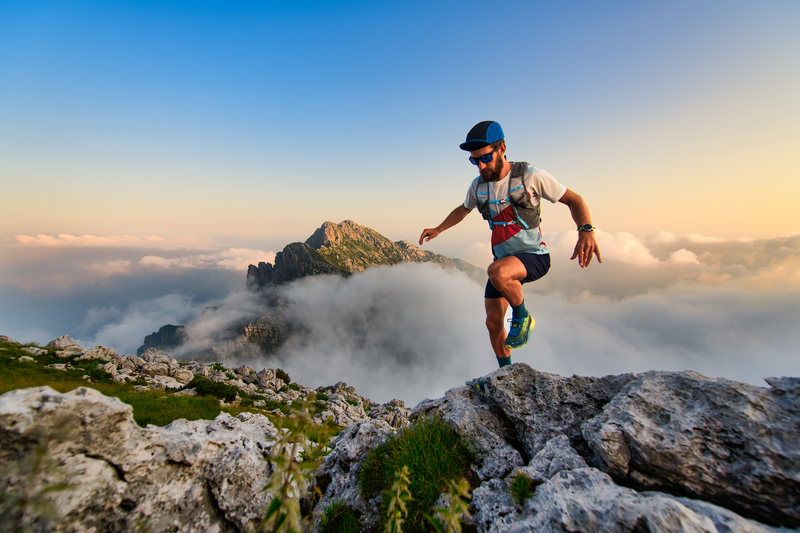
94% of researchers rate our articles as excellent or good
Learn more about the work of our research integrity team to safeguard the quality of each article we publish.
Find out more
REVIEW article
Front. Genet. , 20 August 2020
Sec. Livestock Genomics
Volume 11 - 2020 | https://doi.org/10.3389/fgene.2020.00923
This article is part of the Research Topic High-Throughput Phenotyping in the Genomic Improvement of Livestock View all 17 articles
Parts of this article's content have been modified or rectified in:
Erratum: Infrared Spectrometry as a High-Throughput Phenotyping Technology to Predict Complex Traits in Livestock Systems
High-throughput phenotyping technologies are growing in importance in livestock systems due to their ability to generate real-time, non-invasive, and accurate animal-level information. Collecting such individual-level information can generate novel traits and potentially improve animal selection and management decisions in livestock operations. One of the most relevant tools used in the dairy and beef industry to predict complex traits is infrared spectrometry, which is based on the analysis of the interaction between electromagnetic radiation and matter. The infrared electromagnetic radiation spans an enormous range of wavelengths and frequencies known as the electromagnetic spectrum. The spectrum is divided into different regions, with near- and mid-infrared regions being the main spectral regions used in livestock applications. The advantage of using infrared spectrometry includes speed, non-destructive measurement, and great potential for on-line analysis. This paper aims to review the use of mid- and near-infrared spectrometry techniques as tools to predict complex dairy and beef phenotypes, such as milk composition, feed efficiency, methane emission, fertility, energy balance, health status, and meat quality traits. Although several research studies have used these technologies to predict a wide range of phenotypes, most of them are based on Partial Least Squares (PLS) and did not considered other machine learning (ML) techniques to improve prediction quality. Therefore, we will discuss the role of analytical methods employed on spectral data to improve the predictive ability for complex traits in livestock operations. Furthermore, we will discuss different approaches to reduce data dimensionality and the impact of validation strategies on predictive quality.
For many years dairy and beef cattle breeding have focused on improving the production and profitability of animals through genetics, nutrition, and management, often at the expense of other relevant traits. To remain competitive and meet the world population increase and global climate changes, farmers need to balance production, profitability, and sustainability. There is an extensive list of key phenotypes that must be measured to achieve the emerging breeding goals for the advance of genomic selection (Boichard and Brochard, 2012) and management decisions in the context of precision agriculture. However, recording such phenotypes in large-scale or across different herds and countries is a challenge (Gengler et al., 2016). High-throughput phenotyping technologies have grown in importance in livestock systems because of their ability to generate real-time and accurate animal-level information. Several technologies (e.g., sensors, infrared spectrometry, and image analysis, among others) have been used to generate novel complex traits in dairy and beef cattle, with infrared spectrometry being one of the most relevant tools used in livestock to date (De Marchi et al., 2014; Dixit et al., 2017; Bell and Tzimiropoulos, 2018). Infrared spectrometry is based on the interaction between electromagnetic radiation (infrared light) and matter. The modern Fourier transform infrared spectrometers spans an enormous range of infrared spectrum, which is divided into three main regions: NIR, near-infrared (800–2,500 nm or 4,000–12,500 cm–1); MIR, mid-infrared (2,500–25,000 nm or 400–4,000 cm–1); and FAR, far-infrared (25,000–1,000,000 nm or 10–400 cm–1). NIR and MIR are the main regions used in livestock applications (Griffiths and de Hasenth, 2007). This technology is fast, non-invasive, non-destructive, and has great potential for on-line measurement (De Marchi et al., 2014; Dixit et al., 2017).
Infrared spectrometry, mainly MIR, has been widely used worldwide to predict the concentration of protein, casein, fat, lactose, and urea of milk through regular recording schemes (De Marchi et al., 2014). When cows are milked 2–3 times daily, this biological sample can be more deeply interrogated to generate novel complex traits, which are usually expensive and difficult to be measure on a large scale (e.g., individual milk fatty acids, proteins, feed intake, methane emission, fertility, energy balance, health status, and others). The majority of milk constituents synthesized in the mammary gland are based on the by-products from the digestion of the nutrients ingested in a given day (McParland and Berry, 2016). Therefore, changes in milk composition profile on that day or in the following days can be used as a biomarker for complex phenotypes related to metabolism. Within the beef industry, NIR technology has been shown to be a valuable and cost-effective technology to assess several meat quality attributes (e.g., tenderness, fat content, color, among others) at the same time without any or minimal sample preparation and pretreatment (Prieto et al., 2009a; Dixit et al., 2017; Chapman et al., 2019). Therefore, the NIR technique can be applied directly to the samples, which is an advantage compared to reference methods, and it is also important for the slaughterhouses that can reduce losses with carcass sample assessment and sample preparation. Both technologies (NIR and MIR) have great potential to assess different milk or meat attributes using in-line systems, which could lend deep insights and added efficiencies for both the dairy and beef industries.
Several authors have successfully used infrared spectrometry to predict a range number of traits as reported in previous reviews papers (Prevolnik et al., 2004; Prieto et al., 2009a, 2017; De Marchi et al., 2014; McParland and Berry, 2016; Dixit et al., 2017; Chapman et al., 2019). Although the aforementioned reviews discussed the use of MIR and NIR spectrometry as a tool to predict milk and meat traits, very little attention has been given to analytical methods and validation strategies employed in analyzing such spectral data. Thus, complementary to the previous reviews, the objectives of this review are: to provide a recent update on the use of MIR and NIR techniques as tools to predict several novel complex traits in livestock system, with an emphasis in dairy and beef cattle; and review and discuss the analytical methods employed on spectral data to improve predictive ability, the different approaches used to reduce data dimensionality, and the impact of validation strategies on the prediction quality.
For this review, research articles published in peer-reviewed journals were retrieved from Web of Science using the keywords or the random combination of keywords presented in Table 1. Initially, a total of 348 papers published until May 2020 was found. The papers using NIR or MIR were selected based on the phenotype of interest (e.g., milk composition, feed intake, energy balance, methane emission, fertility, health status, and meat quality traits), and from the 348 studies, only 113 were included in this review. Studies using pre-calibrated or pre-trained models, provided by a company or third party, were not considered in this review. The coefficient of determination (R2) was used as an indicator of prediction quality for continuous variables. Some authors used the correlation coefficient as one of the metrics to report the model prediction quality. As such, the correlation coefficients were converted to R2 in order to have a single statistical metric for model evaluation. Although we have adopted R2 as metric to evaluate prediction quality in this review, due to the number of phenotypes and studies, we also recognize that other important metrics, such as Root Mean Squared Error and Mean Absolute Error, must be considered to better evaluate prediction quality, once R2 could be inflated by one sample or it could be very sensitive to the range of the variable of interest. For discrete distributed traits, the metric for prediction quality, reviewed in the published papers, was the overall accuracy. Thus, only the R2 or accuracy reported by the author in the validation set (internal or external) were considered in this review paper. The validation strategy employed by each author (i.e., data-splitting, leave-one-out cross, or k-folds cross-validation) was reviewed and it is presented in the tables, along with the information of prediction quality for each respective phenotype. However, some papers reviewed here did not completely describe the validation strategy adopted; therefore, it was not reported in the tables of this review. More information related to the compiled validation strategies is presented in the section “Validation Strategies.”
Over the past several years, many studies have investigated the effectiveness of NIR and MIR to predict novel complex phenotypes in dairy and beef cattle, as shown in Figure 1. Since De Marchi et al. (2014) wrote a review paper on milk MIR spectrometry, there has been an exponential increase in the number of studies using milk MIR spectral data to predict a range of complex traits. Overall, these studies include the direct quantification of compounds present in milk (e.g., milk fatty acids and protein profile) as well as the prediction of traits linked to milk spectra (e.g., health status, feed intake, methane emission, fertility, and energy balance). The use of NIR spectrometry to assess meat chemical composition and quality traits was reviewed for different species including beef, chicken, and pork by many authors (Prevolnik et al., 2004; Prieto et al., 2009a, 2017; Dixit et al., 2017; Chapman et al., 2019). The authors have stated that NIR is capable of measuring meat chemical composition and quality associated traits in different species, including beef cattle. However, the interest in using NIR technology as an alternative to predict novel traits in beef cattle since the first review paper (Prevolnik et al., 2004) has been lower than for using MIR spectrometry, based on the amount of paper using each method compiled for this current review (Figure 1). The number of studies developed in the last years using MIR and NIR techniques highlights the growing interest by the scientific community and livestock industry in this topic. Indeed, several novel phenotypes have been recently generated using both MIR and NIR techniques in dairy and beef cattle and they will be covered throughout this review section.
Figure 1. Published papers retrieved from Web of Science based on the combination of keywords presented in Table 1. Scientific papers published up to May 2020.
Beyond the nutritional meaningful for human, milk composition (e.g., protein, fat, lactose, and minerals) has direct implications on the sensory and technological properties of milk products as well as on the economic value of the milk and milk products (Soyeurt et al., 2009; Bastin et al., 2011; Bonfatti et al., 2011; Gengler et al., 2016; Fleming et al., 2017). Thus, over the last few years, efforts have been made by scientists and the dairy industry to quantify milk composition using modern high-throughput phenotyping techniques such as MIR spectrometry. Indeed, milk recording schemes worldwide have used MIR technique to measure total fat, protein, casein, lactose, and urea contents, which is quick and inexpensive when compared with gold standard methods (De Marchi et al., 2014). Given the promising and availability of milk spectra per cow per milking, several studies have reported that the major milk fatty acids (FA) can also be predicted using spectra data (Tables 2, 3).
Table 2. Number of samples (N) and coefficient of determination in the validation set for the milk fatty acids predicted from mid-infrared spectrometry using partial least square methodology in dairy cattle.
Table 3. Coefficient of determination in the validation set for the milk fatty acids predicted from mid-infrared in dairy cattle*.
For FA with less than 16 carbons (C4:0; C6:0; C8:0; C10:0; C12:0; and C14:0) the R2 reported varied from 0.37 to 0.97 in the validation set (Table 2). The feasibility of milk MIR spectra to predict FA with 16 carbons (C16:0 and C16:1) was investigated by several authors. The R2 observed in the validation sets were between the range of 0.33 and 0.95 (Table 2). For the FA with more than 16 carbons (C17:0; C18:0; C18:1 cis-9; C18:2 cis-9, trans-11; C18:2 cis-9, cis-12; and C18:3 cis-9, cis-12, cis-15) the R2 reported in the validation set varied from 0.07 to 0.95 (Table 3). The sums of saturated and monounsaturated FA were predicted with precision (R2) higher than 0.80, whereas for the polyunsaturated FA, the R2 varied from 0.38 to 0.81 in the validation set (Table 3). In general, FA with larger proportion (% of total FA) in the milk (e.g., C14:0, C16:0, and C18:1) presented greater R2 (e.g., C14:0, C16:0, and C18:1), when compared to milk FA of small individual proportion in milk (e.g., C17:0).
Studies have also investigated the effectiveness of using milk spectra data as a potential predictor of total protein, casein, and whey, as well as the individual caseins and whey proteins (Table 4). The R2 in the validation set for total protein and casein were greater than 0.70, except Sørensen et al. (2003); De Marchi et al. (2009), Rutten et al. (2011), and McDermott et al. (2016) that observed R2 in a range of 0.25–0.58. The R2 for total whey varied from 0.42 (McDermott et al., 2016) to 0.69 (Niero et al., 2016) in the validation set. For the individual caseins αS1-CN, αS2-CN, β-CN, and κ-CN R2 in a range of 0.18 (β-CN; Rutten et al., 2011) to 0.78 (αS1-CN; Ferrand et al., 2012) were reported in the validation set. The α-LA whey protein fraction presented the lowest prediction R2, varying from 0.06 (Eskildsen et al., 2016) to 0.48 (Ferrand et al., 2012), compared with β-LG where the prediction R2 were in a range of 0.34 (Eskildsen et al., 2016) to 0.64 (Bonfatti et al., 2011) in the validation set.
Table 4. Number of samples (N) and coefficient of determination in the validation set for the major protein content predicted from milk spectra using partial least square methodology in dairy cattle.
The effectiveness of milk spectra data to predict mineral composition in dairy cattle has also been investigated. The prediction quality reported in the validation set for Ca, Mg, Na, and P is presented in Table 5. Model performances were satisfactory to predict Ca and P (R2 > 0.67) in Soyeurt et al. (2009); Toffanin et al. (2015), Visentin et al. (2016), and Franzoi et al. (2019). But the same minerals were poorly predicted (R2 < 0.55) by Gottardo et al. (2015); Bonfatti et al. (2016), Malacarne et al. (2018), and Fleming et al. (2019). For K, Mg, and Na the R2 reported by the authors varied from 0.25 (Malacarne et al., 2018) to 0.75 (Franzoi et al., 2019) in the validation set.
Table 5. Number of samples (N) and coefficient of determination in the validation set for mineral contents using partial least square methodology.
Divergences in the predictability reported might be related to the different gold standard methodologies used in the reference data, population studied, and the sample size (De Marchi et al., 2014). Overall, the R2 observed in the published papers reviewed here highlight the potential of milk spectra data as a predictor of milk FA, proteins, and minerals. These studies also underline the need for future work using robust analytical data mining techniques and large datasets as a way to improve the model performance for phenotypes that have been inaccurate predicted. The possibility of more frequent predictions of such phenotypes could potentially create discoveries in different areas of animal science, such as genetics/genomics, nutrition, physiology, and reproduction. For example, some authors have demonstrated that milk fatty acids can be good predictors of plasma non-esterified fatty acids concentration (Mann et al., 2016; Dórea et al., 2017), which is an important phenotype associated with negative energy balance in lactating dairy cows. Additionally, such predictions can be used as a powerful tool to improve management decisions on livestock operations.
Given the economic impact of animal feed costs on farmer’s profitability, feed efficiency has been widely discussed as a key phenotype to be included in the selection indexes and for management decisions on livestock operations (Berry and Crowley, 2013; Berry, 2015; Seymour et al., 2019). Selecting animal for feed efficiency is highly attractive, but the practical implementation might be challenging, primarily because individual feed intake records on a large-scale are unavailable to date, and secondly some aspect of production, such as milk output or body weight, and energy sinks including maintenance, need to be accounted to determine individual feed efficiency (Berry and Crowley, 2013; Connor, 2015). Therefore, the use of NIR and MIR spectrometry has been explored as a potential tool to predict traits related to feed efficiency in beef and dairy cattle, as shown in Tables 6, 7. Seven studies evaluated the use of fecal NIR on fecal samples to predict organic or dry matter intake, reporting R2 ranging from 0.44 (Huntington et al., 2011) to 0.98 (Decruyenaere et al., 2004) in the validation set (Table 6). From the studies reviewed, only one study reported the use of feed (grass NIR) to predict dry matter intake, in which the R2 reported in the validation set was 0.71. It is important to point out that the results have not always been consistent, and few studies have compiled data sets of sufficient size to generate robust and accurate prediction equations. Furthermore, the use of NIR spectrometry on grab fecal and grass samples requires preparation and pretreatment, which is laborious, time-consuming, and may not be applicable on a large-scale.
Table 6. Number of samples (N), and coefficient of determination in the validation set (R2) for the prediction of dry matter intake (DMI) and organic matter intake (OMI) traits using grass near-infrared (G-NIR) and fecal near-infrared (F-NIR) spectrometry §.
Table 7. Number of samples (N) and coefficient of determination (R2) for dry matter intake (DMI), residual feed efficiency (RFI), effective energy intake (EEI), net energy intake (NEI), and energy intake (EI) traits using milk mid-infrared spectrometry in dairy cattle.
Due to the difficulties in utilizing fecal or grass samples with NIR to predict intake, the value of milk MIR spectrometry for prediction of feed efficiency has also been evaluated (Table 7). As many milk recording schemes globally already use MIR spectrometry to predict protein, fat, casein, lactose, and urea contents (De Marchi et al., 2014), they can be a useful source of information on large-scale operations. The majority of researchers have aimed to predict dry matter intake, though some have also evaluated predictions for residual feed intake, net energy intake, and effective energy intake. For dry matter intake, the R2 in the validation set was in a range of 0.29 (Wallén et al., 2018) to 0.77 (Shetty et al., 2017b). The studies evaluating the use of milk spectra to predict effective energy intake reported R2 varying from 0.49 (McParland et al., 2014) to 0.74 (McParland et al., 2011). McParland et al. (2015) observed R2 of 0.56 for energy intake in the validation set. McParland et al. (2014) and Shetty et al. (2017b) reported R2 of 0.36 and 0.46, respectively, to predicted residual feed intake from MIR data in the validation set. The majority of the studies observed that combining MIR data with other animal-level variables, such as milk yield, body weight, and feeding behavior resulted in greater prediction precision and accuracy compared to predictions based only on MIR data. The reviewed studies suggest that milk MIR spectra data is a promising tool to predict indicator traits for feed efficiency in dairy cattle. Such a novel source of information has the potential to bring new insights into management decisions and breeding programs.
Dairy cows in early lactation are under high energy demand to meet their requirements for lactation and often energy intake is unable to meet a cow’s requirements, leading animals to enter in a period of negative energy balance (Collard et al., 2000; de Vries and Veerkamp, 2000; McParland et al., 2012). Effective and accurate early assessment of a cow’s energy balance could be useful for management strategies, mitigating the costs associated with detrimental effects of negative energy balance, and future genetic selection (McParland et al., 2012; Grelet et al., 2019). Energy balance has been estimated in dairy cows through alternative methods that are mostly based on the difference between energy intake and energy output or considering the change in body reserves (Coffey et al., 2001; Friggens et al., 2007; Banos and Coffey, 2010). The drawback to these methods is that they require regular measurements of energy intake, body condition score, and body weight, which are expensive to collect on a sufficiently large number of animals, not well suited to assess short-term changes, and vary with intake respectively (McParland et al., 2012). Moving forward to high throughput phenotyping, milk spectra have been used as a potential tool to predict energy balance (Table 8). McParland et al. (2011; 2012; 2014; 2015) reported R2 in a range of 0.29–0.56 in the validation set using evening milk spectra data. A moderate R2 (0.60) was reported by Smith et al. (2019) to predict energy balance in the validation set, whereas Ho et al. (2020) observed low R2 (0.48), which according to the authors could be due to the small dataset used when compared with the previous studies. The predictive ability observed by the authors highlights the potential of milk spectra data to predict herd or individual energy status level; however, more efforts are needed to improve the prediction quality. Furthermore, the models used only require milk spectra and yield, which are both routinely generated during milk recording. Therefore, farmers could have access to the individual animal energy status at the time of milking without additional cost.
Table 8. Number of samples (N) and coefficient of determination (R2) in the validation for energy balance trait using milk mid-infrared spectrometry in dairy cattle§.
Strategies to predict enteric methane emission (CH4) have been widely explored by different research groups worldwide. Such interest is usually driven by concerns regarding the carbon footprint and lower feed efficiency due to energy losses in CH4 (Johnson and Johnson, 1995). Mitigating CH4 emissions may improve the livestock systems’ sustainability and profitability (Knapp et al., 2014). However, the majority of the classical methods used to quantify CH4 in the papers reviewed here (i.e., respiration chamber, sulfur hexafluoride tracer, and sniffer systems) are difficult, expensive, or not feasible to carry out on large scale operations (Hammond et al., 2016; Patra, 2016; Negussie et al., 2017). Several indirect measurements (e.g., feed intake, volatile fatty acids, milk FA, body weight, hindgut, feces, among others) have been proposed as a predictor of CH4 emission, in which milk FA have been stated as a promising CH4 proxy in dairy cattle (Negussie et al., 2017). Many studies have investigated the correlation between different milk FA, quantified using gas chromatography, and enteric CH4 production (Chilliard et al., 2009; Dijkstra et al., 2011; van Lingen et al., 2014; Rico et al., 2016). Based on the moderate to high correlations (0.50–0.80) observed by these authors, milk FA can be considered a potential indicator of individual animal enteric CH4 emissions. Since milk FA can be predicted from milk spectra, as previously discussed in section “Milk Composition,” several researchers have investigated the feasibility of using milk spectra data to predict the volume of CH4 eructed daily by a dairy cow (Table 9). Overall, the R2 reported by the papers reviewed here varied from 0.01 (Wang and Bovenhuis, 2019) to 0.79 (Dehareng et al., 2012) in the validation set. The variation in the predictive ability across studies can be partially explained by the different methods used to determine CH4 emission (e.g., respiration chambers, sulfur hexafluoride tracer, and sniffer method). Based on the prediction quality presented by some of the authors in experimental settings, milk spectra data has the potential to predict enteric CH4 emissions (Vanlierde et al., 2015). The validity of spectra data to predict CH4 emissions under conditions more similar to a commercial herd was only partly confirmed only by Shetty et al. (2017a). In beef cattle, the majority of studies have measured methane emission using classical direct methods, but to the best of our knowledge, no studies have attempted to predict methane emission indirectly using infrared spectrometry data.
Table 9. Number of samples (N) and coefficient of determination in validation set (R2) for methane emission trait predicted from milk mid-infrared spectra data.
Although fertility is a non-yield trait, it is the key to overall profitability in cattle farming as poor fertility increases the replacement rate due to involuntary culling, costs related to fertility treatments, and multiple inseminations, which directly affect animal production (Boichard, 1990; Dekkers, 1991; González-Recio et al., 2004; Berry et al., 2014; Pravia et al., 2014; Kaniyamattam et al., 2016). Since fertility traits are difficult and expensive to measure, early indicator or associated traits (e.g., body condition score, body weight, metabolic and endocrine blood traits, and milk composition) can be used either to enhance indirect genetic improvement of fertility or for reproductive management decisions (Berry et al., 2003; Moraes et al., 2007; Friggens et al., 2008; Diskin and Kenny, 2014). Hempstalk et al. (2015) developed a biological-based ML model to predict the likelihood of conception success given the herd- and cow-specific attributes, with particular attention to the use of milk spectral data. The area under the curve reported by the authors in the external validation set varied from 0.49 to 0.60 across different ML algorithms. However, the inclusion of milk spectra, compared with the same model using only non-MIR data (e.g., days in milk, milk yield, number of inseminations, breeding values, among others) in the ML models, did not improve the accuracy of predicting the likelihood of conception to an insemination. The prediction accuracies of pregnancy status using milk spectra data were also assessed by Toledo-Alvarado et al. (2018). The area under the curve across breed had similar patterns averaging 0.61 for Holsteins and 0.64 for Alpine Grey cows in the cross-validation. The authors concluded that pregnant versus open cows post insemination could be discriminated with promising accuracy using milk spectra, parity, and days in milk. Ho et al. (2019) reported that milk spectra from early lactation cow together with other on-farm data (e.g., days in milk, days from calving to insemination, calving age, milk yield, genotypes, among others) could be used to classify cows that conceived at first insemination or did not conceive within the breading season with reasonable accuracy, based on the area under the curve (0.75), in herd-by-herd external validation. Delhez et al. (2020) observed that milk spectra recorded after 150 days of pregnancy was promising to predict the pregnancy status in Holstein, with the area under the curve around 0.76 in cow-independent external validation. More efforts need to be made to investigate the reliability of milk spectra to predict fertility traits since the accuracies observed to date are not high and the number of studies is very low, although recent. Nevertheless, these studies provide new insights into novel phenotypes that can be used indirectly to improve fertility, especially in dairy cattle, which could become an important tool for management decisions on dairy farms.
Several metabolic disorders and diseases, such as ketosis, mastitis, milk fever, lameness, displaced abomasum, metritis, retained placenta, and cystic ovaries have important impacts on profitability and animal welfare (Kelton et al., 1998; Friggens et al., 2007; McArt et al., 2015; Jamrozik et al., 2016). To mitigate herd losses, producer-recorded events have been used for management decisions at farmer-level and genetic selection (Jamrozik et al., 2013; Miglior et al., 2014; Luke et al., 2019). However, the bottleneck relies on the difficulty of routinely collecting high-quality direct phenotypes on farms (Egger-Danner et al., 2015). Subclinical hyperketonemia or ketosis is one of the most frequent diseases in dairy cattle and it is characterized by increased concentrations of the ketone bodies acetoacetate, β-hydroxybutyrate (BHB), and acetone in blood, milk, and urine (Hansen, 1999). Additionally, blood metabolites such as glucose, non-esterified fatty acids (NEFA), blood urea nitrogen (BUN), and insulin-like growth factor 1 (IGF-1), and glutamic oxaloacetic transaminase (GOT) might also be used as indicators of metabolic status in dairy cows (Fenwick et al., 2008; Benedet et al., 2019; Grelet et al., 2019). Blood metabolic profile testing is the gold standard for diagnosis, however, it is invasive, logistically challenging, and costly (Luke et al., 2019). MIR spectrometry has been explored as possible high-throughput phenotyping technology to predict BHB concentration in blood or milk, and acetone in milk (Table 10). Within the published papers de Roos et al. (2007) and Grelet et al. (2016) predicted the concentration of BHB in milk and the R2 reported by the authors in the validation was 0.62 and 0.63, respectively. Seven published papers evaluated the use of milk spectra as a predictor of BHB in serum and the R2 varied from 0.40 (Belay et al., 2017) to 0.70 (Grelet et al., 2019) in the validation set. Although few studies have focused on predicting acetone in milk from milk spectra, the R2 observed by Hansen (1999) and de Roos et al. (2007) were higher than 0.70, except Grelet et al. (2016) which observed R2 of 0.67 in the validation set. Heuer et al. (2001) observed a standard error of cross-validation, the prediction quality metric used by the authors, of 0.24 to predict acetone in milk. Likewise, the feasibility of using spectral data to predict glucose, NEFA, BUN, and IGF-1 were also investigated in this review (Table 10). The R2 reported by the authors varied between 0.20 (glucose; Benedet et al., 2019) to 0.61 (IGF-1; Grelet et al., 2019) in the validation set.
Table 10. Number of samples (N) and coefficient of determination (R2) in the validation set for β-hydroxybutyrate (BHB), acetone (Ac), non-esterified fatty acids (NEFA), blood urea nitrogen (BUN), glucose (Glu), glutamic oxaloacetic transaminase (GOT), and insuline-like growth factor 1 (IGF-1) using milk mid-infrared spectrometry in dairy cattle.
Mastitis is the most common and costly contagious disease in dairy cattle characterized as an inflammation of the mammary gland and udder tissue. To the best of our knowledge, only Rienesl et al. (2019) investigated the possibility of using milk spectra to predict mastitis, which reported satisfactory accuracy (0.68) in the validation data set (Table 11). Mineur et al. (2017) and Bonfatti et al. (2020) investigated the ability of milk spectra data as a predictor of lameness and the predictions were poor to be employed as an on-farm tool to detect lameness in cows (Table 11). Based on the results presented by the reviewed papers, milk spectra might be useful to predict the concentration of BHB in serum or milk, acetone on milk, and mastitis occurrence in dairy cattle. However, more studies using larger and more diverse calibration data sets are needed, especially across countries, to improve the prediction quality before models can be used for on-farm management or genetic selection purposes.
Table 11. Number of samples (N), accuracy (Acc), sensitive (Sen), and specificity (Spe) in the validation set for mastitis (Mas) and lameness (Lam) traits using milk mid-infrared spectrometry using partial least square in dairy cattle.
Meat quality is a complex concept that involves many attributes such as tenderness, juiciness, flavor, marbling, color, and shelf life (Williams, 2008). Meat tenderness is one of the most important attributes affecting consumers’ acceptability, followed by fat content and visual attributes (Shackelford et al., 2001; Liu et al., 2003; Williams, 2008). Considering the increasing demands for meat and consumers willing to pay higher prices for certified, high-quality meat products, there is a growing interest by the beef meat chain to accurately assess meat quality traits (Andrés et al., 2008; Prieto et al., 2008). To date, meat quality traits have been measured using physical methods, which are time-consuming, expensive, destructive (depreciating the value of the carcass), and unsuitable to perform individually in large-scale (Su et al., 2018; Chapman et al., 2019). To satisfy the requirements of the modern meat industry, NIR spectrometry has been stated as an alternative tool for high throughput phenotyping meat quality traits because it is considered an accurate, fast, non-invasive and non-destructive technique with great potential for in-line application (Prevolnik et al., 2004; Prieto et al., 2009a; Chapman et al., 2019). The feasibility and robustness of NIR technique to predict meat quality traits in cattle have been investigated by several researchers (Tables 12–14). Here our focus will be on meat tenderness, intramuscular fat content, meat color, and cooking loss traits, since such attributes impact consumers’ satisfaction. Meat quality traits can be measured using different methodologies, but in our review, such traits were summarized regardless of the methodology applied. The R2 observed in the reviewed papers for meat tenderness varied from 0.12 (De Marchi et al., 2007) to 0.81 (Prieto et al., 2014) in the validation set (Table 12). The R2 for intramuscular fat content varied from 0.02 (Magalhães et al., 2018) to 0.99 (Su et al., 2014) in the validation set (Table 13). For color traits (L∗, a∗, and b∗), the R2 in the validation set (Table 14) were in a range of 0.16 (Magalhães et al., 2018) to 0.93 (Zhang et al., 2015). The R2 observed for cooking losses varied from 0.001 (Prieto et al., 2008) to 0.61 (Zhang et al., 2015) in the validation set (Table 14). Overall, NIR spectrometry has shown great potential to assess meat quality traits within different breeds. Furthermore, meat quality traits can be generated directly on the raw beef under slaughterhouses conditions as the NIR technique may not require sample pre-preparation. Nevertheless, further research needs to be conducted to validate the models across breeds and use modern data mining approaches to improve prediction quality.
Table 12. Number of samples (N) and coefficient of determination (R2) in the validation set for meat tenderness trait predicted from near-infrared spectrometry in cattle.
Table 13. Number of samples (N) and coefficient of determination (R2) in the validation set for intramuscular fat content predicted from near-infrared spectrometry in cattle.
Table 14. Number of samples (N) and coefficient of determination (R2) in the validation set for L∗ (R2L^*), a∗ (R2a^*), and b∗ (R2b^*) meat color, and cooking losses (R2CL) traits predicted from near-infrared spectrometry in cattle.
Frequently, the main goal of using infrared spectrometry technology in the livestock industry is the development of predictive models to determine the content of specific compounds present in products such as milk, meat, and feedstuffs. However, many compounds present in such products are highly correlated with phenotypes that are difficult to measure in commercial and research settings, such as feed intake, methane emission, energy balance, methane emission, fertility, metabolic diseases, and meat quality traits, as previously discussed. In this context, several research studies have attempted to develop predictive models to predict such complex phenotypes for management decisions or breeding purposes. However, we have noted that factors such as the analytical method chosen to develop the predictive models and the cross-validation strategy used to evaluate the analytical approaches are not deeply discussed in the research studies involving livestock data. If not utilized properly, those two factors can result in (a) poor predictions due to the lack of ability of certain models to capture complex relationships between explanatory and response variables and (b) overoptimistic prediction quality due to high data dependency occurring between training and validation dataset. To developed robust predictive models using spectral data the following three steps should be followed: (1) spectra pretreatment, to remove noise or non-informative wavenumbers, (2) model training (or algorithm training), in which analytical techniques are used to assess the set of coefficients, number of latent variables, or hyperparameters, and (3) model validation, in which an independent dataset is used to evaluate the predictive ability of the model developed using the training dataset. These three main steps will be discussed throughout this review section.
Infrared spectra data comprise signals related to compounds present in the biological sample as well as non-informative signals coming from background, high-frequency noise, baseline shift, and overlapping bands (Rinnan et al., 2009a). Therefore, preprocessing spectral data is a common and crucial strategy that helps to mitigate such undesirable signals present in the raw data, maximizing the relationship between the infrared spectrum and the target phenotype (Rinnan et al., 2009a; De Marchi et al., 2014; McParland and Berry, 2016). Furthermore, preprocessing the spectra data prior to fit the calibration model is used in attempting to obtain robust prediction models and to restrict the insertion of bias into the model. However, applying unsuitable or a high stringent preprocessing strategy might remove important information from the biological sample (Rinnan et al., 2009a). Spectra preprocessing are commonly performed using mathematical pretreatment techniques or variable selection approach. The main mathematical pretreatment techniques used in the reviewed papers to mitigate signal noise can be divided into two groups, scatter-correction methods (e.g., multiplicative scatter correction, standard normal variate, and orthogonal scatter correction) and spectral derivatives (e.g., Savitzky-Golay polynomial derivative). Multiplicative scatter correction (MSC) is used to remove physical effects including particle size and surface blaze from the spectra, which do not carry any chemical or physical information, by correcting differences in the baseline and the trend (Martens et al., 1983). Standard normal variate (SNV) aims to remove the multiplicative effects of scatter and particle size, giving the sample a unit standard deviation (Barnes et al., 1989). Orthogonal scatter correction (OSC) eliminates the parts linearly unrelated (orthogonal) to the response variable (Wold et al., 1998). Savitzky-Golay (SG) first derivative is used to improve the spectra resolution by eliminating constant baseline, whereas the second derivative eliminates both baseline and linear trend (Savitzky and Golay, 1964). More details and theory overview about the mathematical pretreatment techniques employed on NIR and MIR spectra can be found in Rinnan et al. (2009a, b). From the 113 published papers reviewed here, researchers have used spectra data without pretreatment (50), SG first derivative (28), SG second derivative (10), MSC (7), SNV (5), and OSC (2). Eleven authors did not report if some mathematical pretreatment was applied to the spectra data. Several authors also used the combination of more than one pretreatment strategy (e.g., SG first derivative + MSC; SG second derivative + SNV; and SG first derivative + SG second derivative + MSC). Some authors have reported an increase in the prediction quality using pretreated spectra data (Heuer et al., 2001; Rødbotten et al., 2001; Soyeurt et al., 2011; De Marchi et al., 2011), whereas others have observed that the pretreatment failed to improve prediction accuracies (McParland et al., 2011; Cecchinato et al., 2012; Dehareng et al., 2012; Shetty et al., 2017a; Cafferky et al., 2020).
Analytical methods commonly employed on spectra data are suitable to reduce the full-spectrum to a few latent variables or perform some type of regularization (e.g., ridge and lasso). However, even performing such dimensionality reduction, they may also be penalized by noise or non-informative wavenumbers. Furthermore, the number of wavenumbers in infrared spectrometry datasets usually outnumbers the sample size. For example, a dataset containing 30 samples with their respective spectra data (1,060 wavenumbers). Note that the number of parameters p (1,060 wavenumbers) is greater than the number of observations n (30 samples). In such situations, the use of least squares is not appropriate since it will yield a set of coefficient estimates that result in a perfect fit to the data and the residuals will be zero (James et al., 2013). This phenomenon is a concern in data analysis because such perfect fit will potentially lead to overfitting the data (Hastie et al., 2009). Variable selection, therefore, is an important strategy used prior to the model calibration to reduce the data dimensionality by selecting a subset of relevant features from the original space to improve the model robustness and reduce the model complexity (Koljonen et al., 2008; Rinnan et al., 2009a). Although several authors removed the water noise wavenumbers, here we are only taking into account studies that performed variable selection based on a mathematical or statistical approach. Thus, only nine out of 113 published papers reviewed here performed variable selection prior to the calibration model. Variable selection was employed using a genetic algorithm (GA), uninformative variable elimination (UVE), variable importance for projection (VIP), and coefficient of variation (CV) combined with Markov Blanket (MB) techniques by 4, 3, 2, and 1 studies, respectively. Briefly, GA is an algorithm based on the biological evolution theory and natural selection and the main idea is to find within the set of predictor variables the ones that best fitted the model. The best predictor variables need to show high “fitness” and probability to “survive” to be included in the subsequent variable sets used for model refit. An iterative process is performed until the GA has selected the best predictor variable set or the best combination of them (Leardi et al., 1992). UVE approach adds artificial noise predictor variables, multiplied by a constant close to zero in order to eliminate any possible interaction with the original variables, to the reference dataset before fitting the model. The wavenumbers (original variables) that play a less important role in the model (based on the root mean square error, for example) than the random variables are considered uninformative and eliminated from the dataset before the procedure is repeated. The iterative process is performed until the stop criterion is reached (Centner and Massart, 1996). Using the VIP technique, one calculates a coefficient v that represents the importance or influence of each predictor variable on the response variable. Thus, predictor variables with a v < user-defined threshold u (v < 1, for example) are less relevant to fit the model and can be eliminated (Wold et al., 1993). CV is a well-known standardized measure of dispersion and it is used to eliminate wavenumbers that lack variability between samples. The MB notion is widely used in Bayesian Network and is defined as: for a node (target variable), its MB is the minimal set of parents, children, and spouses (wavenumbers) that best represents the node (Pearl, 1988). Studies that performed variable selection prior to the calibration step have reported an improvement in the quality of the predictions for different phenotypes and analytical approaches (Wu et al., 2009, 2012; Gottardo et al., 2015, 2016; Niero et al., 2016; Dórea et al., 2018). Although the authors have observed that spectra pretreatment and variable selection improved the predictions accuracies, there is no consensus in the literature regarding which situations such techniques will effectively result in better prediction ability, especially using larger datasets. Therefore, the effect of spectra data pretreatment and variable selection on the prediction quality should be more deeply investigated. Furthermore, as pointed out by De Marchi et al. (2014) and observed in the published papers reviewed here, the authors usually report only the precision and accuracy for the best model, while information regarding other models is not shown and discussed for full comparison.
From the 113 published papers retrieved from Web of Science, Partial Least Squares (PLS) was the most used statistical approach (101 papers) for the development of predictive equations, when compared to other machine learning (ML) methods. The simple implementation makes PLS a well-established and widely used methodology to generate novel complex traits from infrared spectrometry data in different fields. PLS is a dimension reduction technique suitable when the number of predictors is greater than the number of observations (e.g., infrared spectra data) as well as when strong collinearity exists between predictor variables, i.e., some wavenumbers can be rewritten as a linear function of others (Martens and Naes, 1987). Briefly, PLS maximizes the covariance between the predictor variables and the response variable resulting in a small set of components (latent variables), commonly called factors, that are used to predict target phenotypes in a new dataset (Martens and Jensen, 1982; Martens and Naes, 1987). Multiple linear regression (MLR) has also been used (Mitsumoto et al., 1991; Shackelford et al., 2005) or compared to other methodology (Pralle et al., 2018; Müller et al., 2019) to predict complex phenotypes using spectra data. MLR solves a number of simultaneous equations exploring the linear relationship between several explanatory variables (i.e., wavenumbers) and the continuous response variable (Hastie et al., 2009). Despite the power of such statistical method, Pralle et al. (2018) did not observe improvement in the prediction quality. In contrast, Müller et al. (2019) reported improvement when MLR was implemented compared with other methodologies such as PLS. In addition, the bottleneck of MLR methodology is that its implementation will often face the problem that the number of parameters is greater than the number of samples (Hastie et al., 2009). The PLS method to date has succeeded in predicting some complex traits with high accuracies, whereas for other traits, the prediction quality was poor, as previously reported in section “Complex Traits Predicted by Infrared Spectrometry Data.” This fact highlights the need for more research studies evaluating other analytical strategies able to deal with missing data, non-linear relationships between response and explanatory variables, and high-dimensional data to improve the prediction quality of complex phenotypes. Recently, Bayesian and ML techniques have been implemented to predict a range of phenotypes in livestock; however, it is at a small proportion compared with the number of studies using PLS. Bayesian models have been developed for high-dimensional regression and they are widely used in the context of genomic prediction (Meuwissen et al., 2001). In addition to the possibility of assigning prior information for the marker effects, here substituted by the wavenumbers’ effects, Bayesian methods are also able to perform estimate shrinkage and variable selection. For genomic prediction, Bayesian methods may have greater predictive power than dimension-reduction methods (Meuwissen et al., 2001; de los Campos et al., 2013). Ferragina et al. (2015) reported that Bayes B approach, which is one of the many Bayesian methods available, outperformed PLS methods for predicting milk components and technological properties using infrared spectral data and Bayes B was able to select a small subset of important wavenumbers. Based on Ferragina et al. (2015) finds, Bittante and Cipolat-Gotet (2018); Toledo-Alvarado et al. (2018), and Savoia et al. (2020) also used Bayes B methodology to predict complex phenotypes using spectra data. ML techniques such as Support Vector Machine (SVM) and Artificial Neural Networks (ANN) have been tested as an alternative to the traditional PLS method, because of their ability to search in a high-dimensional space of predictor variables for features that best describe the response variable, with the ability to self-learn. Additionally, such methods can better model the complex relationships (e.g., non-linear and interactions) between the input variables and the response outcome, which could improve the prediction quality (Gianola et al., 2011). Indeed, the authors that used SVM or ANN approaches have reported an increase in prediction quality compared with PLS method (Hempstalk et al., 2015; Qiao et al., 2015; Dórea et al., 2018; Pralle et al., 2018; Grelet et al., 2020). Although ML methodologies are suitable to be implemented in the high-dimensional space of predictor variables, this approach tends to easily overfit, in general because of small datasets and noisy data (Hempstalk et al., 2015). Overfitting is a recurrent issue in ML methods, such as in ANN, and it is clearly identified by high prediction accuracy in the training dataset but very poor in the validation dataset. For such methods, it is very important to perform unbiased validation strategy, therefore ensuring maximum possible data independency between training and validation would be ideal to unbiased predictions (Roberts et al., 2017). Additionally, ML techniques often require an extensive search for hyperparameters (e.g., number of neurons, number of layers, learning rate, among others) before training the algorithms to perform final prediction (Bengio, 2012). Such search is critical in order to define the network architecture, which may detrimentally affect prediction quality if not appropriate (Bengio, 2012). Based on the comparisons between traditional (PLS) and advanced analytical methods (Bayesian and ML) found in the reviewed papers, the use of advanced techniques resulted in improved prediction accuracy for some complex phenotypes. However, the prediction quality is influenced by many factors, including the trait to be predicted (e.g., qualitative or quantitative), the quality of the reference data (observed data) set, the spectra quality, the spectra preprocessing, the sample size used to develop the prediction equations, and the validation strategies used for model development and validation (Karoui et al., 2010; Rutten et al., 2010; Ferragina et al., 2015; Bonfatti et al., 2017; Dórea et al., 2018). In addition, directly predicted traits (e.g., milk fat) usually has a significant signal in the spectra data, whereas indirectly predicted traits (e.g., feed efficiency and methane emission) the signal in the spectra is associated to complex traits through compounds found in the scanned products (milk or meat, for example) (Ferragina et al., 2015). The number of research studies implementing alternative methods to predict complex traits in livestock systems is small and, therefore, more investigation using a different analytical approach, sample size, data pretreatment, and variable selection, is important to shed light on the predictive analytics of complex phenotypes.
Infrared spectrometry data (MIR and NIR) have been stated as an important source of information to generate many novel complex phenotypes in dairy and beef cattle (Prevolnik et al., 2004; De Marchi et al., 2014; McParland and Berry, 2016; Gengler et al., 2016; Chapman et al., 2019). To predict such phenotypes, robust models or equations must be developed using a training dataset that represents the population variability. The approval that ensures prediction quality of the developed models is given through their performance when implemented in a validation dataset, ideally, an external dataset not previously utilized for model development. However, defining the external validation dataset is a non-trivial task in some cases, since the dependency between training and validation sets needs to be reduced as much as possible. The main reason for reducing such dependence between training and validation sets is the need to approximate prediction quality from model validation to real-life implementation, in which very little dependency between training and validation set will occur. In this review, we considered as an external validation set, all datasets in which some level of dependency between training and validation set was broken. For example, research trials using multiple herds where one herd or trial was removed from the dataset to validate the model. Although this is the desired validation strategy, in some situations, it is difficult to define an external validation set due to the hierarchical structure of the dataset. In such case or when datasets are small, internal validation is usually performed, which was the most used strategy in the papers reviewed here (67 out of 113). In this review, we considered internal validation the studies in which hierarchical structure in the dataset (e.g., country, herd, dietary effect, etc.) was not considered for data split strategies (holdout, leave-one-out, k-fold). Therefore, to create the training and internal validation datasets the reviewed studies used one of the data-splitting techniques: holdout (22 studies), leave-one-out cross-validation (20 studies), and k-fold cross-validation (25 studies). An example of each validation strategy technique used for model validation is depicted in Figure 2.
Figure 2. Example of validation strategies employed in the publish papers retrieved from Web of Science. (A) split-data or k-fold cross-validation, (B) leave-one-out cross-validations, and (C) leave-one-group-out cross-validation. Colors represent animals from different herds.
Briefly, in the holdout strategy (Figure 2A), given that animals are sourced in different herds, about 80% of the dataset is randomly used in the model training and the remaining 20% is used for model validation (Stone, 1974; Picard and Berk, 1990). When holdout is adopted, the test error rate can be highly variable depending on which sample is assigned in the training and validation set (Hastie et al., 2009). Leave-one-out cross-validation is an alternative to the holdout approach and involves splitting the dataset into two parts in which N – 1 observations are used to train the model and a single sample N is used to validate the model (Figure 2B). The splitting is iterated N times until all the observations were used in the validation and the model performance is averaged across the N validation sets. Thus, the test error in leave-one-out cross-validation is highly variable compared to holdout, because the training dataset contains almost the same number of observations (N – 1) as the entire dataset (Hastie et al., 2009). Leave-one-out is commonly used when the sample size is small and there is concern about the limited size of the calibration set (Gianola and Schön, 2016). The idea of leave-one-out cross-validation technique can also be adapted to perform leave-one-herd-out (e.g., group, farm, trial, year, among others), which can be a good strategy to reduce data dependence between training and validation dataset (Figure 2C). The k-fold cross-validation is performed by dividing the entire dataset into k disjoint sets of approximately equal size, usually randomly, in which k – 1 sets are used in the training and one set is used to validate the model. Such process is repeated k times until all k sets were used in the validation and the model performance is averaged across all k validation sets (Stone, 1974; Hastie et al., 2009). This process can be repeated many times, wherein each iteration different samples are assigned in both training and validation sets. Due to the larger validation dataset assigned in k-fold cross-validation as well as the test error averaged across the k different subsets, the test error is less sensitive to the partition of the dataset than in the leave-one-out cross-validation (Hastie et al., 2009). The drawback of the three procedures adopted to split the dataset, except for the leave-one-herd-out strategy, is that animals from the same herd or multiple records from the same animal will be present on the training and validation set, creating dependence between them.
Studies comparing different validation strategies using spectra data confirmed the hypothesis that prediction quality was inflated according to the split-data strategy employed to externally validate the model (Shetty et al., 2017b; Dórea et al., 2018; Lahart et al., 2019; Luke et al., 2019; Smith et al., 2019; Wang and Bovenhuis, 2019). Therefore, evaluating the fitted model using only internal validation is not recommended. The performance of the model fitting will be better than it should be, resulting in greater model precision and accuracy than if a true external validation is used, but the prediction accuracies in the external validation set are more realistic and quite often observed in practice. Several authors (32 out of the 112 papers reviewed here) reported that an independent dataset was used to externally validate the model’s performance. However, by reviewing the papers from the 32 studies only seventeen fully performed external model validation. In those studies, the authors validated the models’ performance using an external/independent dataset, which an entire farm, herd, trial, year, study, region, dataset, or batch was removed from the training dataset. The remaining studies (15) only assigned records by animal, individual records, or lactations either randomly or not from the entire dataset to external validate the model. Such strategy does not produce a good independent dataset to test the model against real-life implementation because animals from the same herd or group can be present on the training and validation set. Fourteen out of 112 papers did not report if model performance was evaluated, only stated that cross-validation was employed, or the procedure was not clearly described. Therefore, it is a good practice to use external validation (if large sample size is available), based on data from a different farm, herd, trial, year, region, or batch, before full deployment of such predictive models.
Important advances have been made in the nutrition, reproduction, management, and molecular breeding techniques of beef and dairy cattle in recent years. However, efficient and precise phenotyping remains a bottleneck and, therefore, modern high-throughput techniques should be developed, improved, and applied to take full advantage of the advancements performed in the different animal knowledge fields. Of the techniques currently available, this review summarized the applications of MIR and NIR spectrometry as a novel high-throughput phenotyping technique to generate complex phenotypes in dairy and beef cattle. Furthermore, it presented an overview, status update, and insights into the use of such techniques and the data mining strategies employed to predict the phenotypes of interest. The majority of studies compiled have demonstrated the capability and power of MIR and NIR technique to generate complex traits such as feed efficiency, methane emissions, energy balance, health, and meat quality from different biological samples routinely accessed, without additional cost and at the animal-level. Therefore, these phenotypes would be widely explored in dairy and beef cattle for on-farm decision-making, management, and breeding purpose. MIR and NIR spectrometry has important advantages compared to gold standard methods such as speed, low cost, non-invasive, non-destructive, and potential for in-line application; however, for the implementation of these high-throughput techniques into livestock operations, numerous issues regarding the modeling methodology must be considered. Few studies have used a large dataset as well as machine learning or Bayesian techniques to develop the calibrations models. Therefore, larger datasets and modern data mining approaches should be investigated to improve predictive ability and to confirm the existing calibration models.
TB and JD equally contributed direct and intellectual to the work and approved it for publication. Both authors contributed to the article and approved the submitted version.
The authors declare that the research was conducted in the absence of any commercial or financial relationships that could be construed as a potential conflict of interest.
Afseth, N. K., Martens, H., Randby, S., Gidskehaug, L., Narum, B., Jørgensen, K., et al. (2010). Predicting the fatty acid composition of milk: a comparison of two fourier transform infrared sampling techniques. Appl. Spectrosc. 64, 700–707. doi: 10.1366/000370210791666200
Agnewa, R. E., Park, R. S., Maynea, C. S., and Laidlaw, A. S. (2004). Potential of near infrared spectroscopy to predict the voluntary intake of grazed grass. Anim. Feed. Sci. Technol. 115, 169–178. doi: 10.1016/J.ANIFEEDSCI.2004.01.009
Andrés, S., Silva, A., Soares-Pereira, A. L., Martins, C., Bruno-Soares, A. M., and Murray, I. (2008). The use of visible and near infrared reflectance spectroscopy to predict beef M. longissimus thoracis et lumborum quality attributes. Meat Sci. 78, 217–224. doi: 10.1016/j.meatsci.2007.06.019
Banos, G., and Coffey, M. P. (2010). Genetic association between body energy measured throughout lactation and fertility in dairy cattle. Animal 4, 189–199. doi: 10.1017/S1751731109991182
Barnes, R. J., Dhanoa, M. S., and Lister, S. J. (1989). Standard normal variate transformation and detrending of near-infrared diffuse reflectance spectra. Appl. Spectrosc. 43, 772–777. doi: 10.1366/0003702894202201
Bastin, C., Gengler, N., and Soyeurt, H. (2011). Phenotypic and genetic variability of production traits and milk fatty acid contents across days in milk for Walloon Holstein first-parity cows. J. Dairy Sci. 94, 4152–4163. doi: 10.3168/jds.2010-4108
Belay, T. K., Dagnachew, B. S., Kowalski, Z. M., and Ådnøy, T. (2017). An attempt at predicting blood β-hydroxybutyrate from Fourier-transform mid-infrared spectra of milk using multivariate mixed models in Polish dairy cattle. J. Dairy Sci. 100, 6312–6326. doi: 10.3168/jds.2016-12252
Bell, M. J., and Tzimiropoulos, G. (2018). Novel monitoring systems to obtain dairy cattle phenotypes associated with sustainable production. Front. Sustain. Food Syst. 2:31. doi: 10.3389/fsufs.2018.00031
Benedet, A., Manuelian, C. L., Zidi, A., Penasa, M., and De Marchi, M. (2019). Invited review: β-hydroxybutyrate concentration in blood and milk and its associations with cow performance. Animal 13, 1676–1689. doi: 10.1017/S175173111900034X
Bengio, Y. (2012). “Practical recommendations for gradient-based training of deep architectures,” in Neural Networks: Tricks of the Trade, eds G. Montavon, G. B. Orr, and K.-R. Müller (Heidelberg: Springer), 437–478. doi: 10.1007/978-3-642-35289-8_26
Berry, D. P. (2015). Breeding the dairy cow of the future: What do we need? Anim. Prod. Sci. 55, 823–837. doi: 10.1071/AN14835
Berry, D. P., Buckley, F., Dillon, P., Evans, R. D., Rath, M., and Veerkamp, R. F. (2003). Genetic relationships among body condition score, body weight, milk yield, and fertility in dairy cows. J. Dairy Sci. 86, 2193–2204. doi: 10.3168/jds.S0022-0302(03)73809-0
Berry, D. P., and Crowley, J. J. (2013). Cell biology symposium: genetics of feed efficiency in dairy and beef cattle. J. Anim. Sci. 91, 1594–1613. doi: 10.2527/jas.2012-5862
Berry, D. P., Wall, E., and Pryce, J. E. (2014). Genetics and genomics of reproductive performance in dairy and beef cattle. Animal 8, 105–121. doi: 10.1017/S1751731114000743
Bittante, G., and Cipolat-Gotet, C. (2018). Direct and indirect predictions of enteric methane daily production, yield, and intensity per unit of milk and cheese, from fatty acids and milk Fourier-transform infrared spectra. J. Dairy Sci. 101, 7219–7235. doi: 10.3168/jds.2017-14289
Boichard, D. (1990). Estimation of the economic value of conception rate in dairy cattle. Livest. Prod. Sci. 24, 187–204. doi: 10.1016/0301-6226(90)90001-M
Boichard, D., and Brochard, M. (2012). New phenotypes for new breeding goals in dairy cattle. Animal 6, 544–550. doi: 10.1017/S1751731112000018
Bonfatti, V., Degano, L., Menegoz, A., and Carnier, P. (2016). Short communication: mid-infrared spectroscopy prediction of fine milk composition and technological properties in Italian Simmental. J. Dairy Sci. 99, 8216–8221. doi: 10.3168/jds.2016-10953
Bonfatti, V., Di Martino, G., and Carnier, P. (2011). Effectiveness of mid-infrared spectroscopy for the prediction of detailed protein composition and contents of protein genetic variants of individual milk of Simmental cows. J. Dairy Sci. 94, 5776–5785. doi: 10.3168/JDS.2011-4401
Bonfatti, V., Ho, P. N., and Pryce, J. E. (2020). Usefulness of milk mid-infrared spectroscopy for predicting lameness score in dairy cows. J. Dairy Sci. 103, 2534–2544. doi: 10.3168/jds.2019-17551
Bonfatti, V., Tiezzi, F., Miglior, F., and Carnier, P. (2017). Comparison of Bayesian regression models and partial least squares regression for the development of infrared prediction equations. J. Dairy Sci. 100, 7306–7319. doi: 10.3168/jds.2016-12203
Bonfatti, V., Turner, S.-A., Kuhn-Sherlock, B., Luke, T. D. W., Ho, P. N., Phyn, C. V. C., et al. (2019). Prediction of blood β-hydroxybutyrate content and occurrence of hyperketonemia in early-lactation, pasture-grazed dairy cows using milk infrared spectra. J. Dairy Sci. 102, 6466–6476. doi: 10.3168/jds.2018-15988
Boval, M., Coates, D. B., Lecomte, P., Decruyenaere, V., and Archimède, H. (2004). Faecal near infrared reflectance spectroscopy (NIRS) to assess chemical composition, in vivo digestibility and intake of tropical grass by Creole cattle. Anim. Feed. Sci. Technol. 114, 19–29. doi: 10.1016/j.anifeedsci.2003.12.009
Byrne, C. E., Downey, G., Troy, D. J., and Buckley, D. J. (1998). Non-destructive prediction of selected quality attributes of beef by near-infrared reflectance spectroscopy between 750 and 1098 nm. Meat Sci. 49, 399–409. doi: 10.1016/S0309-1740(98)00005-9
Cafferky, J., Sweeney, T., Allen, P., Sahar, A., Downey, G., Cromie, A. R., et al. (2020). Investigating the use of visible and near infrared spectroscopy to predict sensory and texture attributes of beef M. longissimus thoracis et lumborum. Meat Sci. 159:107915. doi: 10.1016/j.meatsci.2019.107915
Cecchinato, A., De Marchi, M., Boukha, A., Ribeca, C., and Carnier, P. (2009). Genetic correlations between measures of beef quality traits and their predictions by near- infrared spectroscopy in the Piemontese cattle breed. Ital. J. Anim. Sci. 8, 51–53. doi: 10.4081/ijas.2009.s2.51
Cecchinato, A., De Marchi, M., Penasa, M., Albera, A., and Bittante, G. (2011). Near-infrared reflectance spectroscopy predictions as indicator traits in breeding programs for enhanced beef quality. J. Anim. Sci. 89, 2687–2695. doi: 10.2527/jas.2010-3740
Cecchinato, A., De Marchi, M., Penasa, M., Casellas, J., Schiavon, S., and Bittante, G. (2012). Genetic analysis of beef fatty acid composition predicted by near-infrared spectroscopy. J. Anim. Sci. 90, 429–438. doi: 10.2527/jas.2011-4150
Centner, V., and Massart, D.-L. (1996). Elimination of uninformative variables for multivariate calibration. Anal. Chem. 68, 3851–3858. doi: 10.1021/ac960321m
Chapman, J., Elbourne, A., Truong, V. K., and Cozzolino, D. (2019). Shining light into meat - a review on the recent advances in in vivo and carcass applications of near infrared spectroscopy. Int. J. Food Sci. Technol. doi: 10.1111/ijfs.14367
Chilliard, Y., Martin, C., Rouel, J., and Doreau, M. (2009). Milk fatty acids in dairy cows fed whole crude linseed, extruded linseed, or linseed oil, and their relationship with methane output. J. Dairy Sci. 92, 5199–5211. doi: 10.3168/jds.2009-2375
Coffey, M. P., Emmans, G. C., and Brotherstone, S. (2001). Genetic evaluation of dairy bulls for energy balance traits using random regression. Anim. Sci. 73, 29–40. doi: 10.1017/S1357729800058021
Collard, B. L., Boettcher, P. J., Dekkers, J. C. M., Petitclerc, D., and Schaeffer, L. R. (2000). Relationships between energy balance and health traits of dairy cattle in early lactation. J. Dairy Sci. 83, 2683–2690. doi: 10.3168/jds.S0022-0302(00)75162-9
Connor, E. E. (2015). Invited review: improving feed efficiency in dairy production: challenges and possibilities. Animal 9, 395–408. doi: 10.1017/S1751731114002997
Coppa, M., Ferlay, A., Leroux, C., Jestin, M., Chilliard, Y., Martin, B., et al. (2010). Prediction of milk fatty acid composition by near infrared reflectance spectroscopy. Int. Dairy J. 20, 182–189. doi: 10.1016/j.idairyj.2009.11.003
Cozzolino, D., De Mattos, D., and Vaz Martins, D. (2002). Visible/near infrared reflectance spectroscopy for predicting composition and tracing system of production of beef muscle. Anim. Sci. 74, 477–484. doi: 10.1017/S1357729800052632
Cozzolino, D., and Murray, I. (2002). Effect of sample presentation and animal muscle species on the analysis of meat by near infrared reflectance spectroscopy. J. Near Infrared Spec. 10, 37–44. doi: 10.1255/jnirs.319
de los Campos, G., Hickey, J. M., Pong-Wong, R., Daetwyler, H. D., and Calus, M. P. L. (2013). Whole genome regression and prediction methods applied to plant and animal breeding. Genetics 193, 327–345. doi: 10.1534/genetics.112.143313
De Marchi, M. (2013). On-line prediction of beef quality traits using near infrared spectroscopy. Meat Sci. 94, 455–460. doi: 10.1016/j.meatsci.2013.03.003
De Marchi, M., Berzaghi, P., Boukha, A., Mirisola, M., and Galol, L. (2007). Use of near infrared spectroscopy for assessment of beef quality traits. Ital. J. Anim. Sci. 6, 421–423. doi: 10.4081/ijas.2007.1s.421
De Marchi, M., Bonfatti, V., Cecchinato, A., Di Martino, G., and Carnier, P. (2009). Prediction of protein composition of individual cow milk using mid-infrared spectroscopy. Ital. J. Anim. Sci. 8, 399–401. doi: 10.4081/ijas.2009.s2.399
De Marchi, M., Penasa, M., Cecchinato, A., and Bittante, G. (2013). The relevance of different near infrared technologies and sample treatments for predicting meat quality traits in commercial beef cuts. Meat Sci. 93, 329–335. doi: 10.1016/j.meatsci.2012.09.013
De Marchi, M., Penasa, M., Cecchinato, A., Mele, M., Secchiari, P., and Bittante, G. (2011). Effectiveness of mid-infrared spectroscopy to predict fatty acid composition of Brown Swiss bovine milk. Animal 5, 1653–1658. doi: 10.1017/S1751731111000747
De Marchi, M., Toffanin, V., Cassandro, M., and Penasa, M. (2014). Invited review: mid-infrared spectroscopy as phenotyping tool for milk traits. J. Dairy Sci. 97, 1171–1186. doi: 10.3168/jds.2013-6799
de Roos, A. P. W., van den Bijgaart, H. J. C. M., Hørlyk, J., and Jong, G. (2007). Screening for Subclinical ketosis in dairy cattle by fourier transform infrared spectrometry. J. Dairy Sci. 90, 1761–1766. doi: 10.3168/jds.2006-203
de Vries, M. J., and Veerkamp, R. F. (2000). Energy balance of dairy cattle in relation to milk production variables and fertility. J. Dairy Sci. 83, 62–69. doi: 10.3168/jds.s0022-0302(00)74856-9
Decruyenaere, V., Visser, M., de Stilmant, D., Clément, C., and Sinnaeve, G. (2004). Faecal near infrared reflectance spectroscopy for ruminant feed intake prediction. Grassl. Sci. 9, 1034–1036.
Dehareng, F., Delfosse, C., Froidmont, E., and Soyeurt, H. (2012). Potential use of milk mid-infrared spectra to predict individual methane emission of dairy cows. Animal 6, 1694–1701. doi: 10.1017/S1751731112000456
Dekkers, J. C. M. (1991). Estimation of economic values for dairy cattle breeding goals: bias due to sub-optimal management policies. Livest. Prod. Sci. 29, 131–149. doi: 10.1016/0301-6226(91)90062-U
Delhez, P., Ho, P. N., Gengler, N., Soyeurt, H., and Pryce, J. E. (2020). Diagnosing the pregnancy status of dairy cows: How useful is milk mid-infrared spectroscopy? J. Dairy Sci. 103, 3264–3274. doi: 10.3168/jds.2019-17473
Dijkstra, J., van Zijderveld, S. M., Apajalahti, J. A., Bannink, A., Gerrits, W. J. J., Newbold, J. R., et al. (2011). Relationships between methane production and milk fatty acid profiles in dairy cattle. Anim. Feed Sci. Technol. 166-167, 590–595. doi: 10.1016/j.anifeedsci.2011.04.042
Diskin, M. G., and Kenny, D. A. (2014). Optimising reproductive performance of beef cows and replacement heifers. Animal 8, 27–39. doi: 10.1017/S175173111400086X
Dixit, Y., Casado-Gavalda, M. P., Cama-Moncunill, R., Cama-Moncunill, X., Cullena, P. J., and Sullivan, C. (2016). Prediction of beef fat contente simultaneously under static and motion conditions using near infrared spectroscopy. J. Near Infrared Spec. 24, 353–361. doi: 10.1255/jnirs.1221
Dixit, Y., Casado-Gavalda, M. P., Cama-Moncunill, R., Cullen, P. J., and Sullivan, C. (2017). Challenges in model development for meat composition using multipoint nir spectroscopy from at-line to in-line monitoring. J. Food Sci. 81, 1557–1562. doi: 10.1111/1750-3841.13770
Dórea, J. R. R., French, E. A., and Armentano, L. E. (2017). Use of milk fatty acids to estimate plasma nonesterified fatty acid concentrations as an indicator of animal energy balance. J. Dairy Sci. 100, 6164–6176. doi: 10.3168/jds.2016-12466
Dórea, J. R. R., Rosa, G. J. M., Weld, K. A., and Armentano, L. E. (2018). Mining data from milk infrared spectroscopy to improve feed intake predictions in lactating dairy cows. J. Dairy Sci. 101, 5878–5889. doi: 10.3168/jds.2017-13997
Egger-Danner, C., Cole, J. B., Pryce, J. E., Gengler, N., Heringstad, B., Bradley, A., et al. (2015). Invited review: overview of new traits and phenotyping strategies in dairy cattle with a focus on functional traits. Animal 9, 191–207. doi: 10.1017/s1751731114002614
Eijndhoven, M. H. T. M.-V., Soyeurt, H., Dehareng, F., and Calus, M. P. L. (2013). Validation of fatty acid predictions in milk using mid-infrared spectrometry across cattle breeds. Animal 7, 348–354. doi: 10.1017/S1751731112001218
Eskildsen, C. E., Rasmussen, M. A., Engelsen, S. B., Larsen, L. B., Poulsen, N. A., and Skov, T. (2014). Quantification of individual fatty acids in bovine milk by infrared spectroscopy and chemometrics: understanding predictions of highly collinear reference variables. J. Dairy Sci. 97, 7940–7951. doi: 10.3168/jds.2014-8337
Eskildsen, C. E., Skov, T., Hansen, M. S., Larsen, L. B., and Poulsen, N. A. (2016). Quantification of bovine milk protein composition and coagulation properties using infrared spectroscopy and chemometrics: a result of collinearity among reference variables. J. Dairy Sci. 99, 8178–8186. doi: 10.3168/jds.2015-10840
Fenwick, M. A., Llewellyn, S., Fitzpatrick, R., Kenny, D. A., Murphy, J. J., Patton, J., et al. (2008). Negative energy balance in dairy cows is associated with specific changes in IGF-binding protein expression in the oviduct. Reproduction 135, 63–75. doi: 10.1530/REP-07-0243
Ferragina, A., de los Campos, G., Vazquez, A. I., Cecchinato, A., and Bittante, G. (2015). Bayesian regression models outperform partial least squares methods for predicting milk components and technological properties using infrared spectral data. J. Dairy Sci. 98, 8133–8151. doi: 10.3168/jds.2014-9143
Ferrand, M., Huquet, B., Barbey, S., Barillet, F., Faucon, F., Larroque, H., et al. (2011). Determination of fatty acid profile in cow’s milk using mid-infrared spectrometry: interest of applying a variable selection by genetic algorithms before a PLS regression. Chemometr. Intell. Lab. 106, 183–189. doi: 10.1016/j.chemolab.2010.05.004
Ferrand, M., Miranda, G., Larroque, H., Leray, O., Guisnel, S., Lahalle, F., et al. (2012). Determination of protein composition in milk by mid-infrared spectrometry. ICAR Tech. Ser. 16, 41–45.
Ferrand-Calmels, M., Palhière, I., Brochard, M., Leray, O., Astruc, J. M., Aurel, M. R., et al. (2014). Prediction of fatty acid profiles in cow, ewe, and goat milk by mid-infrared spectrometry. J. Dairy Sci. 97, 17–35. doi: 10.3168/jds.2013-6648
Fleming, A., Schenkel, F., Ali, R., Corredig, M., Carta, S., Gregu, C., et al. (2019). Phenotypic investigation of fine milk components in bovine milk and their prediction using mid-infrared spectroscopy. Can. J. Anim. Sci. 99, 218–227. doi: 10.1139/cjas-2018-0058
Fleming, A., Schenkel, F. S., Chen, J., Malchiodi, F., Bonfatti, V., Ali, R. A., et al. (2017). Prediction of milk fatty acid content with mid-infrared spectroscopy in Canadian dairy cattle using differently distributed model development sets. J. Dairy Sci. 100, 5073–5081. doi: 10.3168/jds.2016-12102
Franzoi, M., Niero, G., Visentin, G., Penasa, M., Cassandro, M., and De Marchi, M. (2019). Variation of detailed protein composition of cow milk predicted from a large database of mid-infrared spectra. Animals 9:176. doi: 10.3390/ani9040176
Friggens, N. C., Bjerring, M., Ridder, C., Højsgaard, S., and Larsen, T. (2008). Improved detection of reproductive status in dairy cows using milk progesterone measurements. Reprod. Domest. Anim. 43, 113–121. doi: 10.1111/j.1439-0531.2008.01150.x
Friggens, N. C., Ridder, C., and Løvendahl, P. (2007). On the use of milk composition measures to predict the energy balance of dairy cows. J. Dairy Sci. 90, 5453–5467. doi: 10.3168/jds.2006-821
Garnsworthy, P. C., and Unalt, Y. (2004). Estimation of dry-matter intake and digestibility in group-fed dairy cows using near infrared reflectance spectroscopy. Anim. Sci. 79, 327–334. doi: 10.1017/S1357729800090184
Gengler, N., Soyeurt, H., Dehareng, F., Bastin, C., Colinet, F., Hammami, H., et al. (2016). Capitalizing on fine milk composition for breeding and management of dairy cows. J. Dairy Sci. 99, 4071–4079. doi: 10.3168/jds.2015-10140
Gianola, D., Okut, H., Weigel, K. A., and Rosa, G. J. M. (2011). Predicting complex quantitative traits with Bayesian neural networks: a case study with Jersey cows and wheat. BMC Genet. 12:87. doi: 10.1186/1471-2156-12-87
Gianola, D., and Schön, C.-C. (2016). Cross-validation without doing cross-validation in genome-enabled prediction. G3 6, 3107–3128. doi: 10.1534/g3.116.033381
González-Recio, O., Pérez-Cabal, M. A., and Alenda, R. (2004). Economic value of female fertility and its relationship with profit in Spanish dairy cattle. J. Dairy Sci. 87, 3053–3061. doi: 10.3168/jds.S0022-0302(04)73438-4
Gottardo, P., De Marchi, M., Cassandro, M., and Penasa, M. (2015). Technical note: improving the accuracy of mid-infrared prediction models by selecting the most informative wavelengths. J. Dairy Sci. 98, 4168–4173. doi: 10.3168/jds.2014-8752
Gottardo, P., Penasa, M., Lopez-Villalobos, N., and De Marchi, M. (2016). Variable selection procedures before partial least squares regression enhance the accuracy of milk fatty acid composition predicted by mid-infrared spectroscopy. J. Dairy Sci. 99, 7782–7790. doi: 10.3168/jds.2016-10849
Grelet, C., Bastin, C., Gelé, M., Davière, J.-B., Johan, M., Werner, A., et al. (2016). Development of Fourier transform mid-infrared calibrations to predict acetone, β-hydroxybutyrate, and citrate contents in bovine milk through a European dairy network. J. Dairy Sci. 99, 4816–4825. doi: 10.3168/jds.2015-10477
Grelet, C., Froidmont, E., Foldager, L., Salavati, M., Hostens, M., Ferris, C. P., et al. (2020). Potential of milk mid-infrared spectra to predict nitrogen use efficiency of individual dairy cows in early lactation. J. Dairy Sci. 103, 4435–4445. doi: 10.3168/jds.2019-17910
Grelet, C., Vanlierde, A., Hostens, M., Foldager, L., Salavati, M., Ingvartsen, K. L., et al. (2019). Potential of milk mid-IR spectra to predict metabolic status of cows through blood components and an innovative clustering approach. Animal 13, 649–658. doi: 10.1017/S1751731118001751
Griffiths, P. R., and de Hasenth, J. A. (2007). Fourier Transform Spectrometry, 2nd Edn. Hoboken, NJ: John Wiley and Sons Inc.
Hammond, K., Crompton, L. A., Bannink, A., Dijkstra, J., Yanez-Ruiz, D. R., O’Kiely, P., et al. (2016). Review of current in vivo measurement techniques for quantifying enteric methane emission from ruminants. Anim. Feed Sci. Technol. 219, 13–30. doi: 10.1016/j.anifeedsci.2016.05.018
Hansen, P. W. (1999). Screening of dairy cows for ketosis by use of infrared spectroscopy and multivariate calibration. J. Dairy Sci. 82, 2005–2010. doi: 10.3168/jds.S0022-0302(99)75437-8
Hastie, T., Tibshirani, R., and Friedman, J. (2009). The Elements of Statistical Learning, 2 Edn. New York, NY: Springer.
Hempstalk, K., McParland, S., and Berry, D. P. (2015). Machine learning algorithms for the prediction of conception success to a given insemination in lactating dairy cows. J. Dairy Sci. 98, 5262–5273. doi: 10.3168/jds.2014-8984
Heuer, C., Luinge, H. J., Lutz, E. T. G., Schukken, Y. H., van der Maas, J. H., Wilmink, H., et al. (2001). Determination of acetone in cow milk by fourier transform infrared spectroscopy for the detection of subclinical ketosis. J. Dairy Sci. 84, 575–582. doi: 10.3168/JDS.S0022-0302(01)74510-9
Hildrum, K. I., Nilsen, B. N., Mielnik, M., and Naes, T. (1994). Prediction of sensory characteristics of beef by near-infrared spectroscopy. Meat Sci. 38, 67–80. doi: 10.1016/0309-1740(94)90096-5
Ho, P. N., Bonfatti, V., Luke, T. D. W., and Pryce, J. E. (2019). Classifying the fertility of dairy cows using milk mid-infrared spectroscopy. J. Dairy Sci. 102, 10460–10470. doi: 10.3168/jds.2019-16412
Ho, P. N., Marett, L. C., Wales, W. J., Axford, M., Oakes, E. M., and Pryce, J. E. (2020). Predicting milk fatty acids and energy balance of dairy cows in Australia using milk mid-infrared spectroscopy. Anim. Prod. Sci. 60, 164–168. doi: 10.1071/AN18532
Huntington, G. B., Leonard, E. S., and Burns, J. C. (2011). Technical note: use of near-infrared reflectance spectroscopy to predict intake and digestibility in bulls and steers. J. Anim. Sci. 89, 1163–1166. doi: 10.2527/jas.2010-3376
James, G., Written, D., Hastie, T., and Tibshirani, R. (2013). An Introduction to Statistical Learning with Applications in R. New York, NY: Springer.
Jamrozik, J., Koeck, A., Kistemaker, G. J., and Miglior, F. (2016). Multiple-trait estimates of genetic parameters for metabolic disease traits, fertility disorders, and their predictors in Canadian Holsteins. J. Dairy Sci. 99, 1990–1998. doi: 10.3168/jds.2015-10505
Jamrozik, J., Koeck, A., Miglior, F., Kistemaker, G., Schenkel, F., Kelton, D., et al. (2013). Genetic and genomic evaluation of mastitis resistance in Canada. Interbull Bull. 47, 43–51.
Johnson, J. R., Carstens, G. E., Prince, S. D., Ominski, K. H., Wittenberg, K. M., Undi, M., et al. (2017). Application of fecal near-infrared reflectance spectroscopy profiling for the prediction of diet nutritional characteristics and voluntary intake in beef cattle. J. Dairy Sci. 95, 447–454. doi: 10.2527/jas2016.0845
Johnson, K. A., and Johnson, D. E. (1995). Methane emissions from cattle. J. Anim. Sci. 73, 2483–2492. doi: 10.2527/1995.7382483x
Kaniyamattam, K., Elzo, M. A., Cole, J. B., and De Vries, A. (2016). Stochastic dynamic simulation modeling including multitrait genetics to estimate genetic, technical, and financial consequences of dairy farm reproduction and selection strategies. J. Dairy Sci. 99, 8187–8202. doi: 10.3168/jds.2016-11136
Karoui, R., Downey, G., and Blecker, C. (2010). Mid-infrared spectroscopy coupled with chemometrics: a tool for the analysis of intact food systems and the exploration of their molecular structure quality relationships - A review. Chem. Rev. 110, 6144–6168. doi: 10.1021/cr100090k
Kelton, D. F., Lissemore, K. D., and Martin, R. E. (1998). Recommendations for recording and calculating the incidence of selected clinical diseases of dairy cattle. J. Dairy Sci. 81, 2502–2509. doi: 10.3168/JDS.S0022-0302(98)70142-0
Knapp, J. R., Laur, G. L., Vadas, P. A., Weiss, W. P., and Tricarico, J. M. (2014). Invited review: enteric methane in dairy cattle production: quantifying the opportunities and impact of reducing emissions. J. Dairy Sci. 97, 3231–3261. doi: 10.3168/jds.2013-7234
Koljonen, J., Nordling, T. E. M., and Alander, J. T. (2008). A review of genetic algorithms in near infrared spectroscopy and chemometrics: past and future. J. Near Infrared Spectrosc. 16, 189–197. doi: 10.1255/jnirs.778
Lahart, B., McParland, S., Kennedy, E., Boland, T. M., Condon, T., Williams, M., et al. (2019). Predicting the dry matter intake of grazing dairy cows using infrared reflectance spectroscopy analysis. J. Dairy Sci. 102, 8907–8918. doi: 10.3168/jds.2019-16363
Landau, S. Y., Dvash, L., Roudman, M., Muklada, H., Barkai, D., Yehuda, Y., et al. (2016). Faecal near-IR spectroscopy to determine the nutritional value of diets consumed by beef cattle in east Mediterranean rangelands. Animal 10, 192–202. doi: 10.1017/S175173111500169X
Leardi, R., Boggia, R., and Terrile, M. (1992). Genetic algorithms as a strategy for feature selection. J. Chemom. 6, 267–281. doi: 10.1002/cem.1180060506
Leroy, B., Lambotte, S., Dotreppe, O., Lecocq, H., Istasse, L., and Clinquart, A. (2003). Prediction of technological and organoleptic properties of beef Longissimus thoracis from near-infrared reflectance and transmission spectra. Meat Sci. 66, 45–54. doi: 10.1016/S0309-1740(03)00002-0
Liu, Y., Lyon, B. G., Windham, W. R., Realini, C. E., Pringle, T. D. D., and Duckett, S. (2003). Prediction of color, texture, and sensory characteristics of beef steaks by visible and near infrared reflectance spectroscopy. A feasibility study. Meat Sci. 65, 1107–1115. doi: 10.1016/S0309-1740(02)00328-5
Lopez-Villalobos, N., Spelman, R. J., Melis, J., Davis, S. R., Berry, S. D., Lehnert, K., et al. (2014). Estimation of genetic and crossbreeding parameters of fatty acid concentrations in milk fat predicted by mid-infrared spectroscopy in New Zealand dairy cattle. J. Dairy Res. 81, 340–349. doi: 10.1017/S0022029914000272
Luginbühl, W. (2002). Evaluation of designed calibration samples for casein calibration in Fourier transform infrared analysis of milk. LWT Food Sci. Technol. 35, 554–558. doi: 10.1006/FSTL.2002.0902
Luke, T. D. W., Rochfort, S., Wales, W. J., Bonfatti, V., Marett, L., and Pryce, J. E. (2019). Metabolic profiling of early-lactation dairy cows using milk mid-infrared spectra. J. Dairy Sci. 102, 1747–1760. doi: 10.3168/jds.2018-15103
Magalhães, A. F. B., Teixeira, G. H. A., Ríos, A. C. H., Silva, D. B. S., Mota, L. F. M., Muniz, M. M. M., et al. (2018). Prediction of meat quality traits in Nelore cattle by near-infrared reflectance spectroscopy. J. Anim. Sci. 96, 4229–4237. doi: 10.1093/jas/sky284
Malacarne, M., Visentin, G., Summer, A., Cassandro, M., Penasa, M., Bolzoni, G., et al. (2018). Investigation on the effectiveness of mid-infrared spectroscopy to predict detailed mineral composition of bulk milk. J. Dairy Res. 85, 83–86. doi: 10.1017/S0022029917000826
Mann, S., Nydam, D. V., Lock, A. L., Overton, T. R., and McArt, J. A. A. (2016). Short communication: association of milk fatty acids with early lactation hyperketonemia and elevated concentration of nonesterified fatty acids. J. Dairy Sci. 99, 5851–5857. doi: 10.3168/jds.2016-10920
Martens, H., and Jensen, S. A. (1982). “Partial least squares regression: a new two-stage NIR calibration method,” in Proceedings of the 7th World Cereal and Bread Congress, Amsterdam, 607–647.
Martens, H., Jensen, S. A., and Geladi, P. (1983). “Multivariate linearity transformations for near infrared reflectance spectroscopy,” in Nordic Symposium on Applied Statistics, ed. O. H. J. Christie (Stokkand: Stokkand Forlag), 205–234.
Martens, H., and Naes, T. (1987). Multivariate Calibration by Data Compression. Chichester: John Wiley & Sons.
Martin, A. D., Afseth, N. K., Kohler, A., Randby, Å., Eknæs, M., Waldmann, A., et al. (2015). The relationship between fatty acid profiles in milk identified by Fourier transform infrared spectroscopy and onset of luteal activity in Norwegian dairy cattle. J. Dairy Sci. 98, 5374–5384. doi: 10.3168/jds.2015-9343
McArt, J. A. A., Nydam, D. V., and Overton, M. W. (2015). Hyperketonemia in early lactation dairy cattle: a deterministic estimate of component and total cost per case. J. Dairy Sci. 98, 2043–2054. doi: 10.3168/jds.2014-8740
McDermott, A., Visentin, G., De Marchi, M., Berry, D. P., Fenelon, M. A., O’Connor, P. M., et al. (2016). Prediction of individual milk proteins including free amino acids in bovine milk using mid-infrared spectroscopy and their correlations with milk processing characteristics. J. Dairy Sci. 99, 3171–3182. doi: 10.3168/jds.2015-9747
McParland, S., Banos, G., McCarthy, B., Lewis, E., Coffey, M. P., O’Neill, B., et al. (2012). Validation of mid-infrared spectrometry in milk for predicting body energy status in Holstein-Friesian cows. J. Dairy Sci. 95, 7225–7235. doi: 10.3168/jds.2012-5406
McParland, S., Banos, G., Wall, E., Coffey, M. P., Soyeurt, H., Veerkamp, R. F., et al. (2011). The use of mid-infrared spectrometry to predict body energy status of Holstein cows. J. Dairy Sci. 94, 3651–3661. doi: 10.3168/jds.2010-3965
McParland, S., and Berry, D. P. (2016). The potential of Fourier transform infrared spectroscopy of milk samples to predict energy intake and efficiency in dairy cows. J. Dairy Sci. 99, 4056–4070. doi: 10.3168/jds.2015-10051
McParland, S., Kennedy, E., Lewis, E., Moore, S. G., McCarthy, B., O’Donovan, M., et al. (2015). Genetic parameters of dairy cow energy intake and body energy status predicted using mid-infrared spectrometry of milk. J. Dairy Sci. 98, 1310–1320. doi: 10.3168/jds.2014-8892
McParland, S., Lewis, E., Kennedy, E., Moore, S. G., McCarthy, B., O’Donovan, M., et al. (2014). Mid-infrared spectrometry of milk as a predictor of energy intake and efficiency in lactating dairy cows. J. Dairy Sci. 97, 5863–5871. doi: 10.3168/jds.2014-8214
Meuwissen, T. H. E., Hayes, B. J., and Goddard, M. E. (2001). Prediction of total genetic value using genome-wide dense marker maps. Genetics 157, 1819–1829.
Miglior, F., Koeck, A., Jamrozik, J., Schenkel, F. S., Kelton, D. F., Kistemaker, G. J., et al. (2014). Index for mastitis resistance and use of BHBA for evaluation of health traits in Canadian Holsteins. Interbull Bull. 48, 73–78.
Mineur, A. Köck, A., Grelet, C., Gengler, N., Egger-Danner, C., and Sölkner, J. (2017). First results in the use of milk mid-infrared spectra in the detection of lameness in Austrian dairy cows. Agric. Conspec. Sci. 82, 163–166.
Mitsumoto, M., Maeda, S., Mitsuhashi, T., and Ozawa, S. (1991). Near-infrared spectroscopy determination of physical and chemical characteristics in beef cuts. J. Food Sci. 56, 1493–1496. doi: 10.1111/j.1365-2621.1991.tb08623.x
Moraes, J. C. F., Miguel, J. C., and Souza, C. J. H. (2007). Body condition score to predict the postpartum fertility of crossbred beef cows. Pesq. Agropec. Bras. 42, 741–746. doi: 10.1590/S0100-204X2007000500018
Müller, U., Kessera, J., Kochb, C., Helfrichc, H.-P., and Rietzd, C. (2019). Monitoring predictive and informative indicators of the energy status of dairy cows during early lactation in the context of monthly milk recordings using mid-infrared spectroscopy. Livest. Sci. 221, 6–14. doi: 10.1016/j.livsci.2018.12.020
Negussie, E., de Haas, Y., Dehareng, F., Dewhurst, R. J., Dijkstra, J., Gengler, N., et al. (2017). Invited review: large-scale indirect measurements for enteric methane emissions in dairy cattle: a review of proxies and their potential for use in management and breeding decisions. J. Dairy Sci. 100, 2433–2453. doi: 10.3168/jds.2016-12030
Niero, G., Penasa, M., Gottardo, P., Cassandro, M., and De Marchi, M. (2016). Short communication: selecting the most informative mid-infrared spectra wavenumbers to improve the accuracy of prediction models for detailed milk protein content. J. Dairy Sci. 99, 1853–1858. doi: 10.3168/jds.2015-10318
Park, B., Chen, Y. R., Hruschka, W. R., Shackelford, S. D., and Koohmaraie, M. (1998). Near-infrared reflectance analysis for predicting beef longissimus tenderness. J. Anim. Sci. 76, 2115–2120. doi: 10.2527/1998.7682115x
Patra, A. K. (2016). Recent advances in measurement and dietary mitigation of enteric methane emissions in ruminants. Front. Vet. Sci. 3:39. doi: 10.3389/fvets.2016.00039
Pearl, J. (1988). Probabilistic Reasoning in Intelligent Systems: Networks of Plausible Inference. San Mateo, CA: Morgan Kaufmann.
Picard, R. R., and Berk, K. N. (1990). Data splitting. Am. Stat. 44, 140–147. doi: 10.1080/00031305.1990
Pralle, R. S., Weigel, K. W., and White, H. M. (2018). Predicting blood β-hydroxybutyrate using milk Fourier transform infrared spectrum, milk composition, and producer-reported variables with multiple linear regression, partial least squares regression, and artificial neural network. J. Dairy Sci. 101, 4378–4387. doi: 10.3168/jds.2017-14076
Pravia, M. I., Ravagnolo, O., Urioste, J. I., and Garrick, D. J. (2014). Identification of breeding objectives using a bioeconomic model for a beef cattle production system in Uruguay. Livest. Sci. 160, 21–28. doi: 10.1016/j.livsci.2013.12.006
Prevolnik, M., Èandek-Potokar, M., Škorjanc, D., Velikonja-Bolta, Š., Škrlep, M., Žnidaršiè, T., et al. (2005). Predicting intramuscular fat content in pork and beef by near infrared spectroscopy. Near Infrared Spectrosc. 13, 77–85. doi: 10.1255/jnirs.460
Prevolnik, M., Candek-Potokar, M., and Skorjank, D. (2004). Ability of NIR spectroscopy to predict chemical composition and quality - a review. Czech J. Anim. Sci. 49, 500–510. doi: 10.17221/4337-CJAS
Prieto, N., Andrés, S., Giráldez, F. J., Mantecón, A. R., and Lavín, P. (2008). Ability of near infrared reflectance spectroscopy (NIRS) to estimate physical parameters of adult steers (oxen) and young cattle meat samples. Meat Sci. 79, 692–699. doi: 10.1016/J.MEATSCI.2007.10.035
Prieto, N., López-Campos, Ó., Aalhus, J. L., Dugan, M. E. R., Juárez, M., and Uttaro, B. (2014). Use of near infrared spectroscopy for estimating meat chemical composition, quality traits and fatty acid content from cattle fed sunflower or flaxseed. Meat Sci. 98, 279–288. doi: 10.1016/j.meatsci.2014.06.005
Prieto, N., Pawluczyk, O., Dugan, M. E. R., and Aalhus, J. L. (2017). A review of the principles and applications of near-infrared spectroscopy to characterize meat, fat, and meat products. Appl. Spectrosc. 71, 1403–1426. doi: 10.1177/0003702817709299
Prieto, N., Roehe, R., Lavín, P., Batten, G., and Andrés, S. (2009a). Application of near infrared reflectance spectroscopy to predict meat and meat products quality: a review. Meat Sci. 83, 175–186. doi: 10.1016/j.meatsci.2009.04.016
Prieto, N., Ross, D. W., Navajas, E. A., Nute, G. R., Richardson, R. I., and Hyslop, J. J. (2009b). On-line application of visible and near infrared reflectance spectroscopy to predict chemical-physical and sensory characteristics of beef quality. Meat Sci. 83, 96–103. doi: 10.1016/j.meatsci.2009.04.005
Prieto, N., Ross, D. W., Navajas, E. A., Richardson, R. I., Hyslop, J. J., Simm, G., et al. (2011). Online prediction of fatty acid profiles in crossbred Limousin and Aberdeen Angus beef cattle using near infrared reflectance spectroscopy. Animal 5, 155–165. doi: 10.1017/S1751731110001618
Qiao, T., Ren, J., Craigie, C., Zabalza, J., Maltin, C., and Marshalla, S. (2015). Quantitative prediction of beef quality using visible and nir spectroscopy with large data samples under industry conditions. J. Appl. Spectrosc. 82, 137–144. doi: 10.1007/s10812-015-0076-1
Rico, D. E., Chouinard, P. Y., Hassanat, F., Benchaar, C., and Gervais, R. (2016). Prediction of enteric methane emissions from Holstein dairy cows fed various forage sources. Animal 10, 203–211. doi: 10.1017/S1751731115001949
Rienesl, L., Khayatzadeh, N., Köck, A., Dale, L., Werner, A., Grelet, C., et al. (2019). Mastitis detection from milk mid- infrared (mir) spectroscopy in dairy cows. Acta Univ. Agric. Silvic. Mendel. Brun. 67, 1221–1226. doi: 10.11118/actaun201967051221
Rinnan, A., van den Berg, F., and Engelsen, S. B. (2009a). Review of the most common pre-processing techniques for near-infrared spectra. TrAC Trend Anal. Chem. 28, 1201–1222. doi: 10.1016/j.trac.2009.07.007
Rinnan, Å., Nørgaard, L., van den Berg, F., Thygesen, J., Bro, R., and Engelsen, S. B. (2009b). “Data Pre-processing,” in Infrared Spectroscopy for Food Quality Analysis and Control, ed. D.-W. Sun (New York, NY: Academic Press), 29–50.
Ripoll, G., Albertí, P., Panea, B., Olleta, J. L., and Sañudo, C. (2008). Near-infrared reflectance spectroscopy for predicting chemical, instrumental and sensory quality of beef. Meat Sci. 80, 697–702. doi: 10.1016/j.meatsci.2008.03.009
Roberts, D. R., Bahn, V., Ciuti, S., Boyce, M. S., Elith, J., Guillera-Arroita, G., et al. (2017). Crossvalidation strategies for data with temporal, spatial, hierarchical, or phylogenetic structure. Ecography 40, 913–929. doi: 10.1111/ecog.02881
Rødbotten, R., Mevik, B.-H., and Hildrum, K. I. (2001). Prediction and classification of tenderness in beef from non-invasive diode array detected NIR spectra. J. Near Infrared Spectrosc. 9, 199–210. doi: 10.1255/jnirs.306
Rødbotten, R., Nilsen, B. N., and Hildrum, K. I. (2000). Prediction of beef quality attributes from early post mortem near infrared reflectance spectra. Food Chem. 69, 427–436. doi: 10.1016/S0308-8146(00)00059-5
Rosenvold, K., Micklander, E., Hansen, P. W., Burling-Claridge, R., Challies, M., Devine, C., et al. (2009). Temporal, biochemical and structural factors that influence beef quality measurement using near infrared spectroscopy. Meat Sci. 82, 379–388. doi: 10.1016/j.meatsci.2009.02.010
Rutten, M. J. M., Bovenhuis, H., Heck, J. M. L., and van Arendonk, J. A. M. (2011). Predicting bovine milk protein composition based on Fourier transform infrared spectra. J. Dairy Sci. 94, 5683–5690. doi: 10.3168/jds.2011-4520
Rutten, M. J. M., Bovenhuis, H., Hettinga, K. A., van Valenberg, H. J. F., and van Arendonk, J. A. M. (2009). Predicting bovine milk fat composition using infrared spectroscopy based on milk samples collected in winter and summer. J. Dairy Sci. 92, 6202–6209. doi: 10.3168/jds.2009-2456
Rutten, M. J. M., Bovenhuis, H., and van Arendonk, J. A. M. (2010). The effect of the number of observations used for Fourier transform infrared model calibration for bovine milk fat composition on the estimated genetic parameters of the predicted data. J. Dairy Sci. 93, 4872–4882. doi: 10.3168/jds.2010-3157
Sanderson, R., Lister, S. J., Dhanoa, M. S., Barnes, R. J., and Thomas, C. (1997). Use of near infrared reflectance spectroscopy to predict and compare the composition of carcass samples from young steers. Anim. Sci. 65, 45–54. doi: 10.1017/S1357729800016283
Savitzky, A., and Golay, M. J. E. (1964). Smoothing and differentiation of data by simplified least squares procedures. Anal. Chem. 36, 1627–1639. doi: 10.1021/ac60214a047
Savoia, S., Albera, A., Brugiapaglia, A., Stasio, L. D., Ferragina, A., Cecchinato, A., et al. (2020). Prediction of meat quality traits in the abattoir using portable and hand-held near-infrared spectrometers. Meat Sci. 161:108017. doi: 10.1016/j.meatsci.2019.108017
Seymour, D. J., Cánovas, A., Baes, C. F., Chud, T. C. S., Osborne, V. R., Cant, J. P., et al. (2019). Invited review: determination of large-scale individual dry matter intake phenotypes in dairy cattle. J. Dairy Sci. 102, 7655–7663. doi: 10.3168/jds.2019-16454
Shackelford, S. D., Wheeler, T. L., and Koohmaraie, M. (2005). On-line classification of US Select beef carcasses for longissimus tenderness using visible and near-infrared reflectance spectroscopy. Meat Sci. 69, 409–415. doi: 10.1016/J.MEATSCI.2004.08.011
Shackelford, S. D., Wheeler, T. L., Meade, M. K., Reagan, J. O., Byrnes, B. L., and Koohmaraie, M. (2001). Consumer impressions of tender select beef. J. Anim. Sci. 79, 2605–2614. doi: 10.2527/2001.79102605x
Shetty, N., Difford, G., Lassen, J., Løvendahl, P., and Buitenhuis, A. J. (2017a). Predicting methane emissions of lactating Danish Holstein cows using Fourier transform mid-infrared spectroscopy of milk. J. Dairy Sci. 100, 9052–9060. doi: 10.3168/jds.2017-13014
Shetty, N., Løvendahl, P., Lund, M. S., and Buitenhuis, A. J. (2017b). Prediction and validation of residual feed intake and dry matter intake in Danish lactating dairy cows using mid-infrared spectroscopy of milk. J. Dairy Sci. 100, 253–264. doi: 10.3168/jds.2016-11609
Smith, S. L., Denholm, S. J., Coffey, M. P., and Wall, E. (2019). Energy profiling of dairy cows from routine milk mid-infrared analysis. J. Dairy Sci. 102, 11169–11179. doi: 10.3168/jds.2018-16112
Sørensen, L. K., Lund, M., and Juul, B. (2003). Accuracy of Fourier transform infrared spectrometry in determination of casein in dairy cows’ milk. J. Dairy Res. 70, 445–452. doi: 10.1017/S0022029903006435
Soyeurt, H., Bruwier, D., Romnee, J. M., Gengler, N., Bertozzi, C., Veselko, D., et al. (2009). Potential estimation of major mineral contents in cow milk using mid-infrared spectrometry. J. Dairy Sci. 92, 2444–2454. doi: 10.3168/jds.2008-1734
Soyeurt, H., Dardenne, P., Dehareng, F., Lognay, G., Veselko, D., Marlier, M., et al. (2006). Estimating fatty acid content in cow milk using mid-infrared spectrometry. J. Dairy Sci. 89, 3690–3695. doi: 10.3168/jds.S0022-0302(06)72409-2
Soyeurt, H., Dehareng, F., Gengler, N., McParland, S., Wall, E., Berry, D. P., et al. (2011). Mid-infrared prediction of bovine milk fatty acids across multiple breeds, production systems, and countries. J. Dairy Sci. 94, 1657–1667. doi: 10.3168/jds.2010-3408
Soyeurt, H., Dehareng, F., Mayeres, P., Bertozzi, C., and Gengler, N. (2008). Variation of Δ9-desaturase activity in dairy cattle. J. Dairy Sci. 9, 13211–13224. doi: 10.3168/jds.2007-0518
Stone, M. (1974). Cross-validatory choice and assessment of statistical predictions. J. R. Stat. Soc. B. 36, 111–147.
Su, H., Sha, K., Zhang, L., Zhang, Q., Xu, Y., Zhang, R., et al. (2014). Development of near infrared reflectance spectroscopy to predict chemical composition with a wide range of variability in beef. Meat Sci. 98, 110–114. doi: 10.1016/j.meatsci.2013.12.019
Su, H., Zhang, S., Li, H., Xie, P., and Sun, B. (2018). Using near-infrared reflectance spectroscopy to predict physical parameters of beef. Spectrosc. Lett. 51, 163–168. doi: 10.1080/00387010.2018.1442355
Toffanin, V., De Marchi, M., Lopez-Villalobos, N., and Cassandro, M. (2015). Effectiveness of mid-infrared spectroscopy for prediction of the contents of calcium and phosphorus, and titratable acidity of milk and their relationship with milk quality and coagulation properties. Int. Dairy J. 41, 68–73. doi: 10.1016/j.idairyj.2014.10.002
Toledo-Alvarado, H., Vazquez, A. I., de los Campos, G., Tempelman, R. J., Bittante, G., and Cecchinato, A. (2018). Diagnosing pregnancy status using infrared spectra and milk composition in dairy cows. J. Dairy Sci. 101, 2496–2505. doi: 10.3168/JDS.2017-13647
Tran, H., Salgado, P., Tillard, E., Dardenne, P., Nguyen, X. T., and Lecomte, P. (2010). “Global” and “local” predictions of dairy diet nutritional quality using near infrared reflectance spectroscopy. J. Dairy Sci. 93, 4961–4975. doi: 10.3168/jds.2008-1893
van Gastelen, S., Mollenhorst, H., Antunes-Fernandes, E. C., Hettinga, K. A., van Burgsteden, G. G., Dijkstra, J., et al. (2018). Predicting enteric methane emission of dairy cows with milk Fourier-transform infrared spectra and gas chromatography–based milk fatty acid profiles. J. Dairy Sci. 101, 5582–5598. doi: 10.3168/jds.2017-13052
van Lingen, H. J., Crompton, L. A., Hendriks, W. H., Reynolds, C. K., and Dijkstra, J. (2014). Meta-analysis of relationships between enteric methane yield and milk fatty acid profile in dairy cattle. J. Dairy Sci. 97, 7115–7132. doi: 10.3168/jds.2014-8268
Vanlierde, A., Soyeurt, H., Gengler, N., Colinet, F. G., Froidmont, E., and Kreuzer, M. (2018). Short communication: development of an equation for estimating methane emissions of dairy cows from milk Fourier transform mid-infrared spectra by using reference data obtained exclusively from respiration chambers. J. Dairy Sci. 101, 7618–7624. doi: 10.3168/jds.2018-14472
Vanlierde, A., Vanrobays, M.-L., Dehareng, F., Froidmont, E., Soyeurt, H., and McParland, S. (2015). Hot topic: innovative lactation-stage-dependent prediction of methane emissions from milk mid-infrared spectra. J. Dairy Sci. 98, 5740–5747. doi: 10.3168/jds.2014-8436
Vanlierde, A., Vanrobays, M.-L., Gengler, N., Dardenne, P., Froidmont, E., and Soyeurt, H. (2016). Milk mid-infrared spectra enable prediction of lactation-stage- dependent methane emissions of dairy cattle within routine population-scale milk recording schemes. Anim. Prod. Sci. 56, 258–264. doi: 10.1071/AN15590
Venel, C., Mullen, A. M., Downey, G., and Troy, D. J. (2001). Prediction of tenderness and other quality attributes of beef by near infrared reflectance spectroscopy between 750 and 1100 nm; further studies. J. Near Infrared Spectrosc. 9, 185–198. doi: 10.1255/jnirs.305
Visentin, G., Penasa, M., Gottardo, P., Cassandro, M., and De Marchi, M. (2016). Predictive ability of mid-infrared spectroscopy for major mineral com-position and coagulation traits of bovine milk by using the uninformative variable selection algorithm. J. Dairy Sci. 99, 8137–8145. doi: 10.3168/jds.2016-11053
Wallén, S. E., Prestløkken, E., Meuwissen, T. H. E., McParland, S., and Berry, D. P. (2018). Milk mid-infrared spectral data as a tool to predict feed intake in lactating Norwegian Red dairy cows. J. Dairy Sci. 101, 6232–6243. doi: 10.3168/jds.2017-13874
Wang, Q., and Bovenhuis, H. (2019). Validation strategy can result in an overoptimistic view of the ability of milk infrared spectra to predict methane emission of dairy cattle. J. Dairy Sci. 102, 6288–6295. doi: 10.3168/jds.2018-15684
Williams, J. L. (2008). “Genetic control of meat quality traits,” in Meat biotechnology, ed. F. Toldrá (New York, NY: Springer), 21–61.
Wold, S., Antti, H., Lindgren, F., and Ohman, J. (1998). Orthogonal signal correction of near-infrared spectra. Chemometr. Intell. Lab. Syst. 44, 175–186. doi: 10.1016/S0169-7439(98)00109-9
Wold, S., Johansson, A., and Cochi, M. (1993). PLS-Partial Least Squares Projections to Latent Structures. Leiden: ESCOM Science Publishers.
Wu, D., Chen, X., Shi, P., Wang, S., Feng, F., and He, Y. (2009). Determination of α-linolenic acid and linoleic acid in edible oils using near-infrared spectroscopy improved by wavelet transform and uninformative variable elimination. Anal. Chim. Acta 634, 166–171. doi: 10.1016/j.aca.2008.12.024
Wu, D., Nie, P., He, Y., and Bao, Y. (2012). Determination of calcium content in powdered milk using near and mid-infrared spectroscopy with variable selection and chemometrics. Food Bioprocess Technol. 5, 1402–1410. doi: 10.1007/s11947-010-0492-4
Wyrwisz, J., Moczkowska, M., Kurek, M. A., Karp, S., Atanasov, A. G., and Wierzbicka, A. (2019). Evaluation of WBSF, color, cooking loss of Longissimus lumborum muscle with fiber optic near-infrared spectroscopy (FT-NIR), depending on aging time. Molecules 24:757. doi: 10.3390/molecules24040757
Yancey, J. W. S., Apple, J. K., Meullenet, J.-F., and Sawyer, J. T. (2010). Consumer responses for tenderness and overall impression can be predicted by visible and near-infrared spectroscopy, Meullenet–Owens razor shear, and Warner–Bratzler shear force. Meat Sci. 85, 487–492. doi: 10.1016/j.meatsci.2010.02.020
Keywords: beef cattle, dairy cattle, near-infrared, novel phenotypes, mid-infrared, spectral information
Citation: Bresolin T and Dórea JRR (2020) Infrared Spectrometry as a High-Throughput Phenotyping Technology to Predict Complex Traits in Livestock Systems. Front. Genet. 11:923. doi: 10.3389/fgene.2020.00923
Received: 06 April 2020; Accepted: 24 July 2020;
Published: 20 August 2020.
Edited by:
Fabyano Fonseca Silva, Universidade Federal de Viçosa, BrazilReviewed by:
Wei Shen, Qingdao Agricultural University, ChinaCopyright © 2020 Bresolin and Dórea. This is an open-access article distributed under the terms of the Creative Commons Attribution License (CC BY). The use, distribution or reproduction in other forums is permitted, provided the original author(s) and the copyright owner(s) are credited and that the original publication in this journal is cited, in accordance with accepted academic practice. No use, distribution or reproduction is permitted which does not comply with these terms.
*Correspondence: João R. R. Dórea, am9hby5kb3JlYUB3aXNjLmVkdQ==
Disclaimer: All claims expressed in this article are solely those of the authors and do not necessarily represent those of their affiliated organizations, or those of the publisher, the editors and the reviewers. Any product that may be evaluated in this article or claim that may be made by its manufacturer is not guaranteed or endorsed by the publisher.
Research integrity at Frontiers
Learn more about the work of our research integrity team to safeguard the quality of each article we publish.