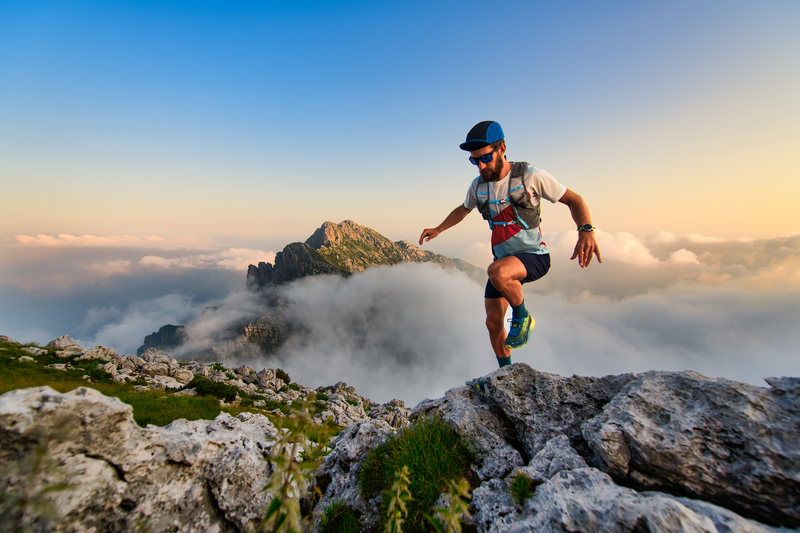
94% of researchers rate our articles as excellent or good
Learn more about the work of our research integrity team to safeguard the quality of each article we publish.
Find out more
ORIGINAL RESEARCH article
Front. Genet. , 23 June 2021
Sec. Systems Biology Archive
Volume 12 - 2021 | https://doi.org/10.3389/fgene.2021.595436
This article is part of the Research Topic Analysis of Bioinformatics Tools in Systems Genetics View all 10 articles
Background: The claudin family is a group of transmembrane proteins related to tight junctions. While their involvement in cancer has been studied extensively, their relationship with the tumor immune microenvironment remains poorly understood. In this research, we focused on genes related to the prognosis of ovarian cancer and explored their relationship with the tumor immune microenvironment.
Methods: The cBioPortal for Cancer Genomics database was used to obtain the genetic variation pattern of the claudin family in ovarian cancer. The ONCOMINE and Gene Expression Profiling Interactive Analysis (GEPIA) databases were used to explore the mRNA expression of claudins in cancers. The prognostic potential of these genes was examined via the Kaplan-Meier plotter. The enrichment of immunological signatures was determined by gene set enrichment analysis (GSEA). The correlations between claudins and the tumor immune microenvironment in ovarian cancer were investigated via the Tumor Immune Estimation Resource (TIMER).
Results: Claudin genes were altered in 363 (62%) of queried patients/samples. Abnormal expression levels of claudins were observed in various cancers. Among them, CLDN3, CLDN4, CLDN6, CLDN10, CLDN15, and CLDN16 were significantly correlated with overall survival in patients with ovarian cancer. GSEA revealed that CLDN6 and CLDN10 were significantly enriched in immunological signatures of B cell, CD4 T cell, and CD8 T cell. Furthermore, CLDN6 and CLDN10 were negatively correlated and positively correlated, respectively, with immune cell infiltration in ovarian cancer. The expression levels of CLDN6 and CLDN10 were also negatively correlated and positively correlated, respectively, with various gene markers of immune cells in ovarian cancer. Thus, CLDN6 and CLDN10 may participate in immune cell infiltration in ovarian cancer, and these mechanisms may be the reason for poor prognosis.
Conclusion: Our study showed that CLDN6 and CLDN10 were prognostic biomarkers correlated with the immune microenvironment in ovarian cancer. These results reveal new roles for CLDN6 and CLDN10 as potential therapeutic targets in the treatment of ovarian cancer.
Ovarian cancer is the most lethal gynecological cancer among women (Siegel et al., 2020). Although surgical techniques and combined chemotherapy applications have progressed since the 1970s, the 5 year survival rate of advanced ovarian cancer is only 40–45% (Henderson et al., 2018). Therefore, improved treatment of ovarian cancer remains an urgent issue. Immunotherapy is an emerging treatment for several solid tumors, which shows improved outcomes in patients. With the application of various immune-based interventions in ovarian cancer, immunotherapy has been proven useful in advanced disease (Bogani et al., 2020).
The claudin (CLDN) family consists of more than 20 transmembrane proteins, which are major components of tight junctions. They serve as a physical barrier to prevent molecules from passing freely through the paracellular space between epithelial or endothelial cell sheets and also play critical roles in maintaining cell polarity and signal transductions (Weinstein et al., 1976; Wodarz, 2000; Tsukita et al., 2001; Kirschner et al., 2013). Previous research has recognized various claudin gene expression patterns and identified several genes dysregulated in cancers (Hewitt et al., 2006). These genes play roles in the tumorigenesis of solid tumors (Swisshelm et al., 2005; Hagen, 2019) and represent promising targets for cancer detection, prognosis, and therapy (Morin, 2005). However, the relationship between claudins and the tumor immune microenvironment has not yet been elucidated. This study comprehensively analyzed claudin expression in ovarian cancer and further explored the relationship between claudins and the immune microenvironment.
The cBioPortal database1 (Cerami et al., 2012; Gao et al., 2013) is an open platform for cancer genomics analysis. In total, 585 samples of ovarian serous cystadenocarcinoma (The Cancer Genome Atlas (TCGA), Pan-Cancer Atlas) were used for genetic variation analyses through the cBioPortal.
Claudin expression levels in various cancers were analyzed via the ONCOMINE database2 (Rhodes et al., 2007), which includes more than 35 types of cancer and normal samples.
GEPIA v23 (Tang et al., 2017) is used to analyze the RNA sequencing expression data of 9736 tumors and 8587 normal samples from the TCGA and GTEx projects using a standard processing pipeline. The expression profile of the claudins in ovarian cancer was explored via GEPIA v2. The p-value cutoff was 0.05 and | log2FC| cutoff was 1.5.
The Kaplan-Meier plotter4 (Gyorffy et al., 2012) assesses the effects of 54,000 genes on survival in 21 cancer types. The largest datasets include breast (n = 6234), ovarian (n = 2190), lung (n = 3452), and gastric (n = 1440) cancer. The system includes gene chip and RNA-seq data-sources from the Gene Expression Omnibus (GEO), European Genome-Phenome Archive (EGA), and TCGA databases. The prognostic significance of claudins in ovarian cancer was analyzed via the online database.
TIMER5 (Li et al., 2017) allows comprehensive analysis of tumor-infiltrating immune cells. The correlation between claudin expression and immune cell infiltration was analyzed using this database. TIMER v2, an updated and enhanced version of TIMER, was used to analyze immune infiltration across diverse cancer types.
The expression levels of claudins are presented as mean ± standard deviation (SD). Kaplan-Meier survival curves were established based on the log-rank test. The hazard ratio (HR) was determined using the Cox model. Spearman correlation was used for correlation analysis. A p-value of < 0.05 was considered to be significant.
Twenty-four reviewed proteins of the claudin family were obtained from the UniProt Knowledgebase (UniProtKB)6 (Table 1) [an additional file shows this in more detail (see Table 1)]. Firstly, we investigated the genetic variation of the claudin family in ovarian cancer using the cBioPortal for Cancer Genomics. Twenty-four genes were queried in 585 samples of ovarian serous cystadenocarcinoma (TCGA, Pan-Cancer Atlas). Figure 1A shows the alteration frequency of genetic variation in serous ovarian cancer. As shown in Figure 1B, the queried genes were altered in 363 (62%) queried patients/samples. The top three gene variations were CLDN11 (24%), CLDN16 (22%), and CLDN1 (16%). Differences in overall survival (OS) between the altered and unaltered groups were compared using the Kruskal-Wallis test. We found that OS was reduced in the altered group compared to the unaltered group (p = 7.981e-3) (Figure 1C). Previous studies have shown that the claudin family is dysregulated in a variety of tumors and is involved in diagnosis, tumorigenesis, and prognosis (Zhang et al., 2013; Barros-Filho et al., 2015; Zhou et al., 2018). Thus, the claudin family is worthy of further research in ovarian cancer.
Figure 1. The genetic variation of the claudin family in ovarian cancer through the cBioPortal. (A) The alteration frequency of the claudin family in serous ovarian cancer. (B) The oncoprint of the claudin family in serous ovarian cancer. (C) The overall survival difference of serous ovarian cancer between the altered and unaltered group (**p < 0.01).
To explore the mRNA expression of the claudin family, we investigated the expression profiles of claudins in various cancers via the ONCOMINE database. The thresholds were: p-value of 0.05, fold change of 1.5, and gene rank of all. Significant analyses are shown in Supplementary Figure 1 (those with < 3 significant analyses were not considered). Results showed that most claudins were dysregulated in various cancers. To verify the expression of claudins in ovarian cancer, GEPIA2 was used to analyze mRNA expression in TCGA and GTEx samples. The | Log2FC| cutoff was set to 1.5 and the p-value cutoff was set to 0.05. As shown in Figure 2, eight genes were overexpressed in ovarian cancer samples compared with normal tissue samples and included CLDN1, CLDN3, CLDN4, CLDN6, CLDN7, CLDN9, CLDN10, and CLDN16. Furthermore, three genes showed low expression in the ovarian cancer samples compared with normal tissue samples and included CLDN5, CLDN11, and CLDN15.
Figure 2. The mRNA expression of claudins in TCGA samples and the GTEx normal samples via GEPIA2. (*p < 0.01).
To identify genes with clinical significance, we studied the relationship between differentially expressed genes (DEGs) and ovarian cancer patient prognosis using the Kaplan-Meier plotter. As shown in Figure 3, overexpressed genes CLDN3, CLDN4, CLDN6, and CLDN16 were significantly correlated with poor OS (Figure 3A) and progression free survival (PFS) (Figure 3B). In addition, high expression of CLDN10 and CLDN15 were predictive of a good prognosis in ovarian cancer patients (Figures 3C,D). Surprisingly, CLDN10 was overexpressed in cancer, but patients with high expression of CLDN10 showed good OS (HR = 0.73, logrank P = 1.6e-06), PFS (HR = 0.83, logrank P = 0.0067), and post progression survival (PPS, HR = 0.73, logrank P = 0.00029). These results are somewhat counterintuitive, and the underlying mechanism requires further exploration.
Figure 3. The relationship between claudin expression and the prognosis of ovarian cancer patients through Kaplan-Meier plotter. The overexpression of CLDN3, CLDN4, CLDN6, and CLDN16 were significantly correlated with poor OS (A) and PFS (B). (C) The overexpression of CLDN10 predicted good OS, PFS, and PPS. (D) The low expression of CLDN15 predicted poor OS in ovarian cancer. OS, overall survival; PFS, progression free survival; PPS, post progression survival.
TCGA projects have identified four molecular subtypes of high-grade serous ovarian carcinoma (HGSOC) (Cancer Genome Atlas Research Network, 2011): (i) the differentiated subtype; (ii) the immunoreactive subtype; (iii) the mesenchymal subtype; and (iv) the proliferative subtype. Among them, T-cell chemokine ligands, CXCL11 and CXCL10, and the receptor, CXCR3, characterized the immunoreactive subtype. Then, Thorsson et al. (2018) developed a global immune classification of solid tumors based on the transcriptomic profiles of 33 cancer types. They identified six distinct immune subtypes: C1 (Wound healing); C2 (IFN-γ dominant); C3 (Inflammatory); C4 (Lymphocyte depleted); C5 (Immunologically quiet); C6 (TGF-β dominant). These six categories represent features of the tumor microenvironment (Charoentong et al., 2017). In this research, we explored the relationships between the expression of differentially expressed genes related to prognosis and molecular subtypes or immune subtypes of ovarian cancer via the TISIDB (Ru et al., 2019). The Kruskal-Wallis test was used. As Supplementary Figure 2 shows, claudins including CLDN3, CLDN6, CLDN10, and CLDN15 are differentially expressed in different immune subtypes. And, claudins including CLDN3, CLDN4, CLDN6, CLDN10, and CLDN16 are differentially expressed in different molecular subtypes (Supplementary Figure 3). Among them, CLDN6 is relatively low expression, and CLDN10 is relatively high expression in the immunoreactive subtype.
To characterize the potential function of claudins, GSEA was performed using gene expression data from TCGA ovarian cancer patients. Immunological signature gene sets were used. As shown in Figure 4, CLDN6 and CLDN10 were related to the effector differentiation of B cell, CD4 T cell, and CD8 T cell.
Figure 4. Gene set enrichment analysis (GSEA) of c7 (immunologic signatures) for CLDN6 and CLDN10. CLDN6 (A) and CLDN10 (B) were related to effector differentiation of B cell, CD8 T cell, and CD4 T cell.
To understand the role of claudins in immunity, we downloaded 379 RNA-seq FPKM (Fragments per kilobase per million) data of ovarian cancer from TCGA. Subsequently, the FPKM was converted to TPM (transcripts per million) (Li et al., 2010). The ESTIMATE algorithm (Yoshihara et al., 2013) was used to predict tumor purity based on TCGA ovarian cancer samples. Then, the relationship between claudin expression and the immune microenvironment was explored. As shown in Figure 5A, a significant negative correlation between CLDN6 expression and the immune score was observed (Spearman correlation = −0.23, p < 0.001). A significant positive correlation between CLDN10 expression and immune score (spearman correlation = 0.21, p < 0.001) was observed (Figure 5B). However, the expression levels of CLDN6 and CLDN10 were not correlated with the stromal score.
Figure 5. Relationship between claudins expression and tumor immune microenvironment. (A) The expression of CLDN6 has a negative correlation with immune score and ESTIMATE score. (B) The expression of CLDN10 has a positive correlation with immune score and ESTIMATE score. (C) The difference of 22 immune cells between the claudin-high group and claudin-low group (*p < 0.05, **p < 0.01).
We next examined the relationship between immune cell infiltration and claudin expression. RNA-seq TPM data (n = 379) from TCGA ovarian cancer were used to assess 22 immune cells subtype concentrations with the CIBERSORT algorithm (Newman et al., 2019). TCGA samples were grouped by the median values of CLDN6 and CLDN10, respectively. Activated dendritic cells differed significantly between the CLDN6_high and CLDN6_low groups. Several cell types were significantly different between the CLDN10_high and CLDN10_low group, including naïve B cells, memory B cells, naïve CD4 T cells, CD4 memory-activated T cells, monocytes, M1 macrophages, and activated dendritic cells (Figure 5C).
The microarray expression values of ovarian cancer were used to calculate the abundances of six immune infiltrates (B cells, CD4+ T cells, CD8+ T cells, Neutrophils, Macrophages, and Dendritic cells) via the TIMER algorithm (Yoshihara et al., 2013). The gene expression levels correlated with tumor purity are displayed in the left-most panel (Figures 6A,B). Results showed that CLDN6 expression was negatively correlated with infiltration of B cell (partial correlation = −0.284, p = 2.21e-10), CD8+ T cells (partial correlation = −0.254, p = 1.64e-08), neutrophils (partial correlation = −0.152, p = 8.29e-04), and dendritic cells (partial correlation = −0.182, p = 6.31e-05) (Figure 6A). In contrast, there was a small but significant positive correlation between CLDN10 expression and infiltration of neutrophils (partial correlation = 0.185, p = 4.66e-05), and dendritic cells (partial correlation = 0.153, p = 7.74e-04) (Figure 6B).
Figure 6. The relationship between immune cell infiltration and claudin expression. Correlation analysis of immune cell infiltration and CLDN6 expression (A), and CLDN10 expression (B) based on the microarray expression values of ovarian cancer through TIMER. Correlation analysis of immune cell infiltration and CLDN6 expression (C), and CLDN10 expression (D) based on available TCGA RNA-seq data of ovarian cancer via TIMER2.
To more accurately describe the relationship between gene expression and immune cell infiltration, we used the TIMER, CIBERSORT, quanTIseq, xCell, MCP-counter, and EPIC algorithms to assess the immune infiltration in tumor tissue (Sturm et al., 2019). TIMER2 provides a platform to analyze immune infiltrates across diverse cancer types based on available TCGA RNA-seq data (Li et al., 2016; Li T. et al., 2020). The correlations between claudin expression (CLDN6 and CLDN10) and immune cell infiltration in ovarian cancer are shown in Table 2. As seen in Figure 6C, CLDN6 was negatively correlated with immune cell infiltration, including that of B cells, CD8+ T cells, effector memory CD4+ T cells, M1 macrophages, and myeloid dendritic cells. In contrast, CLDN10 was positively correlated with immune cell infiltration, including that of B cells, CD8+ T cells, effector memory CD4+ T cells, M1 macrophages, and myeloid dendritic cells (Figure 6D). Relevant evidence suggests that cancer-associated fibroblasts (CAFs) play an important role in the progression of ovarian cancer (Mhawech-Fauceglia et al., 2014; Leung et al., 2018). Interestingly, here, CAFs also showed a positive correlation with CLDN6 expression, but a negative correlation with CLDN10 expression. In ovarian cancer, increased infiltration of tumor-infiltrating lymphocytes (TILs), and more specifically CD8+ T cells, have been proven to be associated with improved clinical outcomes (Sato et al., 2005; Hamanishi et al., 2007; Ovarian Tumor Tissue Analysis et al., 2017). These results suggest that CLDN6 and CLDN10 may participate in immune cell infiltration in ovarian cancer, and these mechanisms may be the reason for poor prognosis.
Table 2. Correlation analysis between claudins and immune infiltration in ovarian cancer via TIMER2.0.
To further illustrate the correlation between claudins and the immune microenvironment, we analyzed the relationship between CLDN6 and CLDN10 expression and gene markers of various immune cells in ovarian cancer (TIMER2 database), including B cells, T cells (general), CD8+ T cells, macrophages, dendritic cells, neutrophils, monocytes, natural killer (NK) cells, and regulatory T cells (Tregs) (Table 3). Purity-adjusted correlation heatmaps are shown in Supplementary Figure 4. After correlation adjustment by purity, CLDN6 expression was negatively correlated with most gene markers of dendritic cells, M1 macrophages, monocytes, NK cells, and tumor-associated macrophages (TAMs) in ovarian cancer. In contrast, CLDN10 expression was positively correlated with gene markers of dendritic cells, T cells (general), and TAMs in ovarian cancer.
Table 3. Correlation analysis between claudins and markers of immune cells in ovarian cancer via TIMER2.0.
Studies have shown that the tumor-infiltrating immune cells mentioned above are related to the tumor immunotherapy response (Rodriguez et al., 2018). Immune cell-based immunotherapy (Baci et al., 2020), including NK Cells (Nersesian et al., 2019) and dendritic cells (Stiff et al., 2013), play important roles in the treatment of ovarian cancer. Taken together, these analyses and our research indicate that CLDN6 and CLDN10 may play important roles in immunotherapy in the future.
CLDN6 and CLDN10 are important components of the claudin family related to tight junctions. Claudins were considered promising targets for diagnosis and therapy since they were involved in uncontrolled cancer growth and metastasis (Martin and Jiang, 2001; Morin, 2005; Bose and Mukhopadhyay, 2010). Moreover, studies have shown that they not only play a vital role in tumorigenesis (Swisshelm et al., 2005; Arabzadeh et al., 2007; Hagen, 2019), but also drug resistance (Gao et al., 2017).
CLDN6 had been demonstrated abnormal expression and can be a prognostic marker in cancers including ovarian cancer (Wang et al., 2013), endometrial cancer (Kojima et al., 2020), gastric cancer (Kohmoto et al., 2020), breast carcinoma (Liu et al., 2016; Jia et al., 2019), and lung cancer (Micke et al., 2014). Bioinformatic analysis has revealed that CLDN6 is regulated by a diverse set of transcription factors and promotes cancer cell behavior via the ASK1-p38/JNK MAPK secretory signaling pathway (Lin et al., 2017). A study revealed that CLDN6 may be a novel targeted therapy for ovarian cancer as a receptor for clostridium perfringens enterotoxin (Lal-Nag et al., 2012). In addition, 6PHU3, a T-cell-engaging bispecific single chain antibody with anti-CD3/anti-CLDN6 specificities, upregulated the cytotoxicity of T cells and made T cells acquire an effector phenotype (Stadler et al., 2016). Another recent study showed that CLDN6 as a chimeric antigen receptor target in solid tumors can be a strategy to overcome inefficient CAR-T cell stimulation in vivo (Reinhard et al., 2020). These studies suggested that CLDN6 has important research value in the treatment of cancer.
CLDN10, a glandular epithelial marker in epithelial ovarian cancer (Seo et al., 2010), was reported to be a key immune-related gene in clear cell renal cell carcinoma (Yang et al., 2021) and papillary thyroid carcinoma (Xiang et al., 2020). Furthermore, CLDN10 expression has proved to be a prognostic marker for ovarian cancer (Li Z. et al., 2020).
The present study combined and analyzed the prognostic potential of CLDN6 and CLDN10 with the tumor immune microenvironment. Consistent with previous reports, both CLDN6 and CLDN10 showed high expression in ovarian cancer. Prognostic analysis showed that the overexpression of CLDN6 was related to a poor prognosis for patients with ovarian cancer. However, CLDN10 overexpression predicted a better prognosis compared to the low CLDN10 expression group. We also found that CLDN6 overexpression was negatively related to immune cell infiltration, whereas CLDN10 overexpression was positively correlated with immune cell infiltration. Moreover, we found that CLDN6 and CLDN10 were related to gene markers of dendritic cells, NK cells, and TAMs. These results may explain why the overexpression of CLDN6 and low expression of CLDN10 predict poor OS in ovarian cancer. This study revealed that the prognostic potential of CLDN6 and CLDN10 is related to the tumor immune microenvironment in ovarian cancer.
Relevant evidence has emerged that immune-related gene expression and TILs are related to the prognosis, recurrence (Ojalvo et al., 2018), and chemotherapeutic response (Choi et al., 2020) of ovarian cancer. Furthermore, the presence of TILs may improve clinical outcomes in ovarian cancer patients (Odunsi, 2017). Immune cell-based immunotherapy (Baci et al., 2020), including NK Cells (Nersesian et al., 2019) and dendritic cells (Stiff et al., 2013), play an important role in the treatment of ovarian cancer. Previous studies and our analyses suggest that CLDN6 may be involved in immune evasion and that they could be an ideal candidate for immunotherapy in ovarian cancer. Future studies on the combined application of claudin-based molecular targeted therapy and immunotherapy are necessary.
CLDN6 and CLDN10 were identified as potential prognostic biomarkers and were correlated with immune cell infiltration in ovarian cancer. Our results revealed new roles for CLDN6 and CLDN10 in ovarian cancer and their potential as therapeutic targets in cancer treatment.
The original contributions presented in the study are included in the article/Supplementary Material, further inquiries can be directed to the corresponding author/s.
PeW was responsible for the study conception and design. PG, TP, CC, and SL were involved in data acquisition, data analysis, and interpretation. PG drafted the manuscript and took charge of supervising the manuscript. All authors read and approved the manuscript.
This work was supported by the Natural Science Foundation of China (81772775 to JW and 82072895 to PeW).
The authors declare that the research was conducted in the absence of any commercial or financial relationships that could be construed as a potential conflict of interest.
This manuscript was released as a pre-print at Research Square (https://www.researchsquare.com/article/rs-40048/v1) (DOI: 10.21203/rs.3.rs-40048/v1) (Gao et al., 2020).
The Supplementary Material for this article can be found online at: https://www.frontiersin.org/articles/10.3389/fgene.2021.595436/full#supplementary-material
Supplementary Figure 1 | The significant unique analyses of claudins expression in ONCOMINE. (Red: overexpression; Blue: low expression).
Supplementary Figure 2 | Associations between the expression of claudins and immune subtypes of ovarian cancer.
Supplementary Figure 3 | Associations between the expression of claudins and molecular subtypes of ovarian cancer.
Supplementary Figure 4 | The correlations between claudins (CLDN6 and CLDN10) expression and gene markers of immune cells across gynecologic oncology (Red: positive correlation; Blue: negative correlation).
GEPIA, Gene Expression Profiling Interactive Analysis; TIMER, Tumor Immune Estimation Resource; GSEA, gene set enrichment analyses; TCGA, The Cancer Genome Atlas; GEO, Gene Expression Omnibus; EGA, European Genome-Phenome Archive; FPKM, Fragments per kilobase per million; TPM, transcripts per million; TILs, tumor-infiltrating lymphocytes; NK, natural killer cells; Tregs, regulatory T cells; CAFs, cancer associated fibroblasts; TAMs, Tumor-associated macrophages; CPE, clostridium perfringens enterotoxin; OS, overall survival; PFS, progression free survival; PPS, post progression survival.
Arabzadeh, A., Troy, T. C., and Turksen, K. (2007). Changes in the distribution pattern of Claudin tight junction proteins during the progression of mouse skin tumorigenesis. BMC Cancer 7:196. doi: 10.1186/1471-2407-7-196
Baci, D., Bosi, A., Gallazzi, M., Rizzi, M., Noonan, D. M., Poggi, A., et al. (2020). The Ovarian Cancer Tumor Immune Microenvironment (TIME) as Target for Therapy: a Focus on Innate Immunity Cells as Therapeutic Effectors. Int. J. Mol. Sci. 21:3125. doi: 10.3390/ijms21093125
Barros-Filho, M. C., Marchi, F. A., Pinto, C. A., Rogatto, S. R., and Kowalski, L. P. (2015). High Diagnostic Accuracy Based on CLDN10, HMGA2, and LAMB3 Transcripts in Papillary Thyroid Carcinoma. J. Clin. Endocrinol. Metab. 100, E890–E899.
Bogani, G., Lopez, S., Mantiero, M., Ducceschi, M., Bosio, S., Ruisi, S., et al. (2020). Immunotherapy for platinum-resistant ovarian cancer. Gynecol. Oncol. 158, 484–488.
Bose, C. K., and Mukhopadhyay, A. (2010). Claudin and ovarian cancer. J. Turk. Ger. Gynecol. Assoc. 11, 48–54.
Cancer Genome Atlas Research Network. (2011). Integrated genomic analyses of ovarian carcinoma. Nature 474, 609–615. doi: 10.1038/nature10166
Cerami, E., Gao, J., Dogrusoz, U., Gross, B. E., Sumer, S. O., Aksoy, B. A., et al. (2012). The cBio Cancer Genomics Portal: an Open Platform for Exploring Multidimensional Cancer Genomics Data. Cancer Discov. 2, 401–404. doi: 10.1158/2159-8290.cd-12-0095
Charoentong, P., Finotello, F., Angelova, M., Mayer, C., Efremova, M., Rieder, D., et al. (2017). Pan-cancer Immunogenomic Analyses Reveal Genotype-Immunophenotype Relationships and Predictors of Response to Checkpoint Blockade. Cell Rep. 18, 248–262. doi: 10.1016/j.celrep.2016.12.019
Choi, K. U., Kim, A., Kim, J. Y., Kim, K. H., Hwang, C., Lee, S. J., et al. (2020). Differences in immune-related gene expressions and tumor-infiltrating lymphocytes according to chemotherapeutic response in ovarian high-grade serous carcinoma. J. Ovarian Res. 13:65.
Gao, J., Aksoy, B. A., Dogrusoz, U., Dresdner, G., Gross, B., Sumer, S. O., et al. (2013). Integrative analysis of complex cancer genomics and clinical profiles using the cBioPortal. Sci. Signal. 6:l1.
Gao, P., Peng, T., Cao, C., Lin, S., Wu, P., Huang, X., et al. (2020). CLDN6 and CLDN10 are Associated with Immune Infiltration of Ovarian Cancer: a Study of Claudin Family. Front. Genet. doi: 10.21203/rs.3.rs-40048/v1 [Preprint].
Gao, Y., Liu, X., Li, T., Wei, L., Yang, A., Lu, Y., et al. (2017). Cross-validation of genes potentially associated with overall survival and drug resistance in ovarian cancer. Oncol. Rep. 37, 3084–3092. doi: 10.3892/or.2017.5534
Gyorffy, B., Lanczky, A., and Szallasi, Z. (2012). Implementing an online tool for genome-wide validation of survival-associated biomarkers in ovarian-cancer using microarray data from 1287 patients. Endocr. Relat. Cancer 19, 197–208. doi: 10.1530/erc-11-0329
Hagen, S. J. (2019). Unraveling a New Role for Claudins in Gastric Tumorigenesis. Cell. Mol. Gastroenterol. Hepatol. 8, 151–152. doi: 10.1016/j.jcmgh.2019.04.004
Hamanishi, J., Mandai, M., Iwasaki, M., Okazaki, T., Tanaka, Y., Yamaguchi, K., et al. (2007). Programmed cell death 1 ligand 1 and tumor-infiltrating CD8+ T lymphocytes are prognostic factors of human ovarian cancer. Proc. Natl. Acad. Sci. U. S. A. 104, 3360–3365. doi: 10.1073/pnas.0611533104
Henderson, J. T., Webber, E. M., and Sawaya, G. F. (2018). Screening for Ovarian Cancer: updated Evidence Report and Systematic Review for the US Preventive Services Task Force. JAMA 319, 595–606. doi: 10.1001/jama.2017.21421
Hewitt, K. J., Agarwal, R., and Morin, P. J. (2006). The claudin gene family: expression in normal and neoplastic tissues. BMC Cancer 6:186. doi: 10.1186/1471-2407-6-186
Jia, H., Chai, X., Li, S., Wu, D., and Fan, Z. (2019). Identification of claudin-2, -6, -11 and -14 as prognostic markers in human breast carcinoma. Int. J. Clin. Exp. Pathol. 12, 2195–2204.
Kirschner, N., Rosenthal, R., Furuse, M., Moll, I., Fromm, M., Brandner, J. M., et al. (2013). Contribution of tight junction proteins to ion, macromolecule, and water barrier in keratinocytes. J. Invest. Dermatol. 133, 1161–1169. doi: 10.1038/jid.2012.507
Kohmoto, T., Masuda, K., Shoda, K., Takahashi, R., Ujiro, S., Tange, S., et al. (2020). Claudin-6 is a single prognostic marker and functions as a tumor-promoting gene in a subgroup of intestinal type gastric cancer. Gastric Cancer 23, 403–417. doi: 10.1007/s10120-019-01014-x
Kojima, M., Sugimoto, K., Tanaka, M., Endo, Y., Kato, H., Honda, T., et al. (2020). Prognostic Significance of Aberrant Claudin-6 Expression in Endometrial Cancer. Cancers 12:2748. doi: 10.3390/cancers12102748
Lal-Nag, M., Battis, M., Santin, A. D., and Morin, P. J. (2012). Claudin-6: a novel receptor for CPE-mediated cytotoxicity in ovarian cancer. Oncogenesis 1:e33. doi: 10.1038/oncsis.2012.32
Leung, C. S., Yeung, T. L., Yip, K. P., Wong, K. K., Ho, S. Y., Mangala, L. S., et al. (2018). Cancer-associated fibroblasts regulate endothelial adhesion protein LPP to promote ovarian cancer chemoresistance. J. Clin. Invest. 128, 589–606. doi: 10.1172/jci95200
Li, B., Ruotti, V., Stewart, R. M., Thomson, J. A., and Dewey, C. N. (2010). RNA-Seq gene expression estimation with read mapping uncertainty. Bioinformatics 26, 493–500. doi: 10.1093/bioinformatics/btp692
Li, B., Severson, E., Pignon, J. C., Zhao, H., Li, T., Novak, J., et al. (2016). Comprehensive analyses of tumor immunity: implications for cancer immunotherapy. Genome Biol. 17:174.
Li, T., Fan, J., Wang, B., Traugh, N., Chen, Q., Liu, J. S., et al. (2017). TIMER: a Web Server for Comprehensive Analysis of Tumor-Infiltrating Immune Cells. Cancer Res. 77, e108–e110.
Li, T., Fu, J., Zeng, Z., Cohen, D., Li, J., Chen, Q., et al. (2020). TIMER2.0 for analysis of tumor-infiltrating immune cells. Nucleic Acids Res. 48, W509–W514.
Li, Z., Xuan, W., Huang, L., Chen, N., Hou, Z., Lu, B., et al. (2020). Claudin 10 acts as a novel biomarker for the prognosis of patients with ovarian cancer. Oncol. Lett. 20, 373–381.
Lin, D., Guo, Y., Li, Y., Ruan, Y., Zhang, M., Jin, X., et al. (2017). Bioinformatic analysis reveals potential properties of human Claudin-6 regulation and functions. Oncol. Rep. 38, 875–885. doi: 10.3892/or.2017.5756
Liu, Y., Jin, X., Li, Y., Ruan, Y., Lu, Y., Yang, M., et al. (2016). DNA methylation of claudin-6 promotes breast cancer cell migration and invasion by recruiting MeCP2 and deacetylating H3Ac and H4Ac. J. Exp. Clin. Cancer Res. 35:120.
Martin, T. A., and Jiang, W. G. (2001). Tight junctions and their role in cancer metastasis. Histol. Histopathol. 16, 1183–1195.
Mhawech-Fauceglia, P., Wang, D., Samrao, D., Kim, G., Lawrenson, K., Meneses, T., et al. (2014). Clinical Implications of Marker Expression of Carcinoma-Associated Fibroblasts (CAFs) in Patients with Epithelial Ovarian Carcinoma After Treatment with Neoadjuvant Chemotherapy. Cancer Microenviron 7, 33–39. doi: 10.1007/s12307-013-0140-4
Micke, P., Mattsson, J. S., Edlund, K., Lohr, M., Jirström, K., Berglund, A., et al. (2014). Aberrantly activated claudin 6 and 18.2 as potential therapy targets in non-small-cell lung cancer. Int. J. Cancer 135, 2206–2214. doi: 10.1002/ijc.28857
Morin, P. J. (2005). Claudin proteins in human cancer: promising new targets for diagnosis and therapy. Cancer Res. 65, 9603–9606. doi: 10.1158/0008-5472.can-05-2782
Nersesian, S., Glazebrook, H., Toulany, J., Grantham, S. R., and Boudreau, J. E. (2019). Naturally Killing the Silent Killer: NK Cell-Based Immunotherapy for Ovarian Cancer. Front. Immunol. 10:1782. doi: 10.3389/fimmu.2019.01782
Newman, A. M., Steen, C. B., Liu, C. L., Gentles, A. J., Chaudhuri, A. A., Scherer, F., et al. (2019). Determining cell type abundance and expression from bulk tissues with digital cytometry. Nat. Biotechnol. 37, 773–782. doi: 10.1038/s41587-019-0114-2
Ojalvo, L. S., Thompson, E. D., Wang, T. L., Meeker, A. K., Shih, I. M., Fader, A. N., et al. (2018). Tumor-associated macrophages and the tumor immune microenvironment of primary and recurrent epithelial ovarian cancer. Hum. Pathol. 74, 135–147. doi: 10.1016/j.humpath.2017.12.010
Ovarian Tumor Tissue Analysis, C., Goode, E. L., Block, M. S., Kalli, K. R., Vierkant, R. A., Chen, W., et al. (2017). Dose-Response Association of CD8+ Tumor-Infiltrating Lymphocytes and Survival Time in High-Grade Serous Ovarian Cancer. JAMA Oncol. 3:e173290.
Reinhard, K., Rengstl, B., Oehm, P., Michel, K., Billmeier, A., Hayduk, N., et al. (2020). An RNA vaccine drives expansion and efficacy of claudin-CAR-T cells against solid tumors. Science 367, 446–453. doi: 10.1126/science.aay5967
Rhodes, D. R., Kalyana-Sundaram, S., Mahavisno, V., Varambally, R., Yu, J., Briggs, B. B., et al. (2007). Oncomine 3.0: genes, pathways, and networks in a collection of 18,000 cancer gene expression profiles. Neoplasia 9, 166–180. doi: 10.1593/neo.07112
Rodriguez, G. M., Galpin, K. J. C., Mccloskey, C. W., and Vanderhyden, B. C. (2018). The Tumor Microenvironment of Epithelial Ovarian Cancer and Its Influence on Response to Immunotherapy. Cancers 10:242. doi: 10.3390/cancers10080242
Ru, B., Wong, C. N., Tong, Y., Zhong, J. Y., Zhong, S. S. W., Wu, W. C., et al. (2019). TISIDB: an integrated repository portal for tumor-immune system interactions. Bioinformatics 35, 4200–4202. doi: 10.1093/bioinformatics/btz210
Sato, E., Olson, S. H., Ahn, J., Bundy, B., Nishikawa, H., Qian, F., et al. (2005). Intraepithelial CD8+ tumor-infiltrating lymphocytes and a high CD8+/regulatory T cell ratio are associated with favorable prognosis in ovarian cancer. Proc. Natl. Acad. Sci. U. S. A. 102, 18538–18543. doi: 10.1073/pnas.0509182102
Seo, H. W., Rengaraj, D., Choi, J. W., Ahn, S. E., Song, Y. S., Song, G., et al. (2010). Claudin 10 is a glandular epithelial marker in the chicken model as human epithelial ovarian cancer. Int. J. Gynecol. Cancer 20, 1465–1473.
Siegel, R. L., Miller, K. D., and Jemal, A. (2020). Cancer statistics, 2020. CA Cancer J. Clin. 70, 7–30.
Stadler, C. R., Bähr-Mahmud, H., Plum, L. M., Schmoldt, K., Kölsch, A. C., and Türeci, Ö, et al. (2016). Characterization of the first-in-class T-cell-engaging bispecific single-chain antibody for targeted immunotherapy of solid tumors expressing the oncofetal protein claudin 6. Oncoimmunology 5:e1091555. doi: 10.1080/2162402x.2015.1091555
Stiff, P. J., Czerlanis, C., and Drakes, M. L. (2013). Dendritic cell immunotherapy in ovarian cancer. Expert Rev. Anticancer Ther. 13, 43–53.
Sturm, G., Finotello, F., Petitprez, F., Zhang, J. D., Baumbach, J., Fridman, W. H., et al. (2019). Comprehensive evaluation of transcriptome-based cell-type quantification methods for immuno-oncology. Bioinformatics 35, i436–i445.
Swisshelm, K., Macek, R., and Kubbies, M. (2005). Role of claudins in tumorigenesis. Adv. Drug Deliv. Rev. 57, 919–928. doi: 10.1016/j.addr.2005.01.006
Tang, Z., Li, C., Kang, B., Gao, G., Li, C., Zhang, Z., et al. (2017). GEPIA: a web server for cancer and normal gene expression profiling and interactive analyses. Nucleic Acids Res. 45, W98–W102.
Thorsson, V., Gibbs, D. L., Brown, S. D., Wolf, D., Bortone, D. S., Ou Yang, T. H., et al. (2018). The Immune Landscape of Cancer. Immunity 48, 812–830.e14.
Tsukita, S., Furuse, M., and Itoh, M. (2001). Multifunctional strands in tight junctions. Nat. Rev. Mol. Cell Biol. 2, 285–293. doi: 10.1038/35067088
Wang, L., Jin, X., Lin, D., Liu, Z., Zhang, X., Lu, Y., et al. (2013). Clinicopathologic significance of claudin-6, occludin, and matrix metalloproteinases -2 expression in ovarian carcinoma. Diagn. Pathol. 8:190.
Weinstein, R. S., Merk, F. B., and Alroy, J. (1976). The structure and function of intercellular junctions in cancer. Adv. Cancer Res. 23, 23–89. doi: 10.1016/s0065-230x(08)60543-6
Wodarz, A. (2000). Tumor suppressors: linking cell polarity and growth control. Curr. Biol. 10, R624–R626.
Xiang, Z., Zhong, C., Chang, A., Ling, J., Zhao, H., Zhou, W., et al. (2020). Immune-related key gene CLDN10 correlates with lymph node metastasis but predicts favorable prognosis in papillary thyroid carcinoma. Aging 12, 2825–2839. doi: 10.18632/aging.102780
Yang, W., Li, L., Zhang, K., Ma, K., Gong, Y., Zhou, J., et al. (2021). CLDN10 associated with immune infiltration is a novel prognostic biomarker for clear cell renal cell carcinoma. Epigenomics 13, 31–45. doi: 10.2217/epi-2020-0256
Yoshihara, K., Shahmoradgoli, M., Martinez, E., Vegesna, R., Kim, H., Torres-Garcia, W., et al. (2013). Inferring tumour purity and stromal and immune cell admixture from expression data. Nat. Commun. 4:2612.
Zhang, Z., Wang, A., Sun, B., Zhan, Z., Chen, K., Wang, C., et al. (2013). Expression of CLDN1 and CLDN10 in lung adenocarcinoma in situ and invasive lepidic predominant adenocarcinoma. J. Cardiothorac. Surg 8:95.
Keywords: ovarian cancer, CLDN6, CLDN10, prognosis, immune microenvironment
Citation: Gao P, Peng T, Cao C, Lin S, Wu P, Huang X, Wei J, Xi L, Yang Q and Wu P (2021) Association of CLDN6 and CLDN10 With Immune Microenvironment in Ovarian Cancer: A Study of the Claudin Family. Front. Genet. 12:595436. doi: 10.3389/fgene.2021.595436
Received: 16 August 2020; Accepted: 05 May 2021;
Published: 23 June 2021.
Edited by:
Bairong Shen, Sichuan University, ChinaReviewed by:
Rajeev K. Singla, Sichuan University, ChinaCopyright © 2021 Gao, Peng, Cao, Lin, Wu, Huang, Wei, Xi, Yang and Wu. This is an open-access article distributed under the terms of the Creative Commons Attribution License (CC BY). The use, distribution or reproduction in other forums is permitted, provided the original author(s) and the copyright owner(s) are credited and that the original publication in this journal is cited, in accordance with accepted academic practice. No use, distribution or reproduction is permitted which does not comply with these terms.
*Correspondence: Peng Wu, cGVuZ3d1ODYyNkB0amgudGptdS5lZHUuY24=; Qin Yang, NzA5MTg0MDRAcXEuY29t
Disclaimer: All claims expressed in this article are solely those of the authors and do not necessarily represent those of their affiliated organizations, or those of the publisher, the editors and the reviewers. Any product that may be evaluated in this article or claim that may be made by its manufacturer is not guaranteed or endorsed by the publisher.
Research integrity at Frontiers
Learn more about the work of our research integrity team to safeguard the quality of each article we publish.