doi: 10.1016/s0021-9150(00)00646-8
Engel, F. B., Hsieh, P. C., Lee, R. T., and Keating, M. T. (2006). FGF1/p38 MAP kinase inhibitor therapy induces cardiomyocyte mitosis, reduces scarring, and rescues function after myocardial infarction. Proc. Natl. Acad. Sci. U.S.A. 103, 15546–15551. doi: 10.1073/pnas.0607382103
Fiordaliso, F., Leri, A., Cesselli, D., Limana, F., Safai, B., Nadal-Ginard, B., et al. (2001). Hyperglycemia activates p53 and p53-regulated genes leading to myocyte cell death. Diabetes 50, 2363–2375. doi: 10.2337/diabetes.50.10.2363
Fu, W., Chen, M., Ou, L., Li, T., Chang, X., Huang, R., et al. (2019). Xiaoyaosan prevents atherosclerotic vulnerable plaque formation through heat shock protein/glucocorticoid receptor axis-mediated mechanism. Am. J Transl. Res. 11, 5531–5545.
Ghosh, J., Das, J., Manna, P., and Sil, P. C. (2009). Taurine prevents arsenic-induced cardiac oxidative stress and apoptotic damage: role of NF-kappa B, p38 and JNK MAPK pathway. Toxicol. Appl. Pharmacol. 240, 73–87. doi: 10.1016/j.taap.2009.07.008
Glicksberg, B. S., Amadori, L., Akers, N. K., Sukhavasi, K., Franzen, O., Li, L., et al. (2019). Integrative analysis of loss-of-function variants in clinical and genomic data reveals novel genes associated with cardiovascular traits. BMC Med. Genomics 12(Suppl. 6):108. doi: 10.1186/s12920-019-0542-3
Hansson, G. K. (2005). Inflammation, atherosclerosis, and coronary artery disease. N. Engl. J. Med. 352, 1685–1695. doi: 10.1056/NEJMra043430
Heid, C. A., Stevens, J., Livak, K. J., and Williams, P. M. (1996). Real time quantitative PCR. Genome Res. 6, 986–994. doi: 10.1101/gr.6.10.986
Herrer, I., Rosello-Lleti, E., Ortega, A., Tarazon, E., Molina-Navarro, M. M., Trivino, J. C., et al. (2015). Gene expression network analysis reveals new transcriptional regulators as novel factors in human ischemic cardiomyopathy. BMC Med. Genomics 8:14. doi: 10.1186/s12920-015-0088-y
Hidaka, M., Wakabayashi, I., Takeda, Y., and Fukuzawa, K. (2013). Vitamin D(3) derivatives increase soluble CD14 release through ERK1/2 activation and decrease IL-8 production in intestinal epithelial cells. Eur. J. Pharmacol. 721, 305–312. doi: 10.1016/j.ejphar.2013.09.014
Hoffman, N. E., Miller, B. A., Wang, J., Elrod, J. W., Rajan, S., Gao, E., et al. (2015). Ca(2)(+) entry via Trpm2 is essential for cardiac myocyte bioenergetics maintenance. Am. J. Physiol. Heart Circ. Physiol. 308, H637–H650. doi: 10.1152/ajpheart.00720.2014
Hung, J., Scanlon, J. P., Mahmoud, A. D., Rodor, J., Ballantyne, M., Fontaine, M. A. C., et al. (2020). Novel plaque enriched long noncoding RNA in atherosclerotic macrophage regulation (PELATON). Arterioscler. Thromb. Vasc. Biol. 40, 697–713. doi: 10.1161/ATVBAHA.119.313430
Ivankovic, D., Chau, K. Y., Schapira, A. H., and Gegg, M. E. (2016). Mitochondrial and lysosomal biogenesis are activated following PINK1/parkin-mediated mitophagy. J. Neurochem. 136, 388–402. doi: 10.1111/jnc.13412
Kang, J. G., Patino, W. D., Matoba, S., and Hwang, P. M. (2006). Genomic analysis of circulating cells: a window into atherosclerosis. Trends Cardiovasc. Med. 16, 163–168. doi: 10.1016/j.tcm.2006.03.006
Khalaf, H., Demirel, I., and Bengtsson, T. (2013). Suppression of inflammatory gene expression in T cells by Porphyromonas gingivalis is mediated by targeting MAPK signaling. Cell Mol. Immunol. 10, 413–422. doi: 10.1038/cmi.2013.23
Kitchens, R. L., Thompson, P. A., O’Keefe, G. E., and Munford, R. S. (2000). Plasma constituents regulate LPS binding to, and release from, the monocyte cell surface. J. Endotoxin Res. 6, 477–482. doi: 10.1179/096805100101532450
Kotepui, M., Thawornkuno, C., Chavalitshewinkoon-Petmitr, P., Punyarit, P., and Petmitr, S. (2012). Quantitative real-time RT-PCR of ITGA7, SVEP1, TNS1, LPHN3, SEMA3G, KLB and MMP13 mRNA expression in breast cancer. Asian Pac. J. Cancer Prev. 13, 5879–5882. doi: 10.7314/apjcp.2012.13.11.5879
Li, H., Fan, J., Zhao, Y., Zhang, X., Dai, B., Zhan, J., et al. (2019). Nuclear miR-320 Mediates diabetes-induced cardiac dysfunction by activating transcription of fatty acid metabolic genes to cause lipotoxicity in the heart. Circ. Res. 125, 1106–1120. doi: 10.1161/CIRCRESAHA.119.314898
Liang, Q., and Molkentin, J. D. (2003). Redefining the roles of p38 and JNK signaling in cardiac hypertrophy: dichotomy between cultured myocytes and animal models. J. Mol. Cell Cardiol. 35, 1385–1394. doi: 10.1016/j.yjmcc.2003.10.001
Lin, R., Wang, Y., Chen, Q., Liu, Z., Xiao, S., Wang, B., et al. (2018). TRPM2 promotes the proliferation and invasion of pancreatic ductal adenocarcinoma. Mol. Med. Rep. 17, 7537–7544. doi: 10.3892/mmr.2018.8816
Maglott, D., Ostell, J., Pruitt, K. D., and Tatusova, T. (2011). Entrez gene: gene-centered information at NCBI. Nucleic Acids Res. 39, D52–D57. doi: 10.1093/nar/gkq1237
Maimaiti, A., Maimaiti, A., Yang, Y., and Ma, Y. (2016). MiR-106b exhibits an anti-angiogenic function by inhibiting STAT3 expression in endothelial cells. Lipids Health Dis. 15:51. doi: 10.1186/s12944-016-0216-5
Maneerat, Y., Prasongsukarn, K., Benjathummarak, S., and Dechkhajorn, W. (2017). PPBP and DEFA1/DEFA3 genes in hyperlipidaemia as feasible synergistic inflammatory biomarkers for coronary heart disease. Lipids Health Dis. 16:80. doi: 10.1186/s12944-017-0471-0
Marques, A. R., Mirzaian, M., Akiyama, H., Wisse, P., Ferraz, M. J., Gaspar, P., et al. (2016). Glucosylated cholesterol in mammalian cells and tissues: formation and degradation by multiple cellular beta-glucosidases. J. Lipid Res. 57, 451–463. doi: 10.1194/jlr.M064923
Messner, M. C., and Cabot, M. C. (2010). Glucosylceramide in humans. Adv. Exp. Med. Biol. 688, 156–164. doi: 10.1007/978-1-4419-6741-1_11
Miller, B. A., Hoffman, N. E., Merali, S., Zhang, X. Q., Wang, J., Rajan, S., et al. (2014). TRPM2 channels protect against cardiac ischemia-reperfusion injury: role of mitochondria. J. Biol. Chem. 289, 7615–7629. doi: 10.1074/jbc.M113.533851
Moore, D. F., Li, H., Jeffries, N., Wright, V., Cooper, R. A. Jr., Elkahloun, A., et al. (2005). Using peripheral blood mononuclear cells to determine a gene expression profile of acute ischemic stroke: a pilot investigation. Circulation 111, 212–221. doi: 10.1161/01.CIR.0000152105.79665.C6
Niki, T., Galli, I., Ariga, H., and Iguchi-Ariga, S. M. (2000). MSSP, a protein binding to an origin of replication in the c-myc gene, interacts with a catalytic subunit of DNA polymerase alpha and stimulates its polymerase activity. FEBS Lett. 475, 209–212. doi: 10.1016/s0014-5793(00)01679-3
Ohno, H., Takimoto, G., and McKeithan, T. W. (1990). The candidate proto-oncogene bcl-3 is related to genes implicated in cell lineage determination and cell cycle control. Cell 60, 991–997. doi: 10.1016/0092-8674(90)90347-h
Park, Y. M. (2014). CD36, a scavenger receptor implicated in atherosclerosis. Exp. Mol. Med. 46:e99. doi: 10.1038/emm.2014.38
Perisic, L., Aldi, S., Sun, Y., Folkersen, L., Razuvaev, A., Roy, J., et al. (2016). Gene expression signatures, pathways and networks in carotid atherosclerosis. J. Intern. Med. 279, 293–308. doi: 10.1111/joim.12448
Pertea, G., and Pertea, M. (2020). GFF Utilities: GffRead and GffCompare. F1000Research 9:304. doi: 10.12688/f1000research.23297.2
Podrez, E. A., Poliakov, E., Shen, Z., Zhang, R., Deng, Y., Sun, M., et al. (2002). A novel family of atherogenic oxidized phospholipids promotes macrophage foam cell formation via the scavenger receptor CD36 and is enriched in atherosclerotic lesions. J Biol. Chem. 277, 38517–38523. doi: 10.1074/jbc.M205924200
Rijnboutt, S., Aerts, H. M., Geuze, H. J., Tager, J. M., and Strous, G. J. (1991). Mannose 6-phosphate-independent membrane association of cathepsin D, glucocerebrosidase, and sphingolipid-activating protein in HepG2 cells. J. Biol. Chem. 266, 4862–4868. doi: 10.1016/s0021-9258(19)67728-8
Robinson, M. D., McCarthy, D. J., and Smyth, G. K. (2010). edgeR: a Bioconductor package for differential expression analysis of digital gene expression data. Bioinformatics 26, 139–140. doi: 10.1093/bioinformatics/btp616
Saucerman, J. J., Tan, P. M., Buchholz, K. S., McCulloch, A. D., and Omens, J. H. (2019). Mechanical regulation of gene expression in cardiac myocytes and fibroblasts. Nat. Rev. Cardiol. 16, 361–378. doi: 10.1038/s41569-019-0155-8
Scarborough, P., Rayner, M., van Dis, I., and Norum, K. (2010). Meta-analysis of effect of saturated fat intake on cardiovascular disease: overadjustment obscures true associations. Am. J. Clin. Nutr. 92, 458–459. doi: 10.3945/ajcn.2010.29504
Scherberich, J. E., and Nockher, W. A. (1999). CD14++ monocytes, CD14+/CD16+ subset and soluble CD14 as biological markers of inflammatory systemic diseases and monitoring immunosuppressive therapy. Clin. Chem. Lab. Med. 37, 209–213. doi: 10.1515/CCLM.1999.039
Scheuner, M. T. (2003). Genetic evaluation for coronary artery disease. Genet Med. 5, 269–285. doi: 10.1097/01.GIM.0000079364.98247.26
Schlitt, A., Heine, G. H., Blankenberg, S., Espinola-Klein, C., Dopheide, J. F., Bickel, C., et al. (2004). CD14+CD16+ monocytes in coronary artery disease and their relationship to serum TNF-alpha levels. Thromb. Haemost. 92, 419–424. doi: 10.1160/TH04-02-0095
Sharov, V. G., Todor, A., Suzuki, G., Morita, H., Tanhehco, E. J., and Sabbah, H. N. (2003). Hypoxia, angiotensin-II, and norepinephrine mediated apoptosis is stimulus specific in canine failed cardiomyocytes: a role for p38 MAPK, Fas-L and cyclin D1. Eur. J. Heart Fail 5, 121–129. doi: 10.1016/s1388-9842(02)00254-4
Sharp, F. R., Xu, H., Lit, L., Walker, W., Pinter, J., Apperson, M., et al. (2007). Genomic profiles of stroke in blood. Stroke 38(Suppl. 2), 691–693. doi: 10.1161/01.STR.0000247940.27518.38
Silverstein, R. L., Baird, M., Lo, S. K., and Yesner, L. M. (1992). Sense and antisense cDNA transfection of CD36 (glycoprotein IV) in melanoma cells. role of CD36 as a thrombospondin receptor. J. Biol. Chem. 267, 16607–16612. doi: 10.1016/s0021-9258(18)42046-7
Simmons, D. L., Tan, S., Tenen, D. G., Nicholson-Weller, A., and Seed, B. (1989). Monocyte antigen CD14 is a phospholipid anchored membrane protein. Blood 73, 284–289. doi: 10.1182/blood.v73.1.284.bloodjournal731284
Soriano-Romani, L., Contreras-Ruiz, L., Garcia-Posadas, L., Lopez-Garcia, A., Masli, S., and Diebold, Y. (2015a). Inflammatory cytokine-mediated regulation of thrombospondin-1 and CD36 in conjunctival cells. J. Ocul. Pharmacol. Ther. 31, 419–428. doi: 10.1089/jop.2015.0029
Soriano-Romani, L., Garcia-Posadas, L., Lopez-Garcia, A., Paraoan, L., and Diebold, Y. (2015b). Thrombospondin-1 induces differential response in human corneal and conjunctival epithelial cells lines under in vitro inflammatory and apoptotic conditions. Exp. Eye Res. 134, 1–14. doi: 10.1016/j.exer.2015.03.004
Stone, N. J., Robinson, J. G., Lichtenstein, A. H., Bairey Merz, C. N., Blum, C. B., Eckel, R. H., et al. (2014). 2013 ACC/AHA guideline on the treatment of blood cholesterol to reduce atherosclerotic cardiovascular risk in adults: a report of the American College of Cardiology/American Heart Association Task Force on practice guidelines. J. Am. Coll. Cardiol. 63(25 Pt. B), 2889–2934. doi: 10.1016/j.jacc.2013.11.002
Susomboon, P., Maneerat, Y., Dekumyoy, P., Kalambaheti, T., Iwagami, M., Komaki-Yasuda, K., et al. (2006). Down-regulation of tight junction mRNAs in human endothelial cells co-cultured with Plasmodium falciparum-infected erythrocytes. Parasitol. Int. 55, 107–112. doi: 10.1016/j.parint.2005.11.054
Tandon, N. N., Kralisz, U., and Jamieson, G. A. (1989). Identification of glycoprotein IV (CD36) as a primary receptor for platelet-collagen adhesion. J. Biol. Chem. 264, 7576–7583. doi: 10.1016/s0021-9258(18)83273-2
Trapnell, C., Hendrickson, D. G., Sauvageau, M., Goff, L., Rinn, J. L., and Pachter, L. (2013). Differential analysis of gene regulation at transcript resolution with RNA-seq. Nat. Biotechnol. 31, 46–53. doi: 10.1038/nbt.2450
Wang, D., Fang, J., Lv, J., Pan, Z., Yin, X., Cheng, H., et al. (2019). Novel polymorphisms in PDLIM3 and PDLIM5 gene encoding Z-line proteins increase risk of idiopathic dilated cardiomyopathy. J. Cell Mol. Med. 23, 7054–7062. doi: 10.1111/jcmm.14607
Weeland, C. J., van den Hoogenhof, M. M., Beqqali, A., and Creemers, E. E. (2015). Insights into alternative splicing of sarcomeric genes in the heart. J. Mol. Cell Cardiol. 81, 107–113. doi: 10.1016/j.yjmcc.2015.02.008
Weber, C., and Noels, H. (2011). Atherosclerosis: current pathogenesis and therapeutic options. Nat. Med. 17, 1410–1422. doi: 10.1038/nm.2538
Wulczyn, F. G., Naumann, M., and Scheidereit, C. (1992). Candidate proto-oncogene bcl-3 encodes a subunit-specific inhibitor of transcription factor NF-kappa B. Nature 358, 597–599. doi: 10.1038/358597a0
Xu, C., Zhang, C., Ji, J., Wang, C., Yang, J., Geng, B., et al. (2018). CD36 deficiency attenuates immune-mediated hepatitis in mice by modulating the proapoptotic effects of CXC chemokine ligand 10. Hepatology 67, 1943–1955. doi: 10.1002/hep.29716
Yao, C., Chen, B. H., Joehanes, R., Otlu, B., Zhang, X., Liu, C., et al. (2015). Integromic analysis of genetic variation and gene expression identifies networks for cardiovascular disease phenotypes. Circulation 131, 536–549. doi: 10.1161/CIRCULATIONAHA.114.010696
Zechner, D., Thuerauf, D. J., Hanford, D. S., McDonough, P. M., and Glembotski, C. C. (1997). A role for the p38 mitogen-activated protein kinase pathway in myocardial cell growth, sarcomeric organization, and cardiac-specific gene expression. J. Cell Biol. 139, 115–127. doi: 10.1083/jcb.139.1.115
Zheng, M., Cheng, H., Banerjee, I., and Chen, J. (2010). ALP/Enigma PDZ-LIM domain proteins in the heart. J. Mol. Cell Biol. 2, 96–102. doi: 10.1093/jmcb/mjp038
Keywords: familial hyperlipidemia, premature coronary heart disease, biomarker, transcriptome, predictive genes
Citation: Prasongsukarn K, Dechkhajorn W, Benjathummarak S and Maneerat Y (2021) TRPM2, PDLIM5, BCL3, CD14, GBA Genes as Feasible Markers for Premature Coronary Heart Disease Risk. Front. Genet. 12:598296. doi: 10.3389/fgene.2021.598296
Received: 24 August 2020; Accepted: 19 April 2021;
Published: 20 May 2021.
Edited by:
Jinwei Tian, The Second Affiliated Hospital of Harbin Medical University, ChinaReviewed by:
Yuki Eshita, Hokkaido University, JapanDiana Baralle, University of Southampton, United Kingdom
Copyright © 2021 Prasongsukarn, Dechkhajorn, Benjathummarak and Maneerat. This is an open-access article distributed under the terms of the Creative Commons Attribution License (CC BY). The use, distribution or reproduction in other forums is permitted, provided the original author(s) and the copyright owner(s) are credited and that the original publication in this journal is cited, in accordance with accepted academic practice. No use, distribution or reproduction is permitted which does not comply with these terms.
*Correspondence: Yaowapa Maneerat, eWFvd2FwYS5tYW5AbWFoaWRvbC5hYy50aA==
†These authors have contributed equally to this work