- 1Department of Genetics and Plant Breeding, College of Agriculture – Mandya, University of Agricultural Sciences, Bengaluru, Bengaluru, India
- 2Corteva Agriscience Pvt. Ltd., Kallinayakanahalli, India
Among various foliar diseases affecting maize yields worldwide, northern corn leaf blight (NCLB) is economically important. The genetics of resistance was worked out to be quantitative in nature thereby suggesting the need for the detection of quantitative trait loci (QTL) to initiate effective marker-aided breeding strategies. From the cross CML153 (susceptible) × SKV50 (resistant), 344 F2:3 progenies were derived and screened for their reaction to NCLB during the rainy season of 2013 and 2014. The identification of QTL affecting resistance to NCLB was carried out using the genetic linkage map constructed with 194 polymorphic SNPs and the disease data recorded on F2:3 progeny families. Three QTL for NCLB resistance were detected on chromosomes 2, 5, and 8 with the QTL qNCLB-8-2 explaining the highest phenotypic variation of 16.34% followed by qNCLB-5 with 10.24%. QTL for resistance to sorghum downy mildew (SDM) and southern corn rust (SCR) were also identified from one season phenotypic data, and the co-location of QTL for resistance to three foliar diseases was investigated. QTL present in chromosome bins 8.03, 5.03, 5.04, and 3.04 for resistance to NCLB, SDM, and SCR were co-localized, indicating their usefulness for the pyramiding of quantitative resistance to multiple foliar pathogens. Marker-assisted selection was practiced in the crosses CM212 × SKV50, HKI162 × SKV50, and CML153 × SKV50 employing markers linked to major QTL on chromosomes 8, 2, and 10 for NCLB, SDM, and SCR resistance, respectively. The populations were advanced to F6 stage to derive multiple disease-resistant inbred lines. Out of the 125 lines developed, 77 lines were tested for their combining ability and 39 inbred lines exhibited high general combining ability with an acceptable level of resistance to major diseases.
Introduction
Maize (Zea mays L.) is a widely cultivated food crop worldwide along with rice and wheat. It also serves as a livestock feed and industrial raw material (Troyer, 2006). Maize was diversified first in the highlands of Mexico where it was domesticated from the wild progenitor teosinte, Z. mays spp. parviglumis (Matsuoka et al., 2002). Globally, maize (Z. mays L.) is cultivated in a wide variety of environments with major cultivation in the warmer parts of temperate regions and in humid–subtropical climate (Dowswell et al., 1996).
Among various biotic constraints, foliar diseases are very important yield-limiting factors worldwide and the prevalence of these diseases varies depending on the region or season (Smith, 1999). About 61 diseases have been recorded on maize in India causing yield losses (Payak et al., 1973; Payak and Sharma, 1985). Northern corn leaf blight incited by Exserohilum turcicum (Pass) Leonard and Suggs (Teliomorph = Setosphaeria turcica (Luttrell), sorghum downy mildew caused by Peronosclerospora sorghi (Weston and Uppal), and southern corn rust caused by Puccinia polysora (Underwood) are considered as the most persistent and destructive diseases of field maize (Pratt and Gordon, 2006).
Northern corn leaf blight is prevalent throughout the world and is known to cause more than 50% yield losses (Raymundo and Hooker, 1981; Perkins and Pederson, 1987). Disease is known to appear in regions whenever moderate temperatures and high humidity prevail (Carson, 1999; Smith, 1999). Northern corn leaf blight disease was first reported in India by Butler (1907), and it causes 16–98% reduction in grain and fodder yield (Kachapur and Hegde, 1988; Harlapur et al., 2000).
Among several management options available, cultivation of resistant cultivars is the most practical and cost-effective approach in the management of diseases (Fehr, 1987; Ward et al., 1997). To breed a genotype with a high level of resistance, the inheritance pattern of resistant reaction in the material being handled is a prerequisite. Earlier studies on the genetics of resistance to northern corn leaf blight (Jenkins et al., 1952; Hughes and Hooker, 1971; Hettiarachchi et al., 2009; Chaudhary and Mani, 2010; Ranganatha et al., 2017) suggest that resistance is complex and polygenic in nature. Selection for resistance to foliar diseases is effectively practiced through conventional breeding, where susceptible genotypes under disease pressure can be eliminated before harvest (Ali and Yan, 2012). However, conventional breeding is time-consuming and less feasible due to the complex nature of resistance reaction. This favored the development of molecular tools to assist conventional breeding efforts to breed resistant cultivars. Identification of quantitative trait loci (QTL) is one such tool to help in marker-assisted selection (MAS) of resistant genotypes. In maize, a large amount of valuable information exists with reference to QTL conditioning resistance to foliar diseases like northern corn leaf blight (Welz et al., 1999; Welz and Geiger, 2000; Brown et al., 2001; Ping et al., 2007; Asea et al., 2009; Balint-Kurti et al., 2010; Chung et al., 2010, 2011; Zwonitzer et al., 2010; Poland et al., 2011; Schaefer and Bernardo, 2013; Chen et al., 2016; Shridhar Hegde et al., 2018; Xia et al., 2020). Resistance to multiple diseases conditioned by the same locus is an important consideration in breeding durable resistant genotypes. The information regarding studies on the application of markers linked to QTL for pyramiding quantitative resistance to multiple foliar pathogens in maize is less. Hence, an attempt was made to identify QTL for resistance to northern corn leaf blight (NCLB), to investigate the co-location of QTL for resistance to NCLB with those for sorghum downy mildew (SDM) and southern corn rust (SCR) diseases detected using the same mapping population, and also to develop multiple disease-resistant inbred lines pyramided with major QTL for these three foliar diseases.
Methodology
Development of Mapping Population
Based on previous experimental data, two inbred lines, viz. CML153 (susceptible inbred, P1) and SKV50 (resistant inbred, P2), with contrasting disease reaction against northern corn leaf blight were selected for the development of the mapping population. The selected inbreds were crossed during the rainy season of 2012. The F1 generation (CML153 × SKV50) was grown during winter 2012–2013 and self-pollinated. The resulting F2 individuals were planted during summer 2013 and selfed to derive 344 F2:3 families. In each F2:3 family, the leaves from five randomly selected plants were collected for genotypic analysis. Later, during the rainy season of 2013 and 2014, F2:3 families were screened for disease reaction against northern corn leaf blight in the national disease nursery maintained at ZARS, V.C. Farm, Mandya.
Genotyping of F2:3 Mapping Population
A set of 768 single nucleotide polymorphisms (SNPs) covering whole maize genome1 was used for the genotyping of parents, and 199 polymorphic SNPs were identified. The 344 F2:3 progenies were genotyped with these polymorphic markers. Leaf samples were pooled from five random plants of each F2:3 family and parents and lyophilized in 96-well plates. Samples were loaded to the Illumina BeadXpress Vera Code Reader for genotyping, according to Illumina protocols2. The polymorphism detected by SNP marker was scored as A = homozygous maternal genotype (CML153), B = homozygous paternal genotype (SKV50), H = heterozygote genotype, and - = missing samples.
Phenotyping of F2:3 Mapping Population
The 344 F2:3 families along with the two parental lines were screened against E. turcicum, causing northern corn leaf blight of maize. The disease screening was conducted in two seasons during the rainy season of 2013 and 2014 in the national disease screening nursery maintained at Mandya. The experimental area was divided into blocks of 3 m width and 32 m length each accommodating 42 progenies. The F2:3 progenies were planted in a single row of 3 m length employing the randomized complete block design with two replications. The spacing of 75 cm between rows and 20 cm between plants was provided.
Screening for Northern Corn Leaf Blight (E. turcicum)
The susceptible inbred CM202 was planted as the first row and the last row in each block and also after every 10th progeny. The artificial inoculation procedure developed by Shekhar and Kumar (2012) was employed to ensure uniform disease development. The initial inoculum for artificial inoculation of E. turcicum was grown in artificial medium under laboratory conditions. The infected leaf tissues were collected from the diseased plants under natural field conditions, washed thrice with sterile water, cultured on potato dextrose agar medium, and then multiplied on sorghum seeds. For this, the sorghum seeds were soaked overnight and transferred to sterilized conical flasks the next day, and the pathogen inoculum was added. The flasks were shaken once in 2 days, and an equal amount of fresh sorghum seeds was mixed after 1 week. The sorghum seeds with pathogen inoculum were ground to a fine powder, and 1–1.5 g of the ground inoculum was added to the leaf whorl of the test entries, followed by a light spray of water to create humidity and initiate infection. Artificial inoculation was made 20 days after sowing between 3:00 and 6:00 p.m., and inoculation was repeated twice after a 1-week interval. The northern corn leaf blight severity was recorded at the flowering stage, i.e., 60th day after sowing by visualizing the leaf area using a standard scale consisting of five broad categories with intermediate ratings (Payak and Sharma, 1983). Based on the disease score, progenies were classified as resistant (<2.5), moderately resistant (2.5 to <3.0), moderately susceptible (3.0 to <3.5), susceptible (3.5 to <4.0), and highly susceptible (4.0–5.0). The disease score data were converted into percent disease severity by using the formula given by Wheeler (1969).
Statistical Analysis
Phenotypic Data Analysis
Transformation of field data
The disease data recorded as percent disease index for northern leaf blight infection ranged from 0 to 100. To make the means and variances independent and normally distributed, the percentage data were subjected to arcsine transformation (Little and Hills, 1978). The analysis of variance was performed on transformed phenotypic data using PROC GLM procedure of SAS package version 9.3. Before pooling the data, Bartlett’s test was conducted to test for homogeneity between environments (Gomez and Gomez, 1984). The components of variance in both the seasons were estimated considering various effects (seasons, replicates, and F3 families) as random in the statistical model. As described by Bohn et al. (1996), transformed entry means were used for the combined analysis of variance. The variance components were estimated as per Searle (1971). The heritability (h2) was calculated following Hallauer and Miranda (1981).
Where r = the number of replications and e = the number of environments.
To understand the nature of distribution of F2:3 progenies with respect to disease incidence, skewness and kurtosis were estimated (Snedecor and Cochran, 1994).
Linkage Map Construction Using iMAS (GMendel)
We used 199 SNP marker data on 344 F2:3 progenies for linkage map construction. Five markers showed segregation distortion (SD) and the remaining markers showed expected Mendelian segregation ratio of 1:2:1 as revealed by the χ2 test. The linkage analysis was performed with 194 markers using GMendel program of iMAS software. For determining linkage groups, a minimum logarithm of odds (LOD) of 3.0 and maximum recombination fraction of 0.40 were set as threshold values. The unique feature of GMendel 2.0 is that it performs multipoint linkage analysis on populations with complex genetic structures. It generates two point maximum likelihood estimates for all pairwise markers. Based on probability rules, linkage phases are correctly assigned and gene order is estimated using an advance multipoint mapping algorithm. Using a powerful method called the simulated annealing algorithm (SAA), multipoint gene order was determined by GMendel 2.0. The validation of marker ordering was carried out by Monte Carlo and bootstrap methods. Using the Haldane mapping function, recombination fraction was converted into map distance in centimorgan (cM) and the linkage map was constructed using intermarker distances calculated from the GMendel program.
QTL Location by WinQTL Cartographer Version 2.5
The analysis of QTL controlling the northern corn leaf blight resistance was performed on the arcsine-transformed means of F2:3 families within each season as well as over seasons. The phenotypic data (rainy season of 2013 and 2014) and genotypic data of 194 SNP markers across 10 chromosomes were used to identify the QTL associated with disease resistance employing WinQTL Cartographer version 2.5 (Wang et al., 2010). The replicated mean data of 344 F3 progenies for northern leaf blight were used for QTL mapping in each season. To determine the QTL across the seasons, replicated means of across-season means of 344 F2:3 progenies were used. The composite interval mapping method (CIM) was used for QTL analysis (Zeng, 1994) employing WinQTL Cartographer 2.5. The presence of putative QTL in an interval was tested using the Bonferroni χ2 approximation (Zeng, 1994) corresponding to genome-wise type-I error by using a critical value for the LOD threshold of 2.5. Both additive and dominance models were used for the analysis in this study as the mapping population is comprised of F2:3 progenies.
The ratio of the absolute value of dominance effect to the absolute value of additive effect, i.e., | d| /| a|, was used to determine gene action with 0–0.20 = additive, 0.21–0.80 = partial dominance, 0.81–1.20 = dominance, and >1.20 = overdominance (Stuber et al., 1987).
Co-localization of QTL for Multiple Foliar Diseases of Maize
The QTL for resistance to SDM and SCR were also identified along with NCLB. These QTL were co-localized to different bins of the chromosomes (Gardiner et al., 1993)3. Pearson correlation coefficients between the means of northern leaf blight, sorghum downy mildew, and southern corn rust were calculated to assess the degree of association between the foliar diseases of maize.
rp (xy) | = | Covp (xy)/√σ2p (x). σ2p (y |
Where,
rp (xy) = Correlation between “x” and “y”
Covp (xy) = Covariance between “x” and “y”
σ2p (x) = Variance of “x”
σ2p (y) = Variance of “y”
Development of Inbred Lines With Multiple Disease Resistance and High General Combining Ability
We attempted to develop inbred lines with resistance to three foliar diseases, namely sorghum downy mildew, northern corn leaf blight, and Polysora rust, using the high combining susceptible inbred lines CML153, CM212, and HKI162. These inbreds were crossed to the resistant inbred SKV50 during the 2014 summer season to derive inbred lines. The three F1s (CM212 × SKV50, HKI162 × SKV50, and CML153 × SKV50) were raised during the rainy season of 2015 and selfed. During the summer season of 2016, F2 plants were raised and screened for three major QTL present on chromosomes 8 (MZA6428-11 and MZA3856-10) for NCLB, 2 (MZA3668-12 and C00324-01) for SDM, and 10 (MZA15331-16 and MZA3922-32) for SCR resistance. The resistant progenies were advanced to the F6 stage employing the plant-to-row approach. These progenies were screened in disease nurseries and 125 progenies with acceptable level of disease reaction were identified from three crosses. These progenies were crossed to the open pollinated tester CM500, 77 test cross progenies were obtained and evaluated during the summer season of 2017 in a single row plot of 4 m length with two replications to identify progenies with high general combining ability. The 77 progenies were evaluated for disease reaction during the rainy season of 2018.
Results
Phenotypic Data Analysis
Weather conditions at Mandya favored the development of severe northern corn leaf blight disease. The percent disease severity and percent disease index values during the rainy season of 2013 and 2014 ranged from 0 to 100% which followed a binomial distribution. An attempt was made to make the means and variances independent and normally distributed by subjecting the data for arcsine transformation. The arcsine-transformed percent disease data of 344 F2:3 progenies were used for statistical analysis and QTL mapping.
The parents CML153 and SKV50 differed significantly in their reaction to the NCLB disease as indicated by their percent disease incidence (Table 1). The parent SKV50 showed resistance reaction and CML153 was susceptible. In F3 progenies, the mean northern corn leaf blight disease incidence was 52.38% in 2013, 40.71% in 2014, and 46.55% when pooled over seasons. The maximum range of disease incidence (17.14–84.29%) was recorded in 2013 followed by 2014 (16.00–74.00%).

Table 1. Mean disease incidence, skewness, kurtosis, variance components, and heritability (H) for reaction of F2:3 progenies to northern corn leaf blight (NCLB) during the rainy season of 2013 and 2014.
The analysis of variance revealed significant differences among the progenies indicating the presence of genetic variability in the F3 progenies (Table 1). Non-significant Bartlett’s χ2 test indicated the homogeneity of error mean sum of squares between seasons, and hence, data were pooled. Variance due to genotype and genotype × season interaction was significant, indicating that the expression of disease incidence significantly varied among F3 families and depends upon testing season.
Genetic Variability Studies in the F2:3 Population of Maize
The estimates of phenotypic coefficient of variation (PCV) and genotypic coefficient of variation (GCV) for northern corn leaf blight were moderate in 2013 and 2014 (Table 1). High heritability and moderate GAM were noticed in 2013 (58.82 and 19.68%, respectively) and 2014 (43.67 and 16.57%, respectively), whereas in the pooled data, the heritability value was 65.72% and GAM was 17.42%.
Test for Normality, Skewness, and Kurtosis
The frequency distribution pattern of F3 families was positively skewed and platykurtic for NCLB disease (Figure 1). The results of the test for normality by the Kolmogorov–Smirnov goodness-of-fit test indicated that the distribution of phenotypic means, within and across the seasons, deviated significantly from normal distribution with majority of the progenies skewed toward resistance in northern corn leaf blight.
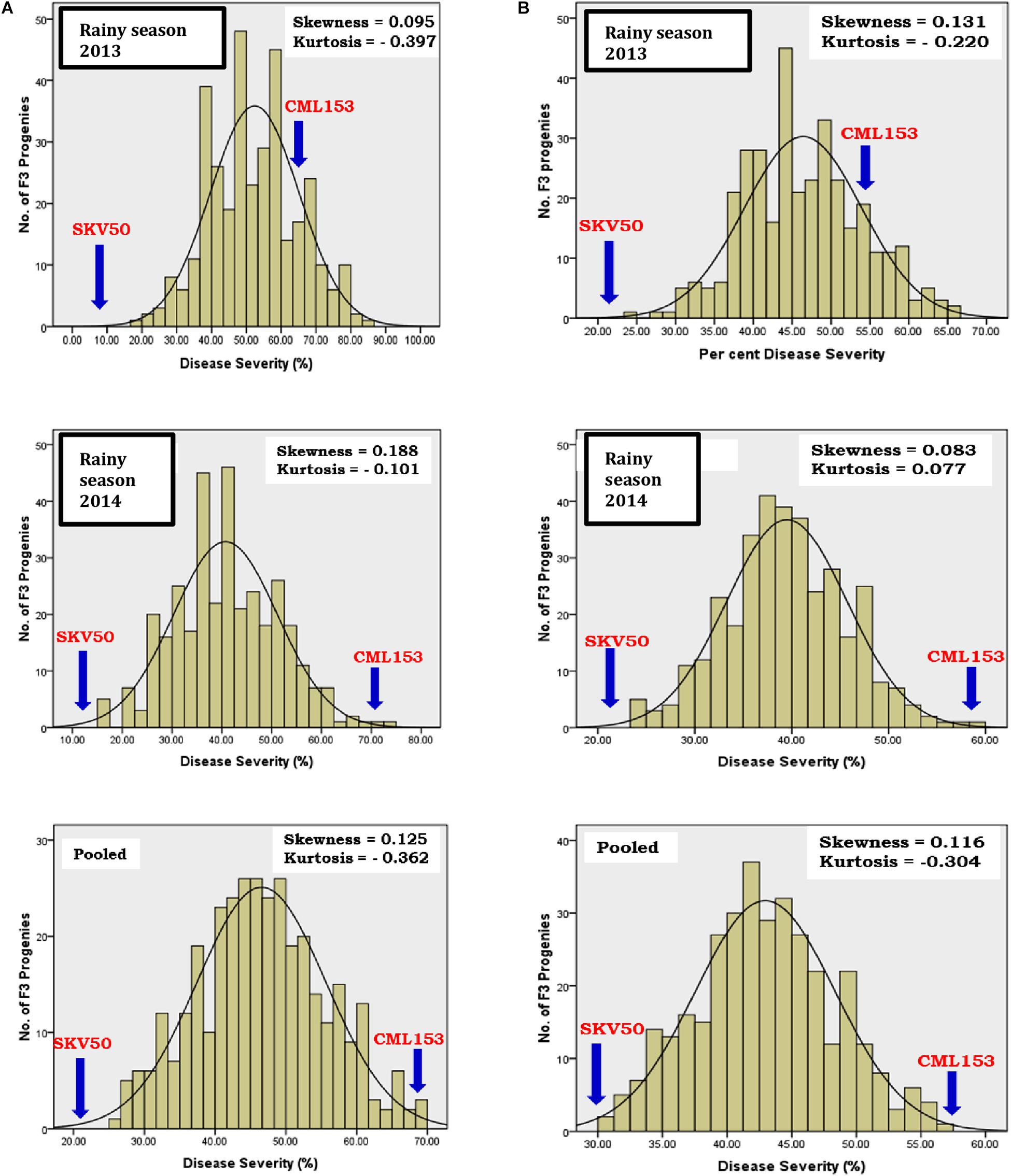
Figure 1. Frequency distribution of mean per cent disease severity of northern corn leaf blight in the F2:3 population derived from the cross CML153 × SKV50 (A, original; B, Arcsine transformed).
Construction of the Linkage Map
Out of the 768 SNP markers, 194 SNP markers were found polymorphic and segregating in Mendelian fashion (1:2:1) and, hence, used for linkage map construction. The markers were mapped on 10 linkage groups (LGs) spanning 2,143.02 cM. The number of markers mapped per linkage group ranged from 6 (LG7) to 33 (LG1). The length of the linkage groups ranged from 152.25 (LG9) to 308.76 cM (LG1) with an average interval distance of 10.77 cM, indicating comparatively high-density SNP linkage map. The SNP map constructed was compared to the maize genome database4,5 and genomically analyzed according to a previous study reported by Jones et al. (2009). This linkage map was used for the identification and mapping of QTL conferring resistance to three foliar diseases of maize.
QTL Analysis
Three QTL positions were identified for northern corn leaf blight resistance during the rainy season of 2013 (Table 2 and Figures 2, 3). One QTL was located on chromosome 2 (qNCLB-2) flanked by markers C00359-01–MZA13360-13 which explained 3.07% phenotypic variation with LOD of 3.06. Two QTL were located on chromosome 8 (qNCLB-8-1 and qNCLB-8-2) flanked by markers, viz. MZA2487-6 and MZA6428-11–MZA3856-10, and these two QTL showed phenotypic variation of 2.46 and 22.97% with LOD score of 2.77 and 3.44, respectively. A major northern corn leaf blight QTL was mapped on chromosome 8 (qNCLB-8-2) which explained maximum phenotypic variation of 22.97%. These three identified QTL explained a total of 28.50% phenotypic variation. Additive gene effects of these three QTL ranged from 1.71 to 1.92, and the favorable alleles for these QTL were contributed by the resistant parent SKV50. The QTL located on chromosome 2 (qNCLB-2) and on chromosome 8 (qNCLB-8-2) exhibited overdominance gene action, while another QTL on chromosome 8 (qNCLB-8-1) revealed a dominance type of gene action.
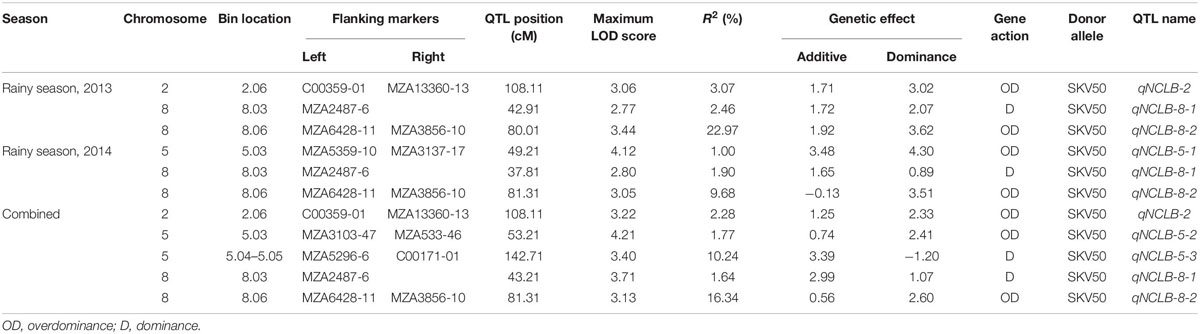
Table 2. QTL detected for northern corn leaf blight resistance during individual seasons and combined over seasons using 344 F2:3 families from the cross CML 153 × SKV 50 (threshold LOD score = 2.50).
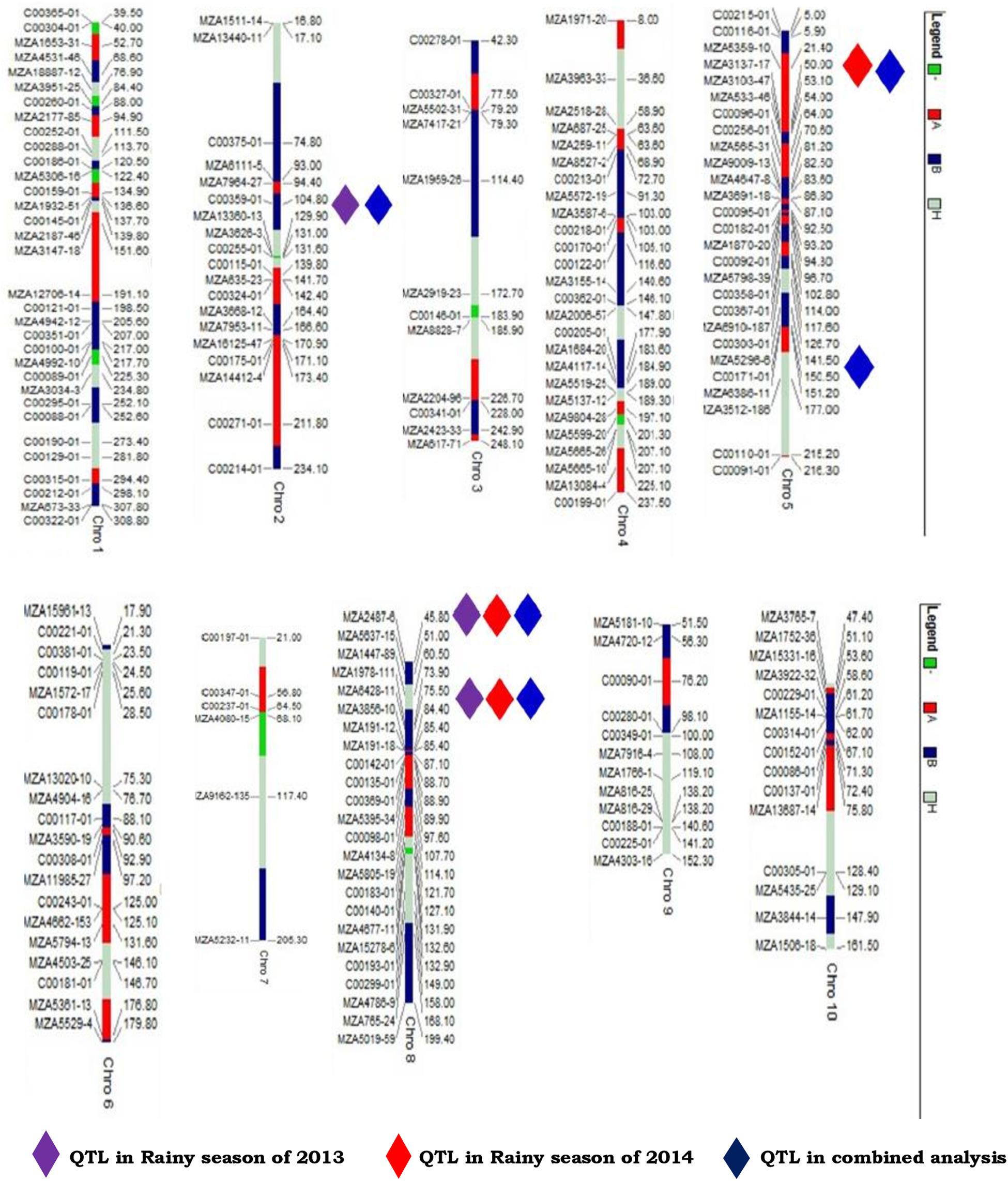
Figure 2. Linkage map and position of the QTL associated with northern corn leaf blight resistance mapped from F2:3 mapping population of the cross CML153 × SKV50.
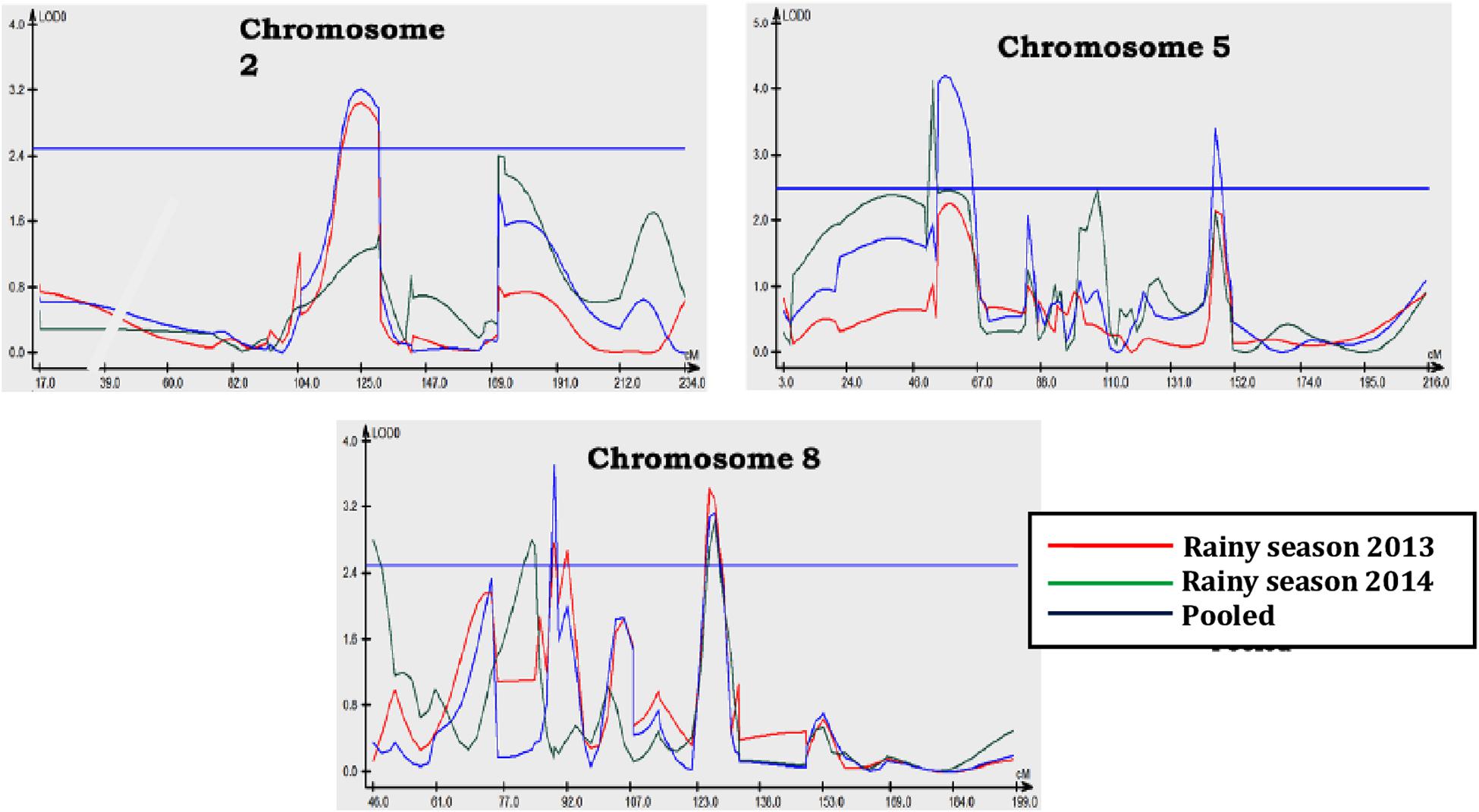
Figure 3. LOD peak for QTL conditioning resistance to northern corn leaf blight on chromosomes 2, 5, and 8 in rainy season (Kharif) of 2013, 2014, and pooled analysis over seasons.
During the rainy season of 2014, three QTL regions conferring resistance to northern corn leaf blight were mapped onto chromosome 5 and chromosome 8. Among these, a QTL bracketed by markers MZA6428-11–MZA3856-10 on chromosome 8 explained the highest phenotypic variation of 16.8% with LOD score 3.05 (qNCLB-8-2) followed by a QTL on chromosome 8 present adjacent to the marker MZA2487-6 which explained 2.90% of phenotypic variation with LOD score of 2.80 (qNCLB-8-1). Other QTL on chromosome 5 flanked by markers MZA5359-10–MZA3137-17 (qNCLB-5-1) contributed 2.00% to the phenotypic variation with LOD score of 4.12. The range of additive genetic effects for these QTL was from −0.13 to 3.48, and the total phenotypic variation of 21.60% was explained by the QTL identified. Favorable allele for QTL located on chromosome 8 (qNCLB-8-2) was contributed by susceptible parent CML153, whereas for other QTL, it was by the resistant parent SKV50. The QTL (qNCLB-8-1) on chromosome 8 showed dominance gene action and two QTL (qNCLB-8-2 and qNCLB-5-1) located on chromosomes 8 and 5 showed overdominance gene action.
In the combined QTL analysis, five QTL were detected on chromosomes 2, 5, and 8. Among these QTL, three QTL flanked by markers C00359-01–MZA1336013 (qNCLB-2), MZA2487-6 (qNCLB-8-1), and MZA6428-11–MZA3856-10 (qNCLB-8-2) were found to be consistent across two seasons with a LOD score of 3.22, 3.71, and 3.13. Two novel QTL were located on chromosome 5 flanked by markers MZA3103-47–MZA533-46 (qNCLB-5-2) and MZA5296-6–C00171-01 (qNCLB-5-3). The QTL qNCLB-8-2 explained the highest phenotypic variation of 16.34% followed by qNCLB-5-3 (10.24%). A total phenotypic variation explained by these QTL was 32.27% in the pooled analysis. An additive genetic effect of these QTL ranged from 0.74 to 3.39, and the favorable allele for these QTL was contributed by the resistant parent SKV50. Out of five QTL, two QTL (qNCLB-5-3 and qNCLB-8-1) located on chromosomes 5 and 8 exhibited dominance, and the other three QTL (qNCLB-2, qNCLB-5-2, and qNCLB-8-2) on chromosomes 2, 5, and 8 showed overdominance gene action in the combined analysis.
Co-localization of QTL Conferring Resistance to Multiple Foliar Pathogens
Since QTL analysis for sorghum downy mildew and southern corn rust was performed from one year data, results were not presented. However, the information generated was used for the estimation of pairwise Pearson correlation coefficient between three diseases (northern corn leaf blight, sorghum downy mildew, and southern corn rust) and co-localization of QTL. The highest significant and positive correlation was noticed between northern corn leaf blight and southern corn rust (r = 0.122), whereas a positive but non-significant pairwise correlation between sorghum downy mildew and southern corn rust (r = 0.061) was observed (Table 3). Between sorghum downy mildew and northern corn leaf blight, a negatively significant correlation was revealed (r = −0.120). This clearly indicated the presence of genes carrying resistance to multiple diseases in the F2:3 population derived from the cross CML153 × SKV50. In the present study, co-localized chromosomal regions harboring QTL for northern corn leaf blight, sorghum downy mildew, and southern corn rust resistance were observed (Table 4). In bin 8.03, QTL conferring resistance to all three foliar diseases, viz. northern corn leaf blight (one QTL), sorghum downy mildew (two QTL), and southern corn rust (two QTL), were co-localized at approximately the same map position (<10 cM difference between the QTL peaks for the three diseases) with the common adjacent marker MZA2487-6. The bin 5.03–5.04 was significantly associated with resistance to northern corn leaf blight and sorghum downy mildew, wherein two QTL for northern corn leaf blight and one QTL for sorghum downy mildew co-localized with a map distance of <3 cM. This co-localized QTL exhibited 10.24 and 5.98% of total phenotypic variation for northern corn leaf blight and sorghum downy mildew, respectively. The QTL for southern corn rust and sorghum downy mildew resistance were co-localized in bin 3.04 at a map distance of 1 cM with a common flanking marker MZA1959-26, while QTL conferring resistance to northern corn leaf blight and sorghum downy mildew were co-localized in chromosome bin 2.06.
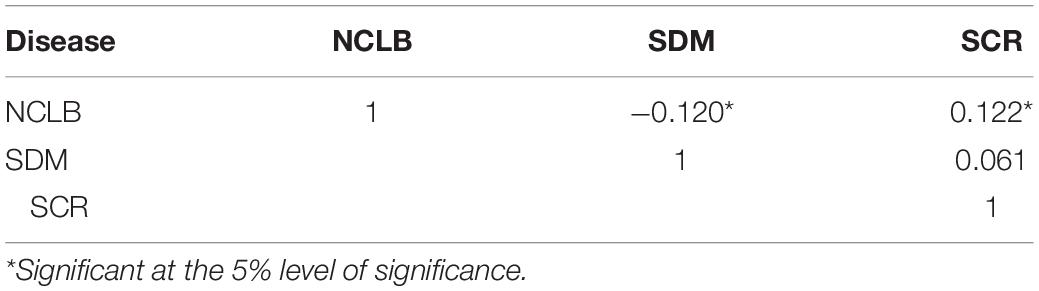
Table 3. Pearson correlation coefficient between mean disease severity for three diseases, viz. northern corn leaf blight (NCLB), sorghum downy mildew (SDM), and southern corn rust (SCR).
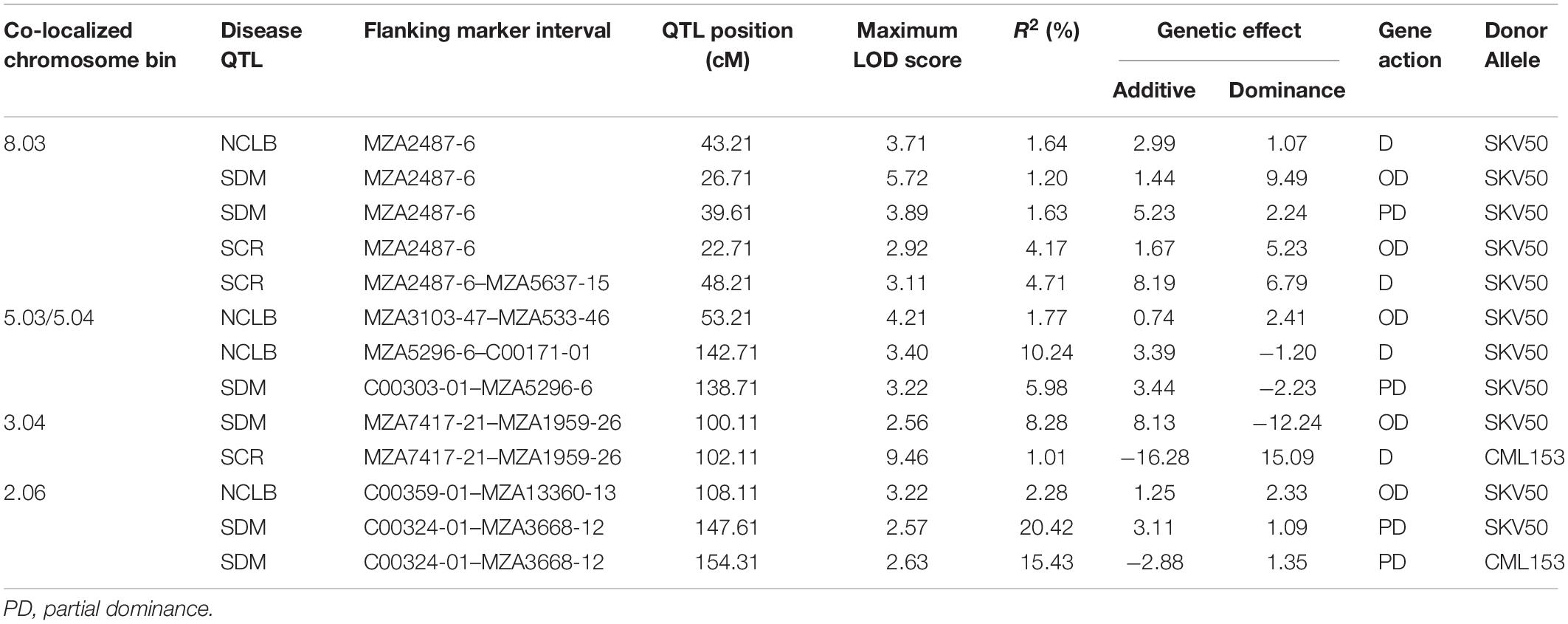
Table 4. Co-located QTL conditioning resistance to northern corn leaf blight (NCLB), sorghum downy mildew (SDM), and southern corn rust (SCR).
Development of Inbred Lines With Resistance to Three Diseases With Good General Combining Ability
We developed 125 lines and crossed with the broad genetic base tester CM500 for testing their combining ability. Only 77 lines produced successful test cross progenies. The evaluation of these test cross progenies resulted in the identification of 39 lines with high general combining ability for grain yield with 17 lines showing significant standard heterosis. These 77 lines exhibited resistance/moderate resistance to NCLB, SDM, and Polysora rust diseases (Supplementary Table 1).
Discussion
Highly diverse parents for multiple pathogen infection were used in the development of F2:3 progenies which resulted in highly significant differences among the progenies for disease reaction against northern corn leaf blight pathogen. The significant difference among means of F3 families for northern corn leaf blight indicated the presence of genotypic variability within the population. Assuming a random effects model, Bartlett’s test proved the homogeneity of error mean sum of squares for northern corn leaf blight data between 2013 and 2014. Therefore, data from these two seasons were pooled. In the pooled analysis, genotype × season interaction was significant, demonstrating the influence of season on northern corn leaf blight incidence (Chen et al., 2016; Shridhar Hegde et al., 2018). In this study, disease pressure was high in 2013 compared with 2014. A significant seasonal effect was observed which indicated that disease development was highly influenced by weather conditions like rainfall, temperature, and relative humidity. Weather data recorded at the V.C. Farm, Mandya indicated comparatively more number of rainy days coupled with high humidity during 2013 resulting in a higher range of disease expression.
Frequency distribution of 344 F3 progenies from the cross CML153 × SKV50 revealed non-normal distribution for the incidence of NCLB. Positively skewed distribution was observed for northern corn leaf blight in each season data and pooled data. Skewed distribution was observed toward the resistant parent SKV50 indicating the dominance of resistance (Schechert et al., 1999; Welz et al., 1999; Brown et al., 2001; Asea et al., 2009). However, the distribution was made near normal through arcsine transformation of the percent disease incidence data. Approximately or near normal distribution in phenotypic data was obtained earlier on F2:3 populations (Holland et al., 1998; Brunelli et al., 2002; Shridhar Hegde et al., 2018). The resistance to NCLB disease appeared to be controlled by a larger number of genes having decreasing effects with the involvement of dominance-based complementary interaction as evidenced by platykurtic and positively skewed distribution (Pooni et al., 1977; Choo and Reinbergs, 1982). High heritability with high genetic advance over mean indicated the reliability of the estimates of variation between F3 families, and a reasonable progress in selection is possible for disease resistance in this population. Similar results were reported for northern corn leaf blight by Freymark et al. (1993, 1994), Schechert et al. (1999), Hakiza et al. (2004), Balint-Kurti et al. (2010), and Zwonitzer et al. (2010).
In the present investigation, 194 SNP markers showed the expected Mendelian segregation ratios and were used to construct relatively high density linkage map consisting of 10 linkage groups. The total map distance covered about 2,143.02 cM with an average interval length of 10.77 cM. The length of a linkage map is influenced by a number of factors, such as the number of markers, size of the mapping population, and genotyping accuracy. The average distance between markers in our linkage map was 10.77 cM, which was longer than the other saturated linkage maps with SNP markers (729.28–2,236.66 cM in length with an interval of 0.66–10 cM) which were successfully utilized for the identification of QTL for various traits in maize (Zou et al., 2012; Chen et al., 2016; Zhou et al., 2016; Su et al., 2017; Xia et al., 2020). However, Doerge (2002) was of the opinion that QTL mapping of important traits can be practiced with marker density up to 20 cM. The near normal distribution pattern of F3 progenies suggested the reliability of the F2:3 mapping population for the identification of QTL for resistance to northern corn leaf blight.
Mapping of QTL Conferring Resistance to Northern Corn Leaf Blight
The identification of QTL with resistance to diseases could serve as a novel strategy to develop disease-resistant lines. The SNP-based linkage maps have been used to precisely map disease resistance through genetic linkage and extensively helped researchers in understanding the function of the chromosomal region or locus at gene levels (St Clair, 2010). In this study, genomic locations of QTL for northern corn leaf blight were identified on chromosomes 2, 5, and 8. The QTL on chromosomes 5 and 8 were major with a high percentage of the phenotypic variance for resistance. The QTL detected on chromosome 8 in bin 8.06 explained 16.34% of the phenotypic variance followed by the QTL on chromosome 5 which explained 10.24% variation in the combined analysis. When considered together, the total variance explained by these QTL was 26.58%. The UMC reference map of maize (Davis et al., 1999) was used to compare QTL positions (Lin et al., 1995; Tuberosa et al., 2002). The major QTL on chromosome bins 5.04–5.05 and 8.06 were also reported by Freymark et al. (1993, 1994) in bins 5.03–5.05 and 8.03–8.06, Dingerdissen et al. (1996) in bins 5.03 and 8.06, Schechert et al. (1999) in bins 5.03–5.05 and 8.06, Welz et al. (1999) in bins 5.03–5.04 and 8.02–8.06, Welz and Geiger (2000) in bins 5.04 and 8.06, Brown et al. (2001) in bin 8.06, Asea et al. (2009) in bins 5.04 and 8.06, Chung et al. (2011) in bins 5.03 and 8.06, and Chen et al. (2016) in bin 5.04. This supports the suggestion from Chung et al. (2010), who fine-mapped northern corn leaf blight QTL on chromosome bin 8.06, that a major QTL region on chromosome 8 affects the response to northern corn leaf blight. Similarly, an important role of QTL on chromosome 5 at bin 4 was deciphered as an additional QTL to that already known in bins 5.03, 5.04, and 5.05 by Welz et al. (1999), Asea et al. (2009), and Chung et al. (2011). Another QTL detected in chromosome bin 2.06 was also reported earlier by Schechert et al. (1999), Welz et al. (1999), Brown et al. (2001), Ping et al. (2007), Balint-Kurti et al. (2010), Zwonitzer et al. (2010); and Shridhar Hegde et al. (2018). The major QTL identified in our study at bin location 8.06 exhibited overdominance (OD) gene action. It could be confirmed that northern corn leaf blight resistance alleles in SKV50 are a good source for marker-assisted selection supporting maize breeding programs. Similar findings were observed by Welz et al. (1999), Welz and Geiger (2000), Brown et al. (2001), Chung et al. (2010), Zwonitzer et al. (2010), and Chung et al. (2011).
One of the major goals of QTL mapping is to identify and use QTL with little QTL × environment interaction (Stuber et al., 1987). This was substantiated in the present study as QTL in bins 8.03 and 8.06 were detected in both seasons and in the pooled analysis. Earlier workers also reported the identification of QTL in all environments with difference in the level of significance and magnitude of genetic effects (Brown et al., 2001; Asea et al., 2009; Balint-Kurti et al., 2010; Chen et al., 2016; Shridhar Hegde et al., 2018). Conclusively, the markers associated with QTL in bins 2.06 (C00359-01 and MZA13360-13), 5.03 (MZA3103-47 and MZA533-46), 5.04 (MZA5296-6 and C00171-01), and 8.06 (MZA6428-11 and MZA3856-10) are the favorites to be used to transfer resistance alleles to susceptible lines (Xia et al., 2020). The QTL localized in chromosome bin 5.04 was previously detected in the NAM population (Poland et al., 2011) and is known to contain a candidate gene, GRMZM2G024612 (Xia et al., 2020). The genomic region in chromosome 8 is important as two resistant genes Ht2 and Htn1 were identified previously (Zaitlin et al., 1992; Simcox and Bennetzen, 1993; Hurni et al., 2015; Kaefer et al., 2017). Wisser et al. (2006) reported Histatin-1 (Htn1) as a maize disease resistance gene against NCLB and is known to encode a wall-associated receptor-like kinase that acts as an important component of the plant innate immune system by perceiving pathogen or host-derived elicitors (Hurni et al., 2015). The gene Ht2 has been further delimited to a region of up to 0.46 Mb via precise mapping, and other candidate genes were predicted and annotated according to the reference genome sequences of B73 (Chung et al., 2010). Except for chromosomes 5 and 8, NCLB resistance-related QTL seems to be relatively rare at other chromosomes (Xia et al., 2020).
Clearly, there is a lack of commonality between the QTL identified for northern corn leaf blight in different populations. This could be because of multiple reasons such as the use of different types and sizes of mapping populations, involvement of different sets of QTL in different crosses, and epistatic interaction between QTL. Furthermore, as noted in previous studies, it is possible that QTL may not be detected in certain segregating populations if alternate alleles of the QTL are not contributed by both parents (Beavis and Keim, 1996; Kearsey and Pooni, 1996; Bohn et al., 1997). In a previous study, a comparison of QTL for disease resistance in multiple segregating populations revealed only few common QTL (Beavis et al., 1991). This is primarily due to environmental conditions existing in a particular region which might affect the QTL expression. Nonetheless, the detection of different QTL present in diverse resistant genotypes could aid in the pyramiding of multiple QTL in cultivars.
Co-localization of QTL Conferring Resistance to Three Foliar Diseases
Mapping resistance loci for multiple pathogens provides an opportunity for co-localizing resistance loci. Such resistance gene combinations are expected to provide more durable protection (Simmonds, 1985) against a variable number of pathogens. In the present study, QTL for northern corn leaf blight, sorghum downy mildew, and southern corn rust resistance were co-localized based on the bin locations and chromosomal regions where QTL were detected. Associations between resistance to northern corn leaf blight, sorghum downy mildew, and southern corn rust were significant in the F2:3 population. These associations were detected most likely because of alleles showing a high level of resistance to one disease and a lower level to another which may not be detected by QTL analysis. Alternatively, it could be because of alleles that confer varied levels of resistance to multiple diseases that are undetectable by QTL analysis. A total of 13 QTL associated with resistance to two or more diseases were detected. These QTL regions may carry a single gene showing multiple disease resistance or due to two closely linked genes causing resistance to different diseases in the F2:3 population. The inbred SKV50 with resistance to foliar diseases, viz. northern corn leaf blight, sorghum downy mildew, and southern corn rust, could be used for pyramiding resistance QTL. The study also indicated the potential of using several target QTL present in chromosome bin 8.03 for resistance to northern corn leaf blight, sorghum downy mildew, and southern corn rust: bins 5.03–5.04 and 2.06 for resistance to northern corn leaf blight and sorghum downy mildew and bin 3.04 for resistance to sorghum downy mildew and southern corn rust for marker-assisted selection to pyramid quantitative resistance to multiple foliar pathogens as described by Pratt and Gordon (2006).
The present study resulted in the identification of markers linked to resistance to northern corn leaf blight, and some common QTL for resistance to three important foliar diseases were identified. It was also possible to validate the major QTL linked to NCLB, SDM, and SCR and to develop 39 inbred lines with high general combining ability which could be used in hybrid development programs.
Data Availability Statement
The original contributions presented in the study are included in the article/Supplementary Material, further inquiries can be directed to the corresponding author/s.
Author Contributions
HL and HR designed and carried out the experiments and contributed to manuscript preparation. AP helped in genotyping of the mapping populations and editing of the manuscript. All authors contributed to the article and approved the submitted version.
Funding
We acknowledge the financial support from the M/s Pioneer Hi-Bred Pvt. Ltd., India, to HL. HR was supported by the DST under the PURSE program.
Conflict of Interest
AP is employed by company Corteva Agriscience Pvt. Ltd.
The remaining authors declare that the research was conducted in the absence of any commercial or financial relationships that could be construed as a potential conflict of interest.
Acknowledgments
The authors are highly indebted to Dr. H. C. Prasanna, Principal Scientist (Plant Breeding), Indian Institute of Horticultural Research, Bengaluru, for carefully editing and providing inputs to improve the manuscript.
Supplementary Material
The Supplementary Material for this article can be found online at: https://www.frontiersin.org/articles/10.3389/fgene.2020.548407/full#supplementary-material
Footnotes
- ^ www.panzea.com
- ^ http://www.illumina.com/
- ^ www.maizegdb.org/bin-viewer
- ^ www.panzea.org
- ^ www.maizeGDB.org
References
Ali, F., and Yan, J. B. (2012). The phenomenon of disease resistance in maize and the role of molecular breeding in defending against global threat. J. Integr. Plant Biol. 54, 134–151. doi: 10.1111/j.1744-7909.2012.01105.x
Asea, G., Vivek, B. S., Bigirwa, G., Lipps, P. E., and Pratt, R. C. (2009). Validation of consensus quantitative trait loci associated with resistance to multiple foliar pathogens of maize. Phytopathology 99, 540–547. doi: 10.1094/phyto-99-5-0540
Balint-Kurti, P. J., Yang, J., Esbroeck, G. V., Jung, J., and Smith, M. E. (2010). Use of a maize advanced intercross line for mapping of QTL for northern leaf blight resistance and multiple disease resistance. Crop Sci. 50, 458–466. doi: 10.2135/cropsci2009.02.0066
Beavis, W. D., Grant, D., Albertsen, M., and Fincher, R. (1991). Quantitative trait loci for plant height in four maize populations and their association with qualitative trait loci. Theor. Appl. Genet. 83, 161–165.
Beavis, W. D., and Keim, P. (1996). “Identification of quantitative trait locithat are affected by environment,” in Genotype by Environment Interaction, eds M. S. Kang and H. G. Gauch Jr. (BocaRaton, FL: CRC Press), 123–149. doi: 10.1201/9781420049374.ch5
Bohn, M., Khairallah, M. M., Gonzalez-de-Leon, D., Hoisington, D., Utz, H. F., Deutsch, J. A., et al. (1996). QTL mapping in tropical maize: I. Genomic regions affecting leaf feeding resistance to sugarcane borer and other traits. Crop Sci. 36, 1352–1361. doi: 10.2135/cropsci1996.0011183x003600050045x
Bohn, M., Khairallah, M. M., Jiang, C. Z., Gonzalez de Leon, D., Hoisington, D., Utz, H. F., et al. (1997). QTL mapping in tropical maize. 2. Comparison of genomic regions for resistance to Diatraea spp. Crop Sci. 37, 1892–1902. doi: 10.2135/cropsci1997.0011183x003700060038x
Brown, A. F., Juvik, J. A., and Pataky, J. K. (2001). Quantitative trait loci in sweet corn associated with partial resistance to Stewart’s Wilt, Northern Corn leaf blight, and common rust. Phytopathology 91, 293–300. doi: 10.1094/phyto.2001.91.3.293
Brunelli, K. R., Silva, H. P., and Camargo, L. E. A. (2002). Mapping of resistance genes to Puccinia polysora in maize. Fitopatol. Brasil. 27, 134–140. doi: 10.1590/S0100-41582002000200003
Butler, E. J. (1907). Some diseases of cereals caused by Sclerospora graminicola. Memorandum Dept. Agri. India Bot. Seri. 2, 1–24.
Carson, M. L. (1999). “Helminthosporium leaf spots and blights,” in Compendium of Corn Diseases, 3rd Edn, ed. D. G. White (St. Paul, MN: The American Phytopathol.), 15–24.
Chaudhary, B., and Mani, V. P. (2010). Genetic analysis of resistance to turcicum leaf blight in semi-temperate early maturing genotypes of maize (Zea mays). Indian J. Genet. 70, 65–70.
Chen, G., Xiaoming, W., Long, S., Jaqueth, J., Li, B., Yan, J., et al. (2016). Mapping of QTL conferring resistance to northern corn leaf blight using high-density SNPs in maize. Mol. Breed. 36, 4. doi: 10.1007/s11032-015-0421-3
Choo, T. M., and Reinbergs, E. (1982). Analyses of skewness and kurtosis for detecting gene interaction in a doubled haploid population. Crop Sci. 22, 231–235. doi: 10.2135/cropsci1982.0011183X002200020008x
Chung, C. L., Jamann, T., Longfellow, J., and Nelson, R. (2010). Characterization and fine-mapping of a resistance locus for northern leaf blight in maize bin 8.06. Theor. Appl. Genet. 121, 205–227. doi: 10.1007/s00122-010-1303-z
Chung, C. L., Poland, P., Kump, K., Benson, J., Longfellow, J., Walsh, E., et al. (2011). Targeted discovery of quantitative trait loci for resistance to northern leaf blight and other diseases of maize. Theor. Appl. Genet. 123, 307–326. doi: 10.1007/s00122-011-1585-9
Davis, G. L., McMullen, M. D., Baysdorf, C., Musket, T., Grant, D., Staebell, M., et al. (1999). A maize map standard with sequenced core markers, grass genome reference points and 932 expressed sequence tagged sites (ESTs) in a 1736-locus map. Genetics 152, 1137–1172.
Dingerdissen, A. L., Geiger, H. H., Lee, M., Schechert, A., and Welz, H. G. (1996). Interval mapping of genes for quantitative resistance of maize to Setosphaeria turcica, cause of northern leaf blight, in a tropical environment. Mol. Breed. 2, 143–156. doi: 10.1007/bf00441429
Doerge, R. W. (2002). Mapping and analysis of quantitative trait loci in experimental populations. Nat. Rev. Genet. 3, 43–52. doi: 10.1038/nrg703
Dowswell, C. R., Paliwal, R. L., and Cantrell, R. P. (1996). Maize in the Third World. Boulder: West view press, 1–37.
Freymark, P. J., Lee, M., Woodman, W. L., and Martinson, C. A. (1993). Quantitative and qualitative trait loci affecting host-plant response to Exserohilum turcicum in maize (Zea mays L.). Theor. Appl. Genet. 87, 537–544. doi: 10.1007/bf00221876
Freymark, P. J., Lee, M., Woodman, W. L., and Martinson, C. A. (1994). Molecular-marker facilitated investigation of host-plant response to Exserohilum turcicum in maize (Zea mays L.): components of resistance. Theor. Appl. Genet. 88, 305–313. doi: 10.1007/bf00223637
Gardiner, J. M., Coe, E. H., Melia-Hancock, S., Hoisington, D. A., and Chao, S. (1993). Development of a core RFLP map in maize using an immortalized F2 population. Genetics 134, 917–930.
Gomez, K. A., and Gomez, A. A. (1984). Statistical Procedures for Agricultural Research, 2nd Edn. New York: John Wiley & Sons.
Hakiza, J. J., Lipps, P. E., Martin, S. S., and Pratt, R. C. (2004). Heritability and number of genes controlling partial resistance to Exserohilum turcicum in maize inbred H99. Maydica 49, 173–182.
Hallauer, A. R., and Miranda, J. B. (1981). Quantitative Genetics in Maize Breeding. Ames, IA: Iowa State University Press.
Harlapur, S. I., Mruthunjaya, C. W., Anahosur, K. H., and Muralikrishna, S. (2000). Survey and surveillance of maize diseases in North Karnataka. Karnataka J. Agri. Sci. 13, 750–751.
Hettiarachchi, K., Prasanna, B. M., Rajan, A., Singh, O. N., Gowda, K. T. P., Pant, S. K., et al. (2009). Generation mean analysis of turcicum leaf blight resistance in maize. Indian J. Genet. 69, 102–108.
Holland, J. B., Uhr, D. V., Jeffers, D., and Goodman, M. M. (1998). Inheritance of resistance to southern corn rust in tropical-by-corn-belt maize populations. Theor. Appl. Genet. 96, 232–241. doi: 10.1007/s001220050732
Hughes, G. R., and Hooker, A. L. (1971). Gene action conditioning resistance to northern leaf blight in maize. Crop Sci. 11, 180–184. doi: 10.2135/cropsci1971.0011183X001100020005x
Hurni, S., Scheuermann, D., Krattinger, S. G., et al. (2015). The maize disease resistance gene Htn1 against northern corn leaf blight encodes a wall associated receptor-like kinase. Proc. Natl. Acad. Sci. U.S.A. 112, 8780–8785. doi: 10.1073/pnas.1502522112
Jenkins, M. T., Robert, A. L., and Findley, W. R. (1952). Inheritance of resistance to Helminthosporium turcicum leaf blight in populations of F3 progenies. Agron. J. 44, 438–442. doi: 10.2134/agronj1952.00021962004400080010x
Jones, E., Chu, W. C., Ayele, M., Ho, J., Bruggeman, E., Yourstone, K., et al. (2009). Development of single nucleotide polymorphism (SNP) markers for use in commercial maize (Zea mays L.). Mol. Breed. 24, 165–176. doi: 10.1007/s11032-009-9281-z
Kachapur, M. R., and Hegde, R. K. (1988). Studies on turcicum leaf blight of maize caused by Exserohilum turcicum. Plant Pathol. 6, 33–35.
Kaefer, K. A. C., Schuelter, A. R., Schuster, I., Marcolin, J., and Vendrusco, E. C. G. (2017). Association mapping and genetic control for Northern leaf blight (Exserohilum turcicum) resistance in maize lines. Aust. J. Crop Sci. 11, 1346–1353.
Kearsey, M. J., and Pooni, H. S. (1996). The Genetical Analysis of Quantitative Traits. London: Chapman and Hall.
Lin, Y. R., Schertz, K. F., and Paterson, A. H. (1995). Comparative analysis of QTLQTL affecting plant height and maturity across the Poaceae, in reference to an interspecific sorghum population. Genetics 141, 391–411.
Little, T. M., and Hills, F. J. (1978). Agricultural Experimentation Design and Analysis. New York: John Wiley and Sons.
Matsuoka, Y., Vigouroux, Y., Goodman, M. M., Sanchez, J. G., Buckler, E., and Doebley, J. (2002). A single domestication for maize shown by multilocus microsatellite genotyping. Proc. Natl. Acad. Sci. U.S.A. 99, 6080–6084. doi: 10.1073/pnas.052125199
Payak, M. M., and Sharma, R. C. (1983). Disease Rating Scales in Maize in India. Techniques of Scoring for Resistance to Important Diseases of Maize. All India Coordinated Maize Improvement Project. New Delhi: IARI, 1–4.
Payak, M. M., and Sharma, R. C. (1985). Maize diseases and approaches to their management in India. Trop. Pest. Manag. 31, 302–310. doi: 10.1080/09670878509371006
Payak, M. M., Sharma, R. C., and Lilaramani, J. (1973). How to control maize diseases? Indian Farm. 23, 20–23.
Perkins, J. M., and Pederson, W. L. (1987). Disease treatment and yield loss associated with northern leaf blight of corn. Plant Dis. 71, 940–943. doi: 10.1094/pd-71-0940
Ping, Z. Z., Hong, L. X., Bi, H. Y., Zhong, L., Chuan, H., and Bo, T. Z. (2007). QTL mapping for resistance to northern corn leaf blight in maize. South West China J. Agric. Sci. 20, 634–637.
Poland, J. A., Bradbury, P. J., Buckler, E. S., and Nelson, R. J. (2011). Genome-wide nested association mapping of quantitative resistance to northern leaf blight in maize. Proc. Natl. Acad. Sci. U.S.A. 108, 6893–6898. doi: 10.1073/pnas.1010894108
Pooni, H. S., Jinks, J. L., and Cornish, M. A. (1977). The causes and consequences of non-normality in pretending the properties of recombinant inbred lines. Heredity 38, 329–338. doi: 10.1038/hdy.1977.95
Pratt, R. C., and Gordon, S. G. (2006). Breeding for resistance to maize foliar pathogens. Plant Breed. Rev. 27, 119–173. doi: 10.1002/9780470650349.ch3
Ranganatha, H. M., Lohithaswa, H. C., and Anand, S. P. (2017). Understanding the genetic architecture of resistance to northern corn leaf blight and southern corn rust in maize (Zea mays L.). Indian J. Genet. 77, 357–363. doi: 10.5958/0975-6906.2017.00048.7
Raymundo, A. D., and Hooker, A. C. (1981). Measuring relationship between northern leaf blight of maize and yield losses. Plant Dis. 65, 325–327. doi: 10.1094/pd-65-325
Schaefer, C. M., and Bernardo, R. (2013). Genomewide association mapping of flowering time, kernel composition, and disease resistance in historical minnesota maize inbreds. Crop Sci. 53, 2518–2529. doi: 10.2135/cropsci2013.02.0121
Schechert, A. L., Welz, H. G., and Geiger, H. H. (1999). QTL for resistance to Setosphaeria turcica in tropical African maize. Crop Sci. 39, 514–523. doi: 10.2135/cropsci1999.0011183x003900020036x
Searle, S. R. (1971). Topics in variance components estimation. Biometrics 27, 1–76. doi: 10.2307/2528928
Shekhar, M., and Kumar, S. (2012). Inoculation Methods and Disease Rating Scales for Maize Diseases. Directorate of Maize. New Delhi: ICAR, 2–16.
Shridhar Hegde, K. M., Mahantesh, G., and Meenakshi, G. N. (2018). Mapping QTLQTL for resistance to northern leaf blight in tropical maize (Zea mays L.). Int. J. Curr. Microbiol. Appl. Sci. 7, 1940–1946. doi: 10.20546/ijcmas.2018.706.230
Simcox, K. D., and Bennetzen, J. L. (1993). The use of molecular markers to study Setosphaeria turcica resistance in maize. Phytopathology 83, 1326–1330.
Simmonds, N. W. (1985). A plant breeder’s perspective of durable resistance. FAO Plant Prot. Bullet. 33, 13–17.
Smith, D. R. (1999). “Global disease assessment of corn,” in Proceedings of the fifty-fourth Annual Corn and Sorghum Research Conference, December 9–10, (Chicago, IL), 54.
Snedecor, G. W., and Cochran, W. G. (1994). Statistical Methods, 5th Edn. Ames, IA: Iowa State University Press.
St Clair, D. A. (2010). Quantitative disease resistance and quantitative resistance Loci in breeding. Annu. Rev. Phytopathol. 48, 247–268. doi: 10.1146/annurev-phyto-080508-081904
Stuber, C. W., Edwards, M. D., and Wendel, J. F. (1987). Molecular marker-facilitated investigations of quantitative trait loci in maize. II. Factors influencing yield and its component traits. Crop Sci. 27, 639–648. doi: 10.2135/cropsci1987.0011183x002700040006x
Su, C., Wang, W., Gong, S., Zuo, J., Li, S., and Xu, S. (2017). High density linkage map construction and mapping of yield trait QTL in maize (Zea mays) using the genotyping-by-sequencing (GBS) technology. Front. Plant Sci. 8:706. doi: 10.3389/fpls.2017.00706
Troyer, A. F. (2006). Adaptedness and heterosis in corn and mule hybrids. Crop Sci. 46, 528–548. doi: 10.2135/cropsci2005.0065
Tuberosa, R., Salvi, S., Sanguineti, M. C., Landi, P., Maccaferri, M., and Conti, S. (2002). Mapping QTLQTL regulating morpho-physiological traits and yield: case studies, shortcomings and perspectives in drought-stressed maize. Ann. Bot. 89, 941–963. doi: 10.1093/aob/mcf134
Wang, S., Basten, C. J., and Zeng, Z. B. (2010). Windows QTL Cartographer 2.5. Department of Statistics. Raleigh, NC: NC State University.
Ward, J. M. J., Laing, M. D., and Rijkenberg, F. H. J. (1997). Frequency and timing of fungicide applications for the control of gray leaf spot in maize. Plant Dis. 81, 141–148.
Welz, H. G., and Geiger, H. H. (2000). Genes for resistance to northern corn leaf blight in diverse maize populations. Plant Breed. 119, 1–14. doi: 10.1046/j.1439-0523.2000.00462.x
Welz, H. G., Xia, X. C., Bassetti, P., Melchinger, A. E., and Lubberstedt, T. (1999). QTLQTL for resistance to Setosphaeria turcica in an early maturing dent × flint maize population. Theor. Appl. Genet. 99, 649–655. doi: 10.1007/s001220051280
Wisser, R. J., Balint-Kurti, P. J., and Nelson, R. J. (2006). The genetic architecture of disease resistance in maize: a synthesis of published studies. Phytopathology 96, 120–129. doi: 10.1094/phyto-96-0120
Xia, H., Gao, W., Qu, J., Dai, L., Gao, Y., Lu, S., et al. (2020). Genetic mapping of northern corn leaf blight-resistant quantitative trait loci in maize. Medicine 99, e21326. doi: 10.1097/MD.0000000000021326
Zaitlin, D., DeMars, S., and Gupta, M. (1992). Linkage of a second gene for NCLB resistance to molecular markers in maize. Maize Genet. Coop. Newsl. 66, 69–70.
Zhou, Z., Zhang, C., Zhou, Y., Hao, Z., Wang, Z., Zeng, X., et al. (2016). Genetic dissection of maize plant architecture with an ultra-high density bin map based on recombinant inbred lines. BMC Genom. 17:178. doi: 10.1186/s12864-016-2555-z
Zou, G., Zhai, G., Feng, Q., Yan, S., Wang, A., Zhao, Q., et al. (2012). Identification of QTL for eight agronomically important traits using an ultra-high-density map based on SNPs generated from high-throughput sequencing in sorghum under contrasting photoperiods. J. Exp. Bot. 63, 5451–5462. doi: 10.1093/jxb/ers205
Zwonitzer, J. C., Coles, N. D., Krakowsky, M. D., Arellano, C., Holland, J. B., McMullen, M. D., et al. (2010). Mapping resistance quantitative trait loci for three foliar diseases in a maize recombinant inbred line population-Evidence for multiple disease resistance? Phytopathology 100, 72–79. doi: 10.1094/phyto-100-1-0072
Keywords: northern corn leaf blight, sorghum downy mildew, southern corn rust, linkage map, QTL, marker assisted selection
Citation: Ranganatha HM, Lohithaswa HC and Pandravada A (2021) Mapping and Validation of Major Quantitative Trait Loci for Resistance to Northern Corn Leaf Blight Along With the Determination of the Relationship Between Resistances to Multiple Foliar Pathogens of Maize (Zea mays L.). Front. Genet. 11:548407. doi: 10.3389/fgene.2020.548407
Received: 02 April 2020; Accepted: 14 December 2020;
Published: 29 January 2021.
Edited by:
Deepmala Sehgal, International Maize and Wheat Improvement Center, MexicoReviewed by:
Kumari Neelam, Punjab Agricultural University, IndiaReyazul Rouf Mir, Sher-e-Kashmir University of Agricultural Sciences and Technology of Jammu, India
Copyright © 2021 Ranganatha, Lohithaswa and Pandravada. This is an open-access article distributed under the terms of the Creative Commons Attribution License (CC BY). The use, distribution or reproduction in other forums is permitted, provided the original author(s) and the copyright owner(s) are credited and that the original publication in this journal is cited, in accordance with accepted academic practice. No use, distribution or reproduction is permitted which does not comply with these terms.
*Correspondence: Hirenallur Chandappa Lohithaswa, lohithaswa.chandappa@gmail.com