- 1Institute of Animal Husbandry and Veterinary Science, Shanghai Academy of Agricultural Sciences, Shanghai, China
- 2Department of Genetics, Genomics, and Informatics, University of Tennessee Health Science Center, Memphis, TN, United States
- 3Department of Bioscience Research, College of Dentistry, University of Tennessee Health Science Center, Memphis, TN, United States
The Chongming white goat (CM) is an indigenous goat breed exhibits unique traits that are adapted to the local environment and artificial selection. By performing whole-genome re-sequencing, we generated 14–20× coverage sequences from 10 domestic goat breeds to explore the genomic characteristics and selection signatures of the CM breed. We identified a total of 23,508,551 single-nucleotide polymorphisms (SNPs) and 2,830,800 insertion–deletion mutations (indels) after read mapping and variant calling. We further specifically identified 1.2% SNPs (271,713) and 0.9% indels (24,843) unique to the CM breed in comparison with the other nine goat breeds. Missense (SIFT < 0.05), frameshift, splice-site, start-loss, stop-loss, and stop-gain variants were identified in 183 protein-coding genes of the CM breed. Of the 183, 36 genes, including AP4E1, FSHR, COL11A2, and DYSF, are involved in phenotype ontology terms related to the nervous system, short stature, and skeletal muscle morphology. Moreover, based on genome-wide FST and pooled heterozygosity (Hp) calculation, we further identified selection signature genes between the CM and the other nine goat breeds. These genes are significantly associated with the nervous system (C2CD3, DNAJB13, UCP2, ZMYND11, CEP126, SCAPER, and TSHR), growth (UCP2, UCP3, TSHR, FGFR1, ERLIN2, and ZNF703), and coat color (KITLG, ASIP, AHCY, RALY, and MC1R). Our results suggest that the CM breed may be differentiated from other goat breeds in terms of nervous system owing to natural or artificial selection. The whole-genome analysis provides an improved understanding of genetic diversity and trait exploration for this indigenous goat breed.
Introduction
Global livestock genetic diversity encompasses species, breeds, strains, and their variations. The diversity between and within breeds rather than species is always considered to be essential for the purposes of conservation biology (Yaro et al., 2017). Goats are important husbandry animals with an ancient domestication history and economic value. It has been reported that their domestication occurred approximately 10,000 years ago and spread worldwide following human migrations and trade routes (Benjelloun et al., 2015; Kim et al., 2019). China is the global leader in terms of goat production (Skapetas and Bampidis, 2016), including many commercial, indigenous, and composite breeds. Owing to the weaker production potential compared with that of some global commercial breeds, indigenous goat breeds are facing the problem of genetic invasion and resource degradation in recent years (Kim et al., 2019; Monau et al., 2020).
Indigenous goats are unique groups that have developed under the forces of natural selection, domestication, and local environmental conditions. Their genetic background, unique traits, and biodiversity, as well as their environmental adaptability, serve as a kind of biological heritage that can be used for the genetic improvement of animal husbandry (Kim et al., 2019). The Chongming white goat (CM) is an indigenous island-type goat breed distributed and bred on Shanghai Chongming Island. Although there have not been sufficient systematic studies on the selection signal genes for island climate adaptation, this may be a direction of research. The CM goats were also marked as special agricultural products with geographical indications registered by the State Administration for Industry and Commerce of China. Moreover, the relatively small size and ease of husbandry make it a suitable animal model for some specific human diseases such as acute spinal cord compression injuries (Huang et al., 2013). However, even with rich domestication history, studies on the genome-wide characteristics of this indigenous goat have not been reported yet to the best of our knowledge.
Whole-genome sequencing (WGS) information bypasses the study limitations of maternal mitochondrial DNA and paternal Y chromosome inheritance in species evolution and population history dynamics. Moreover, WGS can also ameliorate the current problems of breed bias and insufficient markers in genotyping chips, and the new genetic variation information also provides research materials for the further production of high-density chips. The use of WGS and reference genome alignment can identify more comprehensive, genome-wide variation, such as the presence of single-nucleotide polymorphisms (SNPs), insertion–deletion mutations (indels), and structural variants (SVs). Although high-throughput sequencing technology enables the comparison of patterns of polymorphisms at whole-genome levels, characterizing genetic structure from individual sequencing data still remains expensive for non-model species. The DNA pooling strategy (Pool-seq) represents an attractive and cost-effective alternative for SNP discovery (Futschik and Schlötterer, 2010; Hivert et al., 2018) and have been successfully applied to many domestic animal studies such as chicken (Rubin et al., 2010), pig (Rubin et al., 2012), goat (Wang et al., 2016; Guo et al., 2018), and sheep (Liu et al., 2016).
Molecular genetic markers, such as high-density SNPs, are often used to analyze genetic variation at the genome-wide level caused by natural and artificial selection of species. For example, the Chinese Tibetan cashmere goat adapted to a high-altitude area and hypoxic environment. In which, 339 genes were identified through exome sequencing, which are potentially under high-altitude selection (Song et al., 2016). Another study (Kim et al., 2019) analyzed the genome of the Korean indigenous goat (KNG) in comparison with that of nine other goat breeds and revealed that the KNG has selection signatures for Salmonella infection and cardiomyopathy pathways. Wang et al. (2016) generated 9–13× coverage sequences from eight domesticated goat breeds and identified 22 genomic regions that may be associated with specific phenotypic traits, including coat color, body size, cashmere traits, and high-altitude adaptation.
In this study, we aimed to explore the genomic characteristics in the CM goat. Utilizing WGS and genomic approaches, we conducted the first comparative genomic study to reveal the genome-wide variation and selection signatures in the CM breed.
Materials and Methods
Animals
We collected 100 goat samples from 10 breeds, with 10 individuals per breed (five bucks and five does). Samples of each breed were randomly collected from two to three local breeding farms in their province (Figure 1A). The 10 breeds included an indigenous CM breed from the farms of Shanghai Academy of Agricultural Sciences (SAAS), which were located on the Chongming Island of Shanghai. The other nine breeds were from the following regions of China: commercial Boer goats (Bor) from Jiangsu Province; Haimen goats (HM) from Jiangsu Province; Xuhuai goats (XH) from Jiangsu Province; Matou goats (MT) from Hubei Province; Da’er goats (DE) from Sichuan Province; Nanjiang yellow goats (NJ) from Sichuan Province; Macheng black goats (MB) from Hubei Province; Yichang white goats (YC) from Yichang City, Hubei Province; and Qin goats (QS) from Jining City, Shandong Province. The phenotypic descriptions of these 10 breeds can be found in Supplementary Data S1.
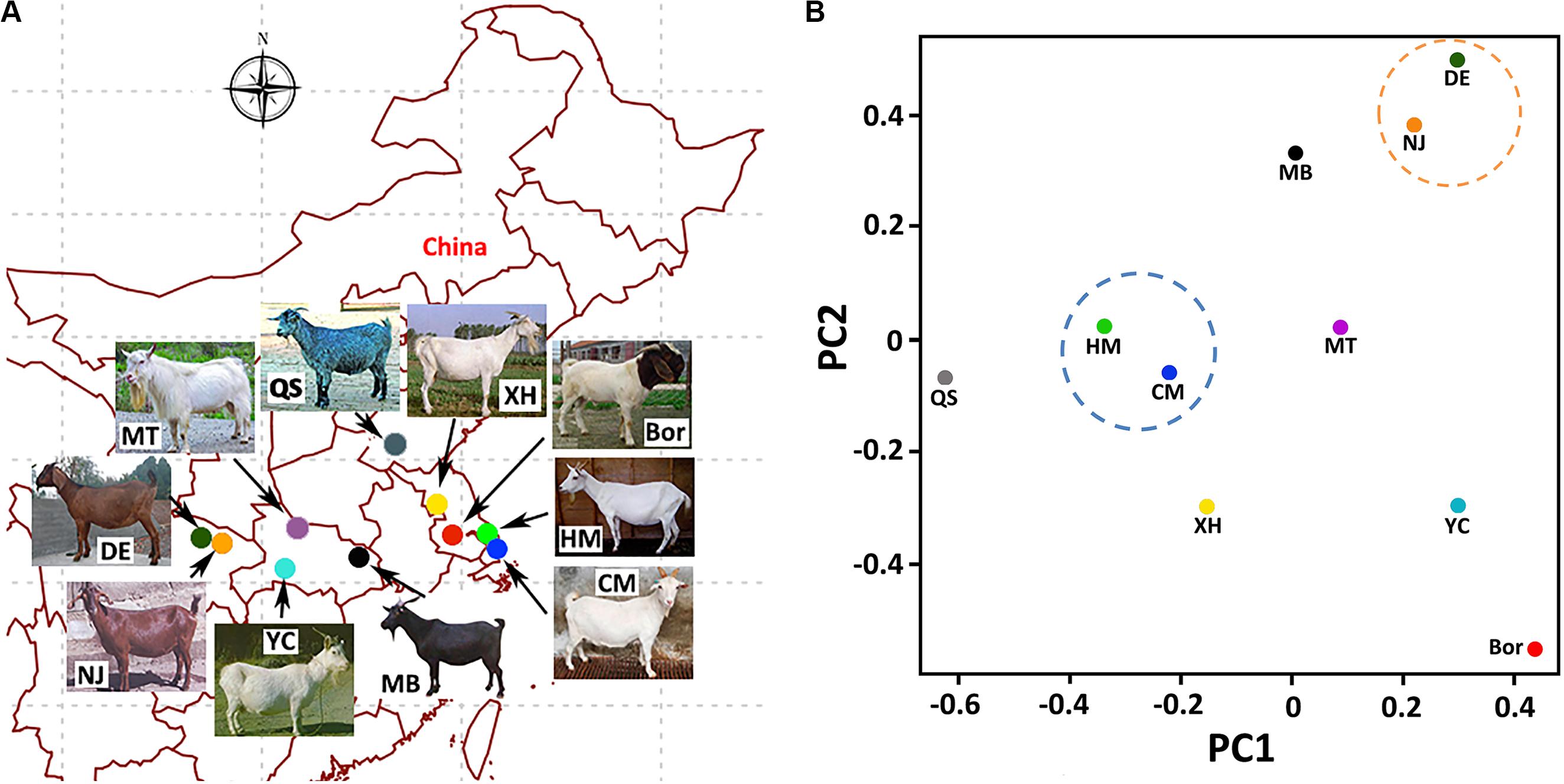
Figure 1. Sampling information and principal component analysis (PCA) of the 10 breeds. (A) Goat breeds and sampling locations. (B) Plot of the first two principal components (PC1 and PC2) of the 10 breeds on the basis of the detected single-nucleotide polymorphisms (SNPs).
Genomic DNA Extraction and Whole-Genome Sequencing
The genomic DNA was extracted from goat ear punches using the cetyl trimethylammonium bromide (CTAB) method (Thomas et al., 1997). DNA isolated from 10 animals per breed was pooled evenly (by μg of DNA) into a single sample. We ensured that the qualities of all the DNA samples were recorded as given OD260/280 = 1.8∼2.0 and OD260/230 ≧ 2.0. One microgram of pooling DNA was used for library preparation using the TruSeqTM Nano DNA Library Prep Kit (Illumina, San Diego, CA, United States) according to the manufacturer’s guidelines. Briefly, the qualified DNA was randomly fragmented using ultrasound-based strategy (Larguinho et al., 2010). The fragments were then subjected to end-repair followed by adaptor ligation to the fragments. Fragments of ∼400 bp in length were selected, enriched by magnetic beads, and further amplified by polymerase chain reaction. High-throughput sequencing was performed using the Illumina HiSeqTM platform (2 × 150 bp read length). The library preparation and sequencing were completed by Shanghai Majorbio Company, China1.
Raw Data Quality Control and Filtering
The raw reads underwent the following filter to get the clean data: (1) remove adaptor sequences; (2) remove bases containing non-AGCT at the 5’ end; (3) trim the end of the reads with the sequencing quality score less than Q20; (4) remove reads containing N > 10%; and (5) discard reads with length less than 25 bp after above filtering.
After the quality control and filtering, the clean reads were summarized with the following statistics: (1) number of the reads; (2) number of the bases; (3) percentages of Q30 bases; (4) average and median insertion size; and (5) average sequencing depth.
Genomic Alignment and Variant Calling
We used the San Clemente goat’s WGS (ARS1) (Bickhart et al., 2017) as the mapping reference genome2, which is the latest de novo assembled sequence (Capra hircus). The clean reads were mapped against the ARS1 by using Burrows Wheeler Alignment (BWA v0.7.15) (Li and Durbin, 2009) with default parameters. We used the Picard v2.23.13 to remove duplicate reads and the GATK (v3.8.0) (McKenna et al., 2010) to call variants (SNPs and indels). The VCFtools v0.1.15 (Li et al., 2009) was used for variant filtering. More precisely, SNPs and indels were filtered by mapping quality >50 and sequencing depth ≧ 10. The BreakDancer v1.3.6 (Chen et al., 2009) was applied to detect the SVs. Variant annotation was conducted with the Ensembl Variant Effect Predictor (VEP4) (McLaren et al., 2016). The Sorting Intolerant from Tolerant (SIFT) was used for predicting whether an amino acid substitution affects protein function on the basis of sequence homology and the physical properties of amino acids (Sim et al., 2012).
Gene Functional Enrichment Analysis
Gene set enrichment analysis was performed at g: Profiler5, a web server for functional enrichment analysis and conversions of gene lists (Raudvere et al., 2019). For gene ontology (GO; biological processes) and Kyoto Encyclopedia of Genes and Genomes (KEGG) pathway, the goat genomic annotation was used. For phenotype ontology, since this information is not available for goat, we used human phenotype ontology (HP) instead to perform the analysis. The P-value generated from the test was automatically adjusted to account for multiple comparisons using the Benjamini and Hochberg correction (Benjamini and Hochberg, 1995). The false discovery rate (FDR) < 0.05 was required to determine the genes that are significantly overrepresented in those categories.
Principal Component Analysis and Detection of Selection Signature Genes
The SNP variant file was transformed to PLINK PED format via PLINK v1.9 (Purcell et al., 2007) to examine the genetic relationships with principal component analysis (PCA) among the 10 goat breeds.
We used two approaches include FST and pooled heterozygosity (Hp) to identify the selection signature differences between the genomes of the CM and the other goat breeds. The weighted population pairwise FST values were calculated for each SNP according to the formula of , in which S2 represents the sampling variance of allele frequencies between two breeds and represents the overall average allele frequency across breeds (Weir and Cockerham, 1984; Guo et al., 2018). FST values were further averaged over SNPs using a 100-kb sliding window with a 50-kb step size and Z-transformed as follows: ZFST = (FST−μFST)/σFST, where μFST and σFST are the mean and standard deviation for the FST, respectively. This analysis was done with popoolation2 v1.201 (Kofler et al., 2011) and R package v3.5.1 (R Core Team, 2013).
The identification of selective sweeps was performed by calculating the ZHp for each pool and SNP. The numbers of reads corresponding to the major (nMaj) and minor (nMin) allele frequencies and for each window in each breed pool, the Hp score is calculated according to the formula of Hp = 2 ∑nMaj∑nMin/(∑nMaj + ∑nMin)2, where ∑nMaj, and ∑nMin are the sums of nMaj and nMin for all SNPs in the window. Individual Hp values were Z transformed as follows: ZHp = (Hp - μHp)/σHp, where μHp and σHp are the mean and standard deviation for the Hp, respectively. Consistent with the FST, we calculated the ZHp value using a 100-kb sliding window with a 50-kb step size.
Windows with extremely high FST values (99% percentile) and low ZHp scores (1% percentile) were proposed to be under selection. The genome-wide scan results of ZFST and ZHp can be found in Supplementary Data S2, S3.
Results
Read Mapping and Variant Identification
In this study, genome sequencing yielded a total of 652.25 Gb of raw data from the 10 goat breeds. Approximately 195.6 (Bor) to 253 (MT) million clean reads were obtained after quality control and filtration. More than 97.5% clean reads were mapped against to the reference genome ARS1 with 5× coverage of ∼94%. The average sequencing depth ranges from 14.24 × (YC) to 20.3 × (QS). These results indicated that high-quality sequences were obtained (Table 1).
A total of 23,508,551 SNPs and 2,830,800 indels were detected, out of which 12,939,052 SNPs and 1,571,878 indels were identified in the CM breed. The number of SNPs in the other nine breeds ranged from 11,871,653 (Bor) to 13,597,084 (MT). Similarly, the lowest number (1,486,903) of indels was detected in the Bor breed and the largest number (1,704,999) in the MT breed. By comparing the other nine breeds, we further identified 1.2% SNPs (271,713) and 0.9% indels (24,843) unique to the CM breed. The other nine breeds’ specific variants ranged from 259,098 (MB) to 540,055 (QS) for SNPs, and 26,031 (NJ) to 59,999 (QS) for indels (Table 2).
Based on the PCA results (Figure 1B), the CM breed showed the closest relationship with the HM breed. The Bor breed demonstrated the most divergent genetic relationships with the CM breed. This is consistent with the fact that the Bor goat is originated from South African regions and was introduced domestically as an excellent commercial meat goat (Malan, 2000). The DE and NJ breeds are found in the Sichuan Province of China and are clustered together on the PCA map. This was also in line with our expectations.
We calculated genome-wide SNP distributions, counted in 100-kb non-overlapping windows, to explore the highly polymorphic regions for the goat breeds. The results demonstrated the average SNP density was ∼5 SNPs/kb among all the 10 breeds. We identified five common high-polymorphic regions with average SNP density >15 SNPs/kb, including 66.3–66.8 Mb on chromosome (Chr) 3, 77.9–78.8 Mb on Chr 10, 14.8–16.2 Mb on Chr 12, 35.9–36.4 Mb on Chr 15, and 23–23.7 Mb on Chr 23 (Figure 2A). For the breed-specific SNPs, the average density was approximately 0.13 SNPs/kb, and only Chr 12: 14.8–16.2 Mb region shows similar high-polymorphic characteristics among the 10 breeds (Figure 1B). Moreover, several breed-specific, highly polymorphic regions were identified, such as 116.2–116.7 Mb on Chr 1 (average density >2.6 SNPs/kb) in Bor, 36.3–36.7 Mb on Chr 15 in CM (average density >1.4 SNPs/kb), and 79.6–80.3 Mb on Chr 6 (average density >3.5 SNPs/kb) in HM (Figure 2B).
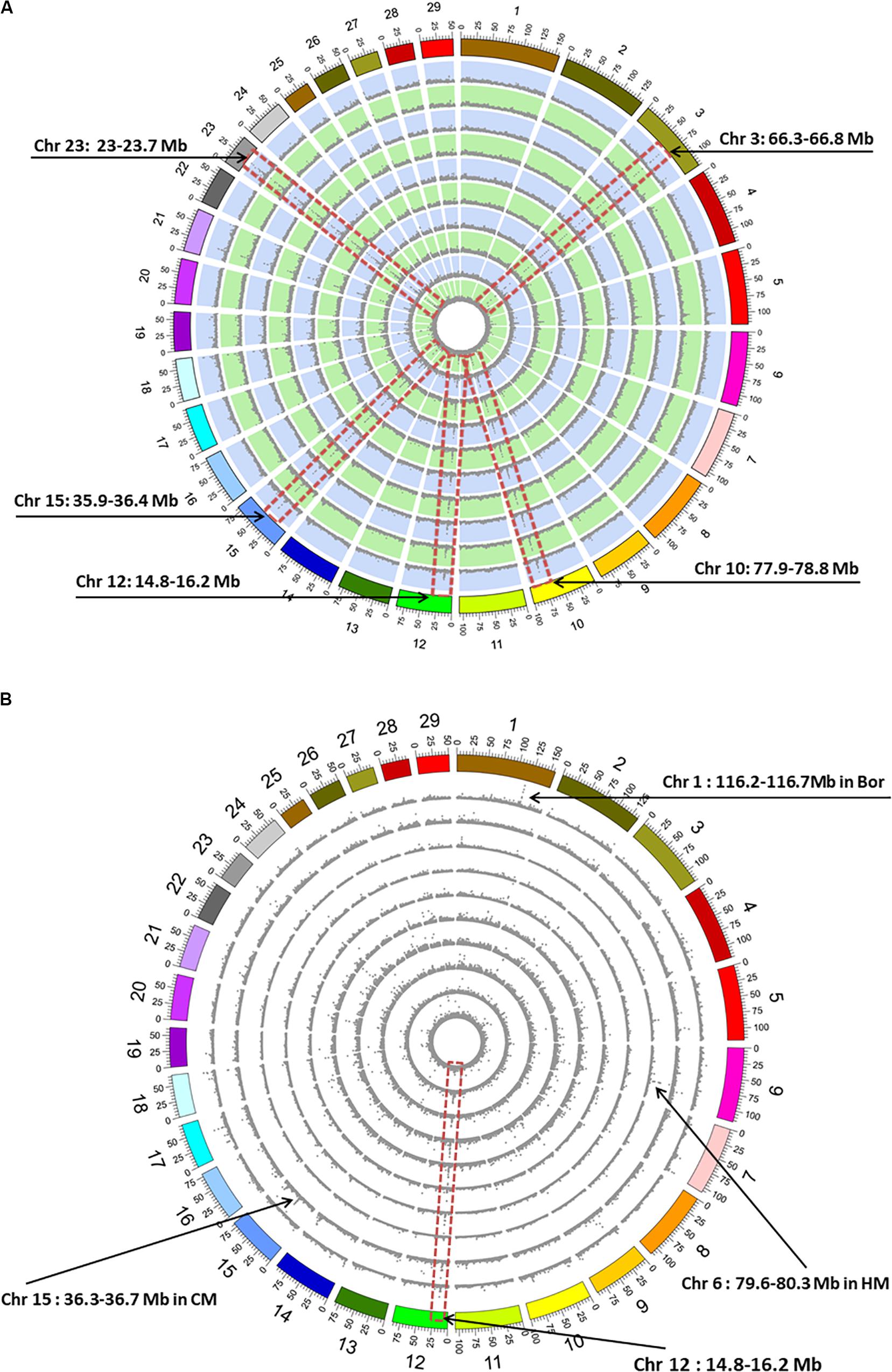
Figure 2. Genome-wide distribution histograms of single-nucleotide polymorphism (SNP) density counted with 100-kb non-overlapping windows. (A) SNP density. (B) Breed-specific SNP density. The outermost circle represents the reference genome (ARS1). The circles from outermost to innermost show the genome-wide distribution of SNP density for the Bor, CM, HM, XH, QS, MB, MT, NJ, DE, and YC breeds. The red dotted frame indicates the consistent SNP high-density location on a specific chromosome (Chr). Mb, megabase; Bor, commercial Boer goats; CM, Chongming white goat; HM, Haimen goats; XH, Xuhuai goats; QS, Qin goats; MB, Macheng black goats; MT, Matou goats; NJ, Nanjiang yellow goats; DE, Da’er goats; YC, Yichang white goats.
Variant Annotation
We performed the variant annotation using VEP, which indicated that 40.9% of variants (SNPs and indels) were located in intergenic regions and the rest in genic regions, including introns (44.5%), upstream (4.6%), and downstream (4.1%) (Figure 3A). Of the total variants identified, ∼0.7% were coding consequence variants (271,960). Of these, 57.7% were synonymous variants (156,873); 38.7% were missense variants (105,257); 1.2% were coding sequence variants (3,371); 1% were frameshift variants (2,816); and ∼0.45% were stop-gained variants (1,243) (Figure 3B). Among the missense variants, ∼15,000 predicted having deleterious effects on protein structure [Sorting Intolerant from Tolerant (SIFT) < 0.05].
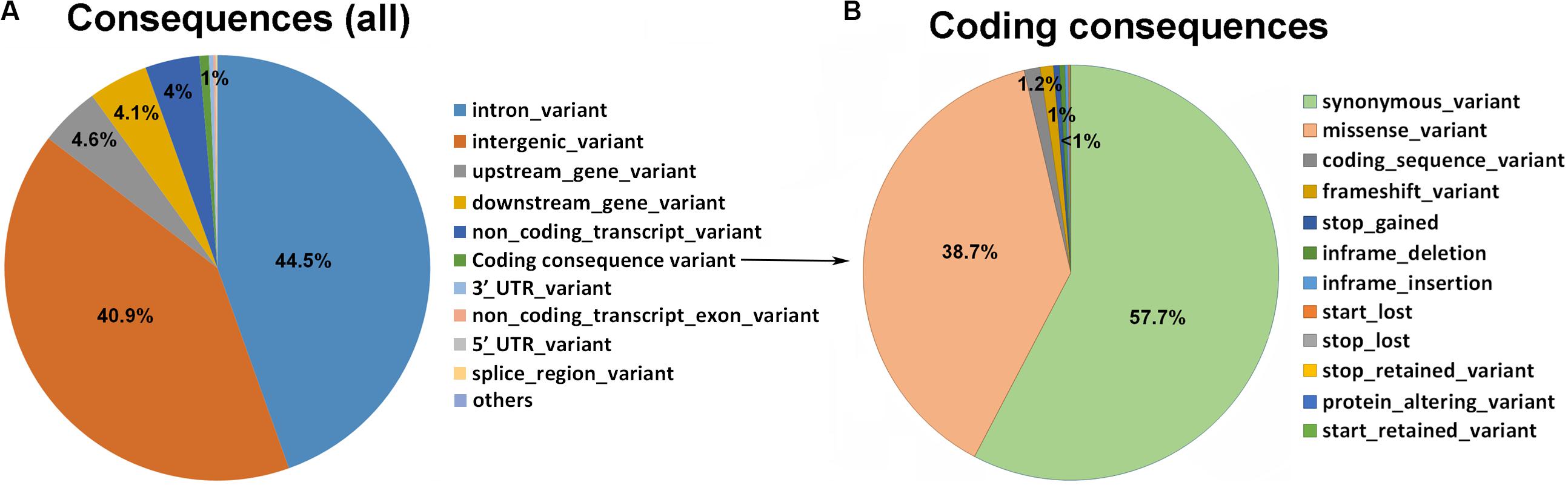
Figure 3. Statistics regarding the variant types of the goat whole genome using VEP Ensemble gene annotation. (A) Pie plot of the total variant annotation. (B) Pie plot of the coding consequence variant annotation.
Filtering resulted in 4,703 genes that harbor ∼20,000 loss-of-function (LoF) variants, including missense (SIFT) < 0.05, frameshift, start-loss, stop-gain or stop-loss, and splice-site variants (Supplementary Data S4). To further understand the function and pathways, we submitted those genes to g: Profiler and performed gene set enrichment analysis. Those genes significantly enriched in the GO terms of biological process (FDR = 9.85E-78), cellular process (FDR = 4.68E-29), and biological regulation (FDR = 7.75E-23). The KEGG pathways (Figure 4A) highlighted the genes significantly enriched in the extracellular matrix (ECM)–receptor interactions (FDR = 1.12E-13), focal adhesion (FDR = 4.47E-7), and metabolic pathways (FDR = 1.69E-6). Phenotype ontology (Figure 4B) suggests these genes are involved in nervous system physiology (FDR = 9.161E-50), skeletal morphology (FDR = 5.39E-37), and the cardiovascular system (FDR = 6.80E-34).
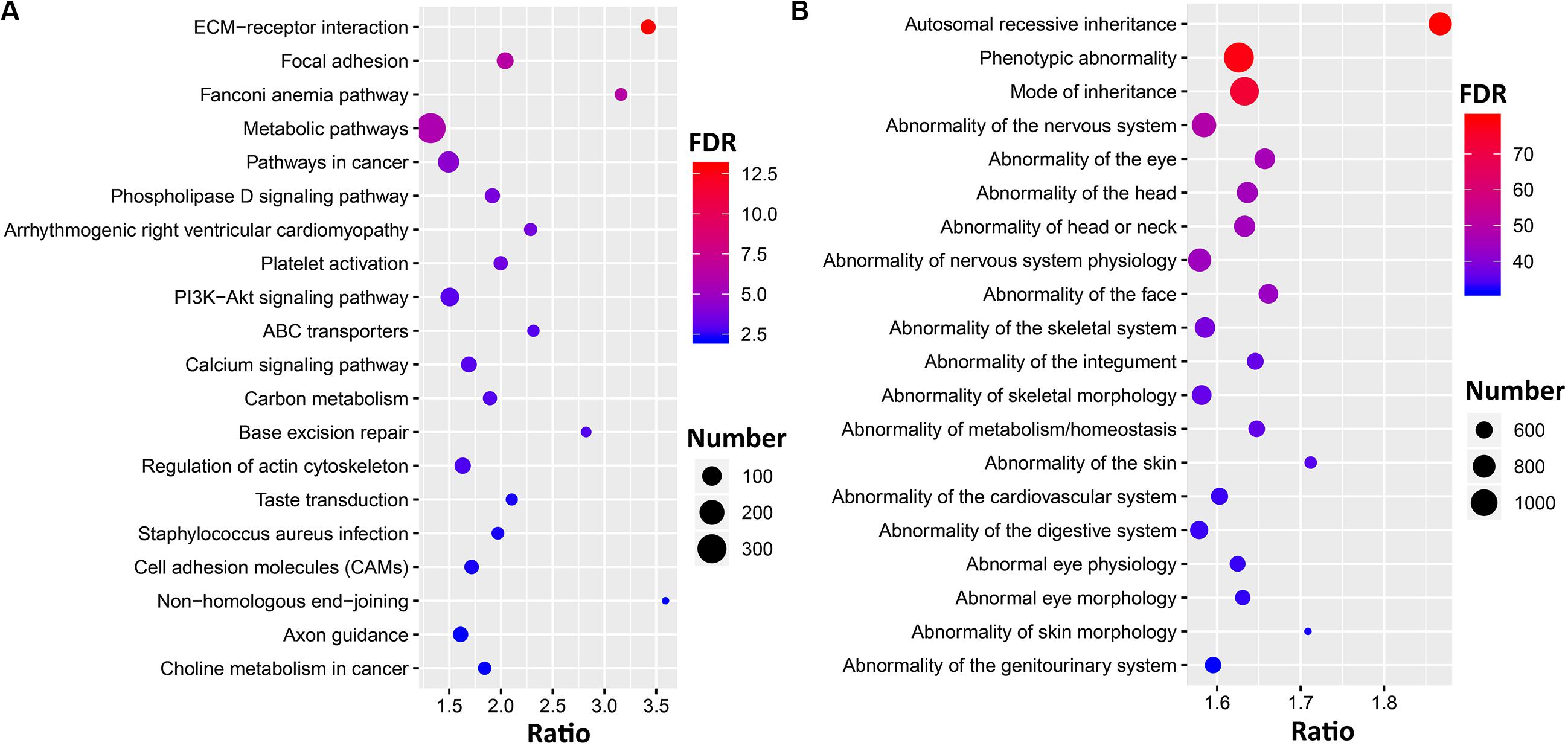
Figure 4. Gene set enrichment analysis for the genes with loss-of-function variants. Bubble plots showing the top 20 significant results [false discovery rate (FDR) < 0.05] for Kyoto Encyclopedia of Genes and Genomes (KEGG) pathways (A) and human phenotype ontology (HP) terms (B). The circles represent the number of enriched genes, and the colors represent log10 transformed FDR values.
Candidate Coding Functional Genes and Related Phenotypes in the Chongming White Goat Breed
The CM breed is our research of interest. Thus, we aimed to further investigate the phenotypes related to the CM-specific genes with LoF variants. In total, 351 LoF variants in 183 coding genes were identified (Supplementary Data S5). We performed the phenotype enrichment analysis for those genes on g: Profiler and obtained 338 HP terms with FDR < 0.05. Next, we filtered the genes with those involved in more than 50 HP terms. This resulted in 36 out of 183 genes. The top 50 phenotype terms related to those 36 genes are listed in Figure 5. Of note, all the genes participated in regulation of the nervous system (HP:0000707, FDR = 0.0002). Furthermore, most of the genes were associated with several other phenotype terms. For instance, 20 genes (e.g., SYNE2, AP4E1, and KBTBD13) were enriched in the “skeletal muscle morphology” (HP:0011805, FDR = 0.0016); 18 genes (e.g., AP4E1, and FSHR, and Col11a2) involved in the “short stature” (HP:0004322, FDR = 0.0025) and “body height” (HP:000002, FDR = 0.0025); and 15 (e.g., IFT172, NALCN, and IARS2) genes are found in the terms of “ear morphology” (HP:0031703, FDR = 0.001106) and “hearing” (HP:0000364, FDR = 0.00098), simultaneously.
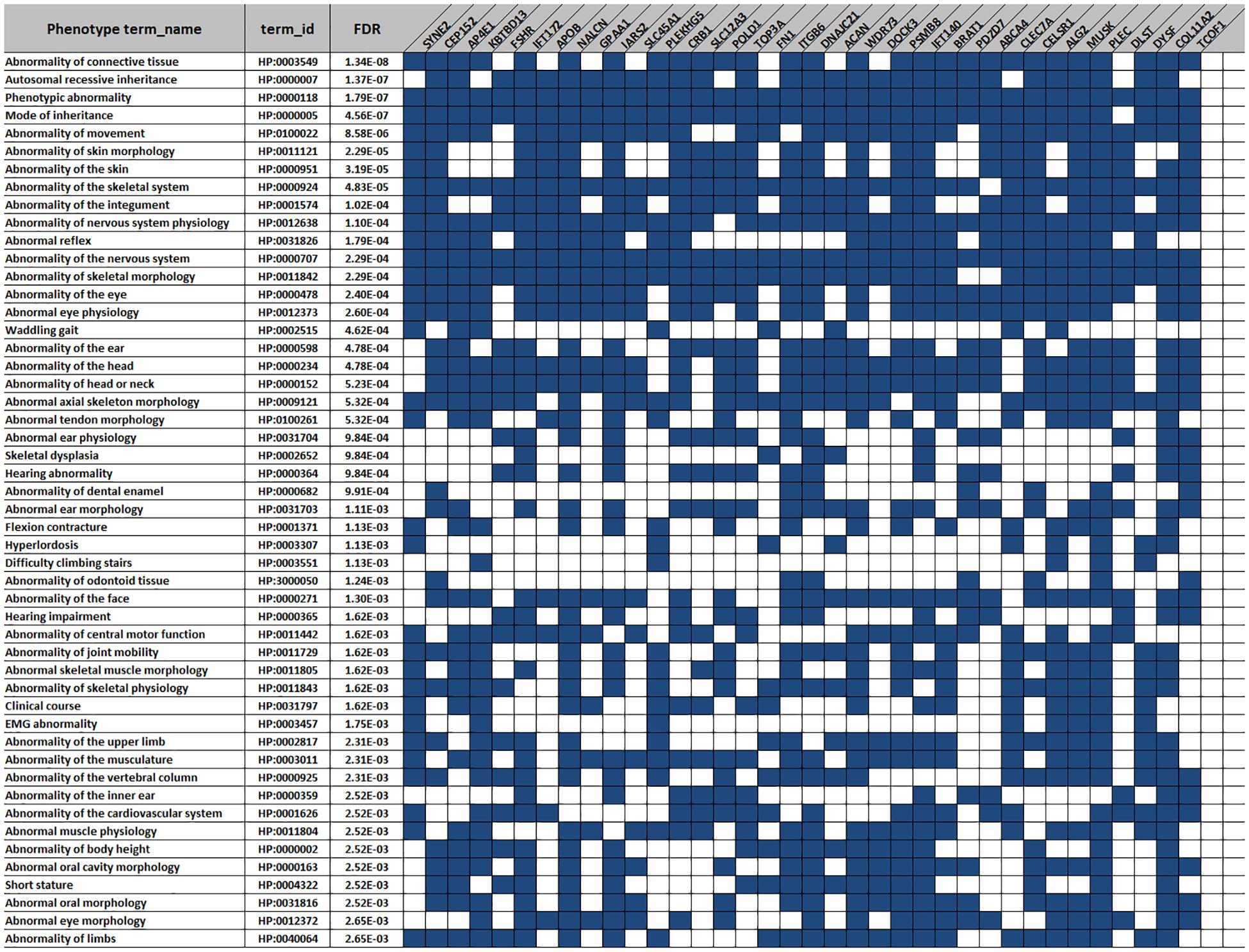
Figure 5. The top 50 phenotype terms for the 36 Chongming white goat (CM) breed-specific genes with the loss-of-function (LoF) variants. Blue squares indicate the genes (columns) involved in the specific phenotype terms (rows).
Selection Signature Genes
Co-selection Signature Genes
The CM goats are domesticated under the natural conditions of Chongming Island in Shanghai. In order to detect the genome-wide selection signatures, we calculated FST values between the CM and each of the other nine breeds using a 100-kb sliding widow with a step size of 50 kb. The 99% percentile Z score transformed FST values for each comparison was calculated and averaged (ZFST = 3.27). This value was used as the selection signature cutoff threshold. On the basis of this, we identified hundreds of genes that underwent selection, ranging from 228 between CM and YC to 330 between CM and DE. Of those, 37 genes were observed having co-selection signatures in more than four comparisons. In addition to FST, we also calculated the ZHp scores for each breed and identified 16 out of the 37 co-selection genes with low ZHp scores < −3 (1% percentile, Figure 6). Of those, seven genes (C2CD3, DNAJB13, UCP2, ZMYND11, CEP126, SCAPER, and TSHR) are related to six nervous system-related terms (HP:0012758, HP:0012759, HP:0000707, HP:0012639, HP:0012638, and HP:0002011). Four genes (UCP2, UCP3, SCAPER, and TSHR) are related to the body weight (HP:0004324 and HP:0004323), and four genes (C2CD3, UCP2, ZMYND11, and TSHR) are involved in modulating the phenotype of globe developmental delay (HP:0001263).
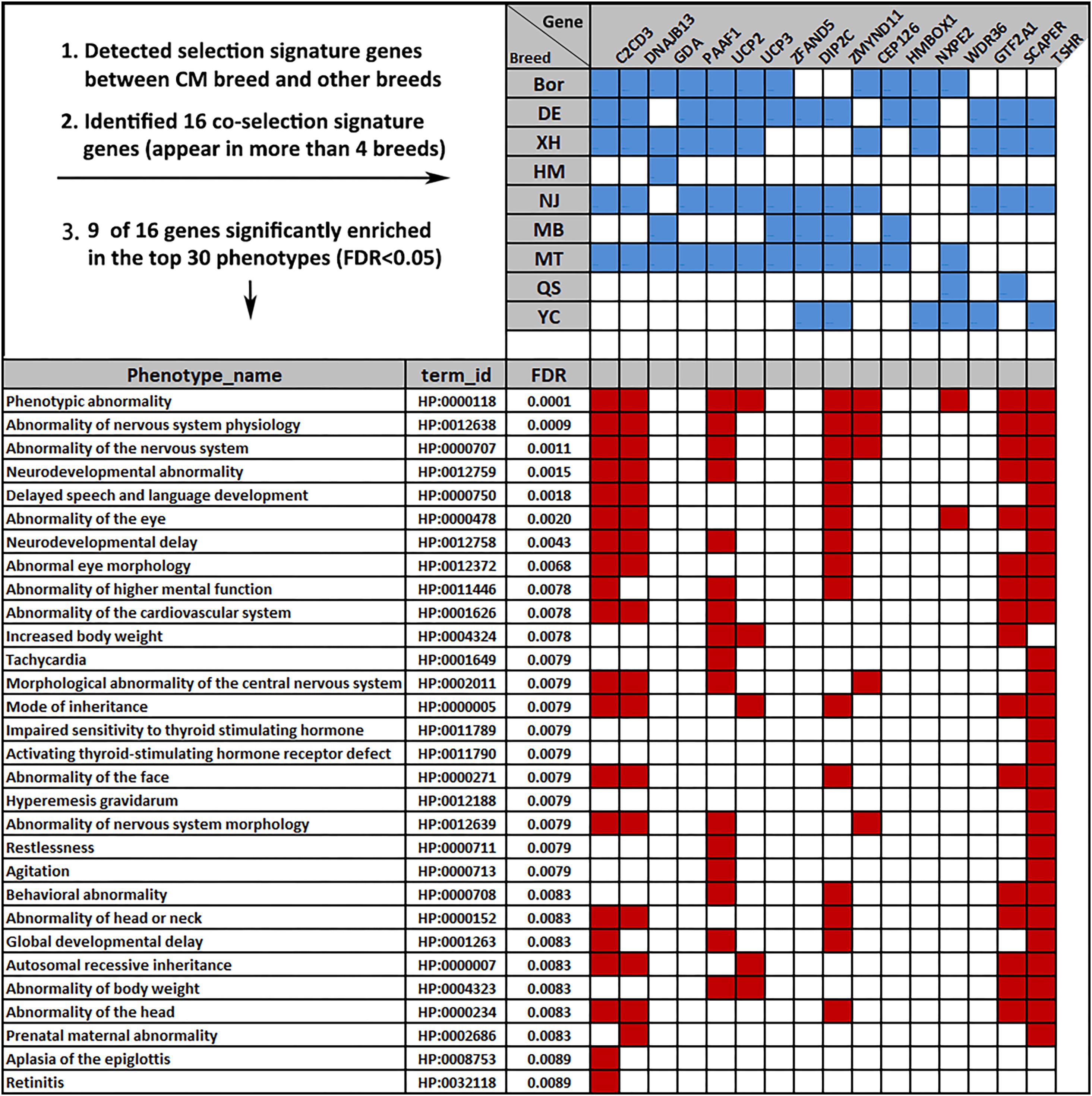
Figure 6. Phenotype overview of the 16 co-selection signature genes. Blue square indicates genes appearing in more than four of 10 breeds [false discovery rate (FDR) < 0.05]. Red squares indicate the nine genes enriched in the top 30 phenotype terms.
Notably, both FST and ZHp indicate that the 29.46–29.65 Mb region on Chr 15 has a strong selection signature in both the CM and HM breeds (Figure 7). This region contains five genes, with C2CD3, DNAJB13, and UCP2 involved in six phenotypes of the nervous system including abnormality of nervous system physiology (HP:0012638), abnormality of nervous system (HP:0000707), neurodevelopmental abnormality (HP:0012759), neurodevelopmental delay (HP:0012758), morphological abnormality of the central nervous system (HP:0002011), and abnormality of nervous system morphology (HP:0012639).
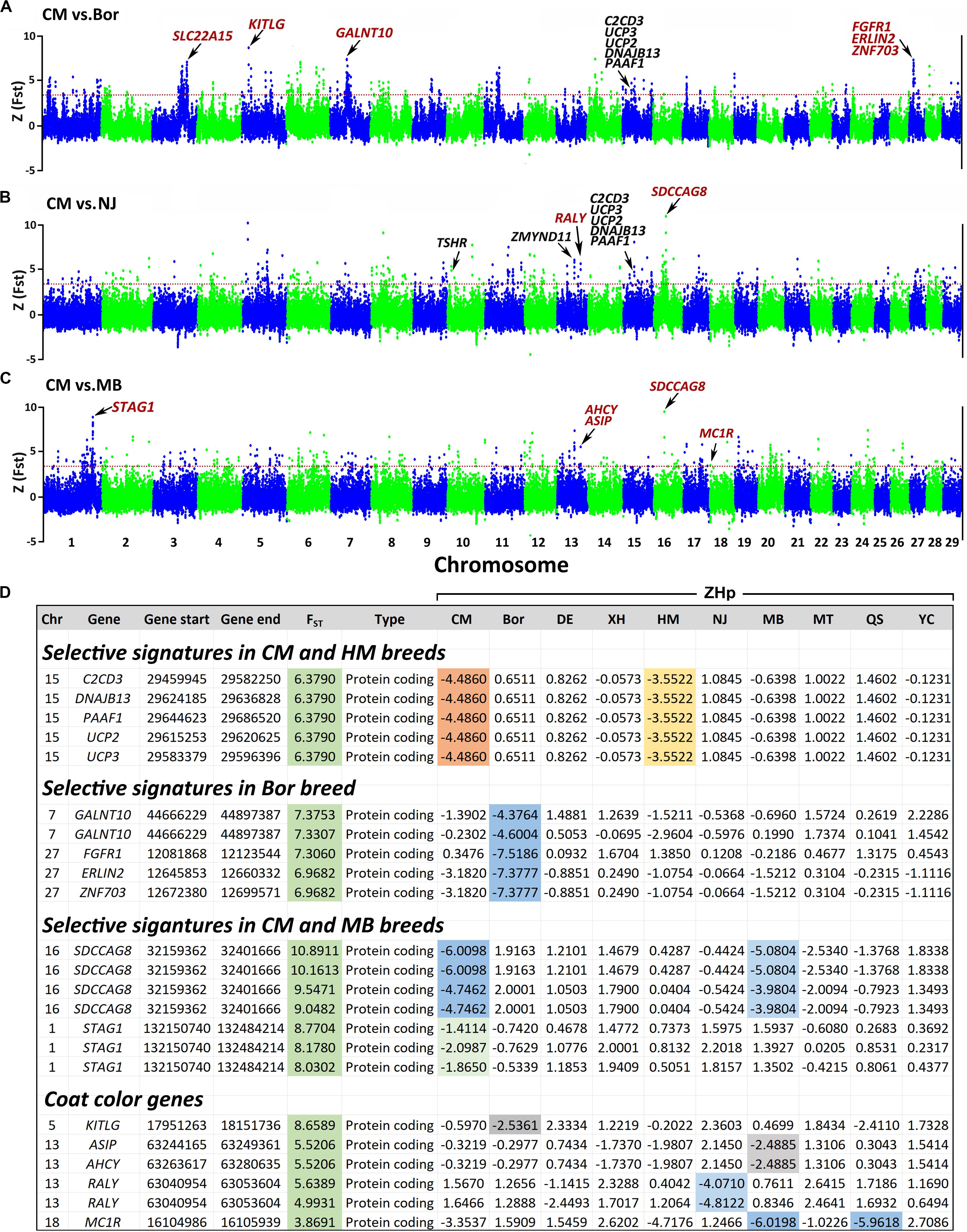
Figure 7. Genome-wide distributions of selection signatures in the CM vs Bor (A), CM vs NJ (B), and CM vs MB (C). ZHp scores of selection genes across the breeds (D). The ZFST and ZHp calculated for each sliding 100-kb window with steps of 50 kb across all autosomes. The red horizontal dashed line indicates the ZFST threshold value of 3.27. CM, Chongming white goat; Bor, commercial Boer goats; NJ, Nanjiang yellow goats; MB, Macheng black goats.
Unique Selection Signature Genes in Chongming White Goat Breed
Through pairwise FST comparisons between the CM and the other breeds, we also identified several unique selection genes such as SLC22A15, KITLG, GALNT10, FGFR1, ERLIN2, and ZNF703 in CM vs Bor (Figure 7A). Among the notable ones are FGFR1, ERLIN2, and ZNF703 located on Chr 27 at 12–12.7 Mb, which is a unique strong selection signal with high FST value (>7) and low ZHp scores (<-7.4) only observed in the Bor breed. Moreover, SDCCAG8 and STAG1 with high ZFST values and low ZHp scores were detected in the CM and MB breeds. Five coat color-related genes (KITLG, ASIP, AHCY, RALY, and MC1R) were identified in the Bor, NJ, and MB breeds (Figure 7). The KITLG gene located on Chr 5 at 17.95–18.15 Mb showed a unique selective signal with FST value of 8.66 and ZHp score of −2.54 in Bor. ASIP, AHCY, and RALY on Chr 13 at 63–63.25 Mb demonstrated with similar FST values of ∼5.5. The −2.49 ZHp score of ASIP and AHCY in the MB breed and −4.81 ZHp score of RALY in the NJ breed were detected. In addition, MC1R with FST = 3.87 and ZHp = −6 in the MB and QS breeds were identified.
Discussion
Genetic and Genomic Studies of the Goat
In this study, we used high-throughput sequencing technologies to perform WGS for 10 goat breeds, pooling libraries from 100 goat individuals. After genome-wide mapping and variant calling, we obtained ∼23.5 million SNPs and 2.8 million indels from the 10 goat breeds. By calculating the distribution of genome-wide SNP polymorphisms in the 100-kb non-overlapping window, we identified five common chromosomal regions with high polymorphism on goat genome. The results are consistent with those reported in previous goat genome study (Guo et al., 2018). Moreover, several breed-specific, highly polymorphic regions were identified. Most of the annotated genes in these genomic regions are uncharacterized LOC symbols, and the biological importance of them remains to be elucidated.
Genomic Characteristics of the Chongming White Goat Breed
Many indigenous breeds, which might represent highly valuable biodiversity resources, are threatened with extinction owing to their substitution by cosmopolitan breeds. The CM goat also faces this dilemma. In our study, we identified 271,713 CM-specific SNPs and 24,843 indels, which is the first whole-genome study to the best of our knowledge to characterize genetic polymorphisms in this breed. The results clearly demonstrate that the CM breed has the closest phylogenetic relationship with the HM and the most divergent relationships with the Bor breed. It has also been argued that both the CM and HM breeds belong to the Yangtze River Delta white goat species in China. The phylogenetic results among these breeds are also consistent with the expectation from the geographical distribution.
LoF variants are typically thought to have deleterious effects on protein-coding genes. The effects of these variants upon protein structure and expression in turn lead to phenotypic differences. In this study, we identified 183 genes with the CM breed-specific LoF variants. Out of these 183, 36 genes are enriched in more than 50 phenotype terms, including “nervous system,” “short stature,” and “skeletal muscle morphology” (Figure 5). The genes significantly enriched in the “nervous system” phenotypes, possibly reflecting the fact that the most critical phenotypic changes during the initial steps of animal domestication affect brain and neuronal development. Variants in these genes may produce behavioral traits that ease the domestication process or be a product of domestication itself (Carneiro et al., 2014).
The body size of the CM breed is relatively small (mature weights for bucks and does are 23–32 and 18–25 kg, respectively) compared with the Bor breed (mature weights for bucks and does are 90–130 and 80–100 kg, respectively (Lu, 2001). It is worth noting that 18 genes (e.g., AP4E1, FSHR, and COL11A2) are related to the terms of “short stature” and “body height.” Mutations in the AP4E1 gene are associated with neurodevelopmental disorders in humans, which are characterized by intellectual disability, global developmental delay, and short stature (Jamra et al., 2011; Moreno-De-Luca et al., 2011). Moreover, Ap4e1 mutant mice display a lean body mass phenotype (Eppig et al., 2005). Follicle-stimulating hormone receptor (FSHR) plays a prominent role in mammalian reproduction (Papadimitriou et al., 2016), and ovarian follicle FSHR expression levels in prolific Lezhi black goats were reported to be significantly higher than those in non-prolific Tibetan goats (Zi et al., 2013). In mice, Fshr mutant females have body weights 20% greater than those of wild-type control females (Danilovich et al., 2000). Similarly, homozygote Col11a2 mutant mice are 25% smaller at birth than their wild-type counterparts (Eppig et al., 2005).
In addition, skeletal muscle is a significant contributor to overall body mass and an important structural component of the mammalian body (Berridge et al., 2018). Dysferlin (encoded by DYSF) is a skeletal muscle protein important for organization and structure of the sarcolemma (Spuler et al., 2008). DYSF mutations are associated with muscular dystrophy in humans (Bashir et al., 1998) and mice (Bittner et al., 1999). DYSF has also been identified as an alternatively spliced gene in goat leg muscle, likely playing crucial roles in skeletal muscle development (Xu et al., 2018). A study has revealed several candidate genes associated with goat ear morphology (Brito et al., 2017). In our study, 15 genes were related to the terms of “ear morphology” and “hearing” simultaneously. Therefore, these 36 genes may play important roles in the CM breed-specific phenotypic traits, and study of the biological underpinnings of these genes in relation to the traits is necessary and important.
Selection Signature Genes of the Chongming White Goat Breed
Co-selection Signature Genes
Through calculated FST values, we filtered 37 genes that have co-selection signals, 16 of which had ZHp scores < -3, which indicate that these genes have significant selection signatures with a high degree of confidence. Moreover, seven genes (C2CD3, DNAJB13, UCP2, ZMYND11, CEP126, SCAPER, and TSHR) were significantly enriched in six nervous system-related phenotypes (Figure 6). Some of them were reported to have clear functions in human or mouse studies. For example, ZMYND11 variants were identified in mice with anxiety-like behavior (Parker et al., 2016). Pathogenic variants in ZMYND11 have been associated with intellectual disability, behavioral abnormalities, and seizures in humans (Yates et al., 2020).
The region on Chr 15 at 29.46–29.65 Mb contains five genes (C2CD3, DNAJB13, UCP2, UCP3, and PAAF1), which is a strong selective signal region only in the CM and HM breeds. Given their close phylogenetic relationship, and the fact that three genes (C2CD3, DNAJB13, and UCP2) are implicated in six nervous system-related traits, we have reason to suppose that the nervous system in the CM and HM breeds may differ from that in the other goat breeds. Owing to the lack of sufficient phenotypic information on the nervous system of goats, the contribution of these genes is still unclear and remains to be further elucidated.
As mentioned above, it is of interest to identify genes important for goat growth and development and how these genes differ between breeds. Five selection signature genes (UCP2, UCP3, ZMYND11, SCAPER, and TSHR) are contributing to the phenotype term of “body weight” or “global developmental delay.” Our results suggested that thyroid-stimulating hormone receptor (TSHR) is one of the co-selective signature genes between the CM and the DE, XH, NJ, and YC breeds (Figure 6). TSHR has a pivotal role in metabolic regulation and photoperiod control of reproduction in vertebrates. Studies had identified TSHR under selective pressure in other domestic animals, such as chicken (Rubin et al., 2010) and sheep (Liu et al., 2016). Accumulating evidence suggests that the uncoupling proteins UCP2 and UCP3 have a role in thermogenesis and energy efficiency (Nagy et al., 2004; de Almeida Brondani et al., 2014). Thus, these five genes, which we identified in this study, may play vital roles in the growth, performance, and metabolism of the goat, but the molecular mechanisms have not been fully elucidated yet.
Unique Selection Signature Genes in Chongming White Goat Breed
Some selection signatures were only identified between one and two breeds. There may be a connection between these unique selective signature genes and the traits of a specific breed. For example, Bor goats are excellent for meat production, grow quickly, and have high fertility rates. We detected a unique selective region located on Chr 27 at 12–12.7 Mb, which includes three genes (FGFR1, ERLIN2, and ZNF703) (Figures 7A,D). Genetic studies have placed the FGFR1 gene at the top of major ontogenic pathways that enable gastrulation, tissue development, and organogenesis (Terranova et al., 2015). Moreover, the genomic region that includes ERLIN2 and ZNF703 was reported to be associated with average daily gain (ADG) in Nellore cattle (Olivieri et al., 2016). Although the function of these unique genes and the relationship with the characteristics of the goat breeds are still unclear, these unique strongly selective genes that include SDCCAG8 and STAG1 in the CM and MB breeds are worthy of further study.
Coat Color Genes
Animal coat color is an important trait that is easy to observe. Studies have shown that some coat color genes have been underwent selections. We identified five coat color-related genes that have selection signatures in this study. The KITLG gene shows a strong selection signature in the Bor breed with unique high FST and low ZHp scores (Figures 7A,D). KITLG encodes for the ligand of c-Kit, which is associated with coat color in pigs (Hadjiconstantouras et al., 2008) and UV-protective eye area pigmentation in cattle (Pausch et al., 2012). KITLG genomic region also reported has a significant effect on skin and hair color of humans (Miller et al., 2007; Sulem et al., 2007). RALY was reported to be associated with saddle tan and black-and-tan phenotypes in the dogs (Dreger et al., 2013). The RALY-ELF2S2-ASIP locus has influence on skin and hair pigmentation in the Nanjiang yellow goat (Guo et al., 2018). In the present study, we also detected the selective signal of RALY in the NJ breed, which further emphasizes that the RALY gene may contribute to the tan color of goats. Agouti signaling protein gene (ASIP) result in various coat patterns in domestic mammals6 including mouse (Voisey and Van Daal, 2002), dog (Kerns et al., 2004), cat (Eizirik et al., 2003), rabbit (Fontanesi et al., 2010), horse (Rieder et al., 2001), sheep (Norris and Whan, 2008), and donkeys (Abitbol et al., 2015). It was also studied as a strong candidate gene associated with the black coat color in a Chinese Taihang black goat (Wang et al., 2016). In this study, only the MB breed has an all-black coat, and the results suggest that the ASIP and AHCY genes may contribute to the black coat color of the MB breed. For the MC1R gene, we observed the low ZHp values in the MB (black) and QS breeds (blue gray). The melanocortin one receptor (MC1R) is a melanocytic Gs protein-coupled receptor that regulates skin pigmentation, UV responses, and melanoma risk (Wolf Horrell et al., 2016). Studies have shown that this gene is important for regulation of eumelanin (black/brown) and phaeomelanin (red/yellow) syntheses in mammalian melanocytes (Wang et al., 2016). The coat color of animals may be determined by the combined action of several genes. These five genes are present in varying degrees of selective signatures in our 10 goat breeds, which highlighted that they play important roles in determining the coat color phenotypes of goats.
Conclusion
In this study, by using the WGS method and genomic approaches, we conducted a comparative genomic study for the first time to reveal the genomic characteristics and selection signatures between the CM and the other nine goat breeds. Our results identified 36 genes with the CM breed-specific LoF variants that were significantly enriched in the phenotype terms of “nervous system,” “short stature,” and “skeletal muscle morphology.” From another perspective, hundreds of selection signature genes were identified in this study that are possibly associated with important traits, such as growth (UCP2, UCP3, ZMYND11, SCAPER, TSHR, FGFR1, ERLIN2, and ZNF703), nervous system (C2CD3, DNAJB13, UCP2, ZMYND11, CEP126, SCAPER, and TSHR), and coat color (KITLG, ASIP, AHCY, RALY, and M1CR). The genes with functional mutations and the selection signatures explored in this study both reflect the fact that the nervous system of the CM breed may differ from the other breeds. Although the trait-related genes require further validation through biological experiments, our findings provide an improved understanding for biodiversity conservation and trait exploration for the domestic goats.
Data Availability Statement
Raw sequence data of 10 sheep breeds in the bam format from this study were deposited in the NCBI Short Read Archive (SRA; http://www.ncbi.nlm.nih.gov/sra) under accession number PRJNA608539.
Ethics Statement
The animal study was reviewed and approved by Experimental Animal Management Committee of SAAS.
Author Contributions
JG and JD managed the project. FX and JG carried out the bioinformatics analyses. YL, DZ, FS, JY, CL, HL, and HY collected the samples. JG and FX wrote the manuscript. KR reviewed and edited the English writing. All authors read and approved the final manuscript.
Funding
This project was funded by the Shanghai Committee of Science and Technology (Grant Nos. 17140900100 and 19140900100) and Shanghai Municipal Agricultural and Rural Committee: Youth Talent Project (Shanghai Academy of Agricultural Sciences Nos. 1–36), China.
Conflict of Interest
The authors declare that the research was conducted in the absence of any commercial or financial relationships that could be construed as a potential conflict of interest.
Supplementary Material
The Supplementary Material for this article can be found online at: https://www.frontiersin.org/articles/10.3389/fgene.2020.00901/full#supplementary-material
DATA S1 | Phenotype description of 10 goat breeds.
DATA S2 | ZFST and ZHp results.
DATA S3 | ZFST and ZHp results.
DATA S4 | 4703 genes harbor LoF variants.
DATA S5 | 183 CM specific genes with LoF variants.
Footnotes
- ^ www.majorbio.com
- ^ www.ncbi.nlm.nih.gov/assembly/GCF_001704415.1
- ^ https://broadinstitute.github.io/picard
- ^ https://useast.ensembl.org/Tools/VEP
- ^ https://biit.cs.ut.ee/gprofiler/gost
- ^ http://omia.angis.org.au
References
Abitbol, M., Legrand, R., and Tiret, L. (2015). A missense mutation in the agouti signaling protein gene (ASIP) is associated with the no light points coat phenotype in donkeys. Genet. Select. Evol. 47:28.
Bashir, R., Britton, S., Strachan, T., Keers, S., Vafiadaki, E., Lako, M., et al. (1998). A gene related to Caenorhabditis elegans spermatogenesis factor fer-1 is mutated in limb-girdle muscular dystrophy type 2B. Nat. Genet. 20, 37–42. doi: 10.1038/1689
Benjamini, Y., and Hochberg, Y. (1995). Controlling the false discovery rate: a practical and powerful approach to multiple testing. J. R. Statist. Soc. Ser. B 57, 289–300. doi: 10.1111/j.2517-6161.1995.tb02031.x
Benjelloun, B., Alberto, F. J., Streeter, I., Boyer, F., Coissac, E., Stucki, S., et al. (2015). Characterizing neutral genomic diversity and selection signatures in indigenous populations of Moroccan goats (Capra hircus) using WGS data. Front. Genet. 6:107. doi: 10.3389/fgene.2015.00107
Berridge, B. R., Bolon, B., and Herman, E. (2018). “Skeletal muscle system,” in Fundamentals of Toxicologic Pathology, eds M. A. Wallig, W. M. Haschek, C. G. Rousseaux, and B. Bolon (Cambridge, MA: Academic Press), 195–212.
Bickhart, D. M., Rosen, B. D., Koren, S., Sayre, B. L., Hastie, A. R., Chan, S., et al. (2017). Single-molecule sequencing and chromatin conformation capture enable de novo reference assembly of the domestic goat genome. Nat. Genet. 49, 643–650. doi: 10.1038/ng.3802
Bittner, R. E., Anderson, L. V., Burkhardt, E., Bashir, R., Vafiadaki, E., Ivanova, S., et al. (1999). Dysferlin deletion in SJL mice (SJL-Dysf) defines a natural model for limb girdle muscular dystrophy 2B. Nat. Genet. 23, 141–142. doi: 10.1038/13770
Brito, L. F., Kijas, J. W., Ventura, R. V., Sargolzaei, M., Porto-Neto, L. R., Cánovas, A., et al. (2017). Genetic diversity and signatures of selection in various goat breeds revealed by genome-wide SNP markers. BMC Genom. 18:229. doi: 10.1186/s12864-017-3610-0
Carneiro, M., Rubin, C.-J., Di Palma, F., Albert, F. W., Alföldi, J., Barrio, A. M., et al. (2014). Rabbit genome analysis reveals a polygenic basis for phenotypic change during domestication. Science 345, 1074–1079. doi: 10.1126/science.1253714
Chen, K., Wallis, J. W., Mclellan, M. D., Larson, D. E., Kalicki, J. M., Pohl, C. S., et al. (2009). BreakDancer: an algorithm for high-resolution mapping of genomic structural variation. Nat. Methods 6, 677–681. doi: 10.1038/nmeth.1363
Danilovich, N., Babu, P. S., Xing, W., Gerdes, M., Krishnamurthy, H., and Sairam, M. R. (2000). Estrogen deficiency, obesity, and skeletal abnormalities in follicle-stimulating hormone receptor knockout (FORKO) female mice. Endocrinology 141, 4295–4308. doi: 10.1210/endo.141.11.7765
de Almeida Brondani, L., De Souza, B. M., Assmann, T. S., Bouças, A. P., Bauer, A. C., Canani, L. H., et al. (2014). Association of the UCP polymorphisms with susceptibility to obesity: case-control study and meta-analysis. Mol. Biol. Rep. 41, 5053–5067. doi: 10.1007/s11033-014-3371-7
Dreger, D. L., Parker, H. G., Ostrander, E. A., and Schmutz, S. M. (2013). Identification of a mutation that is associated with the saddle tan and black-and-tan phenotypes in Basset Hounds and Pembroke Welsh Corgis. J. Hered. 104, 399–406. doi: 10.1093/jhered/est012
Eizirik, E., Yuhki, N., Johnson, W. E., Menotti-Raymond, M., Hannah, S. S., and O’brien, S. J. (2003). Molecular genetics and evolution of melanism in the cat family. Curr. Biol. 13, 448–453. doi: 10.1016/s0960-9822(03)00128-3
Eppig, J. T., Group, M. G. D., Bult, C. J., Group, M. G. D., Kadin, J. A., Group, M. G. D., et al. (2005). The mouse genome database (MGD): from genes to mice—a community resource for mouse biology. Nucleic Acids Res. 33, D471–D475.
Fontanesi, L., Forestier, L., Allain, D., Scotti, E., Beretti, F., Deretz-Picoulet, S., et al. (2010). Characterization of the rabbit agouti signaling protein (ASIP) gene: transcripts and phylogenetic analyses and identification of the causative mutation of the nonagouti black coat colour. Genomics 95, 166–175. doi: 10.1016/j.ygeno.2009.11.003
Futschik, A., and Schlötterer, C. (2010). The next generation of molecular markers from massively parallel sequencing of pooled DNA samples. Genetics 186, 207–218. doi: 10.1534/genetics.110.114397
Guo, J., Tao, H., Li, P., Li, L., Zhong, T., Wang, L., et al. (2018). Whole-genome sequencing reveals selection signatures associated with important traits in six goat breeds. Sci. Rep. 8, 1–11.
Hadjiconstantouras, C., Sargent, C., Skinner, T., Archibald, A., Haley, C., and Plastow, G. (2008). Characterization of the porcine KIT ligand gene: expression analysis, genomic structure, polymorphism detection and association with coat colour traits. Anim. Genet. 39, 217–224. doi: 10.1111/j.1365-2052.2008.01708.x
Hivert, V., Leblois, R., Petit, E. J., Gautier, M., and Vitalis, R. (2018). Measuring genetic differentiation from Pool-seq data. Genetics 210, 315–330. doi: 10.1534/genetics.118.300900
Huang, X., Chen, Z., Wang, K., He, P., Li, F., Zhang, F., et al. (2013). A modified goat model of acute spinal cord compression injury from a percutaneous balloon catheter: method feasibility and preliminary observation. Zhonghua Yi Xue Za Zhi 93, 2993–2996.
Jamra, R. A., Philippe, O., Raas-Rothschild, A., Eck, S. H., Graf, E., Buchert, R., et al. (2011). Adaptor protein complex 4 deficiency causes severe autosomal-recessive intellectual disability, progressive spastic paraplegia, shy character, and short stature. Am. J. Hum. Genet. 88, 788–795. doi: 10.1016/j.ajhg.2011.04.019
Kerns, J. A., Newton, J., Berryere, T. G., Rubin, E. M., Cheng, J.-F., Schmutz, S. M., et al. (2004). Characterization of the dog Agouti gene and a nonagoutimutation in German Shepherd Dogs. Mammal. Genome 15, 798–808. doi: 10.1007/s00335-004-2377-1
Kim, J.-Y., Jeong, S., Kim, K. H., Lim, W.-J., Lee, H.-Y., and Kim, N. (2019). Discovery of genomic characteristics and selection signatures in korean indigenous goats through comparison of 10 goat breeds. Front. Genet. 10:699. doi: 10.3389/fgene.2015.00699
Kofler, R., Pandey, R. V., and Schlötterer, C. (2011). PoPoolation2: identifying differentiation between populations using sequencing of pooled DNA samples (Pool-Seq). Bioinformatics 27, 3435–3436. doi: 10.1093/bioinformatics/btr589
Larguinho, M., Santos, H. M., Doria, G., Scholz, H., Baptista, P. V., and Capelo, J. L. (2010). Development of a fast and efficient ultrasonic-based strategy for DNA fragmentation. Talanta 81, 881–886. doi: 10.1016/j.talanta.2010.01.032
Li, H., and Durbin, R. (2009). Fast and accurate short read alignment with Burrows-Wheeler transform. Bioinformatics 25, 1754–1760. doi: 10.1093/bioinformatics/btp324
Li, H., Handsaker, B., Wysoker, A., Fennell, T., Ruan, J., Homer, N., et al. (2009). The sequence alignment/map format and SAMtools. Bioinformatics 25, 2078–2079. doi: 10.1093/bioinformatics/btp352
Liu, Z., Ji, Z., Wang, G., Chao, T., Hou, L., and Wang, J. (2016). Genome-wide analysis reveals signatures of selection for important traits in domestic sheep from different ecoregions. BMC Genom. 17:863. doi: 10.1186/s12864-017-3610-863
Lu, C. D. (2001). “Boer goat production: progress and perspective,” in Proceedings of the 2001 International Conference on Boer Goats in China, Guizhou.
Malan, S. (2000). The improved boer goat. Small Ruminant Res. 36, 165–170. doi: 10.1016/s0921-4488(99)00160-1
McKenna, A., Hanna, M., Banks, E., Sivachenko, A., Cibulskis, K., Kernytsky, A., et al. (2010). The genome analysis toolkit: a MapReduce framework for analyzing next-generation DNA sequencing data. Genome Res. 20, 1297–1303. doi: 10.1101/gr.107524.110
McLaren, W., Gil, L., Hunt, S. E., Riat, H. S., Ritchie, G. R., Thormann, A., et al. (2016). The ensembl variant effect predictor. Genome Biol. 17:122.
Miller, C. T., Beleza, S., Pollen, A. A., Schluter, D., Kittles, R. A., Shriver, M. D., et al. (2007). cis-Regulatory changes in Kit ligand expression and parallel evolution of pigmentation in sticklebacks and humans. Cell 131, 1179–1189. doi: 10.1016/j.cell.2007.10.055
Monau, P., Raphaka, K., Zvinorova-Chimboza, P., and Gondwe, T. (2020). Sustainable utilization of indigenous goats in Southern Africa. Diversity 12:20. doi: 10.3390/d12010020
Moreno-De-Luca, A., Helmers, S. L., Mao, H., Burns, T. G., Melton, A. M., Schmidt, K. R., et al. (2011). Adaptor protein complex-4 (AP-4) deficiency causes a novel autosomal recessive cerebral palsy syndrome with microcephaly and intellectual disability. J. Med. Genet. 48, 141–144. doi: 10.1136/jmg.2010.082263
Nagy, T. R., Blaylock, M. L., and Garvey, W. T. (2004). Role of UCP2 and UCP3 in nutrition and obesity. Nutrition 20:139. doi: 10.1016/j.nut.2003.09.024
Norris, B. J., and Whan, V. A. (2008). A gene duplication affecting expression of the ovine ASIP gene is responsible for white and black sheep. Genome Res. 18, 1282–1293. doi: 10.1101/gr.072090.107
Olivieri, B. F., Mercadante, M. E. Z., Cyrillo, J. N. D. S. G., Branco, R. H., Bonilha, S. F. M., De Albuquerque, L. G., et al. (2016). Genomic regions associated with feed efficiency indicator traits in an experimental Nellore cattle population. PLoS One 11:e0171845. doi: 10.1371/journal.pone.0171845
Papadimitriou, K., Kountourakis, P., Kottorou, A. E., Antonacopoulou, A. G., Rolfo, C., Peeters, M., et al. (2016). Follicle-stimulating hormone receptor (FSHR): a promising tool in oncology? Mol. Diagn. Ther. 20, 523–530. doi: 10.1007/s40291-016-0218-z
Parker, C. C., Gopalakrishnan, S., Carbonetto, P., Gonzales, N. M., Leung, E., Park, Y. J., et al. (2016). Genome-wide association study of behavioral, physiological and gene expression traits in outbred CFW mice. Nat. Genet. 48, 919–926. doi: 10.1038/ng.3609
Pausch, H., Wang, X., Jung, S., Krogmeier, D., Edel, C., Emmerling, R., et al. (2012). Identification of QTL for UV-protective eye area pigmentation in cattle by progeny phenotyping and genome-wide association analysis. PLoS One 7:e36346. doi: 10.1371/journal.pone.0036346
Purcell, S., Neale, B., Todd-Brown, K., Thomas, L., Ferreira, M. A., Bender, D., et al. (2007). PLINK: a tool set for whole-genome association and population-based linkage analyses. Am. J. Hum. Genet. 81, 559–575. doi: 10.1086/519795
Raudvere, U., Kolberg, L., Kuzmin, I., Arak, T., Adler, P., Peterson, H., et al. (2019). g: Profiler: a web server for functional enrichment analysis and conversions of gene lists (2019 update). Nucleic Acids Res. 47, W191–W198.
Rieder, S., Taourit, S., Mariat, D., Langlois, B., and Guérin, G. (2001). Mutations in the agouti (ASIP), the extension (MC1R), and the brown (TYRP1) loci and their association to coat color phenotypes in horses (Equus caballus). Mammal. Genome 12, 450–455. doi: 10.1007/s003350020017
Rubin, C.-J., Megens, H.-J., Barrio, A. M., Maqbool, K., Sayyab, S., Schwochow, D., et al. (2012). Strong signatures of selection in the domestic pig genome. Proc. Natl. Acad. Sci. U.S.A. 109, 19529–19536. doi: 10.1073/pnas.1217149109
Rubin, C.-J., Zody, M. C., Eriksson, J., Meadows, J. R., Sherwood, E., Webster, M. T., et al. (2010). Whole-genome resequencing reveals loci under selection during chicken domestication. Nature 464, 587–591. doi: 10.1038/nature08832
Sim, N.-L., Kumar, P., Hu, J., Henikoff, S., Schneider, G., and Ng, P. C. (2012). SIFT web server: predicting effects of amino acid substitutions on proteins. Nucleic Acids Res. 40, W452–W457.
Skapetas, B., and Bampidis, V. (2016). Goat production in the World: present situation and trends. Livest Res. Rural Dev. 28:200.
Song, S., Yao, N., Yang, M., Liu, X., Dong, K., Zhao, Q., et al. (2016). Exome sequencing reveals genetic differentiation due to high-altitude adaptation in the Tibetan cashmere goat (Capra hircus). BMC Genomics 17:122. doi: 10.1186/s12864-016-2449-0
Spuler, S., Carl, M., Zabojszcza, J., Straub, V., Bushby, K., Moore, S. A., et al. (2008). Dysferlin-deficient muscular dystrophy features amyloidosis. Ann. Neurol. 63, 323–328. doi: 10.1002/ana.21309
Sulem, P., Gudbjartsson, D. F., Stacey, S. N., Helgason, A., Rafnar, T., Magnusson, K. P., et al. (2007). Genetic determinants of hair, eye and skin pigmentation in Europeans. Nat. Genet. 39, 1443–1452. doi: 10.1038/ng.2007.13
Terranova, C., Narla, S. T., Lee, Y.-W., Bard, J., Parikh, A., Stachowiak, E. K., et al. (2015). Global developmental gene programing involves a nuclear form of fibroblast growth factor receptor-1 (FGFR1). PLoS One 10:e0123380. doi: 10.1371/journal.pone.0123380
Thomas, J. C., Khoury, R., Neeley, C. K., Akroush, A. M., and Davies, E. C. (1997). A fast CTAB method of human DNA isolation for polymerase chain reaction applications. Biochem. Educ. 25, 233–235. doi: 10.1016/s0307-4412(97)00122-2
Voisey, J., and Van Daal, A. (2002). Agouti: from mouse to man, from skin to fat. Pigment Cell Res. 15, 10–18. doi: 10.1034/j.1600-0749.2002.00039.x
Wang, X., Liu, J., Zhou, G., Guo, J., Yan, H., Niu, Y., et al. (2016). Whole-genome sequencing of eight goat populations for the detection of selection signatures underlying production and adaptive traits. Sci. Rep. 6:38932.
Weir, B. S., and Cockerham, C. C. (1984). Estimating F-statistics for the analysis of population structure. Evolution 38, 1358–1370. doi: 10.1111/j.1558-5646.1984.tb05657.x
Wolf Horrell, E. M., Boulanger, M. C., and D’orazio, J. A. (2016). Melanocortin 1 receptor: structure, function, and regulation. Front. Genet. 7:95. doi: 10.3389/fgene.2015.00095
Xu, T., Xu, F., Gu, L., Rong, G., Li, M., Qiao, F., et al. (2018). Landscape of alternative splicing in Capra_hircus. Sci. Rep. 8, 1–15.
Yaro, M., Munyard, K. A., Stear, M. J., and Groth, D. M. (2017). Molecular identification of livestock breeds: a tool for modern conservation biology. Biol. Rev. 92, 993–1010. doi: 10.1111/brv.12265
Yates, T. M., Drucker, M., Barnicoat, A., Low, K., Gerkes, E. H., Fry, A. E., et al. (2020). ZMYND11-related syndromic intellectual disability: 16 patients delineating and expanding the phenotypic spectrum. Hum. Mutat. 41, 1042–1050. doi: 10.1002/humu.24001
Keywords: chongming white goat, whole-genome re-sequencing, genomic characteristics, selection signature, Capra hircus
Citation: Gao J, Lyu Y, Zhang D, Reddi KK, Sun F, Yi J, Liu C, Li H, Yao H, Dai J and Xu F (2020) Genomic Characteristics and Selection Signatures in Indigenous Chongming White Goat (Capra hircus). Front. Genet. 11:901. doi: 10.3389/fgene.2020.00901
Received: 14 May 2020; Accepted: 21 July 2020;
Published: 21 August 2020.
Edited by:
Hans Cheng, United States Department of Agriculture, United StatesReviewed by:
Eugenio López-Cortegano, University of Edinburgh, United KingdomLuiz Brito, Purdue University, United States
Copyright © 2020 Gao, Lyu, Zhang, Reddi, Sun, Yi, Liu, Li, Yao, Dai and Xu. This is an open-access article distributed under the terms of the Creative Commons Attribution License (CC BY). The use, distribution or reproduction in other forums is permitted, provided the original author(s) and the copyright owner(s) are credited and that the original publication in this journal is cited, in accordance with accepted academic practice. No use, distribution or reproduction is permitted which does not comply with these terms.
*Correspondence: Jianjun Dai, blackman0520@126.com; Fuyi Xu, fxu10@uthsc.edu; xufuyiphd@gmail.com
†These authors have contributed equally to this work