- 1Department of Biotechnology, Al-Farabi Kazakh National University, Almaty, Kazakhstan
- 2Department of microbiology, Kazakh Medical University of Continuing Education, Almaty, Kazakhstan
- 3Medical Faculty Mannheim, University of Heidelberg, Heidelberg, Germany
The involvement of genes and miRNAs in the development of atherosclerosis is a challenging problem discussed in recent publications. It is necessary to establish which miRNAs affect the expression of candidate genes. We used known candidate atherosclerosis genes to predict associations. The quantitative characteristics of interactions of miRNAs with mRNA candidate genes were determined using the program, which identifies the localization of miRNA binding sites in mRNA, the free energy interaction of miRNA with mRNA. In mRNAs of GAS6 and NFE2L2 candidate genes, binding sites of 21 miRNAs and of 15 miRNAs, respectively, were identified. In IRS2 mRNA binding sites of 25 miRNAs were located in a cluster of 41 nt. In ADRB3, CD36, FASLG, FLT1, PLA2G7, and PPARGC1A mRNAs, clusters of miR-466, ID00436.3p-miR, and ID01030.3p-miR BS were identified. The organization of overlapping miRNA binding sites in clusters led to their compaction and caused competition among the miRNAs. The binding of 53 miRNAs to the mRNAs of 14 candidate genes with free energy interactions greater than −130 kJ/mole was determined. The miR-619-5p was fully complementary to ADAM17 and CD36 mRNAs, ID01593.5p-miR to ANGPTL4 mRNA, ID01935.5p-miR to NFE2L2, and miR-5096 to IL18 mRNA. Associations of miRNAs and candidate atherosclerosis genes are proposed for the early diagnosis of this disease.
Introduction
The diagnosis, prevention and treatment of atherosclerosis are important tasks of modern medicine (Byrne et al., 2014; Churov et al., 2019; Solly et al., 2019; Shoeibi, 2020). In most cases, polygenic diseases, including atherosclerosis, develop when the expression of different combinations of candidate genes changes. As a result of a review of various publications, it seems that the approaches used to search for markers for the diagnosis and treatment of atherosclerosis, including miRNA, have not yet solved the problem of diagnosing and treating atherosclerosis (Chen et al., 2020; Li et al., 2020; Ryu et al., 2020; Sun et al., 2020; Wang W. et al., 2020; You et al., 2020). In human, it is known about 7000 miRNA and more than 20,000 genes, and it is unknown how many miRNA and genes from them participate in the development of atherosclerosis (Byrne et al., 2014; Toba et al., 2014; Lu et al., 2018; Liu et al., 2020; Shi et al., 2020). As a rule, in publications several miRNA and some candidate genes are studied, so the combination of such attempts is large (Wang C. et al., 2020; Wang M. et al., 2020; Zhang et al., 2020). Considering the limitations of existing prediction methods, we can say that the establishment of adequate miRNA markers for diseases is unrealistic using existing approaches in the coming years. This statement is confirmed by the fact that over 20 years of studying miRNA participation in various diseases, diagnostic methods and therapeutic ways of treating diseases with miRNA are not used. Most researches study only the correlation between changes in miRNA concentration and expression of candidate genes, but this approach does not establish specific associations of miRNA and target gene. A number of publications argue that miRNA is the cause of disease without understanding that changes in miRNA concentration can occur through independent expression from intergenic regions or from host gene introns (Madrigal-Matute et al., 2013; Andreou et al., 2015; Feinberg and Moore, 2016; Solly et al., 2019). In all cases, miRNAs appear to modify the expression of target genes and not to cause the disease themselves. It is not taken into account that one miRNA can affect several or even 100s of genes, and one gene may be a target of several miRNAs (Atambayeva et al., 2017; Kondybayeva et al., 2018). In this article, we discuss what should be taken into consideration when reviewing the problem of miRNA interaction with candidate genes and show the need to use a systematic approach in establishing the most probable associations of miRNA and target genes.
It has been found that miRNAs, which are nanoscale regulatory biomolecules, are involved in many biological processes at all stages of the development of atherosclerosis, from early endothelial dysfunction to the erosion and rupture of an unstable atherosclerotic plaque (Cipollone et al., 2011; Madrigal-Matute et al., 2013; Andreou et al., 2015; Maitrias et al., 2015; Feinberg and Moore, 2016; Santovito et al., 2016; Chen et al., 2018; Ren et al., 2018; Wang et al., 2018). In addition, miRNAs are considered novel non-invasive biomarkers of the instability of atherosclerotic plaques and have been associated ischemic disorders (Churov et al., 2019). Their detection in the blood of patients may be a promising direction for the diagnosis of atherosclerosis complications such as ischemic stroke and myocardial infarction (Kanuri et al., 2018; Desjarlais et al., 2019; Moghaddam et al., 2019; Vargas-Alarcon et al., 2019; Velle-Forbord et al., 2019; Wiese et al., 2019; Kondybayeva et al., 2020). When the pathogenic role of a specific miRNA is confirmed, it can be considered a potential therapeutic target (Friedman et al., 2009; Fang et al., 2013; Moghaddam et al., 2019; Kazemi et al., 2020). According to the miRBase database, miRNAs have been found in many human tissues and are able to regulate the expression of more than 60% of all protein-coding genes (Kanuri et al., 2018; Ren et al., 2018; Wiese et al., 2019). Full complementarity between miRNA and mRNA results in degradation (Leidinger et al., 2012; Alagia and Eritja, 2016). However, incomplete complementarity is most often observed, in which case miRNAs suppress translation, generally by binding to the 3′-untranslated regions (3′ UTRs) of mRNAs (Ivashchenko et al., 2014a,c; Alagia and Eritja, 2016; Atambayeva et al., 2017). In addition, miRNAs can bind to other regions of target mRNAs, including the 5′-untranslated regions (5′ UTR) and coding sequence (CDS) (Orom et al., 2008; Forman and Coller, 2010; Ivashchenko et al., 2013; Zhou and Rigoutsos, 2014; Niyazova et al., 2015; Kondybayeva et al., 2018; Yurikova et al., 2019). We used a program that effectively determines the quantitative characteristics of the interaction of miRNA with mRNA and allows us to identify fundamentally new properties of the binding of miRNA to mRNA. The aim of this work was to identify associations between miRNAs and mRNA candidate genes of atherosclerosis for use as markers for the diagnosis of this disease.
Materials and Methods
The nucleotide sequences of 2565 miRNAs (we name this set as ‘old miRNAs’) were downloaded from the miRBase database1 (Release 22.1) and 3707 miRNAs (we name this set as ‘new miRNAs’) obtained from a report by Londin et al. (2015). Due to this work the number of known miRNAs had more than doubled. The nucleotide sequences of genes were obtained from GenBank2. A database of 68 candidate genes including the names of the genes and publication sources was compiled, confirming the associations of these genes with atherosclerosis (Supplementary Table S1). A search for the target genes of miRNAs was performed using the MirTarget program (Ivashchenko et al., 2014b). This program determines the following binding characteristics: the start of the miRNA binding site (BS) of mRNA; the locations of miRNA BS (3′ UTR, 5′ UTR, CDS); the interaction free energy (ΔG, kJ/mole); and nucleotide interaction schemes between miRNAs and mRNAs. The ratio of ΔG/ΔGm (%) was determined for each BS, where ΔGm is equal to the free energy binding of miRNA with its full complementary nucleotide sequence. The MirTarget program found hydrogen bonds between adenine (A) and uracil (U), guanine (G) and cytosine (C), G and U, A and C. The distances between A and C were equal 1.04 nanometers, between G and C, and between A and U were equal 1.03 nanometers, between G and U equal to 1.02 nanometers (Leontis et al., 2002). The numbers of hydrogen bonds in the G-C, A-U, G-U, and A-C interactions were found to be 3, 2, 1 and 1, respectively (Kool, 2001; Lemieux and Major, 2002). The MirTarget program determines single miRNA BS in mRNA and miRNA BS which are in clusters (BS arranged with overlapping of nucleotide sequences of the same or several miRNAs) (Aisina et al., 2019). Predicted by the MirTarget program binding sites in over 30 genes were confirmed experimentally (Yurikova et al., 2019).
Results
The BSs of the miRNAs and mRNAs of the target genes were not uniform along the length of the mRNAs. Both multiple and single BS were identified. BSs could be sequential or overlap with each other. Having overlapping nucleotide sequences in a cluster lead to the compaction of the mRNA sequence that is the target of several miRNAs. When the ΔG and ΔG/ΔGm values of miRNA BS are close to each other, it can be assumed that in the presence of equal miRNA concentrations, the miRNAs with a larger number of BS will be more likely to bind to the mRNAs of target genes. When the miRNA-mRNA interaction strength and the degree of their complementarity are similar, the miRNA has the highest concentration upon binding.
Characteristics of miRNA Interactions in the 5′ UTRs of the mRNAs of Atherosclerosis Candidate Genes
BSs of miRNAs were identified in the 5′ UTRs of 14 mRNAs. The GAS6 mRNA contains a large cluster with BS of 21 different miRNAs, half of which have two or three BS, while the rest represent single BS (Table 1). The cluster size is 39 nt, starting at the 11 nt position and ending at position 49 nt. The total BS length in the cluster is 824 nt, where the degree of compaction is 21. This compaction allowed this number of miRNA BS to be located in a 5′ UTR with a length of 153 nt. In an association analysis of seven miRNAs with the GAS6 mRNA the free energy interaction of the miRNAs with the mRNA is more than −130 kJ/mole, which indicates that these associations are promising markers of atherosclerosis.
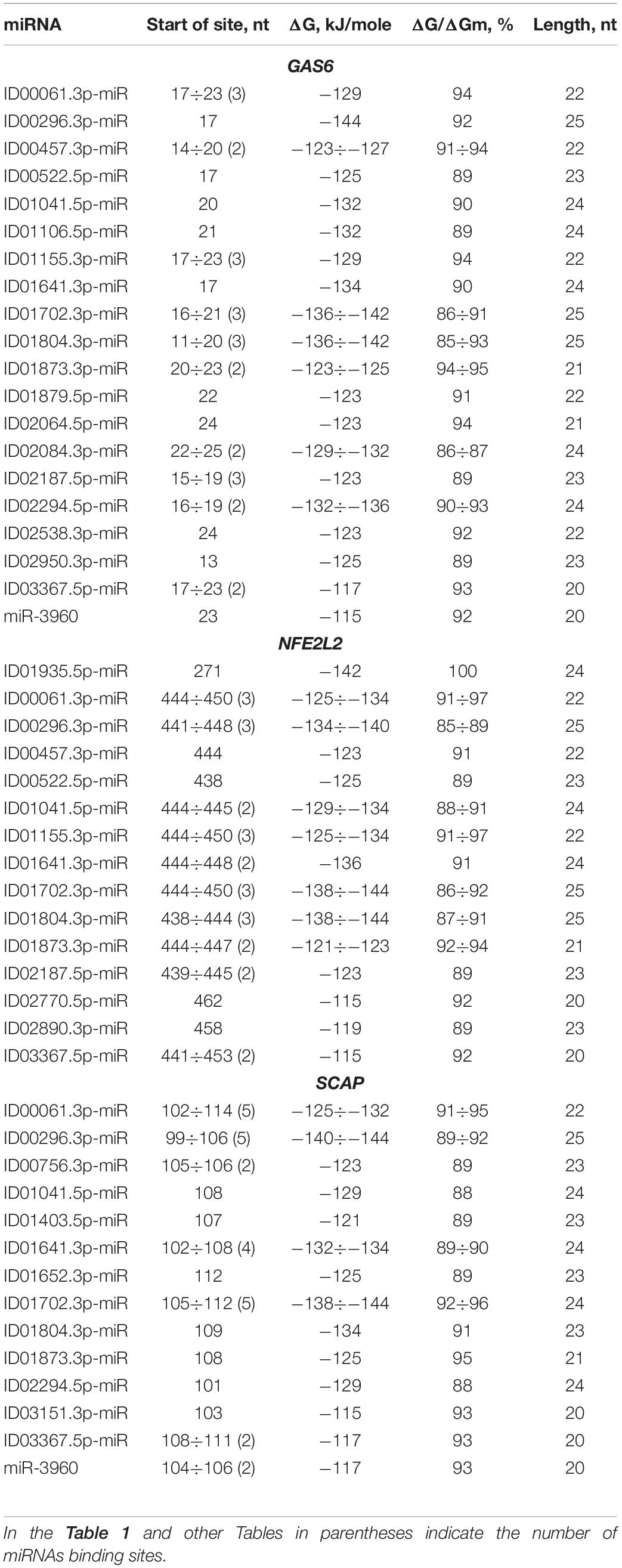
Table 1. Characteristics of miRNAs interaction in the 5′ UTR of mRNA of GAS6, NFE2L2, and SCAP genes.
To prove that the identified clusters are reliable, on the example of GAS6 gene showed that the cluster exists in the mRNA of orthological genes and is conservative in its nucleotide composition (Figure 1). There are mainly new miRNAs in the clusters of BSs in 5′ UTR region of mRNA of GAS6, NFE2L2 and SCAP genes. In mRNA of GAS6 gene the cluster includes 20 new miRNA and one old miRNA, in mRNA of NFE2L2 gene the cluster contains 15 BSs of new miRNA; in mRNA of SCAP gene there is a cluster for binding of 13 new and one old miRNA (Table 1). In the other 11 target genes, there are 28 new and one old miRNAs are linked in 5′ UTR region of mRNA (Supplementary Table S2). These data suggest that the use of new miRNAs significantly increased the number of effective gene expression modulators.
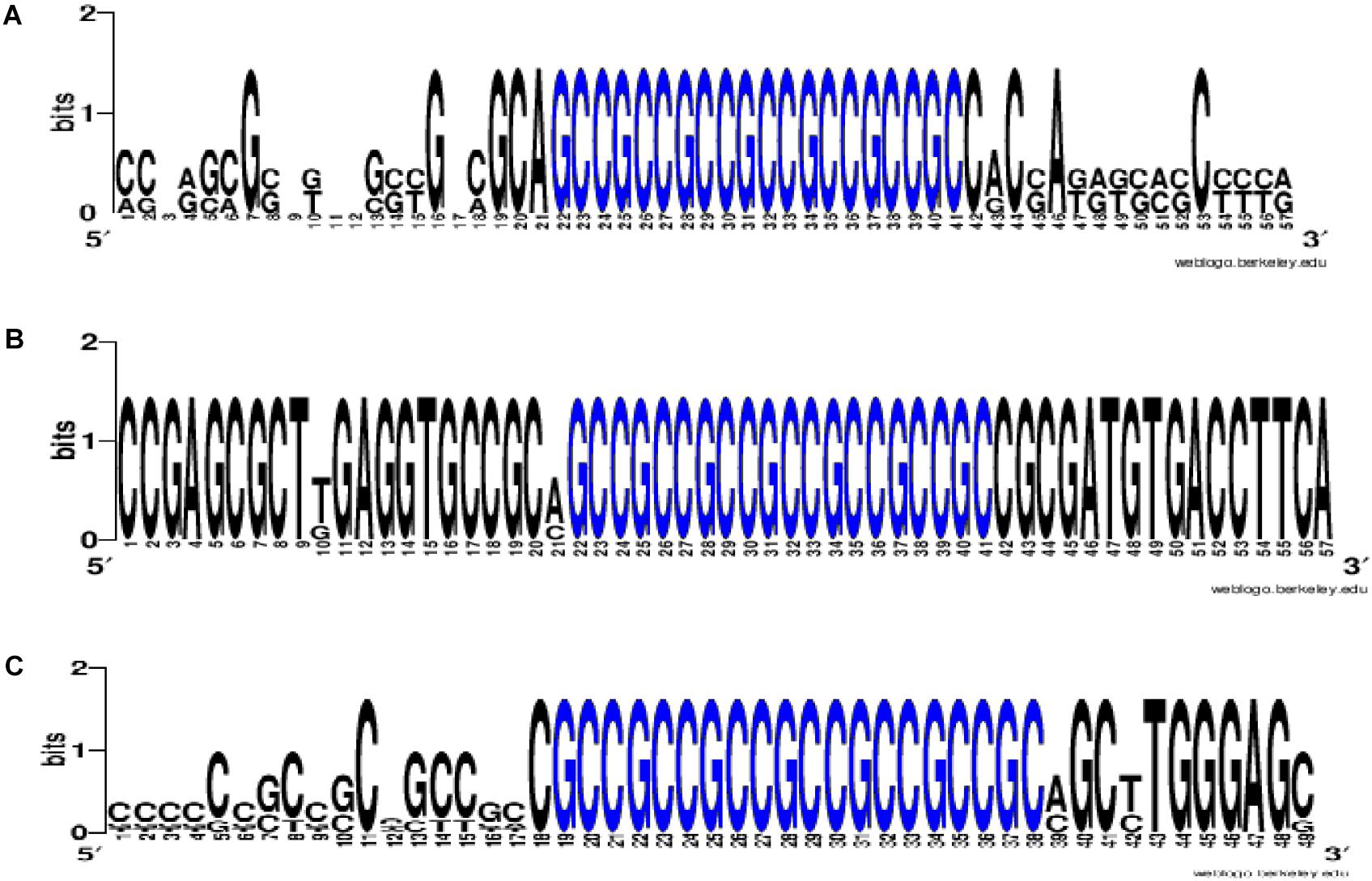
Figure 1. The WebLogo schemes (https://weblogo.berkeley.edu/logo.cgi) of nucleotide sequences for BS clusters in mRNA of GAS6 (A), NFE2L2 (B), SCAP (C) orthological genes. Nucleotides of BS clusters are highlighted in blue. Nucleotides of BS clusters mRNA of GAS6 Ggo (Gîrillà gîrillà), Hsa (Homo sapiens), Mmu (Macaca mulatta), Pab (Pongo abelii), Pan (papio anubis), Ptr (pan troglodytes); nucleotides of BS clusters mRNA of NFE2L2 Ggo, Hsa, Pab, Pan, Ptr; nucleotides of BS clusters mRNA of SCAP Ggo, Hsa, Mmu, Pab, Pan, Ptr.
Some miRNAs have multiple BSs: ID00061.3p-miR, ID01155.3p-miR, ID01702.3p-miR, ID01804.3p-miR, ID02187.5p-miR, ID00296.3p-miR, ID01155.3p-miR by three, ID01641.3p-miR by four, ID00061.3p-miR, ID00296.3p-miR, ID01702.3p-miR by five BSs. These miRNAs are recommended as associations for the diagnosis of atherosclerosis considering the high free energy interaction with mRNA of candidate genes. ID01935.5p-miR has a complete complementarity interaction with mRNA of NFE2L2 gene, which suggests that ID01935.5p-miR and NFE2L2 association should be recommended as a marker. These miRNA correlations clearly demonstrate the predominant influence of new miRNAs on gene expression with BS in 5′ UTR. Consequently, researchers actually do not receive information about the regulation of gene expression by new miRNAs, the effect of which is high when only the old miRNAs are studied.
The following circumstances need to be considered when evaluating the effects of many miRNAs in a cluster of BSs in mRNA. Primarily, miRNA will bind with higher free energy interaction, so quantitative interaction characteristics are needed. The ratio of miRNA concentrations between alternative miRNAs has a significant importance (miRNAs, which bind in the cluster) and especially in relation to the concentration of mRNA. Obviously, the total concentration of alternative miRNAs should be lower than the mRNA concentration, otherwise the protein will not be synthesized. A decrease of concentration of any miRNA below mRNA concentration will not affect protein synthesis. At the same time, an increase of one miRNA above mRNA concentration can completely suppress gene expression. The mRNA of many genes contains clusters of BSs of several similar miRNAs. In this way, nature has optimized the expression of several genes under common miRNA control. It is necessary to consider that many genes are expressed in cells of different tissues to different degrees, which may affect the dependence of protein synthesis from miRNA. However, a large number of binding miRNA may not allow the target gene expression to increase significantly. Many miRNAs have been found in the blood and serum in combination with AGO proteins and within exosomes, which indicates their capability to circulate freely throughout the body and to reach many organs and tissues. It is necessary to remember that the synthesis of intronic miRNAs depends on the expression of host gene, while the synthesis of other miRNAs is made from transcripts of intergenic regions. The reasons for changes in the expression of such miRNA in case of disease should be known. There are known changes of miRNA expression by several orders. Considering the above information, the reports are perceived cautiously that some researchers have been able to detect marker miRNA in specific diseases.
The above examples of presence of BS clusters in mRNA of some genes for several miRNAs suggest that such genes are expressed under the common control of the miRNA group and, consequently, these genes form a network of interconnected genes controlling key metabolic processes. To confirm the reliability of found miRNA and their BSs, it is possible to use data about the presence of such BSs or their clusters in orthological genes (Figure 1).
With the given examples we show the inadequacy of miRNA BSs establishment by programs based only on a miRNA seed sequence. The whole miRNA nucleotide sequence is important, which is confirmed by the conservation of miRNA nucleotide sequences and corresponding BS during millions of years. The nucleotide sequences of miRNA and their BSs have been conserved in the mRNA genes of animal and plant organisms over 10s of millions of years of evolution (Bari et al., 2013; Ivashchenko et al., 2013, 2014b; Yurikova et al., 2019).
ID00061.3p-miR, ID00296.3p-miR, ID00457.3p-miR, ID00522.5p-miR, ID01041.5p-miR, ID01155.3p-miR, ID01641.3p-miR, ID01702.3p-miR, ID01804.3p-miR, ID01873.3p-miR, ID02187.5p-miR and ID03367.5p-miR interact not only with GAS6 mRNA but also with NFE2L2 mRNA, where they form a large cluster together with other miRNAs. The cluster of miRNA BS in the NFE2L2 mRNA is one of the largest clusters of miRNAs associated with atherosclerosis-related genes in which BS are formed in the 5′ UTR. The cluster consists of 14 different miRNAs, ranging from the 438 to 482 nt positions, with a size of 45 nt. The total size of all binding sites is 669 nt, resulting in a degree of compaction of 15. Due to compaction, this length of BS is a small fraction of the 5′ UTR length of 555 nt. A complete complementarity interaction of ID01935.5p-miR with NFE2L2 mRNA was identified. Eight miRNAs interact with NFE2L2 mRNA with a free energy of more than −130 kJ/mole. These associations are recommended as valuable markers of atherosclerosis. The above examples of associations of miRNAs and target genes show that determining the expression level of one or more miRNA without monitoring target gene expression will not provide adequate data on the specific association of these miRNAs with the disease.
The ADAM10 mRNA contains BS for ID02761.3p-miR with overlapping nucleotide sequences (Supplementary Table S2). The ADCY9 mRNA is characterized by the presence of two clusters, each of which is formed by two single BS. In addition to this gene, clusters were identified in the following mRNAs: IRS2 and PIN1, which contain BS for two miRNAs. The PNPLA3 and mRNAs have a cluster consisting of three single BS. The CXCL12 mRNA contains a cluster of four different miRNAs, of which ID02036.3p-miR, ID01293.5p-miR, ID00417.3p-miR and ID02066.5p-miR have two BS. The cluster starts at 65 nt and ends at 93 nt, with a length of 29 nt and an average ΔG/ΔGm of 93%. The sum of the BS lengths of these miRNAs was 170 nt and was six times longer than the cluster length. Due to this compaction, all BS were located in a 5′ UTR with a length of 92 nt. The MMP2 mRNAs contained a cluster of six miRNA BS with a total length of 136 nt and a 5′ UTR length of 311 nt. The PNPLA3 mRNA contains a cluster of three miRNA BS. Among the clusters in the 5′ UTRs of the target genes, one can distinguish the SCAP gene cluster formed by BS from 14 miRNAs from the 99 nt to the 136 nt position, in which both single BS and multiple BS are found, more specifically, seven multiple sites and seven single sites (Table 1). Of the 14 miRNAs with the exception of ID00756.3p-miR, ID01403.5p-miR, ID01652.3p-miR and ID03151.3p-miR all were in the group of miRNAs binding to the GAS6 mRNA. Furthermore, ID00061.3p-miR, ID00296.3p-miR, and ID01702.3p-miR had five BS each, which significantly increased the likelihood of their binding compared to that of competing miRNAs. The total length of miRNA BS was 735 nt, and the degree of compaction was 19. Without compaction, these sites could not be located in this 255 nt long 5′ UTR. This cluster is characterized by a maximum ΔG of −144 kJ/mole for the BS of ID00296.3p-miR and ID01702.3p-miR. Of all the interactions of miRNAs and the 5′ UTRs of target mRNAs, five had a free energy of more than −130 kJ/mole. Convincing evidence of the effectiveness of the MirTarget program in determining the characteristics of miRNA binding to the 5′ UTR, CDS and 3′ UTR of mRNA is given in Figure 2. The diagrams show the interaction of nucleotides along the entire length of the miRNA in the BS of the target mRNA and the characteristics of this interaction.
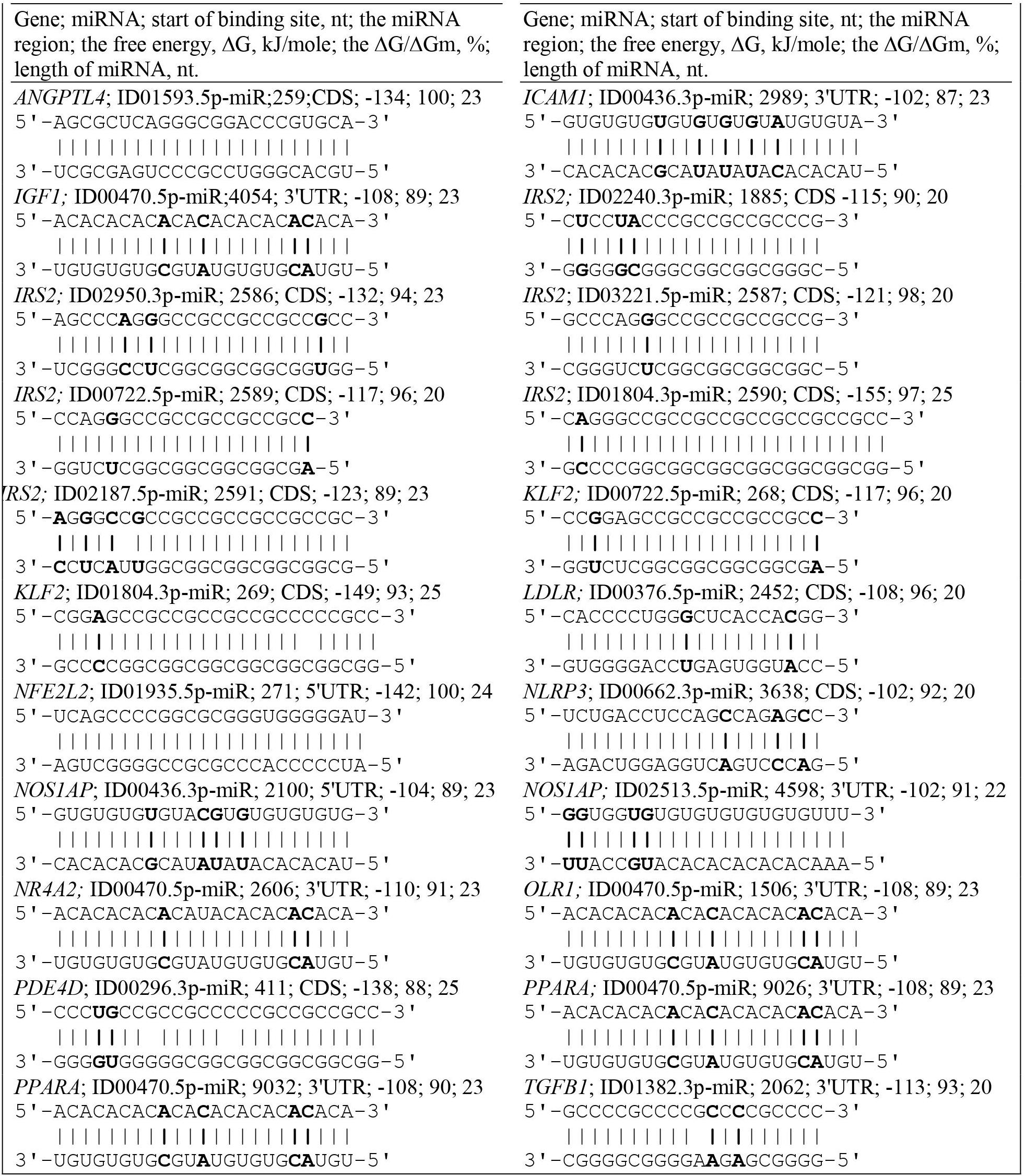
Figure 2. Convincing evidence of the effectiveness of the MirTarget program in determining the characteristics of miRNA binding to the 5′ UTR, CDS and 3′ UTR of mRNA. The diagrams show the interaction of nucleotides along the entire length of the miRNA in the BS of the target mRNA and the characteristics of this interaction. Non-canonical base pairs are in bold.
Characteristics of miRNA Interactions With the CDS of mRNAs of Atherosclerosis Candidate Genes
Most genes contained more one BS for one miRNA in the CDS of mRNAs. There were some genes with several BS or multiple BS where clusters could form. It is worth noting that IRS2 is characterized by the presence of three clusters and a large number of BS compared to other genes. This suggests that this gene is more susceptible to regulation by miRNA (Table 2). The first cluster was composed of 25 different miRNAs, 13 of which had two or more BS (ID00061.3p-miR, ID00457.3p-miR, ID00756.3p-miR, ID01155.3p-miR, ID01702.3p-miR, ID01804.3p-miR, ID01873.3p-miR, ID01879.5p-miR ID02064.5p-miR, ID02187.5p-miR, ID03229.5p-miR, ID03367.5p-miR, miR-3960), and the rest were composed of single BS. The cluster was 41 nt long, starting at 2586 nt and ending at 2626 nt. The total length of the BS in the cluster was 1114 nt, while the degree of cluster compaction was 28. The 2-nd cluster was formed by single BS of seven miRNAs. The 3-day cluster consisted of the BS of nine different miRNAs. ID00296.3p-miR and ID01702.3p-miR had three binding sites. In general, the cluster was 41 nt long, extending from position 4304 to 4344 nt. The total length of all the BS of the three clusters was 1607 nt, which is 40% of the total CDS length of 4017 nt. Due to compaction, the total length of all clusters is 110 nt, that is, only 2.7%. Thirteen miRNAs interacted with the IRS2 mRNA with a free energy of more than −130 kJ/mole, which gives reason to recommend these interactions as diagnostic markers of atherosclerosis. Each of the three clusters in the MR of the orthologous IRS2 genes encoded different highly homologous oligopeptides (Supplementary Table S3).
Clusters consisting of single miRNA BS were found in the following genes (Supplementary Table S4): two BS in ACE with an average ΔG of −128 kJ/mole; five BS in ADRB3 for five miRNAs with an average ΔG of −115 kJ/mole and BS in FASLG for ID00061.3p-miR, ID00296.3p-miR, ID01641.3p-miR, and ID01702.3p-miR. SIRT1 contained two sites for ID03332.3p-miR and single BS for ID00278.3p-miR and ID00811.3p-miR, with an average ΔG of −129 kJ/mole.
TBC1D10B gene is involved in the functioning of cardiovascular endothelial cells (Li et al., 2017) and it is a target of miR-762 with fully complementary binding (Supplementary Table S4). This site encodes the APAPAPAPAPAPA oligopeptide, with the flanking oligopeptides AWVPGSAQTS and VTGSTVVVLTL (Supplementary Table S5).
MRNA of CDKN1C gene (Rodriguez et al., 2007) contains three clusters consisting of both single and multiple BS. The first cluster consists of two BS for miR-762 and ID03129.3p-miR and has a size of 36 nt, extending from 738 to 753 nt. The second cluster is composed of 17 miR-762 BS, 3 ID00099.3p-miR BS, and 1 ID02682.5p-miR BS. ID00036.3p-miR, ID01075.3p-miR and ID00411.5p-miR cover a region of 31 nt, extending from the 888 nt position to 918 nt with an average ΔG value of −125 kJ/mole. The mRNA of the candidate gene CDKN1C has 17 sequentially located binding sites for miR-762 that encode the oligopeptide (AP)18, which indicates a strong dependence of gene expression on this miRNA (Supplementary Table S4). Orthologous primate genes (Supplementary Table S6) encode similar oligopeptides with an AP dipeptide number up to 33. In all primate cases, miR-762 BSs are located between the conserved flanking nucleotide sequences that encode the conserved AAPVAVAVLA and DAAPQESAEQ oligopeptides (Supplementary Table S6). These animals can be used to study the role of miR-762 in the development of atherosclerosis. A similar type of organization of miRNA binding multiple sites has been found in the mRNAs of many genes involved in the development of cardiovascular and other diseases (Kondybayeva et al., 2018; Aisina et al., 2019; Yurikova et al., 2019).
KLF2 is characterized by the presence of two clusters consisting of the BS for 14 and 2 different miRNAs. The first of these clusters is formed by single BS starting from the 264 to 296 nt position with a size of 33 nt (Table 2). The total length of all BS is 305 nt. The degree of compaction of the binding sites in the cluster is nine. The second cluster of this gene is formed only by two single BS. In the CDS of KLF2 mRNA BS were detected for ID00061.3p-miR, ID00457.3p-miR, ID01155.3p-miR, ID01702.3p-miR, ID01804.3p-miR, ID02187.5p-miR, ID03367.5p-miR which have BS in the 5′ UTR of GAS6 mRNA. This indicates an overall control of these genes expression by these miRNAs. The maximum ΔG/ΔGm value (100%) among these genes was detected at the ID01593.5p-miR BS, which interact with the ANGPTL4 mRNA.
PDE4D formed two clusters. The first cluster contained the BS for ID00061.3p-miR, ID01155.3p-miR, ID03064.3p-miR, miR-3960 and ID01702.3p-miR, which had three BS with a free energy value of −134 kJ/mole. ID01641.3p-miR, miR-3960 and ID01702.3p-miR BS were found in the second cluster. The size of the first cluster was 34 nt, extending from position 336 to 369 nt. The second cluster consisted of five multiple BS and eight single BS; the cluster size was 49 nt, extending from 391 to 439 nt. The total length of miRNA BS was 491 nt, and the degree of compaction was 10 (Supplementary Table S4).
There were 39 BS for new miRNAs and two BS for old miRNA in IRS2 mRNA. Two clusters of binding sites in the KLF2 mRNA interacted with 14 new miRNAs and one old miRNA. In addition, there were 15 BS for new miRNA and two BS for miR-3960 in the PDE4D mRNA. A total in CDS mRNA of 40 target genes BS were identified for 148 new miRNAs and 32 old miRNAs.
Some miRNAs had more than two BS: ID01641.3p-miR, ID02064.5p-miR, ID02187.5p-miR, ID02770.5p-miR, ID03324.3p-miR, ID03367.5p-miR – 3 BS; ID00296.3p-miR, ID01377.3p-miR, ID01804.3p-miR, miR-3960 – 4 BS; ID00457.3p-miR, ID01155.3p-miR – 5 BS and ID00061.3p-miR, ID01702.3p-miR – 6 BS. Most of them had free energy interaction above −130 kJ/mole.
Three clusters of miRNA BS in CDS mRNA of orthologic genes encode different oligopeptides because they have different reading frames. Oligopeptides encoded by BS are clearly defined by conservative oligopeptides, which flank oligopeptides encoded by BS. Apparently, flanking oligopeptides carry out the important functional role of a protein, and oligopeptides coded by BS in the first and second clusters change for the reason of an acceptability of incomplete complementary interactions of miRNA and mRNA.
Characteristics of miRNA Interactions in the 3′ UTRs of mRNAs of Atherosclerosis Candidate Genes
The 40 genes involved in the development of atherosclerosis were characterized by BS in the 3′ UTRs. A feature of miRNA interactions with atherosclerosis candidate genes is the presence of BS for miR-466, ID00436.3p-miR, and ID01030.3p-miR in their mRNAs (Table 3 and Supplementary Table S7). The BS of these miRNAs can be single or multiple and could form a cluster. For example, CD36 mRNA contains six BS for ID00436.3p-miR and five BS for miR-466 and ID01030.3p-miR. FASLG mRNA contains six BS for miR-466 and ID00436.3p-miR and five BS for ID01030.3p-miR. The mRNA of the FLT1 gene contains eight BS for miR-466 and seven BS for ID00436.3p-miR and ID01030.3p-miR. Another feature of candidate genes is the presence of clusters of binding sites for miR-5095 and miR-619-5p in their mRNA. There are two clusters of BS of miR-5095 and miR-619-5p located 12 nucleotides apart in IL18 mRNA. In these clusters, the start sites of miR-5095 and miR-619-5p BS are located six nucleotides apart, which is probably due to their common origin. In the mRNAs of CD36 and ADAM17 completely complementary BS with miR-619-5p and BS between the mRNA of IL18 with miR-5096 were revealed. The BS of miR-1273f and miR-1273e form a cluster in the mRNAs of IGF1 gene (Supplementary Table S7). The 3′ UTRs of the mRNAs of many candidate genes contain only single miRNA BS that do not form clusters: ABO, ACE, ADAM17, ADAM33, ANGPT2, APOL1, CD59, FADS2, FOXP3, GPR132, HNF1A, IGF1R, LDLR, LPCAT3, NOS1AP, PNPLA3, PPARA, SOAT1, SOCS3, TFPI, TNC, and ZBTB46. Some genes are characterized by clusters consisting of two single BS: BRCA1, F11R, IGF1, ITGA2, and TNFSF10. Some genes were characterized by mixed clusters formed by both single and multiple BS. NR4A2 had a cluster consisting of both single BS of ID00470.5p-miR and multiple BS of ID02299.5p-miR (Supplementary Table S7).
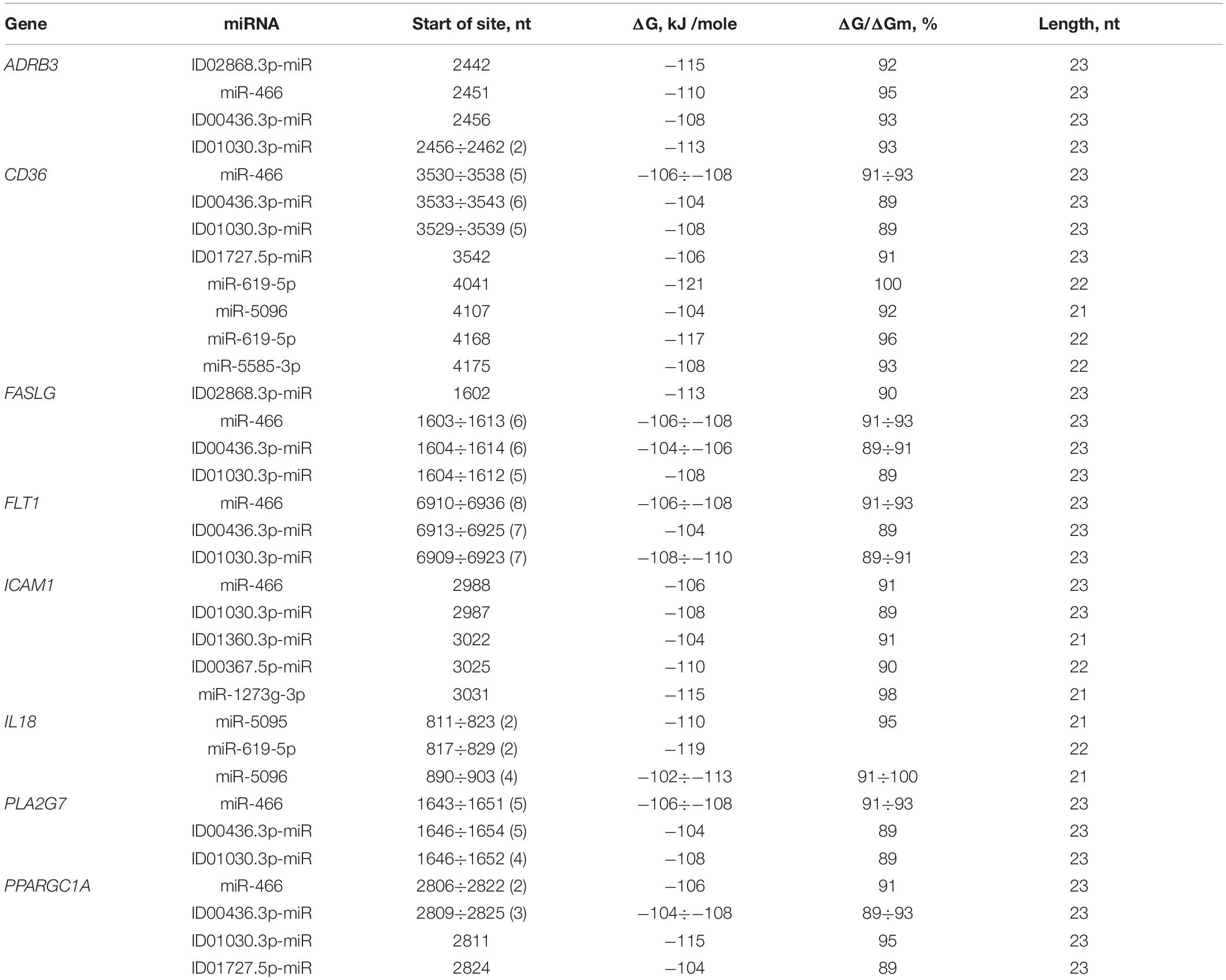
Table 3. Characteristics of miRNAs interaction in the 3′ UTR of mRNA of atherosclerosis candidate genes.
FASLG was characterized by a cluster formed from a miRNA with single BS (ID02868.3p-miR) and three miRNAs with multiple BS consisting of six BS for miR-466, five for ID01030.3p-miR and six for ID00436.3p-miR, extending from 1602 to 1637 nt with an average ΔG value of −108 kJ/mole. In the 3′ UTR of FLT1, there was a cluster of three multiple BS consisting of seven BS for ID01030.3p-miR, seven for ID00436.3p-miR and eight for miR-466, extending from 6909 to 6959 nt and with an average ΔG value of −107 kJ/mole. IL18 had a cluster of two miR-5095 and two miR-619-5p sequences, with a size of 41 nt and an average ΔG value of −114 kJ/mole. MTHFR mRNA contained one cluster, consisting of ID01811.5p-miR, miR-5095 and miR-619-5p with an average ΔG value of −114 kJ/mole. PPARGC1A contained a cluster formed by four different miRNAs, including two miR-466 BS and three ID00436.3p-miR, ID01030.3p-miR, and ID01727.5p-miR sequences. The size of this cluster was 43 nt, extending from 2806 to 2848 nt. There were some genes with more than one cluster. The PLA2G7 mRNA had a cluster that consisted of five miR-466, four ID01030.3p-miR and five ID00436.3p-miR sites and a length of 35 nt, extending from 1643 to 1677 nt, with an average ΔG value of −106 kJ/mole.
ICAM1 was characterized by the presence of two clusters, the first of which consisted of two single BS (ID01030.3p-miR and miR-466), extending from 2987 to 3011 nt. The second cluster consisted of three BS of different miRNAs. ID00470.5p-miR formed BS in OLR1 and NR4A2 mRNAs. The cluster of miR-1273f and miR-1273e BS was formed in IGF1 mRNA.
There were miRNAs that had BS for more than one mRNA. The unique miR-619-5p had BS in the mRNAs of ADAM17, ADAM33, APOL1, BRCA1, F11R, IL18, ITGA2, LDLR, MTHFR, PNPLA3, SOAT1, and TNFSF10. ID00436.3p-miR and ID01030.3p-miR formed clusters that consisted of multiple BS for ADRB3, F11R, FASLG, FLT1, PLA2G7, and PPARGC1A mRNA. ID01727.5p-miR had BS for NOS1AP and PPARGC1A mRNAs.
The CD36 mRNA cluster, consisting of BS to two different miRNAs, miR-619-5p and miR-5585-3p, extended from 4168 to 4197 nt. The CD36 mRNA cluster consisted of both single BS and multiple BS. In general, the cluster consisted of five ID01030.3p-miR, five miR-466, six ID00436.3p-miR and one ID01727.5p-miR sequences. The size was 38 nt, extending from 3529 to 3566 nt, with an average ΔG value of −106 kJ/mole.
Associations of miRNA and target genes having BS in 3′ UTR mRNA are very different from those in 5′ UTR and CDS. In the 3′ UTR mRNA there are no clusters of BS over four miRNAs. The number of old and new miRNAs is comparable. The total number of binding sites of new miRNA is 57 and 63 old miRNA per mRNA of 40 target genes. This is probably due to the fact that the GC content of old miRNAs is comparable to that of 3′ UTRs, while in 5′ UTR and CDS it is about 10% higher. In the new miRNA GC content is comparable to that in 5′ UTR and CDS, so the number of BS for new miRNA in these regions of mRNA is much larger.
The choice of miRNA-gene associations is difficult to offer as miRNAs such as miR-619-5p, miR- 5095-, miR-5096- and miR-5585-3p, which have targets in many genes and most likely play the role of stabilizers of protein expression. Similar to miR-466, ID00436.3p-miR and ID01030.3p-miR, which have multiple BS in the mRNA of many genes, which also gives them the role of stabilizing the expression of their targets. A significant increase in the concentration of any these miRNAs will lead to numerous metabolic disorders and to different diseases consequently. If about 100–200 key miRNAs are identified with miRNA-chips, the range of candidate genes will be significantly reduced because with bioinformatic approaches it could be done easily and quickly.
Discussion
Since it would be advisable to have lower costs for the diagnosis of the disease, it is desirable to minimize the number of associations of miRNA and target genes. However, in the case of polygenic diseases, such methods could be difficult to develop because it is not known which gene and which miRNA cause a particular patient’s disease. Therefore, now it is necessary to select a list of priority genes for diagnosis and then check which miRNA could influence protein expression. With the MirTarget program, this is easily to install and, if alternative miRNAs are involved, it is also simple to identify miRNAs competitiveness. The results of the present research demonstrate the effectiveness of this approach.
It was found that the BS of some miRNAs formed clusters in the 5′ UTR and CDS sequences of mRNA, resulting in competition between these miRNAs for binding to mRNA and, accordingly, for suppressing the expression of the target gene. The quantitative characteristics of miRNA BS with mRNA make it possible to predict which miRNAs can more efficiently bind to mRNA at equal miRNA concentrations. At different concentrations of these miRNAs, kinetic equations can be used to predict their suppressive effect on the expression of the target gene. The revealed associations of several miRNAs that bind to mRNAs of different candidate genes make it possible to predict the effect of these miRNAs on the corresponding genes, which can be expressed to different degrees. For example, GAS6, NFE2L2, and IRS2 are targets of several identical miRNAs and bind with these miRNAs depending on their concentrations in the cell. In cells of different tissues, the ratio of miRNA and target gene concentrations will be different, and the effect of the miRNAs on these target genes will also be different. This brief discussion of the different interactions of miRNAs and candidate genes under different circumstances shows the complexity of these networks and begins to paint a picture of the interactions of miRNA groups with groups of target genes. Without this assumption, it is difficult to predict or evaluate the involvement of a single miRNA or a single gene in the development of a disease. From the identified interactions, it is necessary to choose those that control more genes and miRNAs and reflect the development of atherosclerosis. A number of mRNAs and miRNAs strongly interacting with each other are proposed in this study. For example, PDE4D and ID00296.3p-miR; SCAP and ID00296.3p-miR and ID01702.3p-miR; ADCY9 and ID00296.3p-miR; IRS2 and ID01702.3p-miR, ID03064.3p-miR, ID01804.3p-miR, and ID00296.3p-miR; NFE2L2 and ID01935.5p-miR, ID00296.3p-miR, ID01702.3p-miR, and ID01804.3p-miR. In addition, miRNAs that bind with mRNAs of candidate genes with complete complementarity are of interest. Within addition to this condition, it is necessary to consider miRNAs interacting with a large number of genes, since such miRNAs can simultaneously control the expression of many genes. Genes that are regulated by a large number of miRNAs can also be considered as effective markers. Unfortunately, our knowledge is still insufficient to determine which candidate genes play a key role in the development of the disease, and it is not known which miRNAs are the most likely signals for the development of atherosclerosis. However, one thing is obvious – it is necessary to simultaneously study miRNA and gene interactions in order to improve the likelihood of obtaining a valid result. From the established interactions, it is first necessary to consider the interactions between candidate genes with multiple miRNAs that form clusters of BS and miRNA interactions with high free energy. For example, IRS2 is the target of 41 miRNAs with BS located in the CDS, of which ID00061.3p-miR, ID00296.3p-miR, ID00457.3p-miR, ID01041.5p-miR, ID01155.3p-miR, ID01641.3p-miR, ID01702.3p-miR, ID01778.3p-miR, ID01804.3p-miR, ID01895.5p-miR, ID02052.5p-miR, ID02064.5p-miR, ID02950.3p-miR, and ID03064.3p-miR have free energy interactions above −130 kJ/mole. The mRNA of GAS6 interacts with 21 miRNAs, of which those with ID00296.3p-miR, ID01041.5p-miR, ID01106.5p-miR, ID01641.3p-miR, ID01702.3p-miR, ID01804.3p-miR, ID02084.3p-miR, and ID02294.5p-miR are more efficient. Associations of this type were observed for NFE2L2, ADCY9, PNPLA3, SCAP, KLF2 and PDE4D, which bind to many miRNAs with high free energy. Another type of association is the interaction of one or more miRNA with many genes. For example, miR-466, ID00436.3p-miR and ID01030.3p-miR bind with the mRNAs of ADRB3, CD36, FASLG, FLT1, PLA2G7, and PPARGC1A. In addition, miR-619-5p targets several candidate atherosclerosis genes: CD36, IL18, ADAM17, ADAM33, APOL1, BRCA1, F11R, ITGA2, LDLR, MTHFR, PNPLA3, SOAT1, and TNFSF10. The mRNA of ANGPTL4 is fully complementary with ID01593.5p-miR; the mRNAs of ADAM17 and CD36 with miR-619-5p; the mRNA of IL18 with miR-5096; and the mRNA of NFE2L2 with ID01935.5p-miR.
Here, miRNA interactions with candidate atherosclerosis genes have been established, which consist of one gene and several miRNAs, one gene and one miRNA, one miRNA and several genes, and two or more miRNAs with two or more candidate genes. The revealed cluster organization of miRNA BS in mRNA candidate genes contributes to a more accurate diagnosis of the participation of competing miRNAs in the development of atherosclerosis.
Data Availability Statement
The datasets presented in this study can be found in online repositories. The names of the repository/repositories and accession number(s) can be found in the article/ Supplementary Material.
Author Contributions
AI and DM conceived of the study, drafted the manuscript, and gave final approval of the version to be published. SL, DA, AP, and AR conceived of the study and drafted the manuscript. All authors made substantial contributions to acquisition of data, to interpretation and modification of the data, were involved in subsequent rounds of revisions, and read and approved the final manuscript.
Funding
The work was carried out with the financial support of the Ministry of Education and Science of the Republic of Kazakhstan within the framework of the grant no. AP05132460. We thank the European Union Horizon 2020 research and innovation program (grant agreement no. 645648) ‘Muscle Stress Relief.’
Conflict of Interest
The authors declare that the research was conducted in the absence of any commercial or financial relationships that could be construed as a potential conflict of interest.
Supplementary Material
The Supplementary Material for this article can be found online at: https://www.frontiersin.org/articles/10.3389/fgene.2020.605054/full#supplementary-material
Footnotes
References
Aisina, D., Niyazova, R., Atambayeva, S., and Ivashchenko, A. (2019). Prediction of clusters of miRNA binding sites in mRNA candidate genes of breast cancer subtypes. PeerJ. 7:e8049. doi: 10.7717/peerj.8049
Alagia, A., and Eritja, R. (2016). siRNA and RNAi optimization. Wiley Interdiscip. Rev. RNA. 7, 316–329. doi: 10.1002/wrna.1337
Andreou, I., Sun, X., Stone, P. H., Edelman, E. R., and Feinberg, M. W. (2015). MiRNAs in atherosclerotic plaque initiation, progression, and rupture. Trends Mol. Med. 21, 307–318. doi: 10.1016/j.molmed.2015.02.003
Atambayeva, S., Niyazova, R., Ivashchenko, A., Pyrkova, A., Pinsky, I., Akimniyazova, A., et al. (2017). The binding sites of miR-619-5p in the mRNAs of human and orthologous genes. BMC Genomics 18:428. doi: 10.1186/s12864-017-3811-6
Bari, A., Orazova, S., and Ivashchenko, A. (2013). miR156- and miR171-binding sites in the protein-coding sequences of several plant genes. BioMed Res. Int. 2013:307145. doi: 10.1155/2013/307145
Byrne, M. M., Murphy, R. T., and Ryan, A. W. (2014). Epigenetic modulation in the treatment of atherosclerotic disease. Front. Genet. 5:364. doi: 10.3389/fgene.2014.00364
Chen, W., Yu, F., Di, M., Li, M., Chen, Y., Zhang, Y., et al. (2018). MicroRNA-124-3p inhibits collagen synthesis in atherosclerotic plaques by targeting prolyl 4-hydroxylase subunit alpha-1 (P4HA1) in vascular smooth muscle cells. Atherosclerosis 277, 98–107. doi: 10.1016/j.atherosclerosis.2018.08.034
Chen, F., Ye, X., Jiang, H., Zhu, G., and Miao, S. J. (2020). MicroRNA-151 attenuates apoptosis of endothelial cells induced by oxidized low-density lipoprotein by targeting interleukin-17A (IL-17A). Cardiovasc. Transl. Res. doi: 10.1007/s12265-020-10065-w
Churov, A., Summerhill, V., Grechko, A., Orekhova, V., and Orekhov, A. (2019). MicroRNAs as potential biomarkers in atherosclerosis. Int. J. Mol. Sci. 20:E5547. doi: 10.3390/ijms20225547
Cipollone, F., Felicioni, L., Sarzani, R., Ucchino, S., Spigonardo, F., Mandolini, C., et al. (2011). A unique microRNA signature associated with plaque instability in humans. Stroke 42, 2556–2563. doi: 10.1161/STROKEAHA.110.597575
Desjarlais, M., Dussault, S., Rivard, F., Harel, S., Sanchez, V., Hussain, S. N. A., et al. (2019). Forced expression of microRNA-146b reduces TRAF6-dependent inflammation and improves ischemia-induced neovascularization in hypercholesterolemic conditions. Atherosclerosis 289, 73–84. doi: 10.1016/j.atherosclerosis.2019.08.010
Fang, Z., Du, R., Edwards, A., Flemington, E. K., and Zhang, K. (2013). The sequence structures of human microRNA molecules and their implications. PLoS One 8:e54215. doi: 10.1371/journal.pone.0054215
Feinberg, M. W., and Moore, K. J. (2016). MicroRNA regulation of atherosclerosis. Circ. Res. 118, 703–720. doi: 10.1161/CIRCRESAHA.115.306300
Forman, J. J., and Coller, H. A. (2010). The code within the code: microRNAs target coding regions. Cell Cycle 9, 1533–1541. doi: 10.4161/cc.9.8.11202
Friedman, R. C., Farh, K. K., Burge, C. B., and Bartel, D. P. (2009). Most mammalian mRNAs are conserved targets of microRNAs. Genome Res. 19, 92–105. doi: 10.1101/gr.082701.108
Ivashchenko, A., Berillo, O., Pyrkova, A., and Niyazova, R. (2014a). Binding sites of miR-1273 family on the mRNA of target genes. Biomed Res. Int. 2014:e11. doi: 10.1155/2014/620530
Ivashchenko, A., Berillo, O., Pyrkova, A., Niyazova, R., and Atambayeva, S. (2014b). MiR-3960 binding sites with mRNA of human genes. Bioinformation 10, 423–427. doi: 10.6026/97320630010423
Ivashchenko, A., Berillo, O., Pyrkova, A., Niyazova, R., and Atambayeva, S. (2014c). The properties of binding sites of miR-619-5p, miR-5095, miR-5096 and miR-5585-3p in the mRNAs of human genes. Biomed Res. Int. 2014:e8. doi: 10.1155/2014/720715
Ivashchenko, A., Issabekova, A. S., and Berillo, O. A. (2013). miR-1279, miR-548j, miR-548m, and miR-548d-5p binding sites in CDSs of paralogous and orthologous PTPN12. MSH6, and ZEB1 Genes. Biomed. Res. Int. 2013:e10. doi: 10.1155/2013/902467
Kanuri, S. H., Ipe, J., Kassab, K., Gao, H., Liu, Y., Skaar, T. C., et al. (2018). Next generation MicroRNA sequencing to identify coronary artery disease patients at risk of recurrent myocardial infarction. Atherosclerosis 278, 232–239. doi: 10.1016/j.atherosclerosis.2018.09.021
Kazemi, F. T., Tavakoli, S., Ahmadi, R., Moradi, N., Fadaei, R., Mohammadi, A., et al. (2020). Evaluation of IP10 and miRNA 296-a expression levels in peripheral blood mononuclear cell of coronary artery disease patients and controls. DNA Cell Biol. 39, 1678–1684. doi: 10.1089/dna.2020.5650
Kondybayeva, A., Akimniyazova, A., Kamenova, S., Duchshanova, G., Aisina, D., Goncharova, A., et al. (2020). Prediction of miRNA interaction with mRNA of stroke candidate genes. Neurol. Sci 41, 799–808. doi: 10.1007/s10072-019-04158-x
Kondybayeva, A. M., Akimniyazova, A. N., Kamenova, S. U., and Ivashchenko, A. T. (2018). The characteristics of miRNA binding sites in mRNA of ZFHX3 gene and its orthologs. Vavilov J. Genet. Breed. 22, 438–444. doi: 10.18699/VJ18.380
Kool, E. T. (2001). Hydrogen bonding, base stacking, and steric effects in DNA replication. Annu. Rev. Biophys. Biomol. Struct. 30, 1–22. doi: 10.1146/annurev.biophys.30.1.1
Leidinger, P., Keller, A., and Meese, E. (2012). MicroRNAs – important molecules in lung cancer research. Front. Genet. 2:104. doi: 10.3389/fgene.2011.00104
Lemieux, S., and Major, F. (2002). RNA canonical and non-canonical base pairing types: a recognition method and complete repertoire. Nucleic Acids Res. 30, 4250–4263. doi: 10.1093/nar/gkf540
Leontis, N. B., Stombaugh, J., and Westhof, E. (2002). The non-watson-crick base pairs and their associated isostericity matrices. Nucleic Acids Res. 30, 3497–3531. doi: 10.1093/nar/gkf481
Li, Q., Cui, H. H., Yang, Y. J., Li, X. D., Chen, G. H., Tian, X. Q., et al. (2017). Quantitative proteomics analysis of ischemia/reperfusion injury-modulated proteins in cardiac microvascular endothelial cells and the protective role of tongxinluo. Cell Physiol. Biochem. 41, 1503–1518. doi: 10.1159/000470806
Li, X., He, X., Wang, J., Wang, D., Cong, P., Zhu, A., et al. (2020). The Regulation of Exosome-Derived miRNA on Heterogeneity of Macrophages in Atherosclerotic Plaques. Front. Immunol. 11:2175. doi: 10.3389/fimmu.2020.02175
Liu, D., Song, J., Ji, X., Liu, Z., Li, T., and Hu, B. (2020). PRDM16 upregulation induced by MicroRNA-448 inhibition alleviates atherosclerosis via the TGF-β signaling pathway inactivation. Front. Physiol. 11:846. doi: 10.3389/fphys.2020.00846
Londin, E., Loher, P., Telonis, A. G., Quann, K., Clark, P., Jing, Y., et al. (2015). Analysis of 13 cell types reveals evidence for the expression of numerous novel primate- and tissue-specific microRNAs. Proc. Natl. Acad. Sci. U.S.A. 112, 1106–1115. doi: 10.1073/pnas.1420955112
Lu, Y., Thavarajah, T., Gu, W., Cai, J., and Xu, Q. (2018). Impact of miRNA in Atherosclerosis. Arterioscler. Thromb. Vasc. Biol. 38, e159–e170. doi: 10.1161/ATVBAHA.118.310227
Madrigal-Matute, J., Rotllan, N., Aranda, J. F., and Fernandez-Hernando, C. (2013). MicroRNAs and atherosclerosis. Curr. Atheroscler. Rep. 15:322. doi: 10.1007/s11883-013-0322-z
Maitrias, P., Metzinger-Le Meuth, V., Massy, Z. A., M’Baya-Moutoula, E., Reix, T., Caus, T., et al. (2015). MicroRNA deregulation in symptomatic carotid plaque. J. Vasc. Surg. 62, 1245–1250. doi: 10.1016/j.jvs.2015.06.136
Moghaddam, A. S., Afshari, J. T., Esmaeili, S. A., Saburi, E., Joneidi, Z., and Momtazi-Borojeni, A. A. (2019). Cardioprotective microRNAs: lessons from stem cell-derived exosomal microRNAs to treat cardiovascular disease. Atherosclerosis 285, 1–9. doi: 10.1016/j.atherosclerosis.2019.03.016
Niyazova, R., Berillo, O., Atambayeva, S., Pyrkova, A., Alybayeva, A., and Ivashchenko, A. (2015). MiR-1322 binding sites in paralogous and orthologous genes. BioMed. Res. Int. 1:e7. doi: 10.1155/2015/962637
Orom, U. A., Nielsen, F. C., and Lund, A. H. (2008). MicroRNA-10a binds the 5′UTR of ribosomal protein mRNAs and enhances their translation. Mol. Cell. 30, 460–471. doi: 10.1016/j.molcel.2008.05.001
Ren, K., Zhu, X., Zheng, Z., Mo, Z. C., Peng, X. S., Zeng, Y. Z., et al. (2018). MicroRNA-24 aggravates atherosclerosis by inhibiting selective lipid uptake from HDL cholesterol via the post-transcriptional repression of scavenger receptor class B type I. Atherosclerosis 270, 57–67. doi: 10.1016/j.atherosclerosis.2018.01.045
Rodriguez, I., Coto, E., Reguero, J. R., Gonzalez, P., Andres, V., Lozano, I., et al. (2007). Role of the CDKN1A/p21, CDKN1C/p57, and CDKN2A/p16 genes in the risk of atherosclerosis and myocardial infarction. Cell Cycle 6, 620–625. doi: 10.4161/cc.6.5.3927
Ryu, J., Ahn, Y., Kook, H., and Kim, Y. K. (2020). The roles of non-coding RNAs in vascular calcification and opportunities as therapeutic targets. Pharmacol. Ther. e107675. doi: 10.1016/j.pharmthera.2020.107675
Santovito, D., Egea, V., and Weber, C. (2016). Small but smart: MicroRNAs orchestrate atherosclerosis development and progression. Biochim. Biophys. Acta 1861, 2075–2086. doi: 10.1016/j.bbalip.2015.12.013
Shi, X., Xie, X., Sun, Y., He, H., Huang, H., Liu, Y., et al. (2020). Paeonol inhibits NLRP3 mediated inflammation in rat endothelial cells by elevating hyperlipidemic rats plasma exosomal miRNA-223. Eur. J. Pharmacol. 885:173473. doi: 10.1016/j.ejphar.2020.173473
Shoeibi, S. (2020). Diagnostic and theranostic microRNAs in the pathogenesis of atherosclerosis. Acta Physiol. 228:E13353. doi: 10.1111/apha.13353
Solly, E. L., Dimasi, C. G., Bursill, C. A., Psaltis, P. J., and Tan, J. T. M. (2019). MicroRNAs as therapeutic targets and clinical biomarkers in atherosclerosis. J. Clin. Med. 8:E2199. doi: 10.3390/jcm8122199
Sun, B., Shan, Z., Sun, G., and Wang, X. (2020). MicroRNA-183-5p acts as a potential diagnostic biomarker for atherosclerosis and regulates the growth of vascular smooth muscle cell. J. Chin. Med. Assoc. doi: 10.1097/JCMA.0000000000000433
Toba, H., Lindsey, M. L., and Chilton, R. J. (2014). Applications of miRNA technology for atherosclerosis. Curr. Atheroscler. Rep. 16:386. doi: 10.1007/s11883-013-0386-9
Vargas-Alarcon, G., Perez-Hernandez, N., Rodriguez-Perez, J. M., Fragoso, J. M., Cardoso-Saldana, G., Vazquez-Vazquez, C., et al. (2019). MRE11A polymorphisms are associated with subclinical atherosclerosis and cardiovascular risk factors. a case-control study of the GEA mexican project. Front. Genet. 10:530. doi: 10.3389/fgene.2019.00530
Velle-Forbord, T., Eidlaug, M., Debik, J., Sæther, J. C., Follestad, T., Nauman, J., et al. (2019). Circulating microRNAs as predictive biomarkers of myocardial infarction: evidence from the HUNT study. Atherosclerosis 289, 1–7. doi: 10.1016/j.atherosclerosis.2019.07.024
Wang, C., Yang, W., Liang, X., Song, W., Lin, J., Sun, Y., et al. (2020). MicroRNA-761 modulates foam cell formation and inflammation through autophagy in the progression of atherosclerosis. Mol. Cell. Biochem. 474, 135–146. doi: 10.1007/s11010-020-03839-y
Wang, M., Li, J., Cai, J., Cheng, L., Wang, X., Xu, P., et al. (2020). Overexpression of MicroRNA-16 alleviates atherosclerosis by inhibition of inflammatory pathways. Biomed Res. Int. 2020:8504238. doi: 10.1155/2020/8504238
Wang, W., Ma, F., and Zhang, H. (2020). MicroRNA-374 is a potential diagnostic biomarker for atherosclerosis and regulates the proliferation and migration of vascular smooth muscle cells. Cardiovasc. Diagn. Ther 10, 687–694. doi: 10.21037/cdt-20-444
Wang, Y., Zhu, J., Handberg, A., Overvad, K., Tjønneland, A., Rimm, E. B., et al. (2018). Association between plasma CD36 levels and incident risk of coronary heart disease among Danish men and women. Atherosclerosis 277, 163–168. doi: 10.1016/j.atherosclerosis.2018.08.045
Wiese, C. B., Zhong, J., Xu, Z. Q., Zhang, Y., Ramirez Solano, M. A., Zhu, W., et al. (2019). Dual inhibition of endothelial miR-92a-3p and miR-489-3p reduces renal injury-associated atherosclerosis. Atherosclerosis 282, 121–131. doi: 10.1016/j.atherosclerosis.2019.01.023
You, L., Chen, H., Xu, L., and Li, X. (2020). Overexpression of miR-29a-3p suppresses proliferation, migration, and invasion of vascular smooth muscle cells in atherosclerosis via targeting TNFRSF1A. Biomed. Res. Int. 2020:9627974. doi: 10.1155/2020/9627974
Yurikova, O. Y., Aisina, D. E., Niyazova, R. E., Atambayeva, S. A., Labeit, S., and Ivashchenko, A. T. (2019). The interaction of miRNA-5p and miRNA-3p with the mRNAs of Orthologous Genes. Mol.Biol. 53, 692–704. doi: 10.1134/S0026898419040189
Zhang, R., Song, B., Hong, X., Shen, Z., Sui, L., and Wang, S. (2020). microRNA-9 inhibits vulnerable plaque formation and vascular remodeling via suppression of the SDC2-dependent FAK/ERK signaling pathway in mice with atherosclerosis. Front. Physiol. 11:804. doi: 10.3389/fphys.2020.00804
Keywords: gene, atherosclerosis, miRNA, mRNA, association, binding sites cluster, marker
Citation: Mukushkina D, Aisina D, Pyrkova A, Ryskulova A, Labeit S and Ivashchenko A (2020) In silico Prediction of miRNA Interactions With Candidate Atherosclerosis Gene mRNAs. Front. Genet. 11:605054. doi: 10.3389/fgene.2020.605054
Received: 11 September 2020; Accepted: 15 October 2020;
Published: 04 November 2020.
Edited by:
Yuriy L. Orlov, First Moscow State Medical University, RussiaReviewed by:
Elvira Galieva, Novosibirsk State University, RussiaVladimir N. Uversky, University of South Florida, United States
Copyright © 2020 Mukushkina, Aisina, Pyrkova, Ryskulova, Labeit and Ivashchenko. This is an open-access article distributed under the terms of the Creative Commons Attribution License (CC BY). The use, distribution or reproduction in other forums is permitted, provided the original author(s) and the copyright owner(s) are credited and that the original publication in this journal is cited, in accordance with accepted academic practice. No use, distribution or reproduction is permitted which does not comply with these terms.
*Correspondence: Anatoliy Ivashchenko, a.iavashchenko@gmail.com