- 1Biomedical Engineering Department, Stony Brook University, Stony Brook, NY, United States
- 2Laufer Center for Physical and Quantitative Biology, Stony Brook University, Stony Brook, NY, United States
- 3Stony Brook Medical Scientist Training Program, Stony Brook, NY, United States
- 4Ben May Department for Cancer Research, The University of Chicago, Chicago, IL, United States
Introduction
Cancer metastasis is still the main cause of death for most cancer types (Dillekås et al., 2019). The molecular causes of metastasis are diverse, complex, and poorly understood, including genetic and other molecular changes that transcend genetic sequence. Despite their complexity and diversity, a new emerging theme posits these changes generate cellular heterogeneity that can promote cancer metastasis (Fidler, 1978; Lee et al., 2014; Nguyen et al., 2016; Caswell and Swanton, 2017). Cellular heterogeneity can be genetic or non-genetic. Besides genetic mutations, non-genetic heterogeneity allows otherwise identical cells to develop drastically different phenotypes due to variations in molecular players that accumulate and compound effects over time.
How could cellular heterogeneity affect metastasis? A successfully metastasizing cell must cross multiple physical and molecular barriers: it must detach from the primary site, intravasate, survive the bloodstream or lymphatic vessels, extravasate, overcome immune attack, and start growing. Therefore, understanding how cellular heterogeneity affects barrier crossing is quintessential to understand its role in metastasis. Moreover, other barrier-crossing phenomena, such as drug resistance, may be unexpectedly informative, or even analogous to various steps in metastasis.
Non-genetic heterogeneity and biological noise are broad terms we consider synonymous here. They include variation in essentially any cellular property that is not genetic in origin, such as cell size, protein levels, cell function, and lifespan. A subtype of non-genetic heterogeneity relevant to this article is gene expression noise, which manifests as varying messenger RNA (mRNA) or protein levels in cells with identical genomes. While there are different uses of the term “noise” across the fields of biology, we define noise here as in physics and engineering disciplines besides biology: as a general stochastic process that does not exclude heritability. Indeed, the existence of noise with various spectra (1/f noise, colored noise, etc.) in nature implies that there can be various short- and long-term components of randomness.
Understanding the role of gene expression noise in metastasis can be based on two complementary investigative approaches, which are conceptual generalizations of forward and reverse genetics (Gurumurthy et al., 2016), respectively. Namely, forward (observational) investigation (Schuh et al., 2020; Shaffer et al., 2020) monitors and catalogs naturally occurring gene expression variability at various molecular levels in different cell types, seeking associations with cancer progression from an observational perspective. By contrast, reverse (perturbational) investigation (Kang et al., 2013; Nguyen et al., 2016) studies cancerous phenotypes arising upon artificially imposed gene expression noise, using noise-controlling genetic devices, methods or chemicals (Desai et al., 2020). Here, we discuss both perspectives regarding gene expression noise and metastasis and outline how the reverse approach may be necessary due to a natural coupling between the noise and mean of gene expression, and how it may be accomplished through synthetic biology.
Non-Genetic Heterogeneity and Threshold Crossing in Cellular and Molecular Processes
Genetic heterogeneity among cells, tissues, and organisms has long been known to play roles in generating the phenotypic diversity that life exhibits (Nichol et al., 2019). Genetic heterogeneity can cause two main types of clonal variation in a cell population (Agozzino et al., 2020). First, coding sequence mutations generate clonal populations with protein molecules of missing or altered function (e.g., a mutant enzyme loses or improves its affinity for a substrate). Second, changes in non-coding sequence or gene copy number create clones with altered mRNA or protein levels, without any changes in protein function (e.g., the amount of unaltered enzyme molecules increases or decreases). This second type of genetic heterogeneity resembles non-genetic heterogeneity or gene expression noise, especially if the latter is heritable (i.e., cellular memory is long) (Acar et al., 2005; Nevozhay et al., 2012; Shaffer et al., 2020), since they both manifest as lasting and propagating cell–cell differences in the number of protein or RNA molecules. Indeed, non-genetic variability, like genetic mutations, can play important roles in physiological processes, disease development, and evolution (Brock et al., 2009; Balázsi et al., 2011; Chattwood and Thompson, 2011; Frank and Rosner, 2012; Bai et al., 2013).
Two key characteristics of gene expression noise are its amplitude and its memory. First, the amplitude of gene expression noise [measured by the standard deviation or coefficient of variation (CV)] characterizes how far molecule numbers can deviate from the mean. Noise amplitudes can range from slight (CV < 20%) to dramatic (CV > 200%), giving rise to vastly different cellular phenotypes (Kærn et al., 2005; Raj and van Oudenaarden, 2008). Second, the cellular memory of gene expression noise characterizes the heritable aspects of random cellular differences. Cellular memory is, at least conceptually, independent from the noise amplitude (Acar et al., 2005; Nevozhay et al., 2012) and can range from <1 cell cycle time to hundreds of cell generations (Nevozhay et al., 2012), enabling a non-genetic version of clonal expansion that affects genetic evolutionary dynamics (González et al., 2015; Bódi et al., 2017; Kheir Gouda et al., 2019).
Gene expression noise can have many sources, including biochemical reactions of transcription, translation, post-translational modifications, mRNA/protein degradation, and other cellular processes (Kærn et al., 2005; Balázsi et al., 2011). These processes affect biological noise, which can be segmented as “intrinsic” or “extrinsic” (Thattai and van Oudenaarden, 2001; Elowitz et al., 2002; Swain et al., 2002). Intrinsic noise comprises variation intrinsic to gene expression due to stochastic effects from biochemical reactions involving low copy numbers of molecular species along the central dogma of molecular biology (Ozbudak et al., 2002; Swain et al., 2002; Quarton et al., 2020). This contrasts with extrinsic noise that describes variations in more global factors affecting gene expression, such as global regulators, ribosomes, polymerases, cofactor concentrations, microenvironmental variation, and activity of other cellular players extrinsic to the gene (Swain et al., 2002; Stamatakis et al., 2011). In addition, non-genetic heterogeneity (noise) in protein levels can be preexisting or induced. Unlike tightly regulated deterministic stress programs where most cells turn on the same stress response, induced heterogeneity implies random cell–cell differences emerging upon exposure to stress (Gasch et al., 2017; Farquhar et al., 2019), where some cells turn on various forms of stress response while some other cells do not.
Both intrinsic and extrinsic noise can affect developmental and evolutionary processes. Underlying such effects are threshold-crossing cellular processes that noise can promote or suppress. Noise of drug resistance protein levels can promote microbial and cancer cell populations to cross survival thresholds and thus resist high levels of drug treatment (Blake et al., 2006; Brock et al., 2009; Fraser et al., 2009; Shaffer et al., 2017). On the other hand, noise can also hinder short-term cell survival at low levels of drug treatment (Blake et al., 2006). In addition, recent evidence indicates that preexisting or stress-induced noise can play similar dichotomous roles during long-term evolution of cell populations (Fraser et al., 2009; Marusyk et al., 2012; Farquhar et al., 2019). While less established, there are suggestions that protein noise promotes oncogenesis (Brock et al., 2009), epithelial-to-mesenchymal transition (EMT), mesenchymal–epithelial transition (MET), and the initiation of metastasis (Lee et al., 2014; Nguyen et al., 2016; George et al., 2017; Jolly and Celià-Terrassa, 2019). These findings provoke the question: Could there be common principles of non-genetic heterogeneity underlying all these biologically different, but mechanistically similar processes? For example, higher, heritable noise can cause phenotypic changes in more members of a cell population by pushing and keeping cells above thresholds (Charlebois et al., 2011) that arise from multiple sources (Figure 1A), such as multistability, hypersensitivity, and irreversibility, which we discuss next.
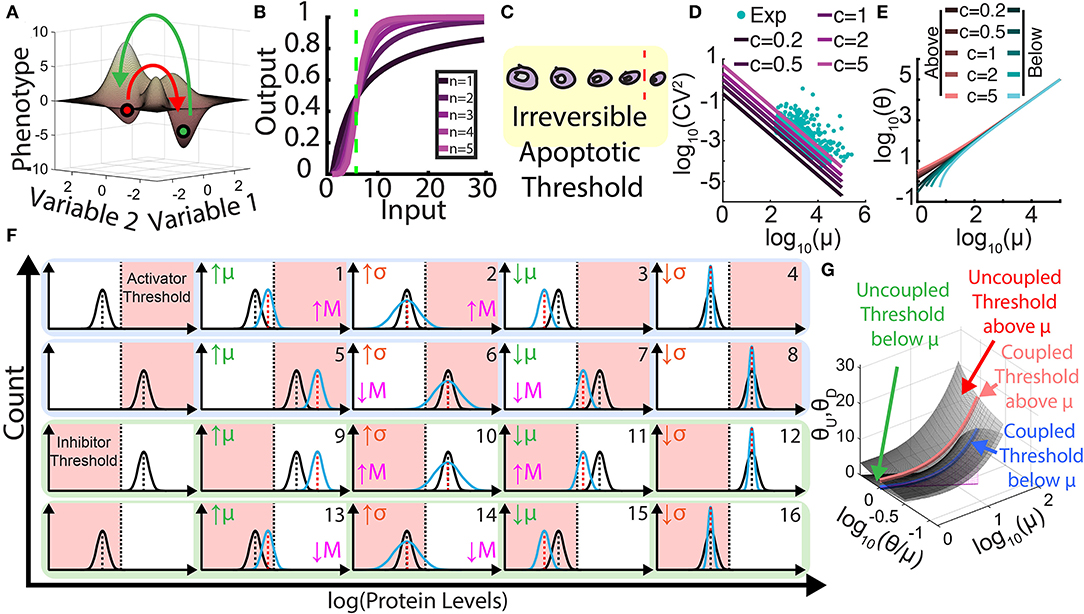
Figure 1. Role of heterogeneity and its control in threshold crossing and metastasis. (A) Multistable landscape illustrating two steady states (phenotypes). Red arrow represents going from the first phenotype to the second across the crest (threshold), and the green arrow represents the reverse transition. (B) Ultrasensitivity is the abrupt change in output for small input changes, captured by Hill functions with high Hill coefficients, n ≥ 2. Green line represents threshold where the system is most sensitive to input. (C) Irreversibility stems from a physical or chemical threshold that acts as a point of no return when cells surpass it. (D) Graph illustrating the natural tendency of gene expression noise [coefficient of variation squared (CV2)] to decrease hyperbolically as mean levels increase: CV2 = c/mean. The values of c ≠ 1 correspond to non-Poisson behaviors, such as bursting (c > 1) or noise suppression (c < 1, e.g., by negative feedback). Teal scatter dots represent rescaled yeast experimental data (Newman et al., 2006) illustrating the natural tendency of coupling between the CV and the mean for hundreds of genes. (E) Graph of threshold levels θ that can be crossed as a function of mean protein levels μ, with natural mean-noise coupling for bursting parameter c. Orange colors represent downward threshold crossing (heuristically, θ < μ – σ) when the mean is above the threshold, whereas the blue colors represent upward threshold crossing (θ > μ + σ) when the mean is below the threshold. (F) Threshold crossing effects of independently changing the mean μ or standard deviation σ for four starting positions of cell populations relative to phenotypic thresholds. The graphs with blue background (1–8) relate to metastatic activator levels, while the graphs with green background (9–16) relate to metastatic inhibitor levels. Pink areas represent metastasis (M), while white areas represent no metastasis, with pink arrows indicating increased or decreased likelihood. (G) Landscapes and curves illustrating phenotypic threshold levels that can be overcome (crossed) when the noise (CV) and mean (μ) vary in a coupled or uncoupled manner, respectively. The higher surface corresponds to upward threshold crossing [top and bottom rows of (F), 1–4 and 13–16], while the lower surface corresponds to downward threshold crossing [middle rows of (F), 5–8, 9–12 ]. Thresholds crossed by naturally coupled mean and noise values are overlaid on each surface as blue and orange lines.
Multistability is the property of a system to permit multiple potential steady states (Gardner et al., 2000; Macía et al., 2009) (two at the minimum), such as restraint vs. commitment to sugar utilization (Novick and Weiner, 1957), oocyte maturation (Xiong and Ferrell, 2003), and stem cell differentiation (Macarthur et al., 2009), which could be imagined as valleys in a landscape (Yuan et al., 2017; Kang et al., 2019; Agozzino et al., 2020) (Figure 1A). Stability of any steady state implies that effects of small, temporary external perturbations decay over time, so cells will return to their valley bottoms after weak noise or transient environmental fluctuations push them slightly away. However, sufficiently large temporary perturbations can alter protein means or noise to a degree that moves cells uniformly or individually beyond the crest (separatrix) separating two valleys, causing them to fall into the neighboring valley. One common theme underlying natural and engineered multistability is positive feedback embedded in biomolecular networks (Gardner et al., 2000; Angeli et al., 2004; Nevozhay et al., 2012), which enable cell survival and resistance to various environmental stresses (Charlebois et al., 2011; Farquhar et al., 2019) and cancer cell transitions (Lee et al., 2014). Two examples of barrier crossing while switching steady states may be EMT and MET, which are vital processes in embryonic development, tissue repair, and cancer metastasis (Zhang et al., 2014; Nieto et al., 2016; Jolly et al., 2017, 2018, 2019; Li and Balazsi, 2018; Gómez Tejeda Zañudo et al., 2019). However, it is increasingly accepted that the phenotypic spectrum between epithelial (E) and mesenchymal (M) cell states includes one or more intermediate states, so noise may induce such intermediate cross-state transitions. Moreover, noise-induced barrier crossing may lead to coexistent E, M, and hybrid E/M phenotypes, as well as emergence of stem-like circulating tumor cells (CTCs), thereby causing collective dissemination of primary tumors (Kudo-Saito et al., 2009; Jolly et al., 2015; Grigore et al., 2016; Bocci et al., 2019a,b), variation in tumor-seeding abilities (Neelakantan et al., 2017; Grosse-Wilde et al., 2018), and differences in drug sensitivity (Creighton et al., 2009; He et al., 2019; Tièche et al., 2019). Therefore, drug resistance, full and partial EMT (Aiello et al., 2018), and metastasis may all have underlying threshold-crossing mechanisms through multistability (Lee et al., 2014; Li and Balazsi, 2018).
Ultrasensitivity is the second threshold-generating property related to sharp input–output transfer functions with switch-like characteristics (Ferrell and Ha, 2014) in monostable systems. Monostable cells are those for which mathematical models predict a single steady state. From an experimental perspective, monostable cells return to their original state (protein and mRNA levels) upon a temporary perturbation, even if they are highly sensitive and thus deviate far. Ultrasensitivity leads to abrupt, large cellular responses to small, persistent input differences within a narrow input range (Figure 1B). For example, monostable cells can be ultrasensitive when their response to an internal or external factor is sigmoidal. For a system exhibiting ultrasensitivity, a threshold can be defined as the stimulus level (i.e., metabolite, protein, or cofactor concentration) at which the system is maximally sensitive (Louis and Becskei, 2002; Zhang et al., 2013).
The last mechanism of threshold generation is irreversibility, whereby external factors restrict or block the reversion of cellular processes, as in embryonic development (Caplan and Ordahl, 1978). Both monostable and multistable cells may approach physical or biochemical barriers that, once crossed, prevent reversion causing permanent outcomes. Such irreversibility can stabilize new cellular states. Typical examples are commencement of DNA synthesis, cell lysis, and apoptosis, which, after a certain progression, cannot revert (Figure 1C). Likewise, when a tumor cell enters the bloodstream and travels away, it is very unlikely to return to its original site (Scott et al., 2013), and even if it does, it will be already altered due to its time in a different environment.
Each of these three threshold-generating mechanisms can produce biological consequences in populations of cells ranging from cell division and neuron depolarization to apoptosis when a certain threshold is exceeded (Mateo et al., 2011; Xie et al., 2011; Sato et al., 2013). A common conceptual way to connect cell population phenotypes, such as drug resistance, oncogenesis, and metastasis to single-cell behaviors is through noise-modulated threshold crossing. However, altering the mean can also move cell populations closer to or farther from thresholds without any change in the noise. Therefore, investigating the role of noise in such processes requires fixing the mean. Yet, in natural systems, the mean and noise of protein levels have a tendency to be coupled (Newman et al., 2006; Dar et al., 2016), where higher means often associate with lower noise, along a hyperbolic (CV2 = c/mean) interdependence. Typically, this relationship holds for various bursting regimes (c > 1 in Figure 1D). From an observational standpoint, naturally occurring mutations or other changes make it rarely possible (Dar et al., 2014; You et al., 2019) to parse out specifically how the noise of a single protein affects threshold crossing and phenotypes independently of the mean. For example, Figure 1E shows the threshold levels that a given protein can naturally overcome based on the experimentally demonstrated inverse relationship (Newman et al., 2006; Dar et al., 2016) between its expression mean and noise (Figure 1D). Overall, due to their natural coupling tendency, both the mean and noise will change and affect threshold crossing. To decipher their individual impact on biological phenotypes, we need engineering approaches to independently control the protein noise and the mean because observing natural decoupling scenarios is far from trivial. In the next section, we explore ways of decoupling means and noise, with implications on drug resistance and metastasis.
Controlling Non-Genetic Heterogeneity of Metastasis Through Synthetic Biological Gene Circuits
To confirm observational suggestions on the role of non-genetic heterogeneity in disease development, stress survival, and metastasis, one must control protein noise independently of the mean in living organisms. Engineering approaches from the field of synthetic biology (Elowitz and Leibler, 2000; Gardner et al., 2000) enabled the inception of such control (Blake et al., 2006; Fraser et al., 2009), followed by identification of many methods that reduce or amplify gene expression noise (Maamar et al., 2007; Cagatay et al., 2009; Nevozhay et al., 2013; Shimoga et al., 2013; Farquhar et al., 2019; Guinn and Balázsi, 2019). In natural systems, a change in a protein's mean will often change the noise since the two parameters tend to be coupled (Figure 1D). Therefore, biological threshold crossing typically does not utilize the mean and noise as two fully independent degrees of freedom (Figure 1E). As opposed to natural mechanisms, synthetic biological systems can allow independent changes in the mean and noise such that they are no longer coupled. Synthetic gene circuits thus provide an increasing number of ways to allow noise-mean decoupling (Aranda-Díaz et al., 2017; Farquhar et al., 2019) in studies of noise-modulated phenotypic transitions.
Two main approaches have accomplished decoupling the gene expression mean and noise from one another in synthetic gene circuits: (i) different gene circuits to express the same gene with different noise-vs.-mean dependencies (Blake et al., 2006; Süel et al., 2006; Kim and Sauro, 2010; Farquhar et al., 2019) and (ii) combinatorial induction of cascaded modules within the same gene circuit (Aranda-Díaz et al., 2017). Decoupling the noise and mean from one another adds a new degree of freedom to tune threshold crossing and reveal individual contributions of mean and noise on cellular processes, such as metastasis.
As an illustrative classification inspired by studies examining how mean and noise affect drug resistance (Blake et al., 2006; Farquhar et al., 2019), we consider a threshold for a metastasis activator (or a threshold for a metastasis inhibitor). Bistability, ultrasensitivity, and irreversibility underlying such thresholds are frequent themes in metastasis and EMT (Lee et al., 2014; Zhang et al., 2014; Jolly et al., 2015). Based on the position of the threshold (above or below) relative to the mean, and the ability to tune the mean and variance independently up and down, there are 16 possible phenotypic scenarios (Figure 1F). To start, assume metastasis activator levels are below a phenotypic threshold when the cells are not metastatic (Figure 1F, 1–4). In such a scenario, both the mean and variance of activator levels can be tuned up or down. Two of these changes (tuning mean or variance up) should promote metastasis by enhanced threshold crossing, while the two opposite changes (tuning mean or variance down) should hinder metastasis. Alternatively, for a metastatic cell population with hyperthreshold activator distribution (Figure 1F, 5–8), tuning the mean down or variance up should hinder metastasis, while the opposite changes should promote metastasis. Overall, for underthreshold populations, elevated activator mean and variance consistently aid overcoming the threshold. In contrast, for hyperthreshold populations, elevated activator variance still aids, but elevated mean hinders threshold crossing. Analogously, non-metastatic and metastatic cell populations could also have metastasis inhibitor levels above or below a phenotypic threshold, respectively (Figure 1F, 9–16). Tuning the variance and mean of a metastasis suppressor up or down will affect threshold crossing according to the above principles but with opposite phenotypic effects. Similar reasoning can predict the effect of simultaneous changes in the mean and the noise, although the scenarios can be numerous and complicated.
Protein mean- and noise-dependent crossings of various thresholds in synthetic and natural scenarios can also be explored visually as landscapes (Figure 1G). Synthetic biological control can explore surfaces with two degrees of freedom, whereas natural coupling restricts movement to a single degree of freedom along paths on such surfaces. These landscapes and curves illustrate what phenotypic threshold levels can be crossed when the noise and mean are coupled or uncoupled, respectively. In the future, it will be important to examine similar but multimolecular threshold crossings in higher dimensions, or the joint effects of the mean, the noise, as well as of the higher moments (i.e., skewness, kurtosis, etc.). Moreover, specific fitness landscapes (Nevozhay et al., 2012; González et al., 2015) will need to replace threshold approximations in many realistic scenarios. Synthetic biological tools will be indispensable for addressing these future questions.
Experimental evidence for how gene expression mean and noise independently affect metastasis threshold crossing is relatively lacking, but synthetic biology is already shedding light on drug resistance (Farquhar et al., 2019), which may give insights to the role of noise in metastasis as an evolutionary process. According to the threshold-crossing principles, high noise aids drug resistance evolution when stress is high but hampers survival when stress is low, mimicking the effects of noise on short-term survival (Blake et al., 2006; Fraser et al., 2009; Farquhar et al., 2019). Analogously, high noise should facilitate metastasis initiation for pre-metastatic cells before dissemination. In contrast, high noise may hinder the rate of metastasis for cells that have already acquired invasive characteristics. These findings relate to where cells sit (below or above) relative to thresholds at which specific phenotypes emerge. We anticipate that utilizing noise amplifying or reducing gene circuits (Becskei et al., 2001; Hooshangi et al., 2005; Weinberger et al., 2005; Tan et al., 2009; Diao et al., 2016; Farquhar et al., 2019) will give similar insights into processes underlying metastasis. Using the growing repertoire of noise-controlling synthetic biology tools and chemicals will certainly uncover unknown roles of gene expression in processes of full or partial EMT (Aiello et al., 2018), metastasis, and oncogenesis.
Author Contributions
MG, YW, and GB conceived and wrote the manuscript. SL surveyed the literature and added the references. DY and MR contributed some critical concepts and feedback on the manuscript. All authors contributed to the article and approved the submitted version.
Funding
This work was supported by the National Institutes of Health, NIGMS MIRA Program R35 GM122561 (to GB), R01 GM121735-01 (to MR), the Rustandy fund for Innovative Cancer Research (to MR), a National Defense Science and Engineering Graduate (NDSEG) Fellowship (to MG), and by the Laufer Center for Physical and Quantitative Biology (to GB).
Conflict of Interest
The authors declare that the research was conducted in the absence of any commercial or financial relationships that could be construed as a potential conflict of interest.
References
Acar, M., Becskei, A., and van Oudenaarden, A. (2005). Enhancement of cellular memory by reducing stochastic transitions. Nature 435, 228–232. doi: 10.1038/nature03524
Agozzino, L., Balázsi, G., Wang, J., and Dill, K. A. (2020). How do cells adapt? Stories told in landscapes. Ann. Rev. Chem. Biomol. Eng. 11, 155–182. doi: 10.1146/annurev-chembioeng-011720-103410
Aiello, N. M., Maddipati, R., Norgard, R. J., Balli, D., Li, J., Yuan, S., et al. (2018). EMT subtype influences epithelial plasticity and mode of cell migration. Dev. Cell 45, 681–695.e684. doi: 10.1016/j.devcel.2018.05.027
Angeli, D., Ferrell, J. E., Sontag, E. D., Angeli, D., Ferrell, J. E., and Sontag, E. D. Jr. (2004). Detection of multistability, bifurcations, and hysteresis in a large class of biological positive-feedback systems. Proc. Natl. Acad. Sci. U.S.A. 101, 1822–1827. doi: 10.1073/pnas.0308265100
Aranda-Díaz, A., Mace, K., Zuleta, I., Harrigan, P., and El-Samad, H. (2017). Robust synthetic circuits for two-dimensional control of gene expression in yeast. ACS Synth. Biol. 6, 545–554. doi: 10.1021/acssynbio.6b00251
Bai, F., Che, Y.-S., Kami-ike, N., Ma, Q., Minamino, T., Sowa, Y., et al. (2013). Populational heterogeneity vs. temporal fluctuation in Escherichia coli flagellar motor switching. Biophys J. 105, 2123–2129. doi: 10.1016/j.bpj.2013.09.043
Balázsi, G., van Oudenaarden, A., and Collins, J. J. (2011). Cellular decision making and biological noise: from microbes to mammals. Cell 144, 910–925. doi: 10.1016/j.cell.2011.01.030
Becskei, A., Séraphin, B., and Serrano, L. (2001). Positive feedback in eukaryotic gene networks: cell differentiation by graded to binary response conversion. EMBO J. 20, 2528–2535. doi: 10.1093/emboj/20.10.2528
Blake, W. J., Balázsi, G., Kohanski, M. A., Isaacs, F. J., Murphy, K. F., Kuang, Y., Blake, W. J., et al. (2006). Phenotypic consequences of promoter-mediated transcriptional noise. Mol. Cell. 24, 853–865. doi: 10.1016/j.molcel.2006.11.003
Bocci, F., Gearhart-Serna, L., Boareto, M., Ribeiro, M., Ben-Jacob, E., Devi, G. R., et al. (2019a). Toward understanding cancer stem cell heterogeneity in the tumor microenvironment. Proc. Natl. Acad. Sci. U.S.A. 116, 148–157. doi: 10.1073/pnas.1815345116
Bocci, F., Levine, H., Onuchic, J., and Jolly, M. K. (2019b). Deciphering the dynamics of epithelial-mesenchymal transition and cancer stem cells in tumor progression. Curr. Stem. Cell Rep. 5, 1–11. doi: 10.1007/s40778-019-0150-3
Bódi, Z., Farkas, Z., Nevozhay, D., Kalapis, D., Lázár, V., Csörgo, B., et al. (2017). Phenotypic heterogeneity promotes adaptive evolution. PLoS Biol. 15:e2000644. doi: 10.1371/journal.pbio.2000644
Brock, A., Chang, H., and Huang, S. (2009). Non-genetic heterogeneity–a mutation-independent driving force for the somatic evolution of tumours. Nat. Rev. Genet. 10, 336–342. doi: 10.1038/nrg2556
Cagatay, T., Turcotte, M., Elowitz, M. B., Garcia-Ojalvo, J., and Süel, G. M. (2009). Architecture-dependent noise discriminates functionally analogous differentiation circuits. Cell 139, 512–522. doi: 10.1016/j.cell.2009.07.046
Caplan, A. I., and Ordahl, C. P. (1978). Irreversible gene repression model for control of development. Science 201:120. doi: 10.1126/science.351805
Caswell, D. R., and Swanton, C. (2017). The role of tumour heterogeneity and clonal cooperativity in metastasis, immune evasion and clinical outcome. BMC Med. 15:133. doi: 10.1186/s12916-017-0900-y
Charlebois, D. A., Abdennur, N., and Kaern, M. (2011). Gene expression noise facilitates adaptation and drug resistance independently of mutation. Phys. Rev. Lett. 107:218101. doi: 10.1103/PhysRevLett.107.218101
Chattwood, A., and Thompson, C. R. (2011). Non-genetic heterogeneity and cell fate choice in Dictyostelium discoideum. Dev. Growth Differ. 53, 558–566. doi: 10.1111/j.1440-169X.2011.01270.x
Creighton, C. J., Li, X., Landis, M., Dixon, J. M., Neumeister, V. M., Sjolund, A., et al. (2009). Residual breast cancers after conventional therapy display mesenchymal as well as tumor-initiating features. Proc. Natl. Acad. Sci. U.S.A. 106, 13820–13825. doi: 10.1073/pnas.0905718106
Dar, R. D., Hosmane, N. N., Arkin, M. R., Siliciano, R. F., and Weinberger, L. S. (2014). Screening for noise in gene expression identifies drug synergies. Science 344, 1392–1396. doi: 10.1126/science.1250220
Dar, R. D., Shaffer, S. M., Singh, A., Razooky, B. S., Simpson, M. L., Raj, A., et al. (2016). Transcriptional bursting explains the noise-versus-mean relationship in mRNA and protein levels. PLoS ONE. 11:e0158298. doi: 10.1371/journal.pone.0158298
Desai, R. V., Hansen, M. M. K., Martin, B., Yu, C., Ding, S., Thomson, M., et al. (2020). Discovery of a cellular mechanism regulating transcriptional noise. bioRxiv 2020.2006.2029.128439v128431.
Diao, J., Charlebois, D. A., Nevozhay, D., Bódi, Z., Pál, C., and Balázsi, G. (2016). Efflux pump control alters synthetic gene circuit function. ACS Synth. Biol. 5, 619–631. doi: 10.1021/acssynbio.5b00154
Dillekås, H., Rogers, M. S., and Straume, O. (2019). Are 90% of deaths from cancer caused by metastases? Cancer Med. 8, 5574–5576 doi: 10.1002/cam4.2474
Elowitz, M. B., and Leibler, S. (2000). A synthetic oscillatory network of transcriptional regulators. Nature 403, 335–338. doi: 10.1038/35002125
Elowitz, M. B., Levine, A. J., Siggia, E. D., and Swain, P. S. (2002). Stochastic gene expression in a single cell. Science 297:1183. doi: 10.1126/science.1070919
Farquhar, K. S., Charlebois, D. A., Szenk, M., Cohen, J., Nevozhay, D., and Balázsi, G. (2019). Role of network-mediated stochasticity in mammalian drug resistance. Nat. Commun. 10:2766. doi: 10.1038/s41467-019-10330-w
Ferrell, J. E., and Ha, S. H. (2014). Ultrasensitivity part I: michaelian responses and zero-order ultrasensitivity. Trends Biochem. Sci. 39, 496–503. doi: 10.1016/j.tibs.2014.08.003
Fidler, I. J. (1978). Tumor heterogeneity and the biology of cancer invasion and metastasis. Cancer Res. 38, 2651–2660.
Frank, S. A., and Rosner, M. R. (2012). Nonheritable cellular variability accelerates the evolutionary processes of cancer. PLoS Biol. 10:e1001296. doi: 10.1371/journal.pbio.1001296
Fraser, D., Kaern, M., Fraser, D., and Kærn, M. (2009). A chance at survival: gene expression noise and phenotypic diversification strategies. Mol. Microbiol. 71, 1333–1340. doi: 10.1111/j.1365-2958.2009.06605.x
Gardner, T. S., Cantor, C. R., and Collins, J. J. (2000). Construction of a genetic toggle switch in Escherichia coli. Nature 403, 339–342. doi: 10.1038/35002131
Gasch, A. P., Yu, F. B., Hose, J., Escalante, L. E., Place, M., Bacher, R., et al. (2017). Single-cell RNA sequencing reveals intrinsic and extrinsic regulatory heterogeneity in yeast responding to stress. PLoS Biol. 15:e2004050. doi: 10.1371/journal.pbio.2004050
George, J. T., Jolly, M. K., Xu, S., Somarelli, J. A., and Levine, H. (2017). Survival outcomes in cancer patients predicted by a partial emt gene expression scoring metric. Cancer Res. 77, 6415–6428. doi: 10.1158/0008-5472.CAN-16-3521
Gómez Tejeda Zañudo, J., Guinn, M. T., Farquhar, K., Szenk, M., Steinway, S. N., Balázsi, G., et al. (2019). Towards control of cellular decision-making networks in the epithelial-to-mesenchymal transition. Phys. Biol. 16, 031002–031002. doi: 10.1088/1478-3975/aaffa1
González, C., Ray, J. C., Manhart, M., Adams, R. M., Nevozhay, D., Morozov, A. V., et al. (2015). Stress-response balance drives the evolution of a network module and its host genome. Mol. Syst. Biol. 11:827. doi: 10.15252/msb.20156185
Grigore, A. D., Jolly, M. K., Jia, D., Farach-Carson, M. C., and Levine, H. (2016). Tumor budding: the name is EMT. Partial EMT. J. Clin. Med. 5:51. doi: 10.3390/jcm5050051
Grosse-Wilde, A., Kuestner, R. E., Skelton, S. M., MacIntosh, E., d'Hérouël, A. F., Ertaylan, G., et al. (2018). Loss of inter-cellular cooperation by complete epithelial-mesenchymal transition supports favorable outcomes in basal breast cancer patients. Oncotarget 9, 20018–20033. doi: 10.18632/oncotarget.25034
Guinn, M. T., and Balázsi, G. (2019). Noise-reducing optogenetic negative-feedback gene circuits in human cells. Nucleic Acids Res. 47, 7703–7714. doi: 10.1093/nar/gkz556
Gurumurthy, C. B., Grati, M., Ohtsuka, M., Schilit, S. L., Quadros, R. M., and Liu, X. Z. (2016). CRISPR: a versatile tool for both forward and reverse genetics research. Hum. Genet. 135, 971–976. doi: 10.1007/s00439-016-1704-4
He, J., Lee, H. J., Saha, S., Ruan, D., Guo, H., Chan, C. H., He, J., et al. (2019). Inhibition of USP2 eliminates cancer stem cells and enhances TNBC responsiveness to chemotherapy. Cell Death Dis. 10:285. doi: 10.1038/s41419-019-1512-6
Hooshangi, S., Thiberge, S., and Weiss, R. (2005). Ultrasensitivity and noise propagation in a synthetic transcriptional cascade. Proc. Natl. Acad. Sci. U.S.A. 102:3581. doi: 10.1073/pnas.0408507102
Jolly, M. K., Boareto, M., Huang, B., Jia, D., Lu, M., Ben-Jacob, E., Jolly, M. K., et al. (2015). Implications of the hybrid epithelial/mesenchymal phenotype in metastasis. Front. Oncol. 5:155. doi: 10.3389/fonc.2015.00155
Jolly, M. K., and Celià-Terrassa, T. (2019). Dynamics of phenotypic heterogeneity associated with EMT and stemness during cancer progression. J. Clin. Med. 8:1542. doi: 10.3390/jcm8101542
Jolly, M. K., Mani, S. A., and Levine, H. (2018). Hybrid epithelial/mesenchymal phenotype(s): the ‘fittest’ for metastasis? Biochim. Biophys. Acta Rev. Cancer 1870, 151–157. doi: 10.1016/j.bbcan.2018.07.001
Jolly, M. K., Somarelli, J. A., Sheth, M., Biddle, A., Tripathi, S. C., Armstrong, A. J., et al. (2019). Hybrid epithelial/mesenchymal phenotypes promote metastasis and therapy resistance across carcinomas. Pharmacol. Ther. 194, 161–184. doi: 10.1016/j.pharmthera.2018.09.007
Jolly, M. K., Ware, K. E., Gilja, S., Somarelli, J. A., and Levine, H. (2017). EMT and MET: necessary or permissive for metastasis? Mol. Oncol. 11, 755–769. doi: 10.1002/1878-0261.12083
Kærn, M., Elston, T. C., Blake, W. J., and Collins, J. J. (2005). Stochasticity in gene expression: from theories to phenotypes. Nat. Rev. Genet. 6, 451–464. doi: 10.1038/nrg1615
Kang, T., White, J. T., Xie, Z., Benenson, Y., Sontag, E., and Bleris, L. (2013). Reverse engineering validation using a benchmark synthetic gene circuit in human cells. ACS Synth. Biol. 2, 255–262. doi: 10.1021/sb300093y
Kang, X., Wang, J., and Li, C. (2019). Exposing the underlying relationship of cancer metastasis to metabolism and epithelial-mesenchymal transitions. iScience 21, 754–772. doi: 10.1016/j.isci.2019.10.060
Kheir Gouda, M., Manhart, M., and Balázsi, G. (2019). Evolutionary regain of lost gene circuit function. Proc. Natl. Acad. Sci. U.S.A. 116, 25162–25171. doi: 10.1073/pnas.1912257116
Kim, K. H., and Sauro, H. M. (2010). Fan-out in gene regulatory networks. J. Biol. Eng. 4:16. doi: 10.1186/1754-1611-4-16
Kudo-Saito, C., Shirako, H., Takeuchi, T., and Kawakami, Y. (2009). Cancer metastasis is accelerated through immunosuppression during Snail-induced EMT of cancer cells. Cancer Cell. 15, 195–206. doi: 10.1016/j.ccr.2009.01.023
Lee, J., Lee, J., Farquhar, K. S., Yun, J., Frankenberger, C. A., Bevilacqua, E., et al. (2014). Network of mutually repressive metastasis regulators can promote cell heterogeneity and metastatic transitions. Proc. Natl. Acad. Sci. U.S.A. 111, E364–E373. doi: 10.1073/pnas.1304840111
Li, C., and Balazsi, G. (2018). A landscape view on the interplay between EMT and cancer metastasis. NPJ Syst. Biol Appl. 4:34. doi: 10.1038/s41540-018-0068-x
Louis, M., and Becskei, A. (2002). Binary and graded responses in gene networks. Sci. STKE 2002:pe33. doi: 10.1126/stke.2002.143.pe33
Maamar, H., Raj, A., and Dubnau, D. (2007). Noise in gene expression determines cell fate in bacillus subtilis. Science 317:526. doi: 10.1126/science.1140818
Macarthur, B. D., Ma'ayan, A., and Lemischka, I. R. (2009). Systems biology of stem cell fate and cellular reprogramming. Nat. Rev. Mol. Cell Biol. 10, 672–681. doi: 10.1038/nrm2766
Macía, J., Widder, S., and Solé, R. (2009). Why are cellular switches Boolean? General conditions for multistable genetic circuits. J. Theor. Biol. 261, 126–135. doi: 10.1016/j.jtbi.2009.07.019
Marusyk, A., Almendro, V., and Polyak, K. (2012). Intra-tumour heterogeneity: a looking glass for cancer? Nat. Rev. Cancer 12, 323–334. doi: 10.1038/nrc3261
Mateo, C., Avermann, M., Gentet, L. J., Zhang, F., Deisseroth, K., and Petersen, C. C. (2011). In vivo optogenetic stimulation of neocortical excitatory neurons drives brain-state-dependent inhibition. Curr. Biol. 21, 1593–1602. doi: 10.1016/j.cub.2011.08.028
Neelakantan, D., Zhou, H., Oliphant, M. U. J., Zhang, X, Simon, L. M., and Henke, D. M. (2017). EMT cells increase breast cancer metastasis via paracrine GLI activation in neighbouring tumour cells. Nat. Commun. 8:15773. doi: 10.1038/ncomms15773
Nevozhay, D., Adams, R. M., Van Itallie, E., Bennett, M. R., and Balázsi, G. (2012). Mapping the environmental fitness landscape of a synthetic gene circuit. PLoS Comput. Biol. 8:e1002480. doi: 10.1371/journal.pcbi.1002480
Nevozhay, D., Zal, T., and Balázsi, G. (2013). Transferring a synthetic gene circuit from yeast to mammalian cells. Nat. Commun. 4:1451. doi: 10.1038/ncomms2471
Newman, J. R., Ghaemmaghami, S., Ihmels, S., Breslow, D. K., Noble, M., et al. (2006). Single-cell proteomic analysis of S. cerevisiae reveals the architecture of biological noise. Nature 441, 840–846. doi: 10.1038/nature04785
Nguyen, A., Yoshida, M., Goodarzi, H., and Tavazoie, S. F. (2016). Highly variable cancer subpopulations that exhibit enhanced transcriptome variability and metastatic fitness. Nat. Commun. 7:11246. doi: 10.1038/ncomms11246
Nichol, D., Robertson-Tessi, M., Anderson, A. R. A., and Jeavons, P. (2019). Model genotype-phenotype mappings and the algorithmic structure of evolution. J. R. Soc. Interf. 16:20190332. doi: 10.1098/rsif.2019.0332
Nieto, M. A., Huang, R. Y., Jackson, R. A., and Thiery, J. P. (2016). EMT: 2016. Cell. 166, 21–45 doi: 10.1016/j.cell.2016.06.028
Novick, A., and Weiner, M. (1957). Enzyme induction as an all-or-none phenomenon. Proc. Natl. Acad. Sci. U.S.A. 43, 553–566. doi: 10.1073/pnas.43.7.553
Ozbudak, E. M., Thattai, M., Kurtser, I., Grossman, A. D., and van Oudenaarden, A. (2002). Regulation of noise in the expression of a single gene. Nat. Genet. 31, 69–73. doi: 10.1038/ng869
Quarton, T., Kang, T., Papakis, V., Nguyen, K., Nowak, C., Li, Y., et al. (2020). Uncoupling gene expression noise along the central dogma using genome engineered human cell lines. Nucleic Acids Res. 48, 9406–9413. doi: 10.1093/nar/gkaa668
Raj, A., and van Oudenaarden, A. (2008). Nature, nurture, or chance: stochastic gene expression and its consequences. Cell 135, 216–226. doi: 10.1016/j.cell.2008.09.050
Sato, Y., Yasuhara, K., Kikuchi, J., and Sato, T. N. (2013). Synthetic cell division system: controlling equal vs. unequal divisions by design. Sci Rep. 3:3475. doi: 10.1038/srep03475
Schuh, L., Saint-Antoine, M., Sanford, E. M., Emert, B. L., Singh, A., Marr, C., et al. (2020). Gene networks with transcriptional bursting recapitulate rare transient coordinated high expression states in cancer. Cell Syst. 10, 363–378 e312. doi: 10.1016/j.cels.2020.03.004
Scott, J. G., Basanta, D., Anderson, A. R., and Gerlee, P. (2013). A mathematical model of tumour self-seeding reveals secondary metastatic deposits as drivers of primary tumour growth. J. R. Soc. Interface. 10:20130011. doi: 10.1098/rsif.2013.0011
Shaffer, S. M., Dunagin, M. C., Torborg, S. R., Torre, E. A., Emert, B., Krepler, C., et al. (2017). Rare cell variability and drug-induced reprogramming as a mode of cancer drug resistance. Nature 546, 431–435. doi: 10.1038/nature22794
Shaffer, S. M., Emert, B. L., Reyes Hueros, R. A., Cote, C., Harmange, G., Schaff, D. L., et al. (2020). Memory sequencing reveals heritable single-cell gene expression programs associated with distinct cellular behaviors. Cell 182, 947–959 e917. doi: 10.1016/j.cell.2020.07.003
Shimoga, V., White, J. T., Li, Y., Sontag, E., and Bleris, L. (2013). Synthetic mammalian transgene negative autoregulation. Mol. Syst. Biol. 9, 670–670. doi: 10.1038/msb.2013.27
Stamatakis, M., Adams, R. M., and Balázsi, G. (2011). A common repressor pool results in indeterminacy of extrinsic noise. Chaos 21:047523. doi: 10.1063/1.3658618
Süel, G. M., Garcia-Ojalvo, J., Liberman, L. M., and Elowitz, M. B. (2006). An excitable gene regulatory circuit induces transient cellular differentiation. Nature 440, 545–550. doi: 10.1038/nature04588
Swain, P. S., Elowitz, M. B., and Siggia, E. D. (2002). Intrinsic and extrinsic contributions to stochasticity in gene expression. Proc. Natl. Acad. Sci. U.S.A. 99:12795. doi: 10.1073/pnas.162041399
Tan, C., Marguet, P., and You, L. (2009). Emergent bistability by a growth-modulating positive feedback circuit. Nat. Chem. Biol. 5, 842–848. doi: 10.1038/nchembio.218
Thattai, M., and van Oudenaarden, A. (2001). Intrinsic noise in gene regulatory networks. Proc. Natl. Acad. Sci. U.S.A. 98:8614. doi: 10.1073/pnas.151588598
Tièche, C. C., Gao, Y., Bührer, E. D., Hobi, N., Berezowska, S. A., Wyler, K., et al. (2019). Tumor initiation capacity and therapy resistance are differential features of EMT-related subpopulations in the NSCLC cell line A549. Neoplasia. 21, 185–196. doi: 10.1016/j.neo.2018.09.008
Weinberger, L. S., Burnett, J. C., Toettcher, J. E., Arkin, A. P., and Schaffer, D. V. (2005). Stochastic gene expression in a lentiviral positive-feedback loop: hiv-1 tat fluctuations drive phenotypic diversity. Cell 122, 169–182. doi: 10.1016/j.cell.2005.06.006
Xie, Z., Wroblewska, L., Prochazka, L., Weiss, R., and Benenson, Y. (2011). Multi-input RNAi-based logic circuit for identification of specific cancer cells. Science 333, 1307–1311. doi: 10.1126/science.1205527
Xiong, W., and Ferrell, J. E. (2003). A positive-feedback-based bistable ‘memory module’ that governs a cell fate decision. Nature 426, 460–465. doi: 10.1038/nature02089
You, S. T., Jhou, Y. T., Kao, C. F., and Leu, J. Y. (2019). Experimental evolution reveals a general role for the methyltransferase Hmt1 in noise buffering. PLoS Biol. 17:e3000433. doi: 10.1371/journal.pbio.3000433
Yuan, R., Zhu, X., Wang, G., Li, S., Ao, P., Yuan, R., et al. (2017). Cancer as robust intrinsic state shaped by evolution: a key issues review. Rep. Prog. Phys. 80:042701. doi: 10.1088/1361-6633/aa538e
Zhang, J., Tian, X. J., Zhang, H., Teng, Y., Li, R., Bai, F., et al. (2014). TGF-beta-induced epithelial-to-mesenchymal transition proceeds through stepwise activation of multiple feedback loops. Sci Signal. 7:ra91. doi: 10.1126/scisignal.2005304
Keywords: synthetic biology, non-genetic heterogeneity, noise, control, metastasis, cancer, drug resistance
Citation: Guinn MT, Wan Y, Levovitz S, Yang D, Rosner MR and Balázsi G (2020) Observation and Control of Gene Expression Noise: Barrier Crossing Analogies Between Drug Resistance and Metastasis. Front. Genet. 11:586726. doi: 10.3389/fgene.2020.586726
Received: 23 July 2020; Accepted: 29 September 2020;
Published: 30 October 2020.
Edited by:
Mohit Kumar Jolly, Indian Institute of Science (IISc), IndiaReviewed by:
Shensi Shen, Institut Gustave Roussy, FranceJean Clairambault, Institut National de Recherche en Informatique et en Automatique (INRIA), France
Copyright © 2020 Guinn, Wan, Levovitz, Yang, Rosner and Balázsi. This is an open-access article distributed under the terms of the Creative Commons Attribution License (CC BY). The use, distribution or reproduction in other forums is permitted, provided the original author(s) and the copyright owner(s) are credited and that the original publication in this journal is cited, in accordance with accepted academic practice. No use, distribution or reproduction is permitted which does not comply with these terms.
*Correspondence: Michael Tyler Guinn, mtguinn@gmail.com; orcid.org/0000-0002-7891-8765; Gábor Balázsi, gabor.balazsi@stonybrook.edu; orcid.org/0000-0002-6865-5818